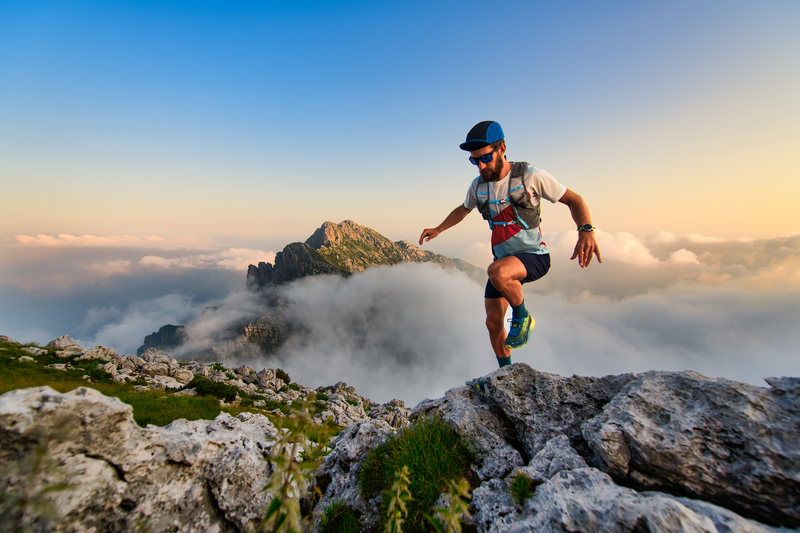
95% of researchers rate our articles as excellent or good
Learn more about the work of our research integrity team to safeguard the quality of each article we publish.
Find out more
ORIGINAL RESEARCH article
Front. Psychol. , 18 October 2022
Sec. Organizational Psychology
Volume 13 - 2022 | https://doi.org/10.3389/fpsyg.2022.999944
As organizations are benefiting from investments in big data analytics capabilities building and education, our study has analyzed the impact of big data analytics capabilities building and education on business model innovation. It has also assessed technological orientation and employee creativity as mediating and moderating variables. Questionnaire data from 499 managers at enterprises in Jiangsu, China have been analyzed using Structural Equation Modeling (SEM) in SmartPLS. Big data analytics capabilities building and education strengthen technological orientation and increase business model innovation. Technology orientation increases business model innovation and plays a mediating role. Employee creativity also boosts innovation. These findings show that business managers should adopt and promote a technological orientation. They should hire and train employees with big data education and training. Organizations can try to select and support employees who show creativity.
Big data has revolutionized old business models (McAfee et al., 2012). The main premise big data analytics builds on is that by analyzing large amounts of data from multiple sources, actionable insights can be extracted that can help businesses achieve an edge over rivals (Chen et al., 2012; Behl et al., 2022; Edu, 2022). Big data can provide valuable information (Hofacker et al., 2016) and provide competitive advantages (Morabito, 2015). Research has found that enterprises that use big data analytics can achieve higher levels of performance, in terms of innovation (Khan and Tao, 2022; Zheng et al., 2022). This is why organizations are making large-scale investments in big data analytics (SAS, 2013; Sharma et al., 2014). To find out more about this topic, big data analytics capabilities have been included in our research model as an antecedent of business model innovation.
Innovation is consistently ranked amongst the top priorities of business executives (Teece and Leih, 2016). The possibility to utilize big data analytics capabilities to pursue innovative strategies (Ciampi et al., 2020; Munir et al., 2022) is generating radical changes in business logics in many industries (Wang and Hajli, 2017; Santoro et al., 2018). To obtain more knowledge, business model innovation has been included as an outcome of big data analytics capabilities in our model.
A growing number of enterprises are making efforts to attain durable competitive advantages by utilizing technologies for business model innovation (Spieth et al., 2019). With support from technology, big data has emerged as the main tool helping businesses in exploitative and explorative activities (McAfee et al., 2012). As technology supports innovation (Dong and Yang, 2019), business capabilities can be utilized more productively if an organization has a technological orientation. Therefore, we have included technology orientation as a mediating variable in the relationship between big data analytics capabilities and business model innovation.
In this context, employee behavior that contributes to production and application of new ideas is favorable because it leads to organizational innovation (Van de Ven, 1986; Amabile, 1988; Scott and Bruce, 1994; Janssen, 2000; Shanker et al., 2017). This shows that dynamic capabilities, including those related to big data, are likely to generate more benefits if an organization has a creative workforce. So this research model includes direct and moderating effects of employee creativity.
The ongoing COVID-19 pandemic is a global challenge (Clark et al., 2020) and businesses need useful recovery mechanisms (Breier et al., 2021) because this crisis will negatively affect existing business models (Ritter and Pedersen, 2020). If major changes are made in the elements of a business model to achieve innovation (Foss and Saebi, 2017), new opportunities can be seized to recover from this crisis (Breier et al., 2021). This means it is important to conduct research to understand the factors affecting business model innovation.
For this research, we have selected China as the appropriate context. World Development Indicators show that China’s research and development expenditure increased 21.90% from 2011 to 2020. The country’s innovation index increased 14.87% over the same period. This shows that creativity and business model innovation are important areas for organizations in China so should be investigated.
The key objectives of our research are to analyze effects of big data analytics capabilities on business model innovation and technological orientation. They include assessing the mediating role of technological orientation and moderating effect of employee creativity. This framework is supported by the dynamic capabilities view. For our study, an online questionnaire was administered to mid-level and senior managers working at Chinese organizations. Data were analyzed using Structural Equation Modeling (SEM). It was found that big data analytics capabilities strengthen technological orientation and increase business model innovation. Technology orientation increases innovation and plays a mediating role in the relationship between big data capabilities and innovation. Employee creativity boosts business innovation. Creativity has a moderating effect on the relationship between big data capabilities and innovation.
This study has made multiple conceptual contributions. It has added to existing literature on the advantages of dynamic capabilities for businesses (Akter et al., 2016; Mikalef et al., 2019). We have responded to calls for more studies on the internal drivers of business model innovation (Martins et al., 2015; Saebi and Foss, 2015; Foss and Saebi, 2017; Frankenberger and Sauer, 2019). These drivers include technological orientation and employee creativity (Ciampi et al., 2021) Further, to the best of our knowledge, no past study has included both mediating and moderating variables in the relationship between big data analytics capabilities and business model innovation. These findings have also provided support for the dynamic capabilities view. To the best of our knowledge, past studies have not analyzed this conceptual model in the Chinese context so this research has made a contextual contribution as well.
This study has also generated valuable managerial implications. Findings show that to achieve business model innovation, managers should adopt and promote a technological orientation. They should also hire and train employees who are most capable of utilizing big data. Organizations should try to select and support employees who show creativity (Ciampi et al., 2021). Further, such workers should be placed in management positions. In this setting, top managers can play an important role (Barton and Court, 2012).
The next section of this paper presents the theoretical background and hypotheses. After that, the research method is described. The next section highlights results obtained. After that, a discussion of the results is provided. Theoretical and managerial implications are presented. Directions for future research are also offered. The paper ends with a conclusion.
Dynamic capabilities are defined as an organization’s abilities for creating, combining and reconfiguring resources to deal with environments that change very quickly (Teece et al., 1997). They include skills and knowledge useful for changing existing resources and generating new value (Teece, 2007; Day, 2014). Dynamic capabilities equip businesses for developing distinguishing procedures and skills, which are essential for retaining success as well as generating new opportunities (Fornell and Larcker, 1981).
The dynamic capabilities view is an appropriate framework for analyzing whether big data analytics capabilities can be leveraged to achieve business model innovation (Ciampi et al., 2021). This view is also appropriate for identifying relevant factors (Ciampi et al., 2021) such as technological orientation and employee creativity. Multiple relevant studies have applied dynamic capabilities as their main theoretical perspective (Akter et al., 2016; Wamba et al., 2017).
In today’s dynamic environment, businesses need to have the capacity to explore as well as exploit changes (Zheng et al., 2011). The dynamic capabilities view highlights the organizational ability to change quickly so it is a suitable theory, in this setting. Successful enterprises need to be data-driven (McAfee et al., 2012) and utilizing big data requires dynamic capabilities (McAfee et al., 2012). Further, dynamic capabilities stimulate innovation (Liao et al., 2009; Tellis et al., 2009; Wei and Lau, 2010). Supporting research has showed that there is a positive relationship between technology usage and organizational performance (Barba-Sanchez et al., 2018). These studies show relevance of the dynamic capabilities view in the contemporary business world. Applying the dynamic capability theory, Li et al. (2022) conducted a study in China. They found that big data analytics positively impact performance. This research highlights relevance of the dynamic capabilities view in the context of China.
The resource-based view is considered one of the most important theories for understanding how organizations can reach higher performance levels (Chae et al., 2014; Hitt et al., 2016a). In the context of technology-oriented businesses also, the theory is useful for improving performance (Cole et al., 2019). This theory states that a business is an amalgamation of different resources and heterogeneity amongst these explains differences in performance (Li et al., 2022). These resources include technological tools such as big data, technology orientation and employee creativity (Li et al., 2022). Keeping these factors in mind, we have selected the dynamic capabilities and resource-based views to support our model.
Big data analytics capabilities are defined as an enterprise’s distinguishing and unique abilities for using big data to obtain strategic insights (Mikalef et al., 2017). Business model innovation is the process of reconfiguring elements in the business value logic (Bucherer et al., 2012). This process requires significant adjustment of at least one core value element so it generates new ways of creating, proposing and/or capturing value (Amit and Zott, 2012). A business model is viewed as successful if it can stay relevant for stakeholders, over time (Gambardella and McGahan, 2010). In this area, businesses can utilize big data analytics to accurately predict environmental changes and update their structures accordingly (Gupta et al., 2020). Analytical data and tools can be utilized to generate business model innovation (Ransbotham and Kiron, 2017). Innovating businesses without the requisite capacities may die even though they are the best at innovation (Teece, 1986).
Supporting research has found that technologies are driving significant innovation in the business world (Mostaghel et al., 2022). Importantly, big data analytics capabilities have a positive impact on business model innovation (Ciampi et al., 2021). Further, the spreading of big data analytics has offered businesses options to upgrade their models (Porter and Heppelmann, 2015). Ransbotham and Kiron (2017) have stated that big data analytics help in detection of new business opportunities by blending diverse sources of data. In this area, big data analytics capabilities improve an organization’s performance in exploration activities (Rialti et al., 2019). They also improve an organization’s speed in generating required responses (Teece et al., 2016) because information flowing freely can clarify what action needs to be taken (Tarafdar and Qrunfleh, 2017). So big data analytics capabilities improve an organization’s capacity for innovation (Kiron et al., 2012, 2014). These dynamic capabilities have been found to positively impact exploratory activities and value creation in Chinese enterprises as well (Shamim et al., 2020).
Importantly, Mikalef et al. (2019) have discovered that big data analytics capabilities have a positive impact on incremental as well as radical innovations. Using big data to understand customers can generate incremental innovation (Story et al., 2011). Big data analytics capabilities can support enterprises in radical innovation, through development of new products or services which can create new markets or radically change existing markets (Erevelles et al., 2016).
In environments that are highly unpredictable, business model innovation can provide opportunities (Giesen et al., 2010) in terms of new methods of generating and capturing value (Amit and Zott, 2010). This is why business model innovation can be a strong response for the COVID-19 crisis. In China, both government and non-government organizations have actively utilized big data technology to prevent and control COVID-19 (Wu et al., 2020). In the country, this technology has played an important role in controlling the disease through tracking, early warnings, medical treatment, resource allocation and recovery efforts (Wu et al., 2020).
Another important contribution of big data analytics capabilities is in the area of environmental welfare. Green management of supply chains has gained a significant amount of attention in academia and business, as environmental awareness has increased (Wang et al., 2020). In China’s manufacturing sector, corporate social responsibility positively impacts green supply chain management and this relationship is strengthened by big data analytics capabilities (Wang et al., 2020). So these capabilities can improve an organization’s capacity to pursue novel and eco-friendly management practices.
Based on this discussion, our first hypothesis is.
H1: Big data analytics capabilities have a direct and positive effect on business model innovation
Past research has highlighted the impact of big data analytics capabilities on several strategic orientations (Ciampi et al., 2021). This shows the importance of investigating the association between big data analytics capabilities and a technological orientation. A technological orientation describes an enterprise’s idea openness and inclination to utilize new technologies as well as integrate these into products (Gatignon and Xuereb, 1997; Hurley and Hult, 1998; Li, 2005; Chen et al., 2014; Tsou et al., 2014). In this topic, Mikalef et al. (2018) have found that enterprises possessing big data analytics capabilities can obtain and analyze data for generating insights by utilizing their technology. So these organizations are more likely to have an inclination for technology.
Research has found that a combination of multiple factors, such as technology, is important for value generation through data. This means that big data can lead to a technology orientation. For example, Limbu et al. (2014) have discovered that a technological orientation can play an important role in the relationship between technological capabilities and organizational outcomes. Through technology, traditional business models can be improved and innovation can be achieved (Wang et al., 2021). In harmony with this, Ryu and Lee (2018) have found that big data analytics are used by businesses with a strong technology orientation to support their operations. Similarly, Mandal (2018) has found that big data analytics capabilities can improve performance and this relationship is supported by technology orientation. China’s Social Credit System is designed to centralize and build surveillance infrastructure, using big data from multiple sources (Liang et al., 2018). The goal is to predict and manage the reliability of citizens, organizations and the government (Liang et al., 2018). In this area, the country’s government is improving its surveillance capacity by using technologies (Creemers, 2017; Hoffman, 2018). So big data has led to the generation of a technological orientation.
Based on these findings, our next hypothesis is
H2: Big data analytics capabilities have a direct and positive effect on technological orientation
Wirtz et al. (2010) have stated that business model innovation is often the outcome of factors such as technology. Multiple studies have found that technology has positive effects on innovation (Morikawa, 2004; Joshi et al., 2010; Kleis et al., 2012; Mithas et al., 2012; Ravichandran et al., 2017; Saldanha et al., 2017; Dong and Yang, 2019). Further, digital transformation provides a boost for business innovation (Wang et al., 2022). In China, technology utilization has a positive impact on business innovation (Chen et al., 2015). So in businesses, a technological orientation is considered important for innovation and maintaining the edge in the market (Dinesh and Sushil., 2019).
Enterprises achieve this innovation through collaboration (Chesbrough and Bogers, 2014). In this area, success depends mostly on trust (Chesbrough and Bogers, 2014). Digital trust, a combination of trust and technology, is a powerful resource for keeping these networks intact (Mubarak and Petraite, 2020). It has been observed that blending knowledge from external sources is important for organizational innovation (Trantopoulos et al., 2017) and technological systems provide help in these operations (Haug et al., 2020). Even when businesses are separated by distance, technology helps them achieve innovation by enabling them to share knowledge (Rai et al., 2006; Nambisan et al., 2017). So technological orientation can be expected to generate business innovation.
Enterprises with this type of orientation are in a better position to develop innovative offerings (Fink and Neumann, 2009). Past studies have revealed that technology can lead to creation of new value propositions, based on better knowledge about customers (Ansari and Mela, 2003; Mithas et al., 2006). However, Hempell and Zwick (2008) have found that technology negatively affects an organization’s ability to innovate. Similar research has revealed that users sometimes respond to technological complexity by working outside these systems (Boudreau and Robey, 2005). This means that knowledge is not shared and innovation processes are negatively affected (Haug et al., 2020). So research has underlined both positive and negative effects of technological orientation, for business model innovation.
Based on this discussion, our third hypothesis is
H3: Technological orientation has a direct and positive effect on business model innovation
Limbu et al. (2014) have stated that an organization’s big data capabilities cannot guarantee improved performance because technological orientation may also be required. Supporting this assertion, research has found that technology systems alone are not sufficient for increasing innovation because technological skills, processes and infrastructure are also needed (Turulja and Bajgorić, 2016). This shows that big data analytics capabilities alone cannot be expected to increase business model innovation and technological orientation is also required.
In China, Zhou et al. (2005) have found that the stronger an enterprise’s technological orientation the larger the advantage offered by its innovation. Similar research has revealed that businesses that are more technology-oriented generate product innovations that are more radical (Gatignon and Xuereb, 1997). This is because technological orientation has a strong impact on how effectively an employee uses information (Hunter and Perreault Jr, 2006; Sundaram et al., 2007). So the information extracted through big data should be expected to improve business model innovation more when there is mediation by a technological orientation.
Relevant studies have investigated mediating variables in the relationship between big data capabilities and innovation, which shows the importance of such factors. Mikalef et al. (2019) have found that big data capabilities can improve dynamic capabilities which have a positive impact on innovation. So dynamic capabilities are a mediator in the relationship between big data capabilities and innovation. They have also found that when there is high environmental diversity, the impact of big data capabilities on innovation is stronger. Su et al. (2021) have found that big data analytics capabilities positively affect performance of Chinese organizations. They have also found that dual innovations mediate this relationship, strengthening the effect of capabilities on outcomes. Related research has showed that technology adoption helps businesses achieve innovation (Cuevas-Vargas et al., 2022).
Based on these findings, our next hypothesis is
H4: Technological orientation mediates the relationship between big data analytics capabilities and business model innovation
Creativity is valuable in the context of innovation (Joas and Beckert, 2002; El-Kassar et al., 2022). Creativity can be defined as the production of novel and useful ideas (Amabile, 1988). Creative ideas are the starting point of innovations (Amabile et al., 1996). Employee creativity positively affects an organization’s innovation capability because creative employees provide ideas that are raw materials for organizational innovation (Oldham and Cummings, 1996; Zhang and Bartol, 2010). This shows that employee creativity can have a direct positive effect on business model innovation. Since managers are also employees of a business, their creativity can also be expected to increase innovation. This is why Martensen and Dahlgaard (1999) have suggested organizations build cultures that encourage and effectively manage creativity. Supporting research has revealed that organisational outcomes are impacted by managerial characteristics (Hambrick and Mason, 1984).
Research has provided useful findings on this topic. Saether (2019) has found that when an organization supports creativity amongst employees, there is an increase in innovation. Chaubey et al. (2019) have found a positive relationship between employee creativity and organizational innovation. Creative employees supply a business with valued inputs for development and utilization of new processes, products and services (Bharadwaj and Menon, 2000). It has been observed that creative employees are likely to become role models for other employees, which leads to the latter also becoming idea generators (Shafique et al., 2019). This is an important finding because novel ideas often need to be mixed, for a breakthrough (Tushman and Anderson, 1986). So if there is a community of creative employees in an enterprise, there is a high probability of innovation (Tushman and Anderson, 1986). In China also, employee creativity has been found to be positively related to business innovation (Jiang et al., 2012).
Interestingly, exposure to foreign cultures improves creative performance of employees (Leung and Chiu, 2010; Maddux et al., 2010) and multicultural experiences increase creativity (Leung and Chiu, 2010). This type of creativity has been found to be positively related to business innovation in China (Jiang et al., 2012). This means that if employees possess foreign education or work experience, they are more likely to achieve business model innovation.
The main advantage of data-driven business model innovation is found in the opportunity to rationalize management’s intuitions and creativity (Cheah and Wang, 2017). Cao et al. (2019) have stated that creativity is necessary for leveraging big data because businesses must develop new analysis methods, which lead to understanding and implementation of new procedures. Therefore, it can be stated that employee creativity plays a moderating role in the relationship between big data analytics capabilities and business model innovation.
Based on this discussion, our next hypotheses are
H5: Employee creativity has a direct and positive effect on business model innovation
H5a: Employee creativity moderates the relationship between big data analytics capabilities and business model innovation so that this link is stronger at higher creativity levels
Research has found that businesses with different sizes adopt dissimilar innovation strategies (Damanpour, 1992) so business size has been included in our model as a control variable. One possible explanation is that larger enterprises have more resources for undertaking research and development (Clark, 2004; Fey and Birkinshaw, 2005). Past research has also discovered that adoption of innovation varies across industries (Bianchi et al., 2011). A possible reason is that organizations in different industries have divergent cultural values that influence their innovation efforts. So the industry of each business has been included as a control factor. Internationalization of enterprises has been found to impact performance, as well (Jantunen et al., 2008). One explanation could be that businesses fine-tune their strategies as the internationalization process happens and knowledge is gained (Jones and Coviello, 2005; Spence and Crick, 2009). Research has also found that employees’ foreign experience is positively related to business innovation (Yuan and Wen, 2018). Both foreign education and work experience positively impact innovation (Yuan and Wen, 2018). This is why firm internationalization is a relevant control variable for our model. Important differences in the elements of Business-to-Business (B2B) and Business-to-Consumer (B2C) markets lead to differences in innovation (Dotzel and Shankar, 2019) which shows that a business’s customer type can impact its performance in terms of innovation. So the customer type is a relevant control factor for our research. Relevant past research has also incorporated these control variables (Crespo Marquez et al., 2020).
The first set of questionnaires was in both Chinese and English. Before administering the final version, we conducted a pilot survey. For this purpose, questionnaires were administered to 30 Chinese and 25 foreign managers. Based on the feedback obtained through this and observations of Chinese language patterns, the final questionnaire was developed. It was administered online and included two parts. The first covered our model’s independent, dependent, mediating and moderating variables while the second covered our control variables. For this research, data was collected in the period from January to July 2021.
The minimum sample size is 212 for conducting SEM1 so our sample size is appropriate. Based on the findings of Hair et al. (2017b) and Cohen’s power theory (Cohen, 2013), we have assessed sample size adequacy. To confirm this sample’s statistical strength, we used the G*power post-hoc test for exogenous factors (with a significance level of 0.05), an effect size of 0.15 and a sample size of 380 (Statista, 2020). There were 4,154 companies listed on the Chinese stock exchange in 2020.2 We used the random sampling method to select 700 from these, representing the population of active local and multinational companies registered in China. This sample comprised mid-level and senior Chinese managers working at multinational companies (40%) and foreign managers at local companies (60%). We distributed 700 questionnaires (1 per organization) out of which 499 were completely filled. Only the complete ones were used for data analysis, so the response rate was 71.29%.
For measuring the constructs in our model, we utilized instruments used in relevant studies (Table 1). Big Data Analytics Capabilities was measured using 13 items, out of 15 (Ravichandran et al., 2005; Aydiner et al., 2019a, 2019b). Two items were dropped because their factor loadings were below 0.5 (Hair et al., 2010). For this construct, the value of Cronbach’s Alpha was 0.958. Business Model Innovation was measured with 5 statements (Asemokha et al., 2019) and Cronbach’s Alpha was 0.911. Technological Orientation was measured using 4 items (Gatignon and Xuereb, 1997) and Cronbach’s Alpha was 0.826. Employee Creativity was measured with 9 statements (Ettlie and O'Keefe, 1982; Tierney et al., 1999). For this construct, the value of Cronbach’s Alpha was 0.942. For these four constructs, a 5-point Likert scale from 1 (strongly disagree) to 5 (strongly agree) was used. Business size was represented by the number of employees (Naqshbandi, 2018). For the industry type, respondents had to choose the sector in which their business was operating (Naqshbandi, 2018). The degree of internationalization was represented by Total Foreign Revenue divided by Total Assets, in terms of US$ (Riahi-Belkaoui, 1999). For the customer type, respondents were asked whether their organizations served businesses or final consumers (Crespo et al., 2020).
Participation in this study was voluntary and the confidentiality of responses was guaranteed (Podsakoff, 2003), to lower the risk of Common Method Bias (CMB). Harman’s one-factor test was conducted to detect CMB (Harman, 1976). We found that the first factor accounted for 47.30% of the variance which is lower than the 50% limit so there is no evidence of CMB (Fuller et al., 2016). To address concerns about CMB, we also utilized established scales and divided the questionnaire into 5 parts, 4 covering our model variables and 1 covering the control variables (Ciampi et al., 2021).
We also compared the size and age of participating and non-participating businesses in our sample. ANOVA provided p values equal to 0.892 and 0.651 respectively, showing that there was no statistically significant difference. We also conducted t-tests, comparing the age and size of early and late responding firms (Armstrong and Overton, 1977). We did not find any statistically significant difference (p values 0.512 and 0.392, respectively).
SEM is a method that is appropriate for analyzing data to identify relationships between variables (Purwanto et al., 2021). In recent years the number of published articles using Partial Least Squares SEM (PLS-SEM) has increased significantly, compared with Covariance-Based SEM (CB-SEM; Hair et al., 2017b). PLS-SEM is now applied in many social science areas, including organizational management (Sosik et al., 2009). An important reason for this trend is that when using PLS-SEM researchers benefit from the higher statistical power, compared with CB-SEM (Reinartz et al., 2009; Hair et al., 2017b). This means that PLS-SEM is more likely to highlight relationships as significant when they are indeed present in the population (Sarstedt and Mooi, 2019). We selected PLS-SEM for data analysis after taking these factors into account. We applied PLS-SEM in the software SmartPLS v3 (Hair et al., 2014; Sarstedt et al., 2019). For hypothesis testing, bootstrapping was used. Moreover, we have used 5,000 bootstrap samples and the two-tailed test.
Results of our study are based on a sample of 499 respondents whose characteristics are presented in Table 1. The sample composition was 54.11% males and 45.89% females. Most of the respondents (48.49%) were in the age group from 31 to 45 years. Amongst the participants, 58.11% had more than 10 years of experience in their industry. Most belonged to the service (35.27%) and manufacturing (28.46%) sectors. In the context of organizational positions, 37.87% of respondents were senior managers and 24.25% were functional managers. In the context of size, 28.66% of the businesses had 1–50 employees and 17.03% had more than 500 employees. More than a quarter of the sample (25.45%) had firm internationalization of 5–9.99 million USD. Business customers were served by 55.11% of the sample while final consumers were served by 44.89%.
It is important to check the reliability and validity of measurement tools utilized in a study. Construct reliability and composite reliability have been assessed (Brown, 2002). The values are provided in Table 2. All are higher than the suggested threshold of 0.70 (Nunally and Bernstein, 1978). The Average Variance Extracted (AVE) values, also given in Table 2, have been used to assess convergent validity. The values are acceptable when compared with the generally used threshold of 0.50 (Henseler et al., 2016). Multicollinearity has been checked (Aiken et al., 1991) and shown in Table 2 in the form of Variance Inflation Factor (VIF) values. The values for all constructs are less than 5 and, therefore, acceptable (Ringle et al., 2015). Values of Rho_A are between Cronbach’s Alpha and composite reliability so are acceptable.
Discriminant validity has been assessed using the Fornell Larcker criterion and the Heterotrait Monotrait (HTMT) ratio, shown in Tables 3, 4 respectively. Both criteria are commonly recognized and other researchers have applied them (Henseler et al., 2016; Neneh, 2019a). Discriminant validity is defined as the square root of AVE (Fornell and Larcker, 1981) and HTMT values must be less than 0.85 (Henseler et al., 2016). The highest HTMT value is 0.123 which shows that all constructs possess discriminant validity.
SmartPLS v3 and the PLS algorithm approach have been deployed to analyze the structural model. The standardized root mean square residual value has been used to assess model fit, with a suggested value of 0.08 (Henseler et al., 2016). This model’s value is 0.048, indicating the model’s overall fitness. Figure 1 presents the R2 value, which shows that this model explains 15.90% of the variance in business model innovation. This R2 value is acceptable because it is not below 10% (Falk and Miller, 1992).
All the direct-effect hypotheses have been accepted. These results are shown in Table 5. Big data analytics capabilities have a significant and positive impact on business model innovation (β = 0.194**, t = 3.252, p < 0.01), providing support for H1. Big data analytics capabilities also have a significant positive effect on technological orientation (β = 0.278**, t = 5.033, p < 0.01) so H2 is accepted. There is significant and positive impact of technological orientation on business model innovation (β = 0.184*, t = 3.189, p < 0.01) so H3 is supported. There is a significant positive effect of employee creativity on business model innovation (β = 0.276**, t = 4.491, p < 0.01) so H5 has been accepted (Figures 2, 3).
Figure 2. Structural model. BDA = big data analytic capabilities, TCO = technological orientation, EMC = employee creativity, and BDI = business model innovation.
Figure 3. Measurement model. BDA = big data analytic capabilities, TCO = technological orientation, EMC = employee creativity, and BDI = business model innovation.
Technology orientation positively partially mediates the relationship between big data analytics capabilities and business model innovation (β = 0.051*, t = 2.450, p < 0.05), providing support for H4. The result is provided in Table 6, with Percentile Confidence Interval (PCI) and Variance Accounted For (VAF) values. To test for mediation 5,000 bootstrap sample were used along with two-tailed testing. To determine the strength of mediation effect Variance Accounted For (VAF) is computed. VAF value shows the ratio of beta co-efficient of indirect effect to the total effect. In SmartPLS software VAF is not calculated automatically therefore we have used the manual calculation to compute it by extracting the values from PLS algorithm. Moreover, the detail calculation is provided in Table 6 notes. If the VAF values comes out to be greater than 80% then it indicates a full mediation, if value lies between 20 and 80% it indicates partial mediation and if the value falls below 20% it indicates no mediation (Hair et al., 2011). The VAF value for this study is 20.8% which lies between 20 and 80% interval so there is partial mediation.
Employee creativity moderates the relationship between big data analytics capabilities and business model innovation (β = 0.114, t = 2.563, p < 0.05), providing support for H5a. Figure 4 further shows the moderating effect (Table 7).
To obtain meaningful and reliable results, we have also evaluated the model’s predictive power using Stone-Geisser’s Q2. It is a cross validity redundancy check performed using a blindfolding procedure. It particularly set omission distance of 7 as a criterion for predictive relevance (Janadari et al., 2016). The general rule is that Q2 values above 0 show the respective predictive relevance (Janadari et al., 2016). We have acquired the Q2 value by using the structural equation model. The Q2 value, presented in Table 8, is 0.126 which shows predictive relevance (Janadari et al., 2016).
There is a lack of empirical research on how big data can be utilized to achieve business innovation (Ciampi et al., 2021). Therefore, our empirical study has made a valuable contribution to existing knowledge about the impact of big data capabilities on business model innovation. It has also provided information about the roles of technological orientation and employee creativity, in this context. Our research has revealed that big data analytics capabilities increase business model innovation, which is consistent with results obtained before the pandemic (Kiron et al., 2012, 2014; Shamim et al., 2020). Big data capabilities strengthen technological orientation, aligned with results of studies before the pandemic (Mandal, 2018; Wang et al., 2021). Technological orientation increases innovation. This finding is also aligned with research completed before COVID-19 (Ravichandran et al., 2017; Saldanha et al., 2017; Dong and Yang, 2019). Technology orientation mediates the relationship between big data capabilities and innovation. This is supported by the work of Limbu et al. (2014), Sundaram et al. (2007) and Zhou et al. (2005) completed before COVID-19. We have found that employee creativity boosts business innovation. It also strengthens the positive relationships between big data capabilities and innovation. These findings are supported by studies conducted before the COVID-19 pandemic (Chaubey et al., 2019; Saether, 2019).
This study has made multiple conceptual contributions. First, it has added to existing literature on the benefits of dynamic capabilities for businesses (Akter et al., 2016; Mikalef et al., 2019). Second, we have responded to calls for more research on the internal drivers of business model innovation (Martins et al., 2015; Saebi and Foss, 2015; Foss and Saebi, 2017; Frankenberger and Sauer, 2019). These drivers include technological orientation and employee creativity (Ciampi et al., 2021). Further, to the best of our knowledge, no past study has included both mediating and moderating variables in the relationship between big data analytics capabilities and business model innovation. Third, the study findings have provided support for the dynamic capabilities view. To the best of our knowledge, past studies have not analyzed this conceptual model in the Chinese context. Therefore, this research has made a contextual contribution as well.
Our findings show that to achieve business model innovation, managers should utilize big data and related technologies. They should also hire and train employees who are most capable of utilizing such resources. Business management should adopt and promote a technological orientation, in this context. Another important implication is that organizations should try to select and support employees who show creativity (Ciampi et al., 2021). Further, such workers should be placed in management positions as they are likely to make positive contributions to business model innovation. In this setting, top managers can play an important role in decisions such as those related to technology infrastructure and recruitment of competent data professionals (Barton and Court, 2012). During the ongoing COVID-19 pandemic, business model innovation is even more important because it is a recovery option for enterprises that have been affected negatively.
Our research has certain limitations which should be viewed as opportunities for future studies. First, a survey with self-reporting was used which means that the threat of cognitive bias exists so future studies should use different methodologies. Second, our work was conducted in China only so researchers should analyze these relationships in different settings to find out if the associations hold. Multigroup analysis can be conducted, based on factors such as industrial sectors and developed/developing economies. This comparative analysis will add to existing knowledge. Third, other mediating variables can be investigated, such as learning orientation, in the relationship between big data capabilities and business performance (Ciampi et al., 2021). Competitive advantage (Anwar et al., 2018) and big data value creation (Shamim et al., 2020) can also play mediating roles. Important moderating factors include resistance to change (Shahbaz et al., 2019), risk tolerance (Hock-Doepgen et al., 2021) and the organizational environment (Mikalef et al., 2019) so these should be investigated to better understand the association between big data capabilities and business performance. Fourth, future researchers can assess the innovation performance of government and humanitarian organizations for a unique view of this topic.
Organizations that use big data analytics can achieve higher levels of performance, including business model innovation. The purpose of this research was to analyze the relationships between big data analytics capabilities and education, business model innovation, technological orientation and employee creativity. Online questionnaire data from a sample of 499 managers at Chinese enterprises was analyzed though PLS-SEM. It has been found that big data analytics capabilities strengthen technological orientation and increase business model innovation. Technological orientation also improves innovation. Employee creativity strengthens the positive relationship between big data capabilities and innovation. These results have provided multiple implications and created opportunities for future researchers. Our findings show that to achieve business model innovation, managers should utilise big data and other technologies. They should hire and train employees who are most capable of utilising such resources. Moreover, organizations should collaborate with education sector especially universities to foster big data analytics basic knowledge at the undergraduate and post graduate curriculum. Business management should adopt and promote a technological orientation. Organizations should try to select and support employees who show creativity (Ciampi et al., 2021). During the ongoing COVID-19 pandemic, innovation is even more important because it is a recovery option for businesses that have been affected negatively.
The raw data supporting the conclusions of this article will be made available by the authors, without undue reservation.
The Jiangsu University review board exempted the research from ethical approval as it was a survey-based study. Informed consent was obtained from all subjects involved in the study while collecting the data through an online questionnaire.
YC and SF contributed to developing the theoretical framework, data analysis, and overall writing of the manuscript. AA, MA, and ZA contributed to data collection and the writing, editing, and organization of the manuscript. All authors contributed to the article and approved the submitted version.
This work was supported by General Program of the Chinese Society of Academic Degrees and Graduate Education for Research and Practice of the Promotion of the Quality of Overseas Engineering Postgraduate Students Education through the Integration between Industry and University under Fund Serial Number (2020MSA350), China Education Association for International Exchange for Research and Practice of the Promotion of the Quality of Overseas Engineering Postgraduate Students Education through the Integration among Government, Industry, and University under Fund Serial Number (Jixieyan 2021-008), Chinese Society of Educational Development Strategy for Research of the Promotion of the Global Competence Cultivation of the Students from University relevant to agriculture through the Integration among Government, Industry and University under Fund Serial Number (SRB202131), and Major Program of the Chinese Society of Academic Degrees and Graduate Education for Exploration and Practice of the Cultivation Mode of the Students from Belt and Road Countries under Fund Serial Number (2020ZAC11).
We acknowledge the contribution of Ayaan Waqar in collection and cleaning of data for analysis.
The authors declare that the research was conducted in the absence of any commercial or financial relationships that could be construed as a potential conflict of interest.
All claims expressed in this article are solely those of the authors and do not necessarily represent those of their affiliated organizations, or those of the publisher, the editors and the reviewers. Any product that may be evaluated in this article, or claim that may be made by its manufacturer, is not guaranteed or endorsed by the publisher.
The Supplementary material for this article can be found online at: https://www.frontiersin.org/articles/10.3389/fpsyg.2022.999944/full#supplementary-material
1. ^https://www.danielsoper.com/statcalc/calculator.aspx?id=89
2. ^https://www.statista.com/statistics/225725/number-of-companies-listed-on-the-chinese-stock-exchange/
Aiken, L. S., West, S. G., and Reno, R. R. (1991). Multiple regression: Testing and interpreting interactions. Thousand Oaks, CA: Sage.
Akter, S., Wamba, S. F., Gunasekaran, A., Dubey, R., and Childe, S. J. (2016). How to improve firm performance using big data analytics capability and business strategy alignment? Int. J. Prod. Econ. 182, 113–131. doi: 10.1016/j.ijpe.2016.08.018
Amabile, T. M. (1988). A model of creativity and innovation in organizations. Res. Organ. Behav., 10Placeholder Text, 123–167.
Amabile, T. M., Conti, R., Coon, H., Lazenby, J., and Herron, M. (1996). Assessing the work environment for creativity. Acad. Manag. J. 39, 1154–1184. doi: 10.5465/256995
Amit, R., and Zott, C. (2010). Business model innovation: Creating value in times of change. SSRN Electron. J. 23, 1–17. doi: 10.2139/ssrn.1701660
Amit, R., and Zott, C. (2012). Creating value through business model innovation. MIT Sloan Manag. Rev. 53, 41–49.
Ansari, A., and Mela, C. F. (2003). E-customization. J. Mark. Res. 40, 131–145. doi: 10.1509/jmkr.40.2.131.19224
Anwar, M., Khan, S. Z., and Shah, S. Z. A. (2018). Big data capabilities and firm’s performance: a mediating role of competitive advantage. J. Inf. Knowl. Manag. 17:1850045. doi: 10.1142/S0219649218500454
Armstrong, J. S., and Overton, T. S. (1977). Estimating nonresponse bias in mail surveys. J. Mark. Res. 14, 396–402. doi: 10.1177/002224377701400320
Asemokha, A., Musona, J., Torkkeli, L., and Saarenketo, S. (2019). Business model innovation and entrepreneurial orientation relationships in SMEs: implications for international performance. J. Int. Entrep. 17, 425–453. doi: 10.1007/s10843-019-00254-3
Aydiner, A. S., Tatoglu, E., Bayraktar, E., and Zaim, S. (2019a). Information system capabilities and firm performance: opening the black box through decision-making performance and business process performance. Int. J. Inf. Manag. 47, 168–182. doi: 10.1016/j.ijinfomgt.2018.12.015
Aydiner, A. S., Tatoglu, E., Bayraktar, E., Zaim, S., and Delen, D. (2019b). Business analytics and firm performance: the mediating role of business process performance. J. Bus. Res. 96, 228–237. doi: 10.1016/j.jbusres.2018.11.028
Barba-Sanchez, V., Calderón-Milán, M. J., and Atienza-Sahuquillo, C. (2018). A study of the value of ICT in improving corporate performance: a corporate competitiveness view. Technol. Econ. Dev. Econ. 24, 1388–1407. doi: 10.3846/tede.2018.3114
Barton, D., and Court, D. (2012). Making advanced analytics work for you. Harv. Bus. Rev. 90, 78–83, 128.
Behl, A., Gaur, J., Pereira, V., Yadav, R., and Laker, B. (2022). Role of big data analytics capabilities to improve sustainable competitive advantage of MSME service firms during COVID-19–a multi-theoretical approach. J. Bus. Res. 148, 378–389. doi: 10.1016/j.jbusres.2022.05.009
Bharadwaj, S., and Menon, A. (2000). Making innovation happen in organizations: individual creativity mechanisms, organizational creativity mechanisms or both? J. Prod. Innov. Manage. 17, 424–434. doi: 10.1111/1540-5885.1760424
Bianchi, M., Cavaliere, A., Chiaroni, D., Frattini, F., and Chiesa, V. (2011). Organisational modes for open innovation in the bio-pharmaceutical industry: an exploratory analysis. Technovation 31, 22–33. doi: 10.1016/j.technovation.2010.03.002
Boudreau, M. C., and Robey, D. (2005). Enacting integrated information technology: a human agency perspective. Organ. Sci. 16, 3–18. doi: 10.1287/orsc.1040.0103
Breier, M., Kallmuenzer, A., Clauss, T., Gast, J., Kraus, S., and Tiberius, V. (2021). The role of business model innovation in the hospitality industry during the COVID-19 crisis. Int. J. Hosp. Manag. 92:102723. doi: 10.1016/j.ijhm.2020.102723
Bucherer, E., Eisert, U., and Gassmann, O. (2012). Towards systematic business model innovation: lessons from product innovation management. Creat. Innov. Manag. 21, 183–198. doi: 10.1111/j.1467-8691.2012.00637.x
Cao, G., Duan, Y., and El Banna, A. (2019). A dynamic capability view of marketing analytics: Evidence from UK firms. Ind. Mark. Manag. 76, 72–83. doi: 10.1016/j.indmarman.2018.08.002
Chae, B. K., Yang, C., Olson, D., and Sheu, C. (2014). The impact of advanced analytics and data accuracy on operational performance: a contingent resource based theory (RBT) perspective. Decis. Support. Syst. 59, 119–126. doi: 10.1016/j.dss.2013.10.012
Chaubey, A., Sahoo, C. K., and Khatri, N. (2019). Relationship of transformational leadership with employee creativity and organizational innovation: a study of mediating and moderating influences. J. Strateg. Manag. 12, 61–82. doi: 10.1108/JSMA-07-2018-0075
Cheah, S., and Wang, S. (2017). Big data-driven business model innovation by traditional industries in the Chinese economy. J. Chin. Econ. Foreign Trade Stud. 10, 229–251. doi: 10.1108/JCEFTS-05-2017-0013
Chen, H., Chiang, R. H., and Storey, V. C. (2012). Business intelligence and analytics: from big data to big impact. MIS Q. 36:1165. doi: 10.2307/41703503
Chen, Y., Tang, G., Jin, J., Xie, Q., and Li, J. (2014). CEO s’ transformational leadership and product innovation performance: the roles of corporate entrepreneurship and technology orientation. J. Prod. Innov. Manag. 31, 2–17. doi: 10.1111/jpim.12188
Chen, Y., Wang, Y., Nevo, S., Benitez-Amado, J., and Kou, G. (2015). IT capabilities and product innovation performance: the roles of corporate entrepreneurship and competitive intensity. Inf. Manag. 52, 643–657. doi: 10.1016/j.im.2015.05.003
Chesbrough, H., and Bogers, M. (2014). Explicating open innovation: Clarifying an emerging paradigm for understanding innovation. New Frontiers in Open Innovation. Oxford: Oxford University Press, Forthcoming, 3–28.
Ciampi, F., Demi, S., Magrini, A., Marzi, G., and Papa, A. (2021). Exploring the impact of big data analytics capabilities on business model innovation: the mediating role of entrepreneurial orientation. J. Bus. Res. 123, 1–13. doi: 10.1016/j.jbusres.2020.09.023
Ciampi, F., Marzi, G., Demi, S., and Faraoni, M. (2020). The big data-business strategy interconnection: a grand challenge for knowledge management. A review and future perspectives. J. Knowl. Manag. 24, 1157–1176. doi: 10.1108/JKM-02-2020-0156
Clark, C., Davila, A., Regis, M., and Kraus, S. (2020). Predictors of COVID-19 voluntary compliance behaviors: an international investigation. Glob. Transit. 2, 76–82. doi: 10.1016/j.glt.2020.06.003
Clark, T. (2004). Strategy viewed from a management fashion perspective. Eur. Manag. Rev. 1, 105–111. doi: 10.1057/palgrave.emr.1500004
Cohen, J. (2013). Statistical power analysis for the behavioral sciences. New York: Academic press. pp. 537.
Cole, R., Stevenson, M., and Aitken, J. (2019). Blockchain technology: implications for operations and supply chain management. Supply Chain Manage.: Int. J. 24, 469–483. doi: 10.1108/SCM-09-2018-0309.
Creemers, R. (2017). Cyber China: upgrading propaganda, public opinion work and social management for the twenty-first century. J. Contemp. China 26, 85–100. doi: 10.1080/10670564.2016.1206281
Crespo Marquez, A., Gomez Fernandez, J. F., Martínez-Galán Fernández, P., and Guillen Lopez, A. (2020). Maintenance management through intelligent asset management platforms (IAMP). Emerging factors, key impact areas and data models. Energies 13:3762. doi: 10.3390/en13153762
Cuevas-Vargas, H., Aguirre, J., and Parga-Montoya, N. (2022). Impact of ICT adoption on absorptive capacity and open innovation for greater firm performance. The mediating role of ACAP. J. Bus. Res. 140, 11–24. doi: 10.1016/j.jbusres.2021.11.058
Damanpour, F. (1992). Organizational size and innovation. Organ. Stud. 13, 375–402. doi: 10.1177/017084069201300304
Day, G. S. (2014). An outside-in approach to resource-based theories. J. Acad. Mark. Sci. 42, 27–28. doi: 10.1007/s11747-013-0348-3
Dinesh, K. K., and Sushil,. (2019). Strategic innovation factors in startups: results of a cross-case analysis of Indian startups. J. Glob. Bus. Adv. 12, 449–470. doi: 10.1504/JGBA.2019.10022956
Dong, J. Q., and Yang, C. H. (2019). Information technology and innovation outcomes: is knowledge recombination the missing link? Eur. J. Inf. Syst. 28, 612–626. doi: 10.1080/0960085X.2019.1627489
Dotzel, T., and Shankar, V. (2019). The relative effects of business-to-business (vs. business-to-consumer) service innovations on firm value and firm risk: an empirical analysis. J. Mark. 83, 133–152. doi: 10.1177/0022242919847221
Edu, A. S. (2022). Positioning big data analytics capabilities towards financial service agility. Aslib. J. Inf. Manag. 74, 569–588. doi: 10.1108/AJIM-08-2021-0240
El-Kassar, A. N., Dagher, G. K., Lythreatis, S., and Azakir, M. (2022). Antecedents and consequences of knowledge hiding: the roles of HR practices, organizational support for creativity, creativity, innovative work behavior, and task performance. J. Bus. Res. 140, 1–10. doi: 10.1016/j.jbusres.2021.11.079
Erevelles, S., Fukawa, N., and Swayne, L. (2016). Big data consumer analytics and the transformation of marketing. J. Bus. Res. 69, 897–904. doi: 10.1016/j.jbusres.2015.07.001
Ettlie, J. E., and O'Keefe, R. D. (1982). Innovative attitudes, values, and intentions in organizations [1]. J. Manag. Stud. 19, 163–182. doi: 10.1111/j.1467-6486.1982.tb00066.x
Fey, C. F., and Birkinshaw, J. (2005). External sources of knowledge, governance mode, and R&D performance. J. Manag. 31, 597–621. doi: 10.1177/0149206304272346
Fink, L., and Neumann, S. (2009). Exploring the perceived business value of the flexibility enabled by information technology infrastructure. Inf. Manag. 46, 90–99. doi: 10.1016/j.im.2008.11.007
Fornell, C., and Larcker, D. F. (1981). Evaluating structural equation models with unobservable variables and measurement error. J. Mark. Res. 18, 39–50. doi: 10.1177/002224378101800104
Foss, N. J., and Saebi, T. (2017). Fifteen years of research on business model innovation: how far have we come, and where should we go? J. Manag. 43, 200–227. doi: 10.1177/0149206316675927
Frankenberger, K., and Sauer, R. (2019). Cognitive antecedents of business models: exploring the link between attention and business model design over time. Long Range Plan. 52, 283–304. doi: 10.1016/j.lrp.2018.05.001
Fuller, C. M., Simmering, M. J., Atinc, G., Atinc, Y., and Babin, B. J. (2016). Common methods variance detection in business research. J. Bus. Res. 69, 3192–3198. doi: 10.1016/j.jbusres.2015.12.008
Gambardella, A., and McGahan, A. M. (2010). Business-model innovation: general purpose technologies and their implications for industry structure. Long Range Plan. 43, 262–271. doi: 10.1016/j.lrp.2009.07.009
Gatignon, H., and Xuereb, J. M. (1997). Strategic orientation of the firm and new product performance. J. Mark. Res. 34, 77–90. doi: 10.1177/002224379703400107
Giesen, E., Riddleberger, E., Christner, R., and Bell, R. (2010). When and how to innovate your business model. Strateg. Leadersh. 38, 17–26. doi: 10.1108/10878571011059700
Gupta, S., Drave, V. A., Dwivedi, Y. K., Baabdullah, A. M., and Ismagilova, E. (2020). Achieving superior organizational performance via big data predictive analytics: a dynamic capability view. Ind. Mark. Manag. 90, 581–592. doi: 10.1016/j.indmarman.2019.11.009
Hair, J. F., Black, W. C., Babin, B. J., and Anderson, R. E. (2010). Multivariate data analysis (7th ed.). Upper Saddle River, NJ, USA: Prentice-Hall, Inc.
Hair, J. F., Hult, G. T. M., Ringle, C. M., and Sarstedt, M. (2014). Partial least squares structural equation modeling (PLS-SEM). India: Sage Publ., 26, pp-106–121.
Hair, J. F., Ringle, C. M., and Sarstedt, M. (2011). PLS-SEM: indeed a silver bullet. J. Mark. Theory Pract. 19, 139–152. doi: 10.2753/MTP1069-6679190202
Hair, J. F. J., Sarstedt, M., Ringle, C. M., and Gudergan, S. P. (2017b). Advanced issues in partial least squares structural equation modeling. Los Angeles, CA: SAGE Publications.
Hambrick, D. C., and Mason, P. A. (1984). Upper echelons: the organization as a reflection of its top managers. Acad. Manag. Rev. 9, 193–206. doi: 10.2307/258434
Haug, A., Adsbøll Wickstrøm, K., Stentoft, J., and Philipsen, K. (2020). The impact of information technology on product innovation in SMEs: the role of technological orientation. J. Small Bus. Manag. 1–27. doi: 10.1080/00472778.2020.1793550
Hempell, T., and Zwick, T. (2008). New technology, work organisation, and innovation. Econ. Innov. New Technol. 17, 331–354. doi: 10.1080/10438590701279649
Henseler, J., Hubona, G., and Ray, P. A. (2016). Using PLS path modeling in new technology research: updated guidelines. Ind. Manag. Data Syst. 116, 2–20. doi: 10.1108/IMDS-09-2015-0382
Hitt, M. A., Carnes, C. M., and Xu, K. (2016a). A current view of resource based theory in operations management: a response to Bromiley and Rau. J. Oper. Manag. 41, 107–109. doi: 10.1016/j.jom.2015.11.004
Hock-Doepgen, M., Clauss, T., Kraus, S., and Cheng, C. F. (2021). Knowledge management capabilities and organizational risk-taking for business model innovation in SMEs. J. Bus. Res. 130, 683–697. doi: 10.1016/j.jbusres.2019.12.001
Hofacker, C. F., Malthouse, E. C., and Sultan, F. (2016). Big data and consumer behavior: imminent opportunities. J. Consum. Mark. 33, 89–97. doi: 10.1108/JCM-04-2015-1399
Hoffman, S. (2018). Managing the state: social credit, surveillance and the CCP’s plan for China. AI, China, Russia, and the Global Order: Technological, Political, Global, and Creative, 42.
Hunter, G. K., and Perreault, W. D. Jr. (2006). Sales technology orientation, information effectiveness, and sales performance. J. Pers. Sell. Sales Manag. 26, 95–113. doi: 10.2753/PSS0885-3134260201
Hurley, R. F., and Hult, G. T. M. (1998). Innovation, market orientation, and organizational learning: an integration and empirical examination. J. Mark. 62, 42–54. doi: 10.1177/002224299806200303
Janadari, M. P. N., Sri Ramalu, S., and Wei, C. (2016). Evaluation of measurment and structural model of the reflective model constructs in PLS–SEM.
Janssen, O. (2000). Job demands, perceptions of effort-reward fairness and innovative work behaviour. J. Occup. Organ. Psychol. 73, 287–302. doi: 10.1348/096317900167038
Jantunen, A., Nummela, N., Puumalainen, K., and Saarenketo, S. (2008). Strategic orientations of born globals—do they really matter? J. World Bus. 43, 158–170. doi: 10.1016/j.jwb.2007.11.015
Jiang, J., Wang, S., and Zhao, S. (2012). Does HRM facilitate employee creativity and organizational innovation? A study of Chinese firms. Int. J. Hum. Resour. Manag. 23, 4025–4047. doi: 10.1080/09585192.2012.690567
Joas, H., and Beckert, J. (2002). A theory of action: pragmatism and the creativity of action. Trans. Viewpoints 1, 1–4.
Jones, M. V., and Coviello, N. E. (2005). Internationalisation: conceptualising an entrepreneurial process of behaviour in time. J. Int. Bus. Stud. 36, 284–303. doi: 10.1057/palgrave.jibs.8400138
Joshi, K. D., Chi, L., Datta, A., and Han, S. (2010). Changing the competitive landscape: continuous innovation through IT-enabled knowledge capabilities. Inf. Syst. Res. 21, 472–495. doi: 10.1287/isre.1100.0298
Khan, A., and Tao, M. (2022). Knowledge absorption capacity's efficacy to enhance innovation performance through big data analytics and digital platform capability. J. Innov. Knowl. 7:100201. doi: 10.1016/j.jik.2022.100201
Kiron, D., Prentice, P. K., and Ferguson, R. B. (2012). Innovating with analytics. MIT Sloan Manag. Rev. 54:47.
Kiron, D., Prentice, P. K., and Ferguson, R. B. (2014). Raising the bar with analytics. MIT Sloan Manag. Rev. 55:29.
Kleis, L., Chwelos, P., Ramirez, R. V., and Cockburn, I. (2012). Information technology and intangible output: the impact of IT investment on innovation productivity. Inf. Syst. Res. 23, 42–59. doi: 10.1287/isre.1100.0338
Leung, A. K. Y., and Chiu, C. Y. (2010). Multicultural experience, idea receptiveness, and creativity. J. Cross-Cult. Psychol. 41, 723–741. doi: 10.1177/0022022110361707
Li, J. J. (2005). The formation of managerial networks of foreign firms in China: the effects of strategic orientations. Asia Pac. J. Manag. 22, 423–443. doi: 10.1007/s10490-005-4118-8
Li, G., Xue, J., Li, N., and Ivanov, D. (2022). Blockchain-supported business model design, supply chain resilience, and firm performance. Transp. Res. E: Logist. Transp. Rev. 163:102773. doi: 10.1016/j.tre.2022.102773
Liang, F., Das, V., Kostyuk, N., and Hussain, M. M. (2018). Constructing a data-driven society: China's social credit system as a state surveillance infrastructure. Policy Internet 10, 415–453. doi: 10.1002/poi3.183
Liao, J., Kickul, J. R., and Ma, H. (2009). Organizational dynamic capability and innovation: an empirical examination of internet firms. J. Small Bus. Manag. 47, 263–286. doi: 10.1111/j.1540-627X.2009.00271.x
Limbu, Y. B., Jayachandran, C., and Babin, B. J. (2014). Does information and communication technology improve job satisfaction? The moderating role of sales technology orientation. Ind. Mark. Manag. 43, 1236–1245. doi: 10.1016/j.indmarman.2014.06.013
Maddux, W. W., Adam, H., and Galinsky, A. D. (2010). When in Rome. Learn why the romans do what they do: how multicultural learning experiences facilitate creativity. Personal. Soc. Psychol. Bull. 36, 731–741. doi: 10.1177/0146167210367786
Mandal, S. (2018). Exploring the influence of big data analytics management capabilities on sustainable tourism supply chain performance: the moderating role of technology orientation. J. Travel Tour. Mark. 35, 1104–1118. doi: 10.1080/10548408.2018.1476302
Martensen, A., and Dahlgaard, J. J. (1999). Strategy and planning for innovation management–supported by creative and learning organisations. Int. J. Qual. Reliab. Manage. 16, 878–891. doi: 10.1108/02656719910289177
Martins, L. L., Rindova, V. P., and Greenbaum, B. E. (2015). Unlocking the hidden value of concepts: a cognitive approach to business model innovation. Strateg. Entrep. J. 9, 99–117. doi: 10.1002/sej.1191
McAfee, A., Brynjolfsson, E., Davenport, T. H., Patil, D. J., and Barton, D. (2012). Big data: the management revolution. Harv. Bus. Rev. 90, 60–6, 68, 128.
Mikalef, P., Boura, M., Lekakos, G., and Krogstie, J. (2019). Big data analytics capabilities and innovation: the mediating role of dynamic capabilities and moderating effect of the environment. Br. J. Manag. 30, 272–298. doi: 10.1111/1467-8551.12343
Mikalef, P., Framnes, V. A., Danielsen, F., Krogstie, J., and Olsen, D. (2017). Big data analytics capability: Antecedents and business value.
Mikalef, P., Pappas, I. O., Krogstie, J., and Giannakos, M. (2018). Big data analytics capabilities: a systematic literature review and research agenda. IseB 16, 547–578. doi: 10.1007/s10257-017-0362-y
Mithas, S., Almirall, D., and Krishnan, M. S. (2006). Do CRM systems cause one-to-one marketing effectiveness? Stat. Sci. 21, 223–233. doi: 10.1214/088342306000000213
Mithas, S., Tafti, A., Bardhan, I., and Goh, J. M. (2012). Information technology and firm profitability: mechanisms and empirical evidence. MIS Q. 36:205. doi: 10.2307/41410414
Morikawa, M. (2004). Information technology and the performance of Japanese SMEs. Small Bus. Econ. 23, 171–177. doi: 10.1023/B:SBEJ.0000032023.11566.4b
Mostaghel, R., Oghazi, P., Parida, V., and Sohrabpour, V. (2022). Digitalization driven retail business model innovation: evaluation of past and avenues for future research trends. J. Bus. Res. 146, 134–145. doi: 10.1016/j.jbusres.2022.03.072
Mubarak, M. F., and Petraite, M. (2020). Industry 4.0 technologies, digital trust and technological orientation: what matters in open innovation? Technol. Forecast. Soc. Chang. 161:120332. doi: 10.1016/j.techfore.2020.120332
Munir, S., Rasid, S. Z. A., Aamir, M., Jamil, F., and Ahmed, I. (2022). Big data analytics capabilities and innovation effect of dynamic capabilities, organizational culture and role of management accountants. Foresight.
Nambisan, S., Lyytinen, K., Majchrzak, A., and Song, M. (2017). Digital innovation management: reinventing innovation management research in a digital world. MIS Q. 41, 223–238. doi: 10.25300/MISQ/2017/41:1.03
Naqshbandi, M. M. (2018). Organizational characteristics and engagement in open innovation: is there a link? Glob. Bus. Rev. 19, S1–S20. doi: 10.1177/0972150918757845
Neneh, B. N. (2019a). From entrepreneurial alertness to entrepreneurial behavior: the role of trait competitiveness and proactive personality. Pers. Individ. Differ. 138, 273–279. doi: 10.1016/j.paid.2018.10.020
Nunally, J. C., and Bernstein, I. J. N. M.-H. (1978). Psychometric theory. New York, NY: McGraw Hill Companies.
Oldham, G. R., and Cummings, A. (1996). Employee creativity: personal and contextual factors at work. Acad. Manag. J. 39, 607–634.
Podsakoff, N. P. (2003). Common method biases in behavioral research: a critical review of the literature and recommended remedies. J. Appl. Psychol. 88, 879–903. doi: 10.1037/0021-9010.88.5.879
Porter, M. E., and Heppelmann, J. E. (2015). How smart, connected products are transforming companies. Harv. Bus. Rev. 93, 96–114.
Purwanto, A., Asbari, M., Santoso, T. I., Sunarsi, D., and Ilham, D. (2021). Education research quantitative analysis for little respondents: comparing of Lisrel, tetrad, GSCA, Amos, SmartPLS, WarpPLS, and SPSS. JSGP. 4. 335–350.
Rai, A., Patnayakuni, R., and Seth, N. (2006). Firm performance impacts of digitally enabled supply chain integration capabilities. MIS Q. 30:225. doi: 10.2307/25148729
Ransbotham, S., and Kiron, D. (2017). Analytics as a source of business innovation. MIT Sloan Manag. Rev. 58.
Ravichandran, T., Han, S., and Mithas, S. (2017). Mitigating diminishing returns to R&D: the role of information technology in innovation. Inf. Syst. Res. 28, 812–827. doi: 10.1287/isre.2017.0717
Ravichandran, T., Lertwongsatien, C., and Lertwongsatien, C. (2005). Effect of information systems resources and capabilities on firm performance: a resource-based perspective. J. Manag. Inf. Syst. 21, 237–276. doi: 10.1080/07421222.2005.11045820
Reinartz, W., Haenlein, M., and Henseler, J. (2009). An empirical comparison of the efficacy of covariance-based and variance-based SEM. Int. J. Res. Mark. 26, 332–344. doi: 10.1016/j.ijresmar.2009.08.001
Riahi-Belkaoui, A. (1999). The degree of internationalization and the value of the firm: theory and evidence. J. Int. Account. Audit. Tax. 8, 189–196. doi: 10.1016/S1061-9518(99)00009-9
Rialti, R., Zollo, L., Ferraris, A., and Alon, I. (2019). Big data analytics capabilities and performance: evidence from a moderated multi-mediation model. Technol. Forecast. Soc. Chang. 149:119781. doi: 10.1016/j.techfore.2019.119781
Ritter, T., and Pedersen, C. L. (2020). Analyzing the impact of the coronavirus crisis on business models. Ind. Mark. Manag. 88, 214–224. doi: 10.1016/j.indmarman.2020.05.014
Ryu, H. S., and Lee, J. N. (2018). Understanding the role of technology in service innovation: comparison of three theoretical perspectives. Inf. Manag. 55, 294–307. doi: 10.1016/j.im.2017.08.003
Saebi, T., and Foss, N. J. (2015). Business models for open innovation: matching heterogeneous open innovation strategies with business model dimensions. Eur. Manag. J. 33, 201–213. doi: 10.1016/j.emj.2014.11.002
Saether, E. A. (2019). Motivational antecedents to high-tech R&D employees’ innovative work behavior: self-determined motivation, person-organization fit, organization support of creativity, and pay justice. J. High Technol. Manage. Res. 30:100350. doi: 10.1016/j.hitech.2019.100350
Saldanha, T. J., Mithas, S., and Krishnan, M. S. (2017). Leveraging customer involvement for fueling innovation: the role of relational and analytical information processing capabilities. MIS Q. 41, 367–396. doi: 10.25300/MISQ/2017/41.1.14
Santoro, G., Fiano, F., Bertoldi, B., and Ciampi, F. (2018). Big data for business management in the retail industry. Manag. Decis. 57, 1980–1992. doi: 10.1108/MD-07-2018-0829
Sarstedt, M., and Mooi, E. (2019). “Regression analysis,” in A concise guide to market research (Berlin, Heidelberg: Springer), 209–256.
Sarstedt, M., Hair, J. F., Cheah, J. H., Becker, J. M., and Ringle, C. M. (2019). How to specify, estimate, and validate higher-order constructs in PLS-SEM. Australas. Mark. J. 27, 197–211. doi: 10.1016/j.ausmj.2019.05.003
SAS (2013). Big data analytics. An assessment of demand for labour and skills, London, England. 2012–2017.
Scott, S. G., and Bruce, R. A. (1994). Determinants of innovative behavior: a path model of individual innovation in the workplace. Acad. Manag. J. 37, 580–607. doi: 10.5465/256701
Shafique, I., Ahmad, B., and Kalyar, M. N. (2019). How ethical leadership influences creativity and organizational innovation: examining the underlying mechanisms. Eur. J. Innov. Manag. 23, 114–133. doi: 10.1108/EJIM-12-2018-0269
Shahbaz, M., Gao, C., Zhai, L., Shahzad, F., and Hu, Y. (2019). Investigating the adoption of big data analytics in healthcare: the moderating role of resistance to change. J. Big Data 6, 1–20. doi: 10.1186/s40537-019-0170-y
Shamim, S., Zeng, J., Choksy, U. S., and Shariq, S. M. (2020). Connecting big data management capabilities with employee ambidexterity in Chinese multinational enterprises through the mediation of big data value creation at the employee level. Int. Bus. Rev. 29:101604. doi: 10.1016/j.ibusrev.2019.101604
Shanker, R., Bhanugopan, R., Van der Heijden, B. I., and Farrell, M. (2017). Organizational climate for innovation and organizational performance: the mediating effect of innovative work behavior. J. Vocat. Behav. 100, 67–77. doi: 10.1016/j.jvb.2017.02.004
Sharma, R., Mithas, S., and Kankanhalli, A. (2014). Transforming decision-making processes: a research agenda for understanding the impact of business analytics on organisations. Eur. J. Inf. Syst. 23, 433–441. doi: 10.1057/ejis.2014.17
Sosik, J. J., Kahai, S. S., and Piovoso, M. J. (2009). Silver bullet or voodoo statistics? A primer for using the partial least squares data analytic technique in group and organization research. Group Org. Manag. 34, 5–36. doi: 10.1177/1059601108329198
Spence, M., and Crick, D. (2009). An exploratory study of Canadian international new venture firms' development in overseas markets. Qual. Mark. Res. Int. J. 12, 208–233. doi: 10.1108/13522750910948798
Spieth, P., Roeth, T., and Meissner, S. (2019). Reinventing a business model in industrial networks: implications for customers' brand perceptions. Ind. Mark. Manag. 83, 275–287. doi: 10.1016/j.indmarman.2019.04.013
Statista (2020). Number of companies listed on the Chinese stock exchanges from 2008 to 2020. statista.com. Available at: https://www.statista.com/statistics/225725/number-of-companies-listed-on-the-chinese-stock-exchange/ (Accessed 20 March 2022).
Story, V., O'Malley, L., and Hart, S. (2011). Roles, role performance, and radical innovation competences. Ind. Mark. Manag. 40, 952–966. doi: 10.1016/j.indmarman.2011.06.025
Su, X., Zeng, W., Zheng, M., Jiang, X., Lin, W., and Xu, A. (2021). Big data analytics capabilities and organizational performance: the mediating effect of dual innovations. Eur. J. Innov. Manag. 25, 1142–1160. doi: 10.1108/EJIM-10-2020-0431
Sundaram, S., Schwarz, A., Jones, E., and Chin, W. W. (2007). Technology use on the front line: how information technology enhances individual performance. J. Acad. Mark. Sci. 35, 101–112. doi: 10.1007/s11747-006-0010-4
Tarafdar, M., and Qrunfleh, S. (2017). Agile supply chain strategy and supply chain performance: complementary roles of supply chain practices and information systems capability for agility. Int. J. Prod. Res. 55, 925–938. doi: 10.1080/00207543.2016.1203079
Teece, D. J. (1986). Profiting from technological innovation: implications for integration, collaboration, licensing and public policy. Res. Policy 15, 285–305. doi: 10.1016/0048-7333(86)90027-2
Teece, D. J. (2007). Explicating dynamic capabilities: the nature and microfoundations of (sustainable) enterprise performance. Strateg. Manag. J. 28, 1319–1350. doi: 10.1002/smj.640
Teece, D. J., Pisano, G., and Shuen, A. (1997). Dynamic capabilities and strategic management. Strateg. Manag. J. 18, 509–533. doi: 10.1002/(SICI)1097-0266(199708)18:7<509::AID-SMJ882>3.0.CO;2-Z
Teece, D., and Leih, S. (2016). Uncertainty, innovation, and dynamic capabilities: an introduction. Calif. Manag. Rev. 58, 5–12. doi: 10.1525/cmr.2016.58.4.5
Teece, D., Peteraf, M., and Leih, S. (2016). Dynamic capabilities and organizational agility: risk, uncertainty, and strategy in the innovation economy. Calif. Manag. Rev. 58, 13–35. doi: 10.1525/cmr.2016.58.4.13
Tellis, G. J., Prabhu, J. C., and Chandy, R. K. (2009). Radical innovation across nations: the preeminence of corporate culture. J. Mark. 73, 3–23. doi: 10.1509/jmkg.73.1.003
Tierney, P., Farmer, S. M., and Graen, G. B. (1999). An examination of leadership and employee creativity: the relevance of traits and relationships. Pers. Psychol. 52, 591–620. doi: 10.1111/j.1744-6570.1999.tb00173.x
Trantopoulos, K., von Krogh, G., Wallin, M. W., and Woerter, M. (2017). External knowledge and information technology: implications for process innovation performance. MIS Q. 41, 287–300. doi: 10.25300/MISQ/2017/41.1.15
Tsou, H. T., Chen, J. S., and Liao, W. H. (2014). Market and technology orientations for service delivery innovation: the link of innovative competence. J. Bus. Ind. Mark. 29, 499–513. doi: 10.1108/JBIM-09-2011-0128
Turulja, L., and Bajgorić, N. (2016). Innovation and information technology capability as antecedents of firms’ success. INDECS 14, 148–156. doi: 10.7906/indecs.14.2.4
Tushman, M. L., and Anderson, P. (1986). Technological discontinuities and organizational environments. Adm. Sci. Q. 31:439. doi: 10.2307/2392832
Van de Ven, A. H. (1986). Central problems in the management of innovation. Manag. Sci. 32, 590–607. doi: 10.1287/mnsc.32.5.590
Wamba, S. F., Gunasekaran, A., Akter, S., Ren, S. J. F., Dubey, R., and Childe, S. J. (2017). Big data analytics and firm performance: effects of dynamic capabilities. J. Bus. Res. 70, 356–365. doi: 10.1016/j.jbusres.2016.08.009
Wang, C., Zhang, Q., and Zhang, W. (2020). Corporate social responsibility, green supply chain management and firm performance: the moderating role of big-data analytics capability. Res. Transp. Bus. Manag. 37:100557. doi: 10.1016/j.rtbm.2020.100557
Wang, Y., and Hajli, N. (2017). Exploring the path to big data analytics success in healthcare. J. Bus. Res. 70, 287–299. doi: 10.1016/j.jbusres.2016.08.002
Wang, Y., Xiuping, S., and Zhang, Q. (2021). Can fintech improve the efficiency of commercial banks?—an analysis based on big data. Res. Int. Bus. Financ. 55:101338. doi: 10.1016/j.ribaf.2020.101338
Wang, Z., Li, M., Lu, J., and Cheng, X. (2022). Business innovation based on artificial intelligence and Blockchain technology. Inf. Process. Manag. 59:102759. doi: 10.1016/j.ipm.2021.102759
Wei, L. Q., and Lau, C. M. (2010). High performance work systems and performance: the role of adaptive capability. Hum. Relat. 63, 1487–1511. doi: 10.1177/0018726709359720
Wirtz, B. W., Schilke, O., and Ullrich, S. (2010). Strategic development of business models: implications of the web 2.0 for creating value on the internet. Long Range Plan. 43, 272–290. doi: 10.1016/j.lrp.2010.01.005
Wu, J., Wang, J., Nicholas, S., Maitland, E., and Fan, Q. (2020). Application of big data technology for COVID-19 prevention and control in China: lessons and recommendations. J. Med. Internet Res. 22:e21980. doi: 10.2196/21980
Yuan, R., and Wen, W. (2018). Managerial foreign experience and corporate innovation. J. Corp. Finan. 48, 752–770. doi: 10.1016/j.jcorpfin.2017.12.015
Zhang, X., and Bartol, K. M. (2010). Linking empowering leadership and employee creativity: the influence of psychological empowerment, intrinsic motivation, and creative process engagement. Acad. Manag. J. 53, 107–128. doi: 10.5465/amj.2010.48037118
Zheng, S., Zhang, W., and Du, J. (2011). Knowledge-based dynamic capabilities and innovation in networked environments. J. Knowl. Manag. 15, 1035–1051. doi: 10.1108/13673271111179352
Zheng, L. J., Zhang, J. Z., Wang, H., and Hong, J. F. (2022). Exploring the impact of big data analytics capabilities on the dual nature of innovative activities in MSMEs: a data-agility-innovation perspective. Ann. Oper. Res. 1–29. doi: 10.1007/s10479-022-04800-6
Keywords: business model innovation, technological orientation, employee creativity, big data analytical capabilities building and education, China
Citation: Cui Y, Firdousi SF, Afzal A, Awais M and Akram Z (2022) The influence of big data analytic capabilities building and education on business model innovation. Front. Psychol. 13:999944. doi: 10.3389/fpsyg.2022.999944
Received: 21 July 2022; Accepted: 09 September 2022;
Published: 18 October 2022.
Edited by:
Umair Akram, RMIT University, VietnamReviewed by:
Virginia Barba-Sánchez, Universidad de Castilla-La Mancha, SpainCopyright © 2022 Cui, Firdousi, Afzal, Awais and Akram. This is an open-access article distributed under the terms of the Creative Commons Attribution License (CC BY). The use, distribution or reproduction in other forums is permitted, provided the original author(s) and the copyright owner(s) are credited and that the original publication in this journal is cited, in accordance with accepted academic practice. No use, distribution or reproduction is permitted which does not comply with these terms.
*Correspondence: Saba Fazal Firdousi, c2FiYWZhemFsZmlyZG91c2lAZ21haWwuY29t
Disclaimer: All claims expressed in this article are solely those of the authors and do not necessarily represent those of their affiliated organizations, or those of the publisher, the editors and the reviewers. Any product that may be evaluated in this article or claim that may be made by its manufacturer is not guaranteed or endorsed by the publisher.
Research integrity at Frontiers
Learn more about the work of our research integrity team to safeguard the quality of each article we publish.