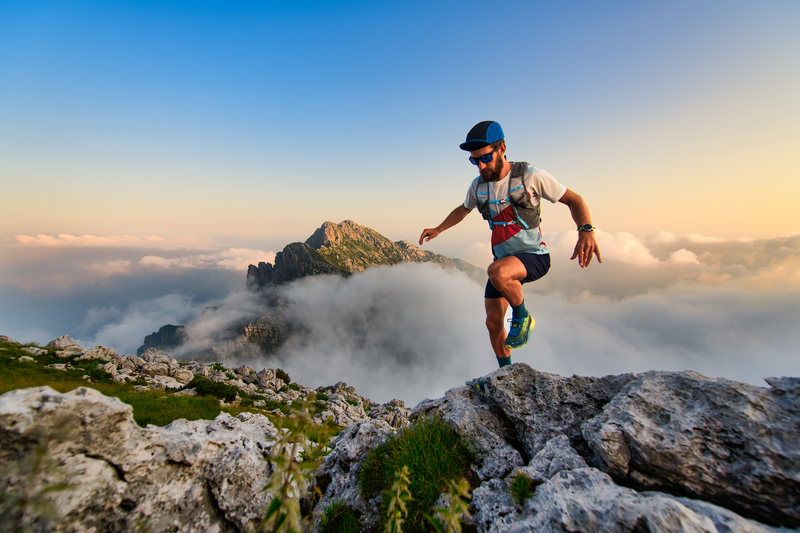
94% of researchers rate our articles as excellent or good
Learn more about the work of our research integrity team to safeguard the quality of each article we publish.
Find out more
SYSTEMATIC REVIEW article
Front. Psychol. , 16 September 2022
Sec. Psychology of Aging
Volume 13 - 2022 | https://doi.org/10.3389/fpsyg.2022.940978
This article is part of the Research Topic Late-Life Psychopathology View all 13 articles
Objective: The presence of two or more chronic diseases results in worse clinical outcomes than expected by a simple combination of diseases. This synergistic effect is expected to be higher when combined with some conditions, depending on the number and severity of diseases. Multimorbidity is a relatively new term, with the first fundamental definitions appearing in 2015. Studies usually define it as the presence of at least two chronic medical illnesses. However, little is known regarding the relationship between mental disorders and other non-psychiatric chronic diseases. This review aims at investigating the association between some mental disorders and non-psychiatric diseases, and their pattern of association.
Methods: We performed a systematic approach to selecting papers that studied relationships between chronic conditions that included one mental disorder from 2015 to 2021. These were processed using Covidence, including quality assessment.
Results: This resulted in the inclusion of 26 papers in this study. It was found that there are strong associations between depression, psychosis, and multimorbidity, but recent studies that evaluated patterns of association of diseases (usually using clustering methods) had heterogeneous results. Quality assessment of the papers generally revealed low quality among the included studies.
Conclusions: There is evidence of an association between depressive disorders, anxiety disorders, and psychosis with multimorbidity. Studies that tried to examine the patterns of association between diseases did not find stable results.
Systematic review registration: https://www.crd.york.ac.uk/prospero/display_record.php?ID=CRD42021216101, identifier: CRD42021216101.
The management of chronic diseases is one of the main challenges faced by health care systems today. Chronic conditions, when they happen together, seem to interact and worsen each other's clinical courses (Mendenhall et al., 2017). This effect is expected to be higher with some combinations of conditions more than others, and with number and severity of diseases (Mendenhall, 2017). However, most research done in the relations between chronic conditions consider them in pairs, within a hierarchy (co-morbid). This paradigm is aligned to the way the biomedical model organizes training of health providing professionals, with each medical specialist within the boundaries of their own field (World Health Organization, 2016). This introduces an arbitrary division and ignores patterns of association that may be clinically relevant and could help reduce morbidity if early addressed by practitioners (MacMahon, 2018). On the other hand, the multimorbidity (MM) concept tries to shift toward a system where there are no index conditions, and the subject of interest is rather the interaction between diseases.
This shift toward the MM concept has implications in several areas. It requires that specialists interact and communicate efficiently with staff from other background training (Sinyor et al., 2019). This type of more integrative care is costly (Valderas et al., 2009), may require reorganizing mental health and general practices (Sinyor et al., 2019) to a more holistic care (arguably simplified in places where a universal health system exists, such as in UK), and impacts research itself, as traditional association statistical methods are better suited for comparisons between two variables.
Most of the studies consider MM as the presence of two or more disorders. MacMahon (2018) declared MM a health priority, as its prevalence is increasing in many regions of the world over the past 20 years. The prevalence is also expected to grow with the increase in life expectancy (MacMahon, 2018). Patients affected by MM have more disability, tend to use more medications (van Oostrom et al., 2014), have worse quality of life (Ralph et al., 2013), more cognitive impairment (Koyanagi et al., 2018; Wang et al., 2020), and may die earlier than non-affected peers (Wei and Mukamal, 2018). Multimorbidity is also linked to increased use and costs of health services (van Oostrom et al., 2014).
The first two major documents on this subject are a summary of a roundtable meeting by The Academy of Medical Sciences (2015) and a report by World Health Organization (2016). This was followed by the Lancet series on Syndemics (Mendenhall et al., 2017), the creation of the Medical Subject Heading (MeSH) term for multimorbidity in National Library of Medicine (2018), the multimorbidity report by the American Academy of Medical Sciences the same year (MacMahon, 2018), and the Lancet Psychiatry Commission Blueprint for protecting the physical health of people with mental illness in 2019 (Firth et al., 2019). This impacts the slow transition of the literature into methods that more accurately assess the interactions between chronic diseases and development, or use of more suited methods to this end (like clustering methods). Furthermore, mental disorders in the context of multimorbidity have been less studied, as clinical conditions have become the main subjects of the field.
The complex relations of mental disorders and chronic diseases is not entirely understood. It is certainly expected to happen, as mental disorders are linked to worse adherence to clinical treatments (Patel and Chatterji, 2015), to lack of physical activity (Bueno-Antequera and Munguía-Izquierdo, 2020), and antipsychotics are linked to metabolic syndromes (Hálfdánarson et al., 2017). The hypothesized mechanisms through which mental disorders may be associated with chronic diseases include: (A) the metabolic syndrome resulting from psychiatric medication; (B) direct inflammatory process from harmful drug/alcohol/tobacco use; (C) childhood adversities as environmental factors for both non-communicable diseases and mental disorders (Patel and Chatterji, 2015); and (D) inflammatory mechanisms such as higher concentration levels of C-reactive protein, interleukin 6 and 12 and tumor necrosis factor in acute depression (Osimo et al., 2020). Additionally, individuals with mental disorders usually make poor lifestyle choices (with less exercises, unhealthy eating habits, tobacco use) and have less access to prevention policies and health care (Patel and Chatterji, 2015). So far studies have found high prevalence of MM with mental disorders among young individuals from deprived backgrounds compared to those resident of more affluent areas (Barnett et al., 2012), and higher rates of multimorbidity with mental disorders in women (Barnett et al., 2012). The majority of studies on MM are concentrated on older individuals from high income countries (MacMahon, 2018). More studies on younger individuals, from more deprived backgrounds are necessary to understand the association between socioeconomic drivers and MM with mental disorders. Previous systematic reviews found higher rates of MM and specific psychiatric disorders, like depression (Read et al., 2017) and schizophrenia (Rodrigues et al., 2021). However, evidence is still fragmented, lacks investigation of trends over time, and rarely uses methods that can estimate the effect of mechanisms.
This review aims at investigating the association between five prevalent mental disorders and non-psychiatric diseases (including the chronic infectious diseases: tuberculosis, and HIV), and the pattern of association between them. It also will organize information in a descriptive synthesis including studies that had multimorbidity as a focus from the start, avoiding those exclusively linked to co-morbidity.
We reported this systematic review according to the PRISMA 2020 guideline (Page et al., 2021). The protocol of this review was registered at PROSPERO (registration number CRD42021216101). The search strategy included MeSH terms with the final expression: (multimorbidity[MeSH Terms]) AND (mental disorder[MeSH Terms] OR anxiety[MeSH Terms] OR depressive disorder[MeSH Terms] OR psychotic disorders[MeSH Terms] OR substance related disorders[MeSH Terms] AND (“2015/01/01”[Date - Entry]: “3000”[Date - Entry])). We searched PubMed and Scielo databases on 31/05/2021 for original articles published in the last 6 years (2015–2021) that studied multimorbidity and at least one of the mental disorders we listed above. We chose studies published from 2015 because that was the year that the Medical Science Academy held the meeting, which led to the “Multimorbidity: a priority for global health research” report in 2018. We also reviewed references from relevant prior systematic reviews, in order to identify any other eligible studies. We selected original studies published in English, Portuguese or Spanish. Furthermore, we report results in a descriptive synthesis, including a quality assessment of the studies.
Any quantitative article that analyzed the association between five psychiatric disorders (depression, anxiety, post-traumatic stress disorder-PTSD, substance use disorder and psychosis) and chronic physical conditions (infectious or non-communicable) were included. In addition to this, studies analyzing multimorbidity patterns that included mental disorders were also added. We excluded gray literature, studies focused on statistical models with no mention of multimorbidity patterns or association and studies that examined prevalence only. Therefore, the minimal definition of MM for this review was the presence of one chronic condition plus one of the five psychiatric disorders listed above.
We performed screening of title and abstract, full text review and data extraction with the Covidence web tool (Veritas Health Innovation, 2021). Two reviewers (NTSF and FC) independently screened titles and abstracts. We discussed the disagreements to reach a consensus. The full text screening followed the same approach. Reasons for exclusion were: wrong outcome (studies reporting only prevalence) or wrong study design (modeling study).
Two reviewers (NTSF and FC) independently extracted data from included studies, with a third reviewer (JAPA) establishing comparison and consensus. We created a data extraction form including information on study characteristics, participant characteristics, setting, statistical method, outcomes, main conclusions and limitations.
We assessed the quality of each included article using the Newcastle-Ottawa Scale (NOS) (Wells et al., 2000) for observational studies. For cohort studies, we used the thresholds for converting the Newcastle-Ottawa scales to Agency for Health Research and Quality (AHRQ) standards: good, fair, and poor quality. We did not assign scores for cross-sectional studies. For these studies, we adapted the scale and included bias listed by the study authors. We did not evaluate the quality of systematic reviews and meta-analysis.
We tabulated our findings as a narrative synthesis, describing the main characteristics of the studies and critical findings. Furthermore, we focused on the association between multimorbidity and psychiatric disorders by using crude and/or adjusted relative risk (RR), risk difference (RD) or odds ratio reported by the studies.
We screened 205 titles and abstracts for eligibility. After reviewing the full text of 56 articles, we included 26 studies in our review (Figure 1). Six articles (23%) were multi-country studies; five (19%) from the USA, two (8%) from China, South Africa and Spain. One (4%) from each Bahrain, Brazil, Canada, Croatia, UK, Italy, Netherlands, Pakistan, and Switzerland. The papers included discussed the following psychiatric conditions: depression, anxiety, bipolar disorder, dysthymia, adjustment and personality disorder, psychosis, schizophrenia, post-traumatic stress disorder (PTSD), dementia, eating disorder dissociative and somatoform disorders, intellectual disabilities, impulse-control, premenstrual dysphoria, insomnia, alcohol abuse, tobacco and substance use disorders. The most common chronic conditions were cardiovascular, respiratory and renal diseases, diabetes, hepatitis and obesity (Table 1). Table 2 summarizes the main findings for the association between multimorbidity and psychiatric disorders. We provided a narrative synthesis below according to specific psychiatric disorders.
Figure 1. PRISMA flow diagram. From: Page et al. (2021).
Most articles discussing multimorbidity and mental disorders were cross-sectional studies (20.76%). Two studies included were systematic reviews and meta-analysis (Read et al., 2017; Rodrigues et al., 2021). Most of the papers were multi-country studies (23%). The single country with more studies was the USA (19%). The study with the largest sample had 3,759,836 individuals (Lenzi et al., 2016) and the smaller had 240 individuals (Jahrami et al., 2017). Fifteen percent of the studies included exclusively older adults (50 years and above). Depression was the psychiatric disorder most evaluated in the reviewed studies (42%), followed by anxiety (23%), and substance abuse (19%). Only four studies addressed multimorbidity among individuals with psychosis. Samples were very heterogeneous and comprised specific groups (veterans, people living with HIV, general population of a region, patients of a psychiatric hospital, patients of primary care units, patients of tuberculosis clinic, etc.). Most study samples came from high income countries (European countries: Italy, Netherlands, Spain, Croatia and Switzerland).
The shift from co-morbidity to the multimorbidity framework resulted in more papers being published aiming at detecting patterns of association between conditions. In particular, it seems that studies that grouped diseases together were the most common. There are a number of these methods and the ones recovered in our systematic review included factor analysis and latent class analysis (Lenzi et al., 2016; Filipčić et al., 2018; Aubert et al., 2019), but there also were papers using non-supervised clustering methods (Violan et al., 2018). In this section, we present the clusters or groupings found through these methods. Only Lenzi et al. (2016) presented ad hoc tests between groups and age, and Filipčić et al. (2018) performed tests between classes and an outcome. Peltzer (2018) identified three patterns of multimorbidity through a factor analysis where the third factor (eigenvalue = 1.10), named substance use disorders, had high loading on daily or almost daily tobacco use (0.81) and alcohol-use disorders (0.81). Aubert et al. (2019) identified five groups as patterns of multimorbidity, Group 5 (“psychiatric diseases”) included psychiatric and neurological disorders, along with chronic heart disease, COPD and bronchiectasis, and arthropathy and arthritis, corresponding to 23% of patients.
Wang et al. (2019) reported patterns of multimorbidity using principal component analysis (PCA) as a clustering method. They used data from a local survey in a large city of Brazil, including 2,713 subjects. The clusters of chronic conditions were distinct among women and men. Among women, the first component was labeled “irritable mood and headache” and encompassed premenstrual dysphoric, mood, anxiety, impulse-control disorders, and headache/migraine. The second component was “chronic diseases and pain” and included hypertension, cardiovascular illnesses, arthritis, diabetes, and musculoskeletal pain. The third component, “substance use,” included heavy drinking and substance use disorders (SUD). For men, the first component was labeled “chronic pain and respiratory disease,” and included headache/migraine, musculoskeletal pain, arthritis, respiratory, and digestive illnesses. The second component was named “psychiatric disorders” and included impulse-control, mood, anxiety disorders, SUD, and heavy drinking. Finally, the third component described a dimension of “chronic diseases” that contained hypertension, cardiovascular illnesses, and diabetes. Of note is their finding that age was not significantly related to the “irritable mood and headache cluster” in women and to the “chronic pain and respiratory disease” cluster in men, whereas age was significantly associated to all other clusters. Furthermore, they report area level (complementing the individual level findings just described) effect sizes and significance for some variables, notably the area violence was significantly associated with the “chronic diseases” cluster in women and with the “chronic pain” cluster in men.
Violan et al. (2018) also defined patterns of multimorbidity by sex using Multiple Correspondence Analysis (MCA) and K-means clustering. In the first cluster, in women had an exclusivity value (fraction of patients with the disease included in the cluster over the total strata of patients with the disease) of 46.1% and included mental and behavioral disorders due to psychoactive substance use (tobacco); in men, the first cluster had an exclusivity value of 35.3% and included metabolic disorders. It also found that the main cluster, for men and women, included the following chronic diseases: metabolic disorders, hypertensive diseases, mental and behavioral disorders due to psychoactive substance use, other dorsopathies and other soft tissue disorders.
Lenzi et al. (2016) using exploratory factor analysis identified 5 patterns of multimorbidity: (1) psychiatric disorders, (2) cardiovascular, renal, pulmonary and cerebrovascular diseases, (3) neurological diseases, (4) liver diseases, AIDS/HIV and substance abuse and (5) tumors. As for the association between factor scores and demographic characteristics, we found that the only correlation coefficient close to 0.30 was that between multimorbidity pattern 2 and age (Spearman's ρ = 0.27, p < 0.001), indicating that the presence of cardiovascular, renal, pulmonary and/or cerebrovascular diseases was more common among older than younger age groups (Spearman's ρ = 0.27, p < 0.001), indicating that the presence of cardiovascular, renal, pulmonary and/or cerebrovascular diseases was more common among older than younger age groups.
Filipčić et al. (2018) report results from self-reports of presence of chronic physical conditions. The authors performed a latent class analysis (LCA) to identify grouping patterns in their data and found that psychiatric patients had 27% higher age-standardized relative risk for chronic physical illness. The LCA identified four groups, labeled as follows: “Relatively healthy,” “Musculoskeletal,” “Hypertension and obesity,” and “Complex multimorbidity.” But the authors report no significant differences in multimorbidity patterns.
These findings will be discussed in more length below. However, it was remarkable how varied results were regarding the clusters recovered. For example, arthritis and other painful conditions have been reported to co-occur with depressive disorders (Miguel et al., 2012), but the algorithms used only grouped arthritis with mental disorders in one (Wang et al., 2019) of the studies. Similarly, only one study grouped mental disorders with metabolic syndrome (Violan et al., 2018), which is one association expected to be found widely due to mechanisms of metabolic alterations via psychiatric medication use. It is important to notice that the list of diseases that were actually included in any of the above papers differed between them, this further hinder comparisons between the clusters reported in these works.
Seven studies evaluated MM in patients with depression. The studies covered the risk of depression in patients with chronic diseases in general (Li et al., 2015; Holvast et al., 2017); the association between depression and HIV, hypertension and diabetes (Petersen et al., 2019) or obesity (Romain et al., 2019) the risk of depression according to the number of chronic diseases (Arokiasamy et al., 2015) the risk of multimorbidity according to the severity of depression (Stubbs et al., 2017) and the risk of depression in patients with and without multimorbidity (Read et al., 2017).
A systematic review and meta-analysis found that individuals with multimorbidity had more than twice the risk (RR 2.13 95% CI: 1.62 to 2.80; p < 0.001) of having a depressive disorder compared to individuals without multimorbidity (Read et al., 2017). In our review, we found two studies investigating the association between depressive symptoms and chronic diseases among people 60 years or older. In China, a cross-sectional study showed significant association (OR = 1.379 [95% CI: 1.291 to 1.473]) (Li et al., 2014) and in the Netherlands, they found a significant association in a primary care sample (OR = 1.55 [95% CI: 1.33 to 1.81]) (Holvast et al., 2017).
We also found one study investigating the association of depression with a chronic infectious disease. In South Africa, HIV was associated with depression (OR = 2.08 [95% CI: 1.53 to 2.83]) while patients with hypertension and diabetes were less likely to have depression (OR = 0.45 [95% CI: 0.23 to 0.89]) (Petersen et al., 2019). In Canada, individuals with physical multimorbidity had higher odds of major depressive episode (OR = 5.16 [95% CI 2.92 to 9.13]) (Romain et al., 2019). Arokiasamy et al. (2015) performed a multi-country study in the adult population (most aged 50 years or older) showing that the odds of depression increased significantly according to the number of chronic diseases (adjusted OR increased from 1.62 [95% CI: 1.42 to 1.84] for one disease to 7.33 [95% CI: 6.24 to 8.61] for four or more diseases) (Arokiasamy et al., 2015). Another multi-country study found that the severity of depression influenced the odds of multimorbidity. Compared with patients with no depression, the odds of multimorbidity was 2.62 times higher (95% CI: 2.17 to 3.15; p < 0.0001) for patients with subsyndromal depression and 3.44 times higher (95% CI: 3.12 to 3.79; p < 0.0001) for patients with depressive episode (Stubbs et al., 2017).
Four studies addressed anxiety and multimorbidity with three of them evaluating the risk of anxiety according to the number of chronic diseases (Vancampfort et al., 2017; Romain et al., 2019; Wong et al., 2020). A fourth study assessed the risk of anxiety in individuals with obesity. In the USA, anxiety was associated with an increased number of medical diseases in adults aged 65 or older (two medical conditions, adjusted OR = 1.79 [95% CI: 1.02 to 3.12]; three or more medical diseases, adjusted OR = 3.04 [95% CI: 1.77 to 5.21]; all p < 0.001) (Gould et al., 2016). Vancampfort et al. (2017) performed a multi-country cross-sectional study with findings indicating that individuals with five or more physical diseases had higher odds of anxiety (adjusted OR 9.66 [95% CI: 6.88 to 13.57]; p < 0.001) compared to those with one physical condition (adjusted OR 2.08 [95% CI: 1.90 to 2.27]; p < 0.001) (Vancampfort et al., 2017). In China, a study conducted during COVID-19 outbreak found no significant association between generalized anxiety disorder and having more than four chronic diseases (reference group: 2 to 4 chronic conditions) (Wong et al., 2020). In Canada, individuals with obesity and physical multimorbidity were more likely to report anxiety disorders (OR = 2.46 [95% CI 1.46 to 4.16]) (Romain et al., 2019).
Two studies looked at the association of anxiety and/or depression with multimorbidity. In Pakistan (Farooq et al., 2019), found that the presence of multimorbidity (hypertension, obesity, dyslipidaemia, diabetes, heart diseases, stroke, migraines, asthma and chronic obstructive pulmonary disease [COPD], anemia, thyroid disease, diseases of bones and joints, dyspepsia/peptic ulcer, hepatitis B or C, chronic kidney diseases including stones, cancer, and/or disability increased 33% the odds of having anxiety and depressive symptoms (adjusted OR 1.33 [95% CI: 1.11 to 1.58]). Additionally, adults with more than five chronic diseases had increased odds of these symptoms (adjusted OR 2.62 [95% CI: 1.66 to 4.13]) when compared with those with one chronic disease (adjusted OR 0.98 [95% CI: 0.69 to 1.14]) (Farooq et al., 2019). In the USA, DiNapoli et al. (2017) evaluated predictors of having depressive and/or anxiety disorder among middle-aged and older veterans. The study also found significant increased odds of depressive and/or anxiety disorder according to the number of chronic diseases (3–5 chronic diseases: OR = 1.70 [95%CI: 1.21 to 2.40]; 10–13 chronic diseases: OR = 6.62 [95%CI: 4.73 to 9.25]) (DiNapoli et al., 2017).
Four studies addressed the association between psychosis and multimorbidity. In the Basque Country (Spain), Gabilondo et al. (2017) analyzed a data set of 2,255,406 individuals covered by public health insurance. Of these, 7,331 (0.3%) had a diagnosis of schizophrenia. Compared with individuals without diagnosis of schizophrenia, individuals with schizophrenia had 1.64 odds (95% CI: 1.56 to 1.73; p < 0.001) of having one chronic physical illness. Similar odds were found for two (OR 1.63 [95% CI: 1.53 to 1.75], p < 0.001; three or more (OR 1.12 [95% CI: 1.05 to 1.19], p < 0.001). In addition, the authors found that patients with schizophrenia were more likely to have a diagnosis of hepatitis, HIV, diabetes and any pulmonary disease (Gabilondo et al., 2017). In Bahrain, a case control study compared patients with schizophrenia with those with no serious mental illness. Compared with controls, cases were significantly more likely to have physical comorbidities, but the odds ratio did not vary according to the number of diseases (one disease OR 3.7 [95%CI: 2.0 to 6.9]; two diseases OR 3.3 [95% CI: 1.8 to 6.0]; three or more diseases OR 3.2 [95%CI: 1.4 to 7.7]). In addition, cases were more likely to have obesity, type 2 diabetes, hypertension, cardiovascular disease and musculoskeletal disorders (Jahrami et al., 2017). In a multi-country study, Stubbs et al. (2016) evaluated the risk of multimorbidity according to the severity of psychosis. The findings indicated that patients with a diagnosis of psychosis had higher odds of multimorbidity (4.05 [95% CI: 3.25 to 5.04], p < 0.001) compared to those with subclinical psychosis (OR 2.20 [95% CI: 2.02 to 2.39], p < 0.001) (Stubbs et al., 2016). In a systematic review and meta-analysis, Rodrigues 2021 found that individuals with psychotic disorder had 1.69 increased risk (RR 1.69 [95% CI: 1.37 to 2.08]) to have multimorbidity, compared with individuals without psychotic disorder (Rodrigues et al., 2021).
Two studies assessed the association between PTSD and multimorbidity (Jacob et al., 2018; Peltzer, 2018). In a study conducted in the UK, Jacob et al. (2018) found that PTSD had adjusted odds of 2.47 (95% CI: 1.71 to 3.56, p < 0.001) to be associated with physical multimorbidity (cancer, diabetes, epilepsy, migraine, cataracts/eyesight problems, ear/hearing problems, stroke, heart attack/angina, high blood pressure, bronchitis/emphysema, asthma, allergies, stomach ulcer or other digestive problems, liver problems, bowel/colon problems, bladder problems/incontinence, arthritis, bone/back/joint/muscle problems, infectious disease, and skin problems). The number of PTSD symptoms was also associated with increasing odds of multimorbidity (OR 1.14 [95%CI = 1.09–1.20], p < 0.001). The authors found that anxiety, depression, and eating disorders were mediators in the association between PTSD and physical multimorbidity (Jacob et al., 2018). In South Africa, a study among patients with tuberculosis found that PTSD was significantly associated with multimorbidity. The association did not change substantially according to the number of non-communicable diseases (one non-communicable disease, adjusted OR 1.42 [95% CI: 1.18 to 1.69]; two non-communicable diseases, adjusted OR 1.79 (1.44 to 2.22); three or more non-communicable diseases, adjusted OR 1.77 [95% CI: 1.30 to 2.41]) (Peltzer, 2018).
Four studies (MacLean et al., 2018; Crawford and Thornton, 2019; Han et al., 2019; Petersen et al., 2019) addressed the association between substance use disorders and multimorbidity. One study included alcohol use disorder (AUD), one was on alcohol and tobacco use disorder (TUD), one on AUD and drug use disorder, and one on drug use disorder (e.g., cannabis, cocaine, methamphetamine, heroin, inhalants, hallucinogens, and prescription drug misuse).
In chronic care patients in South Africa, patients with HIV had twice the odds of having AUD (OR = 2.19 [95% CI: 1.38 to 3.48], p < 0.001) compared with those with no HIV (Petersen et al., 2019). MacLean 2018 et al. performed a comparative cohort study of veterans who had received a diagnostic of AUD or TUD in 2012 in the USA. Compared to patient with AUD only, veterans with concurrent conditions had significantly higher odds of hepatic disease (OR 1.14 [95% CI: 1.11 to 1.17]), diabetes mellitus (OR 0.60 [95% CI: 0.59 to 0.61]) and renal disease (OR 0.76 [95% CI: 0.73 to 0.79]). Compared to veterans with TUD only, those with AUD and TUD had significantly higher odds of hepatic disease (OR 2.18 [95% CI: 2.13 to 2.2]), diabetes mellitus (OR 0.81 [95% CI: 0.80 to 0.83]) and renal disease (OR 0.71 [95% CI: 0.68 to 0.74]) (MacLean et al., 2018). In the USA, Han et al. (2019) analyzed the data of a national survey on drug use. Past-year substance use was reported by 16% of adults with one chronic disease and by 13% of adults with two or more chronic diseases. The correlates of past-year substance use among adults with chronic conditions showed an adjusted odds ratio of 1.16 (95% CI: 0.97, 1.39, p = 0.11) among adults with 3–4 chronic diseases and 0.67 (95% CI: 0.39, 1.15, p = 0.15) among adults with more than five conditions. Results showed lower substance use in individuals with multimorbidity (Han et al., 2019). Another study in the USA among individuals living with HIV found that current alcohol users had a significantly increased risk of multimorbidity compared to never drinkers (adjusted IRR: 1.70 [95% CI: 1.35 to 2.14], p < 0.05). The model was adjusted for age, drug use, smoking status, insurance status, HIV duration, cd4 + cell counts, and log viral load. The unadjusted model showed that drug use was also associated with multimorbidity (IRR: 1.22 [95% CI: 1.01 to 1.47], p < 0.05) (Crawford and Thornton, 2019).
We assessed the quality of three cohorts studies and one case control study. One cohort study obtained fair quality and the remainder were evaluated as poor quality. All cohort studies limited the study population to a selected group of users. The common bias of poor-quality studies were no statement on the comparability of cohorts and adequacy of follow-up. The case-control study was classified as fair quality.
Regarding the cross-sectional studies (20), exposure was assessed through structured interview (55%) or secure record (30%). Outcome was assessed through self-report (65%) or record linkage (35%). The main limitations listed by the authors were cross-sectional study design, self-report data, number, severity and duration of comorbidities included, and other risk factors not included. Details can be found in Table 1.
This systematic review included 26 articles that were published since 2015, the year major publications on the definition of multimorbidity first appeared. Our aim was to frame the relationship between mental disorders and chronic diseases within the multimorbidity framework, in other words, a non-hierarchical framework with multidirectional associations. Such complex structure is harder to fully detect in research and what we found is that we are in a transitional phase where studies are still being designed in a one-to-one type of associative inference, and the studies that ultimately evaluated patterns/groups/clusters reported so distinct groupings that resulted in a very heterogeneous research landscape.
The definition of multimorbidity is still not agreed among researchers. Most studies use a definition based on the number of disorders (2 or more) but some studies use different values (4 or 5 disorders). Even when authors use the same number, there is a problem concerning what diseases are studied. Most studies use a list of conditions, which varies in length and content. Some studies only include chronic conditions, others include acute disorders. The severity of the condition is also not always included. It should be noted, however, that the heterogeneity in our findings can be explained by the broad variety of study types included.
From the small number of studies including mental disorders, the majority (42%) focused on only one disorder (depression). Half the studies only included older individuals (above 50 years), leaving out the young subjects, which are of concern due to high incidence of mental disorders (Ralph et al., 2013). This is surprising given this is a known and important issue acknowledged by the Academy of Medical Sciences in 2015 and the Lancet Psychiatry Commission Blueprint in 2019 (Firth et al., 2019).
The results of this review suggest a strong relationship between depression and multimorbidity. Individuals with multimorbidity had higher odds of having depression or depressive symptoms, with OR ranging between 1.379 (Li et al., 2014) and 1.62 (Arokiasamy et al., 2015). Also, in a systematic review and meta-analysis on multimorbidity and depression, Read et al. (2017) found a risk two times higher of depressive episode in multimorbid patients. The inverse relation was also found, with a multi-country study finding that having depression (subsyndromal or episodic) influenced the odds of having multimorbidity (Stubbs et al., 2017). Although most of these studies were concentrated in samples of older (more than 60 years of age) individuals, they included samples from diverse countries with different income ranges and ethnic groups (Table 1). There is a disproportion of studies concentrated in depression compared to other mental health conditions. Although depression is a highly prevalent disorder (Smith, 2014), there is high comorbidity with other mental health disorders with probable consequences on prevalence of chronic conditions (Steel et al., 2014).
Studies in anxiety disorders found inconsistent results. Whereas, two studies found association between number of chronic conditions and anxiety, with OR that ranged between 1.79 (Gould et al., 2016) and 9.66 (Vancampfort et al., 2017) for more than 5 chronic diseases, another study from China, during the COVID pandemic, found no significant relation between generalized anxiety disorder and more than four chronic diseases (Wong et al., 2020). Two studies focused on anxiety and depression, and both found higher odds of anxiety and depressive symptoms with more chronic diseases (33% in Pakistan and OR of 1.7 in the USA for 3 to 5 chronic conditions) (DiNapoli et al., 2017; Farooq et al., 2019). These studies included younger adults and older individuals.
Individuals with schizophrenia have higher odds of presenting a chronic disease (Gabilondo et al., 2017; Jahrami et al., 2017). Having psychosis increased the chances of having multimorbidity compared to subclinical psychosis (Stubbs et al., 2016). Rodrigues et al. (2021) meta-analysis found a 1.69 risk of multimorbidity among people with psychosis. Studies accounted for different groups of chronic conditions, but diabetes was present in two of them (Gabilondo et al., 2017; Jahrami et al., 2017). These findings are aligned with the large literature showing higher mortality in those with schizophrenia (Walker et al., 2015; Ko et al., 2018; Richmond-Rakerd et al., 2021), which was also observed during the COVID pandemic (Karaoulanis and Christodoulou, 2021).
Both studies with PTSD showed high OR for multimorbidity (Jacob et al., 2018; Peltzer, 2018). The number of PTSD symptoms was related to higher odds of having multimorbidity (Jacob et al., 2018). PTSD was the mental disorder that had fewer studies in this review, its lifetime prevalence in upper-middle income and lower-middle income countries is 2.3 and 2.1 percent, respectively, according to the WHO (Koenen et al., 2017). A previous study reported 17% frequency of comorbidity between PTSD and other coexisting psychiatric disorders, with worse occupational problems, disability and poorer social support among these patients (Solomon and Davidson, 1996). For this reason, the small number of studies evaluating this condition was striking.
Substance use was the subject of four studies. Patients living with HIV had two times the odds of alcohol use disorder (Peterson et al., 2020). In the USA, a study comparing patients with alcohol use disorder and tobacco use disorder showed that the use of both was related with higher odds of having diabetes, renal disease and hepatic disease (MacLean et al., 2018). Koenen et al. (2017) reported that 13% of adults with 2 or more chronic diseases had reported substance use in the last year (Han et al., 2019). Their study found lower rates of substance use among individuals with multimorbidity. This might happen because chronic diseases are more common among older people who are less likely to use substances, and because these individuals stop using substances when they realize they are sick. People in the US living with HIV and current use of alcohol had higher risk of multimorbidity compared to non-drinkers (Crawford and Thornton, 2019). There are not enough studies about the association of substance use and MM. This is particularly important in MM in younger individuals. There is evidence that middle age (40–59) adults with multimorbidity are 1.71 times more likely to be current smokers than non-smokers (Taylor et al., 2010). Tobacco use is related to increased risk for depression, suggesting possible synergistic relation.
This paper should be interpreted in context with its limitations. Comparisons between studies might be hindered due to differences (1) in statistical methods used, (2) in monitored conditions included in each paper, (3) in measuring the conditions, (4) in study type, and (5) due to the broad target population used in the review. Heterogeneity can be in part due to these reasons. This review, as all systematic reviews, only accessed articles published within a specific time frame defined by the authors. Our work is the first to focus on a stricter definition of MM. Previous reviews included primary studies that used MM as a synonym to co-morbidity. Those studies were designed with a notion of hierarchy between disorders that is not compatible with the current MM definition (MacMahon, 2018).
Medicine is organized in specialities that often do not interact seamlessly. Multimorbidity will present a challenge to health service organizations, as it will require better integration of primary care. Furthermore, it will also require changes in how studies are performed. The present review revealed that one approach researchers are taking is to use clustering methodologies. These methods are identifying clinically significant groups, and the non-supervised clustering methods among these have the advantage of becoming starting points to medical automation tools, because of their predictive nature. These algorithms are largely dependent on what conditions are included in the analysis, therefore some kind of standardization will be required in the future, so we can achieve more comparable results. Because of this, we think this field would benefit from the creation of an instrumental scale of diseases/disorders that accounts for number, severity, and weights for clusters that offer higher risk of morbidity/death in multimorbid individuals.
The original contributions presented in the study are included in the article/Supplementary material, further inquiries can be directed to the corresponding author/s.
Material preparation, data collection, and analysis were performed by LC-d-A, FC, NTSF, and ER. The first draft of the manuscript was written by JA, LC-d-A, FC, NTSF, ER, and DM. Contributed reviewing and editing the final version by GL, SD, and MB. All authors contributed to the study conception and design, commented on previous versions of the manuscript, read, and approved the final manuscript.
LC-d-A, ER, MB, and DM were funded by the Medical Research Council - UK, Grant No. MR/T03355X/1 during the study development and by the National Institute of Health no. R01MH128911-01 in the later stages of work.
GL acknowledges support from the UCLH BRC.
The authors declare that the research was conducted in the absence of any commercial or financial relationships that could be construed as a potential conflict of interest.
All claims expressed in this article are solely those of the authors and do not necessarily represent those of their affiliated organizations, or those of the publisher, the editors and the reviewers. Any product that may be evaluated in this article, or claim that may be made by its manufacturer, is not guaranteed or endorsed by the publisher.
The Supplementary Material for this article can be found online at: https://www.frontiersin.org/articles/10.3389/fpsyg.2022.940978/full#supplementary-material
Arokiasamy, P., Uttamacharya, U., Jain, K., Biritwum, R. B., Yawson, A. E., Wu, F., et al. (2015). The impact of multimorbidity on adult physical and mental health in low- and middle-income countries: what does the study on global ageing and adult health (SAGE) reveal? BMC Med. 13:178. doi: 10.1186/s12916-015-0402-8
Aubert, C. E., Fankhauser, N., Marques-Vidal, P., Stirnemann, J., Aujesky, D., Limacher, A., et al. (2019). Patterns of multimorbidity in internal medicine patients in Swiss university hospitals: a multicentre cohort study. Swiss Med. Wkly. 149:w20094. doi: 10.4414/smw.2019.20094
Barnett, K., Mercer, S. W., Norbury, M., Watt, G., Wyke, S., and Guthrie, B. (2012). Epidemiology of multimorbidity and implications for health care, research, and medical education: a cross-sectional study. Lancet 380, 37–43. doi: 10.1016/S0140-6736(12)60240-2
Bueno-Antequera, J., and Munguía-Izquierdo, D. (2020). Exercise and Schizophrenia. Adv. Exp. Med. Biol. 1228, 317–332. doi: 10.1007/978-981-15-1792-1_21
Crawford, T. N., and Thornton, A. C. (2019). Alcohol use and multimorbidity among individuals living with HIV. AIDS Behav. 23, 152–160. doi: 10.1007/s10461-018-2242-y
DiNapoli, E. A., Bramoweth, A. D., Whiteman, K. L., Hanusa, B. H., and Kasckow, J. (2017). Mood disorders in middle-aged and older veterans with multimorbidity. J. Aging Health 29, 657–668. doi: 10.1177/0898264316641082
Farooq, S., Khan, T., Zaheer, S., and Shafique, K. (2019). Prevalence of anxiety and depressive symptoms and their association with multimorbidity and demographic factors: a community-based, cross-sectional survey in Karachi, Pakistan. BMJ Open 9:e029315. doi: 10.1136/bmjopen-2019-029315
Filipčić, I., Šimunovi ć Filipčić, I., Grošić, V., Bakija, I., Šago, D., Benjak, T., et al. (2018). Patterns of chronic physical multimorbidity in psychiatric and general population. J. Psychosom. Res. 114, 72–80. doi: 10.1016/j.jpsychores.2018.09.011
Firth, J., Siddiqi, N., Koyanagi, A., Siskind, D., Rosenbaum, S., Galletly, C., et al. (2019). The Lancet Psychiatry Commission: a blueprint for protecting physical health in people with mental illness. Lancet Psychiatry 6, 675–712. doi: 10.1016/S2215-0366(19)30132-4
Gabilondo, A., Alonso-Moran, E., Nuño-Solinis, R., Orueta, J. F., and Iruin, A. (2017). Comorbidities with chronic physical conditions and gender profiles of illness in schizophrenia. Results from PREST, a new health dataset. J. Psychosom. Res. 93, 102–109. doi: 10.1016/j.jpsychores.2016.12.011
Gould, C. E., O'Hara, R., Goldstein, M. K., and Beaudreau, S. A. (2016). Multimorbidity is associated with anxiety in older adults in the Health and Retirement Study. Int. J. Geriatr. Psychiatry 31, 1105–1115. doi: 10.1002/gps.4532
Hálfdánarson, Ó., Zoëga, H., Aagaard, L., Bernardo, M., Brandt, L., Fusté, A. C., et al. (2017). International trends in antipsychotic use: a study in 16 countries, 2005–2014. Eur. Neuropsychopharmacol. 27, 1064–1076. doi: 10.1016/j.euroneuro.2017.07.001
Han, B. H., Ko, R., and Palamar, J. J. (2019). Substance use by adults with medical multimorbidity in the United States, 2015-2016. J. Gen. Intern. Med. 34, 1394–1396. doi: 10.1007/s11606-019-04980-1
Holvast, F., van Hattem, B. A., Sinnige, J., Schellevis, F., Taxis, K., Burger, H., et al. (2017). Late-life depression and the association with multimorbidity and polypharmacy: a cross-sectional study. Fam. Pract. 34, 539–545. doi: 10.1093/fampra/cmx018
Jacob, L., Haro, J. M., and Koyanagi, A. (2018). Post-traumatic stress symptoms are associated with physical multimorbidity: findings from the Adult Psychiatric Morbidity Survey 2007. J. Affect. Disord. 232, 385–392. doi: 10.1016/j.jad.2018.02.063
Jahrami, H. A., Faris, M. A.-I. E., Saif, Z. Q., and Hammad, L. H. (2017). Assessing dietary and lifestyle risk factors and their associations with disease comorbidities among patients with schizophrenia: a case-control study from Bahrain. Asian J. Psychiatry 28, 115–123. doi: 10.1016/j.ajp.2017.03.036
Karaoulanis, S. E., and Christodoulou, N. G. (2021). Do patients with schizophrenia have higher infection and mortality rates due to COVID-19? A systematic review. Psychiatr. Psychiatr. 32, 219–223. doi: 10.22365/jpsych.2021.027
Ko, Y. S., Tsai, H.-C., Chi, M. H., Su, C.-C., Lee, I. H., Chen, P. S., et al. (2018). Higher mortality and years of potential life lost of suicide in patients with schizophrenia. Psychiatry Res. 270, 531–537. doi: 10.1016/j.psychres.2018.09.038
Koenen, K. C., Ratanatharathorn, A., Ng, L., McLaughlin, K. A., Bromet, E. J., Stein, D. J., et al. (2017). Posttraumatic stress disorder in the World Mental Health Surveys. Psychol. Med. 47, 2260–2274. doi: 10.1017/S0033291717000708
Koyanagi, A., Lara, E., Stubbs, B., Carvalho, A. F., Oh, H., Stickley, A., et al. (2018). Chronic physical conditions, multimorbidity, and mild cognitive impairment in low- and middle-income countries. J. Am. Geriatr. Soc. 66, 721–727. doi: 10.1111/jgs.15288
Lenzi, J., Avaldi, V. M., Rucci, P., Pieri, G., and Fantini, M. P. (2016). Burden of multimorbidity in relation to age, gender and immigrant status: a cross-sectional study based on administrative data. BMJ Open 6:e012812. doi: 10.1136/bmjopen-2016-012812
Li, L. W., Liu, J., Zhang, Z., and Xu, H. (2014). Late-life depression in Rural China: do village infrastructure and availability of community resources matter? Int. J. Geriatr. Psychiatry 30, 729–736. doi: 10.1002/gps.4217
Li, L. W., Liu, J., Zhang, Z., and Xu, H. (2015). Late-life depression in Rural China: do village infrastructure and availability of community resources matter?: Late-life depression in Rural China. Int. J. Geriatr. Psychiatry 30, 729–736.
MacLean, R. R., Sofuoglu, M., and Rosenheck, R. (2018). Tobacco and alcohol use disorders: evaluating multimorbidity. Addict. Behav. 78, 59–66. doi: 10.1016/j.addbeh.2017.11.006
MacMahon, S. (2018). Multimorbidity: A Priority for Global Health. London: The Academy of Medical Sciences.
Mendenhall, E. (2017). Syndemics: a new path for global health research. Lancet 389, 889–891. doi: 10.1016/S0140-6736(17)30602-5
Mendenhall, E., Kohrt, B. A., Norris, S. A., Ndetei, D., and Prabhakaran, D. (2017). Non-communicable disease syndemics: poverty, depression, and diabetes among low-income populations. Lancet 389, 951–963. doi: 10.1016/S0140-6736(17)30402-6
Miguel, R., de, C. C., Dias, R. C., Dias, J. M. D., Silva, S. L. A. da, Menicucci Filho, P. R., and Ribeiro, T. M. S. (2012). Síndrome da fragilidade no idoso comunitário com osteoartrite. Rev. Bras. Reumatol. 52, 339–347. doi: 10.1590/S0482-50042012000300004
National Library of Medicine (2018). Multimorbidity - MeSH Descriptor Data. MeSH Brows. Available online at: https://meshb.nlm.nih.gov/record/ui?ui=D000076322 (accessed January 17, 2022).
Osimo, E. F., Pillinger, T., Rodriguez, I. M., Khandaker, G. M., Pariante, C. M., and Howes, O. D. (2020). Inflammatory markers in depression: a meta-analysis of mean differences and variability in 5,166 patients and 5,083 controls. Brain. Behav. Immun. 87, 901–909. doi: 10.1016/j.bbi.2020.02.010
Page, M. J., McKenzie, J. E., and Bossuyt, P. M. (2021). The PRISMA 2020 statement: an updated guideline for reporting systematic reviews. Syst. Rev. 10:89. doi: 10.1186/s13643-021-01626-4
Patel, V., and Chatterji, S. (2015). Integrating mental health in care for noncommunicable diseases: an imperative for person-centered care. Health Aff. Proj. Hope 34, 1498–1505. doi: 10.1377/hlthaff.2015.0791
Peltzer, K. (2018). Tuberculosis non-communicable disease comorbidity and multimorbidity in public primary care patients in South Africa. Afr. J. Prim. Health Care Fam. Med. 10, e1–e6. doi: 10.4102/phcfm.v10i1.1651
Petersen, I., Rathod, S., Kathree, T., Selohilwe, O., and Bhana, A. (2019). Risk correlates for physical-mental multimorbidities in South Africa: a cross-sectional study. Epidemiol. Psychiatr. Sci. 28, 418–426. doi: 10.1017/S2045796017000737
Peterson, M. D., Kamdar, N., Chiodo, A., and Tate, D. G. (2020). Psychological morbidity and chronic disease among adults with traumatic spinal cord injuries: a longitudinal cohort study of privately insured beneficiaries. Mayo Clin. Proc. 95, 920–928. doi: 10.1016/j.mayocp.2019.11.029
Ralph, N. L., Mielenz, T. J., Parton, H., Flatley, A.-M., and Thorpe, L. E. (2013). Multiple chronic conditions and limitations in activities of daily living in a community-based sample of older adults in New York City, 2009. Prev. Chronic. Dis. 10:130159. doi: 10.5888/pcd10.130159
Read, J. R., Sharpe, L., Modini, M., and Dear, B. F. (2017). Multimorbidity and depression: a systematic review and meta-analysis. J. Affect. Disord. 221, 36–46. doi: 10.1016/j.jad.2017.06.009
Richmond-Rakerd, L. S., D'Souza, S., Milne, B. J., Caspi, A., and Moffitt, T. E. (2021). Longitudinal associations of mental disorders with physical diseases and mortality among 2.3 million New Zealand citizens. JAMA Netw. Open 4:e2033448. doi: 10.1001/jamanetworkopen.2020.33448
Rodrigues, M., Wiener, J. C., Stranges, S., Ryan, B. L., and Anderson, K. K. (2021). The risk of physical multimorbidity in people with psychotic disorders: a systematic review and meta-analysis. J. Psychosom. Res. 140, 110315. doi: 10.1016/j.jpsychores.2020.110315
Romain, A. J., Marleau, J., and Baillot, A. (2019). Association between physical multimorbidity, body mass index and mental health/disorders in a representative sample of people with obesity. J. Epidemiol. Community Health 73, 874–880. doi: 10.1136/jech-2018-211497
Sinyor, M., Goldstein, B. I., and Schaffer, A. (2019). Bridging the mental-physical divide in health care. CMAJ Can. Med. Assoc. J. J. Assoc. Medicale Can. 191, E722–E723. doi: 10.1503/cmaj.190709
Solomon, S. D., and Davidson, J. R. T. (1996). Trauma: prevalence, impairment, service use, and cost. J Clin Psychiatry 58:7.
Steel, Z., Marnane, C., Iranpour, C., Chey, T., Jackson, J. W., Patel, V., et al. (2014). The global prevalence of common mental disorders: a systematic review and meta-analysis 1980–2013. Int. J. Epidemiol. 43, 476–493. doi: 10.1093/ije/dyu038
Stubbs, B., Koyanagi, A., Veronese, N., Vancampfort, D., Solmi, M., Gaughran, F., et al. (2016). Physical multimorbidity and psychosis: comprehensive cross sectional analysis including 242,952 people across 48 low- and middle-income countries. BMC Med. 14:189. doi: 10.1186/s12916-016-0734-z
Stubbs, B., Vancampfort, D., Veronese, N., Kahl, K. G., Mitchell, A. J., Lin, P.-Y., et al. (2017). Depression and physical health multimorbidity: primary data and country-wide meta-analysis of population data from 190 593 people across 43 low- and middle-income countries. Psychol. Med. 47, 2107–2117. doi: 10.1017/S0033291717000551
Taylor, A. W., Price, K., Gill, T. K., Adams, R., Pilkington, R., Carrangis, N., et al. (2010). Multimorbidity - not just an older person's issue. Results from an Australian biomedical study. BMC Public Health 10:718. doi: 10.1186/1471-2458-10-718
The Academy of Medical Sciences (2015). Multiple Morbidities as a Global Health Challenge. Academy of Medical Sciences. Available online at: https://acmedsci.ac.uk/file-download/38330-567965102e84a.pdf (accessed January 4, 2022).
Valderas, J. M., Starfield, B., Sibbald, B., Salisbury, C., and Roland, M. (2009). Defining comorbidity: implications for understanding health and health services. Ann. Fam. Med. 7, 357–363. doi: 10.1370/afm.983
van Oostrom, S. H., Picavet, H. S. J., de Bruin, S. R., Stirbu, I., Korevaar, J. C., Schellevis, F. G., et al. (2014). Multimorbidity of chronic diseases and health care utilization in general practice. BMC Fam. Pract. 15:61. doi: 10.1186/1471-2296-15-61
Vancampfort, D., Koyanagi, A., Hallgren, M., Probst, M., and Stubbs, B. (2017). The relationship between chronic physical conditions, multimorbidity and anxiety in the general population: a global perspective across 42 countries. Gen. Hosp. Psychiatry 45, 1–6. doi: 10.1016/j.genhosppsych.2016.11.002
Veritas Health Innovation (2021). Covidence Systematic Review Software. Available online at: www.covidence.org.
Violan, C., Roso-Llorach, A., Foguet-Boreu, Q., Guisado-Clavero, M., Pons-Vigués, M., Pujol-Ribera, E., et al. (2018). Multimorbidity patterns with K-means nonhierarchical cluster analysis. BMC Fam. Pract. 19:108. doi: 10.1186/s12875-018-0790-x
Walker, E. R., McGee, R. E., and Druss, B. G. (2015). Mortality in mental disorders and global disease burden implications: a systematic review and meta-analysis. JAMA Psychiatry 72, 334–341. doi: 10.1001/jamapsychiatry.2014.2502
Wang, Y., Di, Y., Ye, J., and Wei, W. (2020). Study on the public psychological states and its related factors during the outbreak of coronavirus disease 2019 (COVID-19) in some regions of China. Psychol. Health Med. 26, 13–22. doi: 10.1080/13548506.2020.1746817
Wang, Y.-P., Nunes, B. P., Coêlho, B. M., Santana, G. L., do Nascimento, C. F., Viana, M. C., et al. (2019). Multilevel analysis of the patterns of physical-mental multimorbidity in general population of São Paulo Metropolitan area, Brazil. Sci. Rep. 9:2390. doi: 10.1038/s41598-019-39326-8
Wei, M. Y., and Mukamal, K. J. (2018). Multimorbidity, mortality, and long-term physical functioning in 3 prospective cohorts of community-dwelling adults. Am. J. Epidemiol. 187, 103–112. doi: 10.1093/aje/kwx198
Wells, G., Shea, B., O'Connell, D., Peterson, J., Welch, V., Losos, M., et al. (2000). The Newcastle-Ottawa Scale (NOS) for Assessing the Quality of Nonrandomised Studies in Meta-Analyses. Available online at: https://www.ohri.ca/programs/clinical_epidemiology/oxford.asp
Wong, S. Y. S., Zhang, D., Sit, R. W. S., Yip, B. H. K., Chung, R. Y.-N., Wong, C. K. M., et al. (2020). Impact of COVID-19 on loneliness, mental health, and health service utilisation: a prospective cohort study of older adults with multimorbidity in primary care. Br. J. Gen. Pract. J. R. Coll. Gen. Pract. 70, e817–e824. doi: 10.3399/bjgp20X713021
World Health Organization (2016). Multimorbidity: Technical Series on Safer Primary Care. Geneva: World Health Organization. Available online at: https://www.who.int/publications/i/item/9789241511650 (accessed February 22, 2022).
Keywords: multimorbidity, psychiatric disorders, clustering, aging, patterns
Citation: Castro-de-Araujo LFS, Cortes F, de Siqueira Filha NT, Rodrigues EdS, Machado DB, Araujo JAPd, Lewis G, Denaxas S and Barreto ML (2022) Patterns of multimorbidity and some psychiatric disorders: A systematic review of the literature. Front. Psychol. 13:940978. doi: 10.3389/fpsyg.2022.940978
Received: 10 May 2022; Accepted: 26 July 2022;
Published: 16 September 2022.
Edited by:
Mithat Durak, Abant Izzet Baysal University, TurkeyReviewed by:
Žarko Bajć, Psychiatric Hospital Sveti Ivan, CroatiaCopyright © 2022 Castro-de-Araujo, Cortes, de Siqueira Filha, Rodrigues, Machado, Araujo, Lewis, Denaxas and Barreto. This is an open-access article distributed under the terms of the Creative Commons Attribution License (CC BY). The use, distribution or reproduction in other forums is permitted, provided the original author(s) and the copyright owner(s) are credited and that the original publication in this journal is cited, in accordance with accepted academic practice. No use, distribution or reproduction is permitted which does not comply with these terms.
*Correspondence: Jacyra Azevedo Paiva de Araujo, amFjeXJhLnBhaXZhQGZpb2NydXouY29t
Disclaimer: All claims expressed in this article are solely those of the authors and do not necessarily represent those of their affiliated organizations, or those of the publisher, the editors and the reviewers. Any product that may be evaluated in this article or claim that may be made by its manufacturer is not guaranteed or endorsed by the publisher.
Research integrity at Frontiers
Learn more about the work of our research integrity team to safeguard the quality of each article we publish.