- 1Curtin School of Allied Health, Curtin University, Perth, WA, Australia
- 2St. John of God Midland Public and Private Hospitals, St John of God Health Care, Perth, WA, Australia
- 3Department of Allied Health, The School of Medical and Health Sciences, Edith Cowan University, Perth, WA, Australia
- 4Department Special Needs Education, University of Oslo, Oslo, Norway
- 5Department of Otorhinolaryngology and Head and Neck Surgery, Leiden University Medical Centre, Leiden, Netherlands
- 6Department of Biomedical Imaging and Image-guided Therapy, Medical University of Vienna, Vienna, Austria
- 7Audiologic Phoniatric Service, Otorhinolaryngology Department, Infermi Hospital, AUSL Romagna, Rimini, Italy
- 8Department of Occupational Therapy, Faculty of Medicine, Nursing and Health Sciences, Monash University – Peninsula Campus, Frankston, VIC, Australia
- 9Centre Hospitalier Universitaire de Toulouse, Touloluse, France
- 10Department of Social Work, Education and Community Wellbeing, Northumbria University, Newcastle upon Tyne, United Kingdom
- 11Department of Health and Rehabilitation Sciences, Faculty of Health Sciences, University of Cape Town, Cape Town, South Africa
The provision of quality healthcare relies on scales and measures with robust evidence of their psychometric properties. Using measurement instruments with poor reliability, validity, or feasibility, or those that are not appropriate for the target diagnostic group or construct/dimension under consideration, may be unfavorable for patients, unproductive, and hinder empirical advancement. Resources from the COnsensus-based Standards for the selection of health status Measurement INstruments (COSMIN) group can assist in identifying and developing psychometrically sound measures. The COSMIN initiative is the only international, research-based practice taxonomy and methodological guidelines for measurement in healthcare. This manuscript aims to provide an accessible introduction to theories, principles and practices of psychometrics, instrument properties, and scale development, with applied examples from the COSMIN recommendations. It describes why measurement in healthcare is critical to good practice, explains the concepts of the latent variable and hypothetical construct and their importance in healthcare assessments, explores issues of flawed measurement and briefly explains key theories relevant to psychometrics. The paper also outlines a ten-step process to develop and validate a new measurement instrument, with examples drawn from a recently developed visuoperceptual measure for analysis of disordered swallowing to demonstrate key concepts and provides a guide for understanding properties of and terminology related to measurement instruments. This manuscript serves as a resource for healthcare clinicians, educators, and researchers who seek to develop and validate new measurement instruments or improve the properties of existing ones. It highlights the importance of using psychometrically sound measurement instruments to ensure high-quality healthcare assessments.
1. Introduction
Measurement is integral to clinical practice and evidence-based healthcare. Measurement may be defined as the systematic and orderly categorization of the attributes of an object, phenomenon or concept that creates a shared standard (Bond et al., 2020). As a fundamental activity of science, it serves as a foundation for evidence-based practice (McClimans, 2017; Bond et al., 2020). The journey toward evidence-based medicine in healthcare runs in parallel with progress toward effective measurement, as quantifiable evidence underpins evidence-based care. Accurately quantifying and categorizing observations is vital for attributing clinical meaning to data (Streiner et al., 2015). Strong measurement relies on sound practices in instrument development and validation (Streiner et al., 2015). However, many clinicians, particularly in healthcare disciplines without strong psychology components, may lack training in psychometrics to engage with instrument validation science. Our manuscript seeks to address this gap by presenting concepts, pathways, and applied knowledge in a manner that is easily understandable and applicable for clinicians, and for educators and researchers working in healthcare who have an interest in psychometrics.
1.1. Manuscript structure and aim
This manuscript aims to introduce key principles of psychometrics for healthcare clinicians from medical, nursing and allied health backgrounds and healthcare educators and researchers who are interested in understanding the overall topic measurement and scales, tests and instruments used to measure constructs, factors, attributes, or dimensions. It aims to empower clinicians to navigate psychometrics research, understand relevant criteria and support application of good psychometrics practices to clinical instrument development and selection. Figure 1 outlines the structure of the manuscript, which will describe psychometric terms, introduce the Consensus-based Standards for the selection of health status Measurement Instruments (COSMIN) (Mokkink et al., 2018), present core concepts in measurement, define and provide introductory guidance on understanding the properties and quality of a measurement instrument. The manuscript will also deliver a guide for key steps in measure development through the applied example of a recently developed instrument for difficulty swallowing, also known as dysphagia.
2. Measurement in health
In healthcare, concepts of measurable interest may be considered to be a pin-point or ballung (McClimans, 2017). A pin-point measurement is a discreet category against which a patient may be compared over time, such as weight, age, and mortality. Ballung concepts, which owe their name to the German word “Ballungsgebiet” (English: agglomeration), which refers to a congested urban area with unclear borders, are more challenging to define and articulate (McClimans, 2017). Considering real clinical measurement challenges can lead to the conclusion that most health concepts are ballung. Box 1 outlines an applied example.
BOX 1. The multidimensional nature of health phenomena: coronary artery disease.
The physician interested in tracking a patient with coronary artery disease may choose from a number of aspects to measure; biometrics, such as cardio-respiratory function, haematological markers, electrocardiogram signals or images from coronary angiography (Gillen and Goyal, 2022). Coronary artery disease has a negative effect on health-related quality of life and limits exercise tolerance, while angor amini, a sense of impending doom, is a well-known symptom of acute coronary syndrome and may also be worthy of checking (Goldbeck-Wood, 2019; Huzmeli et al., 2020). Therefore, to complete the clinical picture, the physician may need to gather data from a range of facets. This example shows how a single diagnosis may have multiple clinically relevant variables of interest to the physician.
Dimensions are aspects of a construct; for example, health-related quality of life in diabetes may be impacted by fatigue, reduced participation or changed family roles (De Vet et al., 2011). In healthcare measurement science, the concept of ‘multidimensionality’ acknowledges that many health constructs cannot be comprehensively captured by a unidimensional measure alone. The challenge of accurately capturing the full range of function and dysfunction of ballung, or multidimensional, concepts becomes even more complex in disorders that are not yet fully understood or have a range of clinical presentations (e.g., rare neurological diseases or multifactorial genetic conditions). This can lead to bias or incomplete representation. Despite this, measurement instruments are essential in healthcare as they establish intervention goals, provide comparison baselines, aid diagnosis, monitor changes and intervention impact, and track efficacy and cost–benefit. Indeed, it has been asserted that ‘you cannot manage what you cannot measure’(McClimans, 2017). Therefore, faced with complex constructs and the impossibility of continuous measurement in the context of healthcare’s finite time and resources, clinicians and healthcare professionals must carefully consider what, when, where, and how to measure. Appropriately designed and statistically robust measurement instruments are therefore essential. Understanding key terminology and concepts in instrument design and function improves measurement selection, supporting better clinical practice and research.
2.1. The structure and function of a measurement instrument
In healthcare, various types of measures are used such as surveys, questionnaires, assessment booklets with matching stimuli, specialty devices, and analysis checklists. All of these fall under the category of measurement instruments, measures, or scales. Throughout this manuscript, the term ‘measurement instrument’ or ‘instrument’ will be used to refer to all these concepts for the sake of clarity. Measurement instruments in healthcare can be classified into two broad categories: clinician-reported measures and patient-reported outcome measures (PROMs). Clinician-reported measures are based on the observations, ratings, and assessments made by the clinician. In contrast, PROMs are measurement instruments based on the perspectives and experiences of patients, family members/caregivers, or others affected by the target issues. PROMs are designed to capture the patient’s subjective view of their health status, function, symptoms, and quality of life (De Vet et al., 2011).
Measurement instruments can be further divided into three components: domains, items and response scales (Supplementary Figure S1 describes an applied example). The domain is the content area under investigation, the items are the aspects of the domain that are of interest, and the response scales are the organization of the items into a measurable form (i.e., orderly and unambiguous description or marker of the degree to which the domain is present) (Streiner et al., 2015; Lambert and Newman, 2022). Response scales come in various forms – for example, they may be continuous, dichotomous, ordinal, nominal or interval (Streiner et al., 2015). One of these may be superior, depending on the question the clinician seeks to answer and the construct or dimension under examination. The construct may be considered the core of the instrument. It is the well-defined hypothetical phenomenon, dimension, attribute, factor or concept that the clinician or researcher is interested in measuring (De Vet et al., 2011). It may or may not be possible to observe the construct or dimension directly.
2.2. The hypothetical construct
Most of what is assessed in healthcare is a latent variable reliant on a proxy which is then translated into numerical values. In strict terms, the numbers on a thermometer are not a measure of body temperature. They measure the amount of electrical voltage passed through metal electrodes, which changes depending on how warm or cool the metals are. The numbers of a scale, Celsius or Fahrenheit, are then applied to the resulting resistance, which correlates to the latent variable – human body temperature.
Since it is not feasible or possible to directly observe the latent variable, the challenge in measurement is determining how well the observable maps onto the latent variable (Sechrest, 2005). The issues that these present are outlined in the example in Box 2.
BOX 2. The latent variable: blood pressure and the sphygmomanometer.
Blood pressure can be measured in various ways/positions (standing, sitting, supine, upper arm, ankle) and using different methods (gradient, diastolic/systolic pressure difference) with the application of a pressure cuff (sphygmomanometer). However, the relationship between the instrument's technical properties and the construct (blood pressure) is not as straight-forward as may be assumed. The sphygmomanometer does not directly measure blood pressure but instead uses a strain gauge to convert mechanical pressure into a rise or fall of liquid in a tube (originally mercury). It is then converted to numbers as a proxy for a patient's blood pressure level. The pressure readings are inferred to represent the pressure in blood vessels, but the movement of mercury has no direct relationship to blood pressure (Sechrest, 2005).
Temperature, described above, is often classed as an ‘objective’ measurement. Other common measures in this category include physiological indicators like heart rate, conductance, or even oxygen saturation readings from pulse oximeters. These measurements are considered ‘objective’ because they are typically obtained through standardized and quantifiable methods that are not influenced by subjective judgment or interpretation. However, like temperature these ‘objective measure’ serve as proxies for the underlying construct. As the latent variable moves from the realm of the tangible and observable to the abstract, the bridge between instrument and variable becomes more tenuous, with increased potential for error. The issues of the distance between constructs and their representative proxies are exemplified in the discipline of psychology. For example, constructs and dimensions are constantly evolving, as represented by the 70-year history of dynamic shifts in the Diagnostic and Statistical Manual of Mental Disorders (DSM). A cornerstone of Western medicine and psychiatric treatment, the DSM categories and descriptions are created through consensus and represent their time’s prevailing socio-political, ideological, empirical, and medical knowledge (North, 2015). Changes in the conceptualization of a construct or dimension inevitably result in changes to what, why, and how it is measured.
2.3. The consequences of flawed measurement
Unclear, poorly constructed, culturally biased, or statistically flawed instruments can lead to a multitude of negative consequences for individuals, families, and the wider community. Poor instruments may cause misdiagnoses in healthcare, with subsequent harmful interventions, suffering, and increased morbidity and mortality. This can also lead to reduced quality of life, as well as discrimination and marginalization for patients who are incorrectly labeled with conditions associated with stigma (McClimans, 2017). In the broader context, poor healthcare measurement instruments can result in policies that support or fund unhelpful treatment options. Healthcare providers and funding bodies may waste economic resources on ineffective interventions. In the research sphere, poor instruments can result in the unethical waste of resources, harmful science practices, and the dissemination of false or misleading information (Mokkink et al., 2018).
The issue of poor measurement instruments is demonstrated by Marshall et al. (2000), who examined 300 randomized controlled trials selected from the Cochrane groups register. Authors examined the instruments used in each study as published (in a peer-reviewed journal) or unpublished (i.e., scale or instrument designed by study authors or no published psychometric evidence about the scales or instruments used for data collection purposes). Randomized controlled trials were 40% more likely to claim treatment effects when using unpublished instruments. In non-pharmacological trials, one-third of claims of treatment superiority would not have been made if a published scale or instrument had been utilized. Authors hypothesized that this result might have been linked to unsound instruments, with trials that used unpublished instruments that had dropped unfavorable items (i.e., those that contradicted findings of treatment effectiveness). As the scales and instruments were unpublished, this adjustment (which contravenes good measurement practices) could occur without reviewers or editors being aware. Authors recommended that future research study manuscripts submitted for peer review should not use unpublished (and it may be inferred, unvalidated or unsound) instruments (Marshall et al., 2000). In other words, if studies submitted for review did not meet the inclusion criteria of using instruments with documented evidence of their psychometric properties, then the manuscript would be rejected before going out for peer review.
3. Key concepts
3.1. Psychometrics
Measurement is a wide and diverse field that encompasses various methods and techniques used to quantify and evaluate different attributes, properties, or characteristics of objects, events, phenomena, or concepts. It encompasses physics, engineering, economics, sociology and healthcare. In this broad field of measurement, various subfields and specialized areas exist, each dealing with specific aspects of measurement. One of these subfields is psychometrics, which is of critical significance in health care. In psychometrics, measurement properties are considered the characteristics of an instrument that describe its qualities of accuracy (validity) stability/reproducibility (reliability) and responsiveness. These qualities are therefore commonly known as the ‘psychometric properties’ of an instrument. It should be noted that validity or reliability are not immutable characteristics inherent to a measurement instrument. Instruments possess varying levels of these properties, which are influenced by the construct or factor they are measuring, the context in which they are being applied (including the target population and rater), how they are used and what the scores generated by the instrument are being used for. The latter consideration alludes to the consequence of the scores generated, with some assessments considered ‘high stakes’ (i.e., eligibility for admission to medical school or being selected as a heart transplant recipient). In contrast, others are considered ‘low stakes’ (i.e., ranking within a large group of kindergarten students on school readiness skills). Target population may influence psychometric properties; a quality-of-life instrument may demonstrate strong convergent validity with functional health status instruments in a group of oncology patients but not among patients whose primary concern is diabetes. This may be because the health-related quality of life instrument’s items were designed to link to a construct specific to oncology patients. Sechrest (2005) posits, ‘It is not measures [instruments] that are valid, but the scores that they yield and the interpretations we make of them’. This statement is perhaps an oversimplification of measurement properties, but it highlights a critical point: instrument accuracy depends on the clinician’s ability to make meaning of the scores. If the clinician administers the instrument in a non-standard manner or an untested population, scores will be affected, and validity and reliability may be severely compromised. A grasp of psychometrics is therefore an essential of good measurement and good practice. Foundational theories and terminology of psychometrics will be briefly outlined in the subsections below.
The term psychometrics arises from disciplines that were early adopters of scale and instrument creation, where decisions were based on measurement and clinical evaluation – psychology and education (Streiner, 2003). Psychometrics owes its methodological heritage to these disciplines, and is where key measurement theories such as classical test and item response theories originated (De Vet et al., 2011). Item Response Theory (IRT) and Classical Test Theory (CTT) are both methods for evaluating instrument scores and measuring patient traits. However, they differ in their approach and underlying theoretical assumptions.
3.1.1. Classical test theory
CTT may be considered a ‘traditional’ approach to statistical analysis of reliability (i.e., the degree to which the measurement is free from measurement error) and validity (i.e., the degree to which the instrument truly measures what it purports to measure) (Mokkink et al., 2018). It includes well-known techniques, such as factor analysis, used to analyze numerous healthcare instruments (DeVellis, 2006; Cappelleri et al., 2014). The key concept of both CTT and IRT is that of the latent variable; the target of interest is an unobservable phenomenon. CTT holds that the scores produced by the instrument is a combination of the latent variable (the ‘true score’) and all the errors contributed by other influences on the observed variable (DeVellis, 2006). For example, a self-report pain instrument with a rating scale ranging from 0–10 may have errors introduced due to individual variation in pain thresholds, differences in how respondents understand the concept of pain, phrasing of the instrument items or differences in administration by clinicians. Importantly, statistical analyses utilized in CTT assume that item scores are the ‘true score’ plus evenly distributed random error. This assumption is linked to a notable limitation of CTT; as a result of this assumption, errors related to different items in the response scale can negate each other. This means an instrument with many items is more likely to have a balanced random error distribution. Instrument reliability can therefore be inflated by item numbers, rather than properties of individual items (i.e., the presence of highly reliable items). Redundancy (including multiple items or very similar questions in the instrument) is a foundation of precision in CTT, as it yields stronger scores on correlation analyses. The quest for item redundancy may lead the scale developer to include an excessive number of items or irrelevant items and thus incentivizes the use of semantically and syntactically uniform question structure (e.g., Do you often feel sad? Do you often feel unhappy? Do you often feel miserable?). This can undermine the accuracy and effectiveness of the instrument, as the inclusion of extraneous or overly similar items that do not truly access the underlying construct can result in a less comprehensive or accurately representative sample of the latent variable. CTT-based instruments also tend to be more sensitive to center scores (i.e., scores in the 4–6 range on a 10-point rating scale) than extremes (scores near 1 or 10 on a 10-point rating scale). Where a respondent’s score is on the extreme ends of a scale, differences in the latent variable (i.e., levels of disability, change in the quality-of-life status, or developmental change) may be poorly distinguished or outright missed. For example, a two-point drop from three to one could represent a greater degree of disability than a change from seven to five (DeVellis, 2006).
3.1.2. Item response theory
A relatively modern psychometric paradigm is item response theory (IRT). Like CTT, IRT also assumes the latent variable (underlying trait) is the target of interest but approaches the measurement of the target factor or dimension differently. While CTT looks at the performance of the instrument as a whole, IRT has a ‘micro’ focus (Cappelleri et al., 2014) where it investigates each item individually, allowing for the identification of strong or weak items. This means weak items can be readily identified, revised or removed during the instrument development phase. A further advantage of item-level validation is that they remain valid, even if only a subset of items is administered. In contrast, if a CTT-based instrument is administered incompletely, the resulting score is no longer valid. The latent variable in IRT is based on interval scaling, while in CTT, it is ordinal (Kean and Reilly, 2014). Interval level measurement involves measuring a latent variable using uniform units, while ordinal level measurement involves ranking or rating the latent trait’s components. Ordinal level measurement is less precise and involves inferences; that is, intervals between values are not equal or quantifiable. When interpreting ordinal data, inferences are made based on the rank order of values, assuming higher ranks indicate greater amounts or degrees, but the actual differences between values may vary. In contrast, interval measurement is more exact and standardized. Both interval and ordinal scales can quantify the degree or amount of the latent variable present, but interval scaling provides a more detailed description.
This distinction becomes clearer when considering an analogy from horse racing, illustrated by Kean and Reilly (2014): ‘the results of a horse race can be reported as 1¼ mile times (i.e., interval), as horse lengths of victory (i.e., interval; approximately 8 feet), or as ordinal finish position listed nominally—win, place, show (i.e., ordinal), or as a numerical order—1st, 2nd, 3rd (i.e., ordinal). Ordinally scaled nominal or numerical finish position tell you which horse was best, whereas interval scales such as finish times and lengths of victory tell you how much better the winner was compared with the other horses’ Thus, a CTT-based scale can provide a relative ranking, describing which patient has the most pain. In contrast to CTT, IRT-based instruments describe how much pain patient A experiences compared to patient B. IRT analyses has further advantages; they can tolerate incomplete data sets, and some IRT approaches can provide rankings of individual scale items regarding respondent ability and item difficulty. However, IRT also has limitations – the large sample size required for development (up to 1,000 participants in some models) may be problematic from cost-effectiveness and feasibility perspectives (Kean and Reilly, 2014).
3.1.3. Psychometrics versus clinimetrics versus measurement properties
Lastly, it must be acknowledged that some alternative terms for psychometric properties have been proposed. This includes ‘clinimetrics’ (Feinstein, 1987) which has been suggested to be more attractive to medical and healthcare clinicians who might be uncomfortable with terminology and processes inherited from psychology (Streiner, 2003; De Vet et al., 2003a). Clinimetrics has been further proposed to differ from psychometrics in some methodological issues (De Vet et al., 2003b). However, authors have also suggested that the dichotomy between the two terms is negligible (Streiner, 2003; De Vet et al., 2003a), preferring the term ‘measurement properties’ (De Vet et al., 2011). Readers investigating the field of measurement instrument development and validation should be aware that all three terms may be encountered and broadly describe the same concept; the statistical properties of an instrument that delineate its qualities pertaining to validity, reliability and responsiveness. This manuscript will use ‘psychometrics’ as the preferred terminology, in acknowledgement of the dominance of the term in the field of healthcare measurement (i.e., the search term ‘psychometric*’ resulting in >110,000 abstracts and ‘clinimetric*’ in ~1,500 PubMed free-text search results as of January 2023).
3.2. The COnsensus-based standards for the selection of health status Measurement INstruments
The (COnsensus-based Standards for the selection of health status Measurement INstruments) COSMIN initiative was established in 2005 in response to issues in the measurement literature – extensive unclear, imprecise, or conflicting terminology and definitions for measurement properties, gaps in evidence for many outcome measurement instruments and inconsistency of methodologies used to demonstrate evidence of measurement properties. An international consortium of scientists with specialties in epidemiology, psychometrics, medicine, qualitative research, and healthcare collaborated, intending to improve the quality of studies of measurement properties of clinical scales and instruments. The team mobilized their expertise in measurement design and validation to develop the only international, consensus-based framework for terminology, preferred analysis and reporting of measurement properties. This has led to the development of several resources for evaluating and improving the quality of instruments, including a taxonomy, risk of bias tool, study design checklist, reporting guidelines, a guideline for conducting systematic reviews, and a guideline for selecting measurement instruments for a core outcome set (Mokkink et al., 2018, 2020).
The COSMIN taxonomy categorizes measurement properties of health outcome measurement instruments into three domains: reliability, validity, and responsiveness. These are a broad category of measurement properties that the COSMIN group classifies under nine measurement properties: content validity, structural validity, internal consistency, cross-cultural validity, measurement invariance, reliability, measurement error, criterion validity (which includes criteria for sensitivity and specificity for dichotomous scores), hypotheses testing for construct validity, and responsiveness (Mokkink et al., 2018). Table 1 in the supplements provides a detailed explanation of each of these properties, along with clinical examples to illustrate their application. This table is a conceptual guide to the COSMIN measurement properties and their statistical analysis. Table 2 in the supplements shows how various terms used in psychometric literature are mapped to the COSMIN terminology.
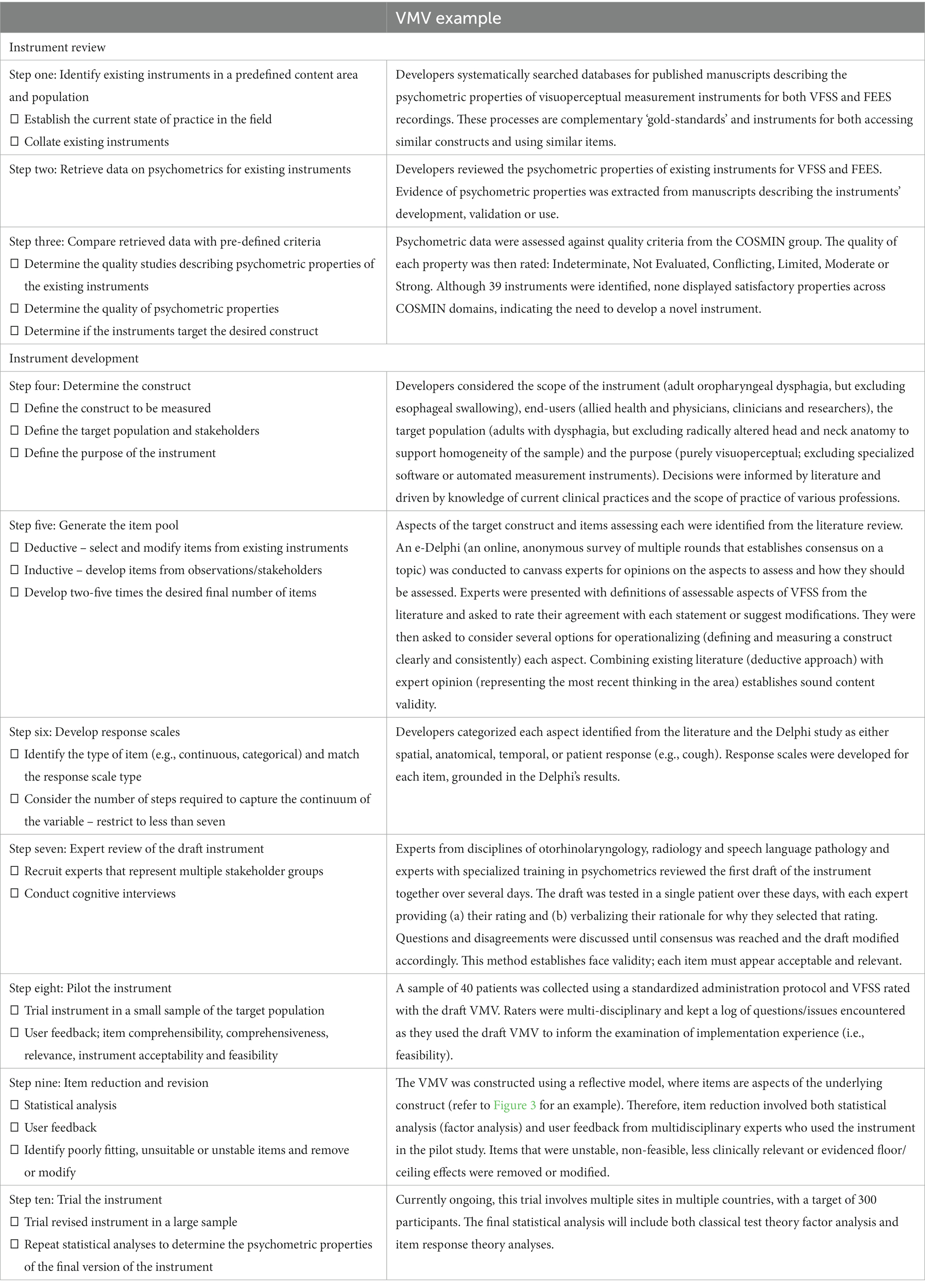
Table 2. Instrument development and review practices applied examples from the VMV (Swan et al., 2022).
Since its inception, COSMIN tools and taxonomy use has experienced substantial growth. The COSMIN group maintains a database of systematic reviews of studies of measurement properties of instruments in healthcare. Although the COSMIN framework was developed specifically for evaluating Patient-Reported Outcome Measures (PROMs), the framework’s principles can be adapted and applied to other types of measurement instruments, including clinical observation instruments (Mokkink et al., 2018). To date, over 350 of the systematic reviews utilized COSMIN terminology or tools.1 The COSMIN taxonomy and tools represent international, research-based practice in measurement and statistics for instruments in healthcare. Therefore, this manuscript will consider measurement properties and instrument development through the lens of the COSMIN.
3.3. Describing and understanding the properties of a measurement instrument
Instrument development necessitates the integration of a robust theoretical framework, sound methodological protocols and appropriate statistical analyses. The results of these analyses describe the properties of an instrument. Like any field of science, there are a variety of methodologies with varying strengths and weaknesses in different statistical approaches. The COSMIN group recommends preferred statistical analyses, sound study design and criteria for good measurement properties (Mokkink et al., 2020). Table 1 lists preferred analysis methods for the psychometric properties recommended by the COSMIN group and briefly introduces the interpretation of these analyses. This table provides an accessible, non-mathematical overview of the suggested statistical analyses, focusing on concepts rather than procedural knowledge. It is designed to support non-statisticians at an introductory level.
4. Instrument development
When the clinician, academic educator, or researcher finds the available instruments are inadequate regarding psychometric properties or feasibility or a poor match to their target population or question, they may be interested in developing a new one. The following section illustrates one possible path for instrument development. Examples are drawn from the visuoperceptual measure for videofluoroscopic swallow studies (VMV), a recently developed instrument for the analysis of videofluoroscopic studies of dysphagia, dynamic x-rays of difficulty swallowing, developed in adherence with COSMIN guidelines and summarized in Table 2 of this manuscript. The ten-step process discussed below briefly demonstrates COSMIN guidelines in the applied practice of instrument development, with the process summarized in Figure 2.
4.1. Step one: identify existing instruments in a predefined content area and population
As with all scientific endeavors, a thorough understanding of existing work and gaps in the respective field is required initially. A detailed and rigorous examination of the existing instruments will result in common themes and identify gaps and future directions. Reviewing the related empirical research literature should be critical and systematic (Streiner et al., 2015; Page et al., 2021). The VMV began with a psychometric review, wherein all visuoperceptual measurement instruments (those which rely on human visual inspection and judgment of images) for ‘gold-standard’ technical assessments of swallowing were collated, and their measurement properties critically examined according to COSMIN quality criteria (Swan et al., 2019). In this context, the term “gold standard” refers to the highest achievable standard backed by evidence and expert agreement (Ralph et al., 2015). The psychometric evaluation therefore focused on instruments used in the analysis of Videofluoroscopic Swallow Studies (VFSS) and Fiberoptic Endoscopic Examinations of Swallowing (FEES) as there is currently no agreement on which measure of VFSS or FEES can be considered a gold standard (Giraldo-Cadavid et al., 2017). The COSMIN initiative specifies detailed recommendations for conducting a systematic review of measurement properties.
4.2. Step two: retrieve data on psychometrics for existing instruments
Experts in health-scale measurement and psychometrics agree that novel instrument development should only be undertaken if absolutely necessary (De Vet et al., 2011; Streiner et al., 2015). Quality instrument development is a time-and resource-intensive process that may take months or years. Clinicians, academic educators, and researchers should consider whether a novel instrument is truly required. This decision should be informed by both the results of step one and their intended purpose for the new instrument. The psychometric review that led to the development of the VMV identified 39 instruments across 45 studies (Swan et al., 2019). Ideally, step one will identify one or more instruments with sound psychometric properties that meet the needs of the clinician, educator or researcher and are validated for use in the target population. However, before selecting an instrument, the healthcare professional must evaluate the quality of existing instruments to determine which is best suited for their intended purposes. This includes considering the instruments’ psychometric properties, as well as their validation in the specific target population of interest.
4.3. Step three: compare retrieved psychometric data with pre-defined criteria
The psychometric data retrieved in the review must be assessed with quality criteria defined a-priori. The COSMIN group generated specific quality criteria per each aspect within domains of validity and reliability and interpretability (Mokkink et al., 2018). For example, 75% of formulated hypotheses for construct validity should be confirmed to meet the requirements for good measurement properties. Hypotheses can refer to the expectation of no significant changes between genders or age groups where age or gender would not mediate the disease. Uld not mediate the disease. The COSMIN initiative provides detailed guidance for this process [7] An instrument may be used without modification if it demonstrates adequate psychometric properties and is both suitable and feasible for the intended target participant group. Often this is not the case, and the measurement instrument requires modification to meet the intended purpose (such as trialing in the target population). If an instrument can be modified to suit the intended purpose, it will require testing before its reliability, validity, and responsiveness can be assumed. Psychometric properties are ‘non-transferrable’ – modification to the instrument or use in an untested population may introduce unknown variables that affect instrument performance and accuracy. The COSMIN guidelines provide instructions on assessing the cross-cultural validity of an instrument and should be applied in this situation (Mokkink et al., 2018).
Alternatively, the clinician, educator or researcher may conclude that instrument development is required. Where there is a need for existing instruments in the construct of interest and target population (e.g., age, diagnosis), unsound psychometrics and poor feasibility of existing measures, the development of the needed instrument should progress. In the case of the VMV’s psychometric review, none of the existing 39 measures demonstrated adequate psychometric properties to recommend them for clinical practice or use in research (Swan et al., 2019). It is beyond the scope of this paper to elaborate on specific methodology for psychometric review and quality analysis; interested readers should refer to COSMIN texts (De Vet et al., 2011; Mokkink et al., 2018) and may refer to Swan et al. (2019) for an example of the review and quality analysis.
4.4. Step four: determine the construct(s)
To develop the VMV, the construct of interest required a formal definition. The construct is the ‘well-defined and precisely demarcated subject of measurement’ (De Vet et al., 2011). It should be defined a priori and specific to the instrument’s intended function. The importance of a well-described construct is particularly evident in the context of an unobservable target construct, a phenomenon common to health-related constructs. It is critical to be precise and unambiguous on what is to be measured and how it differs from other constructs (DeVellis and Thorpe, 2021) as non-specific instruments risk missed or inappropriate diagnoses, inaccurate treatment monitoring or unclear outcome measurement instruments. If a construct is multidimensional, the instrument developer must be clear on the aspect(s) to be measured – for example, anxiety contains multiple aspects linked to personality, stimulus, and contextual situation. The target population (patients and the stakeholders who will use or interpret the instrument) and the purpose of measurement should also be considered at this stage (De Vet et al., 2011). Health measurement instruments may broadly be classified as diagnostic, prognostic or evaluative (McDowell, 2006).
Diagnostic instruments refer to devices or measurement tools that aid in clinical diagnosis. Some examples include sphygmomanometers for hypertension, mental health self-report surveys for anxiety or in this case and VFSS for oropharyngeal dysphagia, and. Prognostic measurement instruments include screening tests like Apgar scores (Apgar, 1952) and the Geriatric Depression Scale (Janneke et al., 2012); these measure future potential of function or dysfunction. Evaluative instruments track change over time. More discrete divisions of the scope of the instrument are also possible, such as discriminative (classifying individuals based on IQ) or instruments concerned with a particular aspect of health, such as quality of life (McDowell, 2006). Clinicians/educators/researchers engaged in instrument development must match the purpose of the instrument to the appropriate instrument design. In the case of the VMV, literature and expert consultation was used to determine the scope and design of the instrument (i.e., excluding esophageal dysphagia from the construct of ‘disordered swallowing’ for the purposes of this measure, creating a measure that was visuoperceptual to reflect both current practices and support future ease of use and access for sites unable to purchase specialty software).
4.5. Step five: generate the item pool
The item pool can be developed after clearly delineating the construct and related aspect(s). At this stage, having clear specifications or operational guidelines for creating the “item pool” is essential because it helps prevent potential bias. Without such guidelines, there’s a risk of disproportionately emphasizing certain indicators over others during the initial phase. This imbalance in measurement can subsequently influence the definition of the construct, leading to an inaccurate representation of the intended concept. There are multiple possible methods, including the ‘deductive’ and ‘inductive’ approaches. In the deductive method, scale item generation results from completing a literature review and collecting and selecting items from previously designed instruments (Boateng et al., 2018). Publicly available item banks of instruments with known psychometric properties, such as the Patient-Reported Outcomes Measurement Information System (PROMIS) and the library of clinical outcome assessments,2 may assist the clinician, educator or researcher at this stage. In contrast, the inductive method relies on key stakeholders’ input to generate items, which may include focus groups, surveys, or observational/exploratory research develops items (Boateng et al., 2018). Focus groups may involve a small group of stakeholders impacted by the target disorder interviewed by a facilitator, with answers recorded, transcribed and analyzed. Care must be taken with selection of the group members with regards to hetero or homogeneity, depending on the aims of the interview. Key informant interviews involve more detailed interviews with a small number of crucial participants with deep subject knowledge. Interviews typically continue until saturation is reached – i.e. interviewing additional informants does not add new knowledge. Qualitative approaches such as these may be an excellent source for developing items assessing subjective symptoms (Streiner et al., 2015). It should be noted that crowd-sourcing of items requires instrument developers to have the construct well-defined to develop well-worded questions that elicit useful responses (De Vet et al., 2011). Combining both methods will likely be most efficacious in most cases, as the literature review provides a sound theoretical basis, while iterative, qualitative data collection generates items closely aligned to the intended population or purpose (Boateng et al., 2018).
Items should be worded with great care. The instrument’s quality largely depends on the quality of its items; that is, it’s content (Streiner et al., 2015). Content validity describes the correspondence of the contents of the instrument to the target construct and is established through relevance and comprehensiveness of the instrument’s items (De Vet et al., 2011). Assessment of content validity is further outlined in Table 1. The COSMIN initiative provides detailed instructions for reviewing content validity (Mokkink et al., 2018). Item phrasing and language should therefore be specific, unambiguous, constrained to one concept per item, avoid negative wording, and be at an appropriate reading level, especially in consideration of the target population’s general level of education and health literacy (De Vet et al., 2011). At this stage in instrument development, the clinician, educator or researcher should aim for the similarity between items. This similarity is termed ‘redundancy’ and is a desirable feature in the early stages of scale item generation (Streiner et al., 2015). As the items are intended to measure the same construct, multiple similar items support good content coverage – that is, a comprehensive and representative sample of the components and aspects that make up the target construct are included (DeVellis and Thorpe, 2021). The consequence of redundancy is that the initial item pool will be considerably larger than the intended end product; authors have suggested that the initial item pool may be two to five times greater than that of the final version of the instrument (Boateng et al., 2018). The VMV’s initial pool included 97 items, developed through both a deductive and inductive approach; that is, literature review, review of existing instruments, consultation with key stakeholders and crowd-sourcing items through the e-Delphi approach (Swan et al., 2022). Step nine of the instrument development details removal and refinement of the items that do not meet pre-defined criteria for internal consistency (excessively or insufficiently high Cronbach’s Alpha).
4.6. Step six: develop response scales
A response scale is the orderly quantification of the item (an aspect of the construct under assessment). Broadly speaking, response scales may be categorical (religion, marital status, eye color) or continuous (mass, pain rated on a 100 mm line, temperature). More specifically, in the categorical division, scales could be nominal (two response categories; e.g., hot or cold, inside or outside, urban or rural) or ordinal (categories with natural ordering; e.g., very unhappy, unhappy, neutral feeling, happy, very happy). If the distance between each step in the scale is equal, the scale is called interval. The variable being measured must match the response scale. For example, an interval scale could measure body temperature in degrees Celsius or be rated as very low, low, within the expected range, high, or very high (Streiner et al., 2015). The type of response scale used informs the response options’ design, formatting, and wording. Certain items may only be suitable for specific types of response scales. A dichotomous scale must have unambiguous language, as there is no middle ground. In contrast, a Likert-type scale must ensure that both extremes of the variable (e.g., strong presence vs. total absence) are clearly captured at the start or end of the scale (Boateng et al., 2018). The steps in the response scale should be presented in a logical order (least to most), and the number of points on the scale should be limited to seven or fewer. The human capacity to process distinctions in categories becomes an unwieldy cognitive load above this number (Miller, 1956). The type of response scale should also consider the target respondent group. For example, response scales used with children would need to consider their developmental level and response scales used with respondents with diverse cultural backgrounds would need to ensure that the wording of items is culture and bias free.
The items of the VMV were developed using the e-Delphi process. The constructs to be assessed were operationalized into a measurable format, such as the fraction of a bolus, the anatomic space filled, or the temporal or spatial movement of a swallowing structure. These were then refined into ordinal scales with less than seven response options that corresponded to the degree of presence or absence of the constructs.
4.7. Step seven: expert review of the draft instrument
A group of experts should review the new draft instrument. Ideally, this should include individuals or groups who are the target end users of the instrument and highly experienced in psychometrics. The group should assess the instrument’s face validity and refine the measurement instrument before pilot testing it. Adequate face validity indicates that the instrument fits the target construct (Boateng et al., 2018). Cognitive interviews are recommended when the unconscious mental processes of completing the instrument or interpreting its items are explicitly verbalized (De Vet et al., 2011; Boateng et al., 2018). The VMV utilized a multi-disciplinary group of experts to provide feedback on aspects of its items in testing the measure across multiple patients (Swan et al., 2022).
4.8. Step eight: pilot testing the instrument
The next step in instrument development is the pilot testing process. The first draft should be tested in a small sample of the target population (24–36 respondents are suggested for this phase) (Johanson and Brooks, 2010) and then refined with due consideration of the psychometric performance and user feedback on the instrument’s implementation and interaction experience. Important concepts to consider in user analysis of the pilot version of the instrument are item comprehensibility, relevance, instrument acceptability, feasibility, the cognitive load required to answer items, time taken to complete items, and comprehensiveness. Acceptability is whether participants are willing to undertake the measurement test or activity, while feasibility denotes whether they have the ability to do so (De Vet et al., 2011). The VMV was piloted with 40 patients, and the measurement instrument tested by a speech-language pathologist two physicians with specialties in radiology and ENT-phoniatrics (Swan et al., 2022).
4.9. Step nine: item reduction and revision
After the pilot testing phase and the results are analyzed, item reduction and revision may occur. Reduction and revision involve removing or modifying items that were unsuitable from user experience perspectives or quality of psychometric properties. Items may be deleted outright, moved to another section of the instrument, collapsed with another item (or more) for a single item, or (more rarely) split to make two novel items. Rating scales are also subject to modification, with removal, rewording or collapse of steps. Item reduction and revision ensure that only the items with the strongest relationship to the construct remain (Boateng et al., 2018). The VMVs items were revised through cognitive interviewing with the raters and statistical analysis to remove or modify weak items (Swan et al., 2022).
The underlying theoretical underpinnings inform item reduction and revision processes of the instrument; that is, whether it was formed using a reflective or formative model (i.e., where the items are assumed to be accessing a single underlying construct or trait versus an instrument that is tapping into multiple sub-constructs or components that make up target construct). Figure 3 demonstrates an applied example of these models, where the items ‘aspiration’, ‘pharyngeal residue’ and ‘piecemeal deglutition’ arise from the single construct ‘dysphagia’ compared to the construct ‘academic achievement, which is comprised of sub-constructs ‘socioeconomic status’, ‘internal motivation’ and ‘language proficiency’.
In the reflective model, factor analysis and item correlation guide both item reduction and identification of viable dimensions. Patient judgment of the importance of items guides retention or rejection of items in instruments constructed with the formative model, such as self-report surveys (De Vet et al., 2011).
4.10. Step ten: trialing the instrument
The natural consequence of item reduction and revision is creation of an instrument that is substantially different to the version that was piloted. The VMV was reduced in length by ~50% between the pilot and trial phases (Swan et al., 2022). The new, reduced version of the instrument requires testing and evaluation of psychometric quality in a larger sample of the target population (De Vet et al., 2011). This version of the instrument will be penultimate; developers can expect to require further revisions after trial and psychometric analysis. At this stage, statistical analyses (e.g., factor analysis, LOA) are appropriate; however, these may require a large sample (in the hundreds, in the case of IRT) to meet their statistical assumptions (Kean and Reilly, 2014). A final round of item removal and edits will ensue, with repeated statistical analyses to judge the impact of individual items and response scales on instrument psychometric properties. Once a satisfactory instrument structure has been attained, the final version is ready for clinical use.
5. Discussion
This paper introduced key concepts in psychometrics for healthcare clinicians, academic educators, and novice researchers interested in measurement and outlined the process of best-practice instrument development and validation in a practical, accessible, manner. This paper used the recently developed VMV (29) as an exemplar of one possible pathway for instrument development that adheres to the COSMIN initiative recommendations and guidelines.
This manuscript has discussed the complexities inherent in measurement in healthcare, the ballung concepts and latent constructs. It has outlined that while validity and reliability constitute important measurement instrument quality domains, validity is the most critical domain. Reliability is moot if an instrument has strong reliability but items that do not adequately represent the latent target variable, regardless of whether the instrument is repeatable or consistent. In other words, validity trumps reliability in the psychometric property hierarchy in instrument development (Lissitz, 2009). An instrument that is reliable but does not have evidence of validity is useless, precisely because of the issues arising from ballung and latent constructs. In most cases, the target construct under investigation in healthcare is often high-stakes, involving a life-threatening disease, expensive long-term management, and significantly impacting quality of life. Therefore, adequate evidence is required before a measurement instrument can be trusted. Psychometrics can provide that empirical evidence that underpins sound measurement (Vetter and Cubbin, 2019). The COSMIN initiative is a resource that can guide clinicians and researchers interested in addressing instrument quality and development.
5.1. Poor measurement in healthcare – a widespread issue
Unfortunately, poor measurement in healthcare is widespread. In 2011, authors (Cano and Hobart, 2011) noted the lack of quality psychometrics of instruments used in healthcare settings, evident in even the most cursory review of the peer-reviewed literature. The authors gave the example of a superficial literature review of randomized controlled Phase III and IV trials in multiple sclerosis published in 2006–2011. Fourteen of 28 articles used some type of rating scale, but only two studies used scales with any published psychometric evidence. Over a decade later, the situation remains essentially unchanged. In a review of 80 outcome measures used for 100 randomized controlled trials in narcolepsy interventions, authors found ‘surprisingly little evidence for the validity, reliability, and responsiveness of [the instrument] frequently used to assess treatment efficacy in narcolepsy’ (Schokman et al., 2022). Unfortunately, this pattern is repeated in various disciplines, across different populations and diagnostic categories. In numerous reviews, authors consistently find that the psychometric evidence for instruments is poor, limited, or lacking in quality (Crellin et al., 2015; Cassidy et al., 2018; Ghai et al., 2022; Thompson et al., 2023).
The dearth of quality instruments in healthcare clearly indicates to the clinicians, educators, and researchers that it is in the patients’ and health professionals’ best interests to engage with psychometrics and upskill in knowledge in the field. Too often, instruments are developed poorly, leading to all subsequent data and treatment findings based on their data being questionable (Schokman et al., 2022). Instrument development is a dynamic, iterative process involving creativity, theory, statistical evaluation, and dedication from the instrument authors. Instrument development should not cease at the first draft; rigorous field testing using best-practices and appropriate statistical analysis is required (De Vet et al., 2011).
5.2. Implications for clinicians, educators, and researchers
• Measurements matter. Healthcare professionals should understand the importance of well-designed and feasible measurement instruments with robust psychometric properties in their work. These instruments play a crucial role in evidence-based practice and good research, as they provide a way to assess the populations, interventions, or other instruments accurately.
• The COSMIN (COnsensus-based Standards for the selection of health Measurement INstruments) initiative provides a wealth of materials and guidelines to support researchers and clinicians in selecting and using psychometric instruments. COSMIN resources can assist clinicians in developing, researching, and selecting measurement instruments with robust psychometric properties that are appropriate for their intended use.
• Psychometrics apply to healthcare across all disciplines, from mental health to internal medicine to rehabilitation science.
• The development and validation of the VMV is one example of applied COSMIN initiative recommendations and may serve as a useful guide for clinicians and researchers interested in instrument development or validation.
• This manuscript calls for a concerted effort among clinicians interested in psychometrics research, serving as a rallying point by presenting key concepts and principles and providing guidance for their practical application in instrument development and selection.
Author contributions
KS, RS, TB, and RC contributed to conception and design of the review. MS, DF, and VW provided data used in the VMV development and contributed to validation, the processes of which are described in this manuscript. KS and RS: original draft preparation. TB and RC: review and editing. All authors have read and agreed to the published version of the manuscript.
Funding
The authors wish to acknowledge Curtin University and the Australian Federal Government for the Curtin University Postgraduate Scholarship (CUPS) and the Australian Postgraduate Award (APA).
Conflict of interest
The authors declare that the research was conducted in the absence of any commercial or financial relationships that could be construed as a potential conflict of interest.
Publisher’s note
All claims expressed in this article are solely those of the authors and do not necessarily represent those of their affiliated organizations, or those of the publisher, the editors and the reviewers. Any product that may be evaluated in this article, or claim that may be made by its manufacturer, is not guaranteed or endorsed by the publisher.
Supplementary material
The Supplementary material for this article can be found online at: https://www.frontiersin.org/articles/10.3389/fpsyg.2023.1225850/full#supplementary-material
Footnotes
References
Apgar, V. (1952). A proposal for a new method of evaluation of the newborn. Classic Papers Crit. Care 32:97.
Asuero, A. G., Sayago, A., and González, A. (2006). The correlation coefficient: an overview. Crit. Rev. Anal. Chem. 36, 41–59. doi: 10.1080/10408340500526766
Boateng, G. O., Neilands, T. B., Frongillo, E. A., Melgar-Quiñonez, H. R., and Young, S. L. (2018). Best practices for developing and validating scales for health, social, and behavioral research: a primer. Front. Public Health 6:149. doi: 10.3389/fpubh.2018.00149
Bond, T. G., Yan, Z., and Heene, M. (2020). Applying the Rasch model: Fundamental measurement in the human sciences, New York: Routledge.
Brown, T. A. (2015). Confirmatory factor analysis for applied research. New York: Guilford Publications.
Brown, G. T., Harris, L. R., O'Quin, C., and Lane, K. E. (2017). Using multi-group confirmatory factor analysis to evaluate cross-cultural research: identifying and understanding non-invariance. Int. J. Res. Method Educ. 40, 66–90. doi: 10.1080/1743727X.2015.1070823
Cano, S. J., and Hobart, J. C. (2011). The problem with health measurement. Patient Prefer. Adherence 5:279. doi: 10.2147/PPA.S14399
Cappelleri, J. C., Lundy, J. J., and Hays, R. D. (2014). Overview of classical test theory and item response theory for the quantitative assessment of items in developing patient-reported outcomes measures. Clin. Ther. 36, 648–662. doi: 10.1016/j.clinthera.2014.04.006
Cassidy, S. A., Bradley, L., Bowen, E., Wigham, S., and Rodgers, J. (2018). Measurement properties of tools used to assess suicidality in autistic and general population adults: a systematic review. Clin. Psychol. Rev. 62, 56–70. doi: 10.1016/j.cpr.2018.05.002
Crellin, D. J., Harrison, D., Santamaria, N., and Babl, F. E. (2015). Systematic review of the face, legs, activity, cry and consolability scale for assessing pain in infants and children: is it reliable, valid, and feasible for use? Pain 156, 2132–2151. doi: 10.1097/j.pain.0000000000000305
de Vet, H. C., and Terwee, C. B. (2010). The minimal detectable change should not replace the minimal important difference. J. Clin. Epidemiol. 63, 804–805. doi: 10.1016/j.jclinepi.2009.12.015
De Vet, H. C., Terwee, C. B., and Bouter, L. M. (2003a). Clinimetrics and psychometrics: two sides of the same coin. J. Clin. Epidemiol. 56, 1146–1147. doi: 10.1016/j.jclinepi.2003.08.010
De Vet, H. C., Terwee, C. B., and Bouter, L. M. (2003b). Current challenges in clinimetrics. J. Clin. Epidemiol. 56, 1137–1141. doi: 10.1016/j.jclinepi.2003.08.012
De Vet, H. C., Terwee, C. B., Mokkink, L. B., and Knol, D. L. (2011). Measurement in medicine: a practical guide. Cambridge: Cambridge university press.
DeVellis, R. F. (2006). Classical test theory. Med. Care 44, S50–S59. doi: 10.1097/01.mlr.0000245426.10853.30
DeVellis, R. F., and Thorpe, C. T. (2021). Scale development: theory and applications. Thousand Oaks, SA: Sage publications.
Geerinck, A., Alekna, V., Beaudart, C., Bautmans, I., Cooper, C., De Souza Orlandi, F., et al. (2019). Standard error of measurement and smallest detectable change of the sarcopenia quality of life (Sar QoL) questionnaire: an analysis of subjects from 9 validation studies. PLoS One 14:e0216065. doi: 10.1371/journal.pone.0216065
Ghai, V., Subramanian, V., Jan, H., and Doumouchtsis, S. K. (2022). A systematic review highlighting poor-quality of evidence for content validity of quality of life (QoL) instruments in female chronic pelvic pain (CPP). J. Clin. Epidemiol. 149, 1–11. doi: 10.1016/j.jclinepi.2022.04.016
Gillen, C., and Goyal, A. (2022). “Stable Angina” in Stat pearls [internet]. (St. Petersburg, FL: Stat Pearls Publishing). Available at: https://www.ncbi.nlm.nih.gov/books/NBK559016 (Accessed February 2, 2023).
Giraldo-Cadavid, L. F., Leal-Leaño, L. R., Leon-Basantes, G. A., Bastidas, A. R., Garcia, R., Ovalle, S., et al. (2017). Accuracy of endoscopic and videofluoroscopic evaluations of swallowing for oropharyngeal dysphagia. Laryngoscope 127, 2002–2010. doi: 10.1002/lary.26419
Goldbeck-Wood, S. (2019). “When bodies speak: psychosomatic illness as hidden communication” in Introduction to psychosexual medicine. eds. P. Brough and M. Denman. (Boca Raton, FL: CRC Press), 9–18.
Hagquist, C., and Andrich, D. (2017). Recent advances in analysis of differential item functioning in health research using the Rasch model. Health Qual. Life Outcomes 15, 1–8. doi: 10.1186/s12955-017-0755-0
Hernaez, R. (2015). Reliability and agreement studies: a guide for clinical investigators. Gut 64, 1018–1027. doi: 10.1136/gutjnl-2014-308619
Hinkin, T. R. (1998). A brief tutorial on the development of measures for use in survey questionnaires. Organ. Res. Methods 1, 104–121. doi: 10.1177/109442819800100106
Huzmeli, I., Ozer, A.-Y., Akkus, O., Katayıfcı, N., Sen, F., Yurdalan, S. U., et al. (2020). Comparison of functional exercise capacity, quality of life and respiratory and peripheral muscle strength between patients with stable angina and healthy controls. J. Int. Med. Res. 48:030006052097921. doi: 10.1177/0300060520979211
Janneke, M., Gooskens, F., Schepers, V. P., Schuurmans, M. J., Lindeman, E., and Hafsteinsdóttir, T. B. (2012). Screening for poststroke depression using the patient health questionnaire. Nurs. Res. 61, 333–341. doi: 10.1097/NNR.0b013e31825d9e9e
Johanson, G. A., and Brooks, G. P. (2010). Initial scale development: sample size for pilot studies. Educ. Psychol. Meas. 70, 394–400. doi: 10.1177/0013164409355692
Kean, J., and Reilly, J. (2014). “Item response theory” in Handbook for Clinical Research: Design, Statistics and Implementation. eds. F. Hammond, J. Malec, T. Nick, and R. Buschbacher (New York: Demos Medical Publishing), 195–198.
Lambert, L. S., and Newman, D. A. (2022). Construct development and validation in three practical steps: recommendations for reviewers, editors, and authors. Organ. Res. Methods. doi: 10.1177/109442812211153
Lissitz, R. W. (2009). The concept of validity: Revisions, new directions and applications. Charlotte, NC: IAP.
Marshall, M., Lockwood, A., Bradley, C., Adams, C., Joy, C., and Fenton, M. (2000). Unpublished rating scales: a major source of bias in randomised controlled trials of treatments for schizophrenia. Br. J. Psychiatry 176, 249–252. doi: 10.1192/bjp.176.3.249
McClimans, L. (2017). Measurement in medicine: Philosophical essays on assessment and evaluation. Lanham, MD: Rowman & Littlefield.
McDowell, I. (2006). Measuring health: a guide to rating scales and questionnaires. Oxford: Oxford University Press.
Miller, G. A. (1956). The magic number seven plus or minus two: some limits on our capacity for processing information. Psychol. Rev. 63, 81–97. doi: 10.1037/h0043158
Mokkink, L. B., Boers, M., Van Der Vleuten, C., Bouter, L. M., Alonso, J., Patrick, D. L., et al. (2020). COSMIN risk of Bias tool to assess the quality of studies on reliability or measurement error of outcome measurement instruments: a Delphi study. BMC Med. Res. Methodol. 20, 1–13. doi: 10.1186/s12874-020-01179-5
Mokkink, L. B., Prinsen, C. A., Patrick, D. L., Alonso, J., Bouter, L. M., De Vet, H. C., et al. (2019). COSMIN study design checklist for patient-reported outcome measurement instruments. Available at: https://www.cosmin.nl/wp-content/uploads/COSMIN-study-designing-checklist_final.pdf (Accessed December, 2022).
Mokkink, L. B., Prinsen, C. A. C., Patrick, D. L., Alonso, J., Bouter, L. M., de Vet, H. C. W., et al. (2018). COSMIN methodology for systematic reviews of patient-reported outcome measures (PROMs) user manual. Amsterdam: Department of Epidemiology and Biostatistics. Available at: http://www.cosmin.nl.
North, C. S. (2015). The classification of hysteria and related disorders: historical and phenomenological considerations. Behav. Sci. 5, 496–517. doi: 10.3390/bs5040496
Page, M. J., McKenzie, J. E., Bossuyt, P. M., Boutron, I., Hoffmann, T. C., Mulrow, C. D., et al. (2021). The PRISMA 2020 statement: an updated guideline for reporting systematic reviews. Syst. Rev. 10, 1–11. doi: 10.1186/s13643-021-01626-4
Parikh, R., Mathai, A., Parikh, S., Sekhar, G. C., and Thomas, R. (2008). Understanding and using sensitivity, specificity and predictive values. Indian J. Ophthalmol. 56, 45–50. doi: 10.4103/0301-4738.37595
Pendergast, L. L., von der Embse, N., Kilgus, S. P., and Eklund, K. R. (2017). Measurement equivalence: a non-technical primer on categorical multi-group confirmatory factor analysis in school psychology. J. Sch. Psychol. 60, 65–82. doi: 10.1016/j.jsp.2016.11.002
Polit, D. F. (2015). Assessing measurement in health: beyond reliability and validity. Int. J. Nurs. Stud. 52, 1746–1753. doi: 10.1016/j.ijnurstu.2015.07.002
Portney, L. G., and Watkins, M. P. (2009). Foundations of clinical research: applications to practice (Vol. 892). Pearson/Prentice Hall: Upper Saddle River, NJ.
Prinsen, C. A., Mokkink, L. B., Bouter, L. M., Alonso, J., Patrick, D. L., De Vet, H. C., et al. (2018). COSMIN guideline for systematic reviews of patient-reported outcome measures. Qual. Life Res. 27, 1147–1157. doi: 10.1007/s11136-018-1798-3
Ralph, G., Aigmueller, T., and Riss, P. (2015). The failed idea of a “gold standard”. 26, pp. 1405–1406): Berlin: Springer.
Riemann, B. L., and Lininger, M. R. (2018). Statistical primer for athletic trainers: the essentials of understanding measures of reliability and minimal important change. J. Athl. Train. 53, 98–103. doi: 10.4085/1062-6050-503-16
Schokman, A., Bin, Y. S., Naehrig, D., Cheung, J. M., Kairaitis, K., and Glozier, N. (2022). Evaluation of psychometric properties of patient-reported outcome measures frequently used in narcolepsy randomized controlled trials: a systematic review. Sleep 45:156. doi: 10.1093/sleep/zsac156
Sechrest, L. (2005). Validity of measures is no simple matter. Health Serv. Res. 40, 1584–1604. doi: 10.1111/j.1475-6773.2005.00443.x
Streiner, D. L. (2003). Clinimetrics vs. psychometrics: an unnecessary distinction. J. Clin. Epidemiol. 56, 1142–1145. doi: 10.1016/j.jclinepi.2003.08.011
Streiner, D. L., Norman, G. R., and Cairney, J. (2015). Health measurement scales: a practical guide to their development and use. Oxford: Oxford University Press.
Swan, K., Cordier, R., Brown, T., and Speyer, R. (2019). Psychometric properties of visuoperceptual measures of videofluoroscopic and fibre-endoscopic evaluations of swallowing: a systematic review. Dysphagia 34, 2–33. doi: 10.1007/s00455-018-9918-3
Swan, K., Speyer, R., Scharitzer, M., Farneti, D., Brown, T., and Cordier, R. (2022). A Visuoperceptual measure for Videofluoroscopic swallow studies (VMV): a pilot study of validity and reliability in adults with dysphagia. J. Clin. Med. 11:724. doi: 10.3390/jcm11030724
Thompson, K., Lo, A. H. Y., McGlashan, H. L., Ownsworth, T., Haslam, C., Pegna, A., et al. (2023). Measures of subjective memory for people with epilepsy: a systematic review of measurement properties. Neuropsychol. Rev. doi: 10.1007/s11065-022-09568-x
Vetter, T. R., and Cubbin, C. (2019). Psychometrics: trust, but verify. Anesth. Analg. 128, 176–181. doi: 10.1213/ANE.0000000000003859
Keywords: psychometrics, measurement, instruments, COSMIN, validation, instrument development
Citation: Swan K, Speyer R, Scharitzer M, Farneti D, Brown T, Woisard V and Cordier R (2023) Measuring what matters in healthcare: a practical guide to psychometric principles and instrument development. Front. Psychol. 14:1225850. doi: 10.3389/fpsyg.2023.1225850
Edited by:
Alessandro Giuliani, National Institute of Health (ISS), ItalyReviewed by:
Franca Crippa, University of Milano-Bicocca, ItalyAlicia Boluarte, Cesar Vallejo University, Peru
Copyright © 2023 Swan, Speyer, Scharitzer, Farneti, Brown, Woisard and Cordier. This is an open-access article distributed under the terms of the Creative Commons Attribution License (CC BY). The use, distribution or reproduction in other forums is permitted, provided the original author(s) and the copyright owner(s) are credited and that the original publication in this journal is cited, in accordance with accepted academic practice. No use, distribution or reproduction is permitted which does not comply with these terms.
*Correspondence: Renee Speyer, cmVuZWUuc3BleWVyQGlzcC51aW8ubm8=
†ORCID: Katina Swan, https://orcid.org/0000-0003-4530-5548
Renee Speyer, https://orcid.org/0000-0003-2828-8897
Martina Scharitzer, https://orcid.org/0000-0003-4427-5463
Daniele Farneti, https://orcid.org/0000-0002-8139-4990
Ted Brown, https://orcid.org/0000-0001-9403-5877
Virginie Woisard, https://orcid.org/0000-0003-3895-2827
Reinie Cordier, https://orcid.org/0000-0002-9906-5300