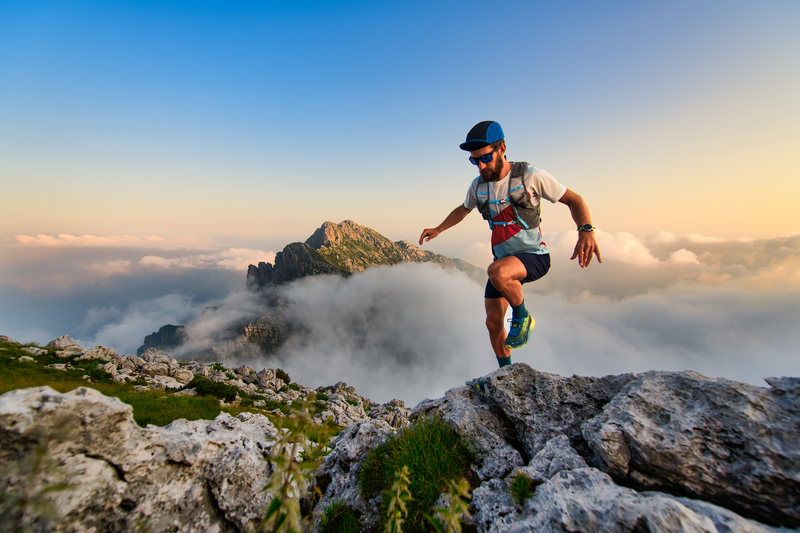
95% of researchers rate our articles as excellent or good
Learn more about the work of our research integrity team to safeguard the quality of each article we publish.
Find out more
ORIGINAL RESEARCH article
Front. Psychol. , 16 February 2023
Sec. Personality and Social Psychology
Volume 14 - 2023 | https://doi.org/10.3389/fpsyg.2023.1085587
This article is part of the Research Topic The Impacts of FinTech on Personality and Social Psychology: Multiple Interdisciplinary Perspectives View all 4 articles
Introduction: The rapid development of fintech has brought opportunities for business operations and economic development. Currently, few researches have focused on how fintech level affects word-of-mouth (WOM) from the perspective of user psychology. Therefore, studying the effect of fintech level on WOM is a worthwhile scientific question.
Methods: Based on motivation theory and reinforcement theory, this paper proposes a new psychology-based theoretical framework model to study the relationship between fintech level and WOM and constructs a structural equation model including fintech level, user experience, user trust, user stickiness and WOM through the analysis of 732 questionnaires.
Results: The results indicate that the improvement of fintech level can enhance WOM. More specifically, fintech level has a significantly positive influence on user stickiness through two mediation variables (user experience and user trust), and further, user stickiness has a significantly positive influence on WOM.
Discussion: This paper analyzes the internal mechanism of fintech level's influence on WOM from the micro psychological perspective, which enriches the psychology theoretical research. And, the conclusions provide specific suggestions for marketing and promotion of financial platforms in the future.
The Financial Stability Board (FSB) provided the definition of financial technology (fintech), which is based on big data, cloud computing, artificial intelligence, blockchain, and a series of technical innovations, and applied to wealth management, retail banking, insurance, trading, settlement, etc. (Vives, 2017). The development of fintech brings new opportunities for social and economic growth and enterprise optimization management mode (Lagna and Ravishankar, 2022; Zhao and Zhou, 2022). Previous research has focused on how the application of fintech can improve the operational efficiency and profits of enterprises (Gomber et al., 2018) but ignored the impact of fintech applications on enterprise marketing, especially on user word of mouth (WOM). In fact, if a financial platform has a high fintech level (the quality of financial products and services provided by the fintech platform to users), it will be able to simplify consumer financial transactions, improve the efficiency of businesses' massive information processing, and quickly meet consumer needs. These advantages are probably to promote the WOM communication of the platform. Once the internal influence mechanism of the fintech level on WOM is found, it will be of great value to the marketing and promotion of the financial platform. To this end, this study aims to answer the following questions: (1) Whether the improvement of the fintech level can promote the WOM communication of financial platforms? (2) What factors play a significant mediation role in the process of the fintech level influencing WOM? (3) How should financial platforms develop their fintech to obtain a good WOM?
In terms of the relationship between fintech and WOM, some work has been explored in previous research. On the one hand, existing researchers have analyzed the relationship between fintech applications and WOM. Tian et al. (2019) found that the innovation and application of fintech not only brought more users to the financial platform but also reduced the security risk of the platform and facilitated the WOM of the platform. Roy et al. (2017) also found that the platform's fintech application increased user satisfaction and WOM in the retail industry. Similarly, Liu et al. (2022) found that electronic payment services, one of the significant fintech, could increase the perceived usefulness and payment efficiency of users, thereby increasing the WOM of financial platforms. On the other hand, existing research has explored the driving influence factors in the process of fintech's influence on WOM. Albayati et al. (2020) found that user trust played a vital mediation role in this process. To be specific, if fintech could be strictly regulated, it would bring users a sense of security and further promote the WOM communication of the platform. Lim et al. (2019) emphasized that in mobile fintech services, perceived usefulness played an important mediation role in the process of fintech's influence on WOM. In addition, Roy et al. (2014) focused on the positive impact of user loyalty in the fintech field on WOM.
According to the previous research progress, we determine that there is theoretical value in studying the relationship between the fintech level and WOM. However, there are weaknesses in the current research. First, although existing research has analyzed the relationship between fintech applications and WOM, most of them focused on analyzing the internal mechanism from the perspective of management theory, ignoring the impact of user psychology. Nevertheless, user psychology plays a crucial role in the process of WOM spread and cannot be ignored. Second, although a few research have paid attention to the psychological factors of individual users, the selection of research variables is not comprehensive enough. For example, Albayati et al. (2020) focused on the influence factors of user trust but ignored the role of user experience and other factors. Similarly, Lim et al. (2019) focused on the effect of perceived usefulness on WOM communication but did not focus on the influence of user trust. In fact, ignoring any one of the key factors may lead to bias in the research results and affect the scientific nature of the conclusion. In general, the lack of a psychological theoretical perspective and systematic research are the shortcomings of previous research.
To fill the gap, this study proposes a psychology-based theoretical framework model of the impact of the fintech level on WOM and six research hypotheses (see Section 2). It attempts to analyze the internal influence mechanism of the fintech level on WOM. Second, this study constructs a structural equation model (SEM) considering multiple factors, focusing on the analysis of the possible mediation effects in the process of the fintech level influencing WOM. Specifically, a questionnaire is designed to obtain user psychological data (including user experience, user trust, and user stickiness). Then an empirical analysis is conducted based on an SEM to systematically discuss the internal rules of the influence of the fintech level on WOM.
Compared with the existing research, our main work and contributions are listed below: (1) based on psychological theories, this study proposes a new theoretical framework for the influence of the fintech level on the platform's WOM, which improves the theoretical basis of the research on the relationship between fintech and WOM and enriches the research work in the field of WOM. (2) This study systematically analyzes and summarizes important influence factors in the process of the fintech level influencing WOM and includes them as mediation variables in the model to verify the two-level mediating effect and make up for the shortcomings of existing research. (3) To the best of our knowledge, this is the first time that researchers have taken Alipay, a famous fintech platform, as the research object and constructed an SEM to analyze the influence of the platform's fintech level on WOM, which provides a reference for subsequent research on the relationship between fintech level and WOM and provide management suggestions for financial platforms.
In order to clearly express the aforementioned work and contributions, the remainder of the study is organized as follows. Section 2 introduces the basic theories and proposes six relevant hypotheses in our research. Section 3 introduces the data sources and the measurement method of constructs in the SEM. Section 4 shows the results of the model assessment and hypothesis verification. Section 5 discusses the research and provides some suggestions. Section 6 provides the summary.
Fintech could help us to develop various technologies for innovating financial products and services (Erel and Liebersohn, 2022). In terms of the fintech level, previous studies have focused on the level of fintech in various regions and interpreted the level of fintech as the fintech development status of a region (Yao et al., 2021; Wu et al., 2022). From a micro-perspective, some studies define the fintech level as the fintech development status of financial institutions such as banks (Cheng and Qu, 2020; Li et al., 2022). The fintech level proposed in our study refers to the fintech development status of a financial platform and also represents the fintech maturity of the financial platform. In fact, a user's perception of the fintech level of a financial platform mainly comes from the quality of its fintech products (Li et al., 2020; Di Maggio and Yao, 2021; Chen et al., 2022). Therefore, this study takes users' perception of fintech products (mobile payment, e-wallet, Alipay Huabei, robo-advisor, intelligent financial management, etc.) as the fintech level of the platform (Hendershott et al., 2021).
User experience is a significant concept in the psychology research field. The ISO 9241-210 standard provided a definition that user experience is people's impression of the product, system, or service that they are using or expect to use. That is, user experience is the sense of whether a financial product or service is convenient and useful (Filieri et al., 2015). Furthermore, in a study of the relationship between virtual reality and user experience, Shin (2021) defined user experience as immersion, which expresses the feelings of users in an interactive process. Similarly, user experience is the feelings and experiences obtained by users when using products or services, which includes both perceptual value and rational value (Park et al., 2018). According to previous research, we consider that user experience refers to the convenience of the Operating System (OS) and the aesthetics measure of the interface when users use a financial platform.
Many scholars have paid attention to the research on the relationship between fintech applications and user experience. For example, Lagna and Ravishankar (2022) affirmed that fintech could provide personalized services and a simple operation process to help low-income people make financial management and investment, which could improve user experience and develop inclusive finance. Nan et al. (2022) stated that face recognition payment (FRP) technology, one of the fintech, had relative advantages over traditional payment methods, which improved user experience and transaction efficiency. Similarly, Youn and Jin (2021) explained that the appearance of chatbots with artificial intelligence (AI) technology improved customer relationship management (CRM) and user experience. From the perspective of technology, Berman and Katona (2020) confirmed the adoption of AI algorithms on the financial platform could innovate specific products and services to satisfy users' preferences and improve user experience. It can be seen that previous research has demonstrated the positive impact of fintech applications on user experience from different research fields. In fact, fintech applications can be quantified in terms of the fintech level (the quality of financial products and services provided by the fintech platform to users). Therefore, we speculate that the fintech level also has a positive influence on user experience.
Hypothesis 1 (H1): The fintech level positively affects user experience.
As for the concept of user stickiness, many scholars have put forward their views from different aspects. On the one hand, Zott et al. (2000) considered that user stickiness is an important ability for enterprises to attract and retain customers. On the other hand, Lin et al. (2010) explained that stickiness is the time that customers spend on the company's social network. Furthermore, Roy et al. (2014) put forward a comprehensive viewpoint that stickiness includes two aspects, one is the visiting time of customers in the company's social network, and the other is the company's ability to retain customers. Therefore, in our study, user stickiness describes the long-term and high-frequency interactive attention of users, which reflects the loyalty of users to the platform.
Previous research has analyzed the relationship between user experience and user stickiness. Zhao and Zhou (2022) found that the user experience of the education platform had a significant and positive impact on the user's repurchase intention, and the user's repurchase intention will further affect user stickiness. In the research on willingness-to-use group purchase platforms, Wang et al. (2016) confirmed that user experience would positively affect user stickiness. In the retail business, Lian (2021) found that digitization retailers will improve user service experience and directly affect consumers' willingness to continue using. In addition, Brakus et al. (2009) revealed that consumers' evaluation of products was influenced by user experience, which would further affect consumers' brand loyalty (Japutra and Molinillo, 2019). From a theoretical point of view, Skinner proposed reinforcement theory, which is an important theory in the field of psychology. A reinforcement theory tells us that when a person takes an action, and the result is favorable to him, the action will be repeated in the future (Gordan and Krishanan, 2014). Therefore, it is reasonable to believe that when a user has a good experience on a platform, he has the motivation to continue using the platform, which increases the platform's user stickiness.
Hypothesis 2 (H2): User experience positively affects user stickiness.
The aforementioned analysis has clearly claimed the concept of fintech level and user stickiness. Although there are few researchers who pay attention to their links, previous research has also confirmed the fact that technology has a significant effect on user stickiness. Vosooghi et al. (2019) claimed that the technology of robots had a positive influence on user stickiness because it could bring them good feelings. The adoption of AI-based chatbots increased user stickiness because it can bring convenience to users in the tourism business (Pillai and Sivathanu, 2020). In terms of mobile fintech, Lim et al. (2019) found that perceived security in mobile fintech services had a significant influence on users' confirmation, which further affected continual intention to use the services. The aforementioned researches illustrate one issue that technology or fintech may have a positive effect on user stickiness. In fact, this phenomenon can be explained by motivation theory. Self-serving is the motivation for self-enhancement (Berger and Milkman, 2012), which is a significant concept in psychology. We can imagine that improving the fintech level will better assist people in making wealth management and enabling users to make self-enhancement come true. Thus, there is a reason to believe that if a platform has a high fintech level, people will be willing to use the platform more frequently to achieve self-enhancement (Pillai and Sivathanu, 2020).
Hypothesis 3 (H3): The fintech level positively affects user stickiness.
Trust can be understood as the confidence of one person in another, and it also can be defined as one's confidence in the reliability of an exchange partner (Morgan and Hunt, 1994). In our study, we focus on trust in fintech platforms (Bart et al., 2005; Zarifis and Cheng, 2022). User trust can also be interpreted as their confidence in the platform, which could bring them a sense of security and a stable income.
According to the previous research on the relationship between fintech level and user trust, Bunnell et al. (2020) proposed a recommendation system framework based on fintech. They verified the positive impact of the fintech application on improving user trust through empirical analysis. Albayati et al. (2020) affirmed that blockchain, one of the fintech, had the advantages of decentralization and asymmetric encryption, and its adoption would enhance users' sense of security and trust. Similarly, based on the constructed user trust evaluation model, Yan et al. (2020) found that with the support of big data mining, user trust can be improved. In fact, compared with manual data processing, mature fintech applications improves accuracy, security, and reliability. From a psychological point of view, establishing user trust is greatly influenced by technology security (Anderson, 2010). That is, if the platform's security can be improved, it will help to promote user trust. Therefore, we speculate that the higher the level of fintech, the more trust users will have in financial platforms.
Hypothesis 4 (H4): The fintech level positively affects user trust.
Previous research has focused on the relationship between user trust and user stickiness in many fields. User trust will effectively improve users' willingness and further improve user stickiness in the robot car rental industry (Vosooghi et al., 2019). In order to explore the influencing factors of the stickiness of member websites, El-Manstrly et al. (2020) found that user trust plays a more positive role than commitment. In addition, Lee and Hyun (2016) found that social networks and community participation had a positive impact on trust, and user trust further had a positive impact on user stickiness. In terms of the use of websites, user trust could motivate people to continue using the websites for a long time (Li et al., 2006). Also, knowledge and perceived security in mobile fintech services have a significant impact on user trust, and user trust positively affects users' intention to continue using the service (Lim et al., 2019). In fact, the theory of reasoned action (TRA) can explain this phenomenon. TRA tells us that people consider both the meaning and the consequences of an action before they do it (Kim et al., 2013). In the process, the individual's belief in the result of the behavior plays an important role, which will affect whether the individual takes the action. As far as this article is concerned, if users have trust in the financial platform, it means that users trust that financial products in the platform can bring them good profits, which may promote users to continue using the platform. Thus, we speculate that user trust has positive effects on user stickiness.
Hypothesis 5 (H5): User trust positively affects user stickiness.
The financial platform's online WOM, including consumer opinions and product reviews, is the main source of information for consumer purchasing decisions (Gu et al., 2012). WOM communication refers to informal interpersonal communication about a product, brand, or service, so it has been the main driving force for the website to acquire new members (Trusov et al., 2009; Baker et al., 2016). Therefore, users' comments and their intention to share the financial platform are uniformly summarized as the WOM of the platform in this study.
Some previous research studies use both user stickiness and WOM as the main indicators to measure user loyalty (Molinsimillo et al., 2020). However, we found that user stickiness and WOM do not belong to the same construct through the exploratory factor analysis, and user stickiness has a positive impact on WOM. In fact, user stickiness and WOM are different concepts (Roy et al., 2014). Specifically, user stickiness is an individual behavior, which mainly reflects the willingness of users to continue to use the platform (Kim et al., 2016). In comparison, owing to the good product quality of the platform, WOM emphasizes the willingness of users to promote the platform (Roy et al., 2017). Currently, a large number of studies have confirmed the positive impact of user stickiness on WOM (Roy et al., 2014; Kim et al., 2016). From the perspective of psychological theory, this phenomenon can be well explained by motivation theory. Specifically, altruistic motivation (Tellis et al., 2019) drives people to share valuable things with others. Because people share these things to show concern for others (Hennig-Thurau et al., 2004) and to try to help others (Lovett et al., 2013). In our research, if the platform's user stickiness is high, it indicates that users recognize the value of the platform, which motivates them to introduce the platform to those around them. Therefore, we propose the following final hypothesis.
Hypothesis 6 (H6): User stickiness positively affects WOM.
According to the previous analysis, this study constructs an SEM that reflects the relationship between fintech level, user experience, user trust, user stickiness, and WOM (see Figure 1) to explore how the fintech level affects WOM and provide managerial suggestions.
Alipay is a famous fintech platform, which is widely used in China to provide consumers with convenient fintech products and services. This study collects data on the fintech level, user experience, user trust, user stickiness, and WOM on Alipay through the survey. The specific workings are as follows in two stages. In the first stage, we make an online survey distributed to 107 users by the initial scales with 37 items first. Moreover, we used the exploratory factor analysis (EFA) to purify the original 37 items and the remaining 25 items in our scales, which eliminated the items with low loadings or high cross-loadings. In the second stage, we redesigned and reorganized the questionnaire in Questionnaire Star (a mainstream platform for academic surveys), distributing them through WeChat, QQ, and Alipay. Through this survey, we obtained 732 questionnaires (the total number of questionnaires is 766) after removing invalid questionnaires, with an effective recovery rate of 95.56%.
The details of the statistical results are as follows (see Table 1). First of all, 51.9% of the respondents were men, and 48.1% were women, with a gender ratio of nearly 1:1. The respondents are mainly aged between 18 and 39, accounting for 97.8%. The reason may be that people over 40 years old have little incentive to answer these questions on account of the infrequent use of fintech. Second, the education level of the respondents is mainly concentrated in the bachelor's degree (38.8%) and the master's degree or above (59.6%). Thus, it can be seen that the users of fintech are concentrated in people with high education. Finally, regarding the annual income of the respondents, the annual income of 50 thousand or less accounted for 32.2%, 50–100 thousand accounted for 21.3%, 100–200 thousand accounted for 33.3%, and 200 to 500 thousand accounted for 13.1%. The preliminary statistical analysis of the data indicates that the respondents have a balanced male-to-female ratio, high education level, stable income, etc. They could understand the meaning of the questionnaire design questions well and give accurate answers, which reflects the good representation of the sampled data in this study. A structural equation modeling methodology was used to determine how well the model fit the data and to identify support for each of the incorporated hypotheses.
The theorized model incorporates five constructs related to fintech level, user experience, users trust, user stickiness, and WOM. The items selected to measure the constructs are shown in Table 2 (for example, C1_Q1 represents the first item of the first construct. Similarly, C1_Q2 represents the second item of the first construct). In order to obtain data on respondents' degree of agreement toward each statement, we used 5-point Likert-type scales with “strongly disagree” and “strongly agree” as anchors (Wongkitrungrueng and Assarut, 2020). The following is an introduction to the specific measures.
The fintech level is assessed with the development and application ability of fintech in the financial platform (Erel and Liebersohn, 2022). Especially, it refers to the ability of technology to help users invest and manage their money. Based on the statement of fintech, artificial intelligence, and other technologies in the existing literature (Hendershott et al., 2021; Shin, 2021; Zarifis and Cheng, 2022), we consider that the advantages of fintech are the progress than the traditional manual services, which can be summarized as two aspects. It can provide users with more personalized financial products and services through big data and artificial intelligence (Lagna and Ravishankar, 2022). Otherwise, products recommended by fintech have greater advantages in terms of security and reliability (Lim et al., 2019). Regarding the fintech level, this study proposes a scale with six items to measure the fintech level, “Financial products pushed by big data and AI are timely,” “Financial products pushed by big data and AI cater to the user preference,” etc.
User experience is assessed with the users' feelings (Park et al., 2018). Based on the statement and scales of the user experience in the existing literature (Lee and Shin, 2018; Ghose et al., 2019), we consider that the core of user experience is to bring more convenient services to people and better meet the individual needs of users. The application of artificial intelligence (AI) technology optimizes the business process and reduces the time of user information review, which provides convenience for users (Youn and Jin, 2021). On the flip side, AI can understand the users' instructions and make a quick response, which meets the timely needs of users. Accordingly, we propose a scale with six items to obtain the data from respondents, “Fintech is easy to operate and use,” “AI can understand your operations and commands,” etc.
User's trust is assessed with the trust of users in the technical capabilities of the platform, which not only includes the consideration of the security of fintech but also includes the trust in the fintech's capacity to bring users more considerable benefits (Zarifis and Cheng, 2022). Based on the previous analysis and existing literature (Bart et al., 2005; Wongkitrungrueng and Assarut, 2020), the previous trust scale is adapted to fit our research scenario. Thus, we propose a scale containing four items, such as “You believe that the big data and AI have improved your return on investment (ROI),” “You believe that big data and AI have improved your investment security,” etc.
User stickiness is assessed by the user's loyalty to the financial platform (Brakus et al., 2009). That is, it is the user's willingness to continue using the platform. Previous researchers have made scales for constructs such as user intention to continue using (Wang et al., 2016), user loyalty (Lim et al., 2019), and user stickiness (Yoon et al., 2022) to explore the relationship between these constructs and the others. On this basis, we propose an evaluation scale for user stickiness to financial platforms, including three items in total, “You are willing to continue using the platform's fintech products and services,” “You are willing to buy more fintech products and services,” etc.
Word of mouth is the willingness of users to promote the financial platform. Previous research has focused on users' willingness to promote WOM on specific websites (Yu et al., 2017), mobile applications (Kim et al., 2016), and smart retail products (Roy et al., 2017) and proposed the scale of WOM measurement, respectively. With the existing results, we propose a quantitative standard containing six items, “You are willing to tell others about this fintech platforms, products and services,” “You are willing to share details about this fintech platforms, products and services,” “You are willing to recommend this fintech platforms, products and services to family members and friends,” etc.
First, the total 25 items' standardized loadings are greater than 0.5, and their related SMC is almost greater than 0.36 (see Table 2). This illustrates that our questions could represent their construct well and suggests convergent validity. Meanwhile, a confirmatory factor analysis (CFA) is also conducted to further validate the measures. After that, the results confirm all the measurement models have good fits in Table 3 (χ2/d.f. the range between 1.088 and 3.547, except for the fintech level; the goodness-of-fit index (GFI) range between 0.990 and 0.999; the adjusted goodness-of-fit index (AGFI) range between 0.948 and 0.990; normed fit index (NFI) range between 0.983 and 0.998; comparative fit index (CFI) range between 0.986 and 1.000; incremental fit index (IFI) range between 0.986 and 1.000; root-mean-square error of approximation (RMSEA) generally less than 0.08; root-mean-square residual (RMR) generally less than 0.05).
Second, the reliability of the measures is confirmed by several criteria. In our study, only one Cronbach's alpha value (user stickiness) is greater than 0.7, and all the others are greater than 0.8, which indicates that our scales are high reliabilities (see Table 2). Moreover, composite reliability (CR) ranges between 0.806 and 0.875 (see Table 4), which indicates that the items are consistent in one construct (Fornell and Larcker, 1981). In addition, the average variance extracted (AVE) for the constructs range between 0.411 and 0.612 in the measurement model (see Table 4), which also illustrates that our constructs have a good ability to explain their items.
Finally, our results also show strong evidence of validity. Convergent validity is indicated by the high loadings, composite reliability, and average variance extracted (Fornell and Larcker, 1981). Especially, there is an evaluation criterion that the model is good if composite reliability >0.7 and average variance extracted >0.36. The results of our data analysis are consistent with the aforementioned criteria (see Table 4). On the flip side, in order to illustrate the discriminant validity, we use the following criterion. The square root of AVE exceeds the other correlation coefficients in the same column or row (see Table 4). The aforementioned results show that our model has good discriminant validity. Thus, the results of the assessment described earlier (i.e., EFA and CFA) indicate the fact that the measurement model is reliable and valid. In addition, the mean value of each variable is approximately 3 (see Table 4). Meanwhile, the skewness of each variable is within ±1, and the kurtosis of each variable is within ±3, which proves that our variables obey the normal distribution (Kline, 2005).
The correlation matrix for the summary variables is presented in Table 4. The results show that the correlation coefficients are positive and significant at the 0.01 level among these constructs in the model, which is basically consistent with the results in Table 5.
In order to evaluate the results of the SEM accurately, we have done two studies. The first is to measure the reliability and validity of the SEM, and the second is to test the significance of constructs' coefficients in the SEM. In our research, Figure 2 shows the results of the structural equation modeling.
Figure 2. Structural equation model with standardized estimates (***significant at 0.001 level; **significant at 0.01 level). χ2/d.f. = 4.022, p ≤ 0.001; GFI = 0.894; CFI = 0.903; IFI = 0.904, RMR = 0.036; RMSEA = 0.064.
On the one hand, the data support the theoretical framework of the model, and the results show a good fit between the model and the observed data (χ2= 1323.30, degrees of freedom (d.f.) = 329, p ≤ 0.001, χ2/d.f. = 4.022; GFI = 0.894; CFI = 0.903; IFI = 0.904, RMR = 0.036; RMSEA = 0.064). Although a χ2/d.f. ratio of <2.0 is a common criterion to evaluate a good-fitting model, a statistically significant χ2/d.f. goodness-of-fit measure of >2.0 is not unusual with large sample sizes (Porter and Donthu, 2008). Therefore, we ought to use other factors to prove that our model is a good fit. Luckily, the value of RMSEA is lower than 0.08, and the value of RMR is lower than 0.05, which illustrates enough that our model is a good fit (Hu and Bentler, 1999).
On the other hand, the majority of hypotheses are supported, except for the relationship between the fintech level and user stickiness (see Figure 2). Specifically, the fintech level to user experience link (H1) is significantly positive, with a standardized estimate of 0.74 and a t-value of 11.83. The link from user experience to user stickiness (H2) is also significantly positive, with a standardized estimate of 0.36 and a t-value of 5.14. The fintech level to user trust link (H4) is significantly positive, with a standardized estimate of 0.62 and a t-value of 12.20. Also, user trust has a significantly positive influence on user stickiness, with a standardized estimate of 0.41 and a t-value of 6.91 (H5). Furthermore, the link from user stickiness to WOM (H6) is significantly positive, with a standardized estimate of 0.86 and a t-value of 11.62. Among the control variables, only one variable, age, plays a significant role. For these reasons, we consider that with the increase of age, people will accumulate more money and have more opportunities to make use of fintech to manage their wealth. Thus, they may easily get a deeper understanding of fintech's advantages and generate a good evaluation (WOM). Conversely, if people are younger and have fewer financial assets, they will have less opportunity and incentive to use fintech, which prevents WOM from being generated. Therefore, it is understandable that age as a control variable has a significantly positive effect on the WOM generation.
However, the lack of support for the hypothesized link between the fintech level and user stickiness (H3) is surprising and unexpected, and this result leads us to rethink the model. To further discuss the relationship between the fintech level and user stickiness, we use stepwise regression (Baron and Kenny, 1986). First, we simplify the model to just three variables (fintech level, user stickiness, and WOM) and test the relationship among them. In Figure 3A, we find that the fintech level to user stickiness link (H3) is significantly positive with a standardized estimate of 0.58 and a t-value of 10.07. Second, we add user experience back into the SEM as a mediation variable from the fintech level to user experience. Through calculation, we find that user experience plays a good mediation effect. Meanwhile, the fintech level to user stickiness link (H3) is still significant, but the standardized coefficient decreases by 0.26 from 0.58 (see Figure 3B). Third, we remove user experience and add user trust to the model as mediation, and Figure 3C shows us a similar result with the second step. The result also shows user trust is a good mediation variable. The fintech level to user stickiness link (H3) is also significant, but the standardized coefficient decreases by 0.30 from 0.58 (see Figure 3C). In the end, we add both user experience and user trust to the model as mediation variables (see Figure 3D, it is exactly the same as Figure 2. This is shown again in Figure 3 for ease of reading). At this time, the result is the same as the previous analysis of Figure 2, the fintech level to user stickiness link (H3) is not significant. This phenomenon illustrates that user experience and user trust jointly play a complete mediation effect, and when either is absent, the mediation effect turns into a partial mediation effect. In our research, there are only two traces from the fintech level to user stickiness, and it must go through two mediation variables (user experience and user trust). It shows that the fintech level cannot directly increase the willingness of users to use their platform again. Still, it can increase user stickiness by improving user experience and enhancing user trust.
Figure 3. Test of mediation effect between fintech level and user stickiness (Solid arrows mean significant, dashed arrows mean insignificant; ***significant at 0.001 level; **significant at 0.01 level). (A) No mediation effects (from fintech level to user experience). (B) User experience as the mediation. (C) User trust as the mediation. (D) Two mediation added (user experience and user trust).
In our research, we want to understand whether the fintech level of the financial platform could promote the improvement of WOM of the fintech platform. What mediation variables play a key role in this process? How should financial platforms develop their fintech to obtain good WOM? First, we find that user stickiness mediates the relationship between the fintech level and WOM, which indicates the fintech level could positively affect WOM via user stickiness. Second, we find user experience and user trust mediate the relationship between the fintech level and user stickiness, which indicates that the fintech level could affect stickiness via user experience or user trust. When there is only one mediation variable in the model (from the fintech level and user stickiness), it acts as a partial mediation (see Figures 3B, C). If we add both user experience and user trust into the model, they jointly play a complete mediation effect (see Figure 3D). This result illustrates that the fintech level could not directly affect user stickiness but could affect it via user experience and user trust. The aforementioned empirical results tell us that developing fintech is the right way for financial platforms to expand their influence. Finally, we also find that only one control variable, user age, has a significantly positive influence on WOM. It is because, with the increasing age of users, their demand for income assets and financial products is also increasing, which allows them to have more exposure and experience the advantages of fintech and generate more positive WOM.
Through the aforementioned research results, we find that if the financial platform wants to form a good WOM, it can adopt two methods: one is to improve user experience, and the other is to enhance user trust. In order to improve user experience, a financial platform could use fintech to extend the functions of online financial services, facilitate users to conduct financial business, and save more time for users. Meanwhile, they could provide users with some electronic instructions on fintech, which can help users better use fintech and have a good experience on the platform. Then, they could further develop the algorithm design so that the platform can better understand the user's command and demand and provide a better communication experience for users. In addition, they could also hire a professional to design the user page. It is because a friendly and beautiful page will brighten people's hearts and provide them good user experience.
On the flip side, to enhance user trust, a financial platform should focus on the development of fintech on improving investment returns. It is because the core of user trust in financial platforms is their trust in their profitability. Fintech can only be trusted by users if it is better able to reward them with revenue. If they want to increase user trust, they also need to further develop the algorithmic design and use fintech to improve platform security and profitability. In addition, users are also more concerned about whether big data and artificial intelligence technology will bring security threats to their private data. In other words, if a financial platform wants to improve users' trust, it should construct data security systems that could maintain and ensure the security of users' private data. For example, they can make use of data classification to manage users' data and use differential privacy technology to realize data sharing and security.
Our research fills the gap of previous research. Existing researchers have studied the relationship between fintech and the platform's WOM from the perspective of management theory (Sheng, 2021; Daud et al., 2022). This study proposes a new psychology-based theoretical framework model to study the relationship between the fintech level and WOM, which better explains how the fintech level affects WOM from a psychological perspective. In addition, although a few researchers have focused on the relationship between the fintech level and WOM, they have not considered comprehensive influencing factors (Roy et al., 2014, 2017; Kim et al., 2016). This study constructs multiple-step multiple mediator models, including three significant mediation variables (user experience, user trust, and user stickiness). We add more influencing variables than previous studies, so our research is more systematic. In addition, we confirm that the user experience and user trust jointly play a complete mediation effect. When either is absent, the mediation effect turns into a partial mediation effect (from the fintech level to user stickiness). This finding is important because it provides a good explanation of the underlying mechanism of the influence of the fintech level on WOM. This result can also provide more reliable management advice for financial platforms.
By analyzing our research's innovation and contribution, we conclude our academic implications. First, from the perspective of user psychology, this study proposes a new theoretical framework for the influence of the fintech level on the platform's WOM, which improves the theoretical basis of the research on the relationship between fintech and WOM and enriches the research work in the field of WOM. Second, based on the previous research and theoretical analysis, this study puts forward the measurement items of fintech level, user experience, user trust, user stickiness, and WOM, which provide a reference for the subsequent marketing promotion research. Third, from the perspective of micro-psychology, this study proposes a number of influence paths from the fintech level to WOM and verifies the positive effects of user experience, user trust, and user stickiness on WOM. This study better explains how the improvement of the fintech level promotes the financial platform's WOM via several significant constructs, which make an in-depth analysis and explanation of the underlying theoretical mechanism.
In terms of practical implications, this study systematically analyzes and summarizes important influence factors in the process of the fintech level influencing WOM and includes them as mediation variables in the model to verify the two-level mediating effect and make up for the shortcomings of existing research. This study has practical significance for brand marketing of financial enterprises. For instance, we have confirmed that the fintech level can have a positive impact on user engagement by influencing user experience and user trust. In real scenarios, financial enterprises can focus on the two key issues of improving user experience and ensuring user privacy security in the process of fintech research and development. In addition, this study is the first time that researchers have taken Alipay, a famous fintech platform, as the research object and constructed an SEM to analyze the influence of the platform's fintech level on WOM, which proposes a scientific paradigm for the study of WOM in other financial enterprises and provides a reference for subsequent research on the relationship between the fintech level and WOM.
Our research links the fintech level, user experience, user trust, user stickiness, and WOM together, studies the interaction mechanism among them, and proposes management suggestions based on academic theories. It is worth noting that our study focuses on how fintech brings convenience to the lives of individual users and promotes WOM communication. In fact, financial platform applications will also serve small- and medium-sized enterprises, so the influences on small- and medium-sized enterprises remain to be further discussed. This is also the issue that we will continue to explore in depth in the next step. This research direction will also help to enrich the theories of interdisciplinary subjects and better reveal the role of fintech development on human society.
Based on motivation theory and reinforcement theory, this study constructs an SEM (including fintech level, user experience, user trust, user stickiness, and WOM) and analyzes 732 questionnaires. After that, this study finds that the fintech level has a significantly positive influence on WOM via user stickiness. Furthermore, we find that user experience and user trust are two important mediation variables, and they jointly play a complete mediation effect. When either is absent, the mediation effect turns into a partial mediation effect (from the fintech level to user stickiness). This illustrates that there are only two traces from the fintech level to user stickiness, and it must go through two mediation variables (user experience and user trust). The conclusion of this study explores the influence path between the fintech level and WOM, which could help us understand the internal mechanism of WOM formation and remind us that WOM on financial platforms can be promoted by improving the fintech level. The research results of this study enriched the application scenarios of psychology and management and also provided a practical basis for the marketing and promotion of financial platforms and enterprises. In addition, from the perspective of psychology, it also provided research ideas for the relationship research between fintech development and social psychology.
The original contributions presented in the study are included in the article/supplementary material, further inquiries can be directed to the corresponding author.
YoL and XM contributed to the conceptualization, research design, data collection, analysis work, and writing the article. YuL contributed to the communication, review, editing, and supervision. RL and HL contributed to the literature collection, analysis, inspection, and correction work. All authors contributed to the article and approved the submitted version.
This study was supported by the Philosophy and Social Sciences Research Planned Project of Heilongjiang Province, China [Grant No. 21XWB124] and the Fundamental Research for the Center Universities [Grant No. FRFCU5710000220].
We greatly thank you for the helpful discussions with Dr. Chao Liu, Dr. Lijuan Xia, and Dr. Baoqiang Zhan.
The authors declare that the research was conducted in the absence of any commercial or financial relationships that could be construed as a potential conflict of interest.
All claims expressed in this article are solely those of the authors and do not necessarily represent those of their affiliated organizations, or those of the publisher, the editors and the reviewers. Any product that may be evaluated in this article, or claim that may be made by its manufacturer, is not guaranteed or endorsed by the publisher.
Albayati, H., Kim, S. K., and Rho, J. J. (2020). Accepting financial transactions using blockchain technology and cryptocurrency: A customer perspective approach. Technol. Soc. 62, 101320. doi: 10.1016/j.techsoc.2020.101320
Anderson, M. R. (2010). Community psychology, political efficacy, and trust. Polit. Psychol. 31, 59–84. doi: 10.1111/j.1467-9221.2009.00734.x
Baker, A. M., Donthu, N., and Kumar, V. (2016). Investigating how word-of-mouth conversations about brands influence purchase and retransmission intentions. J. Mark. Res. 53, 225–239. doi: 10.1509/jmr.14.0099
Baron, R. M., and Kenny, D. A. (1986). The moderator–mediator variable distinction in social psychological research: Conceptual, strategic, and statistical considerations. J. Pers. Soc. Psychol. 51, 1173–1182. doi: 10.1037/0022-3514.51.6.1173
Bart, Y., Shankar, V., Sultan, F., and Urban, G. L. (2005). Are the drivers and role of online trust the same for all web sites and consumers? A large-scale exploratory empirical study. J. Mark. 69, 133–152. doi: 10.1509/jmkg.2005.69.4.133
Berger, J., and Milkman, K. L. (2012). What makes online content viral? J. Mark. Res. 49, 192–205. doi: 10.1509/jmr.10.0353
Berman, R., and Katona, Z. (2020). Curation algorithms and filter bubbles in social networks. Mark. Sci. 39, 296–316. doi: 10.1287/mksc.2019.1208
Brakus, J. J., Schmitt, B. H., and Zarantonello, L. (2009). Brand experience: what is it? How is it measured? Does it affect loyalty? J. Mark. 73, 52–68. doi: 10.1509/jmkg.73.3.052
Bunnell, L., Osei-Bryson, K. M., and Yoon, V. Y. (2020). FinPathlight: framework for an multiagent recommender system designed to increase consumer financial capability. Decis. Support Syst. 134, 113306. doi: 10.1016/j.dss.2020.113306
Chen, T., Huang, Y., Lin, C., and Sheng, Z. (2022). Finance and firm volatility: Evidence from small business lending in China. Manage. Sci. 68, 2226–2249. doi: 10.1287/mnsc.2020.3942
Cheng, M., and Qu, Y. (2020). Does bank FinTech reduce credit risk? Evidence from China. Pac.-Basin Financ. J. 63, 101398. doi: 10.1016/j.pacfin.2020.101398
Daragmeh, A., Lentner, C., and Sági, J. (2021). FinTech payments in the era of COVID-19: Factors influencing behavioral intentions of “Generation X” in Hungary to use mobile payment. J. Behav. Exp. Financ. 32, 100574. doi: 10.1016/j.jbef.2021.100574
Daud, S. N. M., Khalid, A., and Azman-Saini, W. N. W. (2022). FinTech and financial stability: Threat or opportunity? Financ. Res. Lett. 47, 102667. doi: 10.1016/j.frl.2021.102667
Di Maggio, M., and Yao, V. (2021). Fintech borrowers: lax screening or cream-skimming? Rev. Financ. Stud. 34, 4565–4618. doi: 10.1093/rfs/hhaa142
El-Manstrly, D., Ali, F., and Steedman, C. (2020). Virtual travel community members' stickiness behaviour: How and when it develops. Int. J. Hosp. Manag. 88, 102535. doi: 10.1016/j.ijhm.2020.102535
Erel, I., and Liebersohn, J. (2022). Can FinTech reduce disparities in access to finance? Evidence from the Paycheck Protection Program. J. Financ. Econ. 146, 90–118. doi: 10.1016/j.jfineco.2022.05.004
Fang, Y., Qureshi, I., Sun, H., McCole, P., Ramsey, E., and Lim, K. H. (2014). Trust, satisfaction, and online repurchase intention. MIS Q. 38, 407–A9. doi: 10.25300/MISQ/2014/38.2.04
Filieri, R., Alguezaui, S., and McLeay, F. (2015). Why do travelers trust TripAdvisor? Antecedents of trust towards consumer-generated media and its influence on recommendation adoption and word of mouth. Tourism Manage. 51, 174–185. doi: 10.1016/j.tourman.2015.05.007
Fornell, C., and Larcker, D. F. (1981). Evaluating structural equation models with unobservable variables and measurement error. J. Mark. Res. 18, 39–50. doi: 10.1177/002224378101800104
Ghali-Zinoubi, Z., and Toukabri, M. (2019). The antecedents of the consumer purchase intention: Sensitivity to price and involvement in organic product: Moderating role of product regional identity. Trends Food Sci. Technol. 90, 175–179. doi: 10.1016/j.tifs.2019.02.028
Ghose, A., Ipeirotis, P. G., and Li, B. (2019). Modeling consumer footprints on search engines: An interplay with social media. Manage. Sci. 65, 1363–1385. doi: 10.1287/mnsc.2017.2991
Gomber, P., Kauffman, R. J., Parker, C., and Weber, B. W. (2018). On the fintech revolution: Interpreting the forces of innovation, disruption, and transformation in financial services. J. Manage. Inform. Syst. 35, 220–265. doi: 10.1080/07421222.2018.1440766
Gordan, M., and Krishanan, I. A. (2014). A review of BF Skinner's ‘Reinforcement theory of motivation. Int. J. Res. Educ. Methodol. 5, 680–688. doi: 10.24297/ijrem.v5i3.3892
Gu, B., Park, J., and Konana, P. (2012). The impact of external word-of-mouth sources on retailer sales of high-involvement products. Inf. Syst. Res. 23, 182–196. doi: 10.1287/isre.1100.0343
Hendershott, T., Zhang, X., Zhao, J. L., and Zheng, Z. (2021). FinTech as a game changer: Overview of research frontiers. Inf. Syst. Res. 32, 1–17. doi: 10.1287/isre.2021.0997
Hennig-Thurau, T., Gwinner, K. P., Walsh, G., and Gremler, D. D. (2004). Electronic word-of-mouth via consumer-opinion platforms: what motivates consumers to articulate themselves on the internet? J. Interact. Mark. 18, 38–52. doi: 10.1002/dir.10073
Hsu, C. L., and Lin, J. C. C. (2016). Effect of perceived value and social influences on mobile app stickiness and in-app purchase intention. Technol. Forecast. Soc. Chang. 108, 42–53. doi: 10.1016/j.techfore.2016.04.012
Hu, L. T., and Bentler, P. M. (1999). Cutoff criteria for fit indexes in covariance structure analysis: Conventional criteria versus new alternatives. Struct. Equ. Modeling. 6, 1–55. doi: 10.1080/10705519909540118
Japutra, A., and Molinillo, S. (2019). Responsible and active brand personality: On the relationships with brand experience and key relationship constructs. J. Bus. Res. 99, 464–471. doi: 10.1016/j.jbusres.2017.08.027
Kim, S., Baek, T. H., Kim, Y. K., and Yoo, K. (2016). Factors affecting stickiness and word of mouth in mobile applications. J. Res. Interact. Mark. 10, 177–192. doi: 10.1108/JRIM-06-2015-0046
Kim, S., Jeong, S. H., and Hwang, Y. (2013). Predictors of pro-environmental behaviors of American and Korean students: The application of the theory of reasoned action and protection motivation theory. Sci. Commun. 35, 168–188. doi: 10.1177/1075547012441692
Kline, R. B. (2005). Principles and Practice of Structural Equation Modeling (2nd ed.). New York: Guilford publications.
Lagna, A., and Ravishankar, M. N. (2022). Making the world a better place with fintech research. Inf. Syst. J. 32, 61–102. doi: 10.1111/isj.12333
Lee, I., and Shin, Y. J. (2018). Fintech: Ecosystem, business models, investment decisions, and challenges. Bus. Horiz. 61, 35–46. doi: 10.1016/j.bushor.2017.09.003
Lee, K. H., and Hyun, S. S. (2016). A model of value-creating practices, trusting beliefs, and online tourist community behaviors: Risk aversion as a moderating variable. Int. J. Contemp. Hosp. Manag. 28, 1864–1894. doi: 10.1108/IJCHM-01-2015-0002
Li, C., He, S., Tian, Y., Sun, S., and Ning, L. (2022). Does the bank's FinTech innovation reduce its risk-taking? Evidence from China's banking industry. J. Innov. Knowl. 7, 100219. doi: 10.1016/j.jik.2022.100219
Li, D., Browne, G. J., and Wetherbe, J. C. (2006). Why do internet users stick with a specific web site? A relationship perspective. Int. J. Electron. Commer. 10, 105–141. doi: 10.2753/JEC1086-4415100404
Li, Z., Wang, R., Xu, Y., and Gao, Y. (2020). Financial technology efficiency and credit constraints facing the industrial sector: evidence from China. IEEE Access. 8, 57335–57347. doi: 10.1109/ACCESS.2020.2981685
Lian, J. W. (2021). Determinants and consequences of service experience toward small retailer platform business model: Stimulus–organism–response perspective. J. Retail. Consum. Serv. 62, 102631. doi: 10.1016/j.jretconser.2021.102631
Lien, C. H., Cao, Y., and Zhou, X. (2017). Service quality, satisfaction, stickiness, and usage intentions: An exploratory evaluation in the context of WeChat services. Comput. Hum. Behav. 68, 403–410. doi: 10.1016/j.chb.2016.11.061
Lim, S. H., Kim, D. J., Hur, Y., and Park, K. (2019). An empirical study of the impacts of perceived security and knowledge on continuous intention to use mobile fintech payment services. Int. J. Hum. Comput. Interact. 35, 886–898. doi: 10.1080/10447318.2018.1507132
Lin, L., Hu, P. J. H., Sheng, O. R. L., and Lee, J. (2010). Is stickiness profitable for electronic retailers? Commun. ACM. 53, 132–136. doi: 10.1145/1666420.1666454
Liu, T. L., Lin, T. T., and Hsu, S. Y. (2022). Continuance Usage Intention toward E-Payment during the COVID-19 pandemic from the financial sustainable development perspective using perceived usefulness and electronic word of mouth as mediators. Sustainability. 14, 7775. doi: 10.3390/su14137775
Lovett, M. J., Peres, R., and Shachar, R. (2013). On brands and word of mouth. J. Mark. Res. 50, 427–444. doi: 10.1509/jmr.11.0458
Molinillo, S., Anaya-Sánchez, R., and Liébana-Cabanillas, F. (2020). Analyzing the effect of social support and community factors on customer engagement and its impact on loyalty behaviors toward social commerce websites. Comput. Hum. Behav. 108, 105980. doi: 10.1016/j.chb.2019.04.004
Morgan, R. M., and Hunt, S. D. (1994). The commitment-trust theory of relationship marketing. J. Mark. 58, 20–38. doi: 10.1177/002224299405800302
Nan, D., Kim, Y., Huang, J., Jung, H. S., and Kim, J. H. (2022). Factors affecting intention of consumers in using face recognition payment in offline markets: an acceptance model for future payment service. Front. Psychol. 13, 830152. doi: 10.3389/fpsyg.2022.830152
Park, E., Rishika, R., Janakiraman, R., Houston, M. B., and Yoo, B. (2018). Social dollars in online communities: The effect of product, user, and network characteristics. J. Mark. 82, 93–114. doi: 10.1509/jm.16.0271
Pillai, R., and Sivathanu, B. (2020). Adoption of AI-based chatbots for hospitality and tourism. Int. J. Contemp. Hosp. Manag. 32, 3199–3226. doi: 10.1108/IJCHM-04-2020-0259
Porter, C. E., and Donthu, N. (2008). Cultivating trust and harvesting value in virtual communities. Manage. Sci. 54, 113–128. doi: 10.1287/mnsc.1070.0765
Roy, S. K., Balaji, M. S., Sadeque, S., Nguyen, B., and Melewar, T. C. (2017). Constituents and consequences of smart customer experience in retailing. Technol. Forecast. Soc. Chang. 124, 257–270. doi: 10.1016/j.techfore.2016.09.022
Roy, S. K., Lassar, W. M., and Butaney, G. T. (2014). The mediating impact of stickiness and loyalty on word-of-mouth promotion of retail websites: A consumer perspective. Eur. J. Market. 48, 1828–1849. doi: 10.1108/EJM-04-2013-0193
Setia, P., Setia, P., Venkatesh, V., and Joglekar, S. (2013). Leveraging digital technologies: How information quality leads to localized capabilities and customer service performance. MIS Q. 37, 565–590. doi: 10.25300/MISQ/2013/37.2.11
Sheng, T. (2021). The effect of fintech on banks' credit provision to SMEs: Evidence from China. Financ. Res. Lett. 39, 101558. doi: 10.1016/j.frl.2020.101558
Shin, D. (2021). The effects of explainability and causability on perception, trust, and acceptance: Implications for explainable AI. Int. J. Hum.-Comput. Stud. 146, 102551. doi: 10.1016/j.ijhcs.2020.102551
Tellis, G. J., MacInnis, D. J., Tirunillai, S., and Zhang, Y. (2019). What drives virality (sharing) of online digital content? The critical role of information, emotion, and brand prominence. J. Mark. 83, 1–20. doi: 10.1177/0022242919841034
Tian, M. W., Wang, L., Yan, S. R., Tian, X. X., Liu, Z. Q., and Rodrigues, J. J. P. (2019). Research on financial technology innovation and application based on 5G network. IEEE Access. 7, 138614–138623. doi: 10.1109/ACCESS.2019.2936860
Trusov, M., Bucklin, R. E., and Pauwels, K. (2009). Effects of word-of-mouth versus traditional marketing: findings from an internet social networking site. J. Mark. 73, 90–102. doi: 10.1509/jmkg.73.5.90
Vosooghi, R., Kamel, J., Puchinger, J., Leblond, V., and Jankovic, M. (2019). Robo-Taxi service fleet sizing: assessing the impact of user trust and willingness-to-use. Transportation. 46, 1997–2015. doi: 10.1007/s11116-019-10013-x
Wang, W. T., Wang, Y. S., and Liu, E. R. (2016). The stickiness intention of group-buying websites: The integration of the commitment–trust theory and e-commerce success model. Inf. Manage. 53, 625–642. doi: 10.1016/j.im.2016.01.006
Wongkitrungrueng, A., and Assarut, N. (2020). The role of live streaming in building consumer trust and engagement with social commerce sellers. J. Bus. Res. 117, 543–556. doi: 10.1016/j.jbusres.2018.08.032
Wu, Y. H., Bai, L., and Chen, X. (2022). How does the development of fintech affect financial efficiency? Evidence from China. Entrepr. Reg. Develop. 21, 278. doi: 10.1080/1331677X.2022.2106278
Yan, Y., Huang, C., Wang, Q., and Hu, B. (2020). Data mining of customer choice behavior in internet of things within relationship network. Int. J. Inf. Manage. 50, 566–574. doi: 10.1016/j.ijinfomgt.2018.11.013
Yang, H. L., and Lin, C. L. (2014). Why do people stick to Facebook web site? A value theory-based view. Inf. Technol. People. 27, 21–37. doi: 10.1108/ITP-11-2012-0130
Yao, Y., Hu, D., Yang, C., and Tan, Y. (2021). The impact and mechanism of fintech on green total factor productivity. Green Financ. 3, 198–221. doi: 10.3934/GF.2021011
Yoon, J. Y., Lee, C., Choi, J., Chang, S. R., and Kim, J. (2022). The effect of social media apps on shopping apps. J. Bus. Res. 148, 23–32. doi: 10.1016/j.jbusres.2022.04.021
Youn, S., and Jin, S. V. (2021). “In AI we trust?” The effects of parasocial interaction and technopian versus luddite ideological views on chatbot-based customer relationship management in the emerging “feeling economy”. Comput. Hum. Behav. 119, 106721. doi: 10.1016/j.chb.2021.106721
Yu, X., Roy, S. K., Quazi, A., Nguyen, B., and Han, Y. (2017). Internet entrepreneurship and “the sharing of information” in an Internet-of-Things context: The role of interactivity, stickiness, e-satisfaction and word-of-mouth in online SMEs' websites. Internet Res. 27, 74–96. doi: 10.1108/IntR-02-2015-0060
Zarifis, A., and Cheng, X. (2022). A model of trust in Fintech and trust in Insurtech: How Artificial Intelligence and the context influence it. J. Behav. Exp. Financ. 36, 100739. doi: 10.1016/j.jbef.2022.100739
Zhang, M., Guo, L., Hu, M., and Liu, W. (2017). Influence of customer engagement with company social networks on stickiness: Mediating effect of customer value creation. Int. J. Inf. Manage. 37, 229–240. doi: 10.1016/j.ijinfomgt.2016.04.010
Zhao, H., and Zhou, Y. (2022). Understanding the Mechanism of User Experience Role in Educational Livestreaming Platform. Front. Psychol. 13, 907929. doi: 10.3389/fpsyg.2022.907929
Keywords: fintech level, word-of-mouth, user experience, user trust, user stickiness, financial platform, structural equation model
Citation: Li Y, Ma X, Li Y, Li R and Liu H (2023) How does platform's fintech level affect its word of mouth from the perspective of user psychology? Front. Psychol. 14:1085587. doi: 10.3389/fpsyg.2023.1085587
Received: 31 October 2022; Accepted: 26 January 2023;
Published: 16 February 2023.
Edited by:
Tian Xie, Wuhan University, ChinaReviewed by:
Young-Chan Lee, Dongguk University Gyeongju, Republic of KoreaCopyright © 2023 Li, Ma, Li, Li and Liu. This is an open-access article distributed under the terms of the Creative Commons Attribution License (CC BY). The use, distribution or reproduction in other forums is permitted, provided the original author(s) and the copyright owner(s) are credited and that the original publication in this journal is cited, in accordance with accepted academic practice. No use, distribution or reproduction is permitted which does not comply with these terms.
*Correspondence: Yujia Li, d2Vpc2wyMUBtYWlscy5qbHUuZWR1LmNu
Disclaimer: All claims expressed in this article are solely those of the authors and do not necessarily represent those of their affiliated organizations, or those of the publisher, the editors and the reviewers. Any product that may be evaluated in this article or claim that may be made by its manufacturer is not guaranteed or endorsed by the publisher.
Research integrity at Frontiers
Learn more about the work of our research integrity team to safeguard the quality of each article we publish.