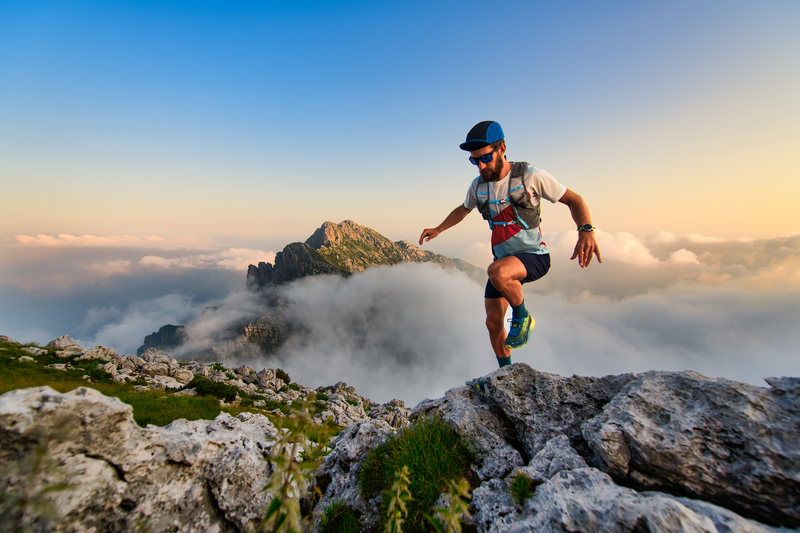
95% of researchers rate our articles as excellent or good
Learn more about the work of our research integrity team to safeguard the quality of each article we publish.
Find out more
ORIGINAL RESEARCH article
Front. Psychol. , 25 March 2024
Sec. Emotion Science
Volume 14 - 2023 | https://doi.org/10.3389/fpsyg.2023.1105875
Using messages posted on Twitter, this study develops a new approach to estimating collective emotions (CEs) within countries. It applies time series methodology to develop and demonstrate a novel application of CEs to identify emotional events that are significant at the societal level. The study analyzes over 200 million words from over 10 million Twitter messages posted in 16 countries during the first 120 days of the COVID-19 pandemic. Daily levels of collective anxiety and positive emotions were estimated using Linguistic Inquiry and Word Count's (LIWC) psychologically validated lexicon. The time series estimates of the two collective emotions were analyzed for structural breaks, which mark a significant change in a series due to an external shock. External shocks to collective emotions come from events that are of shared emotional relevance, and this study develops a new approach to identifying them. In the COVID-19 Twitter posts used in the study, analysis of structural breaks showed that in all 16 countries, a reduction in collective anxiety and an increase in positive emotions followed the WHO's declaration of COVID-19 as a global pandemic. Announcements of economic support packages and social restrictions also had similar impacts in some countries. This indicates that the reduction of uncertainty around the evolving COVID-19 situation had a positive emotional impact on people in all the countries in the study. The study contributes to the field of CEs and applied research in collective psychological phenomena.
Psychology has examined emotions primarily as an individual-level phenomenon. Even when considering group-based emotions, an individual's emotional experiences are regarded as an outcome of their psychological belonging or social membership to the given group (Mackie et al., 2000; Mackie and Smith, 2018). Before the individualization of social psychology (Farr, 1980), there had been considerable interest in collective-level psychological phenomena, including emotions, their transference, and their impact on individuals. For instance, Le Bon (1895, p. na) noted that crowds develop ideas and sentiments, and a “collective mind is formed, doubtless transitory, but presenting very clearly defined characteristics”. Similarly, Durkheim (1915) discussed emotional effervescence and collective emotional excitement in the totemic ceremonies of the Uluru and Kingilli people. More recently, there has been a renewed interest in how group memberships shape individual experiences of emotions due to social identification or bonds between members of the group (Kim, 2016). Despite their name, these emotions remain a type of individual emotion - the individual is still the unit of assessment as the appraisal of the event still remains tied to the individual (Goldenberg et al., 2020).
On the other hand, in situations such as wars or national tragedies, an examination of macrolevel affective processes beyond the smaller group-based emotions becomes necessary (Goldenberg et al., 2020). This has led to the development of the notion of collective emotions (CEs), which do not associate the experience of emotions with group memberships. Instead, they consider that people belonging to a collective may experience an emotion for a range of reasons, not limited to shared identities or bonds. CEs arise in a society in response to events such as wars, national tragedies and triumphs, or indeed other events that dilute the boundaries of identification between groups (Bar-Tal et al., 2007; Goldenberg et al., 2014). Research has shown that CEs tend to cut across identities to engulf entire collectives. For instance, Garcia and Rimé (2019) examined Twitter posts of over 60,000 users in the aftermath of the terrorist attacks in Paris in 2015 and found a long-term increase in lexical indicators for solidarity and pro-sociality. CEs emerge not only in real time but also when collectives recall a significant event. For instance, in Kim's (2016) study several years after 9/11, CEs developed amongst participants discussing the trauma of the terrorist attacks.
In other words, CEs emerge in broader group contexts and, unlike individual level emotions, reflect the emotional state of a collective responding to the same event, phenomenon, or situation. CEs capture the overall emotional states of the systems where individuals activate, amplify, or reflect each other's emotional experiences, resulting in a predominant emotional state. Let us take a hypothetical event that has the possibility of evoking emotional experience in a collective: India winning the Football World Cup. If the collective at hand is of n individuals who watched the final separately on their TVs and remained in a communicative black hole, each of them would individually appraise the event and have an emotional experience. However, in a world where people interact, the emotional experience of the event will also be driven by the experiences of others. In a cricket-mad country, Person A may not care about what the country achieves in football. Yet, by virtue of being embedded in the social world where others do, they may eventually experience joy, pride, or other similar emotions. In summary, when a collective system develops a certain emotional state, individuals activate each other, and instead of individual appraisals, the collective state becomes the relevant trigger for emotional experiences. Figure 1 demonstrates these points. What is more, the Internet and modern technology, more than ever before in human history, have enabled near-real-time conversations about events in the social world. This makes the emerging field of CEs extremely pertinent to what Sheldon Stryker referred to as sociological social psychology. This study makes two significant contributions in this field. First, it makes a contribution by outlining an approach to measuring CEs from what people post on the Internet, and developing daily estimates of CEs in large collectives. Its second and more important contribution is of developing a novel application of CEs to explore the embeddedness of individuals in the collective and the social. The research develops a new approach to identifying events in the social world that have significant impacts on the emotional states of collectives.
Three main methodological issues needed to be tackled in the project. First, an event sufficiently powerful to lead to the development of collective emotions needed to be identified. The COVID-19 pandemic adequately fits this criterion. The spread of the disease had an unprecedented impact on life globally and was marked by almost daily changes to public advisories in most countries. Lockdowns and restrictions on civil liberties affected the work and financial circumstances of people across the world (Bell and Blanchflower, 2020; Brewer and Gardiner, 2020; Nicola et al., 2020) and altered social norms and everyday lives (Alon et al., 2020; Dryhurst et al., 2020; Haleem et al., 2020; Nivette et al., 2020; Tagat and Kapoor, 2020; van der Westhuizen et al., 2020). More than any other new infectious diseases of the recent past, the rapid spread of COVID-19 was a cause of tremendous anxiety, alarm, and uncertainty (Goodwin et al., 2011; Mayor et al., 2013; Idoiaga Mondragon et al., 2017) around the world, and a number of studies have noted the pandemic to be an extremely emotional event (Andrade, 2020; Galea et al., 2020; Groarke et al., 2020). Given the high levels of public uncertainty and near daily changes in government advisories early in the pandemic, the study looked at CEs during the first 120 days1 of the COVID-19 pandemic.
Second, a source of data for estimating collective emotions in response to the said event needed to be identified as CEs develop out of interactions and communications between people dealing with a phenomenon of collective significance. Most debates, discussions, and conversations about the pandemic took place on the Internet as social restrictions and the nature of the disease prevented people meeting others in real life. One of these online public spaces was provided by Twitter, which is widely used for exchanging news, opinions, and feelings in real time. An online space such as Twitter, which people can access at all times, provides a natural means for the contagion, amplification, and reactivation processes and properties of CEs (Goldenberg et al., 2014, 2020). To illustrate, a person expresses their anxiety about the pandemic at time t. Their anxiety decays naturally, like all individual-level emotions, but on reengaging with Twitter, where the CE of anxiety remains high, they may experience a reactivation of their own anxiety. These reasons made Twitter the ideal landscape for examining the development of CEs, and not surprisingly, it has been used in other research on CEs previously (e.g., Garcia and Rimé, 2019).
Third, the project uses countries as the level of the collective at which CEs were tracked. The spread of the disease, hospitalizations, infections, and mortality rates were most commonly discussed at the level of countries. Similarly, the response to the pandemic—lockdowns, restrictions on civil liberties, support for workers and families—also took shape at the level of countries. For these reasons, this research considered countries to be the most appropriate level of the collective for studying CEs in response to the pandemic.
The following sections describe the methodological steps and analytic procedures.
A large publicly available dataset of tweets about COVID-19, maintained by Georgia State University's Panacea Lab, was used (Banda et al., 2020). In line with the privacy regulations, this dataset only provided an identification code (tweet ID) for individual messages posted on Twitter (henceforth, tweets). The dataset did not include retweets. Hydrator2 was used to process tweet IDs and extract text message content along with other associated metadata. At the time of conducting this procedure (Oct–Dec, 2020), nine percent of tweets in the dataset had either been deleted or made publicly unavailable by people who posted them. From each individual tweet ID, the associated text message, the country from which it was posted, and the date of its posting were extracted. For each date, words from individual tweets were combined to form aggregate corpora of words used on a particular date in each country—the study refers to them as metatext. To illustrate, 10,178 unique Twitter messages from the UK were extracted for 15 March 2020 with a total of 254,203 words. These 254,203 words formed the metatext for the UK for this date.
The project began with 25 countries with high numbers of Twitter users. Considering the use of natural language processing through the Linguistic Inquiry and the Word Count program to be undertaken for the next stage (described later), word-volume-based criteria were established with two rules for the inclusion of data from a country. First, in order to systematically eliminate countries with poor data quality, only countries with a minimum average of 5,000 words per day (over the 120-day period) were included. Austria, Costa Rica, Ethiopia, Switzerland, and Tanzania did not meet this criterion and were dropped from the study. Second, early in the pandemic, the number of tweets related to COVID-19 were low. This would result in some countries meeting the first criterion but having poor data at the start of the series. To maintain consistency between data from different countries, the latest start date of an uninterrupted series was set at 1 March 2020. Uninterrupted series were defined as at least seven consecutive calendar dates of 5,000 words in a country's daily tweet corpus. Brazil, Chile, and Peru were dropped because their uninterrupted time series started after 1 March 2020. The 16 countries that were finally included in the study covered five WHO-designated regions: Argentina, Canada, Colombia, Mexico, the United States of America (USA), and Venezuela from the Americas; France, Germany, Spain, and the United Kingdom (UK) from the European region; Nigeria and South Africa (SA) from the African region; India and Singapore from the East Asian region; Australia and New Zealand3 (NZ) from the Eastern Pacific region. Supplementary material 1 provides the details of the data preparation procedures and the software used. The original dataset had a systematic error on 9 February 2020, 28 February 2020, and 29 February 2020. These dates were treated as missing values and were imputed using the AMELIA package (Honaker et al., 2011) in R. Supplementary material 2 provides details of the imputation process.
The final textual dataset used for estimating CEs contained over 200 million words from more than 10 million Tweets posted from 16 countries. See Table 1 for details.
The analytic stage of the research began by developing daily estimates of collective anxiety and collective positive emotions in each country during the 120-day period. The choice of estimating anxiety was driven by the well-documented anxiety-provoking impact of the pandemic globally (Pashazadeh Kan et al., 2021). The umbrella category of positive emotions was used as a foil for comparing levels of anxiety. Daily levels of both emotions were estimated using the Linguistic Inquiry and Word Count (LIWC) application (Tausczik and Pennebaker, 2010). LIWC is the benchmark tool in text analytics with a well-established validity in measuring emotional expression, and its rating of the emotional content of the text has been demonstrated to correspond to human ratings (Alpers et al., 2005; Kahn et al., 2007; Hall et al., 2020). LIWC's psychologically validated dictionary was used to search for lexical indicators of anxiety and positive emotions (Windsor et al., 2019). Each date in each country was searched with LIWC separately and a daily time series estimate of collective anxiety and collective positive emotions was developed. Repeating this process on the text corpora from each country separately provided us with daily time series estimate of the two CEs in all sixteen countries.4 Table 1 provides country-wise details of the time series dataset of the two CEs. Each country's time series of collective anxiety and collective positive emotions became the basis of subsequent analysis.
Abrupt changes in daily values of both CEs were identified by determining structural breaks. Structural breaks refer to statistically significant, abrupt, and unexpected changes in the values of variables measured at regular time intervals. Scholarly works on structural breaks in the context of econometric data consider them as sources of errors, unless they are explained by an external shock to the series (Chow, 1960; Hansen, 2001; Bai and Perron, 2003). For the purposes of this research, however, structural breaks were valuable sources of information as they indicated the onset of exogenous shocks to collective emotions in a country (Casson and Fry, 2011; Cró and Martins, 2017; Zhang et al., 2017; Guan et al., 2018; Petruželka and Barták, 2020). In other words, structural breaks were statistically significant changes in the levels of anxiety and positive emotions and marked the impact of an event that provided the shock. This property of structural breaks was leveraged in the current project to discover key events of collective emotional significance in context of the pandemic.
The most well-known test for a structural break is the Chow test (Chow, 1960), with a null hypothesis of “no structural change”. The test splits the sample into two periods, and tests the equality of parameters estimated for both periods. However, Chow test requires a priori hypothesis of the break point and was not suitable as no hypotheses could be developed about possible significant events causing structural breaks. In an alternative approach to the problem, break dates can be treated as unknown. The method of estimating a single unknown break date (Bai, 1994) has been extended to multiple unknown breaks by Bai (1997a,b) and Bai and Perron (2003). This approach provided the most appropriate way for the identification of the break dates in the context of this study.
R package “strucchange” (Zeileis et al., 2003) was used to implement Bai and Perron's algorithm in the “breakpoints” function to obtain the “break dates” along with their 95% confidence intervals. For each time series, we tested the model
with the null hypothesis that regression coefficients remained constant over time. The alternative hypothesis was that at least one coefficient varies over time.
F statistics were computed for every variable. The supF test from the “supremum” tests of Andrews (1993) was employed, and H0 was rejected for statistically significant values. F statistics plots indicating the supF values at the 5% significance level for all series are provided in Supplementary material 3. The number of potential breakpoints was estimated using the Bayesian information criterion (BIC) according to Bai and Perron's recommendation. Supplementary material 3 also provides residual sum of squares (RSSs) and BIC plots.
It is important to note that the research was able to suppress the impact of individual-specific events (such as the death of a loved one) in the estimation of CEs. Anxiety and positive emotion levels in each country were estimated using its metatext, which aggregated words from individual messages. Therefore, all detected structural breaks necessarily reflected a statistically significant change in lexical indicators within the sample of Twitter traffic from a country and indicated events that were collectively significant to people from that country. For each structural break, instead of the specific date, the study focused on the period spanning the 95% CI of the break to accommodate the latency of public reaction to events and the spread of countries across the International Date Line. Throughout the study, references to detected structural breaks or shocks pertain to the 95% CI period and not the date itself. For each break discovered in anxiety or positive emotions, global- and country-specific timelines of pandemic-related events were examined.
The daily metatext from all the countries contained a higher proportion of positive emotions lexical indicators than anxiety. During the 120-day period, estimated anxiety was highest in the UK () and lowest in Mexico (); positive emotions were highest in South Africa () and lowest in Venezuela (). Figure 2 presents the time series progression of collective anxiety and collective positive emotions in all the countries.5 As the figure captures, consistent with the rapidly changing landscape of the pandemic, both emotions showed considerable volatility until April but became more stable subsequently.
In order to determine the predominant CE, the differences in the relative frequency of the lexical indicators were adjusted to obtain a normalized daily score for both anxiety and positive emotions (see Figure 3).6 As Figure 3 shows, all the countries had a higher expression of anxiety than positive emotions during January and February. In most countries, the pattern reversed during March and positive emotions dominated over anxiety in COVID-19 posts on Twitter.
Shocks to anxiety were detected in 15 countries and in total 21 instances of significant and unexpected changes to this CE were detected. Two shocks to anxiety were detected in Australia, Canada, India, New Zealand, South Africa, and Venezuela. France was the only country where no significant shock to anxiety was detected. Shocks to positive emotions were detected in all 16 countries. Three shocks to positive emotions were detected in the USA, two in Germany and India, and one each in the remaining 13 countries giving a total of 20 shocks across all the countries. Tables 2A, B presents the details.
Dates and CIs for all the detected shocks were examined for overlaps and consistency in the direction of change in the values of both CEs. Periods of shocks to anxiety showed a remarkable overlap—in 12 countries, anxiety significantly and abruptly reduced between 9 March and 17 March (see Table 2A). Interestingly, a similar overlap was also observed with positive emotions. In all 16 countries, positive emotions increased significantly and abruptly between 9 March and 17 March (see Table 2B). Looking at the bigger picture revealed by shocks to both collective emotions, the period between 9 and 17 March stands out across all 16 countries in the study. In other words, the data suggest that the period saw events of global significance within the context of COVID-19—events that altered the levels of collective emotions in all the countries examined in this research. The global and country-specific timelines for COVID-19 related events were examined to identify the likely sources of shocks to the two collective emotions. One global event and two thematic categories of events appear to be the appropriate explanation for an abrupt increase in collective positive emotions and decrease in collective anxiety. There are discussed next.
Between 9 and 17 March, an external shock to collective positive emotions was observed in all countries, and to anxiety in 12 countries (see Tables 2A, B). 28 out of the total 41 shocks (68%) detected in both CEs across all countries falling within this narrow period of nine days strongly points in the direction of an event of global relevance.
The directions of changes in the emotions were also consistent—anxiety declined, and positive emotions increased after the shock event. Within this period, the only event that cut across national boundaries and held relevance for people across the world was the WHO's declaration on 11 March where it formally assigned COVID-19 the label of a pandemic. Indeed, the 95% CI estimates for the shock date include the 11 March in all the countries for at least one CE, and in nine countries, for both CEs (see Tables 2A, B). Thus, the current research suggests that WHO's declaration of pandemic was an emotionally significant event globally where it led to an increase in collective positive emotions and decrease in collective anxiety.
The periods of shocks in nine countries-when anxiety abruptly declined and positive emotions grew-coincided with announcements of national health emergencies, restrictions on civil liberties, and lockdowns. Four countries made these announcements very close to the WHO's declaration of a pandemic. These included Colombia and the USA, where shocks to both emotions coincided with the declaration of emergencies on 12 and 13 March, respectively. In Germany, periods of shocks to both emotions coincided with Chancellor Angela Merkel's first press conference on the situation on 11 March, where she announced a general ban on large public events. Finally, Venezuela's stay-at-home order on 15 March overlapped with shocks detected in both emotions in the country.
In a further five countries, periods of shocks to anxiety coincided with similar measures later in March. In Mexico, it coincided with the declaration of the national health emergency on 30 March, in New Zealand, with a national lockdown on 25 March, and in Nigeria and Spain with restrictions becoming more stringent. Finally, of the two shocks to anxiety detected in South Africa, one coincided with a declaration of a “national state of disaster” on 15 March, while the second was only a day removed from the announcement of a national lockdown on 23 March.
To summarize, announcements of national restrictions and lockdowns were the common denominators of a decline in anxiety or a rise in positive emotions in nine countries. A total of 13 breaks detected in the data can be explained by these announcements acting as an exogenous shock.
Eight shocks to emotions in five countries coincided with the announcement of COVID-19-specific economic support packages in the respective countries. In each instance, they were marked by a reduction in anxiety or an increase in positive emotions. For Australia, the detected shock to anxiety and positive emotions overlapped with the governmental announcements of economic support packages on 12 March and 30 March. In Canada, along with the WHO's declaration of a pandemic, the periods of shock to positive emotions coincided with the government's economic package announcements on 11 and 13 March. Finally, in Singapore, the detected shock and reduction in anxiety coincided with the announcement of the coronavirus resilience budget on 26 March.
Seven breaks in six countries did not map out concretely with any local or global events that could have explained the shocks to the series. These include two positive emotions breaks in the USA (25 February; CI: 23–26 February and 8 April; 7–10 April) and one in India (1 March; CI: 27 February−2 March). Anxiety breaks in Canada (27 March; CI: 26–31 March), Peru (7 March; CI: period of shock: 5–9 March), and Venezuela (31 March; CI: 28 March−3 April) also did not have any clear events that coincided with the breaks. Finally, in one instance positive emotions dropped following a detected break in the time series (USA, break on 8 April; CI: 7 April−10 April). While no significant national event could be identified, it is worth noting that the break was observed a day after President Donald Trump's unsubstantiated criticism of the WHO on Twitter on 7 April.
The work presented in this study is among the first to combine big-data research, natural language processing techniques, and time series analysis to examine collective psychological phenomena. It is also among the first to examine collective emotions in the context of a global phenomenon and study them during the same time period in multiple countries. To the best of the authors' knowledge, this research is the first reported work to move away from survey methodologies to identify significant emotional events within and across countries by using naturally occurring text on Twitter. These methodological developments and the practical application of collective emotions were one of the main motivations for sharing this work with our peers.
Due to resource constraints, the current study did not examine the text of the Twitter posts beyond using LIWC to identify lexical indicators for anxiety and positive emotions. Future studies could, for example, also use topic modeling to scaffold the identification of the main topics as a way of validating the events that explain the shock. Due to the large number of data points and individual posters in the dataset, we did not filter for bots. However, the texts posted by bots and messages with false rumors and misinformation are no different in their contributions to the development of collective emotions.
Findings of this research have implications for public policy during periods of disasters and national crisis. As reported, in five countries, the announcement of economic support packages by their respective governments helped reduce anxiety and enhance positive emotions amidst the pandemic. It is likely that the announcement of economic support packages allowed people to come to terms with job losses and other financial challenges posed by the pandemic. This finding emphasizes the significance of providing economic security to the public during times of unprecedented uncertainty and disruption. A very important finding from this project is regarding the impact of the WHO's declaration of a pandemic on public emotions. The WHO was heavily criticized for declaring H1N1 swine flu as a pandemic in 2009 (Godlee, 2010; Low and McGeer, 2010), but with COVID-19, its reluctance in using the term received significant attention (Green, 2020; Vogel, 2020). In part, the reluctance reflected the conventional wisdom that terms such as “pandemic” and “epidemic” cause or exacerbate fear and panic among the public (Van Damme and Van Lerberghe, 2000; Bonneux and Van Damme, 2006; Barrett and Brown, 2008; Honigsbaum, 2009; Arafat et al., 2020; Maxmen, 2021). The WHO director Tedros Adhanom Ghebreyesus explicitly shared this concern in his press conference on 23 February 2020, where he observed that “using the word pandemic [now] does not fit the facts, but it may certainly cause fear” (WHO, 2020). Fear has an adaptive role in preventing people from harm, and researchers have previously noted that apprehensions about mass panic triggered by disasters and emergencies are exaggerated (Quarantelli, 2001; Gantt and Gantt, 2012; Van Bavel et al., 2020). In this light, the present research adds to the evidence base—instead of reacting with heightened anxiety, people in the 16 countries showed an increase in positive emotions and a decline in anxiety, and around the middle of March, anxiety ceased to be the predominant CE in all countries. This partially follows a natural decay in anxiety, as other studies have also reported (Mertens et al., 2023). However, results from time series analysis undertaken in this research provide strong evidence that the WHO's declaration of the pandemic was likely to have contributed to the decline in anxiety. It is well understood that uncertainty reduction in ambiguous situations lowers anxiety (Reuman et al., 2015; Chen et al., 2018) following evolutionary (Brosschot et al., 2016) and neurobiological (Grupe and Nitschke, 2013) pathways, and one possible explanation is that the WHO's declaration reduced uncertainty and confusion around the threat posed by the novel disease. Finally, this study also provided evidence that national lockdowns, declarations of states of emergency, and announcements of social restrictions did not result in a negative emotional reaction in any country. In none of the countries these measures coincided with an increase in anxiety or decline in positive emotions. On the contrary, Twitter posts in nine countries showed either a reduction in anxiety or an increase in positive emotions following such announcements, suggesting a largely positive reception of these measures in most countries. In this regard, the current study further emphasizes the need to interrogate assumptions around mass panic in the public sphere during periods of disaster and emergency.
Publicly available datasets were analyzed in this study. This data can be found here: https://zenodo.org/record/7213964.
AC, SS, and VB conceptualized the project and were involved in all the stages of the project including planning, data generation, cleaning, analysis, and preparation of the manuscript. AC and VB led the data analysis stage of the project. AC and SS led the writing of the manuscript. HS and JP led the data cleaning and LIWC processing of the dataset. AC led the project overall. All authors have approved the manuscript.
This project was funded by the School of Business and Law, University of Brighton.
The authors thank the School of Business and Law, University of Brighton for their funding, and Prof. Anuradha Patnaik, University of Mumbai, for their comments on the analysis and a previous draft of the paper.
JP was employed by Silence Laboratories. The company was not involved in the study design, collection, analysis, interpretation of data, the writing of this article or the decision to submit it for publication.
The remaining authors declare that the research was conducted in the absence of any commercial or financial relationships that could be construed as a potential conflict of interest.
All claims expressed in this article are solely those of the authors and do not necessarily represent those of their affiliated organizations, or those of the publisher, the editors and the reviewers. Any product that may be evaluated in this article, or claim that may be made by its manufacturer, is not guaranteed or endorsed by the publisher.
The Supplementary Material for this article can be found online at: https://www.frontiersin.org/articles/10.3389/fpsyg.2023.1105875/full#supplementary-material
1. ^As a common global event early in the pandemic, the confirmation of human-to-human transmission of the virus on 20 January 2020 was taken as day 1 of the pandemic.
2. ^Documenting the Now. (2020). Hydrator [Computer Software]. Retrieved from https://github.com/docnow/hydrator.
3. ^New Zealand, with its uninterrupted time series starting on 2nd March, was included as an exception due to its theoretical value as a country with unusually low COVID-19 cases during the period of the study.
4. ^LIWC's validated dictionaries of English, French, and Spanish were used according to the predominant language of the country. It is expected that some Twitter posts in data from all countries were made in a language other than the one identified as its main language. However, given the size of the dataset, this would have been a relatively small proportion, as indicated by high lexicon hit rates observed for all the countries ().
5. ^Smoothened for better visual presentation using LOESS (Cleveland et al., 2017). The parameter α was set to a period of 21 days closest to each data point.
6. ^This was achieved by dividing each observation in each series by the respective mean of the series Xi(t) = Xi(t)/μ(t).
Alon, T. M., Doepke, M., Olmstead-Rumsey, J., and Tertilt, M. (2020). The IMPACT of COVID-19 on Gender Equality. Cambridge: National Bureau of Economic Research.
Alpers, G. W., Winzelberg, A. J., Classen, C., Roberts, H., Dev, P., Koopman, C., et al. (2005). Evaluation of computerized text analysis in an Internet breast cancer support group. Comput. Human Behav. 21, 361–376. doi: 10.1016/j.chb.2004.02.008
Andrade, C. (2020). COVID-19: Humanitarian and health care crisis in a third world country. J. Clin. Psychiatry 81, 0. doi: 10.4088/JCP.20com13383
Andrews, D. W. K. (1993). Tests for parameter instability and structural change with unknown change point. Econometrica 821–856. doi: 10.2307/2951764
Arafat, S. M. Y., Kar, S. K., Marthoenis, M., Sharma, P., Apu, E. H., and Kabir, R. (2020). Psychological underpinning of panic buying during pandemic (COVID-19). Psychiatry Res. 289, 113061. doi: 10.1016/j.psychres.2020.113061
Bai, J. (1994). Least squares estimation of a shift in linear processes. J. Time Series Analy. 15, 453–472. doi: 10.1111/j.1467-9892.1994.tb00204.x
Bai, J. (1997a). Estimating multiple breaks one at a time. Econom. Theory 1997, 315–352. doi: 10.1017/S0266466600005831
Bai, J. (1997b). Estimation of a change point in multiple regression models. Rev. Econ. Statist. 79, 551–563. doi: 10.1162/003465397557132
Bai, J., and Perron, P. (2003). Computation and analysis of multiple structural change models. J. Appl. Economet. 18, 1–22. doi: 10.1002/jae.659
Banda, J. M., Tekumalla, R., Wang, G., Yu, J., Liu, T., Ding, Y., et al. (2020). A large-scale COVID-19 Twitter chatter dataset for open scientific research—an international collaboration. ArXiv. doi: 10.3390/epidemiologia2030024
Barrett, R., and Brown, P. J. (2008). Stigma in the time of influenza: Social and institutional responses to pandemic emergencies. J. Infect. Dis. 197, S34–S37. doi: 10.1086/524986
Bar-Tal, D., Halperin, E., and de Rivera, J. (2007). Collective emotions in conflict situations: societal implications. J. Social Issues. 63, 441–460. doi: 10.1111/j.1540-4560.2007.00518.x
Bell, D. N. F., and Blanchflower, D. G. (2020). US and UK labour markets before and during the Covid-19 crash. Natl. Inst. Econ. Rev. 252, R52–R69. doi: 10.1017/nie.2020.14
Bonneux, L., and Van Damme, W. (2006). An iatrogenic pandemic of panic. BMJ 332, 786–788. doi: 10.1136/bmj.332.7544.786
Brewer, M., and Gardiner, L. (2020). The initial impact of COVID-19 and policy responses on household incomes. Oxford Rev. Econ. Policy 36, S187–S199. doi: 10.1093/oxrep/graa024
Brosschot, J. F., Verkuil, B., and Thayer, J. F. (2016). The default response to uncertainty and the importance of perceived safety in anxiety and stress: an evolution-theoretical perspective. J. Anxiety Disord. 41, 22–34. doi: 10.1016/j.janxdis.2016.04.012
Casson, C., and Fry, J. M. (2011). Revolutionary change and structural breaks: a time series analysis of wages and commodity prices in Britain 1264–1913. Available online at: https://mpra.ub.uni-muenchen.de/27866/
Chen, S., Yao, N., and Qian, M. (2018). The influence of uncertainty and intolerance of uncertainty on anxiety. J. Behav. Ther. Exp. Psychiatry 61, 60–65. doi: 10.1016/j.jbtep.2018.06.005
Chow, G. C. (1960). Tests of equality between sets of coefficients in two linear regressions. Econometrica 28, 591–605. doi: 10.2307/1910133
Cleveland, W. S., Grosse, E., and Shyu, W. M. (2017). “Local regression models,” in Statistical Models in S. London: Routledge, 309–376.
Cró, S., and Martins, A. M. (2017). Structural breaks in international tourism demand: are they caused by crises or disasters? Tourism Manage. 63, 3–9. doi: 10.1016/j.tourman.2017.05.009
Dryhurst, S., Schneider, C. R., Kerr, J., Freeman, A. L. J., Recchia, G., Van Der Bles, A. M., et al. (2020). Risk perceptions of COVID-19 around the world. J. Risk Res. 23, 994–1006. doi: 10.1080/13669877.2020.1758193
Durkheim, E. (1915). The Elementary Forms of ReligiousLife. (George AllenandUnwin Limited). Available online at: https://www.gutenberg.org/files/41360/41360-h/41360-h.htm (accessed September 12, 2022).
Farr, R. M. (1980). “Homo socio-psychologicus,” in Models of Man, eds. A. J. Chapman and D. M. Jones. London: British Psychological Society.
Galea, S., Merchant, R. M., and Lurie, N. (2020). The mental health consequences of COVID-19 and physical distancing: the need for prevention and early intervention. JAMA Intern. Med. 180, 817–818. doi: 10.1001/jamainternmed.2020.1562
Gantt, P., and Gantt, R. (2012). Disaster psychology: dispelling the myths of panic. Prof. Saf. 57, 42–49.
Garcia, D., and Rimé, B. (2019). Collective emotions and social resilience in the digital traces after a terrorist attack. Psychol. Sci. 30, 617–628. doi: 10.1177/0956797619831964
Goldenberg, A., Garcia, D., Halperin, E., and Gross, J. J. (2020). Collective emotions. Curr. Dir. Psychol. Sci. 29, 154–160. doi: 10.1177/0963721420901574
Goldenberg, A., Saguy, T., and Halperin, E. (2014). How group-based emotions are shaped by collective emotions: Evidence for emotional transfer and emotional burden. J. Pers. Soc. Psychol. 107, 581. doi: 10.1037/a0037462
Goodwin, R., Haque, S., Hassan, S. B. S., and Dhanoa, A. (2011). Representations of swine flu: perspectives from a Malaysian pig farm. Public Understand. Sci. 20, 477–490. doi: 10.1177/0963662510392484
Green, M. S. (2020). Did the hesitancy in declaring COVID-19 a pandemic reflect a need to redefine the term? Lancet 395, 1034–1035. doi: 10.1016/S0140-6736(20)30630-9
Groarke, J. M., Berry, E., Graham-Wisener, L., McKenna-Plumley, P. E., McGlinchey, E., and Armour, C. (2020). Loneliness in the UK during the COVID-19 pandemic: Cross-sectional results from the COVID-19 psychological wellbeing study. PLoS ONE 15, e0239698. doi: 10.1371/journal.pone.0239698
Grupe, D. W., and Nitschke, J. B. (2013). Uncertainty and anticipation in anxiety: an integrated neurobiological and psychological perspective. Nat. Rev. Neurosci. 14, 488–501. doi: 10.1038/nrn3524
Guan, D., Meng, J., Reiner, D. M., Zhang, N., Shan, Y., Mi, Z., et al. (2018). Structural decline in China's CO2 emissions through transitions in industry and energy systems. Nat. Geosci. 11, 551–555. doi: 10.1038/s41561-018-0161-1
Haleem, A., Javaid, M., and Vaishya, R. (2020). Effects of COVID 19 pandemic in daily life. Curr. Med. Res. Pract. 78–79. doi: 10.1016/j.cmrp.2020.03.011
Hall, M., Logan, M., Ligon, G. S., and Derrick, D. C. (2020). Do machines replicate humans? Toward a unified understanding of radicalizing content on the open social web. Policy Int. 12, 109–138. doi: 10.1002/poi3.223
Hansen, B. E. (2001). The new econometrics of structural change: dating breaks in US labour productivity. J. Econ. Persp. 15, 117–128. doi: 10.1257/jep.15.4.117
Honaker, J., King, G., and Blackwell, M. (2011). Amelia II: a program for missing data. J. Stat. Softw. 45, 1–47. doi: 10.18637/jss.v045.i07
Idoiaga Mondragon, N., Gil de Montes, L., and Valencia, J. (2017). Understanding an Ebola outbreak: Social representations of emerging infectious diseases. J. Health Psychol. 22, 951–960. doi: 10.1177/1359105315620294
Kahn, J. H., Tobin, R. M., Massey, A. E., and Anderson, J. A. (2007). Measuring emotional expression with the Linguistic inquiry and word count. Am. J. Psychol. 263–286. doi: 10.2307/20445398
Kim, J. (2016). The effects of collective anger and fear on policy support in response to terrorist attacks. J. Soc. Psychol. 156, 455–468. doi: 10.1080/00224545.2015.1119669
Le Bon, G. (1895). The Crowd: A Study of the Popular Mind. Available online at: https://www.gutenberg.org/ebooks/445 (accessed September 12, 2022).
Low, D. E., and McGeer, A. (2010). Pandemic (H1N1) 2009: assessing the response. CMAJ 182, 1874–1878. doi: 10.1503/cmaj.100900
Mackie, D. M., Devos, T., and Smith, E. R. (2000). Intergroup emotions: explaining offensive action tendencies in an intergroup context. J. Pers. Soc. Psychol. 79, 602–616. doi: 10.1037/0022-3514.79.4.602
Mackie, D. M., and Smith, E. R. (2018). “Chapter One - Intergroup Emotions Theory: Production, Regulation, and Modification of Group-Based Emotions,” in Advances in Experimental Social Psychology, eds.J. M. Olson. Cambridge: Academic Press, 1–69.
Maxmen, A. (2021). Why did the world's pandemic warning system fail when COVID hit? Nature 589, 499–500. doi: 10.1038/d41586-021-00162-4
Mayor, E., Eicher, V., Bangerter, A., Gilles, I., Clémence, A., and Green, E. G. T. (2013). Dynamic social representations of the 2009 H1N1 pandemic: shifting patterns of sense-making and blame. Public Underst. Sci. 22, 1011–1024. doi: 10.1177/0963662512443326
Mertens, G., Lodder, P., Smeets, T., and Duijndam, S. (2023). Pandemic panic? Results of a 14-month longitudinal study on fear of COVID-19. J. Affect. Disord. 322, 1523. doi: 10.1016/j.jad.2022.11.008
Nicola, M., Alsafi, Z., Sohrabi, C., Kerwan, A., Al-Jabir, A., Iosifidis, C., et al. (2020). The socio-economic implications of the coronavirus pandemic (COVID-19): a review. Int. J. Surg. (London, England) 78, 185. doi: 10.1016/j.ijsu.2020.04.018
Nivette, A., Ribeaud, D., Murray, A., Steinhoff, A., Bechtiger, L., Hepp, U., et al. (2020). Non-compliance with COVID-19-related public health measures among young adults in Switzerland: Insights from a longitudinal cohort study. Soc. Sci. Med. doi: 10.31235/osf.io/8edbj
Pashazadeh Kan, F., Raoofi, S., Rafiei, S., Khani, S., Hosseinifard, H., Tajik, F., et al. (2021). A systematic review of the prevalence of anxiety among the general population during the COVID-19 pandemic. J. Affect. Disord. 293, 391–398. doi: 10.1016/j.jad.2021.06.073
Petruželka, B., and Barták, M. (2020). The identification of precursor regulation impact on the methamphetamine market and public health indicators in the Czech Republic: time series structural break analysis. Int. J. Environ. Res. Public Health 17, 7840. doi: 10.3390/ijerph17217840
Quarantelli, E. L. (2001). “The sociology of panic,” in International Encyclopedia of the Social and Behavioral Sciences. Oxford: Pergamon Press. doi: 10.1016/B0-08-043076-7/01867-2
Reuman, L., Jacoby, R. J., Fabricant, L. E., Herring, B., and Abramowitz, J. S. (2015). Uncertainty as an anxiety cue at high and low levels of threat. J. Behav. Ther. Exp. Psychiatry 47, 111–119. doi: 10.1016/j.jbtep.2014.12.002
Tagat, A., and Kapoor, H. (2020). Go Corona Go! Cultural beliefs and social norms in India during COVID-19. J. Behav. Econ. Policy 4, 9–15.
Tausczik, Y. R., and Pennebaker, J. W. (2010). The psychological meaning of words: LIWC and computerized text analysis methods. J. Lang. Soc. Psychol. 29, 24–54. doi: 10.1177/0261927X09351676
Van Bavel, J. J., Baicker, K., Boggio, P. S., Capraro, V., Cichocka, A., Cikara, M., et al. (2020). Using social and behavioural science to support COVID-19 pandemic response. Nat. Human Behav. 4, 460–471. doi: 10.1038/s41562-020-0884-z
Van Damme, W., and Van Lerberghe, W. (2000). Epidemics and fear. Trop. Med. Int. Health 5, 511–514. doi: 10.1046/j.1365-3156.2000.00599.x
van der Westhuizen, H.-M., Kotze, K., Tonkin-Crine, S., Gobat, N., and Greenhalgh, T. (2020). Face coverings for covid-19: From medical intervention to social practice. BMJ 370. doi: 10.22541/au.159197439.94809899
Vogel, L. (2020). What's next now that the WHO has declared a COVID-19 pandemic? Can. Med. Assoc. doi: 10.1503/cmaj.1095855
WHO (2020). “WHO Press Conference on 23-02-2020,” in WHO Director-General's Opening Remarks at the Media Briefing on COVID-19. Available online at: https://www.who.int/director-general/speeches/detail/who-director-general-s-opening-remarks-at-the-media-briefing-on-covid-19-−24-february-2020 (accessed September 12, 2022).
Windsor, L. C., Cupit, J. G., and Windsor, A. J. (2019). Automated content analysis across six languages. PLoS ONE 14, e0224425. doi: 10.1371/journal.pone.0224425
Zeileis, A., Kleiber, C., Krämer, W., and Hornik, K. (2003). Testing and dating of structural changes in practice. Computat. Statist. Data Analy. 44, 109–123. doi: 10.1016/S0167-9473(03)00030-6
Keywords: collective emotions, emotions during COVID-19, Twitter, time series, NLP, anxiety in COVID-19
Citation: Chauhan A, Belhekar V, Sehgal S, Singh H and Prakash J (2024) Tracking collective emotions in 16 countries during COVID-19: a novel methodology for identifying major emotional events using Twitter. Front. Psychol. 14:1105875. doi: 10.3389/fpsyg.2023.1105875
Received: 23 November 2022; Accepted: 28 September 2023;
Published: 25 March 2024.
Edited by:
Angel Barrasa, University of Zaragoza, SpainReviewed by:
María Celeste Dávila De León, Complutense University of Madrid, SpainCopyright © 2024 Chauhan, Belhekar, Sehgal, Singh and Prakash. This is an open-access article distributed under the terms of the Creative Commons Attribution License (CC BY). The use, distribution or reproduction in other forums is permitted, provided the original author(s) and the copyright owner(s) are credited and that the original publication in this journal is cited, in accordance with accepted academic practice. No use, distribution or reproduction is permitted which does not comply with these terms.
*Correspondence: Surbhi Sehgal, cy5zZWhnYWxAYnJpZ2h0b24uYWMudWs=
†These authors have contributed equally to this work
Disclaimer: All claims expressed in this article are solely those of the authors and do not necessarily represent those of their affiliated organizations, or those of the publisher, the editors and the reviewers. Any product that may be evaluated in this article or claim that may be made by its manufacturer is not guaranteed or endorsed by the publisher.
Research integrity at Frontiers
Learn more about the work of our research integrity team to safeguard the quality of each article we publish.