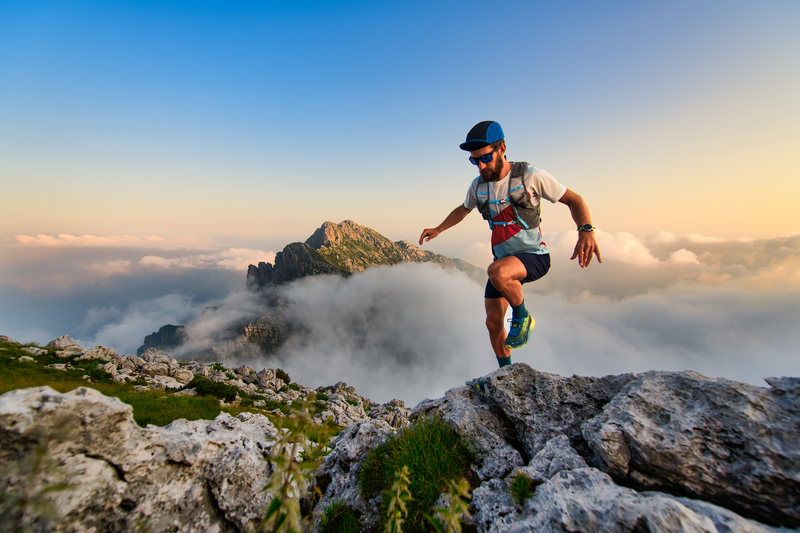
94% of researchers rate our articles as excellent or good
Learn more about the work of our research integrity team to safeguard the quality of each article we publish.
Find out more
CONCEPTUAL ANALYSIS article
Front. Psychol. , 24 January 2023
Sec. Organizational Psychology
Volume 14 - 2023 | https://doi.org/10.3389/fpsyg.2023.1009459
Unlike much research on work engagement, there is not much literature focused on managers that discuss their job demands and resources related to digital challenges in today’s organizations. Grounded in the JD-R model and considering the current digital world context, we build four research propositions and offer a work engagement framework that considers the boundary conditions of digital managerial tasks. Our conceptual framework relates the new job demands and resources to digital managerial tasks: digital adoption tasks and digital business model tasks. This conceptual article has theoretical and practical implications for organizational psychology, organizational behavior, and strategic management scholars and practitioners interested in studying managers’ work engagement and digital managerial tasks.
Technological innovations are rapidly revolutionizing the way in which managers perceive the market and its human-machine interactions (Hsieh and Vergne, 2022). Digitalization refers to digital technologies to change a business model and provide managers with new value-producing resources (Annarelli et al., 2021). Digital businesses based on platforms, big data (BD), machine learning, robots, artificial intelligence (AI), metaverse, and algorithms are reshaping the concept of managerial work because they are altering the practices and processes of human decision-making (Sidner et al., 2005; Brougham and Haar, 2018; Sohn and Kwon, 2020). Digital technologies can take over repetitive, complicated, or heavy tasks, which leaves managers to focus more on cognitively or mentally demanding decisions (Demerouti, 2022). For instance, BD can support managers with business intelligence tools. In contrast, AI can help managers to classify information more efficiently than a team of analysts by using predictive analytics tools. The emergence of these technologies has expanded the need for research on organizational behavior and strategic management that clarifies the latest new job demands and resources that will empower managers to deal with work engagement and task performance in digital managerial tasks.
To date, there has been little agreement on the new job demands and resources related to the digital managerial tasks (Juyumaya and Torres, 2020). The job demands-resources (JD-R) model explains how resources are functional in achieving individuals’ work goals, reducing job demands and the associated physiological and psychological costs, and stimulating organizational growth, learning, and development (Bakker and Demerouti, 2017; Reina-Tamayo et al., 2017). However, the rapid technological changes in digital managerial tasks are having a serious effect on the managers’ work engagement, specifically on their vigor, dedication, and absorption (Bakker et al., 2008; Schaufeli and Bakker, 2010; Demerouti, 2022).
New job demands change managers’ mindsets about the responsibility and vigilance of these new demands. Demerouti (2022) suggests that new digital managerial tasks require individuals who pay sustained attention and react on a timely basis to a visual stimulus. Managers, who use decision-support systems, such as digital dashboards or automatically balanced scorecards, have several indicators that predict outputs automatically and over time. Yet, managers can perceive the new information displayed by the same dashboard differently (Torres et al., 2017), triggering some managers to react positively with vigor to the variation of such visual stimuli. On the other hand, others feel that the new information is overwhelming, generating a state of health impairment, such as burnout.
Furthermore, new jobs and routines demand managers who have acquired new knowledge and continuously learn (Meijerink et al., 2021). However, these changes do not occur automatically; managers face more significant pressure due to adopting new technology, increased workload, and fatigue (Andrade-Valbuena and Torres, 2018). In addition, managers need to develop new personal resources to balance new job demands with the job resources available to perform digital managerial tasks.
Yet the past work from organizational scholars focuses almost exclusively on the positive and negative consequences of the job resources and demands on employees’ states of mind in the workplace, with little consideration of the specific digital managerial tasks’ characteristics or the mechanisms that moderate these effects. What is missing is a coherent theoretical model of how and why new job demands and resources affect digital managerial tasks. To address this issue, we develop a theoretical framework using the JD-R model to explain how managers perceive digital managerial tasks’ job demands and resources (see Figure 1). We argue that the characteristics of digital business models have specific features that affect the manager’s work engagement. This conceptual paper attempts to answer the following questions: What new job demands and resources affect managers’ work engagement in digital managerial tasks? And what are the consequences for future research?
Figure 1. A managers’ work engagement framework for understanding digital managerial tasks. Adapted from Bakker and Demerouti (2017).
Knowing new job demands and resources can help managers, scholars, and policymakers create new positive strategies. In this article, we propose four research propositions. We focus on managers’ work engagement rather than employees to highlight that managers face several workplace changes that can boost or decrease task performance in digital managerial tasks. First, we offer that managers’ work engagement positively relates to task performance in digital adoption and digital business model tasks (Proposition 1). Then, we identify two new job demands (Proposition 2), two new job resources (Proposition 3), and two new personal resources (Proposition 4), which are associated with the manager’s digital managerial tasks. These three research propositions lay the foundations for further empirical explorations.
This paper will benefit practicing managers and academic scholars in research and development (R&D), human resources, innovation management, and, more broadly, organizational behavior and strategic management researchers. We first briefly describe the JD-R model and its extensions to explain digital managerial tasks. We then present several novel research propositions as to how combinations of different job demands and resources influence different aspects of digital task performance through their effects on managers’ engagement. Finally, we describe the implications of our theoretical model for both theory and practice and discuss streams for future studies.
Traditionally, the JD-R model has been used to predict some positive organizational effects of traditional jobs on employees, such as motivation, organizational commitment, work engagement, and task performance (Schaufeli and Salanova, 2007; Christian et al., 2011; Juyumaya, 2022), juxtaposed to adverse organizational outcomes, like stress, exhaustion, burnout, sickness, absenteeism (Schaufeli et al., 2008; Crawford et al., 2010; Breevaart et al., 2015). JD-R model analyzes job characteristics into two categories: job demands and job resources. Job demands refer to the physical, psychological, social, or organizational aspects that require physical and psychological effort and are related to physiological and psychological costs, such as work pressure and emotionally demanding interactions with board members, strategic partners, and clients (El-Kot and Burke, 2010). On the other hand, job resources refer to the physical, psychological, social, or organizational aspects of the job that are (a) functional in achieving work goals; (b) reducing job demands and the associated physiological and psychological costs; or (c) stimulating personal growth, learning, and development (Bakker et al., 2011).
Figure 1 outlines the four propositions within the work engagement framework for digital managerial tasks. It is based on the JD-R model (Bakker and Demerouti, 2017). This framework explores a number of four research propositions about the role of digital managerial tasks within the work engagement framework. It suggests how new job demands and resources could be further explored concerning digital managerial tasks. The framework’s left side depicts new job demands (sustained attention and timely reactions and maintenance and deployment), new job resources (big data and artificial intelligence, and automation), and new personal resources (technological reflectiveness and individual ambidexterity). The right half of the framework illustrates the results of managers’ work engagement. It concerns the impact of job demands and resources on task performance (digital adoption tasks and digital business model tasks), following an individual level of analysis.
Creating and adopting new digital business models is a permanent task for managers that involves new job demands and new resources in digital managerial tasks. For example, these digital managerial tasks allow managers to understand how digital users and customers adapt their products and services. We discuss two digital managerial tasks embedded in the work engagement framework for digital managerial tasks: (1) digital adoption and (2) digital business models.
Digital adoption occurs when a manager acquires managing technology and successfully carries out digital business objectives. Managers that manage digital adoption can (a) understand the potential of digital resources, (b) accept and utilize such resources, and (c) deploy technology to optimize processes and grow innovation. The increased standardization of tasks replaces routine cognitive skills, and automation causes a decline in the active use of skills and an increase in monitoring tasks (Parker and Grote, 2022). Managers need to collect data from different sources to enhance their task performance. However, most captured data are of value only when combined with other data in a specific context. Information silos vary widely across disciplines. The greatest challenge in many cases is to dismantle information silos and incorporate these islands of information. Zhan and Tan (2020) propose an analytic framework for collecting extensive data to enhance performance that comprises five main stages. Stage 1: data capture and management. Stage 2: data cleaning and integration. Stage 3: data analytics. Stage 4: competence set analysis-deduction graph. Stage 5: information interpretation and decision-making.
Digital adoption is a crucial aspect of these five main stages. For instance, in Stage 1, organizations must determine the data sources to create as much value as possible. Therefore, the company needs managers to assume tasks to be able to define the data to be captured and manage such data. In Stage 2, managers must classify and transfer data from different sources. Stage 3 must apply analytics and mining algorithms to identify competence-set information. Then, they use a deduction graph for competence-set analysis in Stage 4. Finally, in Stage 5, they generate a competence network and results for competence-set analysis and decision-making. Managers’ vigor, dedication, and absorption reduce the negative influence of complex job demands, such as sustained attention and timely reaction and deployment and maintenance. As a result, engaged managers are probably more likely to accept, integrate, use and promote new digital technology in their companies (Demerouti, 2022).
Technological innovation does not guarantee business success. Often, products and services can be easily copied, whereas business model innovation can provide more sustainable market success. Consequently, new product or service development efforts should be coupled with a business model that defines go-to-market and value-capturing strategies. Business models can change industries and drive exceptional growth (Johnson et al., 2008). Insight into these effects has caused business models to garner increasing attention in practice and research. The digital and platform economy’s innovative business models are built on software and internet-based technologies such as big data and artificial intelligence (BDAI).
The unprecedented development of information and communication technology (ICT) has led to a phenomenon known as digitalization. Business models are adopted, especially by young enterprises. In this scenario, classical business models funded predominantly on physical activities are disrupted and shifting toward digitalization. Therefore, digitalization affects an organization’s business model and all segments of society, including new relationships and interactions with organizations. Digitalization has consequences for all industries (Buyukozkan and Gocer, 2018).
Although many activities may be digitalized, talented professionals remain a strategic resource. Human capabilities are essential in helping and creating a business model in this new digital context (Sivathanu and Pillai, 2018). The human factor is also vital to a firm’s performance (Xanthopoulou et al., 2009b; Gunasekaran et al., 2017; Afshan and Motwani, 2018). The requirement for new human capabilities challenges an organization’s development. For instance, how can firms support data scientists’ formation? What is the role of the organization following job losses resulting from digitalization?
Croll and Yoskovitz (2013) propose five tasks that managers should implement to build a digital business model: (1) create the acquisition channel, (2) define the selling tactic, (3) formulate the revenue source, (4) build the digital product type, and (5) agree with the form of delivery. These tasks are part of the digital business model tasks. Table 1 shows digital managerial tasks, questions, and tactics.
In the current digital world, new business models and value creation are the mechanisms that allow new job demands and resources to emerge and, consequently, increase managers’ work engagement and task performance. These new job demands and resources are part of a new-thinking business model focused on managers’ work engagement. For instance, for all the digital managerial tasks presented in Table 1, BDAI not only promotes more information about customers (acquisition channel and selling tactic) but also, most importantly, increases the accuracy of this information (Buyukozkan and Gocer, 2018) thanks to the adoption of innovative technology (revenue model). Consequently, BDAI entails smart connections between organizations and their customers. Additionally, customer integration continues to be a vital subject related to an organization’s performance (product type and delivery framework; Al Halbusi et al., 2022) because managers’ work engagement involves the three dimensions of the construct: vigor, dedication, and absorption, especially concerning its cognitive dimensions: (1) vigor: energy and mental resistance; and (2) absorption: visual attention and concentration. Managers must use their energy, mental resistance, visual attention, and concentration to respond to these digital business model tasks.
This framework highlights the need for organizations to consider that managers’ work engagement is critical to success and needs to be managed and developed. Managers’ work engagement increases task performance and generates more resources to face new job demands. Furthermore, managers’ work engagement is positively related to managers’ task performance through job-fit (Venkatesh et al., 2003). Managers can create a better digital business model and adopt new digital technology for their companies when engaged. Consequently,
Proposition 1: Managers’ work engagement is positively related to task performance in digital adoption tasks and digital business model tasks.
Whereas meaningful job demands and resources can be found in almost every top management team, some job demands and resources are unique and related to the digital business context. For instance, physical demands, such as visiting customers across cities, are still essential for traditional real state managers. In contrast, cognitive demands are much more relevant for chief scientist officers in fintech companies who are dealing with the problem of finding the optimal portfolio of real estate assets in a digital platform.
JD-R model proposes two effects in which demands and resources may have a combined effect on managers’ work engagement and indirectly increase their task performance (Bakker and Demerouti, 2017). Firstly, job resources buffer the impact of job demands on the strain. Research has shown that job resources, such as social support, autonomy, performance feedback, and development opportunities, can face managerial demands, including the manager’s burnout (Xanthopoulou et al., 2009a). Therefore, managers with many personal resources can cope better with their job demands. Secondly, job demands amplify the impact of job resources on work engagement (Bakker and Demerouti, 2017). Bakker and Albrecht (2018) suggest that job resources become salient and have the most decisive positive impact on work engagement when job demands are high. When a manager is confronted with challenging job demands, job and personal resources become valuable and foster vigor, dedication, and absorption in the tasks. It includes the investment of cognitive, emotional, and behavioral energies in work, dramatically impacting job satisfaction and performance (Kahn, 1990; Rich et al., 2010).
Based on Bakker and Demerouti (2017), job demands and resources can evoke two separated processes in digital managerial tasks: (1) an energetic process of wearing out in which high job demands exhaust managers’ cognitive resources and may therefore lead to burnout and eventually ill health; and (2) a motivational process in which job resources boost managers’ work engagement. These characteristics do not differ from previous descriptions of employees’ work; nevertheless, digital work also forces managers to reflect on adopting technology and how these technologies will positively impact task performance (Andrade-Valbuena and Torres, 2018). The early focus of studies using the JD-R model centered primarily on front-line employees, nonmanagerial roles employees, and first-line managers. With our framework, we emphasize the analysis on the managers (middle managers and top managers). Further, past research study job attributes focused on inputs, repetitive tasks, traditional skills, clearly defined tasks, siloed metrics, and slow and methodical work (e.g., Luoma, 2000). While our conceptual study discusses the framework primarily focused on outputs, ad hoc activities, e-skills, unclear tasks, integrated functions, KPIs, and fast, agile, and efficient job attributes (e.g., Hofmann and Rusch, 2017).
Empirical evidence suggests that managers experiencing technological failures [e.g., chief technology officers (CTOs)] lead their teams in significant work challenges, such as achieving better standardization and continuously removing dysfunctional routines (Say and Vasudeva, 2020). Firms pursuing an aggressive technology strategy in industries where technology is a critical contingency, such as high R&D spending, encourage CTOs to concentrate entirely on the success of new innovative projects through a higher compensation scheme (Medcof and Lee, 2017). Hence, engaged managers who are highly active, enthusiastic about, and often fully immersed in their work should enhance organizational routines associated with digital managerial tasks. Table 2 compares traditional and digital managerial tasks using the fundamental characteristics of the JD-R framework.
Digital transformation has become mandatory for all firms and businesses. The new context is increased job demands as byproducts of technology introduction or an intrusion into private life facilitated by technology. For instance, evidence shows that the COVID-19 pandemic increases leader workload and work–family conflict. Technology can influence managers’ well-being and make them more exhausted and less engaged. Digitalization can provide opportunities and constraints for managers’ well-being and health and for managers’ abilities to participate and thus co-shape the developments they are affected (Wilkinson et al., 2021).
Demerouti (2022) states that technology takes over the treatment, manipulation, or assembly of smaller objects, parts, or information; the human operator must work with more cognitively or mentally demanding features that require processing a significant amount of data. Additionally, workload variation due to automation, from underload to rapid overload (Parker and Grote, 2022), requires managers to react on a timely basis. As Bainbridge (1983) noted, it is impossible for even a highly motivated human being to maintain adequate visual attention toward a source of information. In cognitive neuroscience, visual attention refers to cognitive operations that mediate the selection of relevant information and filter irrelevant information from cluttered graphic scenes. Currently, managers face more visual attention and timely reaction demands, such as following the latest, complex, balanced scorecard or other business intelligence tools. Job demands based on physical strength have shifted to the demand for timely reactions and sustained attention.
A large amount of data demands that managers pay visual attention and react opportunely. Managers need to respond quickly to various stimuli and then manage the information to deploy a creative/innovative solution to diverse business problems, such as reading algorithm black boxes, giving timely responses to customers and clients, or managing the relationships between the company and stakeholders/shareholders because of the latter’s requests through various digital platforms.
We argue that job demands change as managers take over maintenance and deployment tasks. Under certain conditions, firms can favor employment structures with less skilled routine work but more highly technical professional work related to control, planning, maintenance, and deployment. Combining big data and artificial intelligence with other technologies creates additional capabilities for digitally transformed firms. These capabilities can develop economies of scale and scope for the organization. Maintenance tasks are required to ensure optimal operating systems. Paradoxically, technology has supported and reduced specific tasks but has introduced new workloads associated with maintaining software, robots, and platforms that provide management control and monitoring. Managers involved in digital managerial tasks increase the job demands of surveillance and performance monitoring (Parker and Grote, 2022) associated with these digital environments.
On the other hand, managers face deployment tasks (Parker and Grote, 2022). Deployment is where data mining yields results that impact task performance. The Cross-Industry Standard Data Mining Process, known as CRISP-DM, is an open standard process framework that indicates approaches used by data mining practitioners. According to CRISP-DM (Shearer, 2000), managers use data information to improve the way to do business or generate new forms of income through a value proposition embedded in a digital business model. Managers must continuously acquire new knowledge to validate, sort, and analyze big data to make strategic decisions (Meijerink et al., 2021). These new tasks require specific know-how and skills related to exploration and exploitation.
In digital managerial tasks, the purpose of human engagement with machines has a dual process, where managers or highly skilled employees enhance their decision-making process by augmenting their managerial capabilities. Individual ambidexterity can be an appropriate personal resource for managers to help complete deployment tasks (Torres et al., 2015). However, low-qualified employees are reduced to only those aspects where robotic machines still lack capabilities. For example, the gig worker is controlled by digital platforms and algorithms. Still, gig managers can make critical decisions across different countries with detailed information in real-time (cf. Ravenelle, 2019).
Managers see their autonomy and job complexity augmented as there is a shift toward valuing “mental work” over “manual work” (Sennett, 2008). Human engagement is significantly mediated or replaced by mechanization and algorithmic control. The work process is highly structured and overdetermined; standardization is vital for efficiency and consistency. Most deployment and maintenance tasks are highly structured, involving significant time and cognitive stress. The effect of a job resource is dependent upon the micro-context. For example, job demands or other job resources are determined by the level and nature of job demands (van Veldhoven et al., 2020). In digital managerial tasks, job demands and resources are characterized by the micro-tasks described before (e.g., sustained attention, timely reaction, and maintenance and deployment). Therefore, the managers’ work engagement proposes that job demands can increase or decrease managers’ work engagement. This could be further explored for a more nuanced perspective of job demands. Thus,
Proposition 2: Sustained attention, timely reaction, and maintenance and deployment are job demands in digital managerial tasks.
Our proposed framework groups big data and artificial intelligence (BDAI) with several job resources, such as big data, predictive analysis, machine learning, and other computational algorithms. The traditional JD-R model considers resources in terms of physical capital, human capital, technological capital, and tangible or intangible capital (Gunasekaran et al., 2016). We consider big data a job resource because this body of knowledge is responsible for analyzing and extracting data from large sets that are too complex to be dealt with by traditional data-processing tools (Chen and Zhang, 2014). Big data resources include capturing, storing, analyzing, searching, sharing, transferring, visualization, querying, and updating information privacy and data sources (McAfee and Brynjolfsson, 2012). New interpretations of the JD-R model consider AI and automation because they enable a firm to deal with tasks that are difficult for humans because of the complexity of the operations and procedures (Gunasekaran et al., 2016).
Despite the numerous advancements of BDAI, a large part of the existing literature considers them to be domains related only to a firm’s processes and infrastructures (Chen and Zhang, 2014), while a few articles have put attention on the role of managerial cognition in facing new job demands based on BDAI (Caputo et al., 2019). An organization must develop BDAI acceptance and assimilation capabilities through BDAI routinization (Hazen et al., 2012). BDAI requires as much theoretical knowledge as it does a wide array of quantitative skills. Our framework supports the idea that BDAI is a job resource that can increase managers’ work engagement because it involves a cognitive process of knowledge assimilation.
Managers are more likely to feel more engaged when their company’s system and infrastructure have platforms and BDAI infrastructure because they need to address rapidly changing environments that integrate building and reconfigure internal and external data (Hazen et al., 2012). From a dynamic managerial capability perspective (Helfat and Peteraf, 2015), an organization needs to develop BDAI acceptance and assimilation capabilities through the mediating construct of BDAI routinization. Routinization is the permanent adjustment of an organization’s governance system to incorporate technology. Hazen et al. (2012) argue that routinization is the second stage of a threefold process: acceptance, routinization, and assimilation. Organizations must accept, routinize, and assimilate technologies to generate BDAI assimilation (Gunasekaran et al., 2016).
We argue that companies with BDAI resources could increase work engagement because managers perceive such resources to be relevant in dealing with new job demands. Caputo et al. (2019) state that it is necessary to accept, establish routines, and assimilate new technologies to obtain benefits. In this regard, in the BDAI process, the first stage is acceptance, routinization, and finally, assimilation. BDAI resources also include technology and market surveillance processes, enabling managers to understand the current and latest trends in industries and markets. BDAI assimilation empowers a manager to think about the impact of a technological product on its users and society in general because BDAI environments stimulate this type of thinking (Gunasekaran et al., 2016).
Automation is a new technology by which a process or procedure is performed without direct human assistance (Parker and Grote, 2022). For instance, managers can actively change the design of their jobs by choosing tasks, negotiating different job content, and assigning meaning to their tasks or jobs. The availability of healthy-designed employment and working conditions facilitates manager motivation and reduces stress (Demerouti, 2022).
Automation uses various equipment operating control systems, such as machinery, processes in factories, boilers, heat-treating ovens, switching on telephone networks, steering and stabilizing ships, aircraft, and other applications and vehicles with minimal or reduced human intervention. Following the analysis of Demerouti (2022), automation can contribute to work-related health if (a) they are designed to support the work of individuals, (b) individuals are in control and can craft their use, (c) job resources are maximized, and job demands are affordable, (d) economic growth is shared among stakeholders, including managers, and (e) authorities protect managers and employees.
Job crafting represents a bottom-up adjustment of the tasks and characteristics of the job to fit one’s preferences and find meaning in the position. According to the JD-R model, managers can craft their job by expanding (i.e., seeking resources and challenges) or reducing their scope (i.e., diminishing or optimizing demands; Demerouti, 2022). Automation and job crafting may be an excellent combination of resources to increase managers’ work engagement in digital managerial tasks. Accordingly, automation reduces cognitive and physical goals because it supports a manager’s digital managerial tasks. To turn BDAI and automation into job resources, we need to analyze the nature and amount of the job resource (Van Veldhoven et al., 2020), as well as the way the job resource is valued by the managers as regards the task goal under consideration. Then,
Proposition 3: Big data and artificial intelligence, and automation are job resources related to digital managerial tasks.
We recognize a unique personal resource called technological reflectiveness, an individual’s tendency to think about the impact of a technical product on different stakeholders, such as employees, customers, and shareholders (Schweitzer et al., 2015). Technological reflectiveness draws on theories on reflection and reflexivity (Trapnell and Campbell, 1999). The managers’ work engagement framework proposes that technological reflectiveness is a personal resource that can increase work engagement in the digital workplace characterized by digital managerial tasks. In fact, managers are more likely to feel more engaged when they have the individual tendency of technological reflectiveness (Andrade-Valbuena and Torres, 2018).
The literature about technological reflectiveness aims to understand which individuals are effective contributors to technical innovations because they take society into account. In light of companies’ R&D areas increasing endeavors to open up the innovation process and seize the abilities and skills of external sources in the innovation process (Gassmann, 2006), the measure of technological reflectiveness can be used to recruit externals with high technology reflectiveness scores to contribute to the innovation process. Despite the significance of technological reflectiveness, no previous studies link this resource to work engagement. Technological reflectiveness can supplement other job and personal resources, such as autonomy, supervisory encouragement, team support, and managerial self-efficacy (Tierney and Farmer, 2002), to achieve managers’ work engagement.
A creative work environment may enhance a manager’s feeling of control and resiliency, motivating them intrinsically (Amabile, 1997), a prerequisite for work engagement (Xanthopoulou et al., 2013). Managers in digital managerial tasks must reflect deeply on how new technologies impact technical solutions in the firm, market, and society (Heavin and Power, 2018). Technological reflectiveness is significantly related to the individual’s willingness to promote new technologies (Andrade-Valbuena and Torres, 2018). Hence, technological reflectiveness can be a crucial resource in achieving managers’ work engagement because thinking about the impact of a technical product on its users and society can be critical in digital environments (Venkatesh et al., 2003).
According to Mom et al. (2009), individual ambidexterity is a managerial orientation toward combining exploration-and exploitation-related activities within a particular timeframe. In the last decade, individual ambidexterity has become more concentrated on how leaders act ambidextrously to increase individual or group performance (Tushman and O’Reilly, 1996).
The digital JD-R approach offers some arguments to support the idea that individual ambidexterity can be a personal resource that can boost managers’ work engagement (McCauley et al., 1994). Managers prepare strategic plans that involve short-and long-term investments (e.g., capital expenditures). Such initiatives must be approved annually by the board of directors. Strategy formulation requires significant resource mobilization, coordination, and integration to maintain exploitation and exploration activities (Gibson and Birkinshaw, 2004).
Managers are involved in several tasks, such as information sharing and knowledge processing, that require balancing short-and long-term tensions (Rosenkopf and Nerkar, 2001). Moreover, managers can access the most valuable and diverse information to avoid separating explorative and exploitative behaviors (Sidhu et al., 2004).
Ambidextrous managers engage in complex cognitive processes such as integrative or paradoxical thinking to reconcile the tensions that may emerge in their pursuit of a range of different opportunities, goals, and needs that seem to conflict in terms of time horizon, risk profile, relationship to the current strategy, and managerial responsibilities (Audia et al., 2000). Ambidextrous managers are skilled at not stressing the polarity of seemingly conflicting opportunities, goals, and needs (March, 1991). They are motivated to develop creative solutions that combine all aspects by emphasizing their interrelatedness.
Another commonality is that ambidextrous managers are skilled and motivated to engage in a vast repertoire of different or opposing activities and roles, such as conducting routine and nonroutine activities, leadership roles, and performing entrepreneurial and creative tasks (Adler et al., 1999). Further, managers can shift attention quickly between such different behaviors and functions, depending on the situation and the broader interests of the organization (Bernett, 2008). In this sense, ambidextrous managers have been called multitaskers and generalists rather than specialists (Mom et al., 2015).
Previous studies indicate that ambidextrous managers have the skills and motivation to engage in learning activities, such as reliability enhancement, and increase learning activities to refine and refresh their knowledge and skills (Torres et al., 2015). They build internal linkages to cooperate, combine efforts with others, and develop and maintain rather large, dense personal networks to share knowledge and information (Van Wijk et al., 2008).
We believe that individual ambidexterity can be a critical personal resource for managers that fosters explorative and exploitative behavior in managers by increasing or reducing the flexibility of desirable behavior (Mom et al., 2009). Considering the nature of digital managerial tasks, these individual tendencies of technological reflectiveness and individual ambidexterity can be essential personal resources for managers’ task performance. Therefore,
Proposition 4: Technological reflectiveness and individual ambidexterity are personal resources related to digital managerial tasks.
This conceptual article has important theoretical implications for organizational behavior and strategic management scholars interested in digital managerial tasks. First, we highlighted the central role of understanding managers’ work engagement in digital managerial tasks. We explain how digital businesses can affect job demands and resources with consequences for task performance. We also argue that managers’ work engagement positively relates to task performance in digital adoption and digital business model tasks.
The framework presented in this paper links digital managerial tasks with job demands and resources using the traditional JD-R model (Bakker and Demerouti, 2017). Hence, companies should be explicitly considered human-centered design principles such as work engagement in developing and procuring new technologies in their digital adoption strategies and the design process of new digital business models (cf. Table 1).
We propose that sustained attention, timely reaction, and maintenance and deployment are job demands for digital managerial tasks. Theoretically, the selection of a new digital task involves job demands that managers should perceive as relevant for training new executives (Gunasekaran et al., 2017). Managers must formulate investment plans to acquire new job resources related to digital managerial tasks, such as BDAI and automation. Otherwise, the imbalance between the new job demands and the lack of new job resources can trigger managers to fall into negative states of mind, such as exhaustion or burnout. Our theoretical framework proposes that companies with digital managerial tasks should facilitate the development of new job and personal resources to make new job demands affordable (Demerouti, 2022). We discussed that technological reflectiveness and individual ambidexterity are personal resources for managers involved in digital managerial tasks (Torres et al., 2015; Andrade-Valbuena and Torres, 2018). Furthermore, companies with digital business models must focus on upskilling and reskilling managers, board members, and other stakeholders to help them adapt to technology shifts (Meijerink et al., 2021). Finally, all these contributions open exciting avenues for further development, like empirical studies that confirm or challenge our propositions.
This paper also contributes to practice. In particular, it emphasizes the importance of managers increasing their level of work engagement. We suggest that companies with digital business models formulate long-term investments to increase BDAI assimilation and automation. Additionally, the chief executive officer (CEO), the top management team (TMT), and middle managers must understand the boundaries of the automation process in their organization and develop and train specific personal resources to make strategic decisions (Torres et al., 2015). New digital demands put pressure on managers to acquire more personal resources continuously. The development of personal resources also presents companies with challenges of how managers overcome cognitive limitations to exploit new resources (Dierickx and Cool, 1989).
Nevertheless, from this cognitive perspective, managers’ mental limitations also affect how they understand and respond to new job demands, but above all, how managers make decisions in dynamic and complex environments (Torres et al., 2017). Companies with digital business models should consider that a misperception of job resources might affect how managers cope with new job demands (e.g., difficulty understanding and perceiving new job resources; Sterman and Dogan, 2015). In such a line, the JD-R model could trace new elements based on which managerial cognition researchers can improve efficiency in the relationship between a firm’s human capital and the digital operations dimension (Tehseen et al., 2021).
Finally, using the JD-R model, our theoretical framework describes several contingency factors that may predict managers’ well-being and task performance. For instance, a company needs to generate managers’ work engagement to perform better. Engaged managers are more creative in promoting an innovative climate for other employees and have better creative performance (Juyumaya and Torres, 2023). Firms need to address these issues via diverse strategies, such as talent management, training and development, and incentive programs to handle manager work engagement in the workplace (Asija and Ringov, 2021). Firms must integrate work engagement into the strategy and operations to design new and better job positions in the proposed JD-R framework (cf. Figure 1).
The conceptual framework presented here opens up several exciting avenues for further research. A new venue is adopting a managerial cognitive perspective to explain how managers deal with new job demands. Drawing upon the JD-R model, organizational psychology, organizational behavior and strategic management researchers can discover new biases and decision-making heuristics when managers adopt digital managerial tasks (Tversky and Kahneman, 1974). Besides, the individual’s quest for more job and personal resources is a crucial challenge for further research. Nearby to personal resources, the JD-R model can be expanded to consider personal demands (Bakker and Demerouti, 2017). Future studies could explore how managers face their cognitive limitations (e.g., misperception) to face new job and personal demands.
Due to managers facing more visual attention and timely reaction demands, visual perception play a crucial role. New research can analyze the manager’s visual strategies to understand work engagement and task performance. Scholars can use the lens of the JD-R model to explain shifts in work engagement or one of the work engagement dimensions, such as absorption. Owing to the nature of visual perception, researchers can use neuroscience methods (e.g., eye-tracking data) to develop a multifaceted investigation about managers’ work engagement linking the new job demands and resources presented in this paper.
Another essential direction is the empirical test of our four research propositions using scales that can capture new job demands and resources. On the other hand, qualitative researchers can collect individuals’ perceptions to build new definitions of the job demands, job resources, and personal resources presented in this research. For instance, managers’ work engagement stories could be collected to analyze the degree of technological reflectiveness and individual ambidexterity on managers.
Since its inception, the JD-R model has inspired hundreds of studies and papers to predict positive and negative consequences of job resources and job demands on employees’ states of mind in the workplace. We further develop and understand managers’ work engagement in the context of digital managerial tasks and complement the traditional JD-R model by studying managers’ work engagement. Introducing new job demands and resources helps managers, scholars, researchers, and policymakers understand the manager’s psychological consequences of implementing digital business models. In this line, this work aims to inspire research to increase the opportunities to build manager well-being and achieve organizational functioning considering the challenging digital world of the following years of the XXI century. We hope this research contributes to developing new theoretical and empirical studies about managers’ work engagement and digital managerial tasks.
JJ supervised, conceptualized, visualized, edited, and wrote the article. JT conceptualized, visualized, edited, and wrote the article. All authors contributed to the article and approved the submitted version.
This article was supported by the Agencia Nacional de Investigación y Desarrollo (ANID) under grant Beca de Doctorado Nacional 21190010 and grant Fondecyt de Iniciación 11190146.
The authors declare that the research was conducted in the absence of any commercial or financial relationships that could be construed as a potential conflict of interest.
All claims expressed in this article are solely those of the authors and do not necessarily represent those of their affiliated organizations, or those of the publisher, the editors and the reviewers. Any product that may be evaluated in this article, or claim that may be made by its manufacturer, is not guaranteed or endorsed by the publisher.
Adler, P., Goldoftas, B., and Levine, D. (1999). Flexibility versus efficiency? A case study of model changeovers in the Toyota production system. Organ. Sci. 10, 43–68. doi: 10.1287/orsc.10.1.43
Afshan, N., and Motwani, J. (2018). The mediating role of customer-related performance outcomes on the relationship between customer integration and firm performance. BIJ 25, 2184–2197. doi: 10.1108/BIJ-11-2016-0178
Al Halbusi, H., Alhaidan, H., Abdelfattah, F., Ramayah, T., and Cheah, J. H. (2022). Exploring social media adoption in small and medium enterprises in Iraq: pivotal role of social media network capability and customer involvement. Tech. Anal. Strat. Manag., 1–18. doi: 10.1080/09537325.2022.2125374
Amabile, T. M. (1997). Entrepreneurial creativity through motivational synergy. J. Creat. Behav. 31, 18–26. doi: 10.1002/j.2162-6057.1997.tb00778.x
Andrade-Valbuena, N., and Torres, J. P. (2018). Technological reflectiveness from a managerial capability perspective. Technol. Forecast. Soc. Chang. 134, 84–97. doi: 10.1016/j.techfore.2018.05.019
Annarelli, A., Battistella, C., Nonino, F., Parida, V., and Pessot, E. (2021). Literature review on digitalization capabilities: cocitation analysis of antecedents, conceptualization, and consequences. Technol. Forecast. Soc. Chang. 166, 120635–120665. doi: 10.1016/j.techfore.2021.120635
Asija, A., and Ringov, D. (2021). Dynamic capabilities: the role of board monitoring and managerial incentives. Bus. Res. Q. 24, 268–280. doi: 10.1177/2340944420916309
Audia, P. G., Locke, E. A., and Smith, K. G. (2000). The paradox of success: an archival and a laboratory study of strategic persistence following radical environmental change. Acad. Manag. J. 43, 837–853. doi: 10.2307/1556413
Bainbridge, L. (1983). Ironies of automation. Automatica 19, 775–779. doi: 10.1016/0005-1098(83)90046-8
Bakker, A. B., and Albrecht, S. (2018). Work engagement: current trends. Career Dev. Int. 23, 4–11. doi: 10.1108/CDI-11-2017-0207
Bakker, A. B., Albrecht, S. L., and Leiter, M. P. (2011). Key questions regarding work engagement. Eur. J. Work Organ. Psychol. 20, 4–28. doi: 10.1080/1359432X.2010.485352
Bakker, A. B., and Costa, P. (2014). Chronic job burnout and daily functioning: a theoretical analysis. Burn. Res. 1, 112–119. doi: 10.1016/j.burn.2014.04.003
Bakker, A. B., and Demerouti, E. (2017). Job demands-resources theory: taking stock and looking forward. J. Occup. Health Psychol. 22, 273–285. doi: 10.1037/ocp0000056
Bakker, A. B., Schaufeli, W. B., Leiter, M. P., and Taris, T. W. (2008). Work engagement: an emerging concept in occupational health psychology. Work Stress. 22, 187–200. doi: 10.1080/02678370802393649
Bernett, M. (2008). An attention-based view of real options reasoning. Acad. Manag. Rev. 33, 606–628. doi: 10.5465/amr.2008.32465698
Breevaart, K., Bakker, A. B., Demerouti, E., and van den Heuvel, M. (2015). Leader-member exchange, work engagement, and job performance. J. Manag. Psychol. 30, 754–770. doi: 10.1108/JMP-03-2013-0088
Brougham, D., and Haar, J. (2018). Smart technology, artificial intelligence, robotics, and algorithms (STARA): employees’ perceptions of our future workplace. J. Manag. Organ. 24, 239–257. doi: 10.1017/jmo.2016.55
Buyukozkan, G., and Gocer, F. (2018). Digital supply chain: literature review and a proposed framework for future research. Comput. Ind. 97, 157–177. doi: 10.1016/j.compind.2018.02.010
Caputo, F., Cillo, V., Candelo, E., and Liu, Y. P. (2019). Innovating through digital revolution: the role of soft skills and big data in increasing firm performance. Manag. Decis. 57, 2032–2051. doi: 10.1108/MD-07-2018-0833
Chen, C. L. P., and Zhang, C. Y. (2014). Data-intensive applications, challenges, techniques, and technologies: a survey on big data. Inf. Sci. 275, 314–347. doi: 10.1016/j.ins.2014.01.015
Christian, M. S., Garza, A. S., and Slaughter, J. E. (2011). Work engagement: a quantitative review and test of its relations with task and contextual performance. Pers. Psychol. 64, 89–136. doi: 10.1111/j.1744-6570.2010.01203.x
Crawford, E. R., LePine, J. A., and Rich, B. L. (2010). Linking job demands and resources to employee engagement and burnout: a theoretical extension and meta-analytic test. J. Appl. Psychol. 95, 834–848. doi: 10.1037/a0019364
Croll, A., and Yoskovitz, B. (2013). Lean Analytics: Use Data to Build a Better Startup Faster. Sebastopol, CA: O’Reilly.
Demerouti, E. (2022). Turn digitalization and automation to a job resource. Appl. Psychol. 71, 1205–1209. doi: 10.1111/apps.12270
Demerouti, E., Bakker, A. B., and Bulters, A. J. (2004). The loss spiral of work pressure, work-home interference, and exhaustion: reciprocal relations in a three-wave study. J. Vocat. Behav. 64, 131–149. doi: 10.1016/S0001-8791(03)00030-7
Demerouti, E., Bakker, A. B., Nachreiner, F., and Schaufeli, W. B. (2001). The job demands-resources framework of burnout. J. Appl. Psychol. 86, 499–512. doi: 10.1037/0021-9010.86.3.499
Dierickx, I., and Cool, K. (1989). Asset stock accumulation and sustainability of competitive advantage. Manag. Sci. 35, 1504–1511. doi: 10.1287/mnsc.35.12.1504
El-Kot, G., and Burke, R. (2010). Work engagement among managers and professionals in Egypt. Afr. J. Econ. Manag. Stud. 1, 42–60. doi: 10.1108/20400701011028158
Gassmann, O. (2006). Opening up the innovation process: toward an agenda. R&D Manag. 36, 223–228. doi: 10.1111/j.1467-9310.2006.00437.x
Gibson, C. B., and Birkinshaw, J. (2004). The antecedents, consequences, and mediating role of organizational ambidexterity. Acad. Manag. J. 47, 209–226. doi: 10.2307/20159573
Gunasekaran, A., Papadopoulos, T., Dubey, R., Wamba, S. F., Childe, S. J., Hazen, B., et al. (2017). Big data and predictive analytics for supply chain and organizational performance. J. Bus. Res. 70, 308–317. doi: 10.1016/j.jbusres.2016.08.004
Gunasekaran, A., Tiwari, M. K., Dubey, R., and Wamba, S. F. (2016). Big data and predictive analytics applications in supply chain management. Comput. Ind. Eng. 101, 525–527. doi: 10.1016/j.cie.2016.10.020
Hakanen, J. J., Bakker, A. B., and Demerouti, E. (2005). How dentists cope with their job demands and stay engaged: the moderating role of job resources. Eur. J. Oral Sci. 113, 479–487. doi: 10.1111/j.1600-0722.2005.00250.x
Hazen, B. T., Overstreet, R. E., and Cegielski, C. G. (2012). Supply chain innovation diffusion: going beyond adoption. Int. J. Logist. Manag. 23, 119–134. doi: 10.1108/09574091211226957
Heavin, C., and Power, D. J. (2018). Challenges for digital transformation – towards a conceptual decision support guide for managers. J. Decis. Syst. 27, 38–45. doi: 10.1080/12460125.2018.1468697
Helfat, C., and Peteraf, M. (2015). Managerial cognitive capabilities and the microfoundations of dynamic capabilities. Strateg. Manag. J. 36, 831–850. doi: 10.1002/smj.2247
Hofmann, E., and Rusch, M. (2017). Industry 4.0 and the current status as well as prospects on logistics. Comput. Ind. 89, 23–34. doi: 10.1016/j.compind.2017.04.002
Holman, D., and Axtell, C. (2015). Can job redesign interventions influence a broad range of employee outcomes by changing multiple job characteristics? A quasi-experimental study. J. Occup. Health Psychol. 21, 284–295. doi: 10.1037/a0039962
Hsieh, Y. Y., and Vergne, J. P. (2022). The future of the web? The coordination and early-stage growth of decentralized platforms. Strateg. Manag. J. 1–29. doi: 10.1002/smj.3455
Johnson, G., Scholes, K., and Whittington, R. (2008). Exploring Corporate Strategy: Text and Cases. 8th Edn. Harlow: Prentice-Hall.
Jong, J., and Ford, M. T. (2016). The lagged effects of job demands and resources on organizational commitment in federal government agencies: a multi-level analysis. J. Public Adm. Res. Theory 26, 475–492. doi: 10.1093/jopart/muv040
Juyumaya, J. (2022). How psychological empowerment impacts task performance: the mediation role of work engagement and moderating role of age. Front. Psychol. 13:889936. doi: 10.3389/fpsyg.2022.889936
Juyumaya, J., and Torres, J. P. (2020). Work engagement in a digital disruption era: new job demands and resources. Acad.Manag. Proc. 2020:17251. doi: 10.5465/AMBPP.2020.17251abstract
Juyumaya, J., and Torres, J. P. (2023). Effects of transformational leadership and work engagement on managers’ creative performance. Balt. J. Manag. 18, 34–53. doi: 10.1108/BJM-11-2021-0449
Kahn, W. A. (1990). Psychological conditions of personal engagement and disengagement at work. Acad. Manag. J. 33, 692–724. doi: 10.2307/256287
Luoma, M. (2000). Developing people for business success: capability-driven HRD in practice. Manag. Decis. 38, 145–153. doi: 10.1108/EUM0000000005342
Mäkikangas, A., Schaufeli, W., Tolvanen, A., and Feldt, T. (2013). Engaged managers are not workaholics: evidence from a longitudinal person-centered analysis. J. Work Organ. Psychol. 29, 135–143. doi: 10.5093/tr2013a19
March, J. G. (1991). Exploration and exploitation in organizational learning. Organ. Sci. 2, 71–87. doi: 10.1287/orsc.2.1.71
McAfee, A., and Brynjolfsson, E. (2012). Big data: the management revolution. Harv. Bus. Rev., 90, 60–66, 68, 128
McCauley, C. D., Ruderman, M. N., Ohlott, P. J., and Morrow, J. E. (1994). Assessing the developmental components of managerial jobs. J. Appl. Psychol. 79, 544–560. doi: 10.1037/0021-9010.79.4.544
Medcof, J. W., and Lee, T. (2017). The effects of the chief technology officer and firm and industry R&D intensity on organizational performance. R&D Manag. 47, 767–781. doi: 10.1111/radm.12275
Meijerink, J., Boons, M., Keegan, A., and Marler, J. (2021). Algorithmic human resource management: synthesizing developments and cross-disciplinary insights on digital HRM. Int. J. Hum. Resour. Manag. 32, 2545–2562. doi: 10.1080/09585192.2021.1925326
Mom, T., Fourné, S., and Jansen, J. (2015). Managers’ work experience, ambidexterity, and performance: the contingency role of the work context. Hum. Resour. Manag. 54, s133–s153. doi: 10.1002/hrm.21663
Mom, T., Van Den Bosch, F., and Volberda, H. (2009). Understanding variation in managers’ individual ambidexterity: investigating direct and interaction effects of formal structural and personal coordination mechanisms. Organ. Sci. 20, 812–828. doi: 10.1287/orsc.1090.0427
Parker, S. K., and Grote, G. (2022). Automation, algorithms, and beyond: why work design matters more than ever in a digital world. Appl. Psychol. 71, 1171–1204. doi: 10.1111/apps.12241
Ravenelle, A. (2019). Hustle and Gig: Struggling and Surviving in the Sharing Economy. Berkeley: Univ. Calif. Press.
Reina-Tamayo, A., Bakker, A. B., and Derks, D. (2017). Episodic demands, resources, and engagement. J. Pers. Psychol. 16, 125–136. doi: 10.1027/1866-5888/a000177
Rich, B. L., Lepine, J. A., and Crawford, E. R. (2010). Job engagement: antecedents and effects on job performance. Acad. Manag. J. 53, 617–635. doi: 10.5465/amj.2010.51468988
Rosenkopf, L., and Nerkar, A. (2001). Beyond local search: boundary spanning, exploration, and impact in the optical disk industry. Strateg. Manag. J. 22, 287–306. doi: 10.1002/smj.160
Say, G., and Vasudeva, G. (2020). Learning from digital failures? The effectiveness of firms’ divestiture and management turnover responses to data breaches. Strategy Sci. 5, 117–142. doi: 10.1287/stsc.2020.0106
Schaufeli, W. B., and Bakker, A. B. (2010). “We are defining and measuring work engagement: bringing clarity to the concept” in Work Engagement: A Handbook of Essential Theory and Research. eds. A. B. Bakker and M. P. Leiter (London, UK: Psychology Press), 10–24.
Schaufeli, W. B., and Salanova, M. (2007). Efficacy of inefficacy is the question: burnout and work engagement and their relationships with efficacy beliefs. Anxiety Stress Coping 20, 177–196. doi: 10.1080/10615800701217878
Schaufeli, W. B., Taris, T. W., and Van Rhenen, W. (2008). Workaholism, burnout, and work engagement: three of a kind or three different kinds of employee well-being? Appl. Psychol. 57, 173–203. doi: 10.1111/j.1464-0597.2007.00285.x
Schweitzer, F., Rau, C., Gassmann, O., and van den Hende, E. (2015). Technologically reflective individuals as enablers of social innovation. J. Prod. Innov. Manag. 32, 847–860. doi: 10.1111/jpim.12269
Shearer, C. (2000). The CRISP-DM framework: the new blueprint for data mining. J. Data Warehousing 5, 13–22.
Sidhu, J. S., Volberda, H. W., and Commandeur, H. R. (2004). Exploring exploration orientation and its determinants: some empirical evidence. J. Manag. Stud. 41, 913–932. doi: 10.1111/j.1467-6486.2004.00460.x
Sidner, C. L., Lee, C., Kidd, C. D., Lesh, N., and Rich, C. (2005). Explorations in engagement for humans and robots. Artif. Intell. 166, 140–164. doi: 10.1016/j.artint.2005.03.005
Sivathanu, B., and Pillai, R. (2018). Smart HR 4.0-how industry 4.0 is disrupting HR. Hum. Resour. Manag. Int. Dig. 26, 7–11. doi: 10.1108/HRMID-04-2018-0059
Sohn, K., and Kwon, O. (2020). Technology acceptance theories and factors influencing artificial intelligence-based intelligent products. Telematics Inform. 47:101324. doi: 10.1016/j.tele.2019.101324
Sterman, J. D., and Dogan, G. (2015). “I’m not hoarding, i’m just stocking up before the hoarders get here.”: behavioral causes of phantom ordering in supply chains. J. Oper. Manag. 39–40, 6–22. doi: 10.1016/j.jom.2015.07.002
Tanveer, A., Zeng, S., Irfan, M., and Peng, R. (2021). Do perceived risk, perception of self-efficacy, and openness to technology matter for solar PV adoption? An application of the extended theory of planned behavior. Energies 14:5008. doi: 10.3390/en14165008
Tehseen, S., Johara, F., Halbusi, H. A., Islam, M. A., and Fattah, F. A. M. A. (2021). Measuring dimensions of perceived business success among Malaysian and Bangladeshi SME owners. Rajagiri Manag. J. doi: 10.1108/RAMJ-05-2021-0045 [Epub ahead of print].
Tierney, P., and Farmer, S. M. (2002). Creative self-efficacy: its potential antecedents and relationship to creative performance. Acad. Manag. J. 45, 1137–1148. doi: 10.2307/3069429
Torres, J. P., Drago, C., and Aqueveque, C. (2015). Knowledge inflows effects on middle managers’ ambidexterity and performance. Manag. Decis. 53, 2303–2320. doi: 10.1108/MD-04-2015-0133
Torres, J. P., Kunc, M., and O’Brien, F. (2017). Supporting strategy using system dynamics. Eur. J. Oper. Res. 260, 1081–1094. doi: 10.1016/j.ejor.2017.01.018
Trapnell, P. D., and Campbell, J. D. (1999). Private self-consciousness and the five-factor framework of personality: distinguishing rumination from reflection. J. Pers. Soc. Psychol. 76, 284–304. doi: 10.1037/0022-3514.76.2.284
Tushman, M. L., and O’Reilly, C. A. (1996). Ambidextrous organizations: managing evolutionary and revolutionary change. Calif. Manag. Rev. 38, 8–29. doi: 10.2307/41165852
Tversky, A., and Kahneman, D. (1974). Judgment under uncertainty: heuristics and biases. Science 185, 1124–1131. doi: 10.1126/science.185.4157.1124
Van Veldhoven, M., Van den Broeck, A., Daniels, K., Bakker, A. B., Taveres, S., and Ogbonnaya, C. (2020). Challenging the universality of job resources: why, when, and for whom are they beneficial? Appl. Psychol. 69, 5–29. doi: 10.1111/apps.12211
Van Wijk, R., Jansen, J. J., and Lyles, M. A. (2008). Inter-and intra-organizational knowledge transfer: a meta-analytic review and assessment of its antecedents and consequences. J. Manag. Stud. 45, 830–853. doi: 10.1111/j.1467-6486.2008.00771.x
Venkatesh, V., Morris, M. G., Davis, G. B., and Davis, F. D. (2003). User acceptance of information technology: toward a unified view. MIS Q. 27, 425–478. doi: 10.2307/30036540
Wilkinson, A., Knoll, M., Mowbray, P. K., and Dundon, T. (2021). New trajectories in worker voice: integrating and applying contemporary challenges in the organization of work. Br. J. Manag. 32, 693–707. doi: 10.1111/1467-8551.12528
Xanthopoulou, D., Bakker, A. B., Demerouti, E., and Schaufeli, W. B. (2009a). Reciprocal relationships between job resources, personal resources, and work engagement. J. Vocat. Behav. 74, 235–244. doi: 10.1016/j.jvb.2008.11.003
Xanthopoulou, D., Bakker, A. B., Demerouti, E., and Schaufeli, W. B. (2009b). Work engagement and financial returns: a diary study on the role of job and personal resources. J. Occup. Organ. Psychol. 82, 183–200. doi: 10.1348/096317908X285633
Xanthopoulou, D., Bakker, A. B., and Fischbach, A. (2013). Work engagement among employees facing emotional demands the role of personal resources. J. Pers. Psychol. 12, 74–84. doi: 10.1027/1866-5888/a000085
Keywords: job demands – resources model, managers, work engagement, digital managerial tasks, digital tasks
Citation: Juyumaya J and Torres JP (2023) A managers’ work engagement framework for the digital tasks. Front. Psychol. 14:1009459. doi: 10.3389/fpsyg.2023.1009459
Received: 01 August 2022; Accepted: 06 January 2023;
Published: 24 January 2023.
Edited by:
Majid Murad, Jiangsu University, ChinaReviewed by:
Nelson Andres Andrade Valbuena, Catholic University of the Most Holy Conception, ChileCopyright © 2023 Juyumaya and Torres. This is an open-access article distributed under the terms of the Creative Commons Attribution License (CC BY). The use, distribution or reproduction in other forums is permitted, provided the original author(s) and the copyright owner(s) are credited and that the original publication in this journal is cited, in accordance with accepted academic practice. No use, distribution or reproduction is permitted which does not comply with these terms.
*Correspondence: Jesus Juyumaya, ✉ amVzdXNqdXl1bWF5YWZ1QHNhbnRvdG9tYXMuY2w=
Disclaimer: All claims expressed in this article are solely those of the authors and do not necessarily represent those of their affiliated organizations, or those of the publisher, the editors and the reviewers. Any product that may be evaluated in this article or claim that may be made by its manufacturer is not guaranteed or endorsed by the publisher.
Research integrity at Frontiers
Learn more about the work of our research integrity team to safeguard the quality of each article we publish.