- 1Unit of Psychiatry, Department of Public Health and Medicinal Administration, and Institute of Translational Medicine, Faculty of Health Sciences, University of Macau, Macao, Macao SAR, China
- 2Centre for Cognitive and Brain Sciences, University of Macau, Macao, Macao SAR, China
- 3The National Clinical Research Center for Mental Disorders and Beijing Key Laboratory of Mental Disorders, Beijing Anding Hospital and The Advanced Innovation Center for Human Brain Protection, Capital Medical University, Beijing, China
- 4Kiang Wu Nursing College of Macao, Macau, Macao SAR, China
- 5Faculty of Health Sciences and Sports, Macao Polytechnic University, Macao, Macao SAR, China
- 6School of Public Health, Southeast University, Nanjing, China
- 7School of Nursing, Hong Kong Polytechnic University, Hong Kong, Hong Kong SAR, China
- 8University of Notre Dame Australia, Fremantle, WA, Australia
- 9Division of Psychiatry, School of Medicine, University of Western Australia/Graylands Hospital, Mount Claremont, WA, Australia
- 10Department of Psychiatry, The Melbourne Clinic and St Vincent's Hospital, University of Melbourne, Richmond, VIC, Australia
Background: In the summer of 2022, Macau experienced a surge of COVID-19 infections (the 618 COVID-19 wave), which had serious effects on mental health and quality of life (QoL). However, there is scant research on mental health problems and QoL among Macau residents during the 618 COVID-19 wave. This study examined the network structure of depressive symptoms (hereafter depression), and the interconnection between different depressive symptoms and QoL among Macau residents during this period.
Method: A cross-sectional study was conducted between 26th July and 9th September 2022. Depressive symptoms were measured with the 9-item Patient Health Questionnaire (PHQ-9), while the global QoL was measured with the two items of the World Health Organization Quality of Life-brief version (WHOQOL-BREF). Correlates of depression were explored using univariate and multivariate analyses. The association between depression and QoL was investigated using analysis of covariance (ANCOVA). Network analysis was used to evaluate the structure of depression. The centrality index “Expected Influence” (EI) was used to identify the most central symptoms and the flow function was used to identify depressive symptoms that had a direct bearing on QoL.
Results: A total 1,008 participants were included in this study. The overall prevalence of depression was 62.5% (n = 630; 95% CI = 60.00–65.00%). Having depression was significantly associated with younger age (OR = 0.970; p < 0.001), anxiety (OR = 1.515; p < 0.001), fatigue (OR = 1.338; p < 0.001), and economic loss (OR = 1.933; p = 0.026). Participants with depression had lower QoL F (1, 1,008) =5.538, p = 0.019). The most central symptoms included PHQ2 (“Sad Mood”) (EI: 1.044), PHQ4 (“Fatigue”) (EI: 1.016), and PHQ6 (“Guilt”) (EI: 0.975) in the depression network model, while PHQ4 (“Fatigue”), PHQ9 (“Suicide”), and PHQ6 (“Guilt”) had strong negative associations with QoL.
Conclusion: Depression was common among Macao residents during the 618 COVID-19 wave. Given the negative impact of depression on QoL, interventions targeting central symptoms identified in the network model (e.g., cognitive behavioral therapy) should be developed and implemented for Macau residents with depression.
1. Introduction
Since COVID-19 was first reported in China in early 2020, approximately 600 million people had been infected globally as of the end of 2022 (World Health Organization, 2022). Located on the west of the Pearl River Delta estuary in southern China, Macau is a Special Administrative Region of China (Macau SAR) with a different political and economic system from mainland China. However, Macao adopted a similar COVID management strategy as mainland China (Chen et al., 2023), since the local economy relies heavily on tourism and gambling revenues from mainland China (McCartney, 2021). The latest surge of the COVID-19 infections occurred from June 18 to August 7, 2022 in Macau, known as the “618 COVID-19 wave” (Portal do Governo da Região Administrativa Especial de Macau da República Popular da China, 2022), which caused 1,816 confirmed cases and 6 deaths (Exmoo News, 2022; Macao Daily, 2022d). To prevent the spread of the virus, the quarantine-free border policy between Zhuhai and Macau was suspended, while in Macau the territory lockdown measures were implemented (Radio Television Hong Kong, 2022; Macao Daily, 2022e). From July 11 to July 23, 2022 Macau adopted a “relative standstill” policy; i.e., all businesses and industry activities (including casinos and construction projects) were suspended (Macao Daily, 2022a,b,c). Following the COVID strategy in mainland China, Macau government implemented a Dynamic Zero-COVID policy (Zhao et al., 2022a) and adopted stringent health management and quarantine measures when necessary, which might increase the risk of mental health problems (Zhong et al., 2020; Bai et al., 2022a) such as depressive symptoms (depression hereafter) (Xiong et al., 2020).
Depression is one of the most common mental health problems, especially during the COVID-19 pandemic. Previous studies found that the prevalence of depression were 38.5% (95% CI: 35.5%–41.5%) among Macau residents (Bai et al., 2022b) and 35.2% (95% CI, 32.2–38.3%) among Chinese college students (Li et al., 2020) in the early stage of the pandemic. In contrast, several studies before the COVID-19 pandemic on the epidemiology of depression in Macau found that the prevalence rate was 8.0% in the general population (Hall et al., 2017), 8.6% in older men (Chan and Zeng, 2011) and 11.9% in older women (Chan and Zeng, 2009). Depression is associated with a number of negative outcomes such as impaired functioning (Geiselman and Bauer, 2000; Wieman et al., 2022), lowered quality of life (QoL) (Bertha and Balázs, 2013), and an increased risk of suicidality (Hegerl, 2016; Chen et al., 2022). In order to facilitate health resource allocation and develop appropriate preventive strategies to reduce the likelihood of depression and its negative outcomes, it is important to understand the epidemiology and associated factors of depression.
Most research on depression was traditionally based on the common cause framework (Cramer et al., 2010; Schmittmann et al., 2013; Fried, 2015), in which all symptoms originate from the underlying disease (Lux and Kendler, 2010; Fried, 2015) and all symptoms are clinically equivalent and interchangeable (Cramer et al., 2010; Lux and Kendler, 2010). However, the complexities and ongoing interaction between different depressive symptoms could not be examined from such perspective (Marchetti, 2019; Mullarkey et al., 2019).
In recent years, the network approach has been a novel approach that could conceptualize psychological phenomena (Borsboom and Cramer, 2013). According to network theory, depressive states may result from the co-occurrence of depressive symptoms and the interactions without latent factors (Borsboom, 2008; Borsboom and Cramer, 2013; Santos et al., 2017). Individual psychiatric symptoms are viewed as nodes, while the associations between nodes are viewed as edges in a network model (Epskamp et al., 2012). Network analysis could help determine nodes that are the most central (influential) in the network model and could be targeted for prevention and intervention (Borsboom and Cramer, 2013; Cramer et al., 2016; Marchetti, 2019). In the past years network analysis has been widely applied in different populations during the COVID-19 pandemic such as adolescents (Cai et al., 2022a), the general population (Cheung et al., 2021; Zavlis et al., 2021), older people (Jin et al., 2022), college students (Bai et al., 2021), clinicians (Cai et al., 2022c), and psychiatric patients (Kim et al., 2022). Evidence showed that the pattern and clinical features of depression were highly dependent on the socioeconomic context (Kleinman, 2004; Compton et al., 2006); therefore, the network structure of the depressive symptoms should be examined separately for the population living in areas with different socioeconomic contexts (Cheung et al., 2021). However, no network analysis of depression in Macau residents during the 618 COVID-19 wave has been published.
This study investigated the prevalence and the related factors of depression among Macau residents during the 618 COVID-19 wave, examined the central symptoms of the depression network model, and explored the association between depressive symptoms and QoL. We hypothesized that depression would be common and negatively associated with QoL among Macau residents in this wave.
2. 2. Methods
2.1. Participants and procedure
This was a cross-sectional study conducted between 26th July 2022 and 9th September 2022 using snowball sampling method. A Quick Response code (QR code) linked with the invitation and the study assessment was distributed via major social media platforms including WeChat, Facebook and Instagram in Macau. To be eligible, participants met the following inclusion criteria: (1) aged 18 years or above, and able to understand the purpose and content of the assessments; (2) Macau residents living in Macau during the 618 COVID-19 wave. There were no exclusion criteria in this study. Due to the risk of COVID-19 infection, face-to-face assessments were not adopted. Following other studies (Bai et al., 2022b; Cai et al., 2022b) the “Questionnaire Star” program was conducted in data collection. The participants provided online written informed consent on a voluntary and confidential basis. The study protocol was approved by the Institutional Review Board (IRB) of the University of Macau.
2.2. Measures
Socio-demographic information, such as age, gender, marital status, education level, employment status, and monthly income during the COVID-19 wave, was collected. Variables related to COVID-19 were also measured, including the level of economic loss caused by the COVID-19 wave, concerns about the COVID-19 pandemic, being quarantined, and having COVID-19 infection, fatigue, and regular physical exercise during the wave. In addition, the presence of chronic physical diseases, history of psychiatric disorders, and suicidality during the COVID-19 wave were also collected.
The validated Chinese version of the 9-item Patient Health Questionnaire (PHQ-9) was used to measure the presence and severity of depressive symptoms (Kroenke et al., 2001; Chen et al., 2015), which consisted of nine dimensions: including (1) Anhedonia; (2) Sad Mood; (3) Sleep; (4) Fatigue; (5) Appetite; (6) Guilt; (7) Concentration; (8) Motor disturbances; and (9) Suicide. The PHQ-9 items are developed based on the Major depressive disorder criteria from the Diagnostic and Statistical Manual of Mental Disorders-IV (DSM-IV). Each item ranges from 0 (not at all) to 3 (nearly every day), with the total score ranging between 0 and 27. Following the previous study of Wang et al. (2014), a PHQ-9 total score of ≥5 was considered “having depressive symptoms”; specifically, a total score of 5, 10, 15, and 20 were used as the cut-off values for having mild depressive symptoms”, “moderate depressive symptoms”, “moderately severe depressive symptoms” and “severe depressive symptoms respectively (Kroenke et al., 2001). The psychometric properties of PHQ-9 Chinese version in Chinese populations are considered satisfactory.
The severity of anxiety was assessed using the validated Chinese version of the seven-item Generalized Anxiety Disorder scale (GAD-7) (Spitzer et al., 2006; He et al., 2010). The GAD-7 comprises 7 items with each scored from 0 (not at all) to 3 (nearly every day), and the total score ranges from 0 to 21 with higher scores indicating more severe anxiety symptoms. Global quality of life (QoL) was measured with the total score of the first two items of the World Health Organization Quality of Life-brief version (WHOQOL-BREF) (The WHOQOL Group, 1998; Skevington et al., 2004), with higher total scores indicating higher QoL. The Chinese version of the WHOQOL-BREF has been validated in Chinese populations (Hao et al., 2006; Xia et al., 2012).
2.3. Statistical analysis
2.3.1. Univariate and multivariate analyses
SPSS version 26.0 (SPSS Inc., Chicago, Illinois, USA) was used to conduct univariate and multivariate analyses. Continuous variables were tested for normal distributions using Kolmogorov–Smirnov tests and Q-Q plots. Socio-demographic and clinical data, and COVID-19-related variables between “with depression” and “without depression” groups were analyzed using Chi-square test, Student’s t-test or Mann–Whitney U tests, as appropriate. Analysis of covariance (ANCOVA) was used to compare QoL between “with depression” and “without depression” groups, after controlling for variables with significant differences in univariate analysis. Binary logistic regression analysis with the “Enter” method was used to test independent correlates of depression. Those with significant group differences in univariate analyses were entered as independent variables, while having depression was the dependent variable. The significance level was set at p < 0.05 for all tests (two-tailed).
2.3.2. Network structure
The R software (R Core Team, 2013) was used to conduct the network analysis. In the network model, each symptom is represented as a node, and the association between two nodes is represented as an edge (Beard et al., 2016). The estimate and visualization of the network were performed using the R-packages “qgraph” (Version 1.6.5) (Epskamp et al., 2012) and “bootnet” (Version 1.4.3) (Epskamp et al., 2018). Edges in the network were shrunk and relevant tuning parameters were selected using the least absolute shrinkage and selection operator (LASSO) and extended Bayesian information criteria (EBIC) to make the symptom network sparser and simpler to understand (Epskamp et al., 2018), which could improve the accuracy of the prediction, sparse, and interpretability of the network model (Yang et al., 2022). The correlation between two nodes in green color indicated positive correlations, while red color indicated negative correlations, with thicker edges representing stronger correlations. To determine central (influential) symptoms in the network, expected Influence (EI) as a reliable centrality index was adopted (Robinaugh et al., 2016). The predictability of each node was estimated using the R package “mgm” (Haslbeck and Waldorp, 2015), which is defined as the variance of a node explained by all other nodes in the network model. Further, the “flow” function in R package “qgraph” was conducted to identify individual depressive symptoms that were directly associated with QoL (Epskamp et al., 2012).
To evaluate the robustness of the results, the stability and accuracy of the network were evaluated using the R package “bootnet” (version 1.4.3) (Epskamp et al., 2018). Case-dropping bootstrap was used to estimate the stability of the network. The network was deemed stable if samples could be removed from the dataset without causing significant changes in the node’s centrality index. Stability was graphically represented and quantified by calculating the Correlation Stability Coefficient (CS-C), with moderate stability indicated by values higher than 0.25, while strong stability indicated by values larger than 0.5 (Koo and Li, 2016). Bootstrapped 95% confidence intervals (Cis) were used to measure edge accuracy, with a narrower CI indicating a more reliable network (Epskamp et al., 2018).
3. Results
3.1. Participant characteristics
Of a total of 1,020 Macau residents invited to participate in this study, 1,008 (739 female, 269 male) met the study entry criteria and were included in this study, giving a participation rate of 98.8%. The mean age of participants were 34.85 (SD = 11.5) years, while 831 (82.4%) had high education (i.e., undergraduate/college or above), 687 (68.2%) were employed during the COVID-19 wave, 471 (46.7%) were married, and 935 (92.8%) lived with others.
3.2. Prevalence and correlates
The overall prevalence of depression (PHQ-9 total score ≥ 5) was 62.5% (n = 630; 95% CI = 60.00–65.00%); specifically, 343 (34.0%; 95% CI = 31.10–36.96%) had mild depression, while 163 (16.2%; 95% CI = 13.89–18.45%) had moderate depression 74 (7.3%; 95% CI = 5.73–8.95%) had moderately severe depression, and 50 (5.0%; 95% CI = 3.62–6.30%) had severe depression. Table 1 summarizes the demographic characteristics of participants with depression and those without depression.
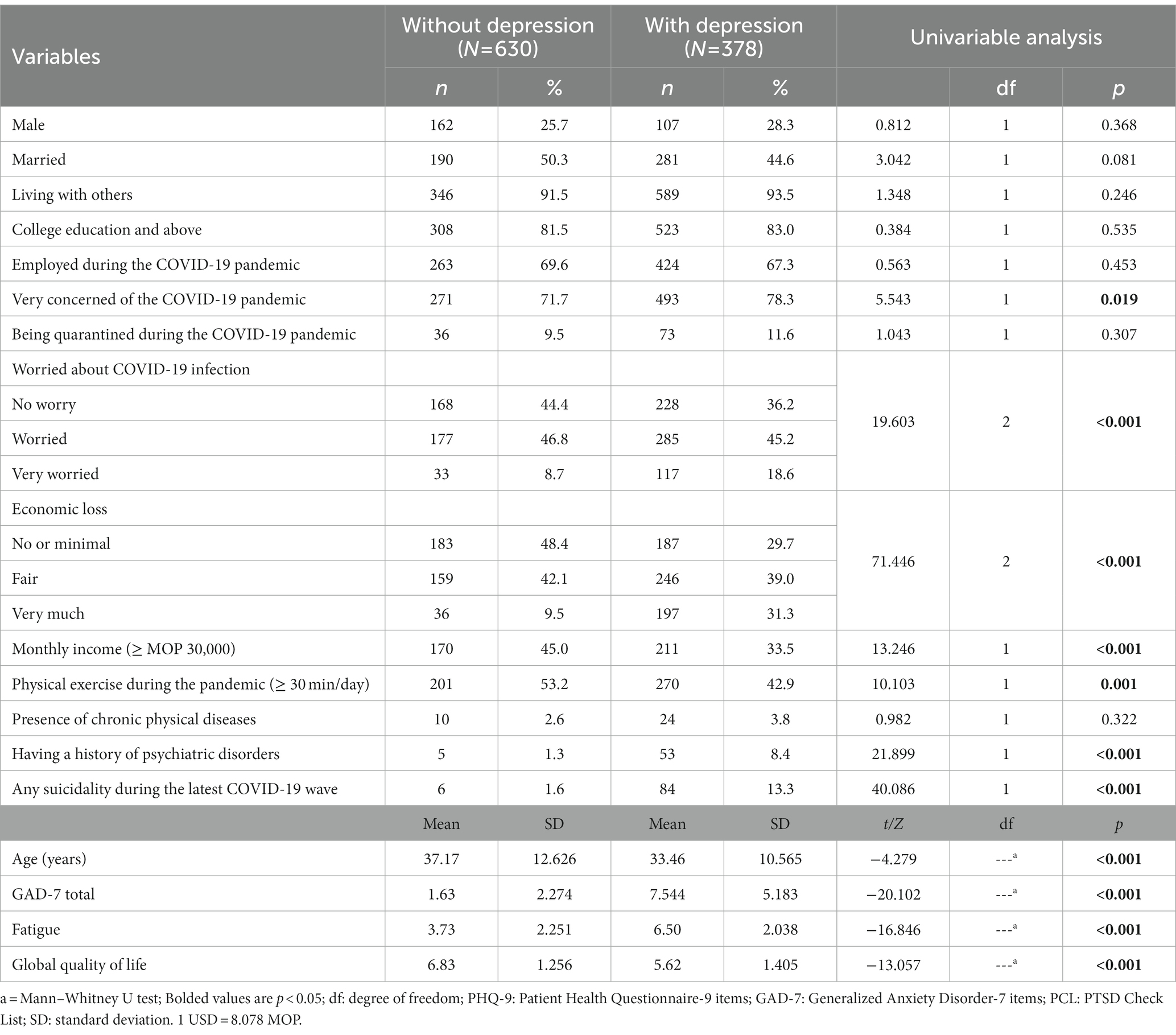
Table 1. Comparison between Macau residents with and without depressive symptoms with respect to demographic and clinical variables.
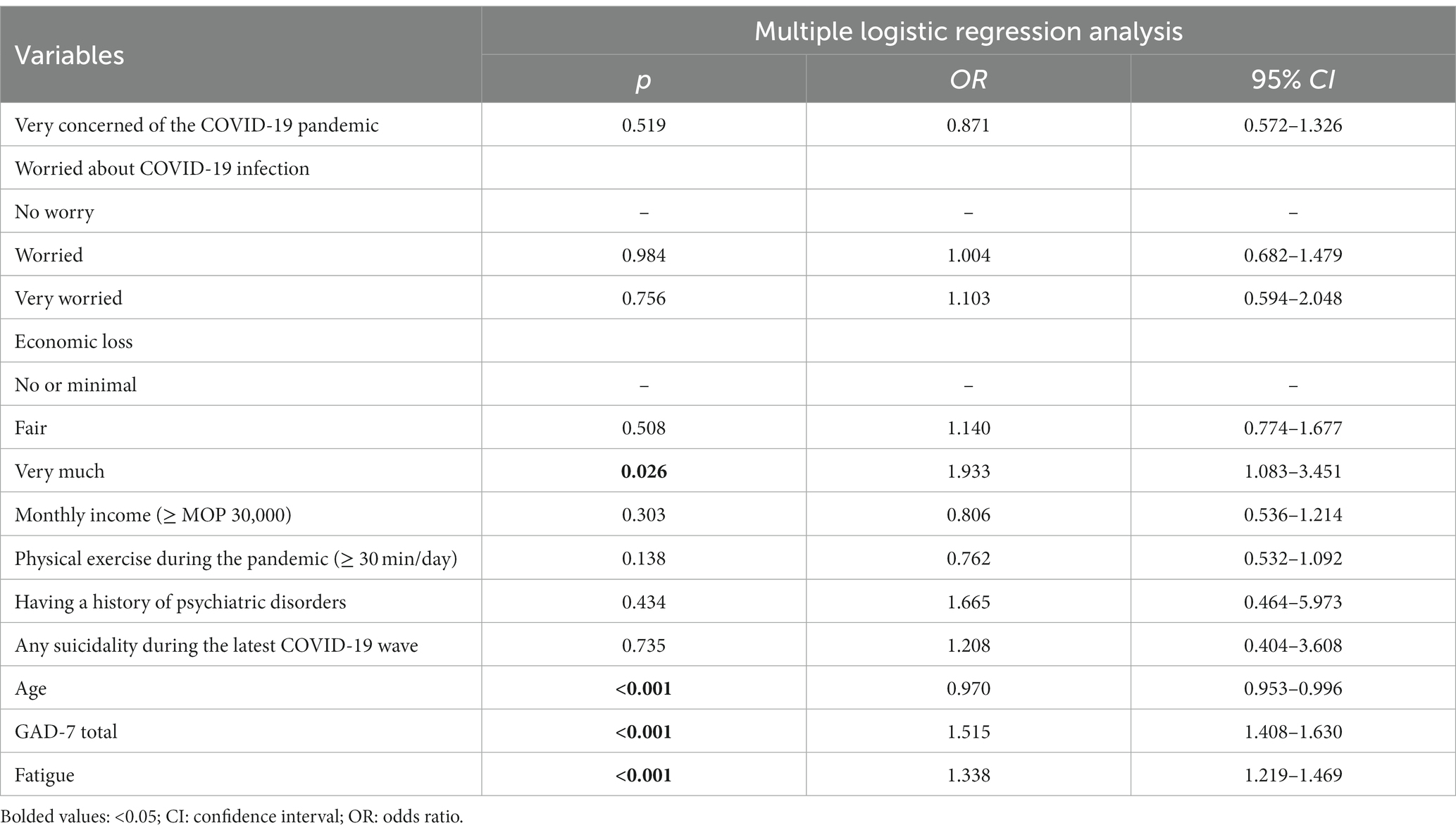
Table 2. Independent correlates of depressive symptoms among Macau residents during the 618 COVID-19 wave (N = 1,008).
Univariable analyses revealed that residents with depressive symptoms were more likely to be concerned about COVID-19 pandemic (p = 0.019), more worried about having COVID-19 infection (p < 0.001), had more economic loss during the COVID-19 wave (p < 0.001), had a history of psychiatric disorders (p < 0.001), and had suicidality during the COVID-19 wave (p < 0.001). Compared with those without depression, those with depression were less likely to have a higher monthly income (≥MOP30,000) (p < 0.001), and physical exercise 30 min or above every day during the pandemic (p = 0.001). Furthermore, the residents with depression were more likely to report a higher total score of GAD-7 (p < 0.001), fatigue (p < 0.001), and a lower QoL (p < 0.001). After controlling for variables with significant group differences in univariate analyses, residents with depression still had lower QOL (F (1, 1,008) = 5.538, p = 0.019) compared to those without depression. Binary logistic regression analysis revealed that participants with depression were more likely to be younger (OR = 0.97;
3.3. Network structure of depressive symptoms
The network structure of depressive symptoms as measured by PHQ items is shown in Figure 1. PHQ2 (“Sad Mood”; EI: 1.044), PHQ4 (“Fatigue,” EI: 1.016), and PHQ6 (“Guilt”; EI: 0.975) were the top three nodes with the highest EI. The mean predictability was 0.535, indicating that on average 53.5% of the variance for each node could be explained by neighboring nodes in the model. Supplementary Table S1 provides the descriptive details and network centrality indices for each depressive symptom. Figure 2 presents the results of flow network model showing that PHQ4 (“Fatigue”; average edge weight = −0.1162), PHQ9 (“Suicide”; average edge weight = −0.1029), and PHQ6 (“Guilt”; average edge weight = −0.0981) had strong negative associations with QoL.
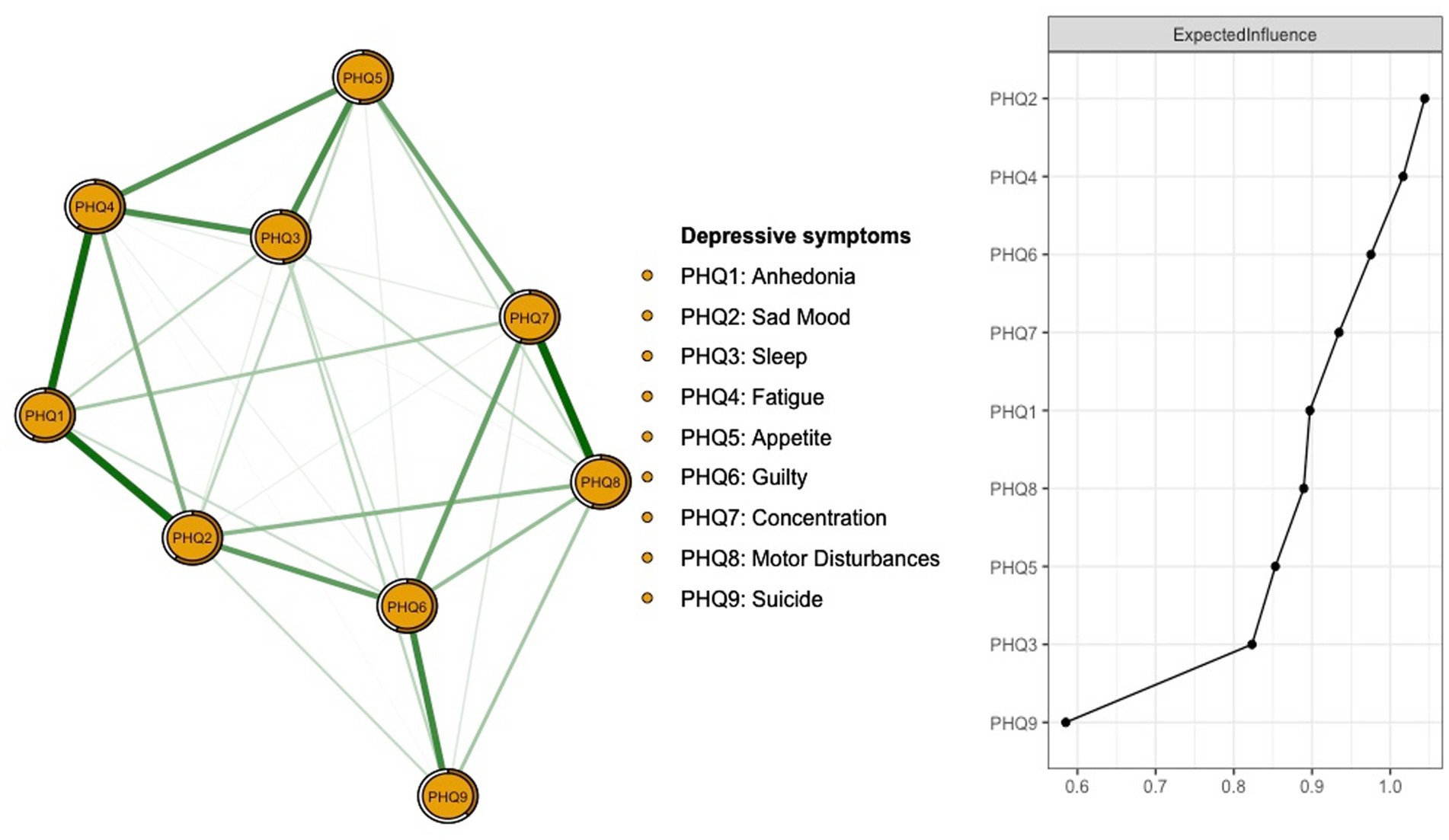
Figure 1. Network structure of depressive symptoms among Macau residents during the 618 COVID-19 wave. Number of nodes: 9; Number of non-zero edges:35/36; Mean weight: 0.111.
Figure 3 shows the stability of the network. Based on the case-dropping bootstrap procedure, the CS-C of EI was 0.75, showing that the network model was very stable, which indicates that 75% of the sample could be dropped and the structure of the network would not significantly change (Figure 3). As shown in Supplementary Figure S1, the bootstrap 95% CI for estimating edge weights for the accuracy of the network indicated a limited range, and most of the edge weights were non-zero, indicating that most of the edges were stable and accurate. These comparisons were statistically significant based on a bootstrap difference test, showing the network model’s reliability (Supplementary Figure S2).
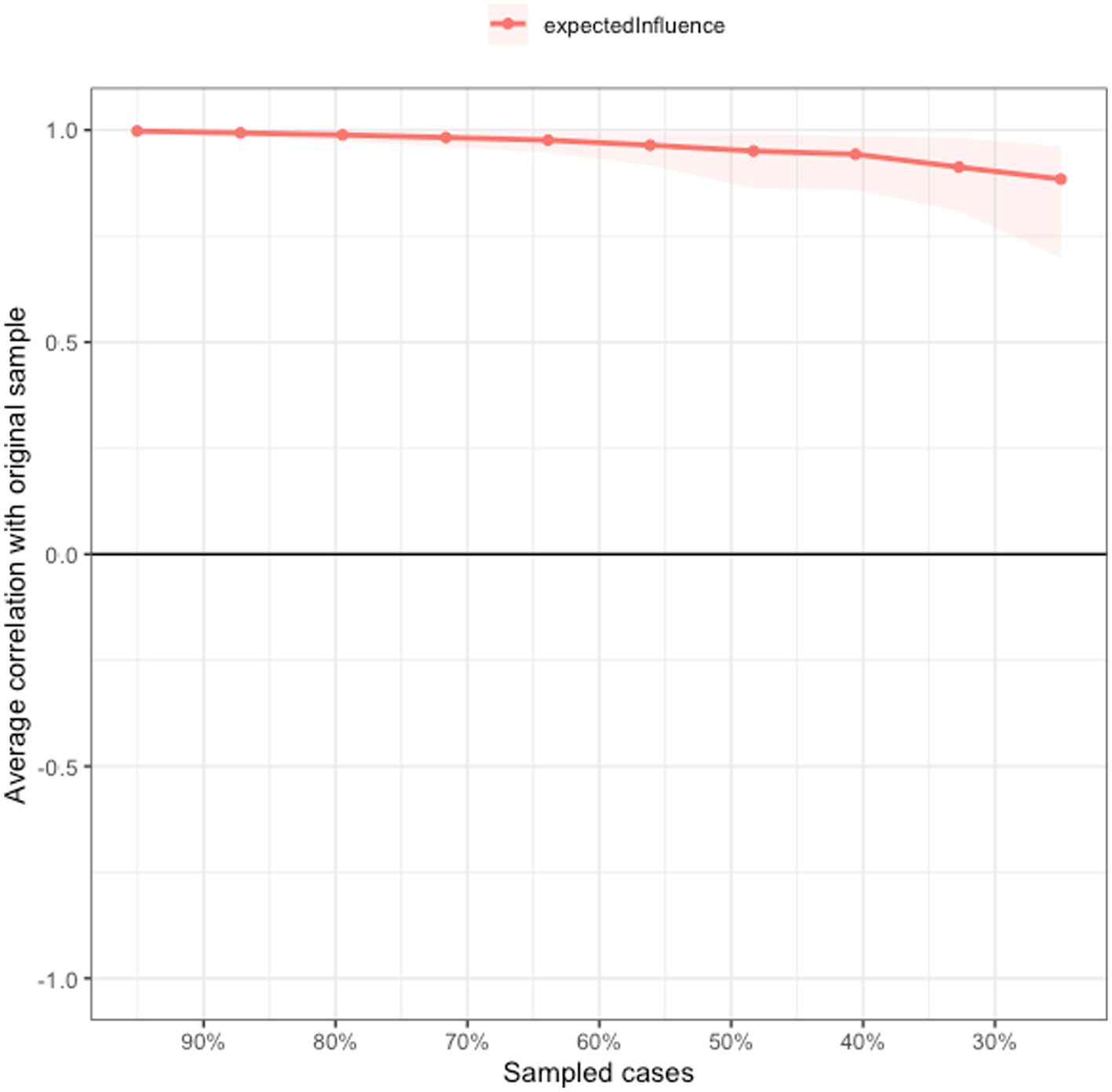
Figure 3. Network stability of depressive symptoms among Macau residents during the 618 COVID-19 wave.
4. Discussion
This was the first study to explore the prevalence, related factors, and network structure of depression as well as its relationship with QoL of among Macau residents shortly after the 618 COVID-19 wave.
The prevalence of depressive symptoms (PHQ-9 total score ≥ 5) was 62.5% (95% CI = 60.0–65.0%) in Macau residents during the 618 COVID-19 wave, which was much higher than the corresponding figures (38.5%; 95% CI = 35.5%–41.5%) during the first wave of the COVID-19 infections in Macau (Bai et al., 2022b) and in most other areas such as the general population in mainland China (37.1%) (Ahmed et al., 2020), in Italy (32.8%) (Mazza et al., 2020) and in Hong Kong (48.8%) (Choi et al., 2020). The higher infection and death cases together with the more stringent public health measures during the 618 COVID-19 wave compared to the first wave of the COVID-19 infections in Macau could explain the higher prevalence of depression. Previous research showed that strict quarantine measures, social distancing, and isolation (Xiao, 2020; Ustun, 2021) could increase the risk of mental health problems including depression (Xiao, 2020).
More severe anxiety was associated with higher risk of depression in this study. During the 618 COVID-19 wave, mass media in Macau continuously reported the latest news about the wave, which caused stress for many people. High anxiety levels were associated with being constantly exposed to COVID-19 news (Moghanibashi-Mansourieh, 2020; WHO, 2020; Yu et al., 2023). A meta-analysis by Jacobson and Newman (2017) found that anxiety was closely associated with depression, and anxiety could often predict depression. We also found that participants who experienced a heavier economic loss during the COVID-19 wave were more likely to suffer from depressive symptoms, which is consistent with previous findings (Hertz-Palmor et al., 2020; Argabright et al., 2022). People who were economically disadvantaged were more likely to experience financial insecurity, unhealthy lifestyles, poor living conditions, and reduced social capital (Lund and Cois, 2018; Jin et al., 2020; Ding et al., 2022). All these factors might lead to a higher risk of depression. In addition, people with limited financial resources usually worry more about their financial insecurity, which could also lead to depression (Asebedo and Wilmarth, 2017).
Severe fatigue is another associated risk factor of depression. Compared with previous waves of the COVID-19 infections, the 618 COVID-19 wave lasted longer and had more stringent measures such as repeated nucleic acid testing, which could lead to subjective fatigue among residents. This might also be physiologically related due to increased stress hormones released in people in response to the rapidly growing 618 COVID-19 wave (Haktanir et al., 2022), which might result in fatigue over time. According to the psychological resource theory (Hobfoll, 2002) and the cognitive load theory (Sweller, 1988), people who experience fatigue with limited psychological resources may be less resilient to stress-related symptoms when subjected to ongoing negative mental or emotional states, which might, in turn, increase the risk of depression (Barsevick et al., 2006; Lee and Kim, 2006).
This study found a lower risk of depression (e.g., lower PHQ-9 scores) among older Macau residents, which is consistent with the findings of previous studies (Nwachukwu et al., 2020; García-Portilla et al., 2021). This might be attributed to the development of resilience or successful adaption to difficult or challenging life experiences, which is a personal characteristic that could be enhanced through practice (Southwick et al., 2014). Due to more exposure to stressful experiences compared to their young counterparts, older adults are usually more resilient (Silva Júnior et al., 2019; García-Portilla et al., 2021), particularly in terms of emotional regulation and problem-solving (Gooding et al., 2012). Hence, resilience in older adults are usually associated with positive outcomes, including adaptive coping, optimism, and a reduced risk of depression (Gooding et al., 2012; MacLeod et al., 2016).
“Sad mood” (PHQ2) was the most central symptom in the depression network model among Macau residents during the 618 COVID-19 wave, which is consistent with the previous findings in a German general population study (Hartung et al., 2019) and in a study of older adults in Hong Kong (Jin et al., 2022) during the COVID-19 pandemic. According to the Diagnostic and Statistical Manual of Mental Disorder-5 (DSM-5) (APA, 2013), “sad mood” is a core symptom of major depressive disorder (MDD), and persistent sadness is also a risk factor for depression (Wolff, 1999; Davidson and Henriques, 2000). Sadness was likely a normal and common reaction to a loss, disappointment, problem, or other difficult situations during the 618 COVID-19 wave.
Characterized by emotional tiredness, an inability to work efficiently, loss of motivation, difficulty falling asleep, helplessness, and resentment (Queen and Harding, 2020; Tanhan et al., 2020; Vindegaard and Benros, 2020), “Fatigue” (PHQ4) was also a central depressive symptom in the network model. This finding is consistent with the results of a study of college students during the late stage of the COVID-19 pandemic (Bai et al., 2021). Moreover, “guilt” (PHQ6) was another central symptom in the model among Macau residents, which is consistent with the findings among Hong Kong (Cheung et al., 2021) and Wuhan residents during the COVID-19 pandemic (Zhao et al., 2021). Guilt may manifest as helplessness, hopelessness, worthlessness, powerlessness, poor self-esteem, and self-doubt (Bademci et al., 2016; Gambin and Sharp, 2018), which may lead to negative outcomes such as a reduction in motivation and self-care (Luck and Luck-Sikorski, 2021) as well as depression. Further, guilt is not only closely related to grief, but also to suicidal thoughts (Jeon et al., 2014).
In the flow network model of QoL and depression, the top three symptoms that negatively correlated with QoL included “Fatigue” (PHQ4), “Suicide” (PHQ9), and “Guilt” (PHQ6). During the 618 COVID-19 wave, fatigue might be caused by lifestyle changes due to the lockdown measures and decreased outdoor physical activities (McIlvenny et al., 2000; Booth et al., 2012; Zhao et al., 2022b). As such, adequate physical exercise could improve physical performance and increase QOL (Mehnert et al., 2011; Jakobsen et al., 2017; Dauwan et al., 2021). Suicidality was another symptom which negatively correlated with QoL, which is expected since many people became vulnerable to mental health problems and suicidality during the pandemic (Gunnell et al., 2020; Luo et al., 2021). Loss of employment and financial stress during the pandemic are identified as risk factors for lowered QoL (Stuckler et al., 2009; Kokaliari, 2018; Norström et al., 2019). To improve QoL, the government needs to provide financial support (Gunnell et al., 2020), and maintain an active labor market program when necessary (Stuckler et al., 2009). Moreover, the symptom “guilt” (PHQ6) was also negatively associated with QOL in the network model. Untreated guilt caused by stressful events might be associated with severe mental health problems, which could lower QoL (Griffin et al., 2019; Cavalera, 2020). Previous studies found that sudden changes in the lifestyle of individuals due to social distancing and lockdown during COVID-19 pandemic increased feelings of guilt (Brooks et al., 2020; Cavalera, 2020; Sahoo et al., 2020), which could lower QoL (Tilghman-Osborne et al., 2012; Luck and Luck-Sikorski, 2021).
The strengths of this study included the relatively large sample size and the use of network analysis to explore the network structures of depression and the correlation between depression and QoL. However, this study has several limitations. First, this was a cross-sectional study; therefore, causal relationships between QoL and depressive symptoms could not be inferred. Second, the PHQ-9 is a self-report measure of depressive symptoms rather than a clinical interview assessment or diagnosis. The possibility of recall bias could not be excluded. Third, this study was conducted in Macau, hence the findings could not be generalized to other areas. Fourth, for logistical reasons, random sampling was not used in this study, which might bias the representativeness of the study sample to an uncertain extent.
In conclusion, depression was common among Macao residents during the 618 COVID-19 wave, particularly among older residents and those who had more severe anxiety, fatigue and economic loss. Due to the negative impact of depression on QoL, interventions targeting central symptoms (e.g., “Sad Mood”, “Fatigue”, “Guilt” and “Suicide”) identified in the network model should be developed and implemented for depressed Macau residents, such as the provision of public education, Internet counseling, and increased physical activity.
Data availability statement
The datasets presented in this article are not readily available because the Institutional Review Board (IRB) of the University of Macau that approved the study prohibits the authors from disseminating the research dataset of clinical studies publicly. Requests to access the datasets should be directed to eHl1dGx5QGdtYWlsLmNvbQ==.
Ethics statement
The studies involving human participants were reviewed and approved by Institutional Review Board (IRB) of the University of Macau. The patients/participants provided their written informed consent to participate in this study.
Author contributions
LZ, YF, SS, and Y-TX study design. TS, PC, ML, K-IL, IC, J-XL, Y-YW, ZS, TC, and GU data collection, analysis, and interpretation. TS, PC, and Y-TX drafting of the manuscript. GU and CN critical revision of the manuscript. All authors contributed to the article and approved the submitted version.
Funding
The study was supported by the National Science and Technology Major Project for investigational new drug (2018ZX09201-014), the Beijing Hospitals Authority Clinical Medicine Development of special funding support (XMLX202128), and the University of Macau (MYRG2019-00066-FHS and MYRG2022-00187-FHS).
Acknowledgments
The authors are grateful to all participants and clinicians involved in this study.
Conflict of interest
The authors declare that the research was conducted in the absence of any commercial or financial relationships that could be construed as a potential conflict of interest.
Publisher’s note
All claims expressed in this article are solely those of the authors and do not necessarily represent those of their affiliated organizations, or those of the publisher, the editors and the reviewers. Any product that may be evaluated in this article, or claim that may be made by its manufacturer, is not guaranteed or endorsed by the publisher.
Supplementary material
The Supplementary material for this article can be found online at: https://www.frontiersin.org/articles/10.3389/fpsyg.2023.1164232/full#supplementary-material
References
Ahmed, M. Z., Ahmed, O., Aibao, Z., Hanbin, S., Siyu, L., and Ahmad, A. (2020). Epidemic of COVID-19 in China and associated psychological problems. Asian J. Psychiatr. 51:102092. doi: 10.1016/j.ajp.2020.102092
APA (2013). Diagnostic and statistical manual of mental disorders: DSM-5™. Washington, DC: American Psychiatric Publishing, a division of American Psychiatric Association.
Argabright, S. T., Tran, K. T., Visoki, E., DiDomenico, G. E., Moore, T. M., and Barzilay, R. (2022). COVID-19-related financial strain and adolescent mental health. Lancet Reg. Health Am. 16:100391. doi: 10.1016/j.lana.2022.100391
Asebedo, S. D., and Wilmarth, M. J. (2017). Does how we feel about financial strain matter for mental health? J. Financ. Ther. 8:5. doi: 10.4148/1944-9771.1130
Bademci, H. Ö., Karadayı, E. F., Pur Karabulut, İ. G., and Warfa, N. (2016). Who is the helper? Who is being helped? The benefits of psychosocial support to correctional officers in Turkey. Psychodyn. Pract. 22, 351–365. doi: 10.1080/14753634.2016.1234946
Bai, W., Cai, H., Liu, S., Chen, X., Sha, S., Cheung, T., et al. (2021). Anxiety and depressive symptoms in college students during the late stage of the COVID-19 outbreak: a network approach. Transl. Psychiatry 11, 638–637. doi: 10.1038/s41398-021-01738-4
Bai, W., Sha, S., Cheung, T., Su, Z., Jackson, T., and Xiang, Y. T. (2022a). Optimizing the dynamic zero-COVID policy in China. Int. J. Biol. Sci. 18, 5314–5316. doi: 10.7150/ijbs.75699
Bai, W., Zhao, Y. J., Cai, H., Sha, S., Zhang, Q., Lei, S. M., et al. (2022b). Network analysis of depression, anxiety, insomnia and quality of life among Macau residents during the COVID-19 pandemic. J. Affect. Disord. 311, 181–188. doi: 10.1016/j.jad.2022.05.061
Barsevick, A. M., Dudley, W. N., and Beck, S. L. (2006). Cancer-related fatigue, depressive symptoms, and functional status: a mediation model. Nurs. Res. 55, 366–372. doi: 10.1097/00006199-200609000-00009
Beard, C., Millner, A. J., Forgeard, M. J., Fried, E. I., Hsu, K. J., Treadway, M., et al. (2016). Network analysis of depression and anxiety symptom relationships in a psychiatric sample. Psychol. Med. 46, 3359–3369. doi: 10.1017/S0033291716002300
Bertha, E. A., and Balázs, J. (2013). Subthreshold depression in adolescence: a systematic review. Eur. Child Adolesc. Psychiatry 22, 589–603. doi: 10.1007/s00787-013-0411-0
Booth, F. W., Roberts, C. K., and Laye, M. J. (2012). Lack of exercise is a major cause of chronic diseases. Compr. Physiol. 2, 1143–1211. doi: 10.1002/cphy.c110025
Borsboom, D. (2008). Psychometric perspectives on diagnostic systems. J. Clin. Psychol. 64, 1089–1108. doi: 10.1002/jclp.20503
Borsboom, D., and Cramer, A. O. J. (2013). Network analysis: an integrative approach to the structure of psychopathology. Annu. Rev. Clin. Psychol. 9, 91–121. doi: 10.1146/annurev-clinpsy-050212-185608
Brooks, S. K., Webster, R. K., Smith, L. E., Woodland, L., Wessely, S., Greenberg, N., et al. (2020). The psychological impact of quarantine and how to reduce it: rapid review of the evidence. Lancet 395, 912–920. doi: 10.1016/S0140-6736(20)30460-8
Cai, H., Bai, W., Liu, H., Chen, X., Qi, H., Liu, R., et al. (2022a). Network analysis of depressive and anxiety symptoms in adolescents during the later stage of the COVID-19 pandemic. Transl. Psychiatry 12:98. doi: 10.1038/s41398-022-01838-9
Cai, H., Bai, W., Sha, S., Zhang, L., Chow, I. H. I., Lei, S. M., et al. (2022b). Identification of central symptoms in internet addictions and depression among adolescents in Macau: a network analysis. J. Affect. Disord. 302, 415–423. doi: 10.1016/j.jad.2022.01.068
Cai, H., Zhao, Y.-J., Xing, X., Tian, T., Qian, W., Liang, S., et al. (2022c). Network analysis of comorbid anxiety and insomnia among clinicians with depressive symptoms during the late stage of the COVID-19 pandemic: a cross-sectional study. Nat Sci. Sleep 14, 1351–1362. doi: 10.2147/NSS.S367974
Cavalera, C. (2020). COVID-19 psychological implications: the role of shame and guilt. Front. Psychol. 11:571828. doi: 10.3389/fpsyg.2020.571828
Chan, M. F., and Zeng, W. (2009). Investigating factors associated with depression of older women in Macau. J. Clin. Nurs. 18, 2969–2977. doi: 10.1111/j.1365-2702.2009.02867.x
Chan, M. F., and Zeng, W. (2011). Exploring risk factors for depression among older men residing in Macau. J. Clin. Nurs. 20, 2645–2654. doi: 10.1111/j.1365-2702.2010.03689.x
Chen, W. C., Chen, S. J., and Zhong, B. L. (2022). Sense of alienation and its associations with depressive symptoms and poor sleep quality in older adults who experienced the lockdown in Wuhan, China, during the COVID-19 pandemic. J. Geriatr. Psychiatry Neurol. 35, 215–222. doi: 10.1177/08919887221078564
Chen, M., Sheng, L., and Qu, S. (2015). Diagnostic test of screening depressive disorders in general hospital with the patient health questionnaire. Chin. Ment. Health J., 241–245.
Chen, P., Zhang, L., Sha, S., Lam, M. I., Lok, K. I., Chow, I. H. I., et al. (2023). Prevalence of insomnia and its association with quality of life among Macau residents shortly after the summer 2022 COVID-19 outbreak: a network analysis perspective. Front. Psych. 14:1113122. doi: 10.3389/fpsyt.2023.1113122
Cheung, T., Jin, Y., Lam, S., Su, Z., Hall, B. J., Xiang, Y.-T., et al. (2021). Network analysis of depressive symptoms in Hong Kong residents during the COVID-19 pandemic. Transl. Psychiatry 11:460. doi: 10.1038/s41398-021-01543-z
Choi, E. P. H., Hui, B. P. H., and Wan, E. Y. F. (2020). Depression and anxiety in Hong Kong during COVID-19. Int. J. Environ. Res. Public Health 17:3740. doi: 10.3390/ijerph17103740
Compton, W. M., Conway, K. P., Stinson, F. S., and Grant, B. F. (2006). Changes in the prevalence of major depression and comorbid substance use disorders in the United States between 1991–1992 and 2001–2002. Am. J. Psychiatr. 163, 2141–2147. doi: 10.1176/ajp.2006.163.12.2141
Cramer, A. O. J., van Borkulo, C. D., Giltay, E. J., van der Maas, H. L. J., Kendler, K. S., Scheffer, M., et al. (2016). Major depression as a complex dynamic system. PLoS One 11:e0167490. doi: 10.1371/journal.pone.0167490
Cramer, A. O., Waldorp, L. J., Van Der Maas, H. L., and Borsboom, D. (2010). Comorbidity: a network perspective. Behav. Brain Sci. 33, 137–150. doi: 10.1017/S0140525X09991567
Dauwan, M., Begemann, M. J., Slot, M. I., Lee, E. H., Scheltens, P., and Sommer, I. E. (2021). Physical exercise improves quality of life, depressive symptoms, and cognition across chronic brain disorders: a transdiagnostic systematic review and meta-analysis of randomized controlled trials. J. Neurol. 268, 1222–1246. doi: 10.1007/s00415-019-09493-9
Davidson, R. J., and Henriques, J. (2000). “Regional brain function in sadness and depression” in The neuropsychology of emotion. ed. J. C. Borod (Oxford, United Kingdom: Oxford University Press), 269–297.
Ding, K.-R., Wang, S.-B., Xu, W.-Q., Lin, L.-H., Liao, D.-D., Chen, H.-B., et al. (2022). Low mental health literacy and its association with depression, anxiety and poor sleep quality in Chinese elderly. Asia Pac. Psychiatry 14:e12520. doi: 10.1111/appy.12520
Epskamp, S., Borsboom, D., and Fried, E. I. (2018). Estimating psychological networks and their accuracy: a tutorial paper. Behav. Res. Methods 50, 195–212. doi: 10.3758/s13428-017-0862-1
Epskamp, S., Cramer, A. O., Waldorp, L. J., Schmittmann, V. D., and Borsboom, D. (2012). Qgraph: network visualizations of relationships in psychometric data. J. Stat. Softw. 48, 1–18. doi: 10.18637/jss.v048.i04
Exmoo News (2022). A total of 1,816 cases, 38% of whom have symptoms, Macao's social situation has been “cleared” for three consecutive days. Macao: Available online: https://www.exmoo.com/article/203463.html
Fried, E. I. (2015). Problematic assumptions have slowed down depression research: why symptoms, not syndromes are the way forward. Front. Psychol. 6:309. doi: 10.3389/fpsyg.2015.00309
Gambin, M., and Sharp, C. (2018). The relations between empathy, guilt, shame and depression in inpatient adolescents. J. Affect. Disord. 241, 381–387. doi: 10.1016/j.jad.2018.08.068
García-Portilla, P., de la Fuente Tomás, L., Bobes-Bascarán, T., Jiménez Treviño, L., Zurrón Madera, P., Suárez Álvarez, M., et al. (2021). Are older adults also at higher psychological risk from COVID-19? Aging Ment. Health 25, 1297–1304. doi: 10.1080/13607863.2020.1805723
Geiselman, B., and Bauer, M. (2000). Subthreshold depression in the elderly: qualitative or quantitative distinction? Compr. Psychiatry 41, 32–38. doi: 10.1016/S0010-440X(00)80006-1
Gooding, P., Hurst, A., Johnson, J., and Tarrier, N. (2012). Psychological resilience in young and older adults. Int. J. Geriatr. Psychiatry 27, 262–270. doi: 10.1002/gps.2712
Griffin, B. J., Purcell, N., Burkman, K., Litz, B. T., Bryan, C. J., Schmitz, M., et al. (2019). Moral injury: an integrative review. J. Trauma. Stress. 32, 350–362. doi: 10.1002/jts.22362
Gunnell, D., Appleby, L., Arensman, E., Hawton, K., John, A., Kapur, N., et al. (2020). Suicide risk and prevention during the COVID-19 pandemic. Lancet Psychiatry 7, 468–471. doi: 10.1016/S2215-0366(20)30171-1
Haktanir, A., Can, N., Seki, T., Kurnaz, M. F., and Dilmaç, B. (2022). Do we experience pandemic fatigue? Current state, predictors, and prevention. Curr. Psychol. 41, 7314–7325. doi: 10.1007/s12144-021-02397-w
Hall, B. J., Lam, A. I. F., Wu, T. L., Hou, W.-K., Latkin, C., and Galea, S. (2017). The epidemiology of current depression in Macau, China: towards a plan for mental health action. Soc. Psychiatry Psychiatr. Epidemiol. 52, 1227–1235. doi: 10.1007/s00127-017-1415-8
Hao, Y.-T., Fang, J.-Q., and Power, M. (2006). The equivalence of WHOQOL-BREF among 13 culture versions. Chin. Ment. Health J.
Hartung, T. J., Fried, E. I., Mehnert, A., Hinz, A., and Vehling, S. (2019). Frequency and network analysis of depressive symptoms in patients with cancer compared to the general population. J. Affect. Disord. 256, 295–301. doi: 10.1016/j.jad.2019.06.009
Haslbeck, J., and Waldorp, L.J. (2015). Mgm: estimating time-varying mixed graphical models in high-dimensional data. arXiv preprint arXiv:1510.06871 [Preprint]. doi: 10.48550/arXiv.1510.06871.
He, X., Li, C., Qian, J., Cui, H., and Wu, W. (2010). A study on the reliability and validity of generalized anxiety scale in general hospitals. Shanghai Psychiatry 22, 200–203.
Hegerl, U. (2016). Prevention of suicidal behavior. Dialogues Clin. Neurosci. 18, 183–190. doi: 10.31887/DCNS.2016.18.2/uhegerl
Hertz-Palmor, N., Moore, T. M., Gothelf, D., DiDomenico, G. E., Dekel, I., Greenberg, D. M., et al. (2020). Association among income loss, financial strain and depressive symptoms during COVID-19: evidence from two longitudinal studies. medRxiv. doi: 10.1101/2020.09.15.20195339
Hobfoll, S. E. (2002). Social and psychological resources and adaptation. Rev. Gen. Psychol. 6, 307–324. doi: 10.1037//1089-2680.6.4.307
Jacobson, N. C., and Newman, M. G. (2017). Anxiety and depression as bidirectional risk factors for one another: a meta-analysis of longitudinal studies. Psychol. Bull. 143, 1155–1200. doi: 10.1037/bul0000111
Jakobsen, M. D., Sundstrup, E., Brandt, M., and Andersen, L. L. (2017). Psychosocial benefits of workplace physical exercise: cluster randomized controlled trial. BMC Public Health 17:798. doi: 10.1186/s12889-017-4728-3
Jeon, H. J., Park, J.-I., Fava, M., Mischoulon, D., Sohn, J. H., Seong, S., et al. (2014). Feelings of worthlessness, traumatic experience, and their comorbidity in relation to lifetime suicide attempt in community adults with major depressive disorder. J. Affect. Disord. 166, 206–212. doi: 10.1016/j.jad.2014.05.010
Jin, Y., Sun, H.-L., Lam, S. C., Su, Z., Hall, B. J., Cheung, T., et al. (2022). Depressive symptoms and gender differences in older adults in Hong Kong during the COVID-19 pandemic: a network analysis approach. Int. J. Biol. Sci. 18, 3934–3941. doi: 10.7150/ijbs.69460
Jin, Y., Zhu, D., and He, P. (2020). Social causation or social selection? The longitudinal interrelationship between poverty and depressive symptoms in China. Soc. Sci. Med. 249:112848. doi: 10.1016/j.socscimed.2020.112848
Kim, S. T., Seo, J. H., Lee, S., Jeon, S., Park, C. I., Kim, S. J., et al. (2022). Dysfunctional coronavirus anxiety in nonpsychotic psychiatric outpatients during the COVID-19 pandemic: a network analysis. Depress. Anxiety 39, 556–563. doi: 10.1002/da.23256
Kleinman, A. (2004). Culture and depression. N. Engl. J. Med. 351, 951–953. doi: 10.1056/NEJMp048078
Kokaliari, E. (2018). Quality of life, anxiety, depression, and stress among adults in Greece following the global financial crisis. Int. Soc. Work. 61, 410–424. doi: 10.1177/0020872816651701
Koo, T. K., and Li, M. Y. (2016). A guideline of selecting and reporting Intraclass correlation coefficients for reliability research. J. Chiropr. Med. 15, 155–163. doi: 10.1016/j.jcm.2016.02.012
Kroenke, K., Spitzer, R. L., and Williams, J. B. (2001). The PHQ-9: validity of a brief depression severity measure. J. Gen. Intern. Med. 16, 606–613. doi: 10.1046/j.1525-1497.2001.016009606.x
Lee, W. H., and Kim, C. J. (2006). The relationship between depression, perceived stress, fatigue and anger in clinical nurses. J. Korean Acad. Nurs. 36, 925–932. doi: 10.4040/jkan.2006.36.6.925
Li, L., Lok, G. K. I., Mei, S.-L., Cui, X.-L., An, F.-R., Li, L., et al. (2020). Prevalence of depression and its relationship with quality of life among university students in Macau, Hong Kong and mainland China. Sci. Rep. 10:15798. doi: 10.1038/s41598-020-72458-w
Luck, T., and Luck-Sikorski, C. (2021). Feelings of guilt in the general adult population: prevalence, intensity and association with depression. Psychol. Health Med. 26, 1143–1153. doi: 10.1080/13548506.2020.1859558
Lund, C., and Cois, A. (2018). Simultaneous social causation and social drift: longitudinal analysis of depression and poverty in South Africa. J. Affect. Disord. 229, 396–402. doi: 10.1016/j.jad.2017.12.050
Luo, W., Zhong, B. L., and Chiu, H. F. (2021). Prevalence of depressive symptoms among Chinese university students amid the COVID-19 pandemic: a systematic review and meta-analysis. Epidemiol. Psychiatr. Sci. 30:e31. doi: 10.1017/s2045796021000202
Lux, V., and Kendler, K. (2010). Deconstructing major depression: a validation study of the DSM-IV symptomatic criteria. Psychol. Med. 40, 1679–1690. doi: 10.1017/S0033291709992157
Macao Daily (2022a). The city will be suspended for seven days from tomorrow. https://appimg.modaily.cn/app/szb/pc/content/202207/10/content_203699.html
Macao Daily (2022b). ‘Continued relative standstill’ non-life-sustaining business activities suspended until next Friday. http://www.macaodaily.com/html/2022-07/17/content_1610099.htm
Macao Daily (2022c). Industrial and commercial premises closed for a week on Monday. https://appimg.modaily.cn/app/displayTemplate1/dist/index.html#/newsDetail/9833199/?isView=true
Macao Daily (2022d). Long-term patient in 90s dies of new crown. http://www.macaodaily.com/html/2022-07/22/content_1610775.htm
Macao Daily (2022e). Macao and Zhuhai actively negotiate entry and exit. http://www.macaodaily.com/html/2022-06/20/content_1604773.htm
MacLeod, S., Musich, S., Hawkins, K., Alsgaard, K., and Wicker, E. R. (2016). The impact of resilience among older adults. Geriatr. Nurs. 37, 266–272. doi: 10.1016/j.gerinurse.2016.02.014
Marchetti, I. (2019). Hopelessness: a network analysis. Cogn. Ther. Res. 43, 611–619. doi: 10.1007/s10608-018-9981-y
Mazza, C., Ricci, E., Biondi, S., Colasanti, M., Ferracuti, S., Napoli, C., et al. (2020). A nationwide survey of psychological distress among Italian people during the COVID-19 pandemic: immediate psychological responses and associated factors. Int. J. Environ. Res. Public Health 17:3165. doi: 10.3390/ijerph17093165
McCartney, G. (2021). The impact of the coronavirus outbreak on Macao. From tourism lockdown to tourism recovery. Curr. Issue Tour. 24, 2683–2692. doi: 10.1080/13683500.2020.1762549
McIlvenny, S., DeGlume, A., Elewa, M., Fernandez, O., and Dormer, P. (2000). Factors associated with fatigue in a family medicine clinic in the United Arab Emirates. Fam. Pract. 17, 408–413. doi: 10.1093/fampra/17.5.408
Mehnert, A., Veers, S., Howaldt, D., Braumann, K.-M., Koch, U., and Schulz, K.-H. (2011). Effects of a physical exercise rehabilitation group program on anxiety, depression, body image, and health-related quality of life among breast cancer patients. Oncol. Res. Treat. 34, 248–253. doi: 10.1159/000327813
Moghanibashi-Mansourieh, A. (2020). Assessing the anxiety level of Iranian general population during COVID-19 outbreak. Asian J. Psychiatr. 51:102076. doi: 10.1016/j.ajp.2020.102076
Mullarkey, M. C., Marchetti, I., and Beevers, C. G. (2019). Using network analysis to identify central symptoms of adolescent depression. J. Clin. Child Adolesc. Psychol. 48, 656–668. doi: 10.1080/15374416.2018.1437735
Norström, F., Waenerlund, A.-K., Lindholm, L., Nygren, R., Sahlén, K.-G., and Brydsten, A. (2019). Does unemployment contribute to poorer health-related quality of life among Swedish adults? BMC Public Health 19, 457–412. doi: 10.1186/s12889-019-6825-y
Nwachukwu, I., Nkire, N., Shalaby, R., Hrabok, M., Vuong, W., Gusnowski, A., et al. (2020). COVID-19 pandemic: age-related differences in measures of stress, anxiety and depression in Canada. Int. J. Environ. Res. Public Health 17:6366. doi: 10.3390/ijerph17176366
Portal do Governo da Região Administrativa Especial de Macau da República Popular da China (2022). Report on the Macao SAR Government's response to the “618” pandemic.
Queen, D., and Harding, K. (2020). Societal pandemic burnout: a COVID legacy. Int. Wound J. 17, 873–874. doi: 10.1111/iwj.13441
R Core Team (2013). R: a language and environment for statistical computing. R foundation for statistical computing, Vienna, Austria: R Core Team.
Radio Television Hong Kong (2022). Zhuhai immediately adjusts the health management measures for Macao inbound persons.
Robinaugh, D. J., Millner, A. J., and McNally, R. J. (2016). Identifying highly influential nodes in the complicated grief network. J. Abnorm. Psychol. 125, 747–757. doi: 10.1037/abn0000181
Sahoo, S., Mehra, A., Suri, V., Malhotra, P., Yaddanapudi, L. N., Puri, G. D., et al. (2020). Lived experiences of the corona survivors (patients admitted in COVID wards): a narrative real-life documented summaries of internalized guilt, shame, stigma, anger. Asian J. Psychiatr. 53:102187. doi: 10.1016/j.ajp.2020.102187
Santos, H. Jr., Fried, E. I., Asafu-Adjei, J., and Ruiz, R. J. (2017). Network structure of perinatal depressive symptoms in Latinas: relationship to stress and reproductive biomarkers. Res. Nurs. Health 40, 218–228. doi: 10.1002/nur.21784
Schmittmann, V. D., Cramer, A. O., Waldorp, L. J., Epskamp, S., Kievit, R. A., and Borsboom, D. (2013). Deconstructing the construct: a network perspective on psychological phenomena. New Ideas Psychol. 31, 43–53. doi: 10.1016/j.newideapsych.2011.02.007
Silva Júnior, E. G. D., Eulálio, M. D. C., Souto, R. Q., Santos, K. L., Melo, R. L. P., and Lacerda, A. R. (2019). The capacity for resilience and social support in the urban elderly. Ciencia & saude coletiva 24, 7–16. doi: 10.1590/1413-81232018241.32722016
Skevington, S. M., Lotfy, M., and O'Connell, K. A. (2004). The World Health Organization's WHOQOL-BREF quality of life assessment: psychometric properties and results of the international field trial. A report from the WHOQOL group. Qual. Life Res. 13, 299–310. doi: 10.1023/B:QURE.0000018486.91360.00
Southwick, S. M., Bonanno, G. A., Masten, A. S., Panter-Brick, C., and Yehuda, R. (2014). Resilience definitions, theory, and challenges: interdisciplinary perspectives. Eur. J. Psychotraumatol. 5:25338. doi: 10.3402/ejpt.v5.25338
Spitzer, R. L., Kroenke, K., Williams, J. B. W., and Löwe, B. (2006). A brief measure for assessing generalized anxiety disorder: the GAD-7. Arch. Intern. Med. 166, 1092–1097. doi: 10.1001/archinte.166.10.1092
Stuckler, D., Basu, S., Suhrcke, M., Coutts, A., and McKee, M. (2009). The public health effect of economic crises and alternative policy responses in Europe: an empirical analysis. Lancet 374, 315–323. doi: 10.1016/S0140-6736(09)61124-7
Sweller, J. (1988). Cognitive load during problem solving: effects on learning. Cogn. Sci. 12, 257–285. doi: 10.1016/0364-0213(88)90023-7
Tanhan, A., Yavuz, K. F., Young, J. S., Nalbant, A., Arslan, G., Yıldırım, M., et al. (2020). A proposed framework based on literature review of online contextual mental health services to enhance wellbeing and address psychopathology during COVID-19. Electron. J. Gen. Med. 17:Article No: em254. doi: 10.29333/ejgm/8316
The WHOQOL Group (1998). Development of the World Health Organization WHOQOL-BREF quality of life assessment. Psychol. Med. 28, 551–558. doi: 10.1017/S0033291798006667
Tilghman-Osborne, C., Cole, D. A., and Felton, J. W. (2012). Inappropriate and excessive guilt: instrument validation and developmental differences in relation to depression. J. Abnorm. Child Psychol. 40, 607–620. doi: 10.1007/s10802-011-9591-6
Ustun, G. (2021). Determining depression and related factors in a society affected by COVID-19 pandemic. Int. J. Soc. Psychiatry 67, 54–63. doi: 10.1177/0020764020938807
Vindegaard, N., and Benros, M. E. (2020). COVID-19 pandemic and mental health consequences: systematic review of the current evidence. Brain Behav. Immun. 89, 531–542. doi: 10.1016/j.bbi.2020.05.048
Wang, W., Bian, Q., Zhao, Y., Li, X., Wang, W., du, J., et al. (2014). Reliability and validity of the Chinese version of the patient health questionnaire (PHQ-9) in the general population. Gen. Hosp. Psychiatry 36, 539–544. doi: 10.1016/j.genhosppsych.2014.05.021
WHO (2020). Mental health and psychosocial considerations during the COVID-19 outbreak. Geneva: World Health Organization).
Wieman, S. T., Arditte Hall, K. A., MacDonald, H. Z., Gallagher, M. W., Suvak, M. K., Rando, A. A., et al. (2022). Relationships among sleep disturbance, reward system functioning, Anhedonia, and depressive symptoms. Behav. Ther. 53, 105–118. doi: 10.1016/j.beth.2021.06.006
World Health Organization (2022). WHO coronavirus (COVID-19) dashboard. https://covid19.who.int/
Xia, P., Li, N., Hau, K.-T., Liu, C., and Lu, Y. (2012). Quality of life of Chinese urban community residents: a psychometric study of the mainland Chinese version of the WHOQOL-BREF. BMC Med. Res. Methodol. 12, 1–11. doi: 10.1186/1471-2288-12-37
Xiao, C. (2020). A novel approach of consultation on 2019 novel coronavirus (COVID-19)-related psychological and mental problems: structured letter therapy. Psychiatry Investig. 17, 175–176. doi: 10.30773/pi.2020.0047
Xiong, J., Lipsitz, O., Nasri, F., Lui, L. M. W., Gill, H., Phan, L., et al. (2020). Impact of COVID-19 pandemic on mental health in the general population: a systematic review. J. Affect. Disord. 277, 55–64. doi: 10.1016/j.jad.2020.08.001
Yang, Y., Zhang, D.-Y., Li, Y.-L., Zhang, M., Wang, P.-H., Liu, X.-H., et al. (2022). Prevalence, correlates, and network analysis of internet addiction symptoms among Chinese pregnant and postpartum women. J. Affect. Disord. 298, 126–133. doi: 10.1016/j.jad.2021.10.092
Yu, Y., Liu, J., Skokauskas, N., Liu, F., Zhang, L., Teng, T., et al. (2023). Prevalence of depression and anxiety, and associated factors, among Chinese primary and high school students: a cross-sectional, epidemiological study. Asia Pac. Psychiatry 15:e12523. doi: 10.1111/appy.12523
Zavlis, O., Butter, S., Bennett, K., Hartman, T. K., Hyland, P., Mason, L., et al. (2021). How does the COVID-19 pandemic impact on population mental health? A network analysis of COVID influences on depression, anxiety and traumatic stress in the UK population. Psychol. Med. 52, 3825–3833. doi: 10.1017/S0033291721000635
Zhao, Y.-J., Bai, W., Cai, H., Sha, S., Zhang, Q., Lei, S. M., et al. (2022a). The backbone symptoms of depression: a network analysis after the initial wave of the COVID-19 pandemic in Macao. PeerJ 10:e13840. doi: 10.7717/peerj.13840
Zhao, N., Li, W., Zhang, S. F., Yang, B. X., Sha, S., Cheung, T., et al. (2021). Network analysis of depressive symptoms among residents of Wuhan in the later stage of the COVID-19 pandemic. Front. Psych. 12:735973. doi: 10.3389/fpsyt.2021.735973
Zhao, Y.-J., Zhang, S.-F., Li, W., Zhang, L., Guo, T., Cheung, T., et al. (2022b). Associations between depressive symptoms and quality of life among residents of Wuhan, China during the later stage of the COVID-19 pandemic: a network analysis. J. Affect. Disord. 318, 456–464. doi: 10.1016/j.jad.2022.08.104
Keywords: depression, quality of life, prevalence, COVID-19, network analysis
Citation: Si TL, Chen P, Zhang L, Sha S, Lam MI, Lok K-I, Chow IHI, Li J-X, Wang Y-Y, Su Z, Cheung T, Ungvari GS, Ng CH, Feng Y and Xiang Y-T (2023) Depression and quality of life among Macau residents in the 2022 COVID-19 pandemic wave from the perspective of network analysis. Front. Psychol. 14:1164232. doi: 10.3389/fpsyg.2023.1164232
Edited by:
Bao-Liang Zhong, Wuhan Mental Health Center, ChinaCopyright © 2023 Si, Chen, Zhang, Sha, Lam, Lok, Chow, Li, Wang, Su, Cheung, Ungvari, Ng, Feng and Xiang. This is an open-access article distributed under the terms of the Creative Commons Attribution License (CC BY). The use, distribution or reproduction in other forums is permitted, provided the original author(s) and the copyright owner(s) are credited and that the original publication in this journal is cited, in accordance with accepted academic practice. No use, distribution or reproduction is permitted which does not comply with these terms.
*Correspondence: Yuan Feng, MTk1NTgwNTFAcXEuY29t; Chee H. Ng, Y25nQHVuaW1lbGIuZWR1LmF1
†These authors have contributed equally to this work