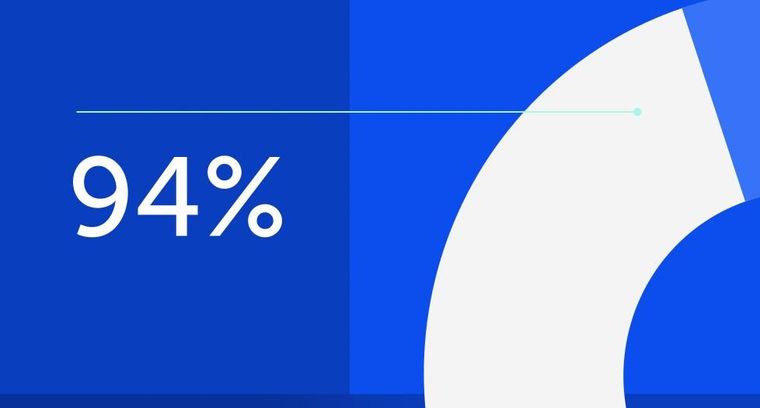
94% of researchers rate our articles as excellent or good
Learn more about the work of our research integrity team to safeguard the quality of each article we publish.
Find out more
ORIGINAL RESEARCH article
Front. Psychol., 20 April 2023
Sec. Neuropsychology
Volume 14 - 2023 | https://doi.org/10.3389/fpsyg.2023.1150540
This article is part of the Research TopicEarly Indicators of Cognitive Decline, Alzheimer’s Disease, and Related Dementias Captured by Neurophysiological ToolsView all 14 articles
Introduction: Differentiating between the two most common forms of dementia, Alzheimer’s dementia and dementia with Lewy bodies (DLB) remains difficult and requires the use of invasive, expensive, and resource-intensive techniques. We aimed to investigate the sensitivity and specificity of electroencephalography quantified using the statistical pattern recognition method (qEEG-SPR) for identifying dementia and DLB.
Methods: Thirty-two outpatients and 16 controls underwent clinical assessment (by two blinded neurologists), EEG recording, and a 6-month follow-up clinical assessment. EEG data were processed using a qEEG-SPR protocol to derive a Dementia Index (positive or negative) and DLB index (positive or negative) for each participant which was compared against the diagnosis given at clinical assessment. Confusion matrices were used to calculate sensitivity, specificity, and predictive values for identifying dementia and DLB specifically.
Results: Clinical assessment identified 30 cases of dementia, 2 of which were diagnosed clinically with possible DLB, 14 with probable DLB and DLB was excluded in 14 patients. qEEG-SPR confirmed the dementia diagnosis in 26 out of the 32 patients and led to 6.3% of false positives (FP) and 9.4% of false negatives (FN). qEEG-SPR was used to provide a DLB diagnosis among patients who received a positive or inconclusive result of Dementia index and led to 13.6% of FP and 13.6% of FN. Confusion matrices indicated a sensitivity of 80%, a specificity of 89%, a positive predictive value of 92%, a negative predictive value of 72%, and an accuracy of 83% to diagnose dementia. The DLB index showed a sensitivity of 60%, a specificity of 90%, a positive predictive value of 75%, a negative predictive value of 81%, and an accuracy of 75%. Neuropsychological scores did not differ significantly between DLB and non- DLB patients. Head trauma or story of stroke were identified as possible causes of FP results for DLB diagnosis.
Conclusion: qEEG-SPR is a sensitive and specific tool for diagnosing dementia and differentiating DLB from other forms of dementia in the initial state. This non-invasive, low-cost, and environmentally friendly method is a promising diagnostic tool for dementia diagnosis which could be implemented in local care settings.
Alzheimer’s disease (AD) and other forms of dementia are significant causes of disability and dependency among older people, worldwide (Lisko et al., 2021). While no curative therapies are currently available for dementia, there are considerable benefits to the early diagnosis of dementia and early differentiation between dementia subtypes. These benefits include better patient counseling and disease prognostication, appropriate selection of pharmacological and non-pharmacological options for symptomatic management, and early modification of cardiovascular risk factors which adversely affect disease progression. Early disease identification is also considered critical to develop both symptomatic and disease modifying therapies (Rasmussen and Langerman, 2019). The recent approval of aducanumab, a monoclonal antibody targeting amyloid-β fibrils, by the U.S. Food and Drug Administration, has been controversial; however, this potentially disease modifying treatment for AD further emphasizes the need for early and specific diagnosis of AD, as the phase 3 trial evidence for aducanumab suggests that it may exert a clinically significant effect, slowing the progression of cognitive decline in AD, but only in the early phase of the disease (Cummings et al., 2021).
After AD, the most common form of dementia is dementia with Lewy bodies (DLB) (Walker et al., 2015; McKeith et al., 2017; Arvanitakis et al., 2019). Reports suggest that DLB is under-diagnosed in clinical practice (Mok et al., 2004; Toledo et al., 2013) with difficulties in making an early diagnosis and differentiating DLB from AD posing the greatest challenge (Walker et al., 2015). Currently, the diagnosis of DLB is based on the identification of core clinical features: cognitive fluctuations (a particularly difficult clinical feature to elicit accurately), visual hallucinations, parkinsonism, and RBD (McKeith et al., 2017). Supportive clinical features and indicative biomarkers (including Positron Emission Tomography (PET), Single Positron Emission Computed Tomography (SPECT) and Magnetic Resonance Imaging (MRI), electroencephalography (EEG) and polysomnography (PSG)) can provide further indications for the diagnosis of DLB (McKeith et al., 2017). The accurate, early diagnosis of DLB is particularly important in order to ensure the appropriate selection of symptomatic pharmacotherapy as certain medications, namely most antipsychotics, which may be used to manage hallucinations or agitation, can generate potentially severe adverse reactions in approximately half of patients with DLB (Aarsland et al., 2008).
In the DLB diagnosis process, routine clinical assessments including physical examinations, blood tests, and basic neuropsychological tests must generally be supplemented by increasingly specialist assessments such as neuro-immunological analysis of cerebrospinal fluid (CSF), requiring an invasive lumbar puncture, complex neuropsychological and electrophysiological tests requiring specialist expertise and equipment and expensive, resource-intensive neuroimaging assessments (Walker et al., 2015; McKeith et al., 2017). These assessments, though effective, require many heavy resources and are therefore costly, in terms of time, money, and their environmental impact and are often only available in specialist centers. Thus, there is a need to develop and promote the use of robust but inexpensive, sustainable and easy-to-use diagnostic tools which can be implemented in small clinical centers and which can be used to streamline the assessment process, giving an indication of which patients warrant more in depth assessment or indeed a diagnostic tool which could provide a robust diagnosis without the other measures.
Electroencephalography (EEG) is a non-invasive diagnostic method which is relatively simple to implement, is inexpensive and therefore, could be provided in most clinical centers. Quantitative EEG analyses (qEEG), an EEG analysis methodology utilizing different computational algorithms such as fast Fourier transform (FFT) or auto regressive (AR) models, has been shown to be a reliable method for measuring modulations in cerebral activity in dementia, with the ability to differentiate AD from other forms of dementia, such as frontotemporal dementia or DLB (Caso et al., 2012; Engedal et al., 2015). EEG of patients with DLB are characterized by theta and delta activity in the posterior, anterior and temporal regions (van der Zande et al., 2018). Slower background activity has been constantly reported in DLB patients compared to AD with the mean dominant frequency ranging between 6.7–7.5 Hz for DLB and 7.5–8.8 Hz for AD (Law et al., 2020). Moreover, alpha relative power in occipital regions is reduced in AD compared to DLB while delta relative.
Power is higher in DLB than AD (Babiloni et al., 2017, 2018). Increased theta/delta power or activities would be more prominent in the posterior region in DLB patients (Kai et al., 2005; Bonanni et al., 2015; Babiloni et al., 2017). Although the dominant frequency was lower with more pre-alpha activities in the anterior region, the diagnostic accuracy of posterior pre-alpha rhythm was higher in differentiating DLB from AD (Bonanni et al., 2008, 2015, 2016). Studies of connectivity showed that phase lag index within the alpha range was lower in DLB than AD, indicating more severe changes in connectivity in DLB (van Dellen et al., 2015; Dauwan et al., 2018; van der Zande et al., 2018). Analyses of event-related potentials also showed differential abnormalities between DLB and AD patients, with delayed auditory or visual P300 in DLB patients (Bonanni et al., 2010; Kurita et al., 2010). Regarding the early stages of the various forms of dementia, EEG abnormalities have been reported to be more common in DLB, even at the mild cognitive impairment (MCI) stage (van der Zande et al., 2020). Thus, analysis of EEG features might have a good accuracy in differentiating DLB from other forms of dementia (Law et al., 2020). Regarding the association between EEG analyses and DLB clinical symptoms, EEG slowing has been correlated with cognitive fluctuations (Briel et al., 1999; Walker et al., 2000a,b; Stylianou et al., 2018). Hallucinations have been associated with slowing of dominant rhythm and decreased functional connectivity (Dauwan et al., 2018; Aoki et al., 2019). Regarding the relationship between EEG abnormalities and cognitive functions, severity of EEG abnormalities have been shown to correlate with MMSE scores (Law et al., 2020). Moreover, EEG features in DLB patients have been shown to correlate with specific domains of cognitive function, such as fronto-executive and visual abilities. The correlation coefficient values ranged between 0.29 and 0.60 indicating weak to moderate correlations (Law et al., 2020).
Thus, many EEG algorithms have been proposed to investigate the pathophysiology of DLB. Applying qEEG using the statistical pattern recognition (SPR) method (qEEG-SPR), where EEG data are processed and classified based on comparison with normative data from a well-defined group of patients with various dementia disorders and from healthy controls, has been shown to be effective in identifying patients with subjective cognitive decline and MCI that have a high risk of converting to dementia over a 5-year period (Ferreira et al., 2016). Moreover, in the last decade, several studies have applied the qEEG-SPR method in order to identify patterns in AD, DLB or other dementias (Snaedal et al., 2010, 2012; Ommundsen et al., 2011; Engedal et al., 2015; Ferreira et al., 2016). Such methods could distinguish patients with dementia from healthy controls with a sensitivity of 76.9% and a specificity of 73.2%, and, among patients with dementia, to differentiate patients with DLB from other forms of dementia with a sensitivity of 90.9% and a specificity of 91.1% (Ferreira et al., 2016). To this aim, MentisCura have developed and tested in the last decade a qEEG-SPR protocol based on a database of 1,000 EEG recordings of patients with clinically confirmed dementia subtypes and 500 healthy controls (Gudmundsson et al., 2007). This database has been developed to identify various classifiers contrasting different sub-cohorts. These classifiers can then be applied to subsequent EEG recordings, constituting an independent estimate of the properties of the classifiers (Engedal et al., 2015).
In this study, we used retrospective clinical data to assess the use of the MentisCura qEEG-SPR protocol in a real-world sample of patients who had been referred for dementia assessment. We aimed to assess the utility of this protocol in identifying dementia and in distinguishing between DLB and other forms of dementia, using the clinical diagnosis [based on the diagnosis criteria for dementia and DLB diagnosis (American Psychiatric Association and American Psychiatric Association, 2013; McKeith et al., 2017)] obtained at the time of assessment as our diagnostic standard. We also aimed to assess whether the combination of neurological assessment, EEG, and neuropsychological tests could further improve the sensitivity and specificity of the results.
Thirty-two patients who visited the outpatient clinic of the Department of Rehabilitation and Functional Recovery of the San Raffaele Hospital (Milan, Italy) with suspected initial state of dementia or cognitive impairment were recruited for this study, as well as 16 healthy controls. To participate to this study, patients had to be aged 50 to 85 y.o. and present symptoms of dementia according to the DSM-5, i.e., substantial impairments in one or more cognitive domains, sufficient to interfere with independence in everyday activities (Hugo and Ganguli, 2014). Oral and written consents were obtained from participants, in accordance with the Code of Ethics of the World Medical Association (Declaration of Helsinki) and the study was approved by the local Ethics committee of the San Raffaele Hospital.
Every participant underwent the following visits prior to the inclusion of their data in the study: clinic visit with neurologist, EEG recording visit, and follow-up clinic visit at 6 months. When available, neuropsychological evaluation of patients was gathered for analyses.
Medical history was obtained from both the patient and a close caregiver, in order to characterize the nature, course, and magnitude of cognitive changes (Arvanitakis et al., 2019). The neurologic examination aimed at identifying objective evidence of neurocognitive issues such as aphasia, apraxia or agnosia, and focal neurologic signs of parkinsonism and included a physical examination to identify systemic vascular disease and systemic signs of rare dementia (Arvanitakis et al., 2019). Based on the neurological examination and all the available data, such as neuropsychological evaluation or MRI/PET data, the neurologist gave a diagnosis of dementia or non-dementia. The majority of MRI or PET imaging was performed in different clinical centers, therefore images were not available for analysis in this study, but clinical reports were used for diagnosis. The diagnosis of probable or possible DLB was based on the diagnostic criteria for DLB (McKeith et al., 2017). A follow-up neurological assessment was performed after 6 months. At both visits, patients were seen by two neurologists, who gave their clinical diagnoses independently. At the time of the study, none of the patients were under benzodiazepines or acetylcholinesterase inhibitors.
EEG recordings were obtained the week following the neurological evaluation. EEGs were recorded from 19 Ag/AgCl electrodes fixed on an elastic cap accordingly to the 10–20 International System, referenced to CPz, with the ground in AFz. The 19 recording electrodes were the following: Fp1, Fp2, F3, F4, C3, C4, P3, P4, O1, O2, F7, F8, T3, T4, T5, T6, Fz, Cz, and Pz. Patients were seated on an armchair, with their arms and legs at rest, and were asked to close their eyes. Five-minute resting-state EEGs were recorded for each patient. Signals were sampled at 1 kHz and coded on 16 bits. Impedances were kept below 5 kΩ. EEG data were acquired using the NicoletOne EEG System from Natus®.
Some patients, included in the study, had previously underwent a detailed neuropsychological evaluation. The following tests for different cognitive domains were then analyzed: Mini Mental State Examination (MMSE) (Folstein et al., 1975), Attentive and Raven Matrices (Raven, 2003), Token test [36], Semantic fluency (Novelli et al., 1986), Phonemic fluency (Novelli et al., 1986), naming (Miceli et al., 1994), word picture matching test (Kaplan et al., 1983), Digit span test (Orsini et al., 1987), Digit Span Backward (Wechsler, 1955), Corsi block-tapping test (Corsi, 1972), Rey Complex Figure Test (Carlesimo et al., 1996), Trail making test (Reitan, 1955), Stroop test (Jensen and Rohwer, 1966; Heaton et al., 1993), and Wisconsin Card Sorting test [46].
The analyses methods described below have been employed in previous studies (Snaedal et al., 2010, 2012; Ferreira et al., 2016; Engedal et al., 2020).
The EEG segment used for analysis was selected by a trained technician who chose a segment with minimal presence of artifact and a length of at least 150 s. Prior to feature extraction, the chosen segment was preprocessed by applying an 8th-order Butterworth band-pass filter with the chosen band (0.1–70 Hz) to eliminate potential low- and high-frequency disturbances from the signal. The features extracted from the EEG recording and used in the evaluation of the dementia index (DI) were retrieved according to the recommendations of the Pharmaco-EEG society (Jobert et al., 2013). The society recommends that the signal is segmented into 2-s segments overlapping by 1 s. The signal is then analyzed segment by segment, and the feature values are estimated by evaluating the expected value over all the segments. This can be achieved by various means. For instance, using the average value or an alternative robust measure. Using a robust measure minimizes the impact of outliers and hence reduces the influence of potential signal artifacts. We used the simplest robust estimate, that is, the median of the feature values. The features used were all related to the spectral properties of the recording. Discrete fast Fourier transform was applied to estimate the spectral properties of the signal (Cooley and Tukey, 1965). The analysis relied on the recordings from the 19 electrodes. If the fast Fourier transform components for each of the electrodes, segments, and discrete frequencies considered are denoted by σcij, where c ∈ {1,2,…, 19} indicates the channel, i ∈ {1,…, N} the segment of the N segments considered, and j ∈ {1,…, 90} the discrete frequencies (0.5, 1,…, 45 Hz), the full spectral resolution covariance between channels c and k is then expressed by xij ck = σcij × σ*kij. These covariances constituted the base features used for analysis and evaluation of the classification index values.
The aim of the qEEG-SPR protocol was to sort patients within two classifier indices. The first classifier, the “dementia index” (DI), was constructed to separate healthy individuals from patients presenting with any dementia disorder. This index showed good diagnostic capacity for AD (Snaedal et al., 2012). The second classifier, the “DLB index,” was constructed to detect patients with DLB among the clinical cohort of patients with dementia. To determine the core features relied on, principal components (PCs) were determined based on the Mentis Cura database of EEG recordings. PC analysis was performed on data from dementia subjects in the database. This was done separately for each covariance. PCs were then ranked according to their individual discriminatory properties in separating the subjects in the database. The discriminatory properties were determined according to the area under curve (AUC) of the receiver-operating characteristic curve (ROC). We use the 2 best performing components from each of the covariances to extract the core features used for evaluation of the index. If Pckαj denotes the 2 chosen PCs, α ∈ {1, 2}, for electrode pair (c, k) at frequencies j ∈ {1,…, 90}, the core features considered for analysis then become Cckα = Ei {Σ90 j = 1 xij ck Pckαj}. The PCs can be related to the classical EEG power bands, δ (1–4 Hz), θ (4–8 Hz), α (8–13 Hz), and β (13–30 Hz). Then, PC1 corresponds to the difference between the combined δ and θ power and the β power, while PC2 is a weighted measure of the total power with slightly more emphasis on α and β power. The index value for an individual recording is evaluated from these features by I = ΣckαCckαβckα + βA1 A + βA2 A2 + ρ, where A is the age of the subject in years. The classification coefficients βckα, βAi, and ρ were determined using a combination of genetic algorithms to optimize the number of features used, and SVM (support vector machine), an SPR, was applied in the Mentis Cura database, which contains EEG data from people with various dementia diagnoses and HC. This was done separately for men and women, resulting in separate gender-dependent indices.
Analyses were done with Sigla v.3.3®, by an experimenter blinded for all clinical symptoms, medical history, and diagnosis of patients.
Confusion matrices were built to evaluate the performance of EEG algorithm for the diagnosis of dementia and DLB compared to the clinical diagnosis representing current clinical practice (reference category: clinical diagnosis; predictor: EEG results). Neuropsychological assessments were compared between DLB and non-DLB patients using Mann–Whitney test. Measures of concordance between EEG and neuropsychological tests were performed using Cohen’s test. A correlation analysis between MMSE scores and the Dementia Index was performed using Pearson’s correlation test.
Data were considered significant when p < 0.05. The commercially available software IBM SPSS Statistics v.23 (IBM Corp.©) was used for all statistical tests.
Clinical and EEG data from 32 patients, who were visited in our memory outpatient clinic between September 2019 and January 2021, were utilized for this study. Twenty-four out of 32 patients were male, patients’ mean age was 73.6 ± 7.6 y.o. and their mean education level was 11.7 ± 4.2 y. Sixteen controls were also included in the study (6 female, mean age 70.1 ± 7.6 y.o., mean education level 12.3 ± 5.1 y.).
Patients’ demographic and clinical data are summarized in Table 1.
Table 1. Reports demographic and clinical data of all patients, including EEG results for dementia index and DLB index and clinical diagnosis at follow-up.
Clinical diagnoses for each patient, given after neurological examination at the first visit (V1) are listed in Table 1. At V1, 30 out of 32 patients were diagnosed with dementia. All patients were in the initial phase of the disease (symptoms’ onset <1 year). Of those diagnosed with dementia (n = 30), 2 patients were diagnosed with possible DLB, 14 with probable DLB and 14 had DLB excluded. No diagnoses were revised at follow-up.
qEEG results were reported as a Dementia Index (positive or negative) and a DLB index (positive or negative). Twenty-six out of 32 patients showed a positive Dementia Index result, 3 patients showed a negative Dementia Index result, and 3 showed an inconclusive result (Table 1). Negative Dementia Index was obtained in 13 out of the 16 controls and inconclusive results were obtained for 3 controls.
When comparing qEEG results and the clinical diagnoses of all participants, the EEG dementia index reported 2 false positive (6.3%) and 3 false negative results (9.4%) in the patients’ group.
The confusion matrix indicated a sensitivity of 80%, a specificity of 89%, a positive predictive value of 92%, a negative predictive value of 72% and an accuracy of 83% (Figure 1).
Figure 1. Left: confusion matrix built to evaluate the performance of EEG in the diagnosis of dementia, compared to clinical diagnosis. Right: confusion matrix built to evaluate the performance of EEG in the diagnosis of Lewy Body Dementia, compared to clinical diagnosis.
Among patients with a positive or inconclusive Dementia Index result (n = 29), 12 patients presented with a positive DLB Index result, 10 patients showed a negative DLB Index result, while the DLB index was inconclusive for 7 patients.
Regarding the DLB index, the confusion matrix indicated a sensitivity of 60%, a specificity of 90%, a positive predictive value of 75%, a negative predictive value of 81%, and an accuracy of 75% (Figure 1).
Among the 22 patients who obtained a positive or negative DLB index, 3 patients obtained false positive results (13.6%) and 3 other patients obtained false negative results (13.6%).
Two out of the three patients who presented with false positive DLB index had a history of hemorrhagic stroke or head trauma. The third patient suffered from vascular dementia.
We thus removed from the data analyses patients who had a history of stroke or head trauma (n = 3). Moreover, since only probable DLB is diagnosed as DLB in clinical settings, we also removed patients with possible DLB diagnoses (n = 2).
After removing these patients, when analyzing results of the dementia index, the confusion matrix indicated a sensitivity of 81%, a specificity of 94%, a positive predictive value of 95.5%, a negative predictive value of 76%, and an accuracy of 87.4%.
Regarding the DLB index, the confusion matrix indicated a sensitivity of 657%, a specificity of 96.3%, a positive predictive value of 88.9%, a negative predictive value of 81.2%, and an accuracy of 76.7% of the EEG reports.
Eighteen out of 32 patients underwent neuropsychological tests. Out of these 18 patients, all were diagnosed with dementia, and 6 out of 18 received a diagnosis of DLB at V1. Neuropsychological scores did not differ significantly between patients with or without DLB (Table 2). Cohen’s coefficient indicated a poor concordance between the EEG reports of dementia (dementia index and DLB index) and neuropsychological test scores (Table 2). The correlation analysis showed a tendency for a negative correlation between the Dementia Index and the MMSE scores (R = −0.463, p = 0.053).
Table 2. Reports median scores and (interquartile range) for neuropsychological tests undergone by 18 out of the 32 patients.
This study reports the successful application of machine learning derived indices to a separate and novel clinical dataset. Our data demonstrated that qEEG, using the statistical pattern recognition method, could constitute a sensitive indicator of dementia in a real-world clinical sample and had a high positive predictive value for differentiating DLB from other forms of dementia, even in the initial phase of the disease. This suggests that qEEG has the potential to be a robust method for screening patients for dementia and DLB.
These data confirmed previous evidence showing good sensitivity and specificity for both the dementia and DLB indexes (Engedal et al., 2015, 2020; Ferreira et al., 2016). These diagnoses were confirmed by the clinical examination of patients with clinical diagnoses expressed by two blinded neurologists at V1 and at 6 months follow-up. Our data reported higher sensitivity of the dementia index and lower sensitivity of the DLB index at point value, compared to previous studies (Ferreira et al., 2016). Lower DLB index might have been due to the small number of patients. This method has the advantage to capture multivariate features of the EEG recordings. This leads in general to more robust feature combination allowing for increased test re-test reliability. This particular algorithm of qEEG-SPR utilizes full spectrum analysis of inter-electrode covariances and direct spectral properties at individual electrodes. In this manner, the strategy captures the degrees of freedom related to both classical qEEG features and connectivity/coherence related features through the covariances. The connectivity/coherence related features are functionals of the covariances.
This study demonstrated the robustness and transferability of the qEEG dementia and DLB indices in an outpatient clinic, demonstrating the practical use of such technique for the diagnosis of dementia.
We also observed that the inclusion of patients with a previous history of head trauma, stroke, or neurosurgery might induce false positive results, especially for the DLB index. Indeed, previous evidence has shown that head trauma or chronic stroke can induce long-term changes in the EEG oscillatory activity, such as reduction of the mean alpha frequency or an increase of theta activity (Tebano et al., 1988; Chen et al., 2006; Gosselin et al., 2009; Petrovic et al., 2017; Livint Popa et al., 2020). Similar EEG changes have been evidenced in patients presenting with dementia. In Alzheimer disease, a generalized slowing of the EEG is observed at rest and is expressed by an increased power in the delta and theta frequency bands and a decreased power of the upper alpha and beta bands (Schreiter-Gasser et al., 1993; Huang et al., 2000; Caso et al., 2012). Early EEG slowing may be specific to MCI with Lewy Bodies compared to MCI with AD (Massa et al., 2020; Schumacher et al., 2020). Indeed, in MCI with AD patients, the slowing-down of the qEEG was less severe than in MCI with Lewy Bodies (Massa et al., 2020). These EEG slowing down are especially expressed by the lowering of the alpha/delta ratio. In MCI- DLB, such EEG slowing-down has been mainly observed in the centro-parietal, temporal, and occipital regions (Babiloni et al., 2011; Benz et al., 2014; Massa et al., 2020), although it seems that the slowing observed in the posterior regions might be particularly specific of DLB, compared to AD (Bonanni et al., 2008, 2015, 2016).
Cholinergic deficits, which are more severe and occur earlier in DLB compared to AD, may be the cause of the EEG slowing (Mesulam et al., 2004). EEG frequency is accelerated by cholinergic function and responds to therapy with acetylcholinesterase inhibitors in AD (Fogelson et al., 2003; Babiloni et al., 2013). In DLB, acetylcholinesterase inhibitors may improve global cognitive function, cognitive fluctuations, hallucinations and activities of daily living (Taylor et al., 2020), although only half of patients benefit from this type of treatment (McKeith et al., 2000; Mori et al., 2012; Stinton et al., 2015). These differences in qEEG as well as in EEG connectivity between DLB and AD would explain the strong accuracy of quantitative EEG analyses to differentiate DLB from AD or other dementia (Benz et al., 2014).
Excluding patients with a history of head trauma or stroke, the specificity of the DLB index greatly improved from 90 to 94%. Specificity is of particular importance in the diagnostic process of DLB as it indicates that 94% of the patients with a negative outcome, really do not suffer from DLB. The accuracy of the DLB index also greatly improved from 75 to 87.4%. Such data are similar to previous evidence showing that higher alpha power and lower delta power differentiate AD from DLB with sensitivity and specificity of 65–78% (Babiloni et al., 2017, 2018).
According to clinical criteria for DLB diagnosis, biomarkers are obtained by PET, SPECT, MRI, polysomnographic exams, or EEG. EEG analysis is considered as a supportive biomarker, meaning that EEG data is not considered as indicative as PET or SPECT reports. PET diagnosis of DLB has been shown to have a sensitivity of about 83–92% and a specificity of about 80–87%, similar to previously reported qEEG results (Minoshima et al., 2001; Ishii et al., 2007; Mosconi et al., 2008; Caminiti et al., 2019). Since qEEG has a good accuracy, a high sensitivity and specificity in diagnosing dementia and DLB, considerations could be made to evaluate the possibility to upgrade qEEG analyses from supportive biomarkers to indicative biomarkers. More studies with higher number of patients would be required. Moreover, qEEG analysis represents a diagnostic tool that is non-invasive for the patients, environment-friendly, has a low cost both for the hospital/clinic and patient and can be easily repeated several times a year. Moreover, commercially available software can be used to perform such qEEG-SPR analyses, in order to promote these analyses even in small clinical centers. In these times of pandemic, attention has been brought to patients’ protection and reduction of patients’ displacements to reduce exposure of sensitive populations of patients. Quantitative EEG is a diagnostic tool that can be used even in small clinic centers and could be used as a systematic screening tool for those patients who are suspected of dementia or DLB following neurologic exam and neuropsychological evaluation. Based on the spoke/hub organization of hospitals and clinical centers, patients could undergo neurologic exam, neuropsychological evaluation, and qEEG in spoke centers. Only in the case of positive EEG results, PET/SPECT or MRI could be prescribed in hub centers to confirm the diagnosis.
Our data did not show significant concordance between EEG results and neuropsychological scoring, maybe due to the low number of patients. However, we showed a tendency toward a negative correlation between the EEG Dementia Index and the MMSE scores: the higher the Dementia index, the lower the MMSE. In order to better define such relationship between the Dementia Index and gravity of dementia, such analyses should be reproduced on a larger sample of patients. Evidence has shown that neuropsychological data are highly relevant in dementia and DLB diagnosis (Benz et al., 2014; Zorick et al., 2020; Howard et al., 2021). According to the literature, DLB subjects would have better performance on recall but worse on praxis than patients with AD (Walker et al., 1997). In the early stages, DLB patients present with more visuospatial deficits, compared to AD patients, as shown with the Rosen drawing test (Yoshizawa et al., 2013). Indeed, visuospatial or constructional impairment is present in 74% of patients with early-stage pathologically confirmed DLB compared with 45% of those with AD (Tiraboschi et al., 2006). Moreover, the authors showed that, among clinical variables, history of visual hallucinations was the most specific symptom to DLB (99%), and visuospatial impairment was the most sensitive (74%) (Tiraboschi et al., 2006). MCI patients with AD might have more memory storage impairments, as shown by the Free and Cued Selective Recall Reminding Test (FCSRT), testing for verbal episodic memory (Sarazin et al., 2007). In our study, FCSRT showed a minimal concordance with the EEG results. Such analyses should be replicated on a larger group of patients to further investigate the potential of neuropsychological testing associated with qEEG analyses to improve the sensitivity and specificity of such screening method.
This study presented several limitations, the first of which being the small number of patients. We also showed that certain pathologies or conditions could confound DLB index, such as head trauma or stroke, showing the necessity for exclusion criteria before running qEEG testing. To address these issues, future studies should involve larger cohort of patients, including non-demented patients. Comorbidities should be evaluated to exclude patients with a history of head trauma or stroke. Neuropsychological data should be gathered in all patients to define whether the combination of clinical data, qEEG, and neuropsychological tests could further improve the sensitivity and specificity of differential dementia diagnosis.
This study confirmed previous findings that qEEG constitutes a highly sensitive and specific tool to perform diagnosis of dementia and differential diagnosis of DLB in the early phase of the disease [12,13,15]. Additional studies, with larger cohorts of patients and control subjects, should be conducted to further confirm the present results to assess whether qEEG algorithms, such as qEEG-SPR, could be upgraded from supportive to indicative biomarker in the process of DLB diagnosis, since its sensibility and specificity are similar to the ones of MRI and PET/SPECT analyses. Quantitative EEG is a non-invasive, low-cost, environment-friendly tool that can be easily installed and used in small clinical centers (spoke). EEG tools should thus be used to streamline the assessment process in dementia diagnosis: EEG exams should be run first and give an indication whether or not more invasive assessments should be undergone to further define the diagnosis. Such invasive assessments are often available only in major clinical centers (hub). Conversely, EEG analyses can be easily implemented in small memory clinics.
The original contributions presented in the study are included in the article/supplementary material, further inquiries can be directed to the corresponding author/s.
The studies involving human participants were reviewed and approved by Comitato Etico San Raffaele, San Raffaele Scientific Institute. The patients/participants provided their written informed consent to participate in this study.
SI: participated in study design, data collection, interpretation of data, and paper writing. EH: participated in data collection, data analyses, interpretation of data, and paper writing. AS: participated in data analyses. GN: participated in data analyses. FA: participated in study design, data interpretation, and paper writing. All authors contributed to the article and approved the submitted version.
The authors thank MentisCura for EEG data analyses and reports.
The authors declare that the research was conducted in the absence of any commercial or financial relationships that could be construed as a potential conflict of interest.
All claims expressed in this article are solely those of the authors and do not necessarily represent those of their affiliated organizations, or those of the publisher, the editors and the reviewers. Any product that may be evaluated in this article, or claim that may be made by its manufacturer, is not guaranteed or endorsed by the publisher.
Aarsland, D., Rongve, A., Nore, S. P., Skogseth, R., Skulstad, S., Ehrt, U., et al. (2008). Frequency and case identification of dementia with Lewy bodies using the revised consensus criteria. Dement. Geriatr. Cogn. Disord. 26, 445–452. doi: 10.1159/000165917
American Psychiatric Association and American Psychiatric Association (Eds.) (2013). Diagnostic and Statistical Manual of Mental Disorders: DSM-5. 5th. Washington, D.C: American Psychiatric Association.
Aoki, Y., Kazui, H., Pascal-Marqui, R. D., Ishii, R., Yoshiyama, K., Kanemoto, H., et al. (2019). EEG resting-state networks in dementia with Lewy bodies associated with clinical symptoms. Neuropsychobiology 77, 206–218. doi: 10.1159/000495620
Arvanitakis, Z., Shah, R. C., and Bennett, D. A. (2019). Diagnosis and Management of Dementia: review. JAMA 322, 1589–1599. doi: 10.1001/jama.2019.4782
Babiloni, C., De Pandis, M. F., Vecchio, F., Buffo, P., Sorpresi, F., Frisoni, G. B., et al. (2011). Cortical sources of resting state electroencephalographic rhythms in Parkinson’s disease related dementia and Alzheimer’s disease. Clin. Neurophysiol. 122, 2355–2364. doi: 10.1016/j.clinph.2011.03.029
Babiloni, C., Del Percio, C., Bordet, R., Bourriez, J.-L., Bentivoglio, M., Payoux, P., et al. (2013). Effects of acetylcholinesterase inhibitors and memantine on resting-state electroencephalographic rhythms in Alzheimer’s disease patients. Clin. Neurophysiol. 124, 837–850. doi: 10.1016/j.clinph.2012.09.017
Babiloni, C., Del Percio, C., Lizio, R., Noce, G., Cordone, S., Lopez, S., et al. (2017). Abnormalities of cortical neural synchronization mechanisms in patients with dementia due to Alzheimer’s and Lewy body diseases: an EEG study. Neurobiol. Aging 55, 143–158. doi: 10.1016/j.neurobiolaging.2017.03.030
Babiloni, C., Del Percio, C., Lizio, R., Noce, G., Lopez, S., Soricelli, A., et al. (2018). Abnormalities of resting state cortical EEG rhythms in subjects with mild cognitive impairment due to Alzheimer’s and Lewy body diseases. J. Alzheimers Dis. 62, 247–268. doi: 10.3233/JAD-170703
Benz, N., Hatz, F., Bousleiman, H., Ehrensperger, M. M., Gschwandtner, U., Hardmeier, M., et al. (2014). Slowing of EEG background activity in Parkinson’s and Alzheimer’s disease with early cognitive dysfunction. Front. Aging Neurosci. 6:314. doi: 10.3389/fnagi.2014.00314
Bonanni, L., Franciotti, R., Nobili, F., Kramberger, M. G., Taylor, J.-P., Garcia-Ptacek, S., et al. (2016). EEG markers of dementia with Lewy bodies: a multicenter cohort study. J. Alzheimers Dis. 54, 1649–1657. doi: 10.3233/JAD-160435
Bonanni, L., Franciotti, R., Onofrj, V., Anzellotti, F., Mancino, E., Monaco, D., et al. (2010). Revisiting P300 cognitive studies for dementia diagnosis: early dementia with Lewy bodies (DLB) and Alzheimer disease (AD). Neurophysiol. Clin. 40, 255–265. doi: 10.1016/j.neucli.2010.08.001
Bonanni, L., Perfetti, B., Bifolchetti, S., Taylor, J.-P., Franciotti, R., Parnetti, L., et al. (2015). Quantitative electroencephalogram utility in predicting conversion of mild cognitive impairment to dementia with Lewy bodies. Neurobiol. Aging 36, 434–445. doi: 10.1016/j.neurobiolaging.2014.07.009
Bonanni, L., Thomas, A., Tiraboschi, P., Perfetti, B., Varanese, S., and Onofrj, M. (2008). EEG comparisons in early Alzheimer’s disease, dementia with Lewy bodies and Parkinson’s disease with dementia patients with a 2-year follow-up. Brain J. Neurol. 131, 690–705. doi: 10.1093/brain/awm322
Briel, R. C., McKeith, I. G., Barker, W. A., Hewitt, Y., Perry, R. H., Ince, P. G., et al. (1999). EEG findings in dementia with Lewy bodies and Alzheimer’s disease. J. Neurol. Neurosurg. Psychiatry 66, 401–403. doi: 10.1136/jnnp.66.3.401
Caminiti, S. P., Sala, A., Iaccarino, L., Beretta, L., Pilotto, A., Gianolli, L., et al. (2019). Brain glucose metabolism in Lewy body dementia: implications for diagnostic criteria. Alzheimers Res. Ther. 11:20. doi: 10.1186/s13195-019-0473-4
Carlesimo, G. A., Caltagirone, C., and Gainotti, G. (1996). The mental deterioration battery: normative data, diagnostic reliability and qualitative analyses of cognitive impairment. The Group for the Standardization of the mental deterioration battery. Eur. Neurol. 36, 378–384. doi: 10.1159/000117297
Caso, F., Cursi, M., Magnani, G., Fanelli, G., Falautano, M., Comi, G., et al. (2012). Quantitative EEG and LORETA: valuable tools in discerning FTD from AD? Neurobiol. Aging 33, 2343–2356. doi: 10.1016/j.neurobiolaging.2011.12.011
Chen, X.-P., Tao, L.-Y., and Chen, A. C. N. (2006). Electroencephalogram and evoked potential parameters examined in Chinese mild head injury patients for forensic medicine. Neurosci. Bull. 22, 165–170.
Cooley, J. W., and Tukey, J. W. (1965). An algorithm for the machine calculation of complex Fourier series. Math. Comput. 19, 297–301.
Corsi, M. (1972). Human memory and the medial temporal region of the brain. Diss. Abstr. Int 34:891B.
Cummings, J., Aisen, P., Lemere, C., Atri, A., Sabbagh, M., and Salloway, S. (2021). Aducanumab produced a clinically meaningful benefit in association with amyloid lowering. Alzheimers Res. Ther. 13:98. doi: 10.1186/s13195-021-00838-z
Dauwan, M., Linszen, M. M. J., Lemstra, A. W., Scheltens, P., Stam, C. J., and Sommer, I. E. (2018). EEG-based neurophysiological indicators of hallucinations in Alzheimer’s disease: comparison with dementia with Lewy bodies. Neurobiol. Aging 67, 75–83. doi: 10.1016/j.neurobiolaging.2018.03.013
Engedal, K., Barca, M. L., Høgh, P., Bo Andersen, B., Winther Dombernowsky, N., Naik, M., et al. (2020). The power of EEG to predict conversion from mild cognitive impairment and subjective cognitive decline to dementia. Dement. Geriatr. Cogn. Disord. 49, 38–47. doi: 10.1159/000508392
Engedal, K., Snaedal, J., Hoegh, P., Jelic, V., Bo Andersen, B., Naik, M., et al. (2015). Quantitative EEG applying the statistical recognition pattern method: a useful tool in dementia diagnostic workup. Dement. Geriatr. Cogn. Disord. 40, 1–12. doi: 10.1159/000381016
Ferreira, D., Jelic, V., Cavallin, L., Oeksengaard, A.-R., Snaedal, J., Høgh, P., et al. (2016). Electroencephalography is a good complement to currently established dementia biomarkers. Dement. Geriatr. Cogn. Disord. 42, 80–92. doi: 10.1159/000448394
Fogelson, N., Kogan, E., Korczyn, A. D., Giladi, N., Shabtai, H., and Neufeld, M. Y. (2003). Effects of rivastigmine on the quantitative EEG in demented parkinsonian patients. Acta Neurol. Scand. 107, 252–255. doi: 10.1034/j.1600-0404.2003.00081.x
Folstein, M. F., Folstein, S. E., and McHugh, P. R. (1975). Mini-mental state. A practical method for grading the cognitive state of patients for the clinician. J. Psychiatr. Res. 12, 189–198. doi: 10.1016/0022-3956(75)90026-6
Gosselin, N., Lassonde, M., Petit, D., Leclerc, S., Mongrain, V., Collie, A., et al. (2009). Sleep following sport-related concussions. Sleep Med. 10, 35–46. doi: 10.1016/j.sleep.2007.11.023
Gudmundsson, S., Runarsson, T. P., Sigurdsson, S., Eiriksdottir, G., and Johnsen, K. (2007). Reliability of quantitative EEG features. Clin. Neurophysiol. 118, 2162–2171. doi: 10.1016/j.clinph.2007.06.018
Heaton, R., Chelune, G., Talley, J., and Kay, G. (1993). Wisconsin Card Sorting Test Manual: Revised and Expanded. 1993rd. Odessa, FL: Psychological Assessment Resources Inc.
Howard, E., Irwin, D. J., Rascovsky, K., Nevler, N., Shellikeri, S., Tropea, T. F., et al. (2021). Cognitive profile and markers of Alzheimer disease-type pathology in patients with Lewy body dementias. Neurology 96, e1855–e1864. doi: 10.1212/WNL.0000000000011699
Huang, C., Wahlund, L., Dierks, T., Julin, P., Winblad, B., and Jelic, V. (2000). Discrimination of Alzheimer’s disease and mild cognitive impairment by equivalent EEG sources: a cross-sectional and longitudinal study. Clin. Neurophysiol. 111, 1961–1967. doi: 10.1016/s1388-2457(00)00454-5
Hugo, J., and Ganguli, M. (2014). Dementia and cognitive impairment: epidemiology, diagnosis, and treatment. Clin. Geriatr. Med. 30, 421–442. doi: 10.1016/j.cger.2014.04.001
Ishii, K., Soma, T., Kono, A. K., Sofue, K., Miyamoto, N., Yoshikawa, T., et al. (2007). Comparison of regional brain volume and glucose metabolism between patients with mild dementia with lewy bodies and those with mild Alzheimer’s disease. J. Nucl. Med. 48, 704–711. doi: 10.2967/jnumed.106.035691
Jensen, A. R., and Rohwer, W. D. (1966). The Stroop color-word test: a review. Acta Psychol. 25, 36–93. doi: 10.1016/0001-6918(66)90004-7
Jobert, M., Wilson, F. J., Roth, T., Ruigt, G. S. F., Anderer, P., Drinkenburg, W. H. I. M., et al. (2013). Guidelines for the recording and evaluation of pharmaco-sleep studies in man: the international Pharmaco-EEG society (IPEG). Neuropsychobiology 67, 127–167. doi: 10.1159/000343449
Raven, J. (2003). “Raven progressive matrices,” in Handbook of Nonverbal Assessment. ed. R. S. McCallum (Boston, MA: Springer), 223–237.
Kai, T., Asai, Y., Sakuma, K., Koeda, T., and Nakashima, K. (2005). Quantitative electroencephalogram analysis in dementia with Lewy bodies and Alzheimer’s disease. J. Neurol. Sci. 237, 89–95. doi: 10.1016/j.jns.2005.05.017
Kaplan, E., Goodglass, H., Weintraub, S., and Goodglass, H. (1983). Boston Naming Test. Philadelphia: Lea & Febiger.
Kurita, A., Murakami, M., Takagi, S., Matsushima, M., and Suzuki, M. (2010). Visual hallucinations and altered visual information processing in Parkinson disease and dementia with Lewy bodies. Mov. Disord. 25, 167–171. doi: 10.1002/mds.22919
Law, Z. K., Todd, C., Mehraram, R., Schumacher, J., Baker, M. R., LeBeau, F. E. N., et al. (2020). The role of EEG in the diagnosis, prognosis and clinical correlations of dementia with Lewy bodies—a systematic review. Diagnostics 10:616. doi: 10.3390/diagnostics10090616
Lisko, I., Kulmala, J., Annetorp, M., Ngandu, T., Mangialasche, F., and Kivipelto, M. (2021). How can dementia and disability be prevented in older adults: where are we today and where are we going? J. Intern. Med. 289, 807–830. doi: 10.1111/joim.13227
Livint Popa, L., Dragos, H., Pantelemon, C., Verisezan Rosu, O., and Strilciuc, S. (2020). The role of quantitative EEG in the diagnosis of neuropsychiatric disorders. J. Med. Life 13, 8–15. doi: 10.25122/jml-2019-0085
Massa, F., Meli, R., Grazzini, M., Famà, F., De Carli, F., Filippi, L., et al. (2020). Utility of quantitative EEG in early Lewy body disease. Parkinsonism Relat. Disord. 75, 70–75. doi: 10.1016/j.parkreldis.2020.05.007
McKeith, I. G., Boeve, B. F., Dickson, D. W., Halliday, G., Taylor, J.-P., Weintraub, D., et al. (2017). Diagnosis and management of dementia with Lewy bodies: fourth consensus report of the DLB consortium. Neurology 89, 88–100. doi: 10.1212/WNL.0000000000004058
McKeith, I., Del Ser, T., Spano, P., Emre, M., Wesnes, K., Anand, R., et al. (2000). Efficacy of rivastigmine in dementia with Lewy bodies: a randomised, double-blind, placebo-controlled international study. Lancet Lond. Engl. 356, 2031–2036. doi: 10.1016/S0140-6736(00)03399-7
Mesulam, M., Shaw, P., Mash, D., and Weintraub, S. (2004). Cholinergic nucleus basalis tauopathy emerges early in the aging-MCI-AD continuum. Ann. Neurol. 55, 815–828. doi: 10.1002/ana.20100
Miceli, G., Laudanna, A., Burani, C., and Capasso, R. (1994). Batteria per l’analisi dei Deficit Afasici. B.A.D.A. Available at: https://www.iris.unisa.it/handle/11386/3828878?mode=full.19#.W1CYCvkzaUk (Accessed July 19, 2018).
Minoshima, S., Foster, N. L., Sima, A. A., Frey, K. A., Albin, R. L., and Kuhl, D. E. (2001). Alzheimer’s disease versus dementia with Lewy bodies: cerebral metabolic distinction with autopsy confirmation. Ann. Neurol. 50, 358–365. doi: 10.1002/ana.1133
Mok, W., Chow, T. W., Zheng, L., Mack, W. J., and Miller, C. (2004). Clinicopathological concordance of dementia diagnoses by community versus tertiary care clinicians. Am. J. Alzheimers Dis. Other Dement. 19, 161–165. doi: 10.1177/153331750401900309
Mori, E., Ikeda, M., and Kosaka, K., Donepezil-DLB Study Investigators (2012). Donepezil for dementia with Lewy bodies: a randomized, placebo-controlled trial. Ann. Neurol. 72, 41–52. doi: 10.1002/ana.23557
Mosconi, L., Tsui, W. H., Herholz, K., Pupi, A., Drzezga, A., Lucignani, G., et al. (2008). Multicenter standardized 18F-FDG PET diagnosis of mild cognitive impairment, Alzheimer’s disease, and other dementias. J. Nucl. Med. 49, 390–398. doi: 10.2967/jnumed.107.045385
Novelli, G., Papagno, C., Capitani, E., Laiacona, M., Vallar, G., and Cappa, S. (1986). Tre test clinici di ricerca e produzione lessicale. Taratura su soggetti normal. Arch. Psicol. Neurol. Psichiatr. 47, 477–506.
Ommundsen, N., Engedal, K., and Øksengård, A. R. (2011). Validity of the quantitative EEG statistical pattern recognition method in diagnosing Alzheimer’s disease. Dement. Geriatr. Cogn. Disord. 31, 195–201. doi: 10.1159/000324878
Orsini, A., Grossi, D., Capitani, E., Laiacona, M., Papagno, C., and Vallar, G. (1987). Verbal and spatial immediate memory span: normative data from 1355 adults and 1112 children. Ital. J. Neurol. Sci. 8, 539–548. doi: 10.1007/BF02333660
Petrovic, J., Milosevic, V., Zivkovic, M., Stojanov, D., Milojkovic, O., Kalauzi, A., et al. (2017). Slower EEG alpha generation, synchronization and “flow”-possible biomarkers of cognitive impairment and neuropathology of minor stroke. PeerJ 5:e3839. doi: 10.7717/peerj.3839
Rasmussen, J., and Langerman, H. (2019). Alzheimer’s disease – why we need early diagnosis. Degener. Neurol. Neuromuscul. Dis. 9, 123–130. doi: 10.2147/DNND.S228939
Reitan, R. M. (1955). Investigation of the validity of Halstead’s measures of biological intelligence. A.M.A. Arch. Neurol. Psychiatry 73, 28–35. doi: 10.1001/archneurpsyc.1955.02330070030005
Sarazin, M., Berr, C., De Rotrou, J., Fabrigoule, C., Pasquier, F., Legrain, S., et al. (2007). Amnestic syndrome of the medial temporal type identifies prodromal AD: a longitudinal study. Neurology 69, 1859–1867. doi: 10.1212/01.wnl.0000279336.36610.f7
Schreiter-Gasser, U., Gasser, T., and Ziegler, P. (1993). Quantitative EEG analysis in early onset Alzheimer’s disease: a controlled study. Electroencephalogr. Clin. Neurophysiol. 86, 15–22. doi: 10.1016/0013-4694(93)90063-2
Schumacher, J., Taylor, J.-P., Hamilton, C. A., Firbank, M., Cromarty, R. A., Donaghy, P. C., et al. (2020). Quantitative EEG as a biomarker in mild cognitive impairment with Lewy bodies. Alzheimers Res. Ther. 12:82. doi: 10.1186/s13195-020-00650-1
Snaedal, J., Johannesson, G. H., Gudmundsson, T. E., Blin, N. P., Emilsdottir, A. L., Einarsson, B., et al. (2012). Diagnostic accuracy of statistical pattern recognition of electroencephalogram registration in evaluation of cognitive impairment and dementia. Dement. Geriatr. Cogn. Disord. 34, 51–60. doi: 10.1159/000339996
Snaedal, J., Johannesson, G. H., Gudmundsson, T. E., Gudmundsson, S., Pajdak, T. H., and Johnsen, K. (2010). The use of EEG in Alzheimer’s disease, with and without scopolamine - a pilot study. Clin. Neurophysiol. 121, 836–841. doi: 10.1016/j.clinph.2010.01.008
Stinton, C., McKeith, I., Taylor, J.-P., Lafortune, L., Mioshi, E., Mak, E., et al. (2015). Pharmacological Management of Lewy Body Dementia: a systematic review and meta-analysis. Am. J. Psychiatry 172, 731–742. doi: 10.1176/appi.ajp.2015.14121582
Stylianou, M., Murphy, N., Peraza, L. R., Graziadio, S., Cromarty, R., Killen, A., et al. (2018). Quantitative electroencephalography as a marker of cognitive fluctuations in dementia with Lewy bodies and an aid to differential diagnosis. Clin. Neurophysiol. 129, 1209–1220. doi: 10.1016/j.clinph.2018.03.013
Taylor, J.-P., McKeith, I. G., Burn, D. J., Boeve, B. F., Weintraub, D., Bamford, C., et al. (2020). New evidence on the management of Lewy body dementia. Lancet Neurol. 19, 157–169. doi: 10.1016/S1474-4422(19)30153-X
Tebano, M. T., Cameroni, M., Gallozzi, G., Loizzo, A., Palazzino, G., Pezzini, G., et al. (1988). EEG spectral analysis after minor head injury in man. Electroencephalogr. Clin. Neurophysiol. 70, 185–189. doi: 10.1016/0013-4694(88)90118-6
Tiraboschi, P., Salmon, D. P., Hansen, L. A., Hofstetter, R. C., Thal, L. J., and Corey-Bloom, J. (2006). What best differentiates Lewy body from Alzheimer’s disease in early-stage dementia? Brain. J. Neurol. 129, 729–735. doi: 10.1093/brain/awh725
Toledo, J. B., Cairns, N. J., Da, X., Chen, K., Carter, D., Fleisher, A., et al. (2013). Clinical and multimodal biomarker correlates of ADNI neuropathological findings. Acta Neuropathol. Commun. 1:65. doi: 10.1186/2051-5960-1-65
van Dellen, E., de Waal, H., van der Flier, W. M., Lemstra, A. W., Slooter, A. J. C., Smits, L. L., et al. (2015). Loss of EEG network efficiency is related to cognitive impairment in dementia with Lewy bodies. Mov. Disord. 30, 1785–1793. doi: 10.1002/mds.26309
van der Zande, J. J., Gouw, A. A., van Steenoven, I., Scheltens, P., Stam, C. J., and Lemstra, A. W. (2018). EEG characteristics of dementia with Lewy bodies, Alzheimer’s disease and mixed pathology. Front. Aging Neurosci. 10:190. doi: 10.3389/fnagi.2018.00190
van der Zande, J. J., Gouw, A. A., van Steenoven, I., van de Beek, M., Scheltens, P., Stam, C. J., et al. (2020). Diagnostic and prognostic value of EEG in prodromal dementia with Lewy bodies. Neurology 95, e662–e670. doi: 10.1212/WNL.0000000000009977
Walker, Z., Allen, R. L., Shergill, S., and Katona, C. L. (1997). Neuropsychological performance in Lewy body dementia and Alzheimer’s disease. Br. J. Psychiatry J. Ment. Sci. 170, 156–158. doi: 10.1192/bjp.170.2.156
Walker, M. P., Ayre, G. A., Cummings, J. L., Wesnes, K., McKeith, I. G., O’Brien, J. T., et al. (2000a). Quantifying fluctuation in dementia with Lewy bodies, Alzheimer’s disease, and vascular dementia. Neurology 54, 1616–1625. doi: 10.1212/wnl.54.8.1616
Walker, M. P., Ayre, G. A., Perry, E. K., Wesnes, K., McKeith, I. G., Tovee, M., et al. (2000b). Quantification and characterization of fluctuating cognition in dementia with Lewy bodies and Alzheimer’s disease. Dement. Geriatr. Cogn. Disord. 11, 327–335. doi: 10.1159/000017262
Walker, Z., Possin, K. L., Boeve, B. F., and Aarsland, D. (2015). Lewy body dementias. Lancet Lond. Engl. 386, 1683–1697. doi: 10.1016/S0140-6736(15)00462-6
Wechsler, D. (1955). Manual for the WECHSLER Adult Intelligence Scale. Oxford, England: Psychological Corp.
Yoshizawa, H., Vonsattel, J. P. G., and Honig, L. S. (2013). Early neuropsychological discriminants for Lewy body disease: an autopsy series. J. Neurol. Neurosurg. Psychiatry 84, 1326–1330. doi: 10.1136/jnnp-2012-304381
Keywords: dementia, quantitative electroencephalography, cognition, EEG, dementia with Lewy bodies
Citation: Iannaccone S, Houdayer E, Spina A, Nocera G and Alemanno F (2023) Quantitative EEG for early differential diagnosis of dementia with Lewy bodies. Front. Psychol. 14:1150540. doi: 10.3389/fpsyg.2023.1150540
Received: 25 January 2023; Accepted: 31 March 2023;
Published: 20 April 2023.
Edited by:
Elena Salobrar-Garcia, Complutense University of Madrid, SpainReviewed by:
Tommaso Piccoli, University of Palermo, ItalyCopyright © 2023 Iannaccone, Houdayer, Spina, Nocera and Alemanno. This is an open-access article distributed under the terms of the Creative Commons Attribution License (CC BY). The use, distribution or reproduction in other forums is permitted, provided the original author(s) and the copyright owner(s) are credited and that the original publication in this journal is cited, in accordance with accepted academic practice. No use, distribution or reproduction is permitted which does not comply with these terms.
*Correspondence: Elise Houdayer, aG91ZGF5ZXIuZWxpc2VAaHNyLml0
Disclaimer: All claims expressed in this article are solely those of the authors and do not necessarily represent those of their affiliated organizations, or those of the publisher, the editors and the reviewers. Any product that may be evaluated in this article or claim that may be made by its manufacturer is not guaranteed or endorsed by the publisher.
Research integrity at Frontiers
Learn more about the work of our research integrity team to safeguard the quality of each article we publish.