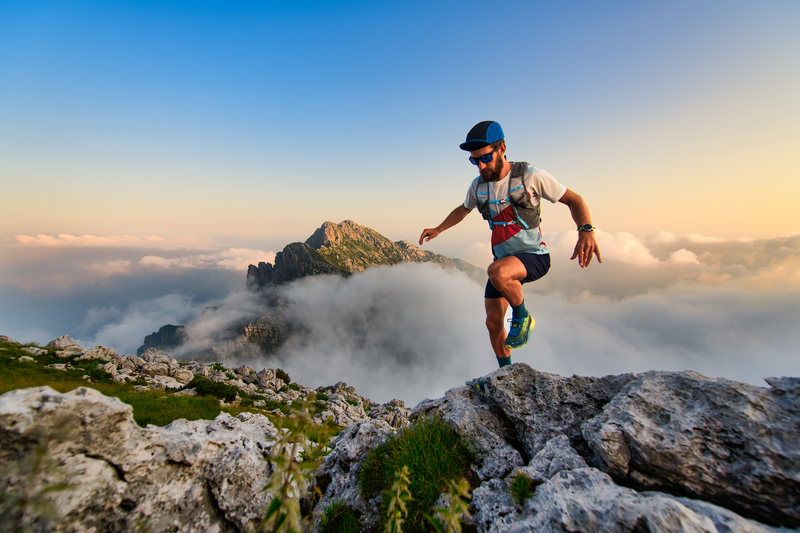
95% of researchers rate our articles as excellent or good
Learn more about the work of our research integrity team to safeguard the quality of each article we publish.
Find out more
ORIGINAL RESEARCH article
Front. Psychol. , 16 November 2022
Sec. Organizational Psychology
Volume 13 - 2022 | https://doi.org/10.3389/fpsyg.2022.994910
This article is part of the Research Topic Innovative Behavior in Entrepreneurship: Analyzing New Perspectives and Challenges View all 16 articles
The aim of this paper is to conduct a revenue management study, generating a theoretical model that establishes the relationship between the factors of a Short-Term Rental apartment offered on the Airbnb marketplace or similar and its optimal rental price set when the property is first put on the market, considering not only the characteristics defined in the platform listing but also the sociodemographic characteristics of the area in which the apartment is located. The research process was structured in six phases as case study for the technology transfer model. First, research planning was conducted to estimate the time, cost, and suitability of the research topic. Second, the study design was determined to establish a technology transfer model focusing on the theory of mixed revenue management. Third, data collection about the city of Madrid was extracted from two technological databases, namely SeeTransparent based mainly on Airbnb (28 internal characteristics of the apartment) and Deskmind Research (9 sociodemographic variables of the area in which the apartment is located). Fourth, the data were prepared to create a new descriptive variable of the apartments based on geolocation. Fifth, the analysis of this study was applied to explore the correlation between the price charged per night, the 28 internal characteristics of the apartments, and the 9 sociodemographic variables of their surrounding areas. Sixth, with this integrated database, the information was transformed into multivariate inferential statistics through Exploratory Factor Analysis and Multiple Linear Regression, creating a technology transfer model (big data algorithm) that allows revenue managers to set the price of an apartment based on known information, prior to having a history of market reactions. This research process and model consider some of the factors affecting the psychological behavior of tourism consumers. Practical implications of the findings indicate that the size/capacity of the apartments used for Short-Term rentals largely determines the initial rental price set (72%). The equipment offered by the apartments has a moderate impact (18%), and the sociodemographic characteristics of the surrounding area have a minor influence (11%).
The presence of marketplaces such as Airbnb, allowing so-called sharing economies to offer peer-to-peer rentals, has raised this market to a different level in terms of volume and number of listings.
Such marketplaces undoubtedly cover a large portion of the demand for overnight stays when traveling. Since the creation of Airbnb in 2008, the number of apartments available to rent for short stays has increased, and indeed this platform has become one of the biggest markets, with a strong presence today. In addition, many other companies have begun to offer this product, connecting landlords with short-term tenants, such as VRBO, Wimdu, Homeaway, and other marketplaces, as well as experts in traditional hotel accommodation such as Booking and Expedia. Even estate agencies and hotel companies such as RoomMate, SpainSelect, GavirRentals, and MyCityHome.es joined the market, as the sector became more professionalized, and progressively more short-term rental accommodation became available to the market.
The development of Short-Term Rental in the current economy as a model of revenue management for these properties, offering fully furnished real estate assets or apartments ready for immediate occupation, offers a significant area of study, in various fields. In this case, the research presented here also considers the psychological behavior of tourism consumers. For example, some research notes that Short-Term Rentals are putting a financial squeeze on housing for regular tenants and further increasing rent and property prices (Horn and Merante, 2017; Barron et al., 2018; Chen et al., 2019; Ayouba et al., 2020; Garcia-López et al., 2020; Koster et al., 2021a; Tood et al., 2021).
However, according to Levin et al. (2002), Sundararajan (2014), and Einav et al. (2016) Short-Term Rental (STR) home-sharing platforms, such as Airbnb, HomeAway, VRBO, and CouchSurfing, provide digital peer-to-peer (P2P) marketplace for landlords to rent out empty lodging spaces to third parties, thereby increasing availability and consequently adjusting the price as per the offer-demand rule, alleviating the suggested financial squeeze on housing by having a larger offer but reacting more dynamically to macroeconomic factors. Moreover, according to Brotman (2021), based on the results of their regressions, the motivation of property owners is also increasing, as they even consider building small annexes behind their primary residences to rent out to visitors as Short-Term Rentals.
Other authors state that, although home-sharing platforms provide major efficiencies and create economic benefits in certain aspects (Gárate Alvarez and Pennington-Cross, 2021), they also disrupt the existing property and hospitality industries and create disturbances in the neighborhood in which the lodgings are provided (Espinosa, 2016; Kim et al., 2017). However, such experiences forced the market to develop in terms of requiring more detailed contracts and regulations, imposing penalties for certain behaviors, and charging security deposits that also affect tenants’ willingness to pay.
Undoubtedly, one important concern of landlords who offer their properties through these marketplaces is how to maximize their revenues. Hence, one of the most important issues in property management is ensuring the asset is correctly priced to meet demand at the best price while providing an experience at the destination (Ali et al., 2022) as well as maximizing the owner’s income. To achieve optimal pricing and profitability for the landlord, it is important to determine which factors will affect this valuation, both directly and indirectly.
Many publications dealing with Revenue Management focus on optimizing pricing, based on experience and adjustments according to the market. Other studies compare Short-Term Rentals with other types of rentals. However, it would appear that no publications have yet tackled the issue of how professionals in this sector decide the starting price of a short term property rental. Interviews conducted with several professionals suggest that landlords largely tend to decide this starting price based on intuition. Interestingly, there does not appear to be empirical data available indicating the factors on which these professionals are basing their decision. Landlords do seem to adjust the price as they begin to gather historical information on the market’s reaction; hence the method used during the initial period is largely intuitive and manual, with a significant time investment required.
This paper aims to provide a practical tool for professionals in the real estate sector, on how to decide the most suitable starting price for a residential real estate asset, and which factors affect this decision, reducing the time investment required when adding a new listing to the market. It contributes to the literature by using a technology transfer model to transform the big data gathered, enabling the effects of different internal or external factors on prices to be measured and modeled.
What are the main applications and uses of understanding the factors affecting the starting price of Short-Term Rentals considering a revenue management model?
By focusing on this analytical perspective, the research presented here aims to answer the following objectives:
• Identify the main applications of factors such as sociodemographic characteristics and property features that are quantitative indicators of Short—Term Rental apartments vs. Pricing, using a technology transfer model.
• Provide future guidelines to develop this technological model proposed here, to include qualitative indicators and correction factors on the grounds of seasonality and market trends.
To test the problems stated before, this research develops a systematic review of the literature to identify the main contributions made to date within this subject area. The results are analyzed by applying Yin’s (2018) case study as a new technology transfer model with data collection from two technological databases and data analysis with multivariate inferential statistics through Exploratory Factor Analysis and Multiple Linear Regression, creating the technology transfer model (big data algorithm). A discussion and future lines of research in this area are then presented.
Following this introduction, section “Literature review” presents the theoretical framework. Section “Methodology development” sets out the methodology used in the study, and section “Analyze of results (Multivariate analysis)” describes the results, enabling revenue managers to set the price of an apartment on the basis of known information, prior to having a history of market reactions. Section “Discussion” presents the discussion, comparing it with similar studies, and section “Conclusion” states the final conclusions, implications, and limitations.
Two of the key concepts in revenue management are Littlewood’s rule (Littlewood, 1972) and expected marginal seat revenue (Belobaba, 1987). This notion was mainly created for airlines and considered seat availability, competition, as well as the expiration date of the plane ticket. In fact, American Airlines developed the world’s first revenue management system in 1985 (Lentz et al., 2021), considering revenue management to be an essential instrument in terms of matching supply and demand by segmenting customers, based on their purchase intentions and assigning them in a way that would maximize the firm’s revenues (El Haddad et al., 2008; Ivanov and Zhechev, 2012). Revenue management has been an area of interest in academia for many years (Tse and Poon, 2012), with research on topics such as pricing (Shoemaker, 2003, 2005), price fairness (Kimes and Rohlfs, 2007; Kimes and Wirtz, 2007; Kimes and Taylor, 2010), pricing research (Shy, 2008), and decision framing (Tversky and Kahneman, 1981). It has also benefited strongly not only from marketing management research but more profoundly from operations (Talluri and van Ryzin, 2005), as well as the impact on consumers (Choi and Mattila, 2004; Heo and Lee, 2010).
Revenue Management is widely understood to mean the management of pricing and external factors to maximize the profitability of an asset. Matsuoka (2022) states that Revenue Management aims to maximize financial performance by setting different prices for the same offerings. Cha et al. (2017) understood pricing to be a complex task that requires in-depth understanding. It entails, among other things, monitoring competition day by day, optimizing revenue and setting pricing strategies as per Cullen (2015) and Demirciftci and Belarmino (2022), integrating new technologies, such as artificial intelligence and robots as per Buhalis et al. (2019), using big data, as per Choi et al. (2018), and interpreting the right data in real time (Buhalis and Sinarta, 2019). Revenue management and pricing became particularly important as a tool and strategy in Yeoman’s (2022) consideration of the hotel industry.
Organization support is crucial to maintain a collaborative workplace climate (Li and Srinivasan, 2019) and requires fairly advanced skills (Wang and Brennan, 2014). In this sense, training for managers and appropriate IT infrastructure for streamlined revenue management are essential elements (Selmi and Dornier, 2011). Innovation has emerged as the main driver of change in a business sector that needs to be flexible and resilient (Saura et al., 2022).
Revenue management is increasingly based on marketing, with tailored practices such as personalized pricing or personalized rooms (Tu et al., 2018). The integration between operations and marketing—as well as between strategy and tactics—is key for successful revenue management. Hotels adopt key performance indicators that go beyond room revenues, such as REVPar (Revenue per available room), which accounts for all outlets in the property, or GopPar (gross operating profit per available room), which accounts for expenses and cross selling (Ferguson and Smith, 2014). Ivanov and Zhechev (2012) provide a detailed overview of the specific revenue centers, including room division, F&B, function rooms, and spa and fitness facilities.
There is a vast body of literature on the subject of revenue management, an increasingly trending topic applied to many more markets. Vast numbers of articles such as those mentioned above allow pricing to be adapted based on events, in other words, competition strategy, expiration date, spikes in demand due to football matches, and a large number of different factors to be applied and considered continuously. However, this adaptation comes from a starting point, a defined unique price per unit, and yet it would appear that existing research has yet to tackle initial price-setting based on empirical evidence.
When asking revenue managers, in companies such as MyCityHome.es, how they set these starting prices, the most common answer is by experience, benchmarking, and personal impression, stating that they do not have a model to perform this calculation, and results are a little too arbitrary for such an important aspect in property revenue management.
Big data refers to the collection of a large amount of data that could be used in the future through the application of technology to learn about behaviors, yield statistics, and establish a decision-making model. The use of mobile applications and other technologies for tourism has seen important changes in the twenty-first century (Saura et al., 2017, 2021).
Elshawi et al. (2018) state that, recently, there have been huge advancements in the scale of data routinely generated and collected through almost all human activity, as well as the ability to exploit modern technologies to process, analyze, and understand such data. The intersection of these trends is known as Big Data Science. According to Ali et al. (2016), “In the modern world we are inundated with data” and “It is estimated that we are generating 2.5 quintillion bytes per day.”
Information is becoming more and more accessible, and so the challenge for big data techniques is to transform this information into useful tools. In this particular case, the aim is to be able to model the data using SPSS and transfer all the knowledge acquired from these data using a simple algorithm or formula, bringing into play the concept of technology transfer.
Also known as transfer of technology (ToT), this is the process of transferring technology from one tech-owning entity to another. Soeder et al. (1990) define it as follows: “Technology transfer is the managed process of conveying a technology from one party to its adoption by another party.”
Technology transfer does not have a universal meaning, according to Kremic (2003), and, in the words of Kutter (1991), it takes place “simply by moving a computer from a laboratory in Boston to a university in Manila” or relocating or exchanging personnel, as per NASA Aeronautics (1993). Others consider international technology transfer complete only when the host economy has absorbed, adapted, and resold the technology (Osman-Gani, 1999).
In order to achieve the goal of the technology transfer study, it is also considered as a process with a Setpoint-Comparator-Feedback loop and post-processing output as per Kremic’s (2003) schema. The set of steps proposed in this paper to establish the Short-Term Rental starting price could be used as a technology transfer model or algorithm.
There are three widely accepted definitions for the different types of rentals among professionals in the market. Bearing in mind that there are no precise or documented definitions, they can broadly be classified as follows:
Short-Term Rentals: apartment rentals by the day. Rental of the apartment for a period that can range from one night to several weeks. This type of rental can include Vacation Rentals, Tourism Rentals, Study Rentals, Exhibitions Rentals, and Workday Rentals. In terms of regulations, different regions have, indeed, defined the maximum term and placed certain limitations on the number of days the apartment can be rented. Koster et al. (2021b) summarize some of them: STR-hosts occupy the property for at least 50% of the time (O’Sullivan, 2016). San Francisco imposes a 14% hotel tax (i.e., a Transient Occupancy Tax) and a cap of 90 rental days maximum per year (Fishman, 2015). Amsterdam even imposes a maximum cap of 30 rental days per year as of 2019. In the region of Madrid, however, duration is not yet regulated, and so solutions such as a 5-day minimum stay or a maximum of 90 days have been put forward but rejected in the current regulations. The sample used in this paper recorded an average duration of 3.3219 nights per stay.
Season-Term Rentals: apartments rented by the month. This type of rental can be confused among professionals since it is unclear or at times difficult to differentiate from Short-Term Rentals. Nevertheless, typically, the general consensus within the property sector is that it refers to properties rented for between 4 and 12 months. Some countries such as Spain have restricted this type of rental to 12 months, as longer stays would be subject to the Spanish Urban Leases Act (“Ley de Arrendamientos Urbanos”) (Jefatura del Estado, 1994).
Long-Term Rentals: apartments rented by the year. As mentioned previously, although there is no precise definition, there are, nonetheless, legal regulations that establish the duration of this rental period. Since this study was conducted in Madrid, Spain, the Urban Leases Act states that such leases will be no less than 6 months and up to 5 or 7 years, if the landlord is a company, unless otherwise agreed by both parties, for a longer lease only. Season-Term Rentals, on the other hand, will never be longer than 12 months (Jefatura del Estado, 1994).
Taking this into consideration, the research presented in this paper defines Short-Term Rentals to be from 1 day to 4 months, Season-Term Rentals from 4 months up to 12 months, and Long-Term Rentals over 12 months.
Different authors, such as Shokoohyar et al. (2020), have found that property location has a significant impact on the rental strategy chosen, underscoring the importance of that well-known refrain “location, location, location” in the property market. Authors such as Benítez-Aurioles (2018) and Gunter and Önder (2018) have focussed previously on geolocation, showing that listing prices are related to distance from the city center, and the response time of the host is negatively correlated with such bookings. Prices are influenced by sociodemographic variables in the surrounding area of the property, and Filippas and Horton (2018) noted that the demand for housing decreases when neighbors see a high turnover of people in their residential area.
As stated previously, this study considers up to 9 sociodemographic variables that may be influencing price.
• Resident population.
• Density of resident population.
• Socioeconomic Index (scale 0–10).
• % of second homes (Over total dwellings).
• No. of active businesses (shops).
• No. of Restaurants/Bars/Cafés.
• No. of Traditional hotels/residences.
• No. of Urban public transport stations.
• No. of Cultural venues (cinemas/theater…).
Adding to the previous paragraph, Shokoohyar et al. (2020) state that “properties with more bedrooms, closer to the historic attractions, in neighborhoods with lower minority rates and higher nightlife vibe are more likely to have a higher return if they are rented out through Short-Term Rental contract.” Cui et al. (2018) assume that “higher-priced homeowners and higher-priced renters are more likely to live in properties with a greater number of bedrooms, near a major employment center, park, or school, as well as in a suburban location[…] school attendance with higher school quality.”
As stated previously, this study considers up to 28 internal variables that may be influencing price. The pre-study variables established are as follows:
• Bathrooms.
• Bedrooms.
• Beds.
• Capacity.
• Available equipment (% Yes).
• Kitchen.
• Washing Machine.
• Heating / Air Conditioning.
• Child Friendly.
• Dryer.
• Pets Allowed.
• Internet.
• Pool.
• Parking.
• Gym.
• Hot Tub.
• Doorman.
• Suitable For Events.
• Wheelchair Accessible.
• Garden.
• Laptop Friendly.
• Workspace.
• Outdoor Grill.
• Patio or Balcony.
• Restaurant.
• Sauna.
• Spa and Wellness Center.
• Terrace View.
These are also the most relevant variables that major marketplaces like Airbnb and Booking request when completing their listings. This article sets out to demonstrate the relevance of these variables empirically, in this study.
In light of the above, the theoretical approach applied in this research, mixing sociodemographic and internal variables to determine Short-Term Rental prices (based on Yin’s case study), contributes analytically to the subject of revenue management, seeking to provide complementary information. Other studies have focused on price-setting for Short-Term Rental holiday apartments. The study of Shokoohyar et al. (2020, mentioned earlier) is somewhat similar to the research presented here, showing how sociodemographic and internal variables influence rental prices, but using different methodological techniques such as logistic regression and applied to learn practices to predict the rental strategy with the highest rate of return for a given property. Most publications on Revenue Management, such as those mentioned earlier, focus chiefly on adapting the price of a given asset over time, on comparing calendar events and the competition that offers a similar product. However, the extant research seems to have largely neglected the issue of the optimal starting price. This initial price sets a course that can be adjusted over time. If the level of this initial price is set incorrectly, it could lead to a substantial loss in the potential profitability of the asset, as revenue management corrections are carried out progressively on the basis of historical data.
Information was gathered initially from several regions in Spain, but during the research process, the decision was made to concentrate solely on the city of Madrid, for the time being, due to several factors.
First, Madrid is a versatile city within this field of study: it offers Short-Term Rental apartments, as well as Vacation Rentals, Tourist Rentals, Study Rentals, Exhibitions Rentals, Workday Rentals, and so on, all included in the Short-Term Rental definition mentioned earlier.
Second, Madrid was also considered as a destination for the purposes of this article because its tourism demand is largely linear, contrary to other regions in Spain such as Marbella or the Balearic Islands, where demand is more seasonal and focused on summer holidays, or Baqueira or the Sierra Nevada where the peak tourist season is in winter.
Third, very reliable and accessible information has been compiled in Madrid, pertaining not only to sociodemographic and environmental factors but also to the internal features of the properties themselves. Other studies conducted previously in Madrid have also used the Deskmind Research (2022) and SeeTransparent Airbnb (2022) databases, which provide up-to-date information on this destination.
Fourth, the personal-professional background of the authors is more concentrated in Madrid. Indeed, they have working experience at an estate agency MyCityHome.es, which mainly focuses on Short-Term rentals and whose headquarters are in Madrid, justifying its selection as a destination.
Moreover, the choice of Airbnb Fast Facts (2022) seems appropriate, as its market share is 57.5%, compared with 36.5% held by Booking.com according to MyCityHome (2022). Furthermore, Airbnb focuses more on Short-Term apartment Rentals whereas Booking.com focuses more on hotel rooms. In addition, according to Zubair and Faiqa (2022), there is a certain level of intended user continuation.
In this context, the study makes three assumptions in this research:
- Assumption 1: the real rental price is taken to be the last price displayed in the Airbnb listing before the listing status changed from “available” to “reserved.”
- Assumption 2: the measurable features of the apartments (quantitative variables, such as beds, bedrooms, and bathrooms) have been taken into account, disregarding the non-measurable features such as users’ reviews, decoration style, or others (qualitative variables).
- Assumption 3: the effects of other variables such as seasonality and competitors have not been taken into consideration, since these variables should be subject to daily correction. The price studied in this paper should be considered a base price and subject to future studies and corrections. Therefore, the data take into consideration an average over the real yearly rental prices.
In light of the above, the final goal of this project is to develop a technology transfer model that establishes a correlation between the different features of a property used for Short-Term Rental in Madrid and the ideal starting price, taking into consideration not only the internal characteristics defined in the Airbnb listing but also the sociodemographic dimensions of the area where the apartment is located.
In this regard, two hypotheses have been formulated for statistical testing, based on Shokoohyar et al. (2020):
- Hypothesis 1: Price per night is influenced by the internal features of the apartment, considering that the price of the apartment is affected by the internal features of the property.
- Hypothesis 2: Price per night is influenced by the sociodemographic characteristics of the area, considering that the price of the apartment is affected by the sociodemographic elements of the surrounding environment.
The data used in this study were obtained from the SeeTransparent database for Short-Term Rentals about Airbnb and from the Deskmind Research database for the sociodemographic variables, to focus on revenue management in apartments for Short-Term Rental as a case study in the city of Madrid. The combination of these two sources represents a form of technology transfer to disseminate knowledge about big data and revenue management issues, such as internal factors (Hypothesis 1) and sociodemographic factors (Hypothesis 2) affecting the pricing of Short-Term Rentals for tourism.
In particular, the design of this technology transfer model is based on Yin’s (2018) as one of the most popular case studies in social sciences and for its theoretical implications. This technology transfer model seems to be an appropriate methodological approach in this research in terms of establishing the relationship between the characteristics of a Short-Term Rental apartment offered through Airbnb or a similar marketplace and its optimal rental price, considering not only the characteristics defined in the platform listing but also the sociodemographic characteristics of the area in which the apartment is located. Yin’s (2018) involves six stages that have been applied in this research: planning, design, data collection, preparation, analysis, and sharing of information.
Research planning was conducted to estimate the time, cost, and suitability of the research topic. The initial plan was to identify a management model for revenue managers using technology transfer from a combination of databases.
The study design was determined with a view to establishing a technology transfer model focusing on the theory of mixed revenue management and its practical implications for the marketing management of Short-Term Rental apartments. The application of revenue techniques and technological software boosts the profit management of these real estate assets.
The dataset was mainly taken from Airbnb, compiling public information and data provided by the listing managers, data obtained through the big data research company, seeTransparent.com, for a sample of more than 1,048,576 day rentals, along with the internal historical data of MyCityhome, among others. The study analyses these technological database sources for apartments booked (with a nightly rate) through Airbnb between May 2021 and April 2022 (inclusive) focusing on the area of Madrid and the representative listings for this study. In total, 569 properties were selected (Table 1) with at least one booking in the research period. On average, these properties had been booked for 51% of the established period (187 days). Moreover, the internal features of each property are specified in the Airbnb database: booking price, day/month/year, rooms, beds, and equipment.
The information provided by the SeeTransparent database included the apartment coordinates. These coordinates can be used to geolocate each apartment. Through these coordinates, each apartment was linked to data about its surrounding environment. The Deskmind Research database, based on data compiled by the INE (National Institute of Statistics), provides sociodemographic information for each census section and was used here as a primary source to establish the characteristics of the apartment’s surrounding area.
The data for each apartment were correlated to the features within a 500-m radius of the apartment location, and the aggregated data from these census sections were linked to each property as a new descriptive variable.
First, the study began with aggregated data collection, working out the nightly rate charged to measure the market-adjusted price based on information from the SeeTransparent database and SPSS software (Table 2 and Figure 1).
Second, the average price per night was calculated for Short-Term Rental apartments booked from May 2021-April 2022. This average was $121 per night with a standard deviation of $97 (Table 2).
Third, the mean values and frequencies of all 28 internal characteristics (number of bathrooms, bedrooms, beds, capacity, kitchen, washing machine…view, terrace) (Table 3). of these 569 Short-Term Rental apartments were calculated. On average, each property has 1.26 bathrooms, 1.39 bedrooms, and 2.08 beds. Furthermore, the vast majority of them have a kitchen (97%), washing machine (90%), heating (89%), and air conditioning (82%).
Fourth, the study includes descriptions of the areas surrounding the 569 properties (within a radius of 500 m) taken from the other technological database used (Deskmind Research) indicating 9 sociodemographic features of these surrounding locations, such as resident population, the density of resident population, socioeconomic index, % of second homes, number of shops, restaurants, bars, hotels, public transport stations, and cultural venues (Table 4). These factors influence the growth of the market as well as the impossibility of renegotiating due to the common approach taken by governments to defend tenants’ interests. The combination of the different databases allows the collection of multiple data sources and further geolocation analysis.
The analysis developed within this study was applied to explore the correlation between the nightly rate charged and the 28 internal characteristics of the apartments (Table 3). Pearson’s coefficient is between 0.36 and 0.42 for bathrooms, bedrooms, beds, and capacity, indicating a moderate correlation.
The correlation between the nightly rate charged and the 9 sociodemographic features of the surrounding area is shown in Table 5. The highest values for Pearson’s coefficients are found for the number of hotels/residences (0.103), public transport stations (0.099), and cultural venues (0.096). As regards the resident population (-0.084) and population density (-0.084), they present a negative correlation with the nightly rate.
The transferability of the algorithm refers to the degree to which the findings of this case study can be generalized in the context of Short-Term Rental apartments through multivariate inferential statistics such as Exploratory Factor Analysis (EFA) and Statistical Regression Technique (SRT). This technological algorithm can estimate a model in which the market-adjusted price could be established for a Short-Term Rental apartment if the location and internal features are known in advance.
The proposed technological algorithm was first used in an Exploratory Factor Analysis of the main factors (28 internal characteristics and 9 sociodemographic features of the surrounding area) that most influence the market-adjusted price of a Short-Term Rental apartment when the location and internal characteristics are known in advance (37 variables in total, 28 + 9).
Exploratory Factor Analysis (EFA) is reduced here to 12 common factors with different descriptive categories and a deep understanding of their influence on the nightly rate. As this subject has never been explored in this way before, EFA was chosen instead of Confirmatory Factor Analysis (CFA) for its validity and reliability. The validity of the new factors was then evaluated using Bartlett’s Test of Sphericity, where p < 0.05 indicates that the matrix is adequate due to the high correlations between the variables. Reliability was confirmed using the KMO test (Kaiser–Mayer–Olkin), where a value of at least 0.6 indicates that partial correlations between variables are acceptable.
An Exploratory Factor Analysis was applied to condense these 37 items into a lower number of dimensions. Bartlett’s Test of Sphericity (p < 0.000) and the KMO index (0.751 higher than 0.7) justify the application of factor analysis (Table 6).
Communalities indicate the amount of variance in each variable that is accounted for. In this EFA, all 38 variables are higher than 0.5, indicating a good explanation capacity for this model (Table 7).
Several iterations were conducted to reach the optimal number of factors. In particular, the total variance explained by these 12 factors/components is 82.721%, showing that the data are useful (Table 8).
The rotated component matrix (Table 9) determines factor composition. In this study, 12 factors are identified:
Following EFA, Multiple Linear Regression was conducted to define an algorithm (technology transfer model) that explains the average rate charged based on 12 factors of the Short-Term Rental apartments related to the internal property features and the sociodemographic data of the surrounding area.
With an R of 0.754 and an adjusted R squared of 0.569, the model obtained is deemed to be reliable (Table 10).
Since the factors are independent of one another, standardized beta coefficients can be used to estimate the weight of each dimension (factor) in terms of the market-adjusted rental price (Table 11).
Comparing findings with the existing literature, the research presented here is focused on the geolocation of the property with regard to its sociodemographic surroundings. In this regard, Benítez-Aurioles (2018) and Gunter and Önder (2018) also focus on geolocation, showing that listing price is related to distance from the city center and that the response time of the host is negatively correlated with such bookings.
Additionally, this study considers prices, influenced by the sociodemographic variables of the property’s surrounding area, as positive. In this regard, Filippas and Horton (2018) focus on the decline in the demand for housing if the neighbors fear a high turnover or unfamiliar people in their neighborhood. In contrast, authors such as Yifei et al. (2022) found that location conditions have a limited impact on price in areas with established transportation networks.
Focusing on internal characteristics such as bedrooms, bathrooms, beds, and capacity, in this case, Shokoohyar et al. (2020) argued that “properties with more bedrooms, closer to the historic attractions, in neighborhoods with lower minority rates and higher nightlife vibe are more likely to have a higher return if they are rented out through a Short-Term Rental contract.”
Looking at internal characteristics, Yifei et al. (2022) show that the quality of a property plays a key role in forming the listing prices. The research presented in this paper shows that the nightly rate charged presents a negative correlation between the resident population and population density. In this regard, Espinosa (2016) and Kim et al. (2017) show that Short Term Rental properties also disrupt existing property and hospitality industries and cause disturbance in the surrounding neighborhood.
Other authors, such as Mody et al. (2021) considered the impact on non-hosting residents’ quality of life, finding that they perceived more positive than negative impacts on price. Similarly, the research takes into account data for Airbnb in Madrid, identifying the correlation between resident population and population density. In this regard, Martínez-Caldentey et al. (2020) state that tourist rentals arranged through platforms such as Airbnb have resulted in over-housing. The historic center of Madrid is an example of this since the Centro district is becoming practically a tourist resort, with the largest number of Airbnb listings concentrated there.
All the factors studied in this research are factors that may be gathered in a kick off state of the commercialization of the asset and are not dependent on the historical data that may be gathered within time. They are specific to the asset and independent of market competition and calendar events that may also affect the pricing of the apartment.
This paper aimed to find a behavioral psychology study, generating a revenue management model that establishes the relationship between the factors of a property listed in the Airbnb marketplace, or similar, for a Short-Term Rental lease and its optimal rental price set when the property is first put on the market. Findings confirm that the price per night of apartments is influenced by both, the internal features of the property and the sociodemographic characteristics of the area. In this context, some theoretical and practical implications are drawn out in the next lines.
The original theoretical scope of this study was to make a comparison between Short-Term Rental and Long-Term Rental revenue. Nevertheless, during the development of the project, it became clear that this initial goal could not be achieved without establishing an empirical foundation to value the different rental models based on the existing literature and adding to it with these new studies. Therefore, based on Yin’s approach, a technology transfer model for establishing the rental price of Short-Term Rental apartments was chosen as a new theoretical implication, narrowing this down to the internal characteristics of the property and sociodemographic variables of the surrounding area. Revenue managers usually set a starting price for Short-Term Rentals through experience, benchmarking, and personal impression, but they do not have a theoretical model to perform this calculation, and results are a little too arbitrary for such an important aspect of property revenue management.
The statistical relationships arising from Yin’s methodological study and these theoretical developments between price and internal characteristics, and between price and sociodemographic variables, confirm the hypotheses mentioned above. “Hypothesis 1” states that the nightly rate is moderately affected by internal characteristics (refer to Table 3, where Pearson’s coefficient sits between 0.36 and 0.42 for bathrooms, bedrooms, beds, and capacity). “Hypothesis 2” indicates that the nightly rate is slightly influenced by the sociodemographic variables of the property’s surrounding area [refer to Table 5, where the highest values for Pearson’s coefficients are found for the number of hotels/residences (0.103), public transport stations (0.099), and cultural venues (0.096)]. Regarding the resident population (-0.084) and population density (-0.084), this study found a negative correlation with the nightly rate.
With regard to the Exploratory Factor Analysis conducted, 37 variables were reduced to 12 common factors with different descriptive categories and a deep understanding of their influence on the rental price. In particular, the total variance explained by these 12 factors/components was 82.721%, validating the data (refer to Table 8).
Finally, Multiple Linear Regression was applied in order to define an algorithm (technology transfer model) that explains the average rental price based on 12 factors related to the internal characteristics of Short-Term Rental apartments and the sociodemographic features of their surrounding area. With an R of 0.754 and an adjusted R squared of 0.569, the model obtained has a strong predictive/explanatory capacity (refer to Table 10).
The practical implications of this research involve the implementation of a technology transfer model for revenue management in Madrid based on the main factors affecting the starting prices of Short-Term Rental apartments (refer to Table 11). Essentially, revenue management should be left to pricing decision-makers who are aware of consistent records regarding internal characteristics of the properties and the sociodemographic environment. In particular, the main practical implications of this proposed technology transfer model are:
a) The size/capacity of the properties offered largely determines the Short-Term Rental price (72%).
b) The equipment available in the property influences the Short-Term Rental price to a much lesser extent than its size/capacity (18%).
c) The characteristics of the surrounding area have an even lower impact on the Short-Term Rental price than the previous two factors (11%).
In short, this technology transfer model allows revenue managers and peers to estimate what the market-adjusted price should be for these Short-Term Rental apartments, whose location and internal characteristics are known in advance, as a starting point.
The model chosen achieves different levels of technological development and shows that certain factors significantly affect the price. These factors are quantitative indicators of Short-Term Rental apartments. However, qualitative indicators, such as reviews of the tenant or tourist users have not been included in the model.
Moreover, the data were collected from April 2021 to May 2022 (inclusive). The COVID-19 pandemic may have affected the price, the commitment of the tenant, and their booking process due to travel restrictions and health requirements. Future lines of research will consider correction factors for this technological model on the grounds of seasonality and market trends. Seasonality and market trends will presumably also influence the rental price of Short-Term Rental apartments. Similarly, Long-Term Rental pricing could be studied and compared with Short-Term Rental for these kinds of fully furnished properties.
The data collected for this study were extracted from two technological databases, namely SeeTransparent, based mainly on Airbnb (28 internal characteristics of the apartment), and Deskmind Research (9 sociodemographic variables of the surrounding area). Requests to access these datasets can be directed to the corresponding author/s.
DJ and DP-B conceptualized the theoretical framework, involved in data preparation, and prepared the first draft of the manuscript. DJ and JE performed the data analyses. DJ, DP-B, and JE revised and improved the multivariate analyses, performed and made important contributions linked to the theoretical literature. All authors read and approved the final version of the text.
The authors declare that the research was conducted in the absence of any commercial or financial relationships that could be construed as a potential conflict of interest.
All claims expressed in this article are solely those of the authors and do not necessarily represent those of their affiliated organizations, or those of the publisher, the editors and the reviewers. Any product that may be evaluated in this article, or claim that may be made by its manufacturer, is not guaranteed or endorsed by the publisher.
All claims expressed in this article are solely those of the authors.
Airbnb Fast Facts, (2022). Available online at: https://press.airbnb.com/about-us (accessed June 7, 2022).
Ali, A., Qadir, J., Rassol, R., Sathiaseelan, A., Zwitter, A., and Crowcroft, J. (2016). Big data for development: Applications and techniques. Big Data Analysis 1:2. doi: 10.1186/s41044-016-0002-4
Ali, J., Yan, L., Hu, Y., and Liu, Y. (2022). An investigation into the effects of destination sensory experiences at visitors’ digital engagement: empirical evidence from sanya, China. Front. Psychol. 13:942078. doi: 10.3389/fpsyg.2022.942078
Ayouba, K., Breuille, M. L., Grivault, C., and Le Gallo, J. (2020). Does airbnb disrupt the private rental market? An empirical analysis for french cities. Int. Regional Sci. Rev. 43, 76–104. doi: 10.1177/0160017618821428
Barron, K., Kung, E., and Proserpio, D. (2018). The sharing economy and housing affordability: Evidence from Airbnb. SSRN Work. Pap. doi: 10.2139/ssrn.3006832
Belobaba, P. P. (1987). Airline yield management: An overview of seat inventory control. Trans. Sci. 21, 63–73. doi: 10.1287/trsc.21.2.63
Benítez-Aurioles, B. (2018). Why are flexible booking policies priced negatively? Tourism Manage. 67, 312–325. doi: 10.1016/j.tourman.2018.02.008
Brotman, B. A. (2021). Portland ordinances: Tiny home and short-term rental permits. Int. J. Housing Markets Analy. 14, 124–136. doi: 10.1108/IJHMA-02-2020-0012
Buhalis, D., and Sinarta, Y. (2019). Real-time co-creation and nowness service: Lessons from tourism and hospitality. J. Travel Tourism Marke. 36, 563–582. doi: 10.1080/10548408.2019.1592059
Buhalis, D., Harwood, T., Bogicevic, V., Viglia, G., Beldona, S., and Hofacker, C. (2019). Technological disruptions in services: Lessons from tourism and hospitality. J. Service Manage. 30, 484–506. doi: 10.1108/JOSM-12-2018-0398
Cha, J., Kim SeungHyun, J., Beck, J., and Knutson, B. J. (2017). Predictors of career success among lodging revenue managers: Investigating roles of proactive work behaviors. Int. J. Hospit. Tour. Administr. 18, 474–490. doi: 10.1080/15256480.2017.1305307
Chen, W., Wei, Z., and Xie, K. (2019). The battle for homes: How does home sharing disrupt local residential markets? SSRN Manage. Sci. doi: 10.2139/ssrn.3257521
Choi, S., and Mattila, A. S. (2004). Hotel revenue management and its impact on customers’ perceptions of fairness. J. Rev. Pricing Manage. 2, 303–314. doi: 10.1057/palgrave.rpm.5170079
Choi, T. M., Wallace, S. W., and Wang, Y. (2018). Big data analytics in operations management. Produc. Operat. Manage. 27, 1868–1883. doi: 10.1111/poms.12838
Cui, N., Gu, H., Shen, T., and Feng, C. (2018). The impact of micro-level influencing factors on home value: A housing price-rent comparison. Sustainability. 10, 4343. doi: 10.3390/su10124343
Cullen, K. (2015). The evolving dynamics of revenue management. Nashville: HSMAI Foundation and STR Publisher.
Demirciftci, T., and Belarmino, A. (2022). A cross-cultural study of competitive intelligence in revenue management. J. Rev. Pricing Manage. 21, 691–699. doi: 10.1057/s41272-022-00379-6
Deskmind Research (2022). Internal database with restricted access. Madrid. Available online at: https://deskmind.es
Einav, L., Farronato, C., and Levin, J. (2016). Peer-to-peer markets. Ann. Rev. Econ. 8, 615–635. doi: 10.1146/annurev-economics-080315-015334
El Haddad, R., Roper, A., and Jones, P. (2008). “The impact of revenue management decisions on customers attitudes and behaviours: A case study of a leading UK budget hotel chain,” in Proceedings of the EuroCHRIE 2008 Congress, Dubai.
Elshawi, R., Sakr, S., and Talia, D. (2018). Big data systems meet machine learning challenges: towards big data science as a service. Big Data Res. 14, 1–11. doi: 10.1016/j.bdr.2018.04.004
Espinosa, T. P. (2016). The cost of sharing and the common law: How to address the negative externalities of home-sharing. Chapman. Law. Review. 19, 597–627.
Ferguson, M., and Smith, S. (2014). The changing landscape of hotel revenue management and the role of the hotel revenue manager. J. Rev. Pricing Manage. 13, 224–232. doi: 10.1057/rpm.2014.11
Filippas, A., and Horton, J. (2018). The tragedy of your upstairs neighbors: when is the home–sharing externality internalized? Nyu stern school of business. New York: Mimeo. doi: 10.1145/3033274.3085097
Fishman, S. (2015). Overview of airbnb law in San Francisco. Available online at: https://www.nolo.com/legal-encyclopedia/overview-airbnb-law-san-francisco.html
Gárate Alvarez, S., and Pennington-Cross, A. (2021). Short-term property rental platforms and the housing market: House prices and liquidity. J. Hous. Res. 2022:3389. doi: 10.1080/10527001.2022.2033389
Garcia-López, M. A., Jofre-Monseny, J., Martínez Mazza, R., and Segú, M. (2020). Do short-term rental platforms affect housing markets? Evidence from airbnb in barcelona. J. Urban Econ. 2020:103278. doi: 10.1016/j.jue.2020.103278
Gunter, U., and Önder, I. (2018). Determinants of airbnb demand in vienna and their implications for the traditional accommodation industry. Tourism Econ. 24, 270–293. doi: 10.1177/1354816617731196
Heo, C. Y., and Lee, S. (2010). Influences of consumer characteristics on fairness perceptions of revenue management pricing in the hotel industry. Int. J. Hospit. Manage. 30, 243–251. doi: 10.1016/j.ijhm.2010.07.002
Horn, K., and Merante, M. (2017). Is home sharing driving up rents? Evidence from airbnb in boston. J. Hous. Econ. 38, 14–24. doi: 10.1016/j.jhe.2017.08.002
Ivanov, S., and Zhechev, V. (2012). Hotel revenue management - a critical literature review. Tourism Rev. 60, 175–197. doi: 10.2139/ssrn.1977467
Jefatura del Estado (1994). Available online at: https://www.boe.es/eli/es/l/1994/11/24/29/con (accessed June 12, 2022)
Kim, J. H., Leung, T., and Wagman, L. (2017). Property rights and neighborhood externalities: Evidence from short-term rental regulations. J. Law Econ. 60, 309–334. doi: 10.1086/694415
Kimes, S. E., and Rohlfs, K. (2007). Customers’ perceptions of best available hotel rates. Cornell Hotel. Restaurant Administr. Quart. 48, 151–162. doi: 10.1177/0010880407300562
Kimes, S. E., and Taylor, W. J. (2010). How hotel guests perceive the fairness of differential room pricing. Cornell Hospit. Rep. 10, 4–14.
Kimes, S. E., and Wirtz, J. (2007). The moderating role of familiarity in fairness perceptions of revenue management pricing. J. Serv. Res. 9, 229–240. doi: 10.1177/1094670506295848
Koster, H. R., Ommeren, J. V., and Volkhausen, N. (2021a). Short-term rentals and the housing market: Quasiexperimental evidence from Airbnb in Los Angeles. J. Urban Econ. 124, 103356. doi: 10.1016/j.jue.2021.103356
Koster, E. S., Philbert, D., and Bouvy, M. L. (2021b). Impact of the COVID-19 epidemic on the provision of pharmaceutical care in community pharmacies. Res. Soc. Adm. Pharm. 17, 2002–2004. doi: 10.1016/j.sapharm.2020.07.001
Kremic, T. (2003). Technology transfer: A contextual approach. J. Technol. Trans. 28, 149–158. doi: 10.1023/A:1022942532139
Kutter, R. (1991). How national security hurts national competitiveness. Harvard Bus. Rev. 69, 140–149.
Lentz, M., Berezan, O., and Raab, C. (2021). Uncovering the relationship between revenue management and hotel loyalty programs. J. Rev. Pricing Manage. 21:331. doi: 10.1057/s41272-021-00331-0
Levin, A., Lin, C. F., and Chu, C. S. J. (2002). Unit root tests in panel data: Asymptotic and finite-sample properties. J. Econ. 108, 1–24. doi: 10.1016/S0304-4076(01)00098-7
Li, H., and Srinivasan, K. (2019). Competitive dynamics in the sharing economy: an analysis in the context of airbnb and hotels. Marke. Sci. 2018:1143. doi: 10.1287/mksc.2018.1143
Littlewood, K. (1972). Forecasting and control of passenger bookings, AGIFORS symposium proceedings. Israel: Nathanya.
Martínez-Caldentey, M. A., Blázquez-Salom, M., and Murray, I. (2020). Habitabilidad y airbnb: el alquiler de la vivienda en el distrito centro de madrid. Cuadernos de Turismo. 46, 367–393. doi: 10.6018/turismo.451881
Matsuoka, K. (2022). Effects of revenue management on perceived value, customer satisfaction, and customer loyalty. J. Bus. Res. 148, 131–148. doi: 10.1016/j.jbusres.2022.04.052
Mody, M., Suess, C., and Dogru, T. (2021). Does airbnb impact non-hosting residents’ quality of life? Comparing media discourse with empirical evidence. Tourism Manage. Perspect. 39:100853. doi: 10.1016/j.tmp.2021.100853
MyCityHome (2022). Internal Database with restricted access. Madrid. Available online at: https://mycityhome.es
NASA Aeronautics (1993). Impact of technology transfer activities is uncertain report to congressional requestors. Available online at: https://www.gao.gov/products/nsiad-93-137
O’Sullivan, F. (2016). The city with the world’s toughest anti-Airbnb laws. CityLab, 1 December. Available online at: https://www.bloomberg.com/news/articles/2016-12-01/the-growing-pains-of-berlin-s-tough-anti-airbnb-laws
Osman-Gani, A. A. M. (1999). nternational technology transfer for competitive advantage: a conceptual analysis of the role of HRD. Competit. Rev. 9, 9–18. doi: 10.1108/eb046370
Saura, J. R., Palos Sánchez, P. R., and Reyes Menéndez, A. (2017). Marketing a través de aplicaciones móviles de turismo (m-tourism): un estudio exploratorio. Int. J. World Tourism. 4, 45–56. doi: 10.12795/IJWT.2017.i08.04
Saura, J. R., Ribeiro- Soriano, D., and Palacios-Marqués, D. (2022). Exploring the boundaries of open innovation: Evidence from social media mining. Technovation 2021:102447. doi: 10.1016/j.technovation.2021.102447
Saura, J. R., Ribeiro- Soriano, D., and Palacios-Marqués, D. (2021). From user-generated data to data-driven innovation: A research agenda to understand user privacy in digital markets. Int. J. Informat. Manage. 60:102331. doi: 10.1016/j.ijinfomgt.2021.102331
SeeTransparent Airbnb (2022). Internal database with restricted access. Madrid. Available online at: https://seetransparent.com
Selmi, N., and Dornier, R. (2011). Yield management in the french hotel business: An assessment of the importance of the human factor. Int. Bus. Res. 4:2. doi: 10.5539/ibr.v4n2p58
Shoemaker, S. (2003). The future of pricing in services. J. Rev. Pricing Manage. 2, 271–279. doi: 10.1057/palgrave.rpm.5170074
Shoemaker, S. (2005). Pricing and the consumer. J. Rev. Pricing Manage. 4, 228–236. doi: 10.1057/palgrave.rpm.5170144
Shokoohyar, S., and Sobhani, A., and Sobhani, A. (2020). Determinants of rental strategy: Short-term vs long-term rental strategy. Int. J. Contemporary Hospit. Manage. 32, 3873–3894. doi: 10.1108/IJCHM-03-2020-0185
Shy, O. (2008). How to price. A guide to pricing techniques and yield management. Cambridge: Cambridge University Press.
Soeder, W. E., Nashar, A. S., and Padmanabhan, V. (1990). A guide to the best technology-transfer practices. J. Technol. 15:5. doi: 10.1007/BF02377652
Sundararajan, A. (2014). Peer-to-peer businesses and the sharing (collaborative) economy: overview, economic effects and regulatory issues.
Talluri, K. T., and van Ryzin, G. (2005). The theory and practice of revenue management. New York: Springer Science+BusinessMedia. doi: 10.1007/b139000
Tood, J., Musah, A., and Cheshire, J. (2021). Assessing the impacts of Airbnb listings on London house prices. Environ. Plan. B 49, 206–222. doi: 10.1177/23998083211001836
Tse, T., and Poon, Y. (2012). Revenue management: resolving a revenue optimization paradox. Int. J. Contemp. Hospit. Manage. 24, 507–521. doi: 10.1108/09596111211226798
Tu, Y., Neuhofer, B., and Viglia, G. (2018). When co-creation pays: stimulating engagement to increase revenues. Int. J. Contemp. Hospit. Manage. 30, 2093–2111. doi: 10.1108/IJCHM-09-2016-0494
Tversky, A., and Kahneman, D. (1981). Prospect theory: An analysis of decision under risk. Econometrica 47, 263–291. doi: 10.2307/1914185
Wang, X. L., and Brennan, R. (2014). A framework for key account management and revenue management integration. Industr. Marke. Manage. 43, 1172–1118. doi: 10.1016/j.indmarman.2014.06.006
Yeoman, I. (2022). The value of revenue management and pricing in a challenging environment. J. Rev. Pricing Manage. 22:395. doi: 10.1057/s41272-022-00395-6
Yifei, J., Honglei, Z., Xianting, C., Ge, W., and Yang, Y. (2022). How to better incorporate geographic variation in Airbnb price modeling? Tourism Econ. 1177:7585. doi: 10.1177/13548166221097585
Yin, R. K. (2018). Case study research and applications: design and methods, 6th Edn. Thousand Oaks, CA: Sage.
Keywords: revenue management, short—term rental, dwellings for tourism use pricing, behavioral psychology, exploratory factor analysis, multiple linear regression, technology transfer model, first price
Citation: de Jaureguizar Cervera D, Pérez-Bustamante Yábar DC and de Esteban Curiel J (2022) Factors affecting short-term rental first price: A revenue management model. Front. Psychol. 13:994910. doi: 10.3389/fpsyg.2022.994910
Received: 15 July 2022; Accepted: 17 October 2022;
Published: 16 November 2022.
Edited by:
Belem Barbosa, University of Porto, PortugalReviewed by:
Francisco Javier S. Lacárcel, University of Alicante, SpainCopyright © 2022 de Jaureguizar Cervera, Pérez-Bustamante Yábar and de Esteban Curiel. This is an open-access article distributed under the terms of the Creative Commons Attribution License (CC BY). The use, distribution or reproduction in other forums is permitted, provided the original author(s) and the copyright owner(s) are credited and that the original publication in this journal is cited, in accordance with accepted academic practice. No use, distribution or reproduction is permitted which does not comply with these terms.
*Correspondence: Diana C. Pérez-Bustamante Yábar, ZGlhbmEucGVyZXpidXN0YW1hbnRlQHVyamMuZXM=
Disclaimer: All claims expressed in this article are solely those of the authors and do not necessarily represent those of their affiliated organizations, or those of the publisher, the editors and the reviewers. Any product that may be evaluated in this article or claim that may be made by its manufacturer is not guaranteed or endorsed by the publisher.
Research integrity at Frontiers
Learn more about the work of our research integrity team to safeguard the quality of each article we publish.