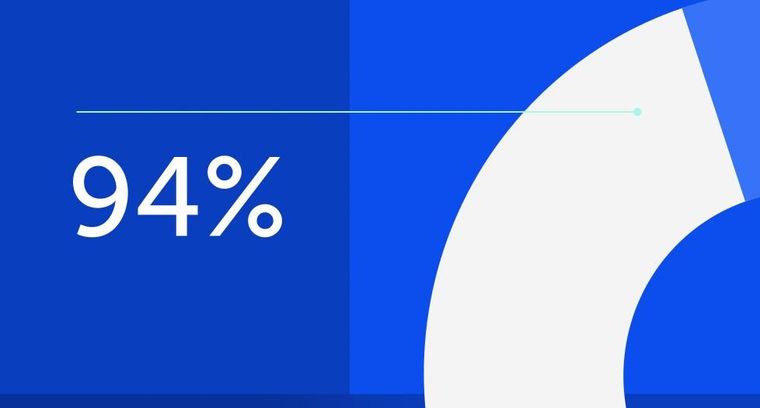
94% of researchers rate our articles as excellent or good
Learn more about the work of our research integrity team to safeguard the quality of each article we publish.
Find out more
ORIGINAL RESEARCH article
Front. Psychol., 17 April 2023
Sec. Human-Media Interaction
Volume 14 - 2023 | https://doi.org/10.3389/fpsyg.2023.1125031
This article is part of the Research TopicTrust in Automated VehiclesView all 16 articles
The present study surveyed actual extensive users of SAE Level 2 partially automated cars to investigate how driver’s characteristics (i.e., socio-demographics, driving experience, personality), system performance, perceived safety, and trust in partial automation influence use of partial automation. 81% of respondents stated that they use their automated car with speed (ACC) and steering assist (LKA) at least 1–2 times a week, and 84 and 92% activate LKA and ACC at least occasionally. Respondents positively rated the performance of Adaptive Cruise Control (ACC) and Lane Keeping Assistance (LKA). ACC was rated higher than LKA and detection of lead vehicles and lane markings was rated higher than smooth control for ACC and LKA, respectively. Respondents reported to primarily disengage (i.e., turn off) partial automation due to a lack of trust in the system and when driving is fun. They rarely disengaged the system when they noticed they become bored or sleepy. Structural equation modelling revealed that trust had a positive effect on driver’s propensity for secondary task engagement during partially automated driving, while the effect of perceived safety was not significant. Regarding driver’s characteristics, we did not find a significant effect of age on perceived safety and trust in partial automation. Neuroticism negatively correlated with perceived safety and trust, while extraversion did not impact perceived safety and trust. The remaining three personality dimensions ‘openness’, ‘conscientiousness’, and ‘agreeableness’ did not form valid and reliable scales in the confirmatory factor analysis, and could thus not be subjected to the structural equation modelling analysis. Future research should re-assess the suitability of the short 10-item scale as measure of the Big-Five personality traits, and investigate the impact on perceived safety, trust, use and use of automation.
SAE Level 2 partially automated driving has been implemented in passenger cars since 2015 combining Adaptive Cruise Control (ACC) and Lane Keeping Assist (LKA). Such systems automate braking, acceleration and lane keeping, while drivers are required to monitor the system whenever the automated driving features are engaged even if feet are off the pedals and the driver is not steering (SAE International, 2021).
Ample scientific studies provide evidence for trust predicting the behavioral intention to use automated cars (Kaur and Rampersad, 2018; Xu et al., 2018; Kettles and Van Belle, 2019; Zhang et al., 2020; Du et al., 2021; Benleulmi and Ramdani, 2022; Kenesei et al., 2022; Meyer-Waarden and Cloarec, 2022; Foroughi et al., 2023). Overtrust can lead to misuse, and undertrust can lead to disuse (Lee and Moray, 1994; Lee, 2008). Overtrust and misuse are key safety concerns for (current) lower automation levels. Undertrust and disuse can diminish the projected benefits of higher automation levels. In our recent interview study with 103 users of Tesla’s Autopilot and Full-Self-Driving (FSD) Beta system, overtrust in Level 2 capability was associated with eyes-off, mind-off and fatigued driving (e.g., drivers actively manipulating the steering wheel, and falling asleep behind the steering wheel with Autopilot engaged) (Nordhoff et al., 2023). Disuse refers to not using automation when it would, in fact, be beneficial (Lee, 2008). Drivers decided to disengage automation, and take back control in anticipation of system failure and lack of trust in the capability of the automation to safely execute a manoeuvre (Dixit et al., 2016; Gershon et al., 2021). Other reasons for disuse were driver’s general negative predispositions towards automation or annoyances caused by automation (‘bells and whistles’ principle), the need to disengage automation, false alarms, and low perceived reliability (De Winter et al., 2022).
It is commonly assumed that (perceived) safety of automated cars is a key requirement for acceptance (Osswald et al., 2012; Dixit et al., 2016; Pyrialakou et al., 2020; Cao et al., 2021; Manfreda et al., 2021). Scientific evidence supporting the role of (perceived) safety as direct predictor of acceptance and use of automated cars is inconclusive. In our previous study, perceived safety did not influence actual use of partial automation (Nordhoff et al., 2021), while in other studies it did influence the intention to use automated cars (Montoro et al., 2019; Detjen et al., 2020; Koul and Eydgahi, 2020).
Technology acceptance models, such as the Unified Theory of Acceptance and Use of Technology (UTAUT) (Venkatesh et al., 2012), assume that performance expectancy (or perceived usefulness) is a key factor impacting the intention to use and actual use of technology. This assumption is supported by scientific evidence showing that the (expected) benefits of automation related to safety, comfort, and efficiency are key drivers impacting the decision to use automated cars (Nordhoff et al., 2020).
Informed by the results of this literature review, the present study derives testable hypotheses as shown by Table 1.
Technology acceptance models also assume that external variables (e.g., driver’s characteristics and system performance) influence the independent factors in the models (Nordhoff et al., 2016; Venkatesh et al., 2016). Driver’s characteristics including demographics and personality can influence trust in and perceived safety of partial automation, and thereby affect use of partial automation. Such relations are still poorly understood (see Modliński et al., 2022). In their theoretical model for trust in automated systems, Hoff and Bashir (2013) assumed that age, gender, and personality influence individual’s level of trust in automated systems. Experiments and surveys provided ambiguous empirical evidence on the relationship between age, gender, and trust in automation. It was found that trust in automated cars decreased (Dikmen and Burns, 2017), or increased with age (Zhang et al., 2020), or that the relationship between age and trust was not significant (Molnar et al., 2018). Yu et al. (2021) revealed that middle-aged drivers reported higher trust in Advanced Driver Assistance Systems (ADAS) than younger drivers. Moody et al. (2020) found that younger people were more likely to have favorable perceptions of safety of automated driving technology.
Males were more likely to report higher ratings of trust in and perceived safety of automated cars. Yu et al. (2021) observed that females had higher levels of trust in ADAS than males. Higher-educated people were more likely than lower-educated people to report more favorable perceptions of safety of automated driving technology (Moody et al., 2020).
Walker et al. (2018) showed substantial changes in driver’s trust ratings after an on-road experience of a partially automated car, with trust ratings decreasing after the experience. Metz et al. (2021) found that trust and perceived safety both increased after experiencing automated driving functions, with respondents spending more time on secondary tasks and less on monitoring the road ahead. In Montoro et al. (2019), driving experience (in years) and experience with driving crashes was positively associated with the perceived safety of automated vehicles. Cao et al. (2021) revealed that familiarity and driving experience correlated positively with the perceived safety of automated cars.
Previous research has shown that people’s attitudes and behavior are influenced by their personality (Devaraj et al., 2008). Personality is commonly captured by the ‘Big Five’ or OCEAN model, representing one of the most comprehensive and parsimonious personality measures (John and Srivastava, 1999; Lovik et al., 2017). The influence of personality on trust and perceived safety in partially automated cars is still poorly understood. Zhang et al. (2020) found a positive effect of the personality trait ‘openness’ on respondents’ trust in automated cars, while the personality trait ‘neuroticism’ had negative effects on trust in automated cars. Li et al. (2020) found a negative relationship between extraversion, openness, and trust in automated cars, respectively, while neuroticism, agreeableness, and conscientiousness did not have significant impacts on trust. Merritt and Ilgen (2008) found that extraverted people were more likely to have high trust in automated driving systems.
Studies have also shown that trust is a function of system performance. Choi and Ji (2015) found positive effects of system transparency, technical competence, and situation management on trust. Wilson et al. (2020) found that trust in partially automated cars was associated with the capability of automated cars to manage corners and regulate speed in response to other traffic. In the study of Dikmen and Burns (2017) drivers who experienced unexpected system performance were less likely to trust Autopilot.
Informed by the results of this literature review, the present study derives additional testable hypotheses as shown by Table 2.
The present study presents the research model in Figure 1.
Data on actual behavior and perception of drivers in partially automated cars is rare. Published studies are largely based on simulators rather than on-road pilots, collecting data from naïve and inexperienced respondents without sustained use of partial automation (De Winter et al., 2014; Montoro et al., 2019; Gershon et al., 2021). The present study probed the perception and behaviour of actual drivers of partially automated cars, through an online survey. We collected information of consumers with a substantial experience with partial automation, and asked them to reflect on their actual on-road behavior regarding automation use. In addition, we probed their perception to investigate trust and perceived safety, and other factors motivating automation use. The following three main research questions were addressed:
i. To what extent do perceived safety and trust, perceived benefits, and secondary task engagement influence use of partial automation?
ii. To what extent do perceived safety and trust influence the perceived benefits of partial automation, and secondary task engagement?
iii. To what extent do driver’s characteristics (i.e., socio-demographics, driving experience, personality), and system performance influence perceived safety and trust in partial automation?
To target current users of partially automated cars, we distributed the survey at Tesla’s supercharging stations near Utrecht, Dordrecht, and Amsterdam in the Netherlands in the form of a QR code. The link was further distributed in specialized communities and forums (e.g., Tesla Owners clubs and forums). Furthermore, the survey was shared in car-and mobility-related forums and groups of Reddit and Facebook, respectively. The authors of the present study further shared the link to the questionnaire on LinkedIn, and an anonymous link to access the questionnaire was sent to employees of Toyota Motor Europe using internal communication mailing.
The questionnaire was implemented using the questionnaire tool Qualtrics (www.qualtrics.com). The questionnaire instructions informed the respondents that it would take around 20 min to complete the questionnaire and that the work is organized by Delft University of Technology in the Netherlands. To improve data quality, Qualtrics applied several technologies preventing respondents from taking the survey more than once, detecting suspicious, non-human (i.e., bot) responses, and preventing search engines from indexing the survey.
Prior to participation in the questionnaire, respondents received a description about the functionality of partially automated cars to ensure that respondents had a sufficient understanding of partially automated cars. This description informed respondents that partially automated cars automate the acceleration, braking, and/or steering of the car. Furthermore, respondents were informed that partially automated cars have gas and brake pedals and a steering wheel and that they as human drivers have to supervise the performance of the car in order to resume manual driving. Their hands have to remain on or periodically touch the steering wheel, and their eyes remain on the road.
After respondents received the instructions, they were asked to provide their written consent to participate in the study. They were asked to declare that they have been informed in a clear manner about the nature and method of the research as described in the instructions at the beginning of the questionnaire. They were further asked to agree, fully and voluntarily, to participate in this research study. They were further informed that they retain the right to withdraw their consent and that they can stop participation in the study at any time. Finally, they were informed that their data will be treated anonymously in scientific publications, and will not be passed to third parties without their permission (Q1).
After respondents provided their written consent to participate in the study, they were asked to provide information about their age (Q2), gender (Q3), highest level of education completed (Q4), and personality (Q5–Q14). They were also asked to indicate access to a valid driver license (Q15), age of car (Q16), brand (Q17), car model (Q18), access to Lane Departure Warning (LDW), Lane Keeping Assist (LKA), and Adaptive Cruise Control (ACC) in their cars (Q19.1–Q19.3), and frequency of activating those systems (Q20.1–Q21.3). Those respondents who indicated that they had access to all three systems (i.e., LDW, LKA, and ACC) or a combination of two of the three systems were allowed to continue with the questionnaire. Respondents were asked to what extent the pandemic COVID–19 affected their mileage in the last 12 months as driver (Q21) and to select the number of kilometers/miles they drove in the last 12 months as driver (Q22). Respondents were also asked how often they used their partially automated car with speed and steering support (Q24). Respondents who did not indicate access to three of these systems or a combination of two of these were directed to the final questionnaire section on the evaluation of Human Machine Interfaces (HMIs) because it was expected that valid responses could be obtained for these questions without sustained use of automation. The questions measuring the evaluation of the HMIs will be subjected to further analysis in future studies. The personality questions were operationalized by the short 10-item measurement scale (Rammstedt and John, 2007), which was also applied in other studies (Kraus et al., 2020).
With the next questions (Q23.1–Q23.7), respondents were asked to report the number of times they have been involved in risky driving-related situations in their lifetime as driver. These risky driving-related situations covered loss of concentration (Q23.1), minor loss of vehicular control while driving (Q23.2), driving fines (Q23.3), nearly having an accident (Q23.4), accident leading to material damage (Q23.5), accident in which the airbag was deployed (Q23.6), and accident leading to personal injury (Q23.7). The questions measuring the involvement in risky driving-related situations were taken from Deffenbacher et al. (2001); Delhomme et al. (2012); Disassa and Kebu (2019).
Respondents were asked to indicate on a Likert scale from strongly disagree (1) to strongly agree (5) to what extent their partially automated car typically accelerates and decelerates smoothly (Q25), makes smooth, gentle steering corrections while they drive actively (Q26), detects lane markings on the roadway (Q27), detects the car ahead in their lane (Q28), starts to change lanes, and then returns to its lane halfway through the process (Q29), and asks them to take over control when they are not ready to take over control (Q30). The formulation of the questions was based on Reagan et al. (2020), while the questions Q29–Q30 were self-developed.
With the next section, respondents were asked to indicate on a scale from strongly disagree (1) to strongly agree (5) to what extent they trust their partially automated car to maintain speed and distance to the car ahead (Q31), and keeping the car centered in the lane (Q32) (Reagan et al., 2020). Next, respondents were asked to indicate to what extent they feel hesitant about activating the partially automated driving mode from time to time (Q33) (self-developed). Next, respondents were asked to rate to what extent they engage in other activities while driving their partially automated car (Q34) (Gold et al., 2015; Xu et al., 2018; Mason et al., 2020), and to what extent they always know when their car is in partially automated driving mode (Q35) (Körber, 2018). With the next self-developed questions, respondents were asked to rate to what extent the surrounding elements detected by their partially automated car are always clear to them (Q36), to what extent their partially automated car reminds them to take back full control (Q37), to keep their hands on the steering wheel (Q38), and to help them to keep using the partially automated car in the manner as advised by the manual (Q39). Next, respondents were asked to rate their trust in their partially automated car (Q40) (Choi and Ji, 2015). The next questions asked respondents to indicate to what extent they are unwilling to hand over control to their partially automated car from time to time (Q41), and to monitor the performance of their partially automated car most of the time (Q42).
With the next questions, respondents were asked to indicate how often they talk to fellow travelers (Q43.1 / Q44.1), watch videos or TV shows (Q43.2 / Q44.2), use the phone for calls (Q43.3 / Q44.3), texting (Q43.4/ Q44.4), music selection (Q43.5/ Q44.5), and setting/updating navigation (Q43.6/ Q44.6), reading text from book/phone (Q43.7/ Q44.7), eating and drinking (Q43.8/ Q44.8), monitoring the road ahead (Q43.9/ Q44.9), sleeping (Q43.10/ Q44.10), and engaging in other activities (Q43.11 / Q44.11) during both manual and partially automated driving. Questions Q43.11/Q44.11 were open-ended questions (“Other”), which were removed from the analysis of the present study.
On a scale from strongly disagree (1) to strongly agree (5), respondents were asked to rate to what extent they use their partially automated car to reach their destination more safely (Q45), comfortably (Q46) (Nordhoff et al., 2020), pleasurable (Q47) (self-developed), and to use their time for other activities unrelated to driving (Q48) (Nordhoff et al., 2020).
On a scale from strongly disagree (1) to strongly agree (5), respondents were further asked to indicate the reasons for disengaging partially automated driving when they notice they become sleepy (Q49), they do not trust it (Q50), they become bored (Q51), driving is fun (Q52), it is not necessary to use it (Q53), and when it is distracting or confusing (Q54). The questions Q49–Q52 were based on the studies of Lin et al. (2018) and Van Huysduynen et al. (2018) and the questions Q53–Q54 were self-developed.
Furthermore, respondents had to indicate to what extent they feel safe (Q55), relaxed (Q56), anxious (Q57) (Xu et al., 2018), and bored most of the time (Q58) when partially automated driving was engaged (Zoellick et al., 2019). With the next question, respondents were asked to indicate to what extent they are concerned about their general safety most of the time (Q59) (Xu et al., 2018). Next, respondents had to rate to what extent they entrust the safety of a close relative to their partially automated car (Q60) (Wien, 2019).
An ordinal Likert-scale was applied, and the order of the questions was randomized to prevent order effects.
The data was analyzed in three steps.
In the first step, descriptive statistics (i.e., means, standard deviations, and frequencies) of the questionnaire items that were subjected to the analysis of the present paper were computed.
In the second step, a confirmatory factor was conducted to estimate the measurement model, i.e., the measurement relations between the questionnaire items and their underlying latent constructs by assessing the internal consistency reliability, (i.e., Cronbach’s alpha), composite reliability, convergent validity, and discriminant validity. Convergent validity was assessed by the following four criteria: (1) Factor loadings should be significant, exceeding the recommended threshold of 0.60 on their scales; (2) Average variance extracted (AVE) should exceed the value of 0.50; (3) Construct reliability (CR) should exceed the threshold of 0.60; and (4) Cronbach’s alpha values should exceed the threshold of 0.60 (Hair, 2009).
Discriminant validity (i.e., uni-dimensionality of latent constructs) is established if the square root of the AVE of each latent construct exceeds the correlation coefficient between two latent constructs. The fit of the model was deemed acceptable if the Comparative Fit Index (CFI) and Tucker Lewis Index (TLI) ≥ 0.90, Root Mean Square Error of Approximation (RMSEA) ≤ 0.08, and the Standardized Root Mean Square Residual (SRMR) ≤ 0.06 (Hair, 2009).
In the third step, a structural equation modeling analysis was performed to assess the structural path relationships between the latent factors in the model. This step involves testing the structural path relationships between the latent factors in the model, reporting the standardized regression coefficients, standard error terms, significance levels, and variance accounted for in the latent constructs.
Between November 24, 2020, and January 30, 2021, 1,557 questionnaires were completed. On average, respondents needed 86.12 min to complete the survey. Respondents who were identified as bots, who did not agree to participate in the study, did not have access to a valid driver license, reported to be younger than 18 years old, and who reported to never and less than monthly use their automated car with speed and steering support were removed from the analysis. In addition, only the responses from respondents reporting to have access to both ACC and LKA representing the functionality of partially automated cars, and who stated to own a car aged 4 years or less were subjected to the analysis given that partially automated driving was only introduced in 2015. “I prefer not to respond” and “Not applicable to me” responses were defined as missing values. 628 responses were maintained for the analysis.
The profile of the respondents is presented in Table 3. 51% of respondents were between 36 and 55 years, and 81% of respondents were male. 77% of respondents reported to have a Bachelor or Master degree. 46% of respondents experienced loss of concentration, 23% nearly had an accident, and 19% received driving fines more than five times in their lifetime as driver. 93% of respondents never experienced accidents leading to personal injury, 90% accidents in which the airbag was deployed, and 36% accidents leading to material damage. 81% of respondents reported that they used their automated car with speed (ACC) and steering assist (LKA) at least 1–2 times a week, and 84 and 92% stated to activate LKA and ACC at least occasionally, respectively.
Figure 2 shows that 76% of respondents reported to disengage partial automation when they do not trust it ( M = 3.94, SD = 1.20), and 68% when driving is fun. Only 22% of respondents disengaged the system when respondents noticed they become sleepy ( M = 2.40, SD = 1.28), and 26% when they noticed they become bored ( M = 2.51, SD = 1.20).
Figure 2. Driver-initiated disengagement of partial automation; stacked bar plots presenting relative proportions of questions pertaining to disengaging partial automation sorted from highest (top) to lowest (bottom). PAD = partially automated driving.
As shown by Figure 3, respondents provided the strongest agreement with the partially automated car detecting the car ahead in their lane ( M = 4.75, SD = 0.54), the lane markings on the roadway ( M = 4.48, SD = 0.69), with 96 and 94% indicating their agreement with these questions. The weakest agreement was obtained for the partially automated car typically starting to change lanes and then returning to its lane halfway throughout the process ( M = 2.15, SD = 1.06), with 16 and 12% of respondents agreeing with these questions.
Figure 3. System performance; stacked bar plots presenting relative proportions of questions pertaining to system performance sorted from highest (top) to lowest (bottom). PAC = partially automated car.
A confirmatory factor analysis was conducted to estimate the relationships between the latent constructs and their observed variables (i.e., questionnaire items). Table 4 shows the standardized factor loadings for all constructs after omitting questionnaire items from the analysis whose loadings did not meet the recommended threshold of 0.60. From the five personality dimensions only neuroticism and extraversion proved to be valid and reliable. The loadings of the indicator variables of the four remaining personality constructs were lower than the recommended threshold of 0.60, and thus dropped from the analysis. As two of the three items loading on driver engagement were below 0.60, the latent construct driver engagement was dropped from the analysis. Cronbach’s alpha and composite reliability was larger than 0.60 for all constructs, which demonstrates sufficient internal consistency reliability of the constructs. The average variance extracted (AVE) exceeded the recommended threshold of 0.50 for all constructs except for extraversion (0.493), and trust (0.459). As shown by Table 5, the square root of the AVE for all constructs was larger than the Spearman correlation coefficients between the constructs, which demonstrates that the latent constructs are sufficiently distinct (discriminant validity). The fit of the measurement model was acceptable, with the indexes exceeding the recommended thresholds [Comparative Fit Index (CFI) = 0.954, Tucker Lewis Index (TLI) = 0.934, Root Mean Square Error of Approximation (RMSEA) = 0.050]. The χ2 test statistic [χ2/df (degrees of freedom)] was 2.286, falling below the recommended threshold of <2.5.
Table 4. Confirmatory factor analysis (M, means, SD, standard deviation, ƛ, lambda, ⍺, Cronbach’s alpha, CR, Composite reliability, AVE, Average variance extracted).
A structural equation modelling analysis was conducted to assess how:
i. Perceived safety, trust, perceived benefits, and secondary task engagement influence use of partial automation;
ii. Perceived safety and trust influence secondary task engagement, and the perceived benefits of partial automation;
iii. Driver’s characteristics (i.e., socio-demographics, driving experience, personality), and system performance influence perceived safety and trust in partial automation.
The structural equation model consisted of valid and reliable latent constructs identified in the confirmatory factor analysis, and single-item indicators measuring driving experience, system performance, and disengaging partial automation. These items did not form valid and reliable composite scales in the confirmatory factor analysis. The use of single item measures in structural equation models is acceptable if single item variables measure specific aspects or behaviors (Hair, 2009)—a condition that is met in the present study. The results of the structural equation modelling analyses are presented in Tables 6, 7, and will be discussed in the subsequent section.
Table 6. Structural equation modelling results (i.e., β = standardized beta coefficients, significance level = *** p < 0.001, **p < 0.01, *p < 0.05, all other correlations are not significant, R2 = variance explained), main model.
Table 7. Structural equation modelling results (i.e., β = standardized beta coefficients, significance level = ***p < 0.001, **p < 0.01, *p < 0.05, all other correlations are not significant, R2 = variance explained), main model + external variables.
This study presents results of an online questionnaire with respondents with actual extensive experience with SAE Level 2 partially automated cars. The main objectives were to investigate how driver’s individual characteristics (i.e., socio-demographics, driving experience, personality), system performance, perceived safety and trust in partial automation influence use of partial automation.
The analysis of descriptive statistics revealed that regarding system performance, the strongest agreement was obtained for the partially automated car detecting the car ahead in their lane. A weaker agreement was obtained for the partially automated car accelerating and decelerating smoothly. Both responses relate to ACC where apparently detection is rated higher than smooth control. The second-highest mean rating was obtained for detecting the lane markings on the roadway, while a weaker agreement was found for the partially automated car making smooth and gentle steering corrections. These responses relate to LKA where again, detection is rated higher than smooth control. The weakest agreement was obtained for the partially automated car typically starting to change lanes and then returning to its lane halfway throughout the process, and for asking respondents to take over control when they are not ready to take over control, with 12% and 16% of respondents (strongly) agreeing with the corresponding questions.
Our study revealed that the number one reason for driver-initiated disengagements was a lack of trust in the system, while sleepiness was a minor reason for driver-initiated disengagements: 76% of respondents agreed (strongly) with disengaging due to a lack of trust, while only 22% agreed (strongly) to disengage when sleepy and 62% even disagreed (strongly) to disengage when sleepy. Van Huysduynen et al. (2018) and Wilson et al. (2020) revealed that respondents most frequently disengaged automation due to a lack of trust in the partially automated car. Lack of trust pertained to the ability of the automation to handle merging manoeuvres, to recognize an obstacle, to handle a situation in poor weather conditions, or because respondents felt uncomfortable travelling too close to another vehicle (Wilson et al., 2020). Other studies associated a lack of trust with technology disuse (Lewandowsky et al., 2000; Choi and Ji, 2015; Lee et al., 2021). Disengaging Tesla’s Autopilot system in situations when drivers became sleepy has been reported by Van Huysduynen et al. (2018). However, in our study only 22% report to disengage partially automated driving when sleepy. This represents a serious safety concern and future studies should investigate which ‘sleepiness level’ (e.g., mind-wandering, nodding off, sleeping) represents a safety concern that diminishes driver performance in critical take-over situations.
The structural equation modelling analysis provided evidence to support several study hypotheses in Tables 1, 2. The analysis revealed that trust had a positive impact on driver’s propensity for secondary task engagement. This corresponds with previous research, which has shown that overtrust in automation was associated with unsafe secondary task engagement during partially automated driving (Casner et al., 2016; Endsley, 2017; Banks et al., 2018; Lin et al., 2018; Casner and Hutchins, 2019; Wilson et al., 2020; Kim et al., 2021). We also found a strong impact of trust on the factor ‘perceived benefits’, showing that an increase in trust in partial automation increases the likelihood to appreciate the perceived benefits of partial automation. It is interesting to note that perceived safety did not influence secondary task engagement. Among the three factors – perceived benefits, secondary task engagement, and trust – only trust did influence actual use of partial automation. Previous research has shown that the perceived benefits of automation strongly influenced the intention to use automated cars (which is regarded as one of the most important predictors for actual use) (Nordhoff et al., 2020).
The structural equation modelling analysis demonstrated a small impact of gender on perceived safety, with males reporting higher levels of perceived safety than females. This aligns with Moody et al. (2020) where males had more favorable perceptions of safety than females. The impact of age on perceived safety and trust was not significant. Other studies found mixed results, where Zhang et al. (2020) found a positive relation between age and trust, whereas in (Moody et al., 2020; Best et al., 2021), younger people had more optimistic safety perceptions than elderly people. Our study findings about age and gender are in line with research investigating attitudes towards automation, which has provided ambiguous evidence about the role of gender and age on key beliefs (e.g., perceived usefulness, ease of use, intention to use) towards automated cars. A common conception held in industry is that users of (automated) cars should be targeted based on key socio-demographic attributes (Christensen et al., 2022). Future research should critically re-assess the role of age and gender, using a representative and balanced sample of the general population of car drivers, considering other personality traits being pivotal for the human-machine collaboration and acceptance of partial automation (e.g., locus of control) e.g., (see Syahrivar et al., 2021).
Our study found a small positive effect of the frequency of experiencing accidents leading to personal injury on trust. This finding is plausible as respondents with severe experiences with accidents are more likely to appreciate the safety benefits of automated cars, and/or may even use these cars to be safer, both objectively and subjectively. Studies have shown more positive (safety) perceptions among individuals being involved in risky driving behavior and accidents (Turner and McClure, 2003; Holland and Hill, 2007; Montoro et al., 2019). It is plausible that accidents with partially automated cars contribute to lower trust in partially automated cars. Lee et al. (2021) revealed that failure of automation contributed to a decrease in trust, which, however, was quickly rebuilt. We also found a positive effect of driving mileage on trust, which shows that experience has a positive effect on trust in automation.
We found a small negative effect of neuroticism on perceived safety, and a moderate effect on trust in partial automation. Likewise, Zhang et al. (2020) revealed that neuroticism had a negative effect on trust in automated cars. The results of the confirmatory factor analysis supported the validity and reliability of two of five personality dimensions: extraversion and neuroticism. This corresponds with the study of Kraus et al. (2020), which reported low Cronbach’s alpha levels for openness, conscientiousness, and agreeableness. Studies applying a longer-item scale to measure the Big-Five personality dimensions produced internally consistent and valid personality dimensions (Braitman and Braitman, 2017; Niranjan et al., 2022).
The study revealed that the ACC, and LKA performance of partial automation did impact respondent’s perceived safety and trust in partially automated cars. This implies that enhancing the reliability and possibly the intuitiveness of the automation controlling the longitudinal and lateral part of the driving task may be an effective means to influence perceived safety and trust in automation. Research has shown that respondents were more comfortable when the automated car had a more defensive driving style, which was characterized by lower speed, and smoother accelerations and decelerations (Beggiato et al., 2020). It should be studied which driving style of the automated vehicle can produce calibrated levels of perceived safety and trust, matching the actual capabilities and limitations of the system.
We also studied the specific conditions for disengaging the system. When trust was high, the likelihood to disengage the system when it was not necessary to use, and when it was distracting or confusing to use decreased. This may imply that respondents with high levels of trust may have a higher level of technological understanding and knowledge of where and when to use automation. We also found that when perceived safety was high, the propensity to disengage the system when it was distracting or confusing, and when drivers noticed they become bored was lower. It should be assessed to what extent boredom during partially automated driving represents a safety-critical physiological state that may compromise driver’s safety, e.g., take-over performance.
First, researchers and respondents may not be able to clearly discriminate between perceived safety and trust. This is illustrated by the labelling of the construct ‘trust in safety’ (Kettles and Van Belle, 2019; Morrison and Belle, 2020), and the measurement of perceived safety by items pertaining to trust in automation (Koul and Eydgahi, 2020). Perceived safety is closely related to perceived risk, and can even be used interchangeably. Perceived risk is generally defined as the level of risk experienced by users of driving automation (He et al., 2022). Finally, risk is also closely related to trust (Hoff and Bashir, 2015; Lazanyi and Maraczi, 2017; Zhang et al., 2019; Tenhundfeld et al., 2020). We recommend future research to re-assess the operationalization of perceived safety and trust in automation.
Second, studies should consider the development of personality scales tailored to the context of road vehicle automation, and investigate the impact on perceived safety, trust, and automation use. The items of existing personality scales such as the Big5 are stated in very generic terms, making the direct transfer to the context of automation challenging.
Third, we also recommend future research to integrate self-efficacy (i.e., individual’s beliefs in own capabilities to produce certain effects) (Bandura and Wessels, 1994), and privacy concerns in models with personality, perceived safety, trust, and automation use. These factors may gain in importance with greater connectivity in automated cars (Duboz et al., 2022). In the study of Zhang et al. (2019) privacy concerns were not associated with trust in automated cars. In another study, privacy concerns had negative effects on trust in automated cars (Meyer-Waarden and Cloarec, 2022), and neurotic individuals were less comfortable with data transmitting (Kyriakidis et al., 2015). Du et al. (2021) found that respondents with higher perceived capabilities to use automated cars had higher trust in automated cars.
Fourth, through on-road observation studies, future research should also relate subjective feelings of safety to more objective indicators measuring perceived safety or risks, e.g., unexpected system disengagements, false alarms (Bauchwitz and Cummings, 2020), and frequency of driver-initiated disengagements.
Fifth, future research should perform validation checks, e.g., by assessing drivers’ knowledge about these systems, to verify whether the system drivers said that they had were available in their cars. It cannot be ruled out that respondents misunderstood our descriptions of the system functionality of partially automated driving.
Sixth, our study is one of the few studies surveying actual users of SAE Level 2 partially automated cars. As the present study mainly used U.S. American communities and forums, the dataset is not fully representative of the European population of drivers of partially automated cars. Respondents may well have an above average interest in and enthusiasm for automated driving (Nordhoff et al., 2021). It is also possible that respondents engaged in socially desirable response behavior, being overly positive to address the challenges associated with partially automated driving, especially Tesla Autopilot (Banks et al., 2018; Lin et al., 2018; Casner and Hutchins, 2019; Wilson et al., 2020). Future research should investigate perceived safety, trust and automation use among a more balanced and representative sample of European car drivers.
The present study surveyed actual extensive users of SAE Level 2 partially automated cars to investigate how driver’s characteristics (i.e., socio-demographics, driving experience, personality), system performance, perceived safety and trust in partial automation influence use of partial automation. People reporting a higher driving mileage had a higher level of trust in partially automated cars, while the effect on perceived safety was not significant. Neuroticism was negatively related to perceived safety, but not to trust in partial automation. System performance (ACC and LKA functionality) influenced perceptions of safety and trust in partial automation. Trust also influenced disuse of partial automation in situations when the system was not necessary, distracting or confusing to use. Respondents positively rated the performance of Adaptive Cruise Control (ACC) and Lane Keeping Assistance (LKA). ACC was rated higher than LKA and detection of lead vehicles and lane markings was rated higher than smooth control for ACC and LKA, respectively. Respondents reported to primarily disengage (i.e., turn off) partial automation due to a lack of trust in the system and when driving is fun. They rarely disengaged the system when they noticed they become bored or sleepy. Structural equation modelling revealed that trust had a positive effect on secondary task engagement during partially automated driving, while the effect of perceived safety on secondary task engagement was not significant. Regarding driver’s characteristics, we did not find a significant effect of age on perceived safety and trust in partial automation. Neuroticism had a negative effect on perceived safety and trust, while extraversion did not impact perceived safety and trust.
The raw data supporting the conclusions of this article will be made available by the authors, without undue reservation.
Ethical review and approval was not required for the study on human participants in accordance with the local legislation and institutional requirements. The patients/participants provided their written informed consent to participate in this study.
SN: conceptualization, methodology, software, validation, formal analysis, investigation, resources, data curation, writing—original draft, writing—review and editing and visualization. JS: writing—review and editing. XH: writing—review and editing. AG: writing review and editing. RH: writing—review and editing, supervision, project administration, funding acquisition. All authors contributed to the article and approved the submitted version.
This research was conducted as part of the project “Investigating Trust in Automation,” co-funded by Toyota Motor Europe NV/SA. The funders had no role in data analysis, decision to publish, or preparation of the manuscript.
AG was employed by Toyota Motor Europe NV/SA.
The remaining authors declare that the research was conducted in the absence of any commercial or financial relationships that could be construed as a potential conflict of interest.
All claims expressed in this article are solely those of the authors and do not necessarily represent those of their affiliated organizations, or those of the publisher, the editors and the reviewers. Any product that may be evaluated in this article, or claim that may be made by its manufacturer, is not guaranteed or endorsed by the publisher.
Bandura, A., and Wessels, S. (1994). “Self-efficacy” in Encyclopedia of Human Behavior. ed. V. S. Ramachaudran , vol. 4 (New York: Academic Press), 71, 71–81.
Banks, V. A., Eriksson, A., O’Donoghue, J., and Stanton, N. A. (2018). Is partially automated driving a bad idea? Observations from an on-road study. Appl. Ergon. 68, 138–145. doi: 10.1016/j.apergo.2017.11.010
Bauchwitz, B. C., and Cummings, M. L. (2020). Evaluating the reliability of tesla model 3 driver assist functions. Available at: https://www.roadsafety.unc.edu/wp-content/uploads/2020/11/R27_interim-deliverable_DMS_experiment_1_summary_CSCRS_report.pdf
Beggiato, M., Hartwich, F., Roßner, P., Dettmann, A., Enhuber, S., Pech, T., et al. (2020). “KomfoPilot—comfortable automated driving” in Smart automotive mobility: reliable technology for the mobile human. ed. G. Meixner (Cham: Springer International Publishing), 71–154.
Benleulmi, A. Z., and Ramdani, B. (2022). Behavioural intention to use fully autonomous vehicles: instrumental, symbolic, and affective motives. Transport. Res. F: Traffic Psychol. Behav. 86, 226–237. doi: 10.1016/j.trf.2022.02.013
Best, R., Fraade-Blanar, L., and Blumenthal, M. S. (2021). Perception of safety of automated vehicles: Age and the influence of the source and content of safety-related messages, No. TRBAM-21-01919.
Braitman, K. A., and Braitman, A. L. (2017). Patterns of distracted driving behaviors among young adult drivers: exploring relationships with personality variables. Transport. Res. F: Traffic Psychol. Behav. 46, 169–176. doi: 10.1016/j.trf.2017.01.015
Cao, J., Lin, L., Zhang, J., Zhang, L., Wang, Y., and Wang, J. (2021). The development and validation of the perceived safety of intelligent connected vehicles scale. Accid. Anal. Prev. 154:106092. doi: 10.1016/j.aap.2021.106092
Casner, S. M., and Hutchins, E. L. (2019). What do we tell the drivers? Toward minimum driver training standards for partially automated cars. J. Cogn. Eng. Decis. Making 13, 55–66. doi: 10.1177/1555343419830901
Casner, S. M., Hutchins, E. L., and Norman, D. (2016). The challenges of partially automated driving. Commun. ACM 59, 70–77. doi: 10.1145/2830565
Choi, J. K., and Ji, Y. G. (2015). Investigating the importance of trust on adopting an autonomous vehicle. Int. J. Hum. Comput. Interact. 31, 692–702. doi: 10.1080/10447318.2015.1070549
Christensen, H. R., Nexø, L. A., Pedersen, S., and Breengaard, M. H. (2022). The lure and limits of smart cars: visual analysis of gender and diversity in car branding. Sustainability 14:6906. doi: 10.3390/su14116906
De Winter, J. C. F., Happee, R., Martens, M. H., and Stanton, N. A. (2014). Effects of adaptive cruise control and highly automated driving on workload and situation awareness: a review of the empirical evidence. Transport. Res. F: Traffic Psychol. Behav. 27, 196–217. doi: 10.1016/j.trf.2014.06.016
De Winter, J. C. F., Petermeijer, S. M., and Abbink, D. A. (2022). Shared control versus traded control in driving: a debate around automation pitfalls. Ergonomics (just-accepted), 1–43. doi: 10.1080/00140139.2022.2153175
Deffenbacher, J. L., Lynch, R. S., Oetting, E. R., and Yingling, D. A. (2001). Driving anger: correlates and a test of state-trait theory. Personal. Individ. Differ. 31, 1321–1331. doi: 10.1016/S0191-8869(00)00226-9
Delhomme, P., Chaurand, N., and Paran, F. (2012). Personality predictors of speeding in young drivers: anger vs. sensation seeking. Transport. Res. F: Traffic Psychol. Behav. 15, 654–666. doi: 10.1016/j.trf.2012.06.006
Detjen, H., Pfleging, B., and Schneegas, S. (2020). “A wizard of oz study to understand non-driving related activities, trust, and acceptance of automated vehicles.” in 12th International Conference on Automotive User Interfaces and Interactive Vehicular Applications, 19–29.
Devaraj, S., Easley, R. F., and Crant, J. M. (2008). Research note: how does personality matter? Relating the fve-factor model to technology acceptance and use. Inf. Syst. Res. 19, 93–105. doi: 10.1287/isre.1070.0153
Dikmen, M., and Burns, C. (2017). “Trust in autonomous vehicles: the case of tesla autopilot and summon.” in 2017 IEEE International Conference on Systems, Man, and Cybernetics (SMC). IEEE, 1093–1098.
Disassa, A., and Kebu, H. (2019). Psychosocial factors as predictors of risky driving behavior and accident involvement among drivers in Oromia region, Ethiopia. Heliyon 5:e01876. doi: 10.1016/j.heliyon.2019.e01876
Dixit, V. V., Chand, S., and Nair, D. J. (2016). Autonomous vehicles: disengagements, accidents and reaction times. PLoS One 11:e0168054. doi: 10.1371/journal.pone.0168054
Du, H., Zhu, G., and Zheng, J. (2021). Why travelers trust and accept self-driving cars: an empirical study. Travel Behav. Soc. 22, 1–9. doi: 10.1016/j.tbs.2020.06.012
Duboz, A., Mourtzouchou, A., Grosso, M., Kolarova, V., Cordera, R., Nägele, S., et al. (2022). Exploring the acceptance of connected and automated vehicles: focus group discussions with experts and non-experts in transport. Transport. Res. F: Traffic Psychol. Behav. 89, 200–221. doi: 10.1016/j.trf.2022.06.013
Endsley, M. R. (2017). Autonomous driving systems: a preliminary naturalistic study of the tesla model S. J. Cogn. Eng. Decis. Making 11, 225–238. doi: 10.1177/1555343417695197
Foroughi, B., Nhan, P. V., Iranmanesh, M., Ghobakhloo, M., Nilashi, M., and Yadegaridehkordi, E. (2023). Determinants of intention to use autonomous vehicles: findings from PLS-SEM and ANFIS. J. Retail. Consum. Serv. 70:103158. doi: 10.1016/j.jretconser.2022.103158
Gershon, P., Seaman, S., Mehler, B., Reimer, B., and Coughlin, J. (2021). Driver behavior and the use of automation in real-world driving. Accid. Anal. Prev. 158:106217. doi: 10.1016/j.aap.2021.106217
Gold, C., Körber, M., Hohenberger, C., Lechner, D., and Bengler, K. (2015). Trust in automation–before and after the experience of take-over scenarios in a highly automated vehicle. Procedia Manuf. 3, 3025–3032. doi: 10.1016/j.promfg.2015.07.847
He, X., Stapel, J., Wang, M., and Happee, R. (2022). Modelling perceived risk and trust in driving automation reacting to merging and braking vehicles. Transport. Res. F: Traffic Psychol. Behav. 86, 178–195. doi: 10.1016/j.trf.2022.02.016
Hoff, K., and Bashir, M. (2013). “A theoretical model for trust in automated systems” in CHI'13 extended abstracts on human factors in computing systems. eds. M. Beaudouin-Lafon, P. Baudisch, and W. E. Mackay (New York: Association for Computing Machinery), 115–120.
Hoff, K. A., and Bashir, M. (2015). Trust in automation: integrating empirical evidence on factors that influence trust. Hum. Factors 57, 407–434. doi: 10.1177/0018720814547570
Holland, C., and Hill, R. (2007). The effect of age, gender and driver status on pedestrians’ intentions to cross the road in risky situations. Accid. Anal. Prev. 39, 224–237. doi: 10.1016/j.aap.2006.07.003
John, O. P., and Srivastava, S. (1999). “The big five trait taxonomy: history, measurement, and theoretical perspectives” in Handbook of personality: Theory and research. 2nd ed (New York, NY: Guilford Press), 102–138.
Kaur, K., and Rampersad, G. (2018). Trust in driverless cars: investigating key factors influencing the adoption of driverless cars. J. Eng. Technol. Manag. 48, 87–96. doi: 10.1016/j.jengtecman.2018.04.006
Kenesei, Z., Ásványi, K., Kökény, L., Jászberényi, M., Miskolczi, M., Gyulavári, T., et al. (2022). Trust and perceived risk: how different manifestations affect the adoption of autonomous vehicles. Transp. Res. A Policy Pract. 164, 379–393. doi: 10.1016/j.tra.2022.08.022
Kettles, N., and Van Belle, J. P. (2019). Investigation into the antecedents of autonomous car acceptance using an enhanced UTAUT model. in 2019 International Conference on Advances in Big Data, Computing and Data Communication Systems (icABCD). IEEE, 1–6.
Kim, H., Song, M., and Doerzaph, Z. (2021). Is driving automation used as intended? Real-world use of partially automated driving systems and their safety consequences. Transp. Res. Rec. 2676, 30–37. doi: 10.1177/03611981211027150
Körber, M. (2018). “Theoretical considerations and development of a questionnaire to measure trust in automation.” in Proceedings of the 20th Congress of the International Ergonomics Association (IEA 2018) Volume VI: Transport Ergonomics and Human Factors (TEHF), Aerospace Human Factors and Ergonomics 20. Springer International Publishing, 13–30.
Koul, S., and Eydgahi, A. (2020). The impact of social influence, technophobia, and perceived safety on autonomous vehicle technology adoption. Period. Polytech. Transp. Eng. 48, 133–142.
Kraus, J., Scholz, D., and Baumann, M. (2020). What’s driving me? Exploration and validation of a hierarchical personality model for trust in automated driving. Hum. Factors 63, 1076–1105. doi: 10.1177/0018720820922653
Kyriakidis, M., Happee, R., and De Winter, J. C. F. (2015). Public opinion on automated driving: results of an international questionnaire study among 5000 respondents. Transportation Research Part F: Traffic Psychology & Behaviour 32, 127–140. doi: 10.1016/j.trf.2015.04.014
Lazanyi, K., and Maraczi, G. (2017). “Dispositional trust – Do we trust autonomous cars?” in Paper presented at the 2017 IEEE 15th international symposium on intelligent systems and informatics (SISY).
Lee, J. D. (2008). Review of a pivotal human factors article: humans and automation: use, misuse, disuse, abuse. Hum. Factors 50, 404–410. doi: 10.1518/001872008X288547
Lee, J., Abe, G., Sato, K., and Itoh, M. (2021). Developing human-machine trust: impacts of prior instruction and automation failure on driver trust in partially automated vehicles. Transport. Res. F: Traffic Psychol. Behav. 81, 384–395. doi: 10.1016/j.trf.2021.06.013
Lee, J. D., and Moray, N. (1994). Trust, self-confidence, and operators’ adaptation to automation. Int. J. Hum. Comput. Stud. 40, 153–184. doi: 10.1006/ijhc.1994.1007
Lewandowsky, S., Mundy, M., and Tan, G. P. (2000). The dynamics of trust: comparing humans to automation. J. Exp. Psychol. Appl. 6, 104–123. doi: 10.1037//1076-898x.6.2.104
Li, W., Yao, N., Shi, Y., Nie, W., Zhang, Y., Li, X., et al. (2020). Personality openness predicts driver trust in automated driving. Automotive Innov. 3, 3–13. doi: 10.1007/s42154-019-00086-w
Lin, R., Ma, L., and Zhang, W. (2018). An interview study exploring tesla drivers’ behavioural adaptation. Appl. Ergon. 72, 37–47. doi: 10.1016/j.apergo.2018.04.006
Lovik, A., Verbeke, G., and Molenberghs, G. (2017). Evaluation of a very short test to measure the big five personality factors on a Flemish sample. J. Psychol. Educ. Res. 25, 7–17.
Manfreda, A., Ljubi, K., and Groznik, A. (2021). Autonomous vehicles in the smart city era: an empirical study of adoption factors important for millennials. Int. J. Inf. Manag. 58:102050. doi: 10.1016/j.ijinfomgt.2019.102050
Mason, J., Classen, S., Wersal, J., and Sisiopiku, V. P. (2020). Establishing face and content validity of a survey to assess users’ perceptions of automated vehicles. Transp. Res. Rec. 2674, 538–547. doi: 10.1177/0361198120930225
Merritt, S. M., and Ilgen, D. R. (2008). Not all trust is created equal: dispositional and history-based trust in human-automation interactions. Hum. Factors 50, 194–210. doi: 10.1518/001872008X288574
Metz, B., Wörle, J., Hanig, M., Schmitt, M., Lutz, A., and Neukum, A. (2021). Repeated usage of a motorway automated driving function: automation level and behavioural adaptation. Transp. Res. Part F Traffic Psychol. Behav. 81, 82–100. doi: 10.1016/j.trf.2021.05.017
Meyer-Waarden, L., and Cloarec, J. (2022). “Baby, you can drive my car”: psychological antecedents that drive consumers’ adoption of AI-powered autonomous vehicles. Technovation 109:102348. doi: 10.1016/j.technovation.2021.102348
Modliński, A., Gwiaździński, E., and Karpińska-Krakowiak, M. (2022). The effects of religiosity and gender on attitudes and trust toward autonomous vehicles. J. High Technol. Managem. Res. 33:100426. doi: 10.1016/j.hitech.2022.100426
Molnar, L. J., Ryan, L. H., Pradhan, A. K., Eby, D. W., Louis, R. M. S., and Zakrajsek, J. S. (2018). Understanding trust and acceptance of automated vehicles: an exploratory simulator study of transfer of control between automated and manual driving. Transport. Res. F: Traffic Psychol. Behav. 58, 319–328. doi: 10.1016/j.trf.2018.06.004
Montoro, L., Useche, S. A., Alonso, F., Lijarcio, I., Bosó-Seguí, P., and Martí-Belda, A. (2019). Perceived safety and attributed value as predictors of the intention to use autonomous vehicles: a national study with Spanish drivers. Saf. Sci. 120, 865–876. doi: 10.1016/j.ssci.2019.07.041
Moody, J., Bailey, N., and Zhao, J. (2020). Public perceptions of autonomous vehicle safety: an international comparison. Saf. Sci. 121, 634–650. doi: 10.1016/j.ssci.2019.07.022
Morrison, G., and Belle, J. P. V., Customer Intentions Towards Autonomous Vehicles in South Africa: An Extended UTAUT Model (2020). 10th international conference on cloud computing. Data Sci. Eng. 2020, 525–531. doi: 10.1109/Confluence47617.2020.9057821
Niranjan, S., Gabaldon, J., Hawkins, T. G., Gupta, V. K., and McBride, M. (2022). The influence of personality and cognitive failures on distracted driving behaviors among young adults. Transport. Res. F: Traffic Psychol. Behav. 84, 313–329. doi: 10.1016/j.trf.2021.12.001
Nordhoff, S., Lee, J. D., Calvert, S. C,, Berge, S., Hagenzieker, M., and Happee, R. (2023). (Mis-)use of standard Autopilot and Full Self-Driving (FSD) Beta: Results from interviews with users of Tesla’s FSD Beta. Front. Psychol. 14.
Nordhoff, S., Louw, T., Innamaa, S., Lehtonen, E., Beuster, A., Torrao, G., et al. (2020). Using the UTAUT2 model to explain public acceptance of conditionally automated (L3) cars: a questionnaire study among 9,118 car drivers from eight European countries. Transport. Res. F: Traffic Psychol. Behav. 74, 280–297. doi: 10.1016/j.trf.2020.07.015
Nordhoff, S., Stapel, J., He, X., Gentner, A., and Happee, R. (2021). Perceived safety and trust in SAE level 2 partially automated cars: results from an online questionnaire. PLoS One 16:e0260953. doi: 10.1371/journal.pone.0260953
Nordhoff, S., Van Arem, B., and Happee, R. (2016). Conceptual model to explain, predict, and improve user acceptance of driverless podlike vehicles. Transp. Res. Rec. 2602, 60–67. doi: 10.3141/2602-08
Osswald, S., Wurhofer, D., Trösterer, S., Beck, E., and Tscheligi, M. (2012). “Predicting information technology usage in the car: towards a car technology acceptance model.” in Proceedings of the 4th International Conference on Automotive User Interfaces and Interactive Vehicular Applications, 51–58.
Pyrialakou, V. D., Gkartzonikas, C., Gatlin, J. D., and Gkritza, K. (2020). Perceptions of safety on a shared road: driving, cycling, or walking near an autonomous vehicle. J. Saf. Res. 72, 249–258. doi: 10.1016/j.jsr.2019.12.017
Rammstedt, B., and John, O. P. (2007). Measuring personality in one minute or less: a 10-item short version of the big five inventory in English and German. J. Res. Pers. 41, 203–212. doi: 10.1016/j.jrp.2006.02.001
Reagan, I. J., Cicchino, J. B., and Kidd, D. G. (2020). Driver acceptance of partial automation after a brief exposure. Transport. Res. F: Traffic Psychol. Behav. 68, 1–14. doi: 10.1016/j.trf.2019.11.015
SAE International . (2021). Taxonomy and definitions for terms related to driving automation Systems for on-Road Motor Vehicles. Warrendale, PA: SAE International.
Syahrivar, J., Gyulavári, T., Jászberényi, M., Ásványi, K., Kökény, L., and Chairy, C. (2021). Surrendering personal control to automation: appalling or appealing? Transport. Res. F: Traffic Psychol. Behav. 80, 90–103. doi: 10.1016/j.trf.2021.03.018
Tenhundfeld, N. L., de Visser, E. J., Ries, A. J., Finomore, V. S., and Tossell, C. C. (2020). Trust and distrust of automated parking in a tesla model X. Hum. Factors 62, 194–210. doi: 10.1177/0018720819865412
Turner, C., and McClure, R. (2003). Age and gender differences in risk-taking behaviour as an explanation for high incidence of motor vehicle crashes as a driver in young males. Inj. Control. Saf. Promot. 10, 123–130. doi: 10.1076/icsp.10.3.123.14560
Van Huysduynen, H. H., Terken, J., and Eggen, B. (2018). “Why disable the autopilot?” in Proceedings of the 10th International Conference on Automotive User Interfaces and Interactive Vehicular Applications, 247–257.
Venkatesh, V., Thong, J. Y., and Xu, X. (2012). Consumer acceptance and use of information technology: extending the unified theory of acceptance and use of technology. MIS Q. 36, 157–178. doi: 10.2307/41410412
Venkatesh, V., Thong, J. Y., and Xu, X. (2016). Unified theory of acceptance and use of technology: a synthesis and the road ahead. J. Assoc. Inf. Syst. 17, 328–376. doi: 10.17705/1jais.00428
Walker, F., Boelhouwer, A., Alkim, T., Verwey, W. B., and Martens, M. H. (2018). Changes in trust after driving level 2 automated cars. J. Adv. Transp. 1045186. doi: 10.1155/2018/1045186
Wien, J. (2019). An assessment of the willingness to choose a self-driving bus for an urban trip: a public transport user’s perspective.
Wilson, K. M., Yang, S., Roady, T., Kuo, J., and Lenné, M. G. (2020). Driver trust & mode confusion in an on-road study of level-2 automated vehicle technology. Saf. Sci. 130:104845. doi: 10.1016/j.ssci.2020.104845
Xu, Z., Zhang, K., Min, H., Wang, Z., Zhao, X., and Liu, P. (2018). What drives people to accept automated vehicles? Findings from a field experiment. Transp. Res. Part C: Emerg. Technol. 95, 320–334. doi: 10.1016/j.trc.2018.07.024
Yu, B., Bao, S., Zhang, Y., Sullivan, J., and Flannagan, M. (2021). Measurement and prediction of driver trust in automated vehicle technologies: an application of hand position transition probability matrix. Transp. Res. Part C Emerg. Technol. 124:102957. doi: 10.1016/j.trc.2020.102957
Zhang, T., Tao, D., Qu, X., Zhang, X., Lin, R., and Zhang, W. (2019). The roles of initial trust and perceived risk in public’s acceptance of automated vehicles. Transp. Res. Part C Emerg. Technol. 98, 207–220. doi: 10.1016/j.trc.2018.11.018
Zhang, T., Tao, D., Qu, X., Zhang, X., Zeng, J., Zhu, H., et al. (2020). Automated vehicle acceptance in China: social influence and initial trust are key determinants. Transp. Res. Part C Emerg. Technol. 112, 220–233. doi: 10.1016/j.trc.2020.01.027
Zoellick, J. C., Kuhlmey, A., Schenk, L., Schindel, D., and Blüher, S. (2019). Amused, accepted, and used? Attitudes and emotions towards automated vehicles, their relationships, and predictive value for usage intention. Transport. Res. F: Traffic Psychol. Behav. 65, 68–78. doi: 10.1016/j.trf.2019.07.009
Keywords: partial automation, system performance, driver-initiated disengagements, perceived safety, trust, personality
Citation: Nordhoff S, Stapel J, He X, Gentner A and Happee R (2023) Do driver’s characteristics, system performance, perceived safety, and trust influence how drivers use partial automation? A structural equation modelling analysis. Front. Psychol. 14:1125031. doi: 10.3389/fpsyg.2023.1125031
Received: 15 December 2022; Accepted: 06 March 2023;
Published: 17 April 2023.
Edited by:
Sebastian Hergeth, BMW (Germany), GermanyReviewed by:
Francesco N. Biondi, University of Windsor, CanadaCopyright © 2023 Nordhoff, Stapel, He, Gentner and Happee. This is an open-access article distributed under the terms of the Creative Commons Attribution License (CC BY). The use, distribution or reproduction in other forums is permitted, provided the original author(s) and the copyright owner(s) are credited and that the original publication in this journal is cited, in accordance with accepted academic practice. No use, distribution or reproduction is permitted which does not comply with these terms.
*Correspondence: Sina Nordhoff, cy5ub3JkaG9mZkB0dWRlbGZ0Lm5s
Disclaimer: All claims expressed in this article are solely those of the authors and do not necessarily represent those of their affiliated organizations, or those of the publisher, the editors and the reviewers. Any product that may be evaluated in this article or claim that may be made by its manufacturer is not guaranteed or endorsed by the publisher.
Research integrity at Frontiers
Learn more about the work of our research integrity team to safeguard the quality of each article we publish.