- 1Centre for Health Technology, University of Plymouth, Plymouth, United Kingdom
- 2Translational and Clinical Research Institute, Newcastle University, Newcastle upon Tyne, United Kingdom
- 3School of Psychology, Faculty of Health, University of Plymouth, Plymouth, United Kingdom
- 4Department of Primary Care and Public Health, School of Public Health, Imperial College London, London, United Kingdom
Introduction: Lack of engagement is a common challenge for digital health interventions. To achieve their potential, it is necessary to understand how best to support users’ engagement with interventions and target health behaviors. The aim of this systematic review was to identify the behavioral theories and behavior change techniques being incorporated into mobile health apps and how they are associated with the different components of engagement.
Methods: The review was structured using the PRISMA and PICOS frameworks and searched six databases in July 2022: PubMed, Embase, CINAHL, APA PsycArticles, ScienceDirect, and Web of Science. Risk of bias was evaluated using the Cochrane Collaboration Risk of Bias 2 and the Mixed Methods Appraisal Tools.
Analysis: A descriptive analysis provided an overview of study and app characteristics and evidence for potential associations between Behavior Change Techniques (BCTs) and engagement was examined.
Results: The final analysis included 28 studies. Six BCTs were repeatedly associated with user engagement: goal setting, self-monitoring of behavior, feedback on behavior, prompts/cues, rewards, and social support. There was insufficient data reported to examine associations with specific components of engagement, but the analysis indicated that the different components were being captured by various measures.
Conclusion: This review provides further evidence supporting the use of common BCTs in mobile health apps. To enable developers to leverage BCTs and other app features to optimize engagement in specific contexts and individual characteristics, we need a better understanding of how BCTs are associated with different components of engagement.
Systematic review registration: https://www.crd.york.ac.uk/prospero/, identifier CRD42022312596.
1. Introduction
Mobile health applications have the potential to empower people to improve their health behaviors and self-manage health conditions (Forman et al., 2016; Moller et al., 2017; Digital Implementation Investment Guide [DIIG], 2020). They are widely available, with a large global market (Grand View Research, 2021), and provide a means of delivering far-reaching behavioral interventions. To have a significant impact on behavioral and health outcomes, mobile health apps need to be able to support sufficient engagement to achieve the aims of the intervention they are delivering (Yardley et al., 2016; Cole-Lewis et al., 2019). Previous research has established an association between engagement with digital interventions and their impact on intended outcomes (Perski et al., 2017; Grady et al., 2018; Mclaughlin et al., 2021), demonstrating the importance of a certain degree of engagement for the efficacy of an intervention. Maintaining engagement is a common challenge for mobile health apps (Birnbaum et al., 2015; Yeager and Benight, 2018; Baumel et al., 2019; Meyerowitz-Katz et al., 2020; Pratap et al., 2020; Torous et al., 2020a) and is a potential reason why evidence of their effectiveness remains mixed (Dounavi and Tsoumani, 2019; Ng et al., 2019; Milne-Ives et al., 2020; Moshe et al., 2021).
The conceptualization of engagement has recently become a focus in digital health research, resulting in several theories and frameworks (O’Brien, 2016; Yardley et al., 2016; Perski et al., 2017; Cole-Lewis et al., 2019; Kelders et al., 2020). It is generally accepted to be multi-faceted, with components relating to dimension (i.e., affective, cognitive, and behavioral) and scale [i.e., small-scale engagement with specific components of the digital intervention (“micro”) and larger-scale engagement with target health behaviors (“macro”)] (Milne-Ives et al., 2022). A variety of strategies have been proposed and incorporated into mobile health apps to help improve user engagement, including design features and behavior change techniques (Garnett et al., 2015; Floryan et al., 2020; Iribarren et al., 2021).
Although evidence is still developing, previous research has emphasized the importance of incorporating behavioral theory into digital health intervention design (Gourlan et al., 2016; Moller et al., 2017; Roberts et al., 2017; Klonoff, 2019; Taj et al., 2019). Behavioral theory, and behavior change techniques (a taxonomy of the smallest intervention components that can support behavior change, e.g., goal setting, self-monitoring, rewards), can provide evidence-based predictions to optimize context-specific intervention design and potential impact (Michie et al., 2013; Morrison, 2015; Moller et al., 2017). Impact on engagement, however, is often measured using only behavioral measures of app use (Torous et al., 2020b; Mclaughlin et al., 2021), which cannot capture the full picture of users’ multi-faceted engagement. For this reason, our understanding of how different components of engagement are associated with app features, engagement strategies, and outcomes is incomplete.
Despite the growing presence of Behavior Change Techniques (BCTs) (Michie et al., 2013) in mobile health apps, there has been limited investigation of how they can help support engagement specifically. Our preliminary review of the literature (Milne-Ives et al., 2022) identified several systematic reviews that have explored questions around this topic (O’Connor et al., 2016; Szinay et al., 2020; Wei et al., 2020; Borghouts et al., 2021), but none that analyzed how BCTs can best support the various components of engagement. The purpose of this review was to investigate how specific BCTs are associated with the different components of engagement (affective, cognitive, behavioral, micro, and macro) to provide insights for the development of mobile health apps. To examine this, the primary objective was to identify BCTs being incorporated in the design and development of mobile health apps and their hypothesized or evidenced associations with engagement and its various components. Learnings from this review will enable future studies to empirically test causal relationships between specific BCTs and specific components of engagement to further improve our understanding of their associations.
2. Methods
2.1. Study design
The Preferred Reporting Items for Systematic Reviews and Meta-analysis (PRISMA) framework (Page et al., 2021) was used to structure this review (Supplementary Appendix 1). The protocol was prospectively registered on PROSPERO (CRD42022312596) and published before searches began (Milne-Ives et al., 2022).
2.2. Eligibility criteria
The eligibility criteria and search strategy were based on the PICOS framework (Table 1; Richardson et al., 1995; Counsell, 1997). The protocol scope was broadened to include development studies to capture research that hypothesized potential associations. Studies published before 2011 were excluded because we wanted to provide an overview of recent evidence [digital technology evolves rapidly (Steinhubl et al., 2015)] and because the conceptualization of engagement as a multi-faceted construct emerged in digital health relatively recently (Kelders et al., 2020).
2.3. Search strategy
PubMed, Embase, Cumulative Index to Nursing and Allied Health Literature (CINAHL), APA PsycArticles, ScienceDirect, and Web of Science were searched using the search structure (Table 2 and Supplementary Appendix 2): engagement (MeSH OR Keywords) AND digital health interventions (MeSH OR Keywords) AND behavior change (MeSH OR Keywords). Searches were conducted on 12 July 2022.
2.4. Screening and article selection
References were imported into EndNote X9 for duplicate removal and initial screening using the EndNote search function (Supplementary Appendix 3). One reviewer screened titles and abstracts of remaining references in Rayyan and then conducted a full-text review.
2.5. Data extraction
Data was extracted by one reviewer into a predetermined form (see Box 1).
BOX 1 Article information and data extraction. |
General study information |
● Year of publication |
● Country of study |
● Sample demographics (eg. age, gender, target population) |
● Initial sample size |
● Analyzed sample size |
● Study duration |
Mobile health app behavioral intervention |
● App name |
● Operating platform (iOS, Android, web) |
● Target health behavior |
● Aim of the intervention |
● Behavioral theory used |
● Number of included Behavior Change Techniques (Michie et al., 2013) (if any) |
● List of included Behavior Change Techniques (Michie et al., 2013) (if any) |
● Other app engagement features |
Evaluation |
● Component(s) of engagement examined |
● Engagement outcome measures |
● Effect of intervention on engagement outcomes |
● Effect of intervention on behavioral outcomes (if reported) |
● Effect of intervention on health outcomes (if reported) |
2.6. Data analysis
A descriptive analysis summarized study and intervention characteristics. A quantitative meta-analysis of effect was not possible due to outcome variety, so extracted evidence was analyzed by one author to map potential associations between BCTs and engagement. Evidence for potential associations was divided into three categories: evidenced, hypothesized, or inferred. Associations were inferred if studies did not report app features as BCTs or did not directly examine associations between features and engagement. Features were coded using the Behaviour Change Technique (BCT) Taxonomy v1 (Michie et al., 2013) as generically as possible (e.g., “non-specific” reward) to ensure that they could be analyzed in line with the rest of the included studies. To examine components of engagement, a basic codebook was created using definitions from the literature (Supplementary Appendix 4) to explore how they were assessed and associated with BCTs.
2.7. Risk of bias and quality assessment
The quality appraisal was conducted using the Cochrane Collaboration Risk of Bias 2 (RoB 2) tool for randomized controlled trials (RCTs) (Higgins et al., 2011; The Cochrane Collaboration, 2021) and the Mixed-Methods Appraisal Tool (MMAT) (Hong et al., 2018). The MMAT tool was used instead of the ROBINS-I tool (Sterne et al., 2016) because it could be applied to the wide range of study types included in the review.
3. Results
3.1. Included studies
In total, 21,185 articles were retrieved and 28 were determined to be eligible for inclusion. The reasons for exclusion in the full-text review stage are included in the PRISMA flow diagram (Figure 1). One of the included references was a Clinicaltrials.gov registration from which a full text article was identified.
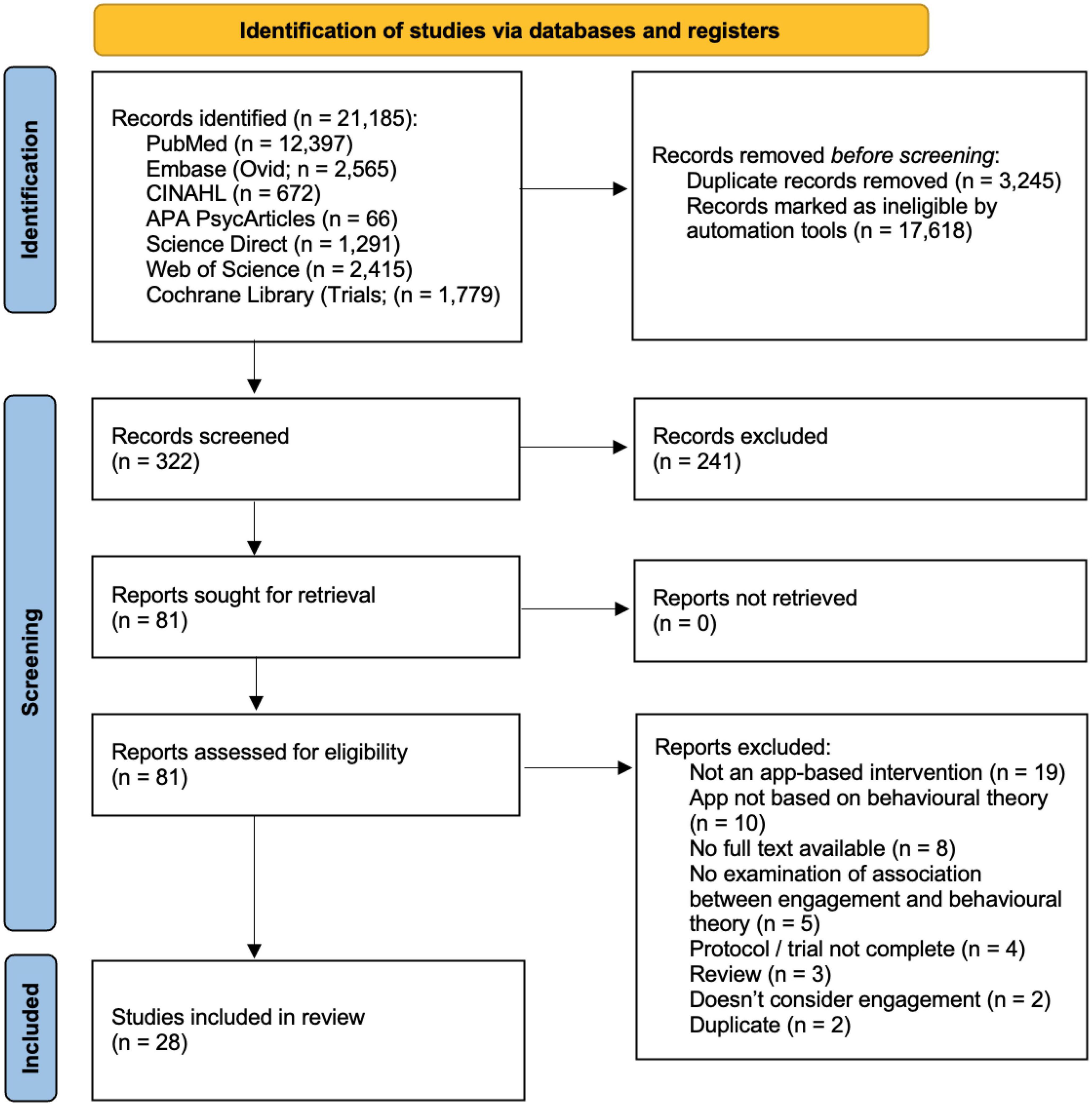
Figure 1. PRISMA flow diagram (Page et al., 2021).
3.2. Study characteristics
The characteristics of the 28 included studies are summarized in Supplementary Appendix 5. Qualitative study designs were most common (8/28, 29%). Half of the studies (14/28) described development or early-stage testing. Reported study durations ranged from 2 (Duff et al., 2018) to 83 weeks (Delmas and Kohli, 2020). Sample sizes ranged from 7 (Garnett et al., 2015) to 2,740 (Delmas and Kohli, 2020). Almost three quarters of the studies (18/25) had fewer than 100 participants; the two studies with the largest sample sizes both retrospectively sampled real world app users (Cueto et al., 2019; Delmas and Kohli, 2020). Two thirds of the studies (17/25) focused on adults, a quarter (6/25) on children and adolescents, and two on adolescents and young adults.
3.3. App characteristics
Twenty-five individual apps were examined in 25 studies (Table 3), with three studies including multiple apps (Tang et al., 2015; Baumel and Kane, 2018; Szinay et al., 2021). The studies referenced a total of 19 unique behavioral theories (Table 4), with a third (9/28) referencing more than one. Just over half the studies (15/28) explicitly reported BCTs, eight of which did not reference a theory (Garnett et al., 2015; Tang et al., 2015; Cueto et al., 2019; Davis and Ellis, 2019; DeSmet et al., 2019; D’Addario et al., 2020; Valle et al., 2020; Thornton et al., 2021). Among the 28 studies, 40 unique BCTs were identified as included in the apps.
3.4. Engagement measures
The most common method of measuring engagement (11/28 studies) was through app usage data (e.g., frequency, duration). Other methods included surveys, qualitative methods, app-collected data (active or passive), and data from synced devices (e.g., activity trackers and smart pill bottle caps, Table 5). Many of the studies that used qualitative methods were focused on user-centered design or early-stage usability or feasibility testing.
3.5. Associations between behavior change techniques and engagement
Table 6 summarizes the authors’ analysis of the potential associations between BCTs and engagement, in the context of the available descriptions and evidence. Methods in the “evidenced” group of studies included comparisons between different mobile conditions (e.g., with and without social support) (Du et al., 2016; Nuijten et al., 2021, 2022), rankings of the effectiveness of particular BCTs for engagement by experts or users (Garnett et al., 2015; Tang et al., 2015; Szinay et al., 2021), and modeling (Delmas and Kohli, 2020; Lin and Mâsse, 2021). Overall, the “evidenced” studies were of moderate quality: of the randomized trials, two had some risk of bias (Du et al., 2016; Nuijten et al., 2022) and one had high risk of bias (Nuijten et al., 2021), but the studies evaluated using the MMAT met most or all of the quality criteria (Garnett et al., 2015; Tang et al., 2015; Delmas and Kohli, 2020; Lin and Mâsse, 2021; Szinay et al., 2021). The six most common BCTs associated with engagement (based on the summed number of studies from the three categories) were social support, goal setting, feedback, prompts/cues, self-monitoring, and rewards (Table 7).

Table 6. Analysis of potential associations between BCTs and engagement and the evidence for the association.
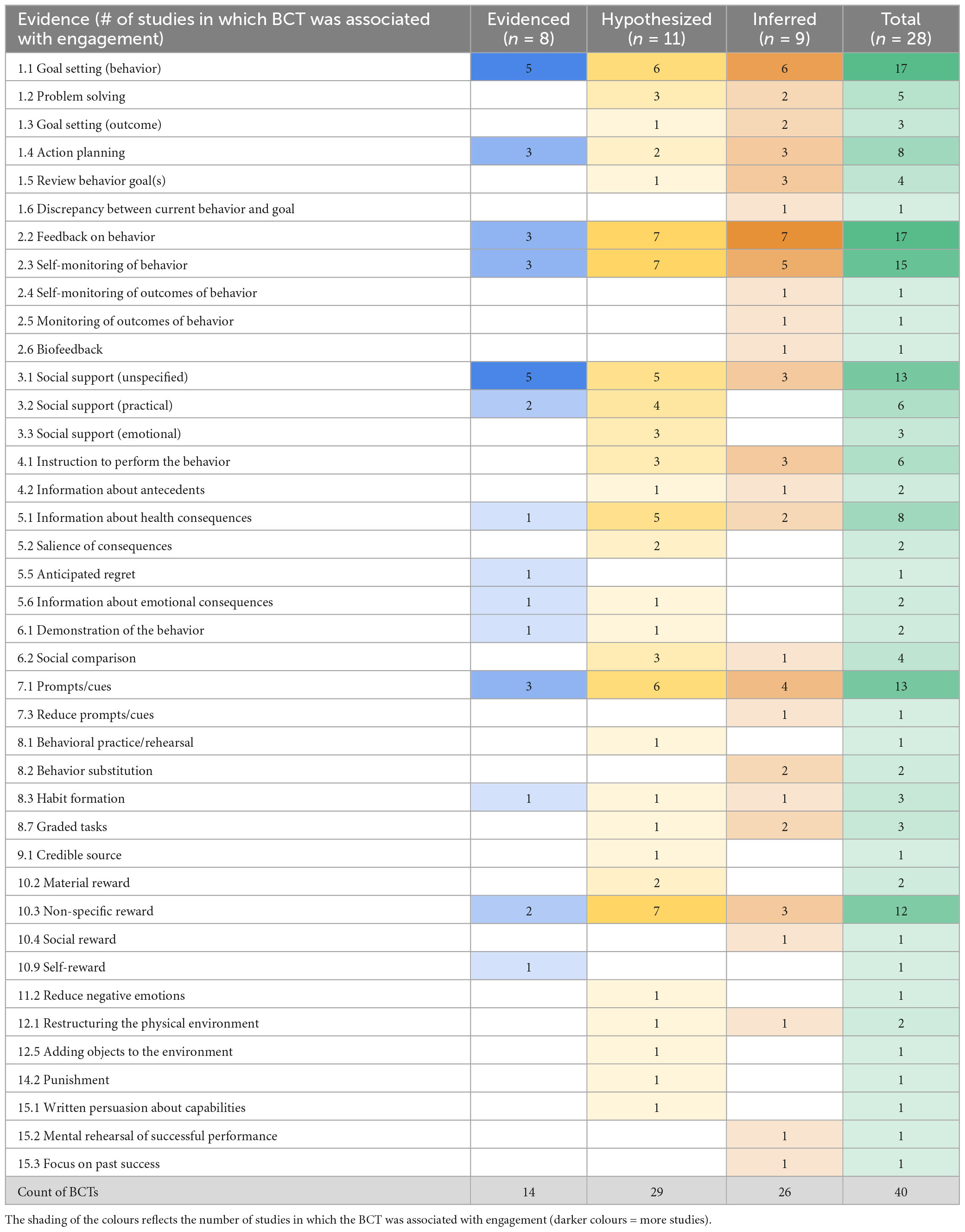
Table 7. Associations between behaviour change techniques (BCTs) and engagement identified in the studies.
3.6. Exploratory analysis of engagement components
Only two studies explicitly discussed multi-faceted conceptualizations of engagement (Nurmi et al., 2020; Szinay et al., 2021). 21 studies included measures of engagement with sufficient description to code various components, most of which captured some form of behavioral engagement (16/21), either with the intervention (12/21) or with the target behavior (11/21). All of the studies that examined engagement with the intervention analyzed app use data, sometimes supplemented by self-report (5/12). Health behavior data was captured through self-report via app or questionnaire in all of the studies but one, which used the app to track step count (Höchsmann et al., 2019).
Around half of the studies captured affective (10/21) and cognitive (11/21) components of engagement. Although affective engagement was seldom a stated outcome, a couple common affective-related themes were identified in several qualitative studies, notably “motivation” and “fun” (Tang et al., 2015; Buman et al., 2016; Hales et al., 2016; Duff et al., 2018; D’Addario et al., 2020; Szinay et al., 2021; Thornton et al., 2021). Participants suggested that features providing social support (D’Addario et al., 2020; Szinay et al., 2021), feedback on behavior (D’Addario et al., 2020), prompts and cues (Duff et al., 2018), rewards (Hales et al., 2016; Szinay et al., 2021), and encouragement (Hales et al., 2016; Szinay et al., 2021) were motivating and that gamification, such as challenges and competition, was both motivating and fun (Tang et al., 2015; Hales et al., 2016; Szinay et al., 2021). Not all participants felt the apps needed to be fun, as that was not the reason they were engaging with them (Duff et al., 2018; D’Addario et al., 2020). Some studies also identified negative emotions (e.g., discouragement, guilt) associated with feedback that increased users’ awareness of their less healthy behaviors (Tang et al., 2015; Buman et al., 2016).
Various constructs related to affective engagement were also captured in various questionnaires: irritation (EQR User Engagement subscale), sense of empathizing from app (EQR Therapeutic Alliance subscale) inspiration/encouragement (EQR Therapeutic Persuasiveness subscale), fun and entertainment (uMARS engagement section), and visual appeal (EQR Visual Design subscale and uMARS aesthetics section) (Baumel and Kane, 2018; Davis and Ellis, 2019), “hedonic motivation” (UTAUT2) (Duff et al., 2018), and “interest/enjoyment” (IMI subscale) (Höchsmann et al., 2019).
Data related to cognitive engagement also came primarily from qualitative feedback (Tang et al., 2015; Buman et al., 2016; Hales et al., 2016; Duff et al., 2018; Whiteley et al., 2019; Nurmi et al., 2020; Szinay et al., 2021; Thornton et al., 2021), although a few questionnaires included at least one item relating to interest (EQR User Engagement subscale (Baumel and Kane, 2018), uMARS engagement section (Davis and Ellis, 2019), IMI “interest/enjoyment” subscale (Höchsmann et al., 2019), and the Client Satisfaction Questionnaire (Whiteley et al., 2019). Qualitative results identified a dichotomous association between cognitive effort and engagement; some feedback suggested that engagement would be negatively affected by a time-consuming app with a high cognitive load and that a basic, easy-to-use app was desirable (Szinay et al., 2021), while other findings indicated that lots of content could help sustain engagement and disliked apps that were too basic (Tang et al., 2015; Thornton et al., 2021).
3.7. Risk of bias and quality assessment
The risk of bias of the six randomized trials was evaluated using the Cochrane Collaboration RoB 2 tool (Higgins et al., 2011; The Cochrane Collaboration, 2021; Table 8 and Supplementary Appendix 6). The domains with the lowest risk of bias were “measurement of the outcome” (because most of the studies used objective measures of engagement) and “missing outcome data” (largely because engagement was the main outcome, so drop-out and attrition were data). Bias in the selection of the reported result could not be determined for the majority of studies, as pre-published protocols or registrations were not found.
The Mixed-Methods Appraisal Tool (MMAT) (Hong et al., 2018) was used to assess the quality of the remaining studies (Supplementary Appendix 6). Of the study types, the qualitative studies met the most criteria; however, as the individual quality criteria are different for each study, a direct comparison is inappropriate. Common issues with the mixed-methods studies assessed were a lack of explicit justification for the mixed-methods design and a lack of detailed interpretation of the integration of results. For the six quantitative studies, half did not have participants representative of the target population. Across all of the study types, there was a common issue regarding the item about “clear research questions”; two-thirds of the studies (13/19) reported aims, but not research questions.
4. Discussion
4.1. Principal findings
In total, 28 studies that developed or examined mobile apps for health behavior change were examined to investigate how behaviour change techniques (BCTs) could influence different components of engagement. Although a variety of methods were used and various components of engagement could be identified in the data captured, most studies did not purposefully examine these different components. Different measures tended to capture different components: affective and cognitive engagement were primarily captured with qualitative methods, micro behavioral engagement with app use data, and macro behavioral engagement with self-report, questionnaires, and app tracking. This emphasizes the importance of mixed methods to fully assess user engagement. By combining study-reported evidence with our coding of BCTs and engagement, we identified patterns of evidence that suggest the potential of 6 BCTs to support engagement with digital health interventions: social support, goal setting, feedback, prompts/cues, self-monitoring, and rewards. A lack of explicit reporting of BCTs and preplanned analyses of engagement components limited our ability to identify potential associations between BCTs and specific components of engagement.
4.2. Comparisons with existing literature
The BCTs identified as having evidence of associations with engagement are in line with previous findings. The mixed influence of social support on engagement has also been observed by previous research (Tong and Laranjo, 2018; Szinay et al., 2020) and could potentially be explained by a difference between hypothetical preferences and practical experiences (Szinay et al., 2020). The implementation of the social support BCT could also be a factor; the studies that found a positive influence of social support on engagement were team-based (Du et al., 2016; Nuijten et al., 2021), provided practical social support, such as connection with healthcare professionals, or offered optional opportunities to interact with other users to get peer support, compete, or be held accountable to their goals (Tang et al., 2015; Szinay et al., 2021). Mixed or negative influences were associated with social comparison and competition (D’Addario et al., 2020).
4.3. Strengths and limitations
The broad scope is both a strength and a limitation; the inclusive search strategy reduced the likelihood of missing relevant studies, but necessitated automated screening. To mitigate the risk of screening out relevant studies, we used a set of six articles identified in preliminary searches and determined to be eligible to check the screening. After each pass in EndNote, if any of the six studies were missing, the search terms were revised and the pass re-run to ensure we were not incorrectly eliminating relevant papers. Although the search terms were developed with a second author, the review was not independently executed by two reviewers, which would have reduced the risk of bias in the analysis and increased the credibility of the conclusions. It was not possible to have a second reviewer due to time and resource constraints.
The diversity of outcomes examined by the studies prohibited a quantitative meta-analysis to examine effect. Additionally, the studies examined a wide variety of health apps, aimed at various populations and behaviors. In including this variety of studies, the review is limited in its ability to explore potential context-specific associations. Contextual factors such as behavior, age, culture, app type, and outcomes could influence engagement in ways that it was not possible to capture with the current sample. The study was also limited to apps that had been evaluated in scientific investigations; this method risks excluding popular publicly-available apps that have not been previously evaluated. A review of the BCTs included in such apps and how they are associated with engagement could provide additional insights to inform app design.
This study instead contributes to the literature by analyzing the body of evidence to hypothesize associations between BCTs and engagement for future testing. The analysis was limited by the available reported data and descriptions of the studies’ apps and measures. The lack of explicit analyses of engagement components in the included studies meant the review relied on author coding and inference to identify potential associations. As such, this review represents a starting point for further investigation of engagement and an identification of current limitations in digital health methodology and reporting.
4.4. Future research and recommendations
One of the challenges of our analysis was that there were no clear and comprehensive definitions of affective, cognitive, and behavioral engagement in the literature. Constructs commonly associated with affective engagement included motivation, positive and negative feelings, and sometimes aesthetic pleasure, while cognitive engagement was described using terms such as interest, attention, thought, and challenge (O’Brien, 2016; Perski et al., 2017; Kelders et al., 2020). Recent discussions in the literature have highlighted the importance of using mixed-methods designs to enable these elements to be assessed (Yardley et al., 2016; Perski et al., 2017; Short et al., 2018), which is supported by our findings. As more studies intentionally examine the different components of engagement and how they influence each other, our understanding of how to use particular BCTs to provide tailored support for different individuals, in different contexts, and at different times in their process of engagement with the intervention will improve.
There was not sufficient evidence available in the included studies to posit associations between BCTs and specific components of engagement. However, the exploratory analysis enables some hypotheses to be suggested for further investigation. Several of the BCTs with potential associations with engagement - goal setting, self-monitoring, feedback, social support, rewards, and prompts and cues - were identified as being “motivating” and “fun” for users. This evidence could indicate associations between these BCTs and affective and cognitive engagement, although further context about how and why they were motivating it would be needed to determine whether the associations were primarily affective, cognitive, or both. A theoretical examination of those components compared to the Behaviour Change Technique (BCT) taxonomy can provide some additional insight.
The Human Behavior Change Project’s Theory and Techniques Tool maps the BCT taxonomy with mechanisms of action (MoA) (Connell Bohlen et al., 2018; Carey et al., 2019; Johnston et al., 2021). A couple of the MoAs associated with the BCTs clearly relate to a component of engagement; for example, the definitions of MoAs with links to goal setting (intention and goals) are theoretically similar to the concept of cognitive engagement as they relate to “conscious decisions” and “mental representations,” as is one of the MoAs linked with prompts/cues (memory, attention, and decision processes). This suggests that, for these two BCTs in particular, their impact on motivation is more likely to be related to cognitive than affective engagement. However, many of the linked MoAs do not provide any additional clarity about how the BCTs relate to different components of engagement. For instance, one of the MoAs for self-monitoring (behavioral regulation) is defined as “behavioral, cognitive and/or emotional skills for managing or changing behavior.” Likewise, the MoA environmental context/resources, which is linked with social support and prompts/cues, is defined as “aspects of a person’s situation or environment that discourage or encourage the behavior,” which could conceivably include cognitive, affective, or behavioral aspects. In digital health, these aspects can include the availability, accessibility, and interactivity of an app (potentially affecting behavioral engagement), the tone of the app (potentially affecting affective engagement), and the personalization to a users’ needs (potentially affecting cognitive engagement) (Szinay et al., 2020, 2021).
Recent studies have identified a lack of justification and guidance for the operationalization of BCTs (Fulton et al., 2018; Lemke and de Vries, 2021). The evidence indicates that the way BCTs such as social support are incorporated into interventions could affect how they are associated with engagement. It will be important to examine if there are certain operationalizations that better support engagement or whether its influence is related primarily to individual characteristics. Understanding when and how they have a positive or negative impact would enable interventions to be tailored to the specific context in which they will be implemented or dynamically to individuals, to emphasize specific BCTs and app features that they are most likely to respond best to.
For app developers, the key takeaways from the evidence gathered in this review is that the inclusion of BCTs such as goal setting, feedback, prompts/cues, self-monitoring, and reward could help support users’ engagement with mobile health apps. Social support also has the potential to support engagement, but can have unintended negative effects, depending on how it is incorporated. Based on the studies reviewed, our recommendation for developers considering incorporating social support is to carefully consider how it will be operationalized and to make that feature optional for users. Practical social support, such as linking patients with healthcare providers, is likely to support engagement and team-based competition seems to be less likely than individual competition to generate negative emotions in users. In view of app design, there appears to be a balance needed to obtain the optimal level of cognitive load. Data from participants indicated a preference for a reduced cognitive load - limited complexity, less data input, limited number of features, not time-consuming (Abuhamdeh et al., 2015; Szinay et al., 2021) - while other data and motivation theory suggests that the app needed to be interesting enough to keep users’ attention (Tang et al., 2015; Thornton et al., 2021) and provide the right level of challenge to achieve optimal motivation (Abuhamdeh et al., 2015). Therefore, apps should be easy to learn and efficient to navigate (Wei et al., 2020), but contain sufficient or varying content to sustain users’ interest. Finally, it is likely to be important for engagement to choose BCTs and other app features carefully to fit the context and the aim of the intervention, rather than incorporating as many BCTs as possible.
5. Conclusion
The purpose of this systematic review was to incorporate theoretical conceptualizations of engagement and synthesize evidence of how mobile health apps and their BCTs are associated with different components of user engagement. Six BCTs were identified as having the most evidence of a potential association with engagement with digital behavior change interventions: goal setting, self-monitoring of behavior, feedback on behavior, prompts/cues, rewards, and social support. An exploratory analysis of the engagement components identified key areas for future research, including further theoretical clarity of engagement components, how less researched BCTs might be theoretically linked to engagement components, and how BCTs can be operationalized in app design to best support affective, cognitive, and behavioral engagement. Recommendations for app development deriving from the findings are the inclusion and further investigation of the 6 BCTs identified to support engagement and the importance of identifying the right level of cognitive involvement for the target population to keep the app interesting without being a burden. This review contributes to the body of evidence for the influence of specific BCTs on engagement by identifying a gap between theoretical conceptualizations of engagement and its evaluation in mobile health apps and hypothesizing potential associations for future empirical investigation. Greater clarity in the definitions of different components of engagement, and further development and incorporation of means of measuring specific types of engagement, could enable future research to examine how different components of engagement are related to specific BCTs and behavioral and health outcomes. This knowledge could inform the design and tailoring of mobile health apps to improve engagement with the intervention and target behaviors.
Data availability statement
The original contributions presented in this study are included in the article/Supplementary material, further inquiries can be directed to the corresponding author.
Author contributions
MM-I conceived the topic, drafted the protocol, conducted the searches, screening, data extraction and analysis, and wrote the first draft of the manuscript. SH and JA contributed to the revisions. EM performed the final review and supervised the execution of the review. All authors contributed to the article and approved the submitted version.
Funding
This research was funded by Health Education England (grant reference number: AM1000393). The funding body was not involved in the study design, data collection or analysis, or the writing and decision to submit the article for publication.
Conflict of interest
The authors declare that the research was conducted in the absence of any commercial or financial relationships that could be construed as a potential conflict of interest.
Publisher’s note
All claims expressed in this article are solely those of the authors and do not necessarily represent those of their affiliated organizations, or those of the publisher, the editors and the reviewers. Any product that may be evaluated in this article, or claim that may be made by its manufacturer, is not guaranteed or endorsed by the publisher.
Author disclaimer
The views expressed in the manuscript belong to the authors and not necessarily those of Health Education England, the University of Plymouth, Newcastle University, or Imperial College London.
Supplementary material
The Supplementary Material for this article can be found online at: https://www.frontiersin.org/articles/10.3389/fpsyg.2023.1227443/full#supplementary-material
Supplementary Appendix 1 | PRISMA checklist.
Supplementary Appendix 2 | Search results by database.
Supplementary Appendix 3 | EndNote screening.
Supplementary Appendix 4 | Engagement components codebook.
Supplementary Appendix 5 | Study characteristics.
Supplementary Appendix 6 | Risk of bias and quality assessment.
References
Abuhamdeh, S., Csikszentmihalyi, M., and Jalal, B. (2015). Enjoying the possibility of defeat: Outcome uncertainty, suspense, and intrinsic motivation. Motiv. Emot. 39, 1–10.
Ahonkhai, A. A., Pierce, L. J., Mbugua, S., Wasula, B., Owino, S., Nmoh, A., et al. (2021). PEERNaija: A gamified mhealth behavioral intervention to improve adherence to antiretroviral treatment among adolescents and young adults in Nigeria. Front. Reprod. Health 3:656507. doi: 10.3389/frph.2021.656507
Baumel, A., and Kane, J. M. (2018). Examining predictors of real-world user engagement with self-guided ehealth interventions: analysis of mobile apps and websites using a novel dataset. J. Med. Internet Res. 20:e11491. doi: 10.2196/11491
Baumel, A., Muench, F., Edan, S., and Kane, J. M. (2019). Objective user engagement with mental health apps: Systematic search and panel-based usage analysis. J. Med. Internet Res. 21:e14567. doi: 10.2196/14567
Birnbaum, F., Lewis, D. M., Rosen, R., and Ranney, M. L. (2015). Patient engagement and the design of digital health. Acad. Emerg. Med. 22:754.
Borghouts, J., Eikey, E., Mark, G., De Leon, C., Schueller, S. M., Schneider, M., et al. (2021). Barriers to and facilitators of user engagement with digital mental health interventions: Systematic review. J. Med. Internet Res. 23:e24387.
Buman, M. P., Epstein, D. R., Gutierrez, M., Herb, C., Hollingshead, K., Huberty, J. L., et al. (2016). BeWell24: development and process evaluation of a smartphone “app” to improve sleep, sedentary, and active behaviors in US Veterans with increased metabolic risk. Transl. Behav. Med. 6, 438–448. doi: 10.1007/s13142-015-0359-3
Caon, M., Prinelli, F., Angelini, L., Carrino, S., Mugellini, E., Orte, S., et al. (2022). PEGASO e-Diary: User engagement and dietary behavior change of a mobile food record for adolescents. Front. Nutr. 9:727480. doi: 10.3389/fnut.2022.727480
Carey, R. N., Connell, L. E., Johnston, M., Rothman, A. J., de Bruin, M., Kelly, M. P., et al. (2019). Behavior change techniques and their mechanisms of action: a synthesis of links described in published intervention literature. Ann. Behav. Med. 53, 693–707. doi: 10.1093/abm/kay078
Cole-Lewis, H., Ezeanochie, N., and Turgiss, J. (2019). Understanding health behavior technology engagement: pathway to measuring digital behavior change interventions. JMIR Form. Res. 3:e14052.
Connell Bohlen, L., Carey, R. N., de Bruin, M., Rothman, A., Johnston, M., Kelly, M. P., et al. (2018). Links between behaviour change techniques and mechanisms of action: an expert consensus study. PsyArXiv [Preprint]. doi: 10.31234/osf.io/fge86
Counsell, C. (1997). Formulating questions and locating primary studies for inclusion in systematic reviews. Ann. Intern. Med. 127, 380–387. doi: 10.7326/0003-4819-127-5-199709010-00008
Cueto, V., Wang, C. J., and Sanders, L. M. (2019). Impact of a mobile app-based health coaching and behavior change program on participant engagement and weight status of overweight and obese children: Retrospective cohort study. JMIR Mhealth Uhealth 7:e14458.
Curtis, K., Lebedev, A., Aguirre, E., and Lobitz, S. (2019). A medication adherence app for children with sickle cell disease: Qualitative study. JMIR Mhealth Uhealth 7:e8130.
D’Addario, M., Baretta, D., Zanatta, F., Greco, A., and Steca, P. (2020). Engagement features in physical activity smartphone apps: Focus group study with sedentary people. JMIR Mhealth Uhealth 8:e20460.
Davis, A., and Ellis, R. (2019). A quasi-experimental investigation of college students’ ratings of two physical activity mobile apps with varied behavior change technique quantity. Digit. Health 5:205520761989134. doi: 10.1177/2055207619891347
Delmas, M. A., and Kohli, A. (2020). Can apps make air pollution visible? Learning about health impacts through engagement with air quality information. J. Bus. Ethics 161, 279–302.
DeSmet, A., De Bourdeaudhuij, I., Chastin, S., Crombez, G., Maddison, R., and Cardon, G. (2019). Adults’ preferences for behavior change techniques and engagement features in a mobile app to promote 24-hour movement behaviors: Cross-sectional survey study. JMIR mHealth and uHealth 7:e15707. doi: 10.2196/15707
Digital Implementation Investment Guide [DIIG] (2020). Integrating Digital Interventions into Health Programmes. Geneva: World Health Organization.
Dounavi, K., and Tsoumani, O. (2019). Mobile health applications in weight management: A systematic literature review. Am. J. Prev. Med. 56, 894–903.
Du, H., Venkatakrishnan, A., Youngblood, G. M., Ram, A., and Pirolli, P. (2016). A group-based mobile application to increase adherence in exercise and nutrition programs: A factorial design feasibility study. JMIR Mhealth Uhealth 4:e4. doi: 10.2196/mhealth.4900
Duff, O., Walsh, D., Malone, S., McDermott, L., Furlong, B., O’Connor, N., et al. (2018). MedFit App, a behavior-changing, theoretically informed mobile app for patient self-management of cardiovascular disease: user-centered development. JMIR Form. Res. 2:e8. doi: 10.2196/formative.9550
Floryan, M., Chow, P. I., Schueller, S. M., and Ritterband, L. M. (2020). The model of gamification principles for digital health interventions: evaluation of validity and potential utility. J. Med. Internet Res. 22:e16506. doi: 10.2196/16506
Forman, E. M., Evans, B. C., Flack, D., and Goldstein, S. P. (2016). Could technology help us tackle the obesity crisis? Future Sci. OA 2:61. doi: 10.4155/fsoa-2016-0061
Fulton, E. A., Kwah, K. L., Wild, S., and Brown, K. E. (2018). Lost in translation: transforming behaviour change techniques into engaging digital content and design for the stopapp. Healthc. Pap. 6:30075. doi: 10.3390/healthcare6030075
Garnett, C., Crane, D., West, R., Brown, J., and Michie, S. (2015). Identification of behavior change techniques and engagement strategies to design a smartphone app to reduce alcohol consumption using a formal consensus method. JMIR Mhealth Uhealth 3:e73. doi: 10.2196/mhealth.3895
Gourlan, M., Bernard, P., Bortolon, C., Romain, A. J., Lareyre, O., Carayol, M., et al. (2016). Efficacy of theory-based interventions to promote physical activity. A meta-analysis of randomised controlled trials. Health Psychol. Rev. 10, 50–66. doi: 10.1080/17437199.2014.981777
Grady, A., Yoong, S., Sutherland, R., Lee, H., Nathan, N., and Wolfenden, L. (2018). Improving the public health impact of eHealth and mHealth interventions. Austral. N. Zeal. J. Public Health 42, 118–119.
Grand View Research (2021). Fitness App Market Size, Share & Growth Report, 2021 - 2028. San Francisco, CA: Grand View Research.
Hales, S., Turner-McGrievy, G., Fahim, A., Freix, A., Wilcox, S., Davis, R. E., et al. (2016). A mixed-methods approach to the development, refinement, and pilot testing of social networks for improving healthy behaviors. JMIR Hum. Factors 3:e8. doi: 10.2196/humanfactors.4512
Higgins, J. P. T., Altman, D. G., Gøtzsche, P. C., Jüni, P., Moher, D., Oxman, A. D., et al. (2011). The Cochrane Collaboration’s tool for assessing risk of bias in randomised trials. BMJ 343:d5928.
Höchsmann, C., Infanger, D., Klenk, C., Königstein, K., Walz, S. P., and Schmidt-Trucksäss, A. (2019). Effectiveness of a behavior change technique-based smartphone game to improve intrinsic motivation and physical activity adherence in patients with type 2 diabetes: randomized controlled trial. JMIR Ser. Games 7:e11444. doi: 10.2196/11444
Hong, Q. N., Pluye, P., Fàbregues, S., Bartlett, G., Boardman, F., Cargo, M., et al. (2018). Mixed Methods Appraisal Tool (MMAT). Gatineau: Canadian Intellectual Property Office.
Iribarren, S. J., Akande, T. O., Kamp, K. J., Barry, D., Kader, Y. G., and Suelzer, E. (2021). Effectiveness of mobile apps to promote health and manage disease: Systematic review and meta-analysis of randomized controlled trials. JMIR mHealth and uHealth 9:e21563.
Johnston, M., Carey, R. N., Connell Bohlen, L. E., Johnston, D. W., Rothman, A. J., de Bruin, M., et al. (2021). Development of an online tool for linking behavior change techniques and mechanisms of action based on triangulation of findings from literature synthesis and expert consensus. Transl. Behav. Med. 11, 1049–1065. doi: 10.1093/tbm/ibaa050
Kelders, S. M., van Zyl, L. E., and Ludden, G. D. S. (2020). The concept and components of engagement in different domains applied to Ehealth: A systematic scoping review. Front. Psychol. 11:926. doi: 10.3389/fpsyg.2020.00926
Klonoff, D. C. (2019). Behavioral theory: The missing ingredient for digital health tools to change behavior and increase adherence. J. Diabetes Sci. Technol. 13, 276–281. doi: 10.1177/1932296818820303
Lemke, M., and de Vries, R. A. J. (2021). Operationalizing behavior change theory as part of persuasive technology: A scoping review on social comparison. Front. Comput. Sci. 3:656873. doi: 10.3389/fcomp.2021.656873
Lin, Y., and Mâsse, L. C. (2021). A look at engagement profiles and behavior change: A profile analysis examining engagement with the Aim2Be lifestyle behavior modification app for teens and their families. Prevent. Med. Rep. 24:101565. doi: 10.1016/j.pmedr.2021.101565
Mclaughlin, M., Delaney, T., Hall, A., Byaruhanga, J., Mackie, P., Grady, A., et al. (2021). Associations between digital health intervention engagement, physical activity, and sedentary behavior: Systematic review and meta-analysis. J. Med. Internet Res. 23:e23180.
Meyerowitz-Katz, G., Ravi, S., Arnolda, L., Feng, X., Maberly, G., and Astell-Burt, T. (2020). Rates of attrition and dropout in app-based interventions for chronic disease: Systematic review and meta-analysis. J. Med. Internet Res. 22:e20283. doi: 10.2196/20283
Michie, S., Richardson, M., Johnston, M., Abraham, C., Francis, J., Hardeman, W., et al. (2013). The behavior change technique taxonomy (v1) of 93 hierarchically clustered techniques: building an international consensus for the reporting of behavior change interventions. Ann. Behav. Med. 46, 81–95. doi: 10.1007/s12160-013-9486-6
Milne-Ives, M., Homer, S., Andrade, J., and Meinert, E. (2022). Associations between behavior change techniques and engagement with mobile health apps: Protocol for a systematic review. JMIR Res. Protoc. 11:e35172. doi: 10.2196/35172
Milne-Ives, M., Lam, C., De Cock, C., Van Velthoven, M. H., and Meinert, E. (2020). Mobile apps for health behavior change in physical activity, diet, drug and alcohol use, and mental health: Systematic review. JMIR Mhealth Uhealth 8:e17046. doi: 10.2196/17046
Moller, A. C., Merchant, G., Conroy, D. E., West, R., Hekler, E., Kugler, K. C., et al. (2017). Applying and advancing behavior change theories and techniques in the context of a digital health revolution: proposals for more effectively realizing untapped potential. J. Behav. Med. 40, 85–98. doi: 10.1007/s10865-016-9818-7
Morrison, L. G. (2015). Theory-based strategies for enhancing the impact and usage of digital health behaviour change interventions: A review. Digit. Health 1:2055207615595335. doi: 10.1177/2055207615595335
Moshe, I., Terhorst, Y., Philippi, P., Domhardt, M., Cuijpers, P., Cristea, I., et al. (2021). Digital interventions for the treatment of depression: A meta-analytic review. Psychol. Bull. 147, 749–786.
Ng, M. M., Firth, J., Minen, M., and Torous, J. (2019). User engagement in mental health apps: A review of measurement, reporting, and validity. Psychiatr. Serv. 70, 538–544. doi: 10.1176/appi.ps.201800519
Nuijten, R., Van Gorp, P., Khanshan, A., Le Blanc, P., van den Berg, P., Kemperman, A., et al. (2022). Evaluating the impact of adaptive personalized goal setting on engagement levels of government staff with a gamified mhealth tool: Results from a 2-month randomized controlled trial. JMIR Mhealth Uhealth 10:e28801. doi: 10.2196/28801
Nuijten, R. C. Y., Van Gorp, P., Borghouts, T., Le Blanc, P., Van den Berg, P., Kemperman, A., et al. (2021). Preadolescent students’ engagement with an mhealth intervention fostering social comparison for health behavior change: crossover experimental study. J. Med. Internet Res. 23:e21202.
Nurmi, J., Knittle, K., Ginchev, T., Khattak, F., Helf, C., Zwickl, P., et al. (2020). Engaging users in the behavior change process with digitalized motivational interviewing and gamification: Development and feasibility testing of the precious app. JMIR Mhealth Uhealth 8:e12884. doi: 10.2196/12884
O’Brien, H. (2016). “Theoretical Perspectives on User Engagement,” in Why Engagement Matters, eds H. O’Brien and P. Cairns (Cham: Springer International Publishing), 1–26.
O’Connor, S., Hanlon, P., O’Donnell, C. A., Garcia, S., Glanville, J., and Mair, F. S. (2016). Understanding factors affecting patient and public engagement and recruitment to digital health interventions: a systematic review of qualitative studies. BMC Med. Inform. Decis. Mak. 16:3. doi: 10.1186/s12911-016-0359-3
Page, M. J., McKenzie, J. E., Bossuyt, P. M., Boutron, I., Hoffmann, T. C., Mulrow, C. D., et al. (2021). The PRISMA 2020 statement: An updated guideline for reporting systematic reviews. PLoS Med 18:e1003583. doi: 10.1371/journal.pmed.1003583
Perski, O., Blandford, A., West, R., and Michie, S. (2017). Conceptualising engagement with digital behaviour change interventions: A systematic review using principles from critical interpretive synthesis. Transl. Behav. Med. 7, 254–267. doi: 10.1007/s13142-016-0453-1
Pratap, A., Neto, E. C., Snyder, P., Stepnowsky, C., Elhadad, N., Grant, D., et al. (2020). Indicators of retention in remote digital health studies: A cross-study evaluation of 100,000 participants. NPJ Digital Medicine 3, 1–10. doi: 10.1038/s41746-020-0224-8
Richardson, W. S., Wilson, M. C., Nishikawa, J., and Hayward, R. S. (1995). The well-built clinical question: A key to evidence-based decisions. ACP J. Club 123, A12–A13.
Roberts, A. L., Fisher, A., Smith, L., Heinrich, M., and Potts, H. W. W. (2017). Digital health behaviour change interventions targeting physical activity and diet in cancer survivors: a systematic review and meta-analysis. J. Cancer Surviv. 11, 704–719. doi: 10.1007/s11764-017-0632-1
Short, C. E., DeSmet, A., Woods, C., Williams, S. L., Maher, C., Middelweerd, A., et al. (2018). Measuring engagement in ehealth and mhealth behavior change interventions: Viewpoint of methodologies. J. Med. Internet Res. 20:e292. doi: 10.2196/jmir.9397
Smith, R., Michalopoulou, M., Reid, H., Riches, S. P., Wango, Y. N., Kenworthy, Y., et al. (2022). Applying the behaviour change wheel to develop a smartphone application “stay-active” to increase physical activity in women with gestational diabetes. BMC Pregnancy Childbirth 22:253. doi: 10.1186/s12884-022-04539-9
Songtaweesin, W. N., LeGrand, S., Bandara, S., Piccone, C., Wongharn, P., Moonwong, J., et al. (2021). Adaptation of a theory-based social networking and gamified app-based intervention to improve pre-exposure prophylaxis adherence among young men who have sex with men in Bangkok, Thailand: Qualitative study. J. Med. Internet Res. 23:e23852. doi: 10.2196/23852
Sporrel, K., De Boer, R. D. D., Wang, S., Nibbeling, N., Simons, M., Deutekom, M., et al. (2020). The design and development of a personalized leisure time physical activity application based on behavior change theories, end-user perceptions, and principles from empirical data mining. Front. Public Health 8:528472. doi: 10.3389/fpubh.2020.528472
Steinhubl, S. R., Muse, E. D., and Topol, E. J. (2015). The emerging field of mobile health. Sci. Transl. Med. 7:283rv3.
Sterne, J. A., Hernán, M. A., Reeves, B. C., Savović, J., Berkman, N. D., Viswanathan, M., et al. (2016). ROBINS-I: A tool for assessing risk of bias in non-randomised studies of interventions. BMJ 355:i4919.
Szinay, D., Jones, A., Chadborn, T., Brown, J., and Naughton, F. (2020). Influences on the uptake of and engagement with health and well-being smartphone apps: Systematic review. J. Med. Internet Res. 22:e17572. doi: 10.2196/17572
Szinay, D., Perski, O., Jones, A., Chadborn, T., Brown, J., and Naughton, F. (2021). Perceptions of factors influencing engagement with health and well-being apps in the United Kingdom: qualitative interview study. JMIR Mhealth Uhealth 9:e29098. doi: 10.2196/29098
Taj, F., Klein, M. C. A., and van Halteren, A. (2019). Digital health behavior change technology: Bibliometric and scoping review of two decades of research. JMIR Mhealth Uhealth 7:e13311.
Tang, J., Abraham, C., Stamp, E., and Greaves, C. (2015). How can weight-loss app designers’ best engage and support users? A qualitative investigation. Br. J. Health Psychol. 20, 151–171. doi: 10.1111/bjhp.12114
Thornton, L., Gardner, L. A., Osman, B., Green, O., Champion, K. E., Bryant, Z., et al. (2021). A multiple health behavior change, self-monitoring mobile app for adolescents: Development and usability study of the health4life app. JMIR Form. Res. 5:e25513. doi: 10.2196/25513
Tong, H. L., and Laranjo, L. (2018). The use of social features in mobile health interventions to promote physical activity: a systematic review. NPJ Digit. Med. 1, 1–10.
Torous, J., Lipschitz, J., Ng, M., and Firth, J. (2020a). Dropout rates in clinical trials of smartphone apps for depressive symptoms: A systematic review and meta-analysis. J. Affect. Disord. 263, 413–419. doi: 10.1016/j.jad.2019.11.167
Torous, J., Michalak, E. E., and O’Brien, H. L. (2020b). Digital health and engagement—looking behind the measures and methods. JAMA Netw Open 3:e2010918. doi: 10.1001/jamanetworkopen.2020.10918
Valle, C. G., Nezami, B. T., and Tate, D. F. (2020). Designing in-app messages to nudge behavior change: Lessons learned from a weight management app for young adults. Organ. Behav. Hum. Decis. Process. 161, 95–101.
Wei, Y., Zheng, P., Deng, H., Wang, X., Li, X., and Fu, H. (2020). Design features for improving mobile health intervention user engagement: Systematic review and thematic analysis. J. Med. Internet Res. 22:e21687. doi: 10.2196/21687
Whiteley, L., Brown, L., Lally, M., Heck, N., and van den Berg, J. J. (2018). A mobile gaming intervention to increase adherence to antiretroviral treatment for youth living with HIV: Development guided by the information, motivation, and behavioral skills model. JMIR Mhealth Uhealth 6:e96. doi: 10.2196/mhealth.8155
Whiteley, L., Mena, L., Craker, L. K., Healy, M. G., and Brown, L. K. (2019). Creating a theoretically grounded gaming app to increase adherence to pre-exposure prophylaxis: Lessons from the development of the viral combat mobile phone game. JMIR Ser. Games 7:e11861. doi: 10.2196/11861
Yardley, L., Spring, B. J., Riper, H., Morrison, L. G., Crane, D. H., Curtis, K., et al. (2016). Understanding and promoting effective engagement with digital behavior change interventions. Am. J. Prev. Med. 51, 833–842.
Keywords: engagement, behaviour change techniques, telemedicine, mobile applications, digital health
Citation: Milne-Ives M, Homer SR, Andrade J and Meinert E (2023) Potential associations between behavior change techniques and engagement with mobile health apps: a systematic review. Front. Psychol. 14:1227443. doi: 10.3389/fpsyg.2023.1227443
Received: 23 May 2023; Accepted: 04 September 2023;
Published: 18 September 2023.
Edited by:
Rüdiger Christoph Pryss, Julius Maximilian University of Würzburg, GermanyReviewed by:
Felix Beierle, National Institute of Informatics, JapanChien-Sing Lee, Sunway University, Malaysia
Johannes Schobel, Neu-Ulm University of Applied Sciences, Germany
Copyright © 2023 Milne-Ives, Homer, Andrade and Meinert. This is an open-access article distributed under the terms of the Creative Commons Attribution License (CC BY). The use, distribution or reproduction in other forums is permitted, provided the original author(s) and the copyright owner(s) are credited and that the original publication in this journal is cited, in accordance with accepted academic practice. No use, distribution or reproduction is permitted which does not comply with these terms.
*Correspondence: Edward Meinert, ZWR3YXJkLm1laW5lcnRAbmV3Y2FzdGxlLmFjLnVr