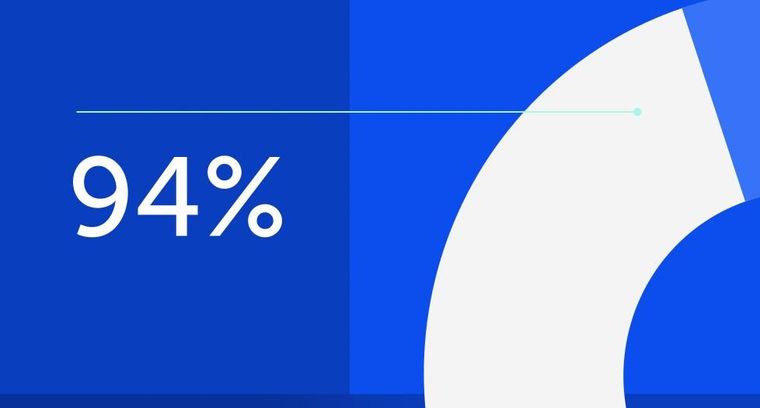
94% of researchers rate our articles as excellent or good
Learn more about the work of our research integrity team to safeguard the quality of each article we publish.
Find out more
ORIGINAL RESEARCH article
Front. Psychol., 23 May 2023
Sec. Educational Psychology
Volume 14 - 2023 | https://doi.org/10.3389/fpsyg.2023.1177160
This research aims to combine the pursuit of literacy cultivation with a focused investigation into the evidence of game-based teaching (GBT). To achieve this, the study employs a mixed-methods approach including the interview method, Delphi method, and network hierarchical analysis (ANP) to analyse Expert opinions and construct a comprehensive GBT evaluation index system. The results indicated that a comprehensive GBT evaluation index system is comprised of five primary indicators: teaching objectives, game-based teaching methods, teaching content, game-based teaching processes, and game-based teaching characteristics. Additionally, there are 19 secondary indicators, such as objective content, game presentation, context construction, and flow experience. This study expects to effectively capture the unique attributes of game-based classes and to assist teachers in improving the design of GBT activities in practical applications.
Game-based teaching (GBT) has emerged as a cutting-edge and innovative pedagogical approach, garnering significant attention in education policies and reports for its numerous advantages. The World Economic Forum’s report “Schools of the Future: Defining a New Education Model for the Fourth Industrial Revolution,” published in January 2020, highlights the potential impact of game-based teaching and learning on personalized and self-paced learning, and the transformative role it can play in the education system (World Economic Forum, 2020). While GBT has predominantly been implemented in K12 education (Hwang et al., 2016; Müller et al., 2018; Yukselturk et al., 2018), research indicates that it can effectively enhance the learning interests of learners across various age groups, including early childhood education (Gallegos et al., 2017; Tobar-Muñoz et al., 2017; Sung and Hwang, 2018; Wu, 2018; Yu et al., 2018). Evidence suggests that GBT stimulates intrinsic motivation and leads to a significant improvement in learning outcomes. Several studies have demonstrated that the adoption of game-based teaching methods in math and science has resulted in a marked improvement in students’ academic performance (Kim and Ke, 2017; Alzubi et al., 2018; Kiili et al., 2018; Brezovszky et al., 2019). In recent years, game-based teaching has been extending to higher education, displaying a broad range of potential applications (Iosup and Epema, 2014; Chen et al., 2018; Perini et al., 2018).
As GBT gains in popularity, a concerning issue has come to light: well-crafted game-based instruction developed by teachers is often met with reluctance or not validated by experts. As a result, it is imperative to establish an effective game-based teaching evaluation index system that can clarify the fundamental tenets of GBT.
GBT falls under the umbrella of GBL research, which focuses on educational games and game-based learning. The former centers around the existence of independent games, while the latter emphasizes the integration of game-based elements into regular instruction. Both areas share several dimensions, including the evaluation of the approach. The evaluation is challenging in most cases but especially when educational games are used (Serrano et al., 2012). The academic community has conducted numerous evaluation studies on educational games, Petri et al. (2016) improved the model based on MEEGA (a model for assessing the quality of educational games) to assess the perceived quality of educational games from the perspective of player experience and perceived learning. Torres-Toukoumidis et al. (2019) integrated theoretical model of gamification (E-MIGA) translated into an evaluation and quantitative assessment instrument based on expert opinion, which is based on the characteristics of the student population, the teacher role RP, the student role (RA), and other actors in the process (OA) 4 dimensions to evaluate 10 educational games with high download numbers in AppStore (IOS) and PlayStore (Android).some of which are based on the fundamental paradigm of “education+game+x,” such as educational, playful, and technical (Mohamed and Jaafar, 2010; Savi et al., 2011). Ye et al. (2009) has differentiated educational evaluation into cognitive and cognitive process dimensions and incorporated game attributes to establish the evaluation index. Faizan et al. (2019) created a strategy for assessing simulation games used in the classroom, indicating the assessment instruments used at different stages and what to assess in terms of pre-, mid-, and post-game. But as Seaborn says, despite the appearance of forming an increasingly cohesive whole, not all examples of gameplay outside of games can be subsumed under these headings or along these research paths (referring to serious games; Seaborn and Fels, 2015).
Simultaneously, Sailer and Homner (2020) elucidated, through the outcomes of a comprehensive meta-analysis, that gamification in contemporary empirical research constitutes an efficacious pedagogical approach. Game-based teaching (GBT) investigations have predominantly concentrated on academic performance, engagement within a system, and the alacrity of task and assignment execution (Majuri et al., 2018). Examining GBT research from an evaluative standpoint, Tsai et al. (2015) devised an online formative assessment game incorporating multi-objective gaming strategies to probe its effectiveness within an online energy education course. Conversely, Tirado-Olivares (Torres-Toukoumidis et al., 2019) undertook a pre-experimental investigation, employing a mixed-methods modality, utilizing experience points, and a technologically adapted traditional class diary to gage the potential of a gamified formative assessment system. This approach proved effective for assessing learning accomplishments and cross-curricular educational aspects, such as collaborative efforts, classroom interest, and daily task revision. ANA (2019) conceived a game, entitled Downtown, explicitly tailored for individuals with intellectual disabilities, such as Down syndrome, specific Autism Spectrum Disorder (ASD) manifestations, or mild cognitive impairments. The game’s objective is to instruct students in public subway system navigation throughout the city, thereby fostering autonomy and enhancing independent living skills. ANA amassed data on total game session durations, average route completion times, inactivity periods, overall minigame performance, and additional observable factors to establish a distinct construct for validating Downtown’s design and development procedures. A substantial body of GBT assessment research exists, with extant inquiries emphasizing the potential benefits for educators and pupils within classroom settings and how such effects transpire. However, these studies often neglect to address the core concept of GBT, displaying minimal cohesion regarding its theoretical foundations and the essence of gamification (Seaborn and Fels, 2015). A salient inquiry arises: can these impacts be attributed to the diverse ways in which games are incorporated into teaching and learning practices within GBT? In other words, does research bias persist due to inadequate comprehension of GBT characteristics? Alternatively, an excessive focus on individual influences within GBT activities may hinder a comprehensive understanding of the approach. To tackle these concerns, the present study establishes a GBT evaluation index system encompassing the entirety of instructional activities, with educators and students as the principal subjects. The research initially formulates an indicator framework, drawing from an extensive literature review and a voluminous dataset of interview data. Subsequently, the Analytic Network Process (ANP) method is employed to allocate weights to the indicators, while regression analysis is performed to corroborate the framework’s effectiveness. This paper aims to answer the following research questions:
RQ1: What indicators can be utilized to illustrate GBT?
RQ2: Which indicators have the most significant influence on the development of GBT?
RQ3: How can a class utilizing GBT be evaluated?
To address the question “What indicators can be used to illustrate GBT?,” the study utilized mixed methods to construct the GBT evaluation index system in three stages. Firstly, the evaluation indices for GBT were developed through the use of the literature research method and interview technique. Secondly, two rounds of expert consultation were conducted via the Delphi method to refine the initial indices. Finally, the network hierarchical analysis (ANP) was used to determine the weightage of each indicator, in order to answer the query, “Which indicators have the most significant influence on the development of GBT?”
The system of evaluation indicators for GBT must incorporate the notion that effective teaching can be enjoyable and engaging. The evaluation indices for GBT are centered around two key dimensions: the pedagogical dimension and the game dimension. In terms of pedagogy, both game-based and traditional teaching share the common goal of delivering the curriculum content within a predetermined time and location. Therefore, the study sought to investigate the similarities and differences between the two methods in terms of the pedagogical dimension. Regarding games, the study delved into which aspects of teaching and learning are affected when games (or game mechanisms) are introduced into the classroom. The exploration of this topic was conducted from a theoretical perspective.
GBT represents an innovation in teaching methods, as it deviates from conventional teaching by incorporating games or game mechanisms. This implies that GBT should be similar to traditional teaching in terms of content. However, GBT offers undeniable advantages in terms of methodology and implementation. In practice, the evaluation of conventional teaching typically revolves around the components of teaching and learning. Table 1 offers a comprehensive overview of the various instruction components.
Table 1 highlights that evaluation of instruction is often closely linked to components such as teachers, students, content, and methods. These categories are fundamental aspects that must be considered when constructing an instruction evaluation index system. Teachers and students are crucial components of the instruction relationship, but they cannot be directly evaluated. Therefore, they are typically incorporated into the evaluation indices as a specific perspective in the development of the evaluation index system. Consequently, this study focuses on six main indicators for the evaluation index system: objectives, content, process, methods, environment, and media.
The incorporation of games (or game mechanics) in teaching and learning is the defining factor that sets it apart from conventional teaching methods. According to Kapp’s definition (Kapp, 2012), games are comprised systems, players, abstraction, challenge, rules, interactivity, feedback, quantifiable outcomes, and emotional responses, and these factors combine to revolutionize teaching and learning. In games, “abstraction” refers to the representation of reality within a virtual context that retains some aspects of reality. In teaching, this concept is often referred to as “contextualization.” The presence and necessity of contextualization in both games and teaching confirms its validity as a category in the evaluation of GBT. Feedback mechanisms play a crucial role in promoting greater immersion in play (Law and Chen, 2016; Pilegard and Mayer, 2016; Yang, 2017). Thus, the timeliness of feedback needs to be explicitly addressed in the evaluation indices. Some research indicates that autonomy, competence, and relevance independently predict enjoyment and future engagement in play (Ryan et al., 2006). In other words, the enjoyment of play emphasizes the degree of autonomy, competence, and relevance of the play to the content in the gamification process, which serves as a precondition for assessing whether the play is fun or not. This is a prerequisite for evaluating whether the game is enjoyable or not. Therefore, the evaluation items of timeliness of feedback, the comprehensiveness of the process, and contextualization have been extracted from the game dimension.
Based on the previous discussion of the two dimensions of teaching and play, the researchers have identified primary indicators such as objectives, content, process, methods, environment, and media, as well as secondary evaluation indicators such as timeliness of feedback, process fun, and contextuality. However, to refine the main indicators into operational and specific indicators, and to take into account any characteristics of GBT that still need to be considered, the researchers conducted in-depth interviews with over 20 teachers who use GBT in practice. The original interview data underwent an open coding process, where the researchers aimed to restore the teachers’ evaluation concept of GBT as exhaustively and comprehensively as possible by retaining the teachers’ original words and text while focusing on measurable relevant statements and concepts. Table 2 illustrates the open coding process for one teacher’s interview data.
The researchers proceeded with an Axial Coding analysis of all the open coding extracted, which involved summarizing and grouping codes with similar essential attributes. For instance, codes such as “objectives should be scientific and reasonable,” “objectives should be specific,” and “objectives should be hierarchical” were coded as “scientific.” The code “teachers should create situations in the class” was coded as “contextual.” This process resulted in 19 Axial codings. Subsequently, the researchers carried out a selective coding process that combined the instructional elements and extracted three core categories: the teacher category, the student category, and the classroom game interaction category. The process of selective coding is presented in Table 3.
After processing the interview data, the study created a preliminary GBT evaluation index system that uses the selective codings as the main categories and associated categories as the primary indicators. The system consists of eight primary indicators, including teaching objectives, instructional methods, and emotional constructs, as well as 20 secondary indicators, such as scientificity, clarity, and playfulness. The primary indicators represent the essential aspects of GBT, while the secondary indicators provide a more detailed and specific evaluation of these aspects. The system aims to provide a comprehensive and objective evaluation of GBT that can help teachers design and implement effective GBT activities.
To ensure the scientific validity of the evaluation indicators and eliminate the influence of theoretical constructs and subjective elements from the interview process, the study used the Delphi method to revise the initially constructed evaluation indicators. The researcher selected 10 experts in the field of game-based learning, including three professors, two associate professors, and five teachers who use GBT, including two expert teachers. In the first round of specialist consultation, the experts pointed out several problems with the proposed index system. These included the slightly confusing dimensions of the indicators at the same level, the lack of uniformity in textual descriptions, and some indicators failing to reflect the characteristics of game-based teaching. As an example, Table 4 shows the statistical results of some secondary indicators and their revision opinions.
The second round of expert consultation received significantly fewer opinions, but some experts still proposed revisions to the indicators. Eventually, one secondary indicator was deleted, and the detailed descriptions of two indicators were revised. The mean, standard deviation, and coefficient of variation of experts’ scores in the second round of consultation were significantly better than those in the first round, indicating that the experts had reached a consensus. As a result, the “GBT Evaluation Index” was revised based on two rounds of expert consultation, which consists of 5 primary indicators and 19 secondary indicators. These are listed in Table 5.
The indicator entries describe factors to be considered in teaching and learning, but they do not indicate the importance of each factor in influencing teaching and learning activities. This study used a weighting system to determine the relative importance of each factor. The network hierarchical analysis (ANP) was chosen to assign weights as it is specific to the field of education and teaching, where many indicators interact and provide feedback dynamically in a classroom setting.
The researchers established the network structure of the index system, with “Evaluation of GBT” as the main objective layer, and five primary indicators as the control criterion layer, including instructional objectives (O), game-based teaching methods (S), instructional materials (M), game-based teaching processes (P), and game-based teaching feature (F). The 19 secondary indicators were divided into different sets of components and numbered as O1 (system architecture), O2 (objective), M1 (knowledge system), and M2 (contextual construction), respectively. Based on the above settings, the study established the network structure of the GBT evaluation system, as shown in Figure 1.
To obtain the weights of the secondary indicators, the researchers followed the same procedure as with the primary indicators, using ANP to establish a multi-level network structure of the evaluation system. The researchers then issued another ANP Expert Opinion Questionnaire to the same group of five experts, asking them to judge the relative importance of the secondary indicators within their corresponding primary indicator sets. The experts were asked to provide a judgment matrix for each set of secondary indicators, and the weights were calculated using the ANP method. The final weights of the secondary indicators were obtained by multiplying the weight of the corresponding primary indicator by the weight of the corresponding secondary indicator within the set. The weights of the secondary indicators are included in the complete evaluation index system presented in Table 6.
After multiple rounds of expert consultation, data coding, and statistical analysis, this study has established a comprehensive evaluation indicator system for GBT that considers the pedagogical dimension and the game dimension, as shown in Table 7. The evaluation system consists of five primary indicators (Instructional objectives, game-based teaching methods, instructional materials, game-based teaching processes, and game-based teaching features) and 19 secondary indicators. The weightings of each indicator have been calculated using the ANP method and are included in the evaluation system. This GBT evaluation system can provide guidance for teachers and educators to create effective and engaging game-based learning environments that foster conceptual understanding and personal development.
An experiment was conducted to answer the second research question, and the results are reported in subsequent sections.
Two undergraduate students majoring in mathematics and interested in GBL were invited to evaluate five game-based class video recordings using the GBT evaluation index system developed in this study. The two raters were selected based on their background and experience in education and GBL. They were both teacher trainees and had some teaching experience, and their research interests were also related to GBL. The raters evaluated the recordings using the GBT evaluation index system and provided feedback on the clarity and usefulness of the system. The feedback was used to further refine the evaluation index system.
In the first stage of the study, the inter-rater agreement between the two raters was tested for reliability using the evaluation index system of GBT. Given that there were only two raters in this study, Spearman’s rank correlation was used to analyze their reliability. The results showed that the reliability coefficient of the two raters reached 0.9 (Sig = 0.037 < 0.05), indicating a high level of agreement between the two observers when using this index system for evaluation.
The dependent variable in the regression analysis is the total score of the scale used in this study. Before conducting the analysis, the normality of the data was tested using both the normality test (Sig1 = 0.106 > 0.05; Sig2 = 0.32 > 0.05) and the Chi-square test (Sig = 0.551 > 0.05), which indicated that the hypothesis of normal distribution of the data cannot be rejected, and the homogeneity of variance can be assumed.
In the second stage of the study, the validity of each of the five primary indicators was verified by subjecting their scores to a linear regression analysis with the overall scale scores. This analysis aimed to use the scores of each primary indicator to predict the scores of the overall scale and determine their validity. A high coefficient of determination (R2) would indicate a strong correlation between the scores of the primary indicators and the overall scale.
After conducting the regression analysis, a high coefficient of determination (R2) was found when using “Instructional objectives” to predict the total score (R2 = 0.947, Sig = 0.000 < 0.05), as shown in Table 8. Figure 2 displays the scatter plot and fit line of the “Instructional objectives” score used to predict the overall scale score.
The study proceeded to conduct an ANOVA test on the regression relationship to determine the presence of a significant linear regression relationship between the overall scale scores and the “Instructional objectives” indicator scores. The results of the regression ANOVA demonstrated a significant linear regression between the “Instructional objectives” indicator scores and the overall scale scores (F = 143.683, p = 0.000 < 0.001). Hence, it can be inferred that the indicators of the “Instructional objectives” dimension can effectively predict the overall scale scores.
After conducting the regression analysis, a high coefficient of determination (R2) was found when using “game-based teaching methods” to predict the total score (R2 = 0.974, Sig = 0.000 < 0.05), as shown in Table 9. Figure 3 displays the scatter plot and fit line of the “game-based teaching methods” score used to predict the overall scale score.
The study proceeded to conduct an ANOVA test on the regression relationship to determine the presence of a significant linear regression relationship between the overall scale scores and the “game-based teaching methods” indicator scores. The results of the regression ANOVA demonstrated a significant linear regression between the “game-based teaching methods” indicator scores and the overall scale scores (F = 300.838, p = 0.000 < 0.001). Hence, it can be inferred that the indicators of the “game-based teaching methods” dimension can effectively predict the overall scale scores.
After conducting the regression analysis, a high coefficient of determination (R2) was found when using “Instructional Materials” to predict the total score (R2 = 0.901, Sig = 0.000 < 0.05), as shown in Table 10. Figure 4 displays the scatter plot and fit line of the “Instructional Materials” score used to predict the overall scale score.
The study proceeded to conduct an ANOVA test on the regression relationship to determine the presence of a significant linear regression relationship between the overall scale scores and the “Instructional Materials” indicator scores. The results of the regression ANOVA demonstrated a significant linear regression between the “Instructional Materials” indicator scores and the overall scale scores (F = 72.803, p = 0.000 < 0.001). Hence, it can be inferred that the indicators of the “Instructional Materials” dimension can effectively predict the overall scale scores.
After conducting the regression analysis, a high coefficient of determination (R2) was found when using “game-based teaching process” to predict the total score (R2 = 0.974, Sig = 0.000 < 0.05), as shown in Table 11. Figure 5 displays the scatter plot and fit line of the “game-based teaching process” score used to predict the overall scale score.
The study proceeded to conduct an ANOVA test on the regression relationship to determine the presence of a significant linear regression relationship between the overall scale scores and the “game-based teaching process” indicator scores. The results of the regression ANOVA demonstrated a significant linear regression between the “game-based teaching process” indicator scores and the overall scale scores (F = 313.331, p = 0.000 < 0.001). Hence, it can be inferred that the indicators of the “game-based teaching process” dimension can effectively predict the overall scale scores.
After conducting the regression analysis, a high coefficient of determination (R2) was found when using “game-based teaching feature” to predict the total score (R2 = 0.957, Sig = 0.000 < 0.05), as shown in Table 12. Figure 6 displays the scatter plot and fit line of the “game-based teaching feature” score used to predict the overall scale score.
The study proceeded to conduct an ANOVA test on the regression relationship to determine the presence of a significant linear regression relationship between the overall scale scores and the “game-based teaching feature” indicator scores. The results of the regression ANOVA demonstrated a significant linear regression between the “game-based teaching feature” indicator scores and the overall scale scores (F = 177.588, p = 0.000 < 0.001). Hence, it can be inferred that the indicators of the “game-based teaching feature” dimension can effectively predict the overall scale scores.
Now, let us return to the research questions of this study:
What indicators can be utilized to illustrate GBT? To achieve this goal, the study first identified two core dimensions of GBT: instructional and game. Based on these dimensions, the study developed a new scale consisting of 5 primary and 29 secondary indicators using a theory-based approach. Upon examining the aforementioned premises, it becomes evident that Game-Based Teaching (GBT) encompasses five distinct categories: instructional objectives, instructional materials, game-based teaching processes, game-based teaching methods, and game-based teaching features. The majority of scholars who have endeavored to delineate the factors integral to devising instructional programs have emphasized the importance of identifying objectives (Glaser, 1966). If educators can effectively devise instructional strategies, anticipated instructional goals should be attainable through predetermined accomplishments, contingent upon student needs (Myers et al., 2017; Browne, 2018; Rapson, 2018). Instructional objectives constitute the cornerstone of GBT, suggesting that when designing GBT for learners, meticulous consideration should be given to which learner needs can be fulfilled by instructional objectives.
Instructional materials pertain to the resources through which learners construct individual knowledge. Moreover, some scholars posit that instructional design (ID) is the scientific discipline concerned with designing, developing, evaluating, and maintaining instructional materials to create comprehensive specifications that facilitate learning and performance (Martin, 2011). Consequently, instructional materials delineate the boundaries of GBT and circumscribe learners’ experiences.
Of the five domains, the most crucial are game-based teaching processes, game-based teaching methods, and game-based teaching features. These domains elucidate the necessity for GBT to actively involve learners in game activities, as GBT not only generates games for learners but also crafts learning activities that progressively introduce concepts and guide users toward the ultimate objective (Pho and Dinscore, 2015). In this context, learners transition from mere consumers to producers of content through their actions and decision-making processes (Gee, 2003). The merit of this study’s indicator system, which portrays the comprehensive framework of GBT through five categories, lies in its capacity to offer a holistic perspective on the influence of play (or gamification) on teaching and learning Activities.
Which indicators have the most significant influence on the development of GBT? In light of the weighted schema employed in the indicator framework, we suggest that Instructional Materials play a crucial role in the progression of Game-Based Teaching (GBT). Some scholars have remarked on the limited presence of games capable of empirically showcasing effectiveness in delivering academic content when used independently in educational contexts (Garris et al., 2017). GBT often receives praise for its propensity to encourage student engagement in gameplay and promote positive social interactions (Gunter et al., 2007). Shi hypothesizes that this occurrence may be attributed to game designers’ skill in devising intriguing games, without necessarily preserving material quality, while educators concentrate on potent instructional resources but may struggle with creating engaging games (Shi and Shih, 2015). This study aligns with the insights of Shi et al. the underlying objective of GBT is not solely to provide amusement and playfulness, but rather to work toward achieving germane academic goals (Cheng and Su, 2012). This leads to the question of how best to design instructional materials that support students’ academic growth. Gunter et al. (2007) contends that integrating newly acquired content within contextual settings is essential for the success of games in reinforcing students’ learning. Fu et al. (2019)highlight the considerable potential of authentic scenarios in making learning more meaningful. The “Contextual Constructs” indicator in the framework, which achieved the highest weight within the Instructional Materials dimension, endorses these viewpoints. This finding suggests that a key consideration in GBT implementation rests in the careful design of Instructional Materials and that ensuring high-quality Contextual Constructs is a vital precondition for realizing GBT.
How can a class utilizing GBT be evaluated? Through the regression analysis, it was discerned that each of the primary indicators more accurately forecasted the cumulative scale scores; consequently, the evaluative framework devised in this investigation succeeded in encouraging disparate evaluators to appraise classrooms employing GBT uniformly, predicated on the content of the indicators. Enhancing the caliber of instruction and learning constitutes a pivotal element in the majority of proposals aimed at augmenting school quality (Hanushek et al., 2016). Certain investigations have advocated the utilization of feedback surveys, inspections (via peer observation), and enigmatic clientele (students) as the three principal methods for monitoring service quality in higher education (Douglas and Douglas, 2006). Educators can elevate the quality of their instruction by refining their pedagogical activities (Hau, 1996). As such, evaluating instruction and learning entails assessing instructors (and their classroom interactions). Educator assessment, designed to enhance instructional quality, embodies a formative assessment that molds teachers’ performance to render it more efficacious (Popham, 1988). The indicator system we devised represents a formative evaluation framework that assists educators in acquiring impartial outcomes in the formative assessment of GBT and in receiving comprehensive guidance within such assessments to bolster the quality of instruction and learning.
This study has developed a GBT evaluation index system with three main considerations. Firstly, it emphasizes the guiding role of the index system and provides an evaluation system for various game-based teaching activities. Secondly, it pays attention to the goal orientation of literacy cultivation to avoid the evaluation indexes leading to a cycle of “literacy without teaching.” Lastly, it focuses on the critical status of sub-disciplinary teaching and meets the established requirements of the index system for sub-disciplinary teaching.
As the indicator system demonstrates, GBT reflects the differences between its and other instruction methods in terms of both instruction and game. We can now provide a powerful response to the basic principles of GBT, namely, effective teaching and fun and games. This principle brings a fresh perspective to GBT, helping us to look at the impact that the vehicle of play brings to teaching and learning and how these impacts occur in the context of the totality of teaching and learning activities. It is worth noting that the assessment function of the indicator system may be an opponent of teachers in some cases, so we need to emphasize that the purpose of the GBT evaluation index system is not to grade teachers who use GBT but to establish a reference system that educators can use to assess their GBT practices. Teachers should utilize this system to reflect on and improve their GBT approaches (Popham, 1988), identify any areas for improvement, and promote the advancement of teaching reform in schools.
This study has focused on evaluating teaching within the field of GBT, but it has not defined the problem and has not conducted an in-depth investigation on classes of different subjects and levels of students. Methodologically, the number of samples for comparison in the difference comparison study is relatively small, which may limit the generalizability of some of the conclusions. In using the Delphi method, the study chose only 10 experts, which may have limited the scope of the evaluation.
Considering the limitations of this study, several suggestions are proposed for future research. Firstly, it is recommended to incorporate students’ views and opinions on GBT in the index system to broaden its scope. Secondly, to ensure the generalizability of the research findings, future studies should increase the sample size of experts, teachers, and GBT recordings. Lastly, it is suggested that different subjects and class levels be included to explore the applicability of the evaluation indicators. These improvements could enhance the comprehensiveness and reliability of the evaluation index system, and provide more practical guidance for GBT practice.
The raw data supporting the conclusions of this article will be made available by the authors, without undue reservation.
YW and SZ: conceptualization. HH: methodology. YW: software, validation, and investigation. SZ: resources, supervision, and funding acquisition. YW and HH: writing–original draft preparation. HW and SZ: writing–review and editing. All authors have read and agreed to the published version of the manuscript.
This research work was supported by the “13th Five-Year Plan” Teacher Education Innovation Project of Zhejiang Province: “Digital Competence Development of Future Teachers” Office of Zhejiang Provincial Education Department [2017], No. 80 and 2021 Ministry of Education Industry-University Cooperation Collaborative Education Project: “Construction of Virtual Reality Teaching Practice Base of Hangzhou Normal University” (202102464071).
The authors declare that the research was conducted in the absence of any commercial or financial relationships that could be construed as a potential conflict of interest.
All claims expressed in this article are solely those of the authors and do not necessarily represent those of their affiliated organizations, or those of the publisher, the editors and the reviewers. Any product that may be evaluated in this article, or claim that may be made by its manufacturer, is not guaranteed or endorsed by the publisher.
Algozzine, B., and Ysseldyke, J. (1992). Strategies and tactics for effective instruction. Educ. Resour. Inf. Centr. 26, 34–36.
Alzubi, T., Fernández, R., Flores, J., Duran, M., and Cotos, J. M. (2018). Improving the working memory during early childhood education through the use of an interactive gesture game-based learning approach. IEEE Access 6, 53998–54009. doi: 10.1109/ACCESS.2018.2870575
Aulls, M. W., and Ibrahim, A. (2012). Pre-service teachers’ perceptions of effective inquiry instruction: are effective instruction and effective inquiry instruction essentially the same? Instr. Sci. 40, 119–139. doi: 10.1007/s11251-010-9164-z
Brezovszky, B., McMullen, J., Veermans, K., Hannula-Sormunen, M. M., Rodríguez-Aflecht, G., Pongsakdi, N., et al. (2019). Effects of a mathematics game-based learning environment on primary school students' adaptive number knowledge. Comput. Educ. 128, 63–74. doi: 10.1016/j.compedu.2018.09.011
Browne, M. (2018). Teachers’ perceptions on the common core standards and effective classroom instruction for students with disabilities: a case study approach. pp. 1–112.
Chen, Z. H., Chen, H. H. J., and Dai, W. J. (2018). Using narrative-based contextual games to enhance language learning: a case study. J. Educ. Technol. Soc. 21, 186–198.
Cheng, C.-H., and Su, C.-H. (2012). A game-based learning system for improving student's learning effectiveness in system analysis course. Procedia. Soc. Behav. Sci. 31, 669–675. doi: 10.1016/j.sbspro.2011.12.122
Dick, W., Carey, L., and Carey, J. O. (2005). The systematic design of instruction. London, UK: Pearson Education.
Douglas, J., and Douglas, A. (2006). Evaluating teaching quality. Qual. High. Educ. 12, 3–13. doi: 10.1080/13538320600685024
Faizan, N. D., Löffler, A., Heininger, R., Utesch, M., and Krcmar, H. (2019). Classification of evaluation methods for the effective assessment of simulation games: results from a literature review. Int. J. Eng. Pedagog. 9, 19–33. doi: 10.3991/ijep.v9i1.9948
Fu, Q.-K., Lin, C.-J., Hwang, G.-J., and Zhang, L. (2019). Impacts of a mind mapping-based contextual gaming approach on EFL students’ writing performance, learning perceptions and generative uses in an English course. Comput. Educ. 137, 59–77. doi: 10.1016/j.compedu.2019.04.005
Gagné, R. M. (1977). “Designing instruction for learning,” in The conditions of learning and theory of instruction, eds. H. Kirk and B. Iginla (New York: Holt, Rinehart and Winston), 303–305.
Gallegos, C., Tesar, A. J., Connor, K., and Martz, K. (2017). The use of a game-based learning platform to engage nursing students: a descriptive, qualitative study. Nurse Educ. Pract. 27, 101–106. doi: 10.1016/j.nepr.2017.08.019
Garris, R., Ahlers, R., and Driskell, J. E. (2017). Games, motivation, and learning: A research and practice model. Simulation in Aviation Training, 475–501. doi: 10.4324/9781315243092-25
Gee, J. P. (2003). What video games have to teach us about learning and literacy. Comput. Entertain. 1:20. doi: 10.1145/950566.950595
Glaser, R. (1966). Teaching machines and programed learning, II: data and directions. AAUP Bull. 52:350. doi: 10.2307/40224180
Gunter, G. A., Kenny, R. F., and Vick, E. H. (2007). Taking educational games seriously: using the retain model to design endogenous fantasy into standalone educational games. Educ. Technol. Res. Dev. 56, 511–537. doi: 10.1007/s11423-007-9073-2
Hanushek, E. A., Machin, S., Woessmann, L., Hanushek, E. A., and Rivkin, S. G. (2016). “Teacher quality” in Handbook of the economics of education. eds. E. A. Hanushek and F. Welch (Amsterdam: Elsevier North Holland), 1051–1078.
Hau, I. (1996). Teaching quality improvement by quality improvement in teaching. Qual. Eng. 9, 77–94. doi: 10.1080/08982119608919019
Hwang, W. Y., Shih, T. K., Ma, Z. H., Shadiev, R., and Chen, S. Y. (2016). Evaluating listening and speaking skills in a mobile game-based learning environment with situational contexts. Comput. Assist. Lang. Learn. 29, 639–657. doi: 10.1080/09588221.2015.1016438
Hwang, G.-J., Sung, H.-Y., Hung, C.-M., Huang, I., and Tsai, C.-C. (2012). Development of a personalized educational computer game based on students’ learning styles. Educ. Technol. Res. Dev. 60, 623–638. doi: 10.1007/s11423-012-9241-x
Iosup, A., and Epema, D. (2014). An experience report on using gamification in technical higher education. In Proceedings of the 45th ACM technical symposium on Computer science education. pp. 27–32.
Popham, W. J. (1988). The dysfunctional marriage of formative and summative teacher evaluation. J. Pers. Eval. Educ. 1, 269–273. doi: 10.1007/bf00123822
Kapp, K. M. (2012). The gamification of learning and instruction: game-based methods and strategies for training and education. New York: John Wiley & Sons, 6–8.
Kiili, K., Moeller, K., and Ninaus, M. (2018). Evaluating the effectiveness of a game-based rational number training-in-game metrics as learning indicators. Comput. Educ. 120, 13–28. doi: 10.1016/j.compedu.2018.01.012
Kim, H., and Ke, F. (2017). Effects of game-based learning in an OpenSim-supported virtual environment on mathematical performance. Interact. Learn. Environ. 25, 543–557. doi: 10.1080/10494820.2016.1167744
Law, V., and Chen, C. H. (2016). Promoting science learning in game-based learning with question prompts and feedback. Comput. Educ. 103, 134–143. doi: 10.1016/j.compedu.2016.10.005
Levin, T., and Long, R. (1981). Effective instruction. New Delhi: Education Resources Information Center.
Majuri, J., Koivisto, J., and Hamari, J. (2018). Gamification of education and learning: A review of empirical literature. Proceedings of the 2nd International GamiFIN Conference.
Martin, F. (2011). Instructional design and the importance of instructional alignment. Community Coll. J. Res. Pract. 35, 955–972. doi: 10.1080/10668920802466483
Merrill, M. D. (2001). Components of instruction toward a theoretical tool for instructional design. Instr. Sci. 29, 291–310. doi: 10.1023/A:1011943808888
Mohamed, H., and Jaafar, A. (2010). Development and potential analysis of heuristic evaluation for educational computer game (PHEG). In: 5th international conference on computer sciences and convergence information technology. IEEE, pp. 222–227.
Müller, A., Son, J. B., Nozawa, K., and Dashtestani, R. (2018). Learning English idioms with a web-based educational game. J. Educ. Comput. Res. 56, 848–865. doi: 10.1177/0735633117729292
Myers, D., Freeman, J., Simonsen, B., and Sugai, G. (2017). Classroom management with exceptional learners. Teach. Except. Child. 49, 223–230. doi: 10.1177/0040059916685064
Perini, S., Luglietti, R., Margoudi, M., Oliveira, M., and Taisch, M. (2018). Learning and motivational effects of digital game-based learning (DGBL) for manufacturing education–the life cycle assessment (LCA) game. Comput. Ind. 102, 40–49. doi: 10.1016/j.compind.2018.08.005
Petri, G., Von Wangenheim, C. G., and Borgatto, A. F. (2016). Meega+: An evolution of a model for the evaluation of Educational Games. Available at: https://www.researchgate.net/publication/309031701_MEEGA_An_Evolution_of_a_Model_for_the_Evaluation_of_Educational_Games [Accessed May 11, 2023].
Pilegard, C., and Mayer, R. E. (2016). Improving academic learning from computer-based narrative games. Contemp. Educ. Psychol. 44-45, 12–20. doi: 10.1016/j.cedpsych.2015.12.002
Loderer, K., Pekrun, R., and Plass, J. L. (2020). “Emotional foundations of game-based learning,” in Handbook of Game-Based Learning, eds. J. L. Plass, R. E. Mayer, and B. D. Homer (Cambridge, MA: The MIT Press), 111–151.
Rapson, A. P. (2018). Exploring special education Teachers' perception of the use of data to inform instruction in the virtual educational environment: a case study. pp. 1–98. Northcentral University ProQuest Dissertations Publishing.
Ryan, R. M., Rigby, C. S., and Przybylski, A. (2006). The motivational pull of video games: a self-determination theory approach. Motiv. Emot. 30, 344–360. doi: 10.1007/s11031-006-9051-8
Sailer, M., and Homner, L. (2020). The Gamification of learning: a Meta-analysis. Educ. Psychol. Rev. 32, 77–112. doi: 10.1007/s10648-019-09498-w
Savi, R., Von Wangenheim, C. G., and Borgatto, A. F. (2011). A model for the evaluation of educational games for teaching software engineering. In 2011 25th Brazilian Symposium on Software Engineering. IEEE, pp. 194–203.
Schoenfeld-Tacher, R., and Sims, M. H. (2013). Course goals, competencies, and instructional objectives. J. Vet. Med. Educ. 40, 139–144. doi: 10.3138/jvme.0411-047r
Seaborn, K., and Fels, D. I. (2015). Gamification in theory and action: a survey. Int. J. Hum. Comput. Stud. 74, 14–31. doi: 10.1016/j.ijhcs.2014.09.006
Serrano, A., Marchiori, E. J., Del Blanco, A., Torrente, J., and Fernández-Manjón, B. (2012) A framework to improve evaluation in educational games, In: Proceedings of the 2012 IEEE global engineering education conference (EDUCON).
Shi, Y.-R., and Shih, J.-L. (2015). Game factors and game-based learning design model. Int. J. Comput. Games Technol. 2015, 1–11. doi: 10.1155/2015/549684
Slavin, R. E. (1995). “A model of effective instruction” in The Educational Forum, vol. 59 (Milton Park: Taylor & Francis), 166–176.
Sung, H. Y., and Hwang, G. J. (2018). Facilitating effective digital game-based learning behaviors and learning performances of students based on a collaborative knowledge construction strategy. Interact. Learn. Environ. 26, 118–134. doi: 10.1080/10494820.2017.1283334
Tanner, K. D. (2010). Order matters: using the 5E model to align teaching with how people learn. CBE Life Sci. Educ. 9, 159–164. doi: 10.1187/cbe.10-06-0082
Tay, J., Goh, Y. M., Safiena, S., and Bound, H. (2022). Designing digital game-based learning for professional upskilling: a systematic literature review. Comput. Educ. 184:104518. doi: 10.1016/j.compedu.2022.104518
Tobar-Muñoz, H., Baldiris, S., and Fabregat, R. (2017). Augmented reality game-based learning: enriching students’ experience during reading comprehension activities. J. Educ. Comput. Res. 55, 901–936. doi: 10.1177/0735633116689789
Torres-Toukoumidis, Á., Ramírez-Montoya, M. S., and Romero-Rodríguez, L. M. (2019). Valoración y evaluación de los Aprendizajes Basados en Juegos (GBL) en contextos e-learning. Educ. Knowl. Soc. EKS 19, 109–128. doi: 10.14201/eks2018194109128
Tsai, F.-H., Tsai, C.-C., and Lin, K.-Y. (2015). The evaluation of different gaming modes and feedback types on game-based formative assessment in an online learning environment. Comput. Educ. 81, 259–269. doi: 10.1016/j.compedu.2014.10.013
Ucus, S. (2015). Elementary school teachers’ views on game-based learning as a teaching method. Procedia. Soc. Behav. Sci. 186, 401–409. doi: 10.1016/j.sbspro.2015.04.216
Van Merriënboer, J. J., Clark, R. E., and De Croock, M. B. (2002). Blueprints for complex learning: the 4C/ID-model. Educ. Technol. Res. Dev. 50, 39–61. doi: 10.1007/BF02504993
Wang, A. I. (2015). The wear out effect of a game-based student response system. Comput. Educ. 82, 217–227. doi: 10.1016/j.compedu.2014.11.004
World Economic Forum. (2020). Available at: https://www.weforum.org/reports/schools-of-the-future-defining-new-models-of-education-for-the-fourth-industrial-revolution (Accessed February 22, 2022).
Wu, T. T. (2018). Improving the effectiveness of English vocabulary review by integrating ARCS with mobile game-based learning. J. Comput. Assist. Learn. 34, 315–323. doi: 10.1111/jcal.12244
Yang, K. H. (2017). Learning behavior and achievement analysis of a digital game-based learning approach integrating mastery learning theory and different feedback models. Interact. Learn. Environ. 25, 235–248. doi: 10.1080/10494820.2017.1286099
Ye, C. Q., Wang, H. Y., and Wang, P. (2009). A three-dimensional evaluation system framework of digital instructional game. J. Distance Educ. 17, 71–78. doi: 10.3969/j.issn.1672-0008.2009.06.014
Yu, H., Sirsat, S. A., and Madera, J. M. (2018). Enhancing hospitality students’ motivation to learn food safety knowledge using tablet personal computer (TPC)-based simulation game class activity. J. Hosp. Leis. Sport Tour. Educ. 23, 82–94. doi: 10.1016/j.jhlste.2018.08.002
Keywords: game-based teaching, class, evaluation index system, literacy cultivation, ANP
Citation: Wang Y, Zhang S and Hong H (2023) Research on the construction of the evaluation index system of game-based teaching. Front. Psychol. 14:1177160. doi: 10.3389/fpsyg.2023.1177160
Received: 01 March 2023; Accepted: 03 May 2023;
Published: 23 May 2023.
Edited by:
Nelly Lagos San Martín, University of the Bío Bío, ChileReviewed by:
Florin Nechita, Transilvania University of Brașov, RomaniaCopyright © 2023 Wang, Zhang and Hong. This is an open-access article distributed under the terms of the Creative Commons Attribution License (CC BY). The use, distribution or reproduction in other forums is permitted, provided the original author(s) and the copyright owner(s) are credited and that the original publication in this journal is cited, in accordance with accepted academic practice. No use, distribution or reproduction is permitted which does not comply with these terms.
*Correspondence: Sujing Zhang, MjFzanpoYW5nQDE2My5jb20=
Disclaimer: All claims expressed in this article are solely those of the authors and do not necessarily represent those of their affiliated organizations, or those of the publisher, the editors and the reviewers. Any product that may be evaluated in this article or claim that may be made by its manufacturer is not guaranteed or endorsed by the publisher.
Research integrity at Frontiers
Learn more about the work of our research integrity team to safeguard the quality of each article we publish.