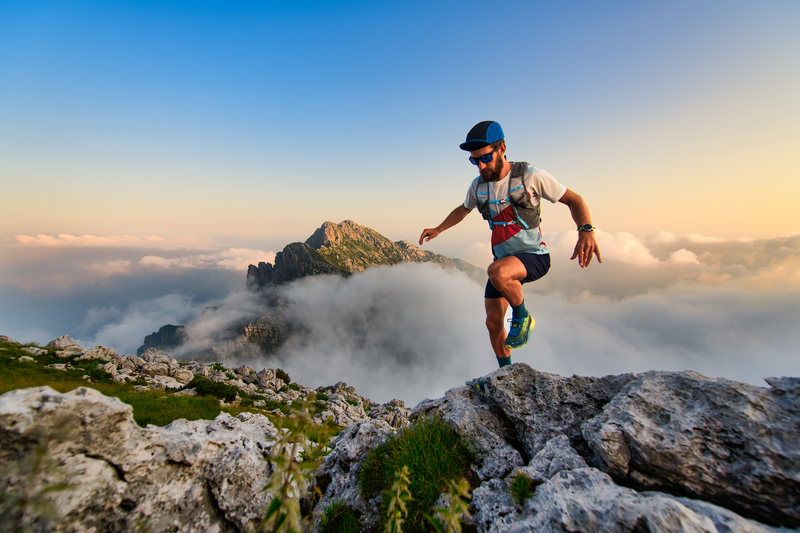
95% of researchers rate our articles as excellent or good
Learn more about the work of our research integrity team to safeguard the quality of each article we publish.
Find out more
ORIGINAL RESEARCH article
Front. Psychol. , 03 January 2023
Sec. Organizational Psychology
Volume 13 - 2022 | https://doi.org/10.3389/fpsyg.2022.948982
This article is part of the Research Topic Governing Sustainability Transitions in Agribusinesses and Food-Systems: A Behavioral Foundations View View all 9 articles
Agricultural retailers face serious challenge of losing consumers due to channel integration, it has become essential to provide an expected consistent and seamless omni-channel shopping experience in an omni-channel environment. Although previous literature has begun to focus on the consumer perspective of the omni-channel experience, little attention has been paid to the process of change from a single retail environment to omni-channel. By combining the research frameworks of unified theory of acceptance and use of technology (UTAUT) and the value-based adoption model (VAM), this study aims to identify the drivers of omni-channel consumer purchase intention in agriculture products retail. This article conducts a questionnaire survey on 620 samples in China. The results show that single-channel shopping cost, reference groups, positive online reviews, and single-channel perceived risk have a significant positive impact on the omni-channel purchase intention of agricultural products, and perceived value plays a mediating role. Moreover, contextual factors partially have a negative moderating effect. When consumers purchase agricultural products, the more suitable the online shopping environment is, the weaker the influence of single-channel shopping costs, reference groups and positive online reviews on consumers’ omni-channel purchase intention. These findings build on the existing literature on the omni-channel retail consumer experience and provide insights for fresh produce retailers to implement and evaluate an omni-channel integration strategy for agriculture products. The findings may shed lights on how to promote the healthy development of the omni-channel sales model of agricultural products.
The spread of COVID-19 drives a huge surge in demand for agricultural products, however, the challenges surrounding the production, service, logistics and labor availability of agricultural products have posed a constant threat (De and Singh, 2021). As a result, the key adjustment for marketers is to move from single-channel to omni-channel collaboration in managing consumer relationships, in other words, using goods to attract people (Huré et al., 2017). Chinese retailers are attempting to innovate their produce distribution models to digitally engage consumers and create a unified and seamless experience across intermingled touch points (Morgenstern, 2021), such as live-streaming vegetable sales, contactless deliveries, and smart food baskets.1 Clearly this demand has been amplified by the enhanced ability of consumers to choose their channels. Digital News Asia (2019) data shows that 90% of Asian consumers prefer a combination of channels to purchase goods (mobile, app, in-store or desktop). By the end of 2021, the scale of fresh food e-commerce transactions will reach 68.9 billion dollars, a year-on-year increase of 27.92%. The penetration rate of the fresh food e-commerce industry reached 7.91% in 2021.2 This new omni-channel environment is blurring the boundaries between offline and online retailing, with each channel taking on a networked distribution to cater consumers’ purchasing needs across time and geography (Zhang et al., 2021). Meeting these expectations can be challenging for retailers, however, if successfully achieved, retailers will improve sales growth and improve customer retention (Fang et al., 2021).
In the traditional agricultural distribution system, farmers are connected to markets through the Farmers-Origin Wholesale Market—Retail Terminal (Ming et al., 2021). However, in China, the long distribution chain and the high cost of transportation and storage often result in negative phenomena—contradictions between supply and demand, slow transmission of information in the supply chain and narrowing of suppliers’ access to goods (Liang et al., 2022). With the development of e-commerce and information technology, from the earliest days when there was only a single channel, it evolved to dual channels, multiple channels and eventually to the current common omni-channel. The omni-channel agricultural supply chain that this study concerned usually consists of a single farmer cooperative and one online retailer offering three shopping options, namely pick-your-own, online purchase and home delivery (Hu and Xu, 2019).
In omni-channel shopping, consumers not only get the same quality of products as in fresh produce shops, but also the convenience of e-commerce (Zhang et al., 2020). Also, integrating online-offline channels offers an innovative way of thinking to solve the “last mile” problem of fresh produce (Janjevic et al., 2021; Wang et al., 2022). However, the plans to connect, collaborate and synchronize the various channels are large and complex for retailers (Abrudan et al., 2020), so it is necessary to measure consumer value to assess the relevance of these efforts (Huré et al., 2017). It will promote better planning by retailers and increase the knowledge of consumers’ willingness to buy agricultural products.
Although omni-channel retailing has generated a lot of interest among marketing researchers, it needs to be explored further in the fresh agri-produce sector. Existing research has focused on pricing and service level decisions in the fresh produce supply chain (Yu and Xiao, 2017; Jin et al., 2018), logistics distribution models (Ailawadi and Farris, 2017; Wollenburg et al., 2018) and digital strategic governance (Saghiri and Mirzabeiki, 2021; Zhang et al., 2021). For example, Jin et al. (2018) explored omni-channel supply chain pricing and order fulfillment on buy–online–pick-up-in-store mode. This illustrates the importance of coordination and optimization of the omni-channel supply chain. In addition, a small number of empirical studies in other areas have begun to recognize shopping value of omni-channel (Murfield et al., 2017; Pallant et al., 2020). Huré et al. (2017) identifies three dimensions for accurately measuring omni-channel shopping value: utilitarian, hedonic and social dimensions. Researchers have also demonstrated that personal innovativeness, effort expectancy, and performance expectancy influence omni-channel consumers’ behavior (Juaneda-Ayensa et al., 2016). But they rarely focus on the process of change from a single retail environment to an omni-channel. What influences consumer behavior? And how retailers are guiding consumers through the transition to an omni-channel environment and how they can leverage various channels to maximize consumer value?
Unlike other industries, fresh produce has a short life cycle, a high wastage rate and market demand fluctuates with the freshness of the product (Zhou et al., 2020; Ming et al., 2021); therefore, providing easier and more comprehensive perceived value is key to innovation in the agricultural supply chain. Moreover, in an omni-channel environment, the characteristics and attributes of touch points vary and consumer perceptions of them are relative (Stein and Ramaseshan, 2016; Huré et al., 2017). In general, based on the perspective of single touch points and other social impacts, we attempt to revisit the focus of omni-channel value measurement. Specifically, this study aims to answer the following three research questions: (1) Do single-channel shopping costs, reference groups, positive online reviews and single-channel perceived risk affect consumers’ perceived value and willingness to purchase agricultural products through omni-channel? (2) Do contextual factors moderate the relationship between perceived value and purchase intention to purchase agricultural products omni-channel? (3) What are the prerequisites for purchasing agricultural products omni-channel?
Understanding the significance of omni-channel for agricultural products is a pre-condition for capturing the value of omni-channel shopping among consumers. Rigby (2011) first introduced omni-channel concept in Harvard Business Review, which states that retailers integrate all available channels (e.g., stores, websites, social media, TV, etc.) to communicate, transact and co-create value with consumers. Compared with single channel or multi-channel, the unique aspects of omni-channel are: multiple interactions across channels and touch points; focus on the interaction between channels and brands; and meet consumers’ expectations for a seamless shopping experience (Huré et al., 2017; Jin et al., 2018). Verhoef et al. (2015) defined omni-channel management as “synergistic management of the numerous available channels and customer touchpoints, in such a wat that the customer experience across channels and the performance over channels is optimized.” Consumers can switch retail channels at multiple stages of the purchase journey based on their personal preferences, and ultimately complete shopping behaviors such as information search, product purchase, shipping, and returns (Asmare and Zewdie, 2022). Therefore, retailers need to develop omni-channel strategies in response to consumers’ changing purchase methods (e.g., in-store or online purchases) and preferences (e.g., home delivery or pick-up in store) (Murfield et al., 2017).
In the field of agricultural products, considering that channel demand is influenced by product freshness and price, researchers have tried to solve difficult supply chain problems (e.g., risk aversion and decision making) (Yu et al., 2018; Yan et al., 2020; Feng et al., 2021). But these studies side-by-side demonstrate the value of omni-channel, for example, that cross-channel integration can contribute to improved cost effectiveness (Hu and Xu, 2019). The above research provides a framework for further research in the area of agricultural products omni-channel retailing, but their usefulness in guiding practice is limited. To fill this gap, we theoretically investigate the impact of single-channel value of fresh produce and other social influences on the shopping value of consumers. And it provides insights in promoting the development of marketing strategies for agricultural products.
Most current retail research on new technology acceptance the theory of reasoned action (TRA), the theory of planned behavior (TPB), unified theory of acceptance and use of technology (UTAUT) and the technology acceptance model (TAM) to explore consumers’ purchase intentions (Herrero-Crespo et al., 2022; Kim et al., 2022; Mathavan et al., 2022).
Venkatesh and Davis (2000) extended TAM and effectively explained perceived usefulness and technology use intentions through social influence and cognitive processes. Social influence is defined as the degree to which an individual perceives that important others believe he or she should use the new system. Venkatesh et al. (2003) further constructed UTAUT, including four key elements, performance expectations, effort expectations, social impact, and convenience. UTAUT has been shown to predict technology acceptance among automotive retail users in an omni-channel context (Kim et al., 2022). One manifestation of implementing an omni-channel strategy is replacing some big stores with online stores. It can reduce operating costs and facilitate the integration of online and offline channels (Kazancoglu and Aydin, 2018). But some consumers are still skeptical of such a model, which implies that contextual factors may influence consumers’ decision-making processes in an omni-channel context (Daugherty et al., 2019). In this regard, this study focuses on the social impact of UTAUT, exploring the role of reference groups, positive online reviews.
Kim et al. (2007) argued that TAM is considered for adoption from the perspective of technology users, while consumers differ from technology users in terms of bearing costs and risks, and therefore proposed the value-based adoption model (VAM). And he analyzed consumers’ adoption of new technologies and their purchase behavior in the e-commerce technology environment from the perspective of value maximization. VAM has been found to be effective in terms of intention to use (Vishwakarma et al., 2020). Overall, considering the special attributes of agricultural products, channel characteristics, channel transfer costs, and changes in perceived value, this study uses a combined framework consisting of UTAUT and VAM, which is more suitable in predicting consumer purchase intention. To be specific, the purpose of this study is to investigate the effects of single-channel shopping costs, single-channel perceived risk, reference groups, and positive online reviews on perceived value and omni-channel purchase intentions. The conceptual model of this paper is shown in Figure 1.
As channel choice opportunities increase, consumers begin to consider the opportunity costs associated with shopping activities, such as product prices, search costs, switching costs, and transportation costs (Lim et al., 2011; Chang et al., 2017; Singh and Jang, 2022). Price is the currency that consumers pay to obtain a product or service (Wood and Scheer, 1996). Many scholars have studied the impact of price on the sales ability of enterprises. Forman et al. (2008) analyzed Amazon’s data and found that online retail prices can significantly affect retailers’ sales. Many previous studies have shown that online channels can effectively reduce consumers’ purchasing costs. Peterson and Merino (2003) pointed out that search cost refers to the time and physical effort that consumers spend in collecting product information during the shopping process. The research of Jepsen (2007) shows that consumers can search for information about purchasing products through online channels, which can break through the limitations of time and space and reduce the search cost. The dissemination of product quality information to consumers breaks through the limitations of time and space, which reduces the search cost of consumers and helps to increase their willingness to buy (Heng et al., 2018). Zheng et al. (2020) pointed out that competitive prices are a key factor for consumers to choose to buy agricultural products online. Zatz et al. (2021) compared consumers’ omni-channel purchase behavior and found that consumers are more likely to compare prices online, save time and convenience, improve consumers’ perceived value, and increase their willingness to buy. When purchasing agricultural products omni-channel, consumers can easily obtain all product-related information, effectively saving time and transportation costs. Consumers can also quickly obtain product information from successfully traded orders, further reducing search and switching costs in the purchasing process (Pozzi, 2012; Richards et al., 2017). Therefore, it is hypothesized that:
H1: Single-channel shopping costs have a significant positive effect on the perceived value of purchasing agricultural products omni-channel.
H2: Single-channel shopping costs have a significant positive effect on the purchase intention to purchase agricultural products omni-channel.
A reference group is a person or group of people who significantly influences an individual’s behavior. This concept is widely used in marketing strategies by marketing practitioners to influence consumers’ product and brand decisions (Bearden and Etzel, 1982; Bearden et al., 1989). (Amaldoss and Jain, 2005a,b, 2015) demonstrated that reference group effects can affect the retail price of firms and the branding decisions of consumers. Studies have shown that reference groups can directly affect individual behavior (Dimanche and Havitz, 1995; Welsch and Kühling, 2009). Escalas and Bettman (2005) pointed out that consistency within reference groups will have a significant positive impact on consumers’ brand perception. Sun et al. (2022) demonstrated that the strength of reference-group effects affects consumers’ product decisions. Thus, the firm will adopt a different introduction strategy for upgraded products. Therefore, it is hypothesized that:
H3: Reference groups have a significant positive effect on the perceived value of purchasing agricultural products omni-channel.
H4: Reference groups have a significant positive effect on the purchase intention of purchase agricultural products omni-channel.
Online reviews are a kind of interpersonal communication. Consumers transmit information related to products or sellers’ services through the Internet based on their own product purchase and use experiences (Hennig-Thurau et al., 2010). Some scholars have found that positive reviews can affect consumers’ perceived value. Online reviews provide consumers with more information, which is helpful for them to identify the quality of products, judge the ability of products to meet their needs, and eliminate their concerns (Kwark et al., 2014). Online reviews affect consumers’ perceived value, and choosing online review management platform services has become a consensus among B2C companies (König et al., 2022). Other studies have shown that positive reviews further influence consumer purchase intentions. Bao and Chang (2014) found that the effective use of online reviews by companies can increase sales. Online reviews will bring value-added effects to consumers, improve the accuracy of their judgments on product quality, and increase consumers’ purchasing intentions (Fan et al., 2020). In the era of mobile internet, nearly 95% of consumers will read online reviews before or during their purchasing decisions (Tom et al., 2020). Therefore, it is hypothesized that:
H5: A positive online review has a significant positive effect on the perceived value of purchasing agricultural products omni-channel.
H6: A positive online review has a significant positive effect on the purchase intention of agricultural products omni-channel.
Perceived risk is the consumer’s expectation of loss when making a purchasing decision (Stone and Grønhaug, 1993). Corbitt et al. (2003) believed that perceived risk affects consumer trust, which in turn affects purchase intention. Online channels cannot directly contact products, and virtual product information displays may be perceived as accurate enough to make suitable judgments (Park et al., 2005). Bennett and Harrell (1975) suggest that lower perceived risk may be related to higher purchase intention. Vijayasarathy and Jones (2000) found that perceived risk plays an important role in the process of making purchase decisions. Ueland et al. (2012) proposed that consumers’ willingness to purchase products depends on perceived risks and perceived benefits. Therefore, the following hypothesis was developed:
H7: Single-channel perceived risk has a significant positive effect on the perceived value of purchasing agricultural products omni-channel.
H8: Single-channel perceived risk has a significant positive effect on the purchase intention to purchase agricultural products omni-channel.
The perceived value constructed by Kim et al. (2007) includes the price paid to purchase the product, the usefulness of the product, and the hedonicity of the product. The selling price of a product directly affects perceived value (Zeithaml, 1988; Dodds William et al., 1991). Early research on perceived value focused on perceived quality and price (Chang and Wildt, 1994; Grewal et al., 1998).
Individuals evaluate the consequences of their actions based on perceived usefulness and choose actions based on the desirability of usefulness. The construction of usefulness is similar to the marketing concept of product quality, which is defined as the customer’s cognitive assessment of product excellence or superiority (Voss et al., 1998). Consumers believe that purchasing agricultural products omni-channel is more conducive to identifying product attributes, which are products with functions that satisfy them. Steenkamp (1990) defines product quality as fit for consumption, that is, the usefulness of a product in meeting consumer needs.
With the upgrade of consumption, consumers purchase products to obtain the functional value and hedonic value of the product (Babin et al., 1994). Davis et al. (1989) pointed out that hedonic value refers to the degree of pleasure brought to consumers by the activities of purchasing and using a product, excluding any expected functional value. Hedonic value represents intrinsic, emotional value. In addition to product usefulness, hedonic value is also an important component of perceived value (Davis et al., 1992). Hedonic value has a positive effect on perceived value (Petrick, 2002).
Neal’s (1999) study shows that perceived value will bring customer loyalty, generate purchase behavior, and further improve customer loyalty. Customer perceived value has a direct impact on purchase intention (Cronin et al., 2000; Sweeney and Soutar, 2001). Therefore, it is hypothesized that:
H9: Perceived value has a significant positive effect on the purchase intention of purchasing agricultural products omni-channel.
Contextual factors are the key factors affecting consumers’ purchase intention (Dhar, 1992). Contextual factors can influence consumers’ purchasing decisions to deviate from stable preferences (Prelec et al., 1997). Wu and Wang (2005) pointed out that in the process of transforming attitude into behavior, it will be affected by contextual factors. Contextual factors such as the price, availability, and convenience of purchasing channels of agricultural products can affect consumers’ attitudes and then determine their purchase intentions (Zepeda and Deal, 2010). Zhu et al. (2013) found that contextual factors such as the convenience of purchase significantly moderate the influence between consumers’ purchase intention and purchase behavior. Therefore, it is hypothesized that:
H10: Contextual factors will positively moderate the relationship between perceived value and purchase intention of purchase agricultural products omni-channel.
The design of the variable scales in the questionnaires in this paper draws on published research papers and is adapted from mature scales combined with the results of market interviews. The measurement items for single-channel shopping cost were adapted from Wood and Scheer (1996) and Jepsen (2007). reference groups was measured using four items adapted from Bearden and Etzel (1982) and Escalas and Bettman (2005). Positive online reviews were measured using five items adapted from Hennig-Thurau et al. (2010) and Fan et al. (2020). Single-channel perceived risk was measured using five items adapted from Stone and Grønhaug (1993) and Corbitt et al. (2003). The measurement of perceived value draws on the studies of, Davis et al. (1992); Voss et al. (1998), and Neal (1999). The measurement of purchase intention draws on the research of Cronin et al. (2000) and is designed from three aspects: willingness to shop omni-channel, possible omni-channel shopping in the future, and recommending others to shop omni-channel. The measurement of online contextual factors draws on the studies of Dhar (1992) and Zepeda and Deal (2010) and is designed from four aspects: purchasing convenience, price, availability, and policies and regulations.
As the original items were in English, we conducted a back translation to ensure translation validity. First, a researcher whose native language was Chinese translated the source items from English into Chinese. Next, another researcher independently translated these items back into English. Subsequently, the two researchers compared the two English versions and jointly revised the first Chinese version of the items. Based on their feedback, minor modifications were made to improve the comprehensiveness and user friendliness of the measurement items. To ensure the reliability and validity of the scale, the research team first consulted experts in relevant fields to propose revisions and then conducted a preliminary survey. The scale was revised according to expert opinions and preinvestigation results, and finally, a formal scale was formed. The final survey questionnaire is presented in Appendix. The dimensionality of all variables are unidimensional, and all items were measured on a 7-point Likert scale, which ranged from 1 (not agree at all) to 7 (absolutely agree).
All items were measured on a 7-point Likert scale, which ranged from 1 (not agree at all) to 7 (absolutely agree). To ensure the reliability and validity of the scale, the research team first consulted experts in relevant fields to propose revisions. We conducted a preliminary survey on the questionnaire with volunteers who have channel switch experience in purchasing agricultural products (146 volunteers in total). The scale was revised according to expert opinions and pre-investigation results, and finally, a formal scale was formed. The final survey questionnaire is presented in Appendix A.
A power analysis using R*power 3.6 computer software was conducted to calculate a suitable sample size (Hua et al., 2020). The results indicated that a sample size of 168 would be sufficient using a one-tailed test and a power (1 – β) = 0.95, given α = 0.05. The survey covered 635 respondents who were selected randomly from the consumers of Missfresh in China who were members of the firm’s site or app. The official survey of the data in this article will be conducted from December 2021 to March 2022. After purification of the questionnaire, incomplete answers were excluded or the same answer was selected for all the question items. We selected consumers who had either the experience or intention of purchasing agricultural products. Finally, we received 620 valid questionnaires, accounting for 93.37% of all the returned questionnaires.
The reasons for choosing Missfresh are as follows. Missfresh was established in November 2014 and was invested by Tencent. It is a technology-driven innovative community retail enterprise dedicated to allowing every family to buy with peace of mind and eat with confidence. As of April 2021, Missfresh ranked first in both the number and amount of financing events for fresh food e-commerce projects in China. Missfresh pioneered the “prewarehouse” model, providing “over 4,000 items of products to tens of millions of households in 16 cities, within 30 min of delivery.”
The demographic information of the final sample is summarized in Table 1. The gender distribution of the samples is dominated by female participants (77.26%), male participants (22.74%). The age distribution is mainly between 25 and 35 years old (82.26%). The monthly income of the sample is (unit: $), below 442.2 (19.53%), 442.2∼737 (49.74%), 737∼1,474 (24.48%), 1,474∼2,948 (4.95%). The most sample were highly educated, including undergraduate (83.87%), post-graduate or above (5.00%), junior college (5.64%), high school or below (5.48%). Dominici et al. (2021) point out that a young, well-educated, female consumer with a very good or adequate overall economic condition will be more likely to buy food online. Overall, the sample structure is basically consistent with the characteristics of cross-channel purchaser agricultural products, and the sample has good typicality and representativeness.
In this paper, SPSS 20.0 and AMOS 22.0 software were used to process the data and construct a structural equation model (SEM). First, this paper tests reliability and validity, including reliability, convergent validity and discriminant validity. Next, the paper constructs a SEM to examine the path coefficients between latent variables. Finally, this paper uses SPSS PROCESS v3.3 to test the moderating effect of contextual factors.
This paper implemented the evaluation of measurements based on their internal consistency reliability, convergent validity, and discriminant validity. Cronbach’s α and composite reliability (CR) are adopted to test internal consistency reliability (Fornell and Larcker, 1981; Hua et al., 2020). As shown in Table 2, the Cronbach’s α coefficient of all measurement items is above 0.701∼0.928, indicating a high level of reliability. The Cronbach’s α coefficients of the latent variables of single-channel shopping cost, reference groups, positive online reviews, single-channel perceived risk, perceived value, purchase intention, and contextual factors are 0.851, 0.798, 0.929, 0.928, 0.875, 0.911, and 0.847, respectively. The CR coefficients of all latent variables are greater than 0.80. The results indicate that all scales designed in this paper can reliably measure latent variables. The scales used in this paper are all representative mature scales, which are adopted from the published documents in Authoritative journals. Therefore, they have good content validity. Convergent validity is the extent of the positive relation between a measure and alternative measures of the same construct (Hair et al., 2014). The average variance extracted (AVE) of the variables was greater than 0.500, the factor loading of each observed variable exceeded 0.700, and the t-values all reached the significance level of p < 0.05 (t > 1.960), indicating that each variable had good convergent validity.
Discriminant validity refers to the extent to which a construct is truly distinct from others by empirical standards (Hair et al., 2014). This paper tests discriminant validity by comparing the correlation coefficient between the square root of the AVE value of the latent variable and other variables. As shown in Table 3, the square root of the AVE value of each construct’s variance inflation factor (single-channel shopping cost, reference groups, positive online reviews, single-channel perceived risk, perceived value, purchase intention, and contextual factors) is greater than its highest correlation coefficient with other variables, indicating that each latent variable’s discriminant validity was adequate.
AMOS 22.0 software is used to construct a SEM to test the conceptual model and the hypotheses shown in Figure 1. The test results of the hypotheses between single-channel shopping cost, reference groups, positive online reviews, single-channel perceived risk, perceived value, and purchase intention are shown in Table 4. As shown in Table 4, single-channel shopping cost has a positive influence on perceived value (β = 0.4, p < 0.001). Thus, hypothesis 1 is supported. In support of hypothesis 2, single-channel shopping cost has a positive influence on purchase intention (β = 0.26, p < 0.001). In support of hypothesis 3, reference groups have a positive influence on perceived value (β = 0.09, p < 0.05). In support of hypothesis 5, positive online reviews have a positive influence on perceived value (β = 0.16, p < 0.001). In support of hypothesis 6, positive online reviews have a positive influence on purchase intention (β = 0.18, p < 0.001). In support of hypothesis 7, single-channel perceived risk has a positive influence on perceived value (β = 0.24, p < 0.001). In support of hypothesis 9, perceived value has a positive influence on purchase intention (β = 0.64, p < 0.001). The overall fitting index of the model basically meets the requirements of the SEM, CMIN/DF = 2.42, less than 3, RMR = 0.06, GFI = 0.92, IFI = 0.97, CFI = 0.97, TLI = 0.96, all greater than 0.9, RMSEA = 0.05, less than 0.08, and the model reflects the relationship between latent variables well. Overall, the empirical results support the research model proposed in this paper, and the conceptual model estimation results are presented in Table 4 and Figure 2.
In this paper, the mediating effects of perceived value in the hypothesized model were tested for significance using the boot-strapping approach (5,000 replications). The boot-strapping procedure enhances the statistical power of mediation analysis, especially for a small or moderate sample size (Preacher and Hayes, 2008). As presented in Table 5, the direct effects of single-channel shopping cost on perceived value, reference groups on perceived value, positive online reviews on perceived value, single-channel perceived risk on perceived value, and perceived value on purchase intention were all significant. The direct effects of single-channel shopping cost on perceived purchase, positive online reviews on perceived purchase, and perceived value on purchase intention were all significant. Last, the direct relationship between single-channel shopping cost and purchase intention (β = 0.32) is significant when we added perceived value as a mediator. Last, the direct relationship between positive online reviews and purchase intention (β = 0.29) is significant when we added perceived value as a mediator. From the preceding results, we can deduce that perceived shopping partially mediates the relationship between single-channel shopping cost, positive online reviews and purchase intention.
To analyze the moderating effect of contextual factors, this paper constructs a moderating effect analysis model. The paper adopts model 14 of SPSS PROCESS v3.3 to analyze the mediating effect of contextual factors. The sample size is set to 5,000, the purchase intention is the dependent variable, the perceived value is the mediator variable, the Contextual factor is the moderator variable, and the purchase cost, reference groups, online comments, and perceived risk are The estimated results of independent variables and moderating effects are shown in Table 6.
The results show that contextual factors negatively moderate the mediation effect of single-channel shopping cost on purchase intention via perceived value [index of moderated mediation = −0.0244, SE = 0.0104, 95% CI = (−0.0442, −0.031); direct effect = 0.1464, SE = 0.0295, 95% CI = (0.0886, 0.2043)]. Contextual factors negatively moderate the mediation effect of reference groups on purchase intention via perceived value [index of moderated mediation = −0.0223, SE = 0.0102, 90% CI = (−0.0398, −0.0050); direct effect = 0.676, SE = 0.0288, 95% CI = (0.0200, 0.1150)]. Contextual factors negatively moderate the mediation effect of positive online reviews on purchase intention via perceived value [index of moderated mediation = −0.0165, SE = 0.0088, 90% CI = (−0.0344, −0.0001); direct effect = 0.1408, SE = 0.0255, 95% CI = (0.0907, 0.1908)]. Contextual factors negatively moderate the mediation effect of single-channel perceived risk on purchase intention via perceived value [index of moderated mediation = −0.0229, SE = 0.0107, 90% CI = (−0.0438, −0.0013); direct effect = −0.0008, SE = 0.0267, 95% CI = (−0.0532, 0.0515)].
This study yielded meaningful conclusions. As the results show, single-channel shopping costs have a significant positive effect on the perceived value of purchasing agricultural products omni-channel (β = 0.4, p < 0.001). Single-channel shopping cost has a significant positive effect on the purchase intention of purchasing agricultural products omni-channel (β = 0.26, p < 0.001). The results are consistent with previous research findings (Guo et al., 2022), indicating that shopping cost is the key factor of perceived value and purchase intention. reference groups has a positive impact on the perceived value (β = 0.09, p < 0.05). Positive online reviews have a positive impact on perceived value (β = 0.16, p < 0.001). Positive online reviews have a positive impact on perceived intention (β = 0.18, p < 0.001). The results show that compared with reference groups, positive online reviews play a more important role in consumers’ purchasing decision processes omni-channel. Single-channel perceived risk has a significant positive effect on the perceived value of purchasing agricultural products omni-channel (β = 0.24, p < 0.001).
Second, the study indicates the mediating effect of perceived value on purchase intention. In the model of “single-channel shopping cost→ perceived valueg willingness to buy agricultural products through dual-channel,” the mediating role of perceived value is significant, and the effect size is 0.3219. In the model of “reference groups→ perceived valueo willingness to buy agricultural products omni-channel,” perceived value played a significant mediating role, and the effect size was 0.3555. In the model of “positive online reviews→ perceived valueo willingness to buy agricultural products omni-channel,” perceived value played a significant mediating role, and the effect size was 0.2895. In the model of “single-channel perceived riskg perceived valuee willingness to buy agricultural products through dual-channel,” perceived value played a significant mediating role, and the effect size was 0.4210.
Finally, our results indicate that contextual factors negatively moderate the mediation effect of single-channel shopping cost on purchase intention via perceived value [index of moderated mediation = −0.0244, SE = 0.0104, 95% CI = (−0.0442, −0.031]; direct effect = 0.1464, SE = 0.0295, 95% CI = (0.0886, 0.2043)]. Contextual factors negatively moderate the mediation effect of reference groups on purchase intention via perceived value (index of moderated mediation = −0.0223, SE = 0.0102, 90% CI = (−0.0398, −0.0050); direct effect = 0.676, SE = 0.0288, 95% CI = (0.0200, 0.1150)]. Contextual factors negatively moderate the mediation effect of positive online reviews on purchase intention via perceived value [index of moderated mediation = −0.0165, SE = 0.0088, 90% CI = (−0.0344, −0.0001); direct effect = 0.1408, SE = 0.0255, 95% CI = (0.0907, 0.1908)]. Contextual factors negatively moderate the mediation effect of single-channel perceived risk on purchase intention via perceived value [index of moderated mediation = −0.0229, SE = 0.0107, 90% CI = (−0.0438, −0.0013); direct effect = −0.0008, SE = 0.0267, 95% CI = (−0.0532, 0.0515)].
Recent researchers have begun to emphasize the importance of understanding omni-channel development from a consumer perspective (Hossain et al., 2019; Shi et al., 2020; Guo et al., 2021). This study offers three important theoretical implications for fresh produce omni-channel technology. First, previous research has focused on studies concerning the drivers of search and purchase channels (Arora et al., 2017), rather than the costs and risks of the channel switching process. By combining the research frameworks of VAM and UTAUT, this study fully captures the key factors influencing consumers’ omni-channel purchase intentions. At the same time, it enriches theoretical insights on how consumers’ perceived value affects purchase intention.
More importantly, our study provides new insights into consumer perceived value in a contextually relevant paradigm. The findings confirm the role of positive online reviews and reference groups. Third, while many fresh agri-produce retailers have adopted an omni-channel retail strategy, it is unknown whether the strategy will help retailers drive a positive consumer response (Yan et al., 2020). Focusing on the lesser-focused fresh produce industry, this study seeks to provide theoretical support for these retailers’ strategies.
This study may provide several implications for practice. First, agricultural retailers should pay full attention to the role of single-channel shopping costs, which have the greatest impact on consumers’ willingness to purchase agricultural products omni-channel. On the one hand, agriculture retailers can continue to strengthen the construction of online websites to reduce the difficulty of consumers judging product information before purchasing. On the other hand, agricultural retailers should innovate planting technology, improve the quality of agricultural products, continuously reduce the purchase cost of consumers’ dual-channel purchase of agricultural products, and make full use of the impact of purchase costs to increase consumers’ willingness to purchase.
Second, agricultural retailers should pay more attention to social influence. Agricultural retailers should choose high-quality websites and anchors with good reputations to carry out online sales. High-quality online reviews should be selected, influential positive online reviewers should be actively discovered, and positive information about product differences and user experience should be promptly delivered to potential consumers. Agriculture retailers can also take corresponding selected comment incentive measures to encourage consumers to post positive online reviews, improve the quantity and quality of positive online reviews on agricultural products, further establish smooth communication channels among customers, and make full use of social influence.
Third, agriculture retailers should keep abreast of consumers’ single-channel perceived risk. Enterprises should pay attention to the investigation of the consumer market and strive to accurately grasp the source of consumers’ single-channel perceived risk. In addition, retailers should ensure consistency of information across channels to reduce uncertainty in omni-channel technology and shopping processes. For example, establish cross-channel feedback mechanisms to meet customer needs and improve competitive advantage.
Finally, the perceived value of consumers should be continuously improved. The process of consumer selection is essentially a process of seeking value. Retailers should enhance their fresh produce logistics and distribution systems to avoid problems such as untimely deliveries and product spoilage. Consumers can reap the same fresh produce whether in-store or at home, thus facilitating a seamless and consistent shopping experience.
Although the results of this study have provided some implications for agricultural product dual-channel development in China, there are still some limitations. Future research could take the following aspects into account.
Most of the research hypotheses and conceptual models in this paper are based on the general online shopping theory and are not very targeted. Future research could consider further modifying and improving the model from the characteristics of omni-channel shopping agricultural products and the characteristics of omni-channel consumers to improve the pertinence and explanatory power of the model in the agricultural product sales model.
In studying the factors of consumers’ channel choice, we compared the attributes of a single channel and omni-channel. This study focuses on the factors that have a significant effect on perceived value. Future research will focus on some other factors that affect consumer attitudes and perceptions of consumers’ willingness to choose channels. such as agricultural product types and green agricultural products.
The raw data supporting the conclusions of this article will be made available by the authors, without undue reservation.
YL designed the study, drafted the initial manuscript, and contributed to the revised manuscript. SZ collected the data, performed statistical analysis, and drafted the initial manuscript. Both authors discussed the results and contributed to the final manuscript.
This research was supported by the Soft Science Research Plan Foundation of Henan Province (Contract No. 212400410133) and the Philosophy and Social Science Foundation of Henan Province (Contract No. 2019CJJ086).
We thank the general editor, guest editors, and reviewers for their comments and helpful feedback.
The authors declare that the research was conducted in the absence of any commercial or financial relationships that could be construed as a potential conflict of interest.
All claims expressed in this article are solely those of the authors and do not necessarily represent those of their affiliated organizations, or those of the publisher, the editors and the reviewers. Any product that may be evaluated in this article, or claim that may be made by its manufacturer, is not guaranteed or endorsed by the publisher.
Abrudan, I. N., Dabija, D. C., and Grant, D. B. (2020). “Omni-channel retailing strategy and research agenda,” in Perspectives on consumer behaviour. Contributions to Management Science, ed. W. Sroka (Cham: Springer), 261–280. doi: 10.1007/978-3-030-47380-8_12
Ailawadi, K. L., and Farris, P. W. (2017). Managing multi- and omni-channel distribution: Metrics and research directions. J. Retail. 93, 120–135. doi: 10.1016/j.jretai.2016.12.003
Amaldoss, W., and Jain, S. (2005a). Conspicuous consumption and sophisticated thinking. Manage. Sci. 51, 1449–1466. doi: 10.1287/mnsc.1050.0399
Amaldoss, W., and Jain, S. (2005b). Pricing of conspicuous goods: A competitive analysis of social effects. J. Mark. Res. 42, 30–42. doi: 10.1509/jmkr.42.1.30.56883
Amaldoss, W., and Jain, S. (2015). Branding conspicuous goods: An analysis of the effects of social influence and competition. Manage. Sci. 61, 2064–2079. doi: 10.1287/mnsc.2014.2078
Arora, S., Singha, K., and Sahney, S. (2017). Understanding consumer’s showrooming behaviour: Extending the theory of planned behavior. Asia Pac. J. Mark. Logist. 29, 409–431. doi: 10.1108/APJML-06-2016-0111
Asmare, A., and Zewdie, S. (2022). Omnichannel retailing strategy: A systematic review. Int. Rev. Retail Distrib. Consum. Res. 32, 59–79. doi: 10.1080/09593969.2021.2024447
Babin, B. J., Darden, W. R., and Griffin, M. (1994). Work and/or fun: Measuring hedonic and utilitarian shopping value. J. Consum. Res. 20, 644–656. doi: 10.1086/209376
Bao, T., and Chang, T. L. S. (2014). Finding disseminators via electronic word of mouth message for effective marketing communications. Decis. Support Syst. 67, 21–29. doi: 10.1016/j.dss.2014.07.006
Bearden, W. O., and Etzel, M. J. (1982). Reference groups influence on product and brand purchase decisions. J. Consum. Res. 9, 183–194. doi: 10.1086/208911
Bearden, W. O., Netemeyer, R. G., and Teel, J. E. (1989). Measurement of consumer susceptibility to interpersonal influence. J. Consum. Res. 15, 473–481. doi: 10.1086/209186
Bennett, P. D., and Harrell, G. D. (1975). The role of confidence in understanding and predicting buyers’ attitudes and purchase intentions. J. Consum. Res. 2, 110–117. doi: 10.1086/208622
Chang, H. H., Wong, K. H., and Li, S. Y. (2017). Applying push-pull-mooring to investigate channel switching behaviors: M-shopping self-efficacy and switching costs as moderators. Electron. Commer. Res. Appl. 24, 50–67. doi: 10.1016/j.elerap.2017.06.002
Chang, T. Z., and Wildt, A. R. (1994). Price, product information, and purchase intention: An empirical study. J. Acad. Mark. Sci. 22, 16–27. doi: 10.1177/0092070394221002
Corbitt, B. J., Thanasankit, T., and Yi, H. (2003). Trust and e-commerce: A study of consumer perceptions. Electron. Commer. Res. Appl. 2, 203–215. doi: 10.1016/S1567-4223(03)00024-3
Cronin, J. J., Brady, M. K., and Hult, G. (2000). Assessing the effects of quality, value, and customer satisfaction on consumer behavioral intentions in service environments. J. Retail. 76, 193–218. doi: 10.1016/S0022-4359(00)00028-2
Daugherty, P. J., Bolumole, Y., and Grawe, S. J. (2019). The new age of customer impatience. Int. J. Phys. Distrib. Logist. Manage. 49, 4–32. doi: 10.1108/IJPDLM-03-2018-0143
Davis, F. D., Bagozzi, R. P., and Warshaw, P. R. (1989). User acceptance of computer technology: A comparison of two theoretical models. Manage. Sci. 35, 982–1003. doi: 10.1287/mnsc.35.8.982
Davis, F. D., Bagozzi, R. P., and Warshaw, P. R. (1992). Extrinsic and intrinsic motivation to use computers in the workplace. J. Appl. Soc. Psychol. 22, 1111–1132. doi: 10.1111/j.1559-1816.1992.tb00945.x
De, A., and Singh, S. P. (2021). A resilient pricing and service quality level decision for fresh agri-product supply chain in post-covid-19 era. Int. J. Logist. Manag. [Epub ahead of print] doi: 10.1108/IJLM-02-2021-0117
Dhar, R. (1992). “To choose or not to choose: This is the question,” in ACR North American Advances, Vol. 19, eds J. F. Sherry and Jr. B. Sternthal (Provo, UT: Association for Consumer Research), 735–738.
Digital News Asia (2019). Asian consumers have high expecataions for omni-channel shopping experiences. Available online at: https://www.digitalnewsasia.com/digital-economy/asian (accessed January 28, 2022).
Dimanche, F., and Havitz, M. E. (1995). Consumer behavior and tourism: Review and extension of four study areas. J. Travel Tour. Mark. 3, 37–57. doi: 10.1300/J073v03n03_03
Dodds William, B., Monroe, K. B., and Dhruv, G. (1991). The effects of price, brand and store ınformation on buyers’ product evaluations. J. Mark. Res. 28, 307–319. doi: 10.1177/002224379102800305
Dominici, A., Boncinelli, F., Gerini, F., and Marone, E. (2021). Determinants of online food purchasing: The impact of socio-demographic and Contextual factors. J. Retail. Consum. Serv. 60, 1–9. doi: 10.1016/j.jretconser.2021.102473
Escalas, J. E., and Bettman, J. R. (2005). Self-construal, Reference groups, and brand meaning.. J. Consum. Res. 32, 378–389. doi: 10.1086/497549
Fan, X., Zhang, J., and Zhu, G. (2020). Effects of consumers’ uncertain valuation-for-quality in a distribution channel. Ann. Oper. Res. 1–26. doi: 10.1007/s10479-020-03847-7
Fang, J., Liu, H., and Li, Y. (2021). Balance cues of online-offline channel integration: Considering the moderating role of customer’s showrooming motivation. Inf. Manage. 58:103535. doi: 10.1016/j.im.2021.103535
Feng, J. Y., Yuan, B. Y., Li, X., Tian, D., and Mu, W. (2021). Evaluation on risks of sustainable supply chain based on optimized BP neural networks in fresh grape industry. Comput. Electron. Agric. 183:105988. doi: 10.1016/j.compag.2021.105988
Forman, C., Ghose, A., and Wiesenfeld, B. (2008). Examining the relationship between reviews and sales: The role of reviewer identity disclosure in electronic markets. Inf. Syst. Res. 19, 291–313. doi: 10.1287/isre.1080.0193
Fornell, C., and Larcker, D. F. (1981). Evaluating structural equation models with unobservable variables and measurement error. J. Mark. Res. 18, 39–50. doi: 10.1177/002224378101800104
Grewal, D., Monroe, K. B., and Krishnan, R. (1998). The effects of price-comparison advertising on buyers’ perceptions of acquisition value, transaction value, and behavioral intentions. J. Mark. 62, 46–59. doi: 10.1177/002224299806200204
Guo, J., Hao, H., Wang, M., and Liu, Z. (2022). An empirical study on consumers’ willingness to buy agricultural products online and its influencing factors. J. Clean. Prod. 336:130403. doi: 10.1016/j.jclepro.2022.130403
Guo, Y., Zhang, M., and Lynette Wang, V. (2021). Webrooming or showrooming? The moderating effect of product attributes. J. Res. Interact. Mark. [Epub ahead of print]. doi: 10.1108/JRIM-08-2020-0161
Hair, J. F., Sarstedt, M., Hopkins, L., and Kuppelwieser, V. G. (2014). Partial least squares structural equation modeling (PLS-SEM): An emerging tool in business research. Eur. Bus. Rev. 26, 106–121. doi: 10.1108/EBR-10-2013-0128
Heng, Y., Gao, Z., Jiang, Y., and Chen, X. (2018). Exploring hidden factors behind online food shopping from amazon reviews: A topic mining approach. J. Retail. Consum. Serv. 42, 161–168. doi: 10.1016/j.jretconser.2018.02.006
Hennig-Thurau, T., Malthouse, E. C., Friege, C., Gensler, S., Lobschat, L., Rangaswamy, A., et al. (2010). The impact of new media on customer relationships. J. Serv. Res. 13, 311–330. doi: 10.1177/1094670510375460
Herrero-Crespo, A., Viejo-Fernández, N., Collado-Agudo, J., and Sanzo Pérez, M. J. (2022). Webrooming or showrooming, that is the question: Explaining omnichannel behavioural intention through the technology acceptance model and exploratory behaviour. J. Fashion Mark. Manage. 26, 401–419. doi: 10.1108/JFMM-05-2020-0091
Hossain, T. M. T., Akter, S., Kattiyapornpong, U., and Dwivedi, Y. K. (2019). Multichannel integration quality: A systematic review and agenda for future research. J. Retail. Consum. Serv. 49, 154–163. doi: 10.1016/j.jretconser.2019.03.019
Hu, Q., and Xu, B. (2019). Differential game analysis of optimal strategies and cooperation in omni-channel organic agricultural supply chain. Sustainability 11:848. doi: 10.3390/su11030848
Hua, Y., Cheng, X., Hou, T., and Luo, R. (2020). Monetary rewards, intrinsic motivators, and work engagement in the IT-enabled sharing economy: A mixed-methods investigation of internet taxi drivers. Decis. Sci. 51, 755–785. doi: 10.1111/deci.12372
Huré, E., Picot-Coupey, K., and Ackermann, C.-L. (2017). Understanding omni-channel shopping value: A mixed-method study. J. Retail. Consum. Serv. 39, 314–330. doi: 10.1016/j.jretconser.2017.08.011
Janjevic, M., Merchán, D., and Winkenbach, M. (2021). Designing multi-tier, multi-service-level, and multi-modal last-mile distribution networks for omni-channel operations. Eur. J. Oper. Res. 294, 1059–1077. doi: 10.1016/j.ejor.2020.08.043
Jepsen, A. L. (2007). Factors affecting consumer use of the internet for information search. J. Interact. Mark. 21, 21–34. doi: 10.1002/dir.20083
Jin, M., Li, G., and Cheng, T. C. E. (2018). Buy online and pick up in-store: Design of the service area. Eur. J. Oper. Res. 268, 613–623. doi: 10.1016/j.ejor.2018.02.002
Juaneda-Ayensa, E., Mosquera, A., and Sierra Murillo, Y. (2016). Omnichannel customer behavior: Key drivers of technology acceptance and use and their effects on purchase intention. Front. Psychol. 7:1117. doi: 10.3389/fpsyg.2016.01117
Kazancoglu, I., and Aydin, H. (2018). An investigation of consumers’ purchase intentions towards omni-channel shopping: A qualitative exploratory study. Int. J. Retail Distrib. Manage. 46, 959–976. doi: 10.1108/IJRDM-04-2018-0074
Kim, H. W., Chan, H. C., and Gupta, S. (2007). Value-based adoption of mobile internet: An empirical investigation. Decis. Support Syst. 43, 111–126. doi: 10.1016/j.dss.2005.05.009
Kim, S., Connerton, T. P., and Park, C. (2022). Transforming the automotive retail: Drivers for customers’ omnichannel bops (buy online & pick up in store) behavior. J. Bus. Res. 139, 411–425. doi: 10.1016/j.jbusres.2021.09.070
König, T. M., Hein, N., and Nimsgern, V. (2022). A value perspective on online review platforms: Profiling preference structures of online shops and traditional companies. J. Bus. Res. 145, 387–401. doi: 10.1016/j.jbusres.2022.02.080
Kwark, Y., Chen, J., and Raghunathan, S. (2014). Online product reviews: Implications for retailers and competing manufacturers. Inf. Syst. Res. 25, 93–110. doi: 10.1287/isre.2013.0511
Liang, P., Sima, M., Huang, Y., and Sun, X. Y. (2022). Assessing the coordinated revenue-sharing contract of china’s farmer-supermarket direct-purchase model. Int. Food Agribus. Manage. Rev. 25, 229–243. doi: 10.22434/IFAMR2020.0196
Lim, G. G., Kang, J. Y., Lee, J. K., and Lee, D. C. (2011). Rule-based personalized comparison shopping including delivery cost. Electron. Commer. Res. Appl. 10, 637–649. doi: 10.1016/j.elerap.2011.07.002
Mathavan, B., Vafaei-Zadeh, A., Hanifah, H., Ramayah, T., and Kurnia, S. (2022). Understanding the purchase intention of fitness wearables: Using value-based adoption model. Asia Pac. J. Bus. Adm. [Epub ahead of print]. doi: 10.1108/APJBA-04-2022-0166
Ming, L., Guo, H. Z., and Wei, W. (2021). Study of the game model of e-commerce information sharing in an agricultural product supply chain based on fuzzy big data and LSGDM. Technol. Forecast. Soc. Change 172:121017. doi: 10.1016/j.techfore.2021.121017
Morgenstern (2021). How brands can create winning omnichannel experiences. Available online at: https://www.mytotalretail.com/article/ (accessed January 28, 2022).
Murfield, M., Boone, C. A., Rutner, P., and Thomas, R. (2017). Investigating logistics service quality in omni-channel retailing. Int. J. Phys. Distrib. Logist. Manage. 47, 263–296.
Neal, W. D. (1999). Satisfaction is nice, but value drives loyalty. Mark. Res. 11, 20–23. doi: 10.1108/IJPDLM-06-2016-0161
Pallant, J. I., Sands, S. J., Ferraro, C. R., and Pallant, J. L. (2020). Self-selection and purchase value of research shoppers. Int. J. Retail Distrib. Manage. 48, 845–863. doi: 10.1108/IJRDM-12-2019-0387
Park, J., Lennon, S. J., and Stoel, L. (2005). On-line product presentation: Effects on mood, perceived risk, and purchase intention. Psychol. Mark. 22, 695–719. doi: 10.1002/mar.20080
Peterson, R. A., and Merino, M. C. (2003). Consumer information search behavior and the Internet. Psychol. Mark. 20, 99–121. doi: 10.1002/mar.10062
Petrick, J. F. (2002). Development of a multi-dimensional scale for measuring the perceived value of a service. J. Leis. Res. 34, 119–134. doi: 10.1080/00222216.2002.11949965
Pozzi, A. (2012). Shopping cost and brand exploration in online grocery. Am. Econ. J. Microecon. 4, 96–120.
Preacher, K. J., and Hayes, A. F. (2008). Asymptotic and resampling strategies for assessing and comparing indirect effects in multiple mediator models. Behav. Res. Methods 40, 879–891. doi: 10.1257/mic.4.3.96
Prelec, D., Wernerfelt, B., and Zettelmeyer, F. (1997). The role of inference in context effects: Inferring what you want from what is available. J. Consum. Res. 24, 118–125. doi: 10.3758/BRM.40.3.879
Richards, T. J., Hamilton, S. F., and Empen, J. (2017). Attribute search in online retailing. Am. J. Agric. Econ. 99, 225–242. doi: 10.1086/209498
Rigby, D. (2011). Marketing; the future of shopping. Harv. Bus. Rev. 89, 65–76. doi: 10.1093/ajae/aaw085
Saghiri, S., and Mirzabeiki, V. (2021). Omni-channel integration: The matter of information and digital technology. Int. J. Oper. Prod. Manage. 41, 1660–1710. doi: 10.1108/IJOPM-04-2021-0262
Shi, S., Wang, Y., Chen, X., and Zhang, Q. (2020). Conceptualization of omnichannel customer experience and its impact on shopping intention: A mixed-method approach. Int. J. Inf. Manage. 50, 325–336. doi: 10.1016/j.ijinfomgt.2019.09.001
Singh, S., and Jang, S. (2022). Search, purchase, and satisfaction in a multiple-channel environment: How have mobile devices changed consumer behaviors? J. Retail. Consum. Serv. 65, 1–11. doi: 10.1016/j.jretconser.2020.102200
Steenkamp, J. B. E. (1990). Conceptual model of the quality perception process. J. Bus. Res. 21, 309–333. doi: 10.1016/0148-2963(90)90019-A
Stein, A., and Ramaseshan, B. (2016). Towards the identification of customer experience touch point elements. J. Retail. Consum. Serv. 30, 8–19. doi: 10.1016/j.jretconser.2015.12.001
Stone, R. N., and Grønhaug, K. (1993). Perceived risk: Further considerations for the marketing discipline. Eur. J. Mark. 27, 39–50. doi: 10.1108/03090569310026637
Sun, J., Xie, J., Chen, T., Li, F., and Wang, G. (2022). Managing reference-group effects in sequential product upgrades. Prod. Oper. Manage. 31, 442–456. doi: 10.1111/poms.13552
Sweeney, J. C., and Soutar, G. N. (2001). Consumer perceived value: The development of a multiple item scale. J. Retail. 77, 203–220. doi: 10.1016/S0022-4359(01)00041-0
Tom, C., Edward, M., Ewa, M., Rebecca, W., Jung, K. S., and Yorgos, A. (2020). How online reviews influence sales: Evidence of the power of online reviews to shape customer behavior, Vol. 602082019. (Evanston, IL: Northwestern University’s spiegel research Center), 275–299.
Ueland, Ø., Gunnlaugsdottir, H., Holm, F., Kalogeras, N., Leino, O., Luteijn, J. M., et al. (2012). State of the art in benefit–risk analysis: Consumer perception. Food Chem. Toxicol. 50, 67–76. doi: 10.1016/j.fct.2011.06.006
Venkatesh, V., and Davis, F. D. (2000). A theoretical extension of the technology acceptance model: Four longitudinal field studies. Manage. Sci. 46, 186–204. doi: 10.1287/mnsc.46.2.186.11926
Venkatesh, V., Morris, M. G., Davis, G. B., and Davis, F. D. (2003). User acceptance of information technology: Toward a unified view. MIS Q. 27, 425–478. doi: 10.2307/30036540
Verhoef, P. C., Kannan, P. K., and Inman, J. J. (2015). From multi-channel retailing to Omni-channel retailing: Introduction to the special issue on multi-channel retailing. J. Retail. 91, 174–181. doi: 10.1016/j.jretai.2015.02.005
Vijayasarathy, L. R., and Jones, J. M. (2000). Print and Internet catalog shopping: assessing attitudes and intentions. Internet Res. 10, 191–202. doi: 10.1108/10662240010331948
Vishwakarma, P., Mukherjee, S., and Datta, B. (2020). Travelers’ intention to adopt virtual reality: A consumer value perspective. J. Dest. Mark. Manage. 17:100456. doi: 10.1016/j.jdmm.2020.100456
Voss, G., Parasuraman, A., and Grewal, D. (1998). The role of price and quality perceptions in prepurchase and postpurchase evaluation of services. J. Mark. 62, 46–61. doi: 10.1177/002224299806200404
Wang, X., Wong, Y. D., Shi, W., and Yuen, K. F. (2022). Shoppers’ logistics activities in omni-channel retailing: A conceptualisation and an exploration on perceptual differences in effort valuation. Transp. Policy 115, 195–208. doi: 10.1016/j.tranpol.2021.11.014
Welsch, H., and Kühling, J. (2009). Determinants of pro-environmental consumption: The role of Reference groups and routine behavior. Ecol. Econ. 69, 166–176. doi: 10.1016/j.ecolecon.2009.08.009
Wollenburg, J., Holzapfel, A., Hübner, A., and Kuhn, H. (2018). Configuring retail fulfillment processes for omni-channel customer steering. Int. J. Electron. Commer. 22, 540–575. doi: 10.1080/10864415.2018.1485085
Wood, C. M., and Scheer, L. K. (1996). Incorporating perceived risk into models of consumer deal assessment and purchase intent. Adv. Consum. Res. 23, 399–404.
Wu, J. H., and Wang, S. C. (2005). What drives mobile commerce?: An empirical evaluation of the revised technology acceptance model. Inf. Manage. 42, 719–729. doi: 10.1016/j.im.2004.07.001
Yan, B., Chen, X., Cai, C., and Guan, S. (2020). Supply chain coordination of fresh agricultural products based on consumer behavior. Comput. Oper. Res. 123:105038. doi: 10.1016/j.cor.2020.105038
Yu, J., Gan, M., Ni, S., and Chen, D. (2018). Multi-objective models and real case study for dual-channel fap supply chain network design with fuzzy information. J. Intell. Manuf. 29, 389–403. doi: 10.1007/s10845-015-1115-8
Yu, Y., and Xiao, T. (2017). Pricing and cold-chain service level decisions in a fresh agri-products supply chain with logistics outsourcing. Comput. Ind. Eng. 111, 56–66. doi: 10.1016/j.cie.2017.07.001
Zatz, L. Y., Moran, A. J., Franckle, R. L., Block, J. P., Hou, T., Blue, D., et al. (2021). Comparing online and in-store grocery purchases. J. Nutr. Educ. Behav. 53, 471–479. doi: 10.1016/j.jneb.2021.03.001
Zeithaml, V. A. (1988). Consumer perceptions of price, quality, and value: A means-end model and synthesis of evidence. J. Mark. 52, 2–22. doi: 10.1177/002224298805200302
Zepeda, L., and Deal, D. (2010). Organic and local food consumer behaviour: Alphabet theory. Int. J. Consum. Stud. 33, 697–705. doi: 10.1111/j.1470-6431.2009.00814.x
Zhang, H., Xu, H., and Pu, X. (2020). Comparisons of pre-sale strategies for a fresh agri-product supply chain with service effort. Agriculture 10:324. doi: 10.3390/agriculture10080324
Zhang, L., Wu, L., Huang, L., and Zhang, Y. (2021). Wield the power of omni-channel retailing strategy: a capability and supply chain resilience perspective. J. Strateg. Mark. 1–25. doi: 10.1080/0965254X.2021.1972440
Zheng, Q., Chen, J., Zhang, R., and Wang, H. H. (2020). What factors affect Chinese consumers’ online grocery shopping? Product attributes, e-vendor characteristics and consumer perceptions. China Agric. Econ. Rev. 12, 193–213. doi: 10.1108/CAER-09-2018-0201
Zhou, J.-H., Han, F., Li, K., and Wang, Y. (2020). Vegetable production under covid-19 pandemic in china: An analysis based on the data of 526 households. J. Integr. Agric. 19, 2854–2865. doi: 10.1016/S2095-3119(20)63366-4
Zhu, Q., Li, Y., Geng, Y., and Qi, Y. (2013). Green food consumption intention, behaviors and influencing factors among Chinese consumers. Food Qual. Prefer. 28, 279–286. doi: 10.1016/j.foodqual.2012.10.005
Keywords: COVID-19 pandemic, purchase intention, omni-channel, structural equation model, value-based adoption model
Citation: Liu Y and Zheng S (2023) Factors affecting consumers’ purchase intention for agriculture products omni-channel. Front. Psychol. 13:948982. doi: 10.3389/fpsyg.2022.948982
Received: 20 May 2022; Accepted: 30 August 2022;
Published: 03 January 2023.
Edited by:
Nikunja Mohan Modak, University of Kalyani, IndiaReviewed by:
Khairul Anuar Mohammad Shah, Universiti Sains Malaysia (USM), MalaysiaCopyright © 2023 Liu and Zheng. This is an open-access article distributed under the terms of the Creative Commons Attribution License (CC BY). The use, distribution or reproduction in other forums is permitted, provided the original author(s) and the copyright owner(s) are credited and that the original publication in this journal is cited, in accordance with accepted academic practice. No use, distribution or reproduction is permitted which does not comply with these terms.
*Correspondence: Shuyin Zheng, c2h1eWluemhlbmcyN0BvdXRsb29rLmNvbQ==
Disclaimer: All claims expressed in this article are solely those of the authors and do not necessarily represent those of their affiliated organizations, or those of the publisher, the editors and the reviewers. Any product that may be evaluated in this article or claim that may be made by its manufacturer is not guaranteed or endorsed by the publisher.
Research integrity at Frontiers
Learn more about the work of our research integrity team to safeguard the quality of each article we publish.