- 1Department of Psychiatry, The Fifth Affiliated Hospital of Sun Yat-sen University, Zhuhai, Guangdong, China
- 2Department of Nursing, Beijing Tongren Hospital, Capital Medical University, Beijing, China
- 3The National Clinical Research Center for Mental Disorders & Beijing Key Laboratory of Mental Disorders, Beijing Anding Hospital & the Advanced Innovation Center for Human Brain Protection, Capital Medical University, Beijing, China
- 4School of Nursing, Hong Kong Polytechnic University, Kowloon, Hong Kong SAR, China
- 5School of Public Health, Southeast University, Nanjing, China
- 6Unit of Psychiatry, Department of Public Health and Medicinal Administration, & Institute of Translational Medicine, Faculty of Health Sciences, University of Macau, Macao, Macao SAR, China
- 7Centre for Cognitive and Brain Sciences, University of Macau, Macao, Macao SAR, China
- 8Department of Psychiatry, The Melbourne Clinic and St Vincent’s Hospital, University of Melbourne, Richmond, VIC, Australia
Background: Nurses in Ophthalmology Department (OD) had a high risk of infection during the novel coronavirus disease 2019 (COVID-19) pandemic. This study examined the prevalence, correlates, and network structure of depression, and explored its association with quality of life (QOL) in Chinese OD nurses.
Methods: Based on a cross-sectional survey, demographic and clinical data were collected. Depression was measured with the 9-item Self-reported Patient Health Questionnaire (PHQ-9), and QOL was measured using the World Health Organization Quality of Life Questionnaire-brief version (WHOQOL-BREF). Univariate analyses, multivariate logistic regression analyses, and network analyses were performed.
Results: Altogether, 2,155 OD nurses were included. The overall prevalence of depression among OD nurses was 32.71% (95%CI: 30.73–34.70%). Multiple logistic regression analysis revealed that having family or friends or colleagues who were infected (OR = 1.760, p = 0.003) was significantly associated with higher risk of depression. After controlling for covariates, nurses with depression reported lower QOL (F(1, 2,155) = 596.784, p < 0.001) than those without depression. Network analyses revealed that ‘Sad Mood’, ‘Energy Loss’ and ‘Worthlessness’ were the key central symptoms.
Conclusion: Depression was common among OD nurses during the COVID-19 pandemic. Considering the negative impact of depression on QOL and daily life, regular screening for depression, timely counselling service, and psychiatric treatment should be provided for OD nurses, especially those who had infected family/friends or colleagues. Central symptoms identified in network analysis should be targeted in the treatment of depression.
1. Introduction
Since the novel coronavirus disease 2019 (COVID-19) emerged in December 2019, over 676 million people were infected globally and about 6.9 million people had died from COVID-19 as of March 10, 2023 (The Johns Hopkins Coronavirus Resource Center, 2023). The high rate of infection has caused immense workload and challenges on healthcare systems worldwide. Frontline health caregivers have played a critical and direct role in caring for infected patients and their families, which put them at a higher risk of infection compared to most of other populations (Chidiebere Okechukwu et al., 2020; Huang et al., 2020; Shah et al., 2021). Among the frontline workers, nurses, who are primarily responsible for infected patients, face a higher risk of infection than other healthcare professionals due to their frequent and prolonged physical contact with patients (Fawaz et al., 2020). In particular, nurses working in Emergency Departments (ED) and fever clinics face the highest risk of infection as they are managing COVID-19 patients who first present for care (Lu et al., 2015; An et al., 2020). However, preliminary evidence indicated that eyecare providers could also be exposed to substantial COVID-19 infection risk (Grossman et al., 2020; Seah et al., 2020). In fact, OD nurses faced high risk of infection in daily clinical practice due to transmission of COVID-19 through ocular fluids from slit-lamp examination and ophthalmoscopy (Williams et al., 2020), and respiratory droplets due to close physical contact (Sadhu et al., 2020). A study found that around 80% of ophthalmology health workers perceived they had a high risk of COVID-19 transmission because of the close proximity to patients and lack of Personal Protective Equipment (Xie et al., 2022) such as protective goggles (Minocha et al., 2020). Some studies found that conjunctivitis could be the first presentation of COVID-19 before the onset of usual symptoms like cough, fever, and fatigue (Seah and Agrawal, 2020; Daruich et al., 2020a, b); therefore, COVID-19 patients with only ocular symptoms might be presenting first to OD staff who were not aware of the transmission risk. A tragic example is Li Wenliang, an well-known OD health worker in Wuhan, who died from COVID-19 in January 2020 after coming into contact with a patient presenting with glaucoma as the primary complaint rather than typical COVID-19 symptoms (Parrish 2nd et al., 2020). Due to high work pressure caused by the COVID-19 pandemic, mental health problems, particularly depression, among frontline healthcare workers have become a major concern (Lee et al., 2022; Mercader Rubio et al., 2022). Several studies on depression among OD doctors during the pandemic have found a wide prevalence ranging from 32.6 to 65% (Almater et al., 2020; Khanna et al., 2020; Durmaz Engin et al., 2021; Grover et al., 2021). However, depression among OD nurses has not been adequately studied despite their higher infection risk due to prolonged close contact with patients (Chidiebere Okechukwu et al., 2020; Shah et al., 2021).
After the peak of the first COVID-19 wave in China in February of 2020, subsequent waves have occurred due to the rapid mutation of the virus and the waning of antibodies resulting from previous infections or vaccinations (Singh and Yi, 2021). This has resulted in significant impact on the work productivity, well-being, cognition, and mental health of healthcare workers. To develop effective public health interventions and strategies, it is important to examine COVID-19 related mental health problems among the vulnerable populations. Understanding the pattern of depression and its related factors among different healthcare workers is crucial for developing appropriate preventive strategies, treatment modalities, and management protocols.
Quality of Life (QOL) refers to the individual’s perception of their position in life in the context of their culture and value systems, and in relation to their goals, expectations, standards, and concerns, all of which could have an impact on their well-being. QOL is also associated with various factors such as economic status, personal relationships, and mental health (World Health Organization, 2023). Research has shown that the QOL of health professionals could affect the quality of medical services and even the prevention of infection outbreaks (Kandula and Wake, 2021). Therefore, addressing the QOL of healthcare professionals is highly important.
In the past decade, network analysis has been widely used to examine the complex associations among individual symptoms of a disorder (Beard et al., 2016), which was based on partial correlations between symptoms (Epskamp et al., 2018b). Network analysis identifies highly central symptoms (defined as nodes) and symptom-symptom interactions (defined as edges), and generates network models in which central nodes are located at the center, while nodes with fewer connections are at the periphery (Mullarkey et al., 2019b). Identifying central nodes and the relevant influential edges using network analysis may be helpful to develop effective treatment and improve health outcomes (Hofmann et al., 2016). Previous studies have examined depression networks among various populations, including adolescents, older adults, and the general population (Zhao et al., 2021; Eli et al., 2022; Xie et al., 2022). For instance, among Chinese adolescents, “fatigue,” “depressed mood,” and “self-blame” were identified as the most central symptoms (Xie et al., 2022). Among the Wuhan residents during the COVID-19 pandemic, “fatigue,” “sad mood,” “guilt,” and “motor disturbances” were the most central symptoms (Zhao et al., 2021). As most studies on depression among ophthalmologists were based on total scale scores, it was previously not possible to examine the syndrome of depression at the symptom level.
This study thus examined the epidemiological patterns and correlates of depression among OD nurses during COVID-19 pandemic in China, constructed the network structure and identified the central symptoms of depression, and explored the relationship between depression and QOL. Based on previous studies and reports, we hypothesized that depression among OD nurses would be common, and certain factors would be significantly associated with depression (Almater et al., 2020; Durmaz Engin et al., 2021; Grover et al., 2021). We also hypothesized that depression would be negatively associated with overall QOL.
2. Methods
2.1. Setting and study sample
This nationwide survey in China was jointly conducted by the Chinese Nursing Association Psychiatry Branch, and the Chinese Nursing Society Ophthalmology Branch from March 15 to March 20, 2020 (i.e., after the peak of the first COVID-19 wave), using a snowball sampling method. At the time the survey, 81,554 people in China were infected and 3,312 had died (Chinese Health Commission, 2020).
Due to the risk of COVID-19 infection, face-to-face assessment were not adopted for safety reasons. Similar to other studies (Zou et al., 2020; Yuan et al., 2021; Liu et al., 2022), the WeChat-based QuestionnaireStar program, a commonly used application for epidemiological surveys (Li et al., 2016; Liu et al., 2022), was used for data collection. A WeChat-based Quick Response code (QR code) linked to the study invitation and questionnaire was distributed by the Chinese Nursing Society Ophthalmology Branch to all OD nurses working in public hospitals in China. Those OD nurses who agreed then participated in this study on an anonymous and voluntary basis. To be eligible, participants met the following inclusion criteria: (1) adults aged 18 years or above; (2) OD nurses working in Chinese public hospitals during the COVID-19 pandemic; (3) able to understand Chinese and provide electronic written informed consent. The protocol of this study was approved by the ethics committee of Beijing Anding Hospital, China.
2.2. Instruments
Basic demographic and clinical data, such as age, gender, marital status, educational level, working experience, duty shift, living circumstances, rank (junior/senior), hospital setting (primary/tertiary), working units (inpatient/outpatient department), current smoking status, and work experience during the 2003 SARS outbreak, were collected. Three additional standard questions were asked: (1) whether they were directly engaged in clinical services for COVID-19 patients; (2) whether they had families, friends or colleagues infected with COVID-19; and (3) whether the local COVID-19 confirmed cases were more than 500 in the province they lived in.
Due to the risk of COVID-19 infection, interviewer-rated measures on depression in a face-to-face interview were not used. The severity of depression was measured with the validated self-report 9-item Patient Health Questionnaire (PHQ-9)-Chinese version, which was one of the most widely used measure on depression in different populations during the pandemic (Liu et al., 2021; Stocker et al., 2021; Shevlin et al., 2022). For example, a meta-analysis showed that over half of studies on depression during the pandemic used the PHQ-9 (Liu et al., 2021). The PHQ-9 was chosen instead of other standardized measures because of its brevity and wide application across different Chinese populations (Wang et al., 2014; Li et al., 2021; Kong et al., 2022). PHQ-9 is also a rapid screening tool for depression in large-scale population surveys (Levis et al., 2019; Sun et al., 2020).
Each item of PHQ-9 is scored from 0 to 3, with the total score of 5 being the cut-off value for “having depression” (Kroenke et al., 2010). The nine PHQ items measure nine cluster of depressive symptoms in accordance with the diagnosis criteria of major depressive disorder in the Diagnostic and Statistical Manual of Mental Disorders (DSM-IV), including ‘Anhedonia’, ‘Sad Mood’, ‘Sleep Disturbance’, ‘Energy Loss’, ‘Appetite Change’, ‘Worthlessness’, ‘Concentration Difficulty’, ‘Psychomotor Issues’, and ‘Suicidal Ideation’(American Psychiatric Association, 2013; Wang et al., 2014; Levis et al., 2019; Sun et al., 2020). Specifically, the PHQ-9 total score of 5–9, 10–14, 15–19, ≥20 indicate ‘mild depression’, ‘moderate depression’, ‘moderate-to-severe depression’, and ‘severe depression’, respectively (Kroenke et al., 2010). Psychometric properties of the Chinese version of PHQ-9 were found to be satisfactory (Cronbach’s alpha = 0.89)(Chen et al., 2015). Global QOL was measured by the sum of the first two items on the global QOL derived from the World Health Organization Quality of Life Questionnaire-brief version (WHOQOL-BREF)-Chinese version (Harper and Power, 1998; Fang and Hao, 1999). Higher score indicates higher QOL (Skevington and Tucker, 1999).
2.3. Data analysis
Data were analysed using the IBM Statistical Package for Social Science (SPSS) software version 23.0. P–P plots were performed to test the normal distribution of continuous variables. We divided participants into two groups according to PHQ-9 total score, depression group (PHQ-9 ≥ 5) and non-depression group (PHQ-9 < 5). To compare the demographic and clinical variables between the two groups, Chi-square test and two samples independent sample t-test were used where appropriate. Multiple logistic regression analysis with the “Enter” method was conducted to examine the independent demographic and clinical correlates of depression, with depression as the dependent variable, and variables with p values of <0.05 in the univariate analyses as independent variables. To compare QOL between the two groups, analysis of covariance (ANCOVA) was conducted after controlling for all the potential confounders. Significant level was set as p value less than 0.05 (two-tailed).
2.4. Network estimation
Following previous studies (Mullarkey et al., 2019; Peng et al., 2023; Wang et al., 2023), we performed network analysis in the depression group (N = 705) to explore the central symptoms and key edges of depression among Chinese OD nurses. Network analyses were conducted using bootnet (Epskamp et al., 2018a) and qgraph (Epskamp et al., 2012) packages in R program (version 4.1.2). In the network analysis, each individual depressive symptom was defined as ‘node’ and relationships between symptoms were defined as ‘edges’ (Beard et al., 2016; Wang et al., 2020). In this study, the PHQ-9 depressive symptoms were included for network analyses. For network visualization, the thickness of edges represented the strength of associations between two nodes. The colours of the edge indicated the direction of the correlations (i.e., green represented positive correlations, while red represented negative correlations).
Following previous studies (Beard et al., 2016; Wang et al., 2020), the ‘estimateNetwork’ function in bootnet package (Epskamp et al., 2018a) was used to estimate the network structure, with ‘EBICglasso’ as default method and 0.5 as default tuning parameter. The network models were estimated using a sparse Graphical Gaussian Model (GGM) combined with graphical least absolute shrinkage and selection operator (LASSO) method (Friedman et al., 2008); model selection was based on the Extended Bayesian Information Criterion (EBIC) (Chen and Chen, 2008 Epskamp, 2016). The GGM method was applied to construct network models. Considering the large number of spurious edges produced due to latent variables (Epskamp et al., 2018b; Montazeri et al., 2020), to reduce the number of spurious edges and improve the interpretability of networks, the network models were regularized using the LASSO, which is a well-established method for regularization (Epskamp et al., 2018a). This algorithm could reduce small associations to zero by removing them from the model as potentially “false positive” edges (Friedman et al., 2008; Heeren et al., 2018; Epskamp et al., 2018a).
To assess the importance of each node in the network, centrality index ‘Strength’ was calculated (Freeman, 1978; Borgatti, 2005; Opsahl et al., 2010; Pan and Liu, 2021), using the ‘centralityPlot’ function in qgraph package (Epskamp et al., 2012). ‘Strength’ is the sum of absolute edge weights of all direct connections between a specific node and all other nodes, reflecting the importance of an individual symptom (Dalege et al., 2017).
2.5. Estimation of network accuracy, stability, and comparisons of edge and node strengths
Similar to previous studies (Beard et al., 2016; Belvederi Murri et al., 2020; Wang et al., 2020), the accuracy and stability of network were tested using R package bootnet (Epskamp et al., 2018a). First, to estimate the accuracy of edge weights, non-parametric bootstrapping (1,000 replicates, 8 cores) was performed to compute 95% confidence intervals (Dobson et al., 2021) of edge values. Second, bootstrapped difference test was used to determine significant differences between edges weight and node strengths. Finally, to determine the stability of centrality indices, case-dropping subset bootstrap (1,000 replicates, 8 cores) was performed to compute correlation stability coefficient (CS). A series of correlation values were calculated between the original centrality indices based on original sample, and subset centrality based on different subset of sample (e.g., 95% of the sample, 80, 70%, …, 25%) (Hevey, 2018; Epskamp et al., 2018a). The CS represents the maximum proportion of cases that could be drooped from the original sample, while the correlation coefficients between centrality indices based on the original networks, and centrality indices based on case-subset network could still reach at least 0.7 (default) (Epskamp et al., 2018a). Generally, the CS should not be lower than 0.25, and is preferably above 0.5 as recommended previously (Epskamp et al., 2018a).
3. Results
3.1. Demographic information
In total, 2,155 OD nurses met the inclusion criteria and completed the survey. The mean age of this sample was 34.32 ± 8.21 years, and 99% were female. The mean duration of work experience was 12.94 ± 9.07 years, and 57.5% were junior nurses. In addition, 10.4% of participants had work experience during SARS in 2003. Overall, 74.5% had worked in the inpatient department, 5.7% had infected family/friends/colleagues, and 6.5% had experience caring for infected patients. Table 1 shows the basic social-demographic characteristics of the participants.
3.2. Prevalence, correlates of depression and descriptive information of symptoms
In the whole sample, the mean (SD) of the PHQ-9 total score was 3.60 (SD: 4.29). The prevalence of depression was 32.71% (95%CI: 30.73–34.70%; PHQ-9 total score of ≥5). Specifically, the prevalence rates of ‘mild depression’, ‘moderate depression’, ‘moderate-to-severe depression’, and ‘severe depression’ were 23.02% (95%CI: 21.27–24.87%), 6.73% (95%CI: 5.73–7.89%), 2.55% (95%CI: 1.94–3.33%) and 0.42% (95%CI, 0.21–0.83%), respectively.
The results of P–P plot showed that continuous variables (e.g., age, work experience, and total QOL scores) followed a normal distribution. Univariate analyses revealed that depression was significantly associated with college education and above (p = 0.096), having family/friends/colleagues who were infected (p = 0.001), and caring for infected patients (p = 0.027). Multiple logistic regression analysis revealed that having infected family/friends/colleagues (OR = 1.760, p = 0.003) was significantly associated with higher risk of depression (Table 2). Table 3 shows the mean, SD, minimum, maximum and frequency (Epskamp) of all the depressive symptoms as measured by PHQ-9 in the depression group. After controlling for variables with significant group differences in univariate analyses, ANCOVA revealed that nurses with depression reported lower QOL than non-depression group (F (1, 2,155) = 596.784, p < 0.001).
3.3. Network structures, accuracy, and stability
Following previous studies (Marchetti, 2019; Mullarkey et al., 2019), after checking for item informativeness (i.e., SD of the item) and item redundancy, we found that no item was poorly informative (i.e., 2.5 SD below the mean level of informativeness (Mullarkey et al., 2019), MSD = 0.635 ± 0.141) or redundant with any other item (i.e., <25% of statistically different correlations). Therefore, all the PHQ-9 items were included in the analyses. The network of depressive symptoms in the depression group is shown in Figure 1. Figure 2 illustrates the centrality measure (i.e., Strength); the symptom ‘Sad Mood’ had the highest strength, followed by ‘Energy Loss’ and ‘Worthlessness’.
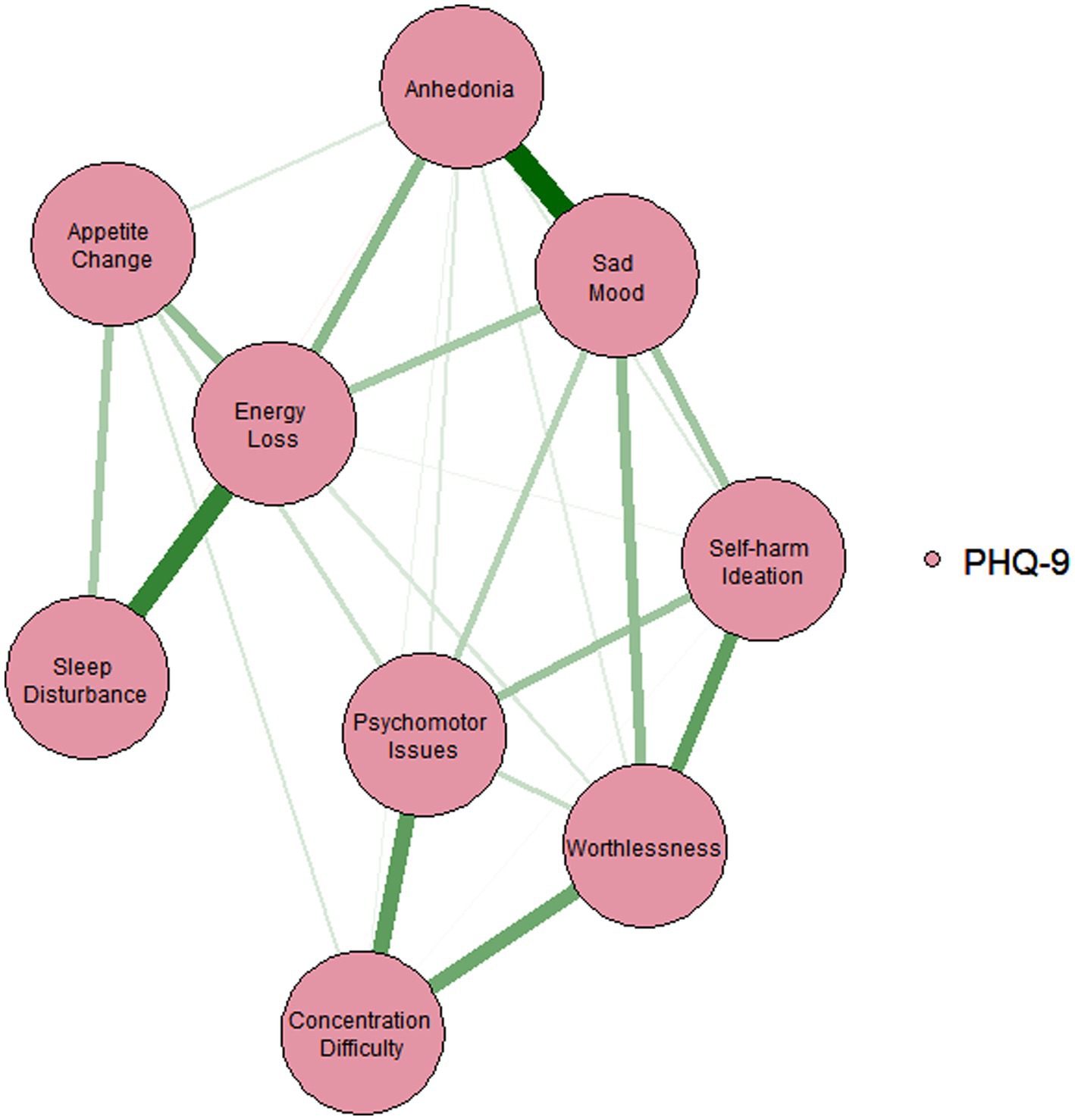
Figure 1. Estimated network model for depressive symptoms in the depression sample. The network models were estimated using the ‘EBICglasso’ model.
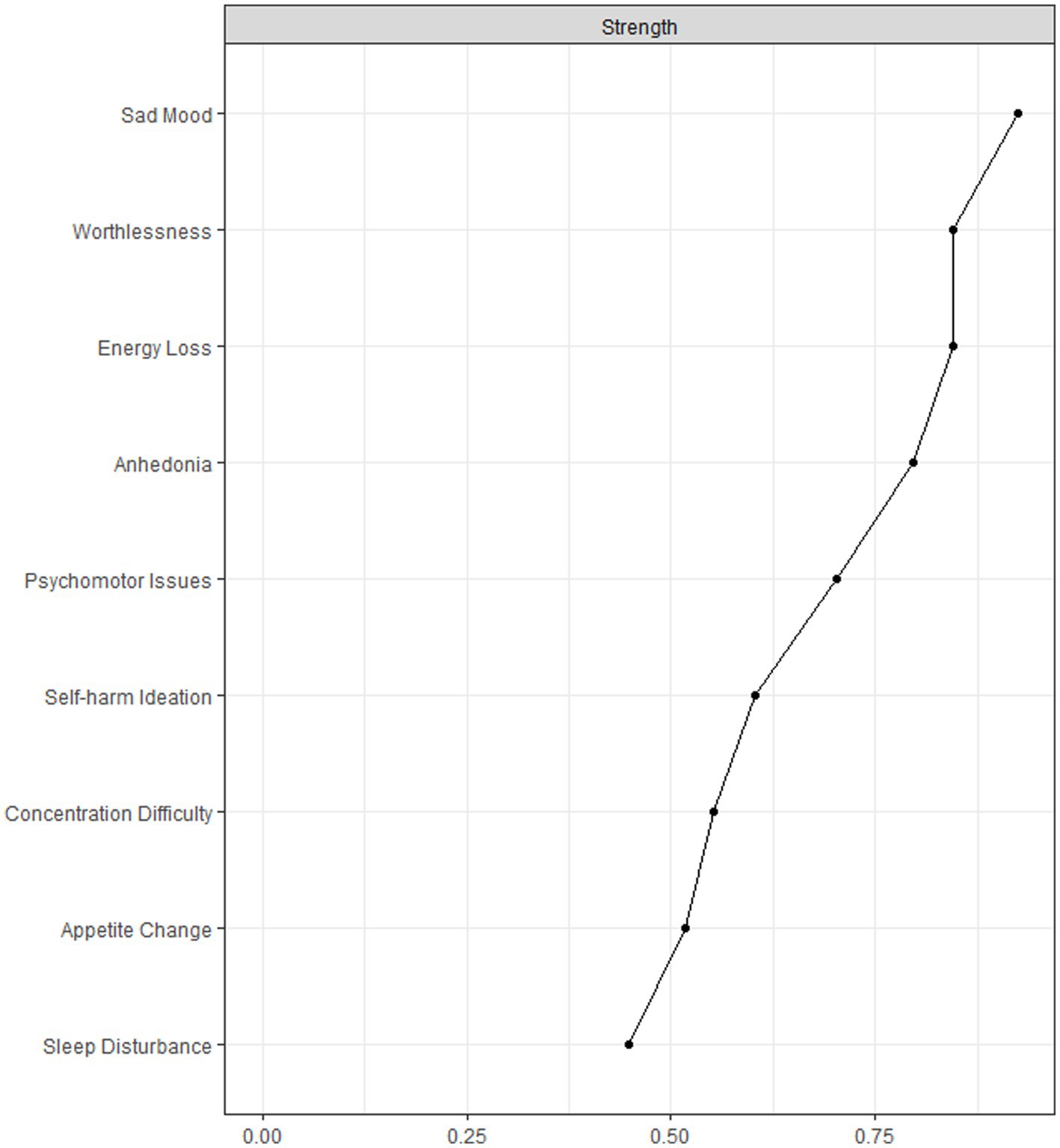
Figure 2. Centrality measures of all symptoms within the network in the depression sample. The figure shows centrality measure (i.e., strength) of all factors within the network (z-scores).
Figure 3 shows the case-dropping subset bootstrap procedure, indicating that the value of strength remained stable even after dropping large proportions of the sample. The CS coefficients for ‘strength’ was 0.675, indicating that 67.5% of the sample could be excluded with a higher correlation (r = 0.7) between the original whole sample and subset of sample, which suggested that the original results were robust and trustworthy. The Bootstrap 95%CI for edges within the network were narrow and the edge weights were consistent with the bootstrapped sample, suggesting that the precision of the edges was acceptable, with smaller CIs indicating more accurate estimation of the edges (Supplementary Figure S1). The bootstrapped difference test revealed that most of edge weights and node strengths were statistically significant from one another in the individual comparisons (Supplementary Figures S2, S3). For edge comparison, ‘Anhedonia-Sad Mood’, and ‘Sleep Disturbance-Energy Loss’ were the strongest edges that were statistically stronger than most of the other edges (Supplementary Figure S3).
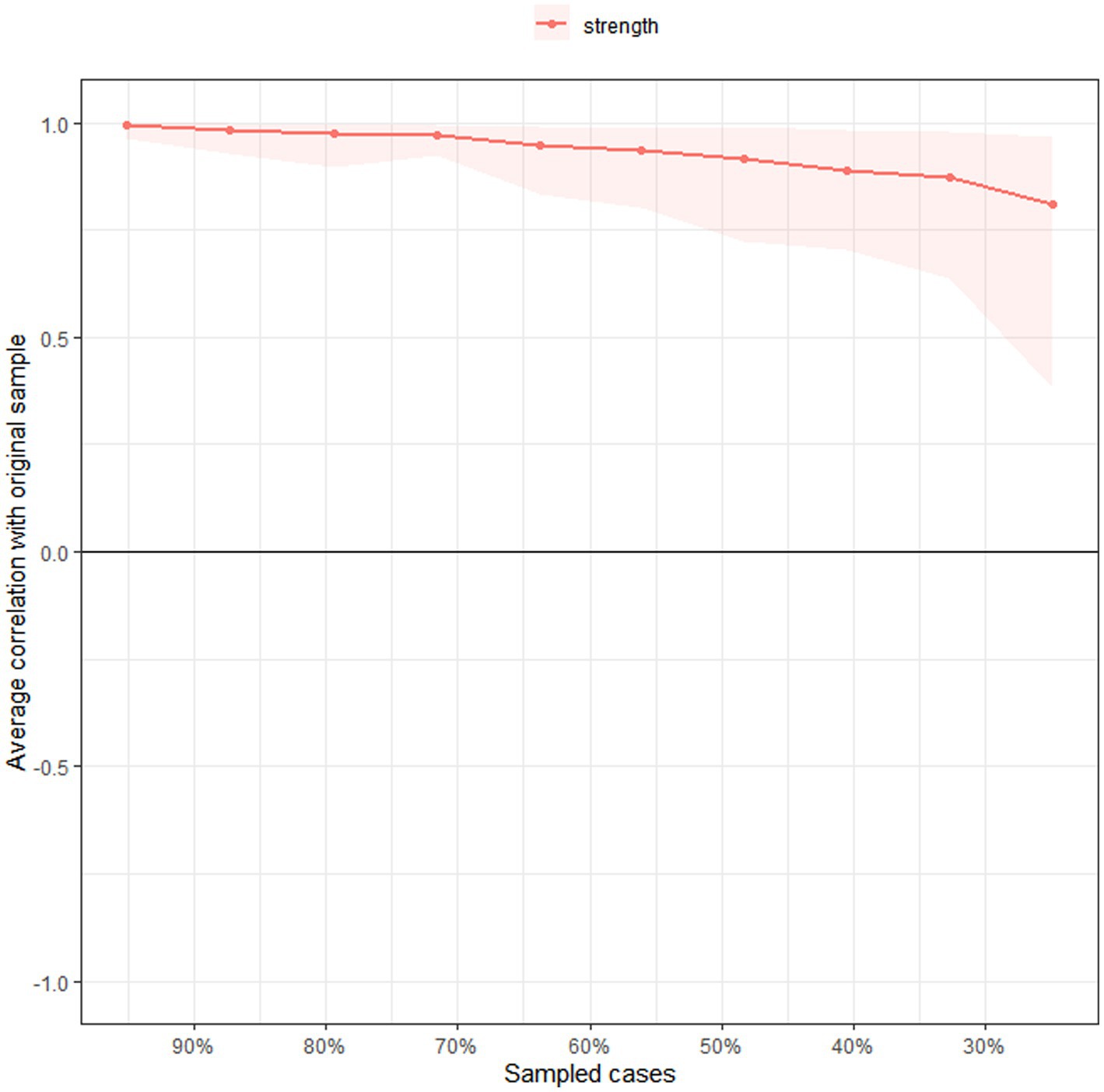
Figure 3. Stability of centrality indices by case dropping subset bootstrap. The x-axis represents the percentage of cases of the original sample used at each step. The y-axis represents the average of correlations between the centrality indices from the original network and the centrality indices from the networks that were re-estimated after excluding increasing percentages of cases.
4. Discussion
This was the first study that investigated the prevalence of depression, associated factors and impact on QOL among OD nurses in public hospitals in China during the peak of the first COVID-19 pandemic wave as well as the first network analysis of depressive symptoms in this population. In this study, we examined the prevalence, correlates, and network structure of depression among OD nurses and also performed a network analysis of depressive symptoms in this population. The findings provided valuable insights into the mental health and well-being of this overlooked group of healthcare professionals during the pandemic. This study focused specifically on OD nurses, who faced substantial risks of infection due to transmission through ocular fluids and respiratory droplets (Seah et al., 2020; Williams et al., 2020; Taha et al., 2022). The presence of COVID-19 patients with ocular symptoms who might not exhibit typical COVID-19 symptoms emphasizes the need for greater awareness and preparedness among ophthalmology healthcare professionals.
This study found that the overall depression prevalence among OD nurses was 32.71% (95% CI: 30.73–34.70%), which is comparable with a Chinese study (31.37%) using the same assessment tool among frontline health workers in China during COVID-19 pandemic (Zheng et al., 2020), and another study among ophthalmologists in India (32.6%) (Khanna et al., 2020). The high risk of infection from the close physical contact to conduct ophthalmoscopy in daily clinical practice could contribute to the high depression rate in our study (Williams et al., 2020; Durmaz Engin et al., 2021). Our findings suggest that even though OD was not a clinical unit with excessive workload burden like ED and fever clinics during the COVID-19 pandemic, OD nurses appeared to have an elevated risk of developing depression. Hence, mental health support should be implemented for OD nurses to address their risk of developing depression.
Although previous studies in frontline healthcare workers found that being directly involved in the care of COVID-19 patients was associated with a higher risk of depressive symptoms (Lai et al., 2020; Hruska et al., 2023), this study did not find any significant association between having depression and caring for infected patients after controlling for covariates. This could probably be due to use of different statistical methods (e.g., univariate vs. multivariate analyses) and different study stages of the COVID-19 pandemic (e.g., very early stage vs. middle stage). Instead, we found that having infected family/friends/colleagues was significantly associated with a higher risk of depression, which could be attributed to the uncertain prognosis of COVID-19 during the early/middle pandemic stages and the fear of being infected by family/friends/colleagues.
Our study also examined the QOL of OD nurses and its association with depression. The QOL of healthcare professionals is important due to its impact on the quality of medical services and infection prevention efforts (Kandula and Wake, 2021). Moreover, depression could affect sleep quality, social function and energy levels which could lead to a low quality of life (Malhi and Mann, 2018). As expected, we found that OD nurses with depression reported lower QOL than those without depression, which is similar to the previous findings (Malhi and Mann, 2018; Shao and Zhang, 2020; Sjöberg et al., 2020).
The network analysis of depressive symptoms provided valuable insights into the interconnected nature of these symptoms among OD nurses. Identifying the central symptoms and their connections could guide the development of targeted interventions. The network analysis revealed that “Sad Mood” was the most central symptom while “Worthlessness” and “Energy loss” were also other central symptoms. These findings are consistent with the notion that depression is characterized by low mood, lack of energy, and sadness, as well as one’s inability to enjoy life (Cui, 2015). Compared to other symptoms in the model, central symptoms are more important targets in treatments (Mullarkey et al., 2019). The network analysis also revealed that ‘Anhedonia-Sad Mood’, and ‘Sleep Disturbance-Energy Loss’ were the strongest edges, which might indicate the potentially strong dynamic relationships between ‘Anhedonia’ and ‘Sad Mood’, and between ‘Sleep Disturbance’ and ‘Energy Loss’. Therefore, improving mood and energy levels could be possible by targeting the symptoms of anhedonia and sleep disturbances in clinical management. For example, optimizing the irregular working schedules might improve the sleep quality among nurses and reduce the symptom of ‘Energy Loss’. This study findings support the implementation of guidelines and strategies such as appropriate patient triage, sufficient PPE supply, and professional infection control training to protect OD nurses from the high infection risk during COVID-19, and decrease their anxiety and sense of vulnerability (Borrelli et al., 2020; Du et al., 2020; Romano et al., 2020; Yu et al., 2020). Another important implication of our findings is the need for accessible and effective mental health services for OD nurses. These interventions could include psychological counselling, stress management programs, and peer support groups that targets the unique difficulties and challenges faced by OD nurses during the pandemic. Furthermore, promoting a supportive work environment that encourages open discussions about mental health, reduces the stigma of mental disorders, and provides resources for help seeking (Jackson, 2021).
The strengths of this study included the relatively large sample size, use of standard instrument on depression and QOL, and the sophisticated network analysis that could analysis the inter-relationships between different depressive symptoms. However, several limitations should be acknowledged. First, the cross-sectional design of the study limits our ability to establish causal relationships between variables. Longitudinal studies are needed to understand the dynamic nature of depression and its impact on the QOL of OD nurses over time. Second, our study focused on OD nurses in China only, and thus may not be generalizable to nurses in other healthcare settings or different countries. Future research should include a more diverse sample to enhance the external validity of the findings. Third, most of the study sample were females, which reflected the actual gender distribution among healthcare workers in China (Han et al., 2021 National Health Commission of China, 2022). Fourth, for logistical and safety reasons, self-report measures were used to assess depression and QOL, which were subject to individual biases and might not fully capture the complexity of these constructs. The inclusion of interviewer-administered assessments and objective measures would strengthen the validity of the results in future studies. Additionally, as only global QOL was measured in this study, there was a lack of data on individual QOL domains. Hence, the network analysis of depressive symptoms and QOL domains was not performed. Fifth, as this was a nationwide online survey, hospital and provincial distribution of the study sample was not recorded. Finally, some variables related to depression were not examined, such as social support and pre-existing psychiatric illnesses.
In conclusion, this study found a high prevalence of depression among OD nurses during the first wave of the COVID-19 pandemic in China. The findings highlighted the need for targeted interventions, support programs, and a supportive work environment to address the mental health needs of this vulnerable group of healthcare professionals. Considering the negative impact of depression on QOL and daily life, regular screening for depression, timely counselling service, and psychiatric treatment should be provided for OD nurses, especially those who had infected family/friends or colleagues. Moreover, interventions for depression, such as cognitive behavioral therapy, should target the central symptoms (e.g., ‘Sad Mood’, ‘Worthlessness’, and ‘Energy Loss’) identified in the network analysis. These findings might have implications for the mental health support and well-being of healthcare professionals globally during the pandemic.
Data availability statement
The datasets presented in this article are not readily available because the ethics committee of Beijing Anding Hospital that approved the study prohibits the authors from disseminating the research dataset of clinical studies publicly. Requests to access the datasets should be directed to eHl1dGx5QGdtYWlsLmNvbQ==.
Ethics statement
The studies involving human participants were reviewed and approved by the ethics committee of Beijing Anding Hospital, China. The patients/participants provided their written informed consent to participate in this study.
Author contributions
F-RA and Y-TX: conception and design. YL, Z-RT, and Y-TX: administrative support. YL, Z-RT, F-RA, and Y-TX: provision of study materials or patients. YL, Z-RT, Y-JZ, PC, and F-RA: collection and assembly of data. Z-HL, TC, ZS, and F-RA: data analysis and interpretation. Z-HL, CN, and Y-TX: manuscript writing and revision. Z-HL, YL, Z-RT, Y-JZ, TC, ZS, PC, CN, F-RA, and Y-TX: final approval of manuscript.
Funding
The study was supported by the National Science and Technology Major Project for investigational new drug (2018ZX09201-014), the Beijing Hospitals Authority Clinical Medicine Development of special funding support (XMLX202128), and the University of Macau (MYRG2019-00066-FHS; MYRG2022-00187-FHS).
Conflict of interest
The authors declare that the research was conducted in the absence of any commercial or financial relationships that could be construed as a potential conflict of interest.
Publisher’s note
All claims expressed in this article are solely those of the authors and do not necessarily represent those of their affiliated organizations, or those of the publisher, the editors and the reviewers. Any product that may be evaluated in this article, or claim that may be made by its manufacturer, is not guaranteed or endorsed by the publisher.
Supplementary material
The Supplementary material for this article can be found online at: https://www.frontiersin.org/articles/10.3389/fpsyg.2023.1218747/full#supplementary-material
References
Almater, A. I., Tobaigy, M. F., Younis, A. S., Alaqeel, M. K., and Abouammoh, M. A. (2020). Effect of 2019 coronavirus pandemic on ophthalmologists practicing in Saudi Arabia: a psychological health assessment. Middle East Afr. J. Ophthalmol. 27, 79–85. doi: 10.4103/meajo.MEAJO_220_20
American Psychiatric Association, (2013). Diagnostic and statistical manual of mental disorders (fifth). Arlington, VA: American Psychiatric Association.
An, Y., Yang, Y., Wang, A., Li, Y., Zhang, Q., Cheung, T., et al. (2020). Prevalence of depression and its impact on quality of life among frontline nurses in emergency departments during the COVID-19 outbreak. J. Affect. Disord. 276, 312–315. doi: 10.1016/j.jad.2020.06.047
Beard, C., Millner, A. J., Forgeard, M. J., Fried, E. I., Hsu, K. J., Treadway, M. T., et al. (2016). Network analysis of depression and anxiety symptom relationships in a psychiatric sample. Psychol. Med. 46, 3359–3369. doi: 10.1017/S0033291716002300
Belvederi Murri, M., Amore, M., Respino, M., and Alexopoulos, G. S. (2020). The symptom network structure of depressive symptoms in late-life: results from a European population study. Mol. Psychiatry 25, 1447–1456. doi: 10.1038/s41380-018-0232-0
Borgatti, S. P. (2005). Centrality and network flow. Soc. Networks 27, 55–71. doi: 10.1016/j.socnet.2004.11.008
Borrelli, E., Sacconi, R., Querques, L., Zucchiatti, I., Prascina, F., Bandello, F., et al. (2020). Taking the right measures to control COVID-19 in ophthalmology: the experience of a tertiary eye care referral center in Italy. Eye 34, 1175–1176. doi: 10.1038/s41433-020-0880-6
Chen, J., and Chen, Z. (2008). Extended Bayesian information criteria for model selection with large model spaces. Biometrika 95, 759–771. doi: 10.1093/biomet/asn034
Chen, M., Sheng, L., and Qu, S. (2015). Diagnostic test of screening depressive disorder in general hospital with the patient health questionnaire (in Chinese). Chin. Ment. Health 29, 241–245.
Chidiebere Okechukwu, E., Tibaldi, L., and La Torre, G. (2020). The impact of COVID-19 pandemic on mental health of nurses. Clin. Ter. 171, e399–e400. doi: 10.7417/CT.2020.2247
China’s State Council of the People’s Republic of China. (2020). The latest situation of the COVID-19 epidemic in China as of 24:00 on march 31 (in Chinese). Available at: https://www.gov.cn/xinwen/2020-04/01/content_5497871.htm (Accessed March 15, 2023).
Cui, R. (2015). Editorial: a systematic review of depression. Curr. Neuropharmacol. 13:480. doi: 10.2174/1570159X1304150831123535
Dalege, J., Borsboom, D., van Harreveld, F., and van der Maas, H. L. J. (2017). Network analysis on attitudes. Soc. Psychol. Personal. Sci. 8, 528–537. doi: 10.1177/1948550617709827
Daruich, A., Martin, D., and Bremond-Gignac, D. (2020a). Ocular manifestation as first sign of coronavirus disease 2019 (COVID-19): interest of telemedicine during the pandemic context. J. Fr. Ophtalmol. 43, 389–391. doi: 10.1016/j.jfo.2020.04.002
Daruich, A., Martin, D., and Bremond-Gignac, D. (2020b). Unilateral conjunctivitis as first presentation of coronavirus disease 2019 (COVID-19): a telemedicine diagnosis. J. Fr. Ophtalmol. 43, 389–391. doi: 10.1016/j.jfo.2020.04.002
Dobson, E. T., Croarkin, P. E., Schroeder, H. K., Varney, S. T., Mossman, S. A., Cecil, K., et al. (2021). Bridging anxiety and depression: a network approach in anxious adolescents. J. Affect. Disord. 280, 305–314. doi: 10.1016/j.jad.2020.11.027
Du, H., Zhang, M., Zhang, H., and Sun, X. (2020). Practical experience on emergency ophthalmic surgery during the prevalence of COVID-19. Graefes Arch. Clin. Exp. Ophthalmol. 258, 1831–1833. doi: 10.1007/s00417-020-04692-x
Durmaz Engin, C., Senel Kara, B., Ozturk, T., and Dadas, O. F. (2021). The impact of COVID-19 pandemic on practice patterns and psychological status of ophthalmologists in Turkey. Cureus 13:e16614. doi: 10.7759/cureus.16614
Eli, B., Zhou, Y., Chen, Y., Huang, X., and Liu, Z. (2022). Symptom structure of depression in older adults on the Qinghai–Tibet plateau: a network analysis. Int. J. Environ. Res. Public Health 19:13810. doi: 10.3390/ijerph192113810
Epskamp, S. (2016). Regularized Gaussian psychological networks: brief report on the performance of extended BIC model selection. doi: 10.48550/arXiv.1606.05771
Epskamp, S., Borsboom, D., and Fried, E. I. (2018a). Estimating psychological networks and their accuracy: a tutorial paper. Behav. Res. Methods 50, 195–212. doi: 10.3758/s13428-017-0862-1
Epskamp, S., Cramer, A., Waldorp, L., Schmittmann, V., and Borsboom, D. (2012). Qgraph: network visualizations of relationships in psychometric data. J. Stat. Softw. 48, 1–8. doi: 10.18637/jss.v048.i04
Epskamp, S., Waldorp, L. J., Mõttus, R., and Borsboom, D. (2018b). The Gaussian graphical model in cross-sectional and time-series data. Multivariate Behav. Res. 53, 453–480. doi: 10.1080/00273171.2018.1454823
Fang, J. Q., and Hao, Y. A. (1999). Reliability and validity for Chinese version of WHO quality of life scale (in Chinese). Chin. Ment. Health J. 13, 203–209.
Fawaz, M., Anshasi, H., and Samaha, A. (2020). Nurses at the front line of COVID-19: roles, responsibilities, risks, and rights. Am. J. Trop. Med. Hyg. 103, 1341–1342. doi: 10.4269/ajtmh.20-0650
Freeman, L. C. (1978). Centrality in social networks conceptual clarification. Soc. Networks 1, 215–239. doi: 10.1016/0378-8733(78)90021-7
Friedman, J., Hastie, T., and Tibshirani, R. (2008). Sparse inverse covariance estimation with the graphical lasso. Biostatistics 9, 432–441. doi: 10.1093/biostatistics/kxm045
Grossman, S. N., Calix, R., Tow, S., Odel, J. G., Sun, L., Balcer, L. J., et al. (2020). Neuro-ophthalmology in the era of COVID-19: future implications of a public health crisis. Ophthalmology 127, e72–e74. doi: 10.1016/j.ophtha.2020.05.004
Grover, R., Dua, P., Juneja, S., Chauhan, L., Agarwal, P., and Khurana, A. (2021). “depression, anxiety and stress” in a cohort of registered practicing ophthalmic surgeons, post lockdown during COVID-19 pandemic in India. Ophthalmic Epidemiol. 28, 322–329. doi: 10.1080/09286586.2020.1846757
Han, L., Luoya, H., Weijiao, Z., Liqiong, S., Shida, J., Mengqi, W., et al. (2021). Trends, composition and distribution of nurse workforce in China: a secondary analysis of national data from 2003 to 2018. BMJ Open 11:e047348. doi: 10.1136/bmjopen-2020-047348
Harper, A., and Power, M. (1998). Development of the World Health Organization WHOQOL-BREF quality of life assessment. Psychol. Med. 28, 551–558. doi: 10.1017/S0033291798006667
Heeren, A., Jones, P. J., and McNally, R. J. (2018). Mapping network connectivity among symptoms of social anxiety and comorbid depression in people with social anxiety disorder. J. Affect. Disord. 228, 75–82. doi: 10.1016/j.jad.2017.12.003
Hevey, D. (2018). Network analysis: a brief overview and tutorial. Health Psychol. Behav. Med. 6, 301–328. doi: 10.1080/21642850.2018.1521283
Hofmann, S. G., Curtiss, J., and McNally, R. J. (2016). A complex network perspective on clinical science. Perspect. Psychol. Sci. 11, 597–605. doi: 10.1177/1745691616639283
Hruska, B., Patterson, P. D., Doshi, A. A., Guyette, M. K., Wong, A. H., Chang, B. P., et al. (2023). Examining the prevalence and health impairment associated with subthreshold PTSD symptoms (PTSS) among frontline healthcare workers during the COVID-19 pandemic. J. Psychiatr. Res. 158, 202–208. doi: 10.1016/j.jpsychires.2022.12.045
Huang, L., Lei, W., Xu, F., Liu, H., and Yu, L. (2020). Emotional responses and coping strategies in nurses and nursing students during COVID-19 outbreak: a comparative study. PLoS One 15:e0237303. doi: 10.1371/journal.pone.0237303
Jackson, J. (2021). Supporting nurses’ recovery during and following the COVID-19 pandemic. Nurs. Stand. 36, 31–34. doi: 10.7748/ns.2021.e11661
Kandula, U. R., and Wake, A. D. (2021). Assessment of quality of life among health professionals during COVID-19: review. J. Multidiscip. Healthc. 14, 3571–3585. doi: 10.2147/JMDH.S344055
Khanna, R. C., Honavar, S. G., Metla, A. L., Bhattacharya, A., and Maulik, P. K. (2020). Psychological impact of COVID-19 on ophthalmologists-in-training and practising ophthalmologists in India. Indian J. Ophthalmol. 68, 994–998. doi: 10.4103/ijo.IJO_1458_20
Kong, L.-Z., Lai, J.-B., and Hu, S.-H. (2022). China initiates depression screening in children and adolescents. Lancet Psychiatry 9, 107–108. doi: 10.1016/S2215-0366(21)00479-X
Kroenke, K., Spitzer, R. L., Williams, J. B., and Löwe, B. (2010). The patient health questionnaire somatic, anxiety, and depressive symptom scales: a systematic review. Gen. Hosp. Psychiatry 32, 345–359. doi: 10.1016/j.genhosppsych.2010.03.006
Lai, J., Ma, S., Wang, Y., Cai, Z., Hu, J., Wei, N., et al. (2020). Factors associated with mental health outcomes among health care workers exposed to coronavirus disease 2019. JAMA Netw. Open 3:e203976. doi: 10.1001/jamanetworkopen.2020.3976
Lee, Y.-R., Chung, Y.-C., Kim, J. J., Kang, S. H., Lee, B. J., Lee, S.-H., et al. (2022). Effects of COVID-19-related stress and fear on depression in schizophrenia patients and the general population. Schizophrenia 8:15. doi: 10.1038/s41537-022-00213-3
Levis, B., Benedetti, A., and Thombs, B. D. (2019). Accuracy of patient health Questionnaire-9 (PHQ-9) for screening to detect major depression: individual participant data meta-analysis. BMJ 365:l1476. doi: 10.1136/bmj.l1476
Li, F., Wu, J., Mai, X., Ning, K., Chen, K., Chao, L., et al. (2016). Internalized homophobia and depression in homosexuals: the role of self-concept clarity (in Chinese). Chin. J. Clin. Psych. 3, 475–479.
Li, W., Yang, Y., Liu, Z.-H., Zhao, Y.-J., Zhang, L., Cheung, T., et al. (2021). The first national action plan on depression in China: progress and challenges. Lancet Reg. Health West Pac. 7:100078. doi: 10.1016/j.lanwpc.2020.100078
Liu, Z. H., Cai, H., Bai, W., Liu, S., Liu, H., Chen, X., et al. (2022). Gender differences in body appreciation and its associations with psychiatric symptoms among Chinese college students: a Nationwide survey. Front. Psych. 13:771398. doi: 10.3389/fpsyt.2022.771398
Liu, X., Zhu, M., Zhang, R., Zhang, J., Zhang, C., Liu, P., et al. (2021). Public mental health problems during COVID-19 pandemic: a large-scale meta-analysis of the evidence. Transl. Psychiatry 11:384. doi: 10.1038/s41398-021-01501-9
Lu, D. M., Sun, N., Hong, S., Fan, Y. Y., Kong, F. Y., and Li, Q. J. (2015). Occupational stress and coping strategies among emergency department nurses of China. Arch. Psychiatr. Nurs. 29, 208–212. doi: 10.1016/j.apnu.2014.11.006
Malhi, G. S., and Mann, J. J. (2018). Depression. Lancet 392, 2299–2312. doi: 10.1016/S0140-6736(18)31948-2
Marchetti, I. (2019). Hopelessness: a network analysis. Cogn. Ther. Res. 43, 611–619. doi: 10.1007/s10608-018-9981-y
Mercader Rubio, I., Sánchez-López, P., Ángel, N. G., and Ruiz, N. F. O. (2022). Psychological consequences of fear of COVID-19: symptom analysis of triggered anxiety and depression disorders in adolescents and young adults. Int. J. Environ. Res. Public Health 19:14171. doi: 10.3390/ijerph192114171
Minocha, A., Sim, S. Y., Than, J., and Vakros, G. (2020). Survey of ophthalmology practitioners in a&E on current COVID-19 guidance at three major UK eye hospitals. Eye 34, 1243–1245. doi: 10.1038/s41433-020-0857-5
Montazeri, F., de Bildt, A., Dekker, V., and Anderson, G. M. (2020). Network analysis of Behaviors in the depression and autism realms: inter-relationships and clinical implications. J. Autism Dev. Disord. 50, 1580–1595. doi: 10.1007/s10803-019-03914-4
Mullarkey, M. C., Marchetti, I., and Beevers, C. G. (2019). Using network analysis to identify central symptoms of adolescent depression. J. Clin. Child Adolesc. Psychol. 48, 656–668. doi: 10.1080/15374416.2018.1437735
National Health Commission of China, (2022). China health statistics yearbook 2022. Beijing Union Medical College Press, Beijing.
Opsahl, T., Agneessens, F., and Skvoretz, J. (2010). Node centrality in weighted networks: generalizing degree and shortest paths. Soc. Net. 32, 245–251. doi: 10.1016/j.socnet.2010.03.006
Pan, H., and Liu, Q. (2021). Difference of depression between widowed and non-widowed older people in China: a network analysis approach. J. Affect. Disord. 280, 68–76. doi: 10.1016/j.jad.2020.11.058
Parrish, R. K. 2nd, Stewart, M. W., and Duncan Powers, S. L. (2020). Ophthalmologists are more than eye doctors-in memoriam Li Wenliang. Am. J. Ophthalmol. 213, A1–a2. doi: 10.1016/j.ajo.2020.02.014
Peng, P., Wang, Q., Lang, X. E., Liu, T., and Zhang, X. Y. (2023). Association between thyroid dysfunction, metabolic disturbances, and clinical symptoms in first-episode, untreated Chinese patients with major depressive disorder: undirected and Bayesian network analyses. Front. Endocrinol. 14:1138233. doi: 10.3389/fendo.2023.1138233
Romano, M. R., Montericcio, A., Montalbano, C., Raimondi, R., Allegrini, D., Ricciardelli, G., et al. (2020). Facing COVID-19 in ophthalmology department. Curr. Eye Res. 45, 653–658. doi: 10.1080/02713683.2020.1752737
Sadhu, S., Agrawal, R., Pyare, R., Pavesio, C., Zierhut, M., Khatri, A., et al. (2020). COVID-19: limiting the risks for eye care professionals. Ocul. Immunol. Inflamm. 28, 714–720. doi: 10.1080/09273948.2020.1755442
Seah, I., and Agrawal, R. (2020). Can the coronavirus disease 2019 (COVID-19) affect the eyes? A review of coronaviruses and ocular implications in humans and animals. Ocul. Immunol. Inflamm. 28, 391–395. doi: 10.1080/09273948.2020.1738501
Seah, X. S., and Lingam, G. (2020). Revisiting the dangers of the coronavirus in the ophthalmology practice. Eye 34, 1155–1157. doi: 10.1038/s41433-020-0790-7
Shah, M., Roggenkamp, M., Ferrer, L., Burger, V., and Brassil, K. J. (2021). Mental health and COVID-19: the psychological implications of a pandemic for nurses. Clin. J. Oncol. Nurs. 25, 69–75. doi: 10.1188/21.CJON.69-75
Shao, Y., and Zhang, W. (2020). Psychological and ocular surface state of ophthalmologists and ophthalmic nurses working with patients with coronavirus disease 2019. JAMA Ophthalmol. 138, 907–910. doi: 10.1001/jamaophthalmol.2020.2289
Shevlin, M., Butter, S., McBride, O., Murphy, J., Gibson-Miller, J., Hartman, T. K., et al. (2022). Measurement invariance of the patient health questionnaire (PHQ-9) and generalized anxiety disorder scale (GAD-7) across four European countries during the COVID-19 pandemic. BMC Psychiatry 22:154. doi: 10.1186/s12888-022-03787-5
Singh, D., and Yi, S. V. (2021). On the origin and evolution of SARS-CoV-2. Exp. Mol. Med. 53, 537–547. doi: 10.1038/s12276-021-00604-z
Sjöberg, A., Pettersson-Strömbäck, A., Sahlén, K. G., Lindholm, L., and Norström, F. (2020). The burden of high workload on the health-related quality of life among home care workers in northern Sweden. Int. Arch. Occup. Environ. Health 93, 747–764. doi: 10.1007/s00420-020-01530-9
Skevington, S. M., and Tucker, C. (1999). Designing response scales for cross-cultural use in health care: data from the development of the UK WHOQOL. Br. J. Med. Psychol. 72, 51–61. doi: 10.1348/000711299159817
Stocker, R., Tran, T., Hammarberg, K., Nguyen, H., Rowe, H., and Fisher, J. (2021). Patient health questionnaire 9 (PHQ-9) and general anxiety disorder 7 (GAD-7) data contributed by 13,829 respondents to a national survey about COVID-19 restrictions in Australia. Psychiatry Res. 298:113792. doi: 10.1016/j.psychres.2021.113792
Sun, Y., Fu, Z., Bo, Q., Mao, Z., Ma, X., and Wang, C. (2020). The reliability and validity of PHQ-9 in patients with major depressive disorder in psychiatric hospital. BMC Psychiatry 20:474. doi: 10.1186/s12888-020-02885-6
Taha, M. J. J., Abuawwad, M. T., Alrubasy, W. A., Sameer, S. K., Alsafi, T., Al-Bustanji, Y., et al. (2022). Ocular manifestations of recent viral pandemics: a literature review. Front. Med. 9:1011335. doi: 10.3389/fmed.2022.1011335
The Johns Hopkins Coronavirus Resource Center. (2023). COVID-19 map – Johns Hopkins coronavirus resource Center. Available at: https://coronavirus.jhu.edu/map.html (Accessed March 15, 2023).
Wang, W., Bian, Q., Zhao, Y., Li, X., Wang, W., Du, J., et al. (2014). Reliability and validity of the Chinese version of the patient health questionnaire (PHQ-9) in the general population. Gen. Hosp. Psychiatry 36, 539–544. doi: 10.1016/j.genhosppsych.2014.05.021
Wang, Y., Hu, Z., Feng, Y., Wilson, A., and Chen, R. (2020). Changes in network centrality of psychopathology symptoms between the COVID-19 outbreak and after peak. Mol. Psychiatry 25, 3140–3149. doi: 10.1038/s41380-020-00881-6
Wang, Y., Zhang, S., Liu, X., Shi, H., and Deng, X. (2023). Differences in central symptoms of anxiety and depression between college students with different academic performance: a network analysis. Front. Psychol. 14:1071936. doi: 10.3389/fpsyg.2023.1071936
Williams, A. M., Kalra, G., Commiskey, P. W., Bowers, E. M. R., Rudolph, B. R., Pitcher, M. D., et al. (2020). Ophthalmology practice during the coronavirus disease 2019 pandemic: the University of Pittsburgh experience in promoting clinic safety and embracing video visits. Ophthalmol. Ther. 9, 1–9. doi: 10.1007/s40123-020-00255-9
World Health Organization. (2023). WHOQOL: measuring quality of life. Available at: https://www.who.int/tools/whoqol (Accessed March 15, 2023).
Xie, T., Wen, J., Liu, X., Wang, J., and Poppen, P. J. (2022). Utilizing network analysis to understand the structure of depression in Chinese adolescents: replication with three depression scales. Curr. Psychol., 1–12. doi: 10.1007/s12144-022-03201-z
Yu, A. Y., Tu, R., Shao, X., Pan, A., Zhou, K., and Huang, J. (2020). A comprehensive Chinese experience against SARS-CoV-2 in ophthalmology. Eye Vis. 7:19. doi: 10.1186/s40662-020-00187-2
Yuan, Y., Liu, Z. H., Zhao, Y. J., Zhang, Q., Zhang, L., Cheung, T., et al. (2021). Prevalence of post-traumatic stress symptoms and its associations with quality of life, demographic and clinical characteristics in COVID-19 survivors during the post-COVID-19 era. Front. Psych. 12:665507. doi: 10.3389/fpsyt.2021.665507
Zhao, N., Li, W., Zhang, S.-F., Yang, B. X., Sha, S., Cheung, T., et al. (2021). Network analysis of depressive symptoms among residents of Wuhan in the later stage of the COVID-19 pandemic. Front. Psych. 12:735973. doi: 10.3389/fpsyt.2021.735973
Zheng, C. M., Shen, F., Tian, G. Q., and Zhang, L. (2020). Investigation on the stress level and depression of medical staff during the new coronavirus pneumonia outbreak (in Chinese). Zhejiang Med. 42, 406–414.
Zou, S., Liu, Z. H., Yan, X., Wang, H., Li, Y., Xu, X., et al. (2020). Prevalence and correlates of fatigue and its association with quality of life among clinically stable older psychiatric patients during the COVID-19 outbreak: a cross-sectional study. Glob. Health 16:119. doi: 10.1186/s12992-020-00644-6
Keywords: COVID-19, depression, ophthalmology, nurse, network analysis
Citation: Liu Z-H, Li Y, Tian Z-R, Zhao Y-J, Cheung T, Su Z, Chen P, Ng CH, An F-R and Xiang Y-T (2023) Prevalence, correlates, and network analysis of depression and its associated quality of life among ophthalmology nurses during the COVID-19 pandemic. Front. Psychol. 14:1218747. doi: 10.3389/fpsyg.2023.1218747
Edited by:
Yi Nam Suen, The University of Hong Kong, Hong Kong SAR, ChinaReviewed by:
Rahim Badrfam, Alborz University of Medical Sciences, IranHongguang Chen, Peking University Sixth Hospital, China
Copyright © 2023 Liu, Li, Tian, Zhao, Cheung, Su, Chen, Ng, An and Xiang. This is an open-access article distributed under the terms of the Creative Commons Attribution License (CC BY). The use, distribution or reproduction in other forums is permitted, provided the original author(s) and the copyright owner(s) are credited and that the original publication in this journal is cited, in accordance with accepted academic practice. No use, distribution or reproduction is permitted which does not comply with these terms.
*Correspondence: Pan Chen, eWMxNzYwOUB1bS5lZHUubW8=; Feng-Rong An, YWZyeWxtQHNpbmEuY29t; Chee H. Ng, Y25nQHVuaW1lbGIuZWR1LmF1
†These authors have contributed equally to this work