Corrigendum: Bias in measurement of autism symptoms by spoken language level and non-verbal mental age in minimally verbal children with neurodevelopmental disorders
- 1Department of Psychiatry and Behavioral Sciences, Weill Institute for Neurosciences, University of California, San Francisco, San Francisco, CA, United States
- 2Feinberg School of Medicine, Northwestern University, Chicago, IL, United States
- 3Neurodevelopmental and Behavioral Phenotyping Service, National Institute of Mental Health, Bethesda, MD, United States
- 4Department of Pediatrics, University of Minnesota, Minneapolis, MN, United States
- 5Masonic Institute for the Developing Brain, University of Minnesota, Minneapolis, MN, United States
- 6Center for Autism and the Developing Brain, Weill Cornell Medical College, White Plains, NY, United States
- 7Offord Centre for Child Studies, McMaster University, Hamilton, ON, Canada
- 8UCLA Semel Institute for Neuroscience & Human Behavior, Center for Autism Research and Treatment, David Geffen School of Medicine, University of California, Los Angeles, Los Angeles, CA, United States
- 9Thompson Center for Autism and Neurodevelopmental Disorders, University of Missouri, Columbia, MO, United States
- 10Department of Health Psychology, University of Missouri, Columbia, MO, United States
- 11Department of Psychology, University of South Carolina, Columbia, SC, United States
Increasing numbers of children with known genetic conditions and/or intellectual disability are referred for evaluation of autism spectrum disorder (ASD), highlighting the need to refine autism symptom measures to facilitate differential diagnoses in children with cognitive and language impairments. Previous studies have reported decreased specificity of ASD screening and diagnostic measures in children with intellectual disability. However, little is known about how cognitive and language abilities impact the measurement of specific ASD symptoms in this group. We aggregated a large sample of young children (N = 1196; aged 31–119 months) to examine measurement invariance of ASD symptoms among minimally verbal children within the context of the Autism Diagnostic Observation Schedule (ADOS) Module 1. Using confirmatory factor analysis (CFA) and moderated non-linear factor analysis (MNLFA), we examined how discrete behaviors were differentially associated with the latent symptom domains of social communication impairments (SCI) and restricted and repetitive behaviors (RRB) across spoken language levels and non-verbal mental age groupings. While the two-factor structure of SCI and RRB held consistently across language and cognitive levels, only partial invariance was observed for both ASD symptom domains of SCI and RRB. Specifically, four out of the 15 SCI items and one out of the three RRB items examined showed differential item functioning between children with “Few to No Words” and those with “Some Words”; and one SCI item and one RRB item showed differential item functioning across non-verbal mental age groups. Moreover, even after adjusting for the differential item functioning to reduce measurement bias across groups, there were still differences in ASD symptom domain scores across spoken language levels. These findings further underscore the influence of spoken language level on measurement of ASD symptoms and the importance of measuring ASD symptoms within refined spoken language levels, even among those with minimal verbal abilities.
Introduction
Evidence of social communication impairments (SCI) and restricted and repetitive behaviors (RRB) is required for a diagnosis of autism spectrum disorder (ASD) (American Psychiatric Association., 2013). However, symptoms in these two domains occur commonly in children with a range of other neurodevelopmental disorders (NDDs), greatly complicating differential diagnosis (Grzadzinski et al., 2011; Hepburn and Moody, 2011; Bishop et al., 2019; Lord and Bishop, 2021). Differential diagnosis of ASD is especially challenging in the context of intellectual disability (ID) (Moss and Howlin, 2009; Thurm et al., 2019). By definition, children with ID exhibit delays in social communication relative to same-aged peers (American Psychiatric Association., 2013), and they often present with RRBs (Evans and Gray, 2000; Moss et al., 2009; Burbidge et al., 2010; Wolff et al., 2012; Hoch et al., 2016). Not surprisingly, therefore, children with lower IQ/mental age often receive elevated scores on ASD symptom measures, regardless of whether they ultimately receive a clinical diagnosis of ASD (Havdahl et al., 2016).
Decreased specificity (i.e., higher false positive rate) of commonly used diagnostic instruments such as the Autism Diagnostic Interview-Revised (ADI-R) (Lord et al., 1994; Rutter et al., 2005) and Autism Diagnostic Observation Schedule (ADOS)/ADOS-2 (Lord et al., 2000, 2012) is particularly pronounced among children with very low mental ages and/or non-verbal IQ below 50 (Risi et al., 2006). Thus, the authors have cautioned against interpreting scores in children with non-verbal mental ages below 15–18 months for the ADOS and below 24 months for the ADI-R (Lord et al., 1994, 2012). Nevertheless, these measures are still widely applied in clinical and research samples of children with very low levels of language and cognitive abilities. Especially as DSM-5 now explicitly allows for the diagnosis of ASD with a range of other conditions, a growing number of children with known genetic diagnoses, many of whom have severe to profound intellectual disability (ID), are being referred for assessment of ASD (Hepburn and Moody, 2011; King et al., 2014; Richards et al., 2015; Abbeduto et al., 2019).
Understanding how cognitive and/or language ability affects the measurement of ASD symptoms has implications for clinical practice and research involving children with ASD, other NDDs, and/or genetic conditions (Thurm et al., 2019). Inaccurate diagnosis may lead to delayed or inappropriate clinical services, and in the research context, presents a serious threat to the validity of ASD case vs. control studies. Further, if measures systematically provide higher or lower symptom scores for individuals with certain characteristics (regardless of ASD status), the score differences will fail to represent true differences in abilities/impairments across groups. Numerous studies have established that both language and cognitive ability influence the manifestation of ASD-related symptoms, which in turn may affect accuracy of classifications yielded by ASD symptom measures in certain groups (Risi et al., 2006; Corsello et al., 2007; Gotham et al., 2007; Kim and Lord, 2012; Hus et al., 2013; Havdahl et al., 2016). However, there is much less work on how specific aspects of ASD symptom measurement are affected by developmental and/or language level. This information is needed to increase precision of measurement of ASD symptoms in the context of extreme developmental variability that characterizes NDD clinical and research populations.
Examining measurement invariance (MI)/differential item functioning (DIF) across groups defined based on certain characteristics is one way to advance ASD measurement in this area. MI refers to “the situation in which scales provide the same results across different samples or populations” (Zedeck, 2014, p. 211), which is a critical property of measures that allows factor scores to be compared meaningfully across groups. MI is often tested in a stepwise fashion with increasingly strict standards for equivalence. Specifically, MI is commonly tested across three levels of equivalence: (1) configural invariance of the number of factors and loading pattern, (2) metric invariance of the factor loadings, which reflect the strength of the associations between the items and the factors (i.e., latent constructs), and (3) scalar invariance of the intercepts, which indicates the means of item scores across groups were reflective of means of the latent construct. Adequate MI is established by demonstrating that constraints on each of the parameters described above do not significantly worsen model fit. For more information on MI and differential item functioning, please see Widaman and Reise (1997); Teresi and Fleishman (2007), and Bauer et al. (2020).
In recent years, multiple studies on MI/DIF have been carried out with different ASD symptom measures, including the Childhood Autism Rating Scale (CARS) (Schopler et al., 1988, 2010), ADOS (Lord et al., 2000, 2012), Social Responsiveness Scale, and ADI-R (Constantino, 2005; Constantino and Gruber, 2012). These studies primarily focused on the effects of race/ethnicity, sex/gender and chronological age on scores (ADOS: Harrison et al., 2017; Ronkin et al., 2021; Burrows et al., 2022; Kalb et al., 2022; CARS: Stevanovic et al., 2021; SRS and ADI-R: Frazier and Hardan, 2017), with a few studies also investigating MI across groups with or without ID (Sturm et al., 2017; Dovgan et al., 2019). While these studies provided preliminary evidence that ASD symptom measures should take the impact of cognitive abilities into account, understanding of how cognitive or language abilities influence the measurement of specific ASD symptoms is still limited. Thus, the current study chose to focus on children with developmental delays to clarify the impact of finer divisions of cognitive and language abilities on the measurement of ASD symptom domains within this population. This information is necessary to improve the measurement of ASD symptoms within this special group, wherein differential diagnosis of ASD is especially challenging.
The ADOS is one of the most commonly used measures in the diagnostic assessment of ASD. Module 1 is designed for individuals with chronological age over 31 months who are not yet using flexible phrase speech; thus, children receiving Module 1 present with clinically significant delays in language and/or overall development. However, even among this group, there is substantial variability in age and non-verbal cognitive ability, as well as in expressive language ability (i.e., from no word approximations or words to beginning use of multiple word combinations). Therefore, examining MI of the latent constructs of ASD symptom domains in the context of the ADOS Module 1 provides a unique opportunity to elucidate the impact of mental age and spoken language level on the measurement of ASD symptoms in children with developmental delays.
Materials and methods
Participants
Data for the current analyses were aggregated from multiple sites to obtain a large sample of children who received ADOS Module 1 as part of a comprehensive diagnostic evaluation. Participants were included in the current analysis if they: (1) were between 31 and119 months at the time of ADOS administration; (2) had undergone a comprehensive diagnostic evaluation to determine a best-estimate diagnosis of ASD or another non-ASD NDD; (3) had complete data on the selected items from ADOS Module 1; (4) received a developmental/cognitive assessment at the time of the ADOS Module 1 administration; and (5) had cognitive assessment information available for the calculation of non-verbal age equivalents. This resulted in 1043 children with ASD and 153 without ASD from seven sites (see Supplementary material for details about data sources and sample aggregation). Table 1 shows the demographic characteristics of the study sample.
Measures
The Autism Diagnostic Observation Schedule (Lord et al., 2000, 2012) is a standardized, semi-structured observational assessment designed to elicit social communication and restricted and repetitive behaviors associated with a diagnosis of ASD. It was designed to accommodate the assessment of ASD symptoms across language levels, with developmentally appropriate activities and codes organized into Modules (Lord et al., 2000, 2012). In the current analysis, we only included participants who were administered Module 1, designed for individuals who do not yet use flexible phrase speech. Consistent with scoring conventions, item scores of 0,1, and 2 were included in the analysis as they were, scores of 3 were converted to 2s for analysis, and scores of 8 (“Not applicable”) and 9 (“Unknown”) were converted to 0s.
As reflected in DSM-5 diagnostic criteria for ASD (American Psychiatric Association., 2013), previous factor analyses of the ADOS have consistently identified two core symptom domains of SCI and RRB (Gotham et al., 2007, 2008; Huerta et al., 2012; Harrison et al., 2017). Therefore, the current analyses focused on a subset of items mapping onto the two latent constructs of interest, SCI and RRB. Items on play (Section C) and other abnormal behaviors (Section E) were excluded. We also excluded the following items, as they were later added in the ADOS-2 and therefore missing for older cases who received ADOS-G: B13a Amount of Social Overtures/Maintenance to Attention: Examiner; B13b Amount of Social Overtures/Maintenance to Attention: Parent/Caregiver; B14 Quality of Social Response; B15 Level of Engagement; B16 Overall Quality of Rapport. Item A6 Use of Another’s Body was excluded as, unlike the other SCI items, it reflects the presence of abnormal behavior rather than the absence of developmentally expected behavior. For RRB, we excluded items that were dependent on sufficient spoken language to exhibit the abnormality (A3 Intonation of Vocalizations, A4 Immediate Echolalia, A5 Stereotyped/Idiosyncratic Use of Words or Phrases). We also excluded Item D3 Self-Injurious Behavior due to an extremely low rate of endorsement (<9% endorsing 1s or 2s). In total, 15 items assessing SCI and three items assessing RRB were included in the analyses (see Table 2).
Spoken Language Level. We derived the language level classification based on Item A1 “Overall Level of Non-Echoed Spoken Language” from the ADOS Module 1. Consistent with instructions for use of the revised algorithms (Gotham et al., 2007), participants who received scores of 3 or 4 were assigned to “Few to No words” and participants who received scores of 0, 1, or 2 were assigned to “Some words” group. The validity of these spoken language groups is further supported by previous studies showing differences between “Few to No Words” and “Some Words” on other measures of expressive language and cognitive ability (Bal et al., 2016; Mazurek et al., 2019).
Non-verbal mental age. Participants included in the aggregated dataset were administered at least one measure of cognitive ability based on site-specific protocols and/or clinician judgment about the developmentally appropriate test: the Mullen Scales of Early Learning (MSEL; (Mullen, 1995), the Differential Ability Scales (DAS) (Elliott, 2007), and/or the Merrill-Palmer Scales of Development (Roid and Sampers, 2004). The MSEL was used for 89% of the non-ASD sample and 75% of the ASD sample. For each participant, a non-verbal mental age was derived based on averaging available age equivalents from the non-verbal subtests. For those who received the MSEL, the age equivalents from the Fine Motor and Visual Reception subscales were averaged to represent NVMA (see Bishop et al., 2011; Farmer et al., 2016).
We dichotomized the NVMA variable to NVMA under 24 months vs. NVMA of 24 months and above for both practical and theoretical reasons: (1) given different tests were administered across sites, the binary categories will achieve more reliable grouping by avoiding the point estimates of the NVMA; (2) the cut point at 24 months allows sufficient sample sizes in both groups; (3) moreover, 24 months is an age at which children would be expected to use phrase speech in typical development (Sheldrick et al., 2019); thus, children with a non-verbal mental age above 24 months who receive Module 1 (rather than Module 2 or 3) show evidence of a discrepancy between their non-verbal mental age and their spoken language level. Therefore, we might expect that items developed for children with a very low spoken language level (i.e., language abilities characteristic of children under 24 months) might function differently in those with higher NVMA.
Best Estimate Diagnosis of ASD. All participants underwent multi-disciplinary evaluations by experienced clinicians and/or researchers who had established and maintained research reliability on the ADOS/ADOS-2. Best-estimate clinical diagnoses of ASD or the absence of ASD (i.e., Non-ASD) were determined based on all available information, including parent interviews of developmental history and direct observation of ASD symptoms (including the ADOS), as well as tests of cognitive and adaptive functioning.
Statistical analyses
Confirmatory factor analyses
Separate CFA with two factors (SCI and RRB; see Table 2 for ADOS Module 1 item mapping onto the two factors) were conducted across two spoken language level groups and two NVMA groups, respectively, to examine configural invariance (i.e., the number of factors and loading pattern) (Widaman and Reise, 1997). Factor analyses were conducted in Mplus with WLSMV estimator for ordered categorical variables. The chi-square statistics, comparative fit index (CFI), the root mean square error of approximation (RMSEA) and its 90% confidence interval (CI), and the standardized root mean square residual (SRMR) were examined for CFA model fit, with CFI larger than 0.95, RMSEA and SRMR smaller than 0.08 indicating a good fit (Hu and Bentler, 1999).
Moderated non-linear factor analysis
Once configural invariance was established through the CFA across NVMA and spoken language level groups, we proceeded to examine higher levels of structural validity testing of the two latent constructs across covariate groupings of interests (both of which were analyzed using effects coding): spoken language level (i.e., −1 = Few to No words vs. 1 = Some words) and developmental level (i.e., NVMA: −1 = under 24 months vs. 1 = 24 months and above). Moderated Non-linear Factor Analysis (MNLFA) is similar to both the multiple-group CFA and the multiple-indicator multiple-cause (MIMIC) methods for evaluating measurement invariance, but it extends both to multiple groups, categorical or count data, and the inclusion of multiple grouping variables at the same time. In the MNLFA model, MI/DIF is viewed as a form of parameter moderation; and thus, tested in the model for statistical significance as moderators of factor and item parameters. That is, moderation of the intercepts would indicate uniform DIF, whereas moderation of the factor loadings would indicate non-uniform DIF. We recommend that interested readers refer to Bauer (2017) for more details. Since MNLFA only accommodates unidimensional factor structure, separate analyses were conducted for SCI and RRB. The MNLFA method allows testing of the impact of spoken language levels and NVMA groups at the same time on the mean and variances of latent constructs, as well as their impacts on the intercept and loading of each item on the latent constructs. MNLFA involves an iterative process where each item is tested independently, then the significant (p < 0.05) effects are retained and tested simultaneously in one model. Lastly, a final model was estimated using the statistically significant parameters after the Benjamini-Hochberg false discovery rate correction to adjust for multiple comparisons. Moderated item effects were examined and reported to understand the impact of NVMA and spoken language level. The resulting model was then used to estimate the factor scores of the two latent constructs of SCI and RRB.
We employed an updated version of the R package aMNLFA Version 1.1.2 (Gottfredson et al., 2019)1 to streamline the generation of the MPlus codes and automate the process of integrating all effects into one model. We carefully reviewed and modified the automated MPlus codes to fit our dataset and research questions.
While there are multiple measures of impact of DIF on the overall measurement of the latent constructs (Meade, 2010), no recommended metric is available for the assessment of overall differential test functioning (DTF) in the context of MNLFA with simultaneous testing of multiple grouping variables. Therefore, to evaluate the differences between DIF-adjusted latent construct scores based on the group-specific information and the factor scores of latent constructs assuming full measurement invariance, we chose to adapt the Root Expected Mean Square Difference (REMSD) which was developed to index subpopulation invariance of linking and equating relationships (Dorans and Holland, 2000). Although MNLFA and equating analyses are distinct, the contrast between group-specific (i.e., with DIF) and overall (i.e., invariant) item parameters in the MNLFA context is comparable to the group-specific and overall equating relationship from which the REMSD statistic was originally derived. The adapted REMSD metric was calculated as the square root of the expected value of squared differences between the DIF-adjusted latent construct scores (FSmnlfa) and the factor scores assuming full measurement invariance of the item parameters (FSFI), divided by the standard deviation of the latent factor score (fixed to 1):
Further, effect sizes (Cohen’s d) were calculated for group comparisons of the latent construct factor scores.
Results
The majority of the aggregated sample was diagnosed with ASD, which is expected given the data were mostly drawn from autism specialty clinics or research projects focused on ASD (see Table 1). The descriptive statistics showed that, compared to those without ASD, children in the ASD group were more likely to be male (χ2 = 13.05, p = 0.003), to have “Few to No words” (χ2 = 10.02, p = 0.002), and to have an NVMA of 24 months and over (χ2 = 18.92, p < 0.001).
The two-factor structure with SCI and RRB showed a good fit, supporting configural invariance of the ADOS across the two spoken language levels and the two NVMA groups (see Table 3). Table 4 shows item factor loadings onto the two factors of SCI and RRB, respectively, across the two spoken language levels and two NVMA groups.
For each latent construct, ensuing MNLFAs were conducted separately. For the latent construct of SCI, we observed a significant effect of spoken language level on the measured SCI scores (Estimate = −0.45, SE = 0.034, p < 0.001), with individuals with Few to No words showing higher levels of SCI symptoms. Multiple items showed loading and intercept DIF across language levels on the latent construct of SCI, including Unusual Eye Contact, Integration of Gaze and Other Behaviors during Social Overtures, Requesting, and Showing. Only one item, Frequency of Vocalization, showed significant loading and intercept DIF across the NVMA groups on the SCI (see Table 5 upper panel for parameter estimates and Figure 1 for the final SCI measurement model). For the latent construct of RRB, the mean level of measured RRB differed across language levels (Estimate = −0.249, SE = 0.046, p < 0.001). There were also loading DIFs of Item “Hand/finger and Other Complex Mannerisms” across spoken language levels and “Unusually Repetitive Interests or Stereotyped Behaviors” across NVMA groups (see Table 5 bottom panel for parameter estimates and Figure 2 for the final RRB measurement models of the two latent constructs). That is, these items show different levels of associations with the latent constructs of SCI and RRB, as well as varying item difficulties. In sum, metric invariance did not hold for several items on both SCI and RRB latent constructs, with subsets of items functioning differently across groups.
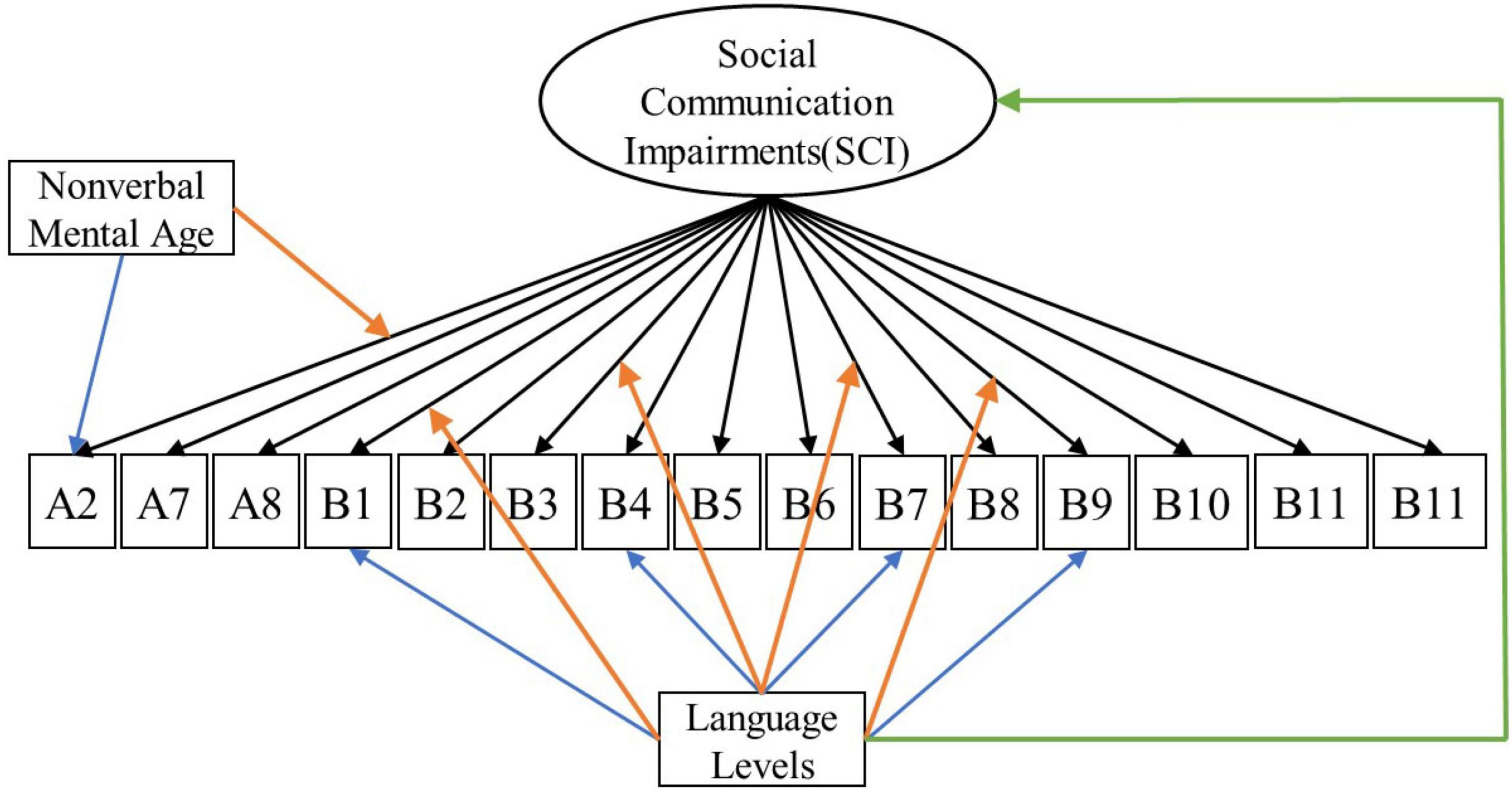
Figure 1. Measurement model for social communication impairments (SCI). Black arrows indicate factor loadings of each item examined on the SCI latent construct. Colored Arrows in the figure showing significant impact of the covariate on the factor and item parameters: (1) Green arrow represents the impact of language level on the mean of the latent construct; (2) Orange arrows represent the impact of covariates (NVMA and language level groups) on the relationships between the item and the latent construct (non-uniform DIF); (3) Blue arrows represent the impact of covariates on the levels of items when the overall level of the latent construct is similar across groups (uniform DIF). For specific item names, please refer to Table 2.
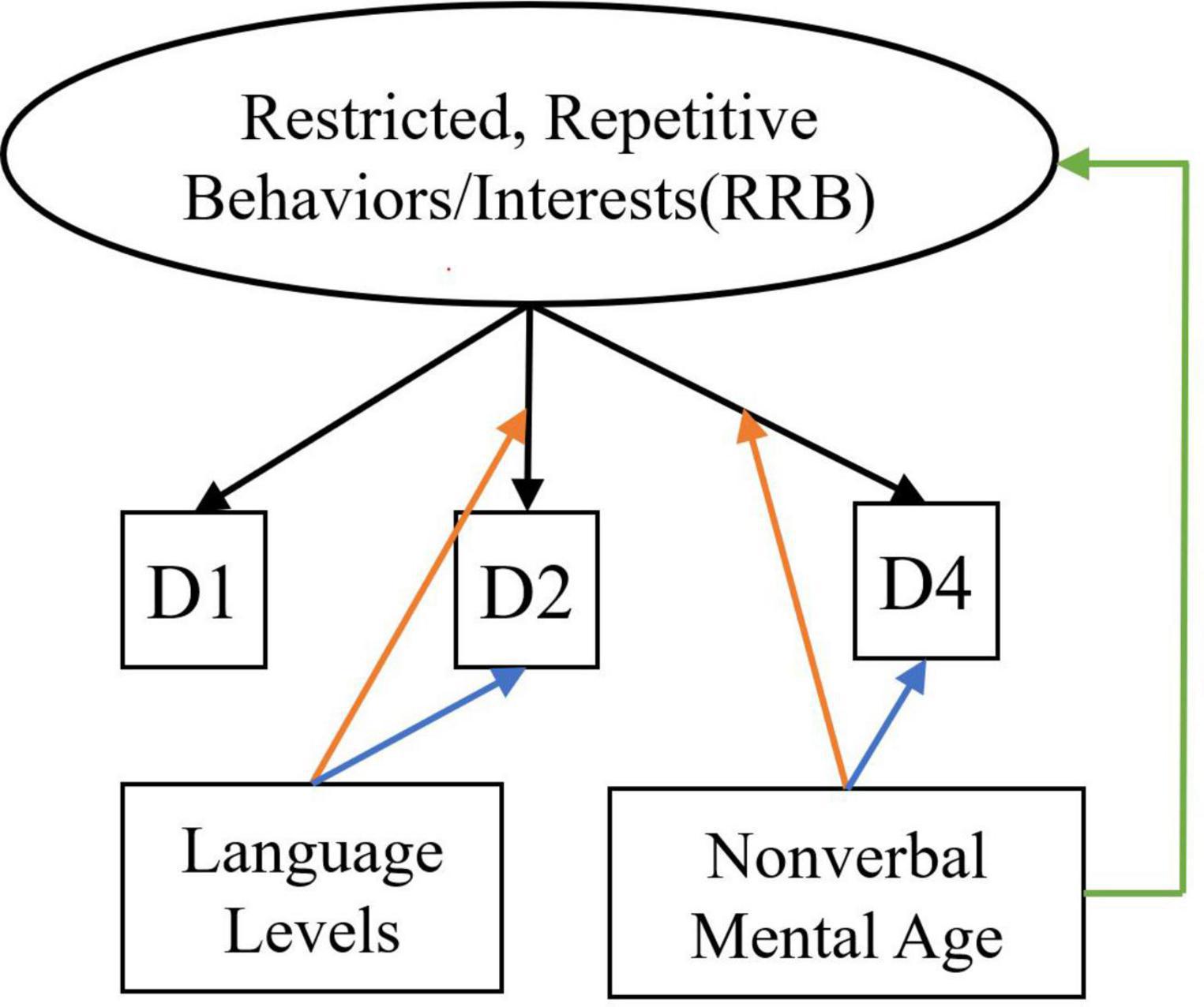
Figure 2. Measurement model for restricted, repetitive behaviors/interests. Black arrows indicate factor loadings of each item examined on the RRB latent construct. Colored Arrows in the figure showing significant impact of the covariate on the factor and item parameters: (1) Green arrow represents the impact of language level on the mean of the latent construct; (2) Orange arrows represent the impact of covariates (NVMA and language level groups) on the relationships between the item and the latent construct (non-uniform DIF); (3) Blue arrows represent the impact of covariates on the levels of items when the overall level of the latent construct is similar across groups (uniform DIF). For specific item names, please refer to Table 2.
Item-level DIF has moderate to large impact on the score of the two latent constructs: REMSDSCI = 0.66 and REMSDRRB = 0.74, indicating the need to consider measurement bias in interpreting the measured scores of the two latent constructs. Effect sizes of the DIF-adjusted SCI factor scores indicated that children with “Few to No Words” scored about 1 standard deviation (SD) higher than those with “Some Words” in SCI severity factor scores (Cohen’s d = 1.01); similarly, for the RRB factor scores, children with “Few to No Words” scored 0.75 SD higher. On the other hand, small ES were observed for the group comparisons across NVMA of both latent constructs (SCI: ES = 0.34, RRB: ES = 0.27).
Discussion
The current study was conducted to provide more explicit guidance about how the measurement of ASD symptoms (as indexed by selected items from Module 1 of the ADOS) might be affected by language and developmental level. While decades of research indicate that both language and cognitive ability influence the manifestation and measurement of ASD-related symptoms, there is much less work about specific aspects of ASD symptom measurement that may be problematic when comparing children developmental and spoken language levels. Greater understanding of this issue is important given the extreme developmental heterogeneity that characterizes ASD and NDD clinical and research populations.
Consistent with previous studies of ASD symptom structure, which ultimately informed DSM-5 diagnostic criteria (Huerta et al., 2012; Frazier et al., 2014), findings from the current CFA of the ADOS Module 1indicate two core symptom domains (i.e., SCI and RRB). This structure held across spoken language levels and NVMA groupings, supporting configural invariance of the measure. However, when examining the mean levels of latent constructs for both SCI and RRB, children with “Few to No Words” scored systematically higher (i.e., more impairments) than those with “Some Words”.
When looking at where the ASD symptom measurements showed biases, stricter levels of measurement invariance did not hold at the item level for some items in the MNLFA models for either SCI or RRB latent construct. For the measurement of SCI, loading and intercept DIF was observed for four items across spoken language levels [Unusual Eye Contact (B1), Integration of Gaze and Other Behaviors during Social Overtures (B4), Requesting (B7), and Showing (B9)], and one item [Frequency of Vocalization(A2)] across NVMA groups. All four SCI items that showed DIF across spoken language levels involved the use of eye contact with the examiner, highlighting the potential role of spoken language level even when measuring basic non-verbal social communication skills such as eye contact. Even though only a small subset of items (n = 5) showed any measurement bias on the latent construct SCI, the DIFs showed impact on the overall latent construct scores, underscoring the need to carefully consider the impact of spoken language levels when making score comparisons between individuals. On the other hand, two out of three RRB items (i.e., Hand and Finger and Other Complex Mannerisms and Unusually Repetitive Interests or Stereotyped Behaviors) included in the analyses showed bias across either spoken language or NVMA, indicating that the measurement of RRBs with only the three selected items is likely problematic. This is consistent with previous item response theory analyses done with ADOS Modules 3 and 4 (Kuhfeld and Sturm, 2018).
To further understand different levels of autism symptoms across spoken language levels, we compared SCI and RRB factor scores after adjusting for measurement biases identified at the item level, and found that they still differed significantly across spoken language levels, with higher severity scores seen in children with “Few to No Words”. These findings suggest that there are likely true differences in the levels of SCI and RRB symptom severity, as measured using Module 1 of the ADOS, between children with “Few to No Words” vs. “Some Words”. This provides further evidence for the decision to create separate algorithms based on finer language-level divisions within Module 1 (Gotham et al., 2007, 2008). Given that some items on the ADOS Module 1 function differently for children of different spoken language levels, even among those with minimal verbal abilities, clinicians and researchers should follow the algorithm guidelines to derive scores for the two spoken language levels separately and only interpret scores at the domain and scale levels, but not at the item level.
To our knowledge, this study is the first to examine MI of ASD symptoms within children with developmental delays across cognitive and spoken language levels. A deeper understanding of how ASD symptom measurement is affected by developmental level is critical, particularly given increased interests in behavioral phenotyping of rare genetic conditions, many of which are associated with severe to profound ID (Arvio and Sillanpää, 2003; Richards et al., 2015; Abbeduto et al., 2019; Burdeus-Olavarrieta et al., 2021). We focused on ADOS Module 1 to specifically home in on the effects of mental age and expressive language in children with lower cognitive and language abilities. However, this sample does not represent the full range of minimally verbal individuals who have even more severe delays. Importantly, valid administration of the ADOS requires that a child be able to walk, see, and hear at the time of assessment, meaning that it is not even valid for a significant proportion of children with severe to profound ID. Moreover, given the reduced specificity of the measure, the test developers advised against using the ADOS in children with NVMA below 15 months, resulting in very few such cases available for the current analyses: Non-ASD = 8 (5.2%), ASD = 26 (2.5%). Therefore, the present findings have limited applicability to individuals with severe to profound ID and/or sensory and motor impairments, and do not change the recommendation that ADOS scores may not be valid in this group. Yet, the fact remains that clinicians and researchers are increasingly faced with the challenges of assessing ASD symptoms in individuals for whom current measures were not validated, highlighting the need for empirical evidence to measure ASD symptoms validly and reliably in this population. Further, children develop over time and some gain cognitive and language skills as they grow and receive intervention. Thus, future longitudinal studies should examine intra-individual changes as children shift from “Few to No Words” to “Some Words” and/or from lower NVMA group to higher NVMA levels.
The current study represents a first step in understanding ASD symptom measurement for those who are minimally verbal. Even within Module 1, which is already only applicable to children within a relatively narrow developmental range, our findings highlight the need for finer divisions based on spoken language level (e.g., “Few to No Words” and “Some Words”) and/or mental age to optimize measurement of ASD symptoms. Thus, to advance measurement of SCI and RRB in the extremely heterogeneous population of children with neurodevelopmental disorders, the field must work to enhance developmentally appropriate measurement strategies (Bishop et al., 2019). Moreover, it is imperative that clinicians and researchers implement best-practice methods for carefully considering developmental profiles, including cognitive and spoken language levels, in their assessment of ASD-related symptoms and behaviors.
Data availability statement
Publicly available datasets were analyzed in this study. Data from the Simons Simplex Collections is available to qualified researchers. Approved researchers can obtain the SSC population dataset described in this study by applying at https://base.sfari.org. Data from other sources can be requested by contacting the principal investigators and directors at each site.
Ethics statement
Ethical review and approval was not required for the study on human participants in accordance with the local legislation and institutional requirements. Written informed consent to participate in this study was provided by the participants or their legal guardian/next of kin.
Author contributions
SZ and SB conceptualized and designed the study with consultation from AK, CF, and AT. SZ aggregated datasets, conducted statistical analyses, interpreted the results, and drafted the manuscript. AK and CB provided consultation on data analysis and result interpretation. SB together with AK, CF, and AT provided feedback on previous versions of the manuscript. AT, CB, SK, CL, AE, NT, KN, EW, JR, and SB contributed to data collection. SB secured funding for the current study. All authors provided feedback on and approved the final version of the manuscript.
Funding
This work was supported by grants from the Eunice Kennedy Shriver National Institute of Child Health and Human Development (NICHD; R01HD093012 to SB), and in part by the Intramural Research Program of the NIMH (1ZICMH002961 to AT).
Acknowledgments
We are grateful to all of the families at the participating Simons Simplex Collection (SSC) sites, as well as the principal investigators (A. Beaudet, R. Bernier, J. Constantino, E. Cook, E. Fombonne, D. Geschwind, R. Goin-Kochel, E. Hanson, D. Grice, A. Klin, D. Ledbetter, C. Lord, C. Martin, D. Martin, R. Maxim, J. Miles, O. Ousley, K. Pelphrey, B. Peterson, J. Piggot, C. Saulnier, M. State, W. Stone, J. Sutcliffe, C. Walsh, Z. Warren, E. Wijsman). We appreciate obtaining access to phenotypic data on the Simons Foundation Autism Research Initiative (SFARI) Base. Approved researchers can obtain the SSC population dataset described in this study by applying at https://base.sfari.org. We are also grateful to all the clinicians (Robin Rumsey, Desirae Rambeck, Chimei Lee, Rebekah Hudock, and Jane Nofer) from the University of Minnesota Autism and Neurodevelopment Clinic and all other clinics who contributed to the data collection.
Conflict of interest
CL and SB have received royalties from the ADOS-2, and all profits are donated to charity.The remaining authors declare that the research was conducted in the absence of any commercial or financial relationships that could be construed as a potential conflict of interest.
Publisher’s note
All claims expressed in this article are solely those of the authors and do not necessarily represent those of their affiliated organizations, or those of the publisher, the editors and the reviewers. Any product that may be evaluated in this article, or claim that may be made by its manufacturer, is not guaranteed or endorsed by the publisher.
Supplementary material
The Supplementary Material for this article can be found online at: https://www.frontiersin.org/articles/10.3389/fpsyg.2022.927847/full#supplementary-material
Footnotes
References
Abbeduto, L., Thurman, A. J., McDuffie, A., Klusek, J., Feigles, R. T., Brown, W. T., et al. (2019). ASD comorbidity in Fragile X Syndrome: symptom profile and predictors of symptom severity in adolescent and young adult males. J. Autism Dev. Disord. 49, 960–977. doi: 10.1007/s10803-018-3796-2
American Psychiatric Association. (2013). Diagnostic and Statistical Manual of Mental Disorders, 5th Edn. Washington, DC: American Psychiatry Publishing.
Arvio, M., and Sillanpää, M. (2003). Prevalence, aetiology and comorbidity of severe and profound intellectual disability in Finland. J. Intellect. Disabil. Res. 47, 108–112. doi: 10.1046/j.1365-2788.2003.00447.x
Bal, V. H., Katz, T., Bishop, S. L., and Krasileva, K. (2016). Understanding definitions of minimally verbal across instruments: Evidence for subgroups within minimally verbal children and adolescents with autism spectrum disorder. J. Child Psychol. Psychiatry 57, 1424–1433. doi: 10.1111/jcpp.12609
Bauer, D. J. (2017). A more general model for testing measurement invariance and differential item functioning. Psychol. Methods 22, 507–526. doi: 10.1037/met0000077
Bauer, D. J., Belzak, W. C. M., and Cole, V. T. (2020). Simplifying the assessment of measurement invariance over multiple background variables: using Regularized Moderated Nonlinear Factor Analysis to detect differential item functioning. Struct. Equ. Model. 27, 43–55. doi: 10.1080/10705511.2019.1642754
Bishop, S. L., Guthrie, W., Coffing, M., and Lord, C. (2011). Convergent validity of the Mullen Scales of Early Learning and the Differential Ability Scales in children with autism spectrum disorders. Am. J. Intellect. Dev. Disabil. 116, 331–343. doi: 10.1352/1944-7558-116.5.331
Bishop, S., Farmer, C., Kaat, A., Georgiades, S., Kanne, S., and Thurm, A. (2019). The need for a developmentally based measure of social-communication skills. J. Am. Acad. Child Adolesc. Psychiatry 58, 555–560. doi: 10.1016/j.jaac.2018.12.010
Burbidge, C., Oliver, C., Moss, J., Arron, K., Berg, K., Furniss, F., et al. (2010). The association between repetitive behaviours, impulsivity and hyperactivity in people with intellectual disability. J. Intellect. Disabil. Res. 54, 1078–1092. doi: 10.1111/j.1365-2788.2010.01338.x
Burdeus-Olavarrieta, M., San José-Cáceres, A., García-Alcón, A., González-Peñas, J., Hernández-Jusdado, P., and Parellada-Redondo, M. (2021). Characterisation of the clinical phenotype in Phelan-McDermid syndrome. J. Neurodev. Disord. 13:26. doi: 10.1186/s11689-021-09370-5
Burrows, C. A., Grzadzinski, R. L., Donovan, K., Stallworthy, I. C., Rutsohn, J., St. John, T., et al. (2022). A data driven approach in an unbiased sample reveals equivalent sex ratio of autism spectrum disorder associated impairment in early childhood. Biol. Psychiatry doi: 10.1016/j.biopsych.2022.05.027
Constantino, J. N., and Gruber, C. P. (2012). Social Responsiveness Scale, Second Edition (SRS-2). Los Angeles, CA: Western Psychological Services.
Corsello, C., Hus, V., Pickles, A., Risi, S., Cook, E. H., Leventhal, B. L., et al. (2007). Between a ROC and a hard place: Decision making and making decisions about using the SCQ. J. Child Psychol. Psychiatry 48, 932–940. doi: 10.1111/j.1469-7610.2007.01762.x
Dorans, N. J., and Holland, P. W. (2000). Population invariance and the equatability of tests: basic theory and the linear case. J. Educ. Measur. 37, 281–306.
Dovgan, K., Mazurek, M. O., and Hansen, J. (2019). Measurement invariance of the child behavior checklist in children with autism spectrum disorder with and without intellectual disability: follow-up study. Res. Autism Spectr. Disord. 58, 19–29. doi: 10.1016/j.rasd.2018.11.009
Evans, D. W., and Gray, F. L. (2000). Compulsive-like behavior in individuals with Down Syndrome: its relation to mental age level, adaptive and maladaptive behavior. Child Dev. 71, 288–300. doi: 10.1111/1467-8624.00144
Farmer, C., Golden, C., and Thurm, A. (2016). Concurrent validity of the differential ability scales, second edition with the Mullen Scales of Early Learning in young children with and without neurodevelopmental disorders. Child Neuropsychol. 22, 556–569. doi: 10.1080/09297049.2015.1020775
Frazier, T. W., and Hardan, A. Y. (2017). Equivalence of symptom dimensions in females and males with autism. Autism 21, 749–759. doi: 10.1177/1362361316660066
Frazier, T. W., Ratliff, K. R., Gruber, C., Zhang, Y., Law, P. A., and Constantino, J. N. (2014). Confirmatory factor analytic structure and measurement invariance of quantitative autistic traits measured by the Social Responsiveness Scale-2. Autism 18, 31–44. doi: 10.1177/1362361313500382
Gotham, K., Risi, S., Dawson, G., Tager-flusberg, H., Joseph, R., Carter, A., et al. (2008). A Replication of the Autism Diagnostic Observation Schedule (ADOS) Revised Algorithms. J. Am. Acad. Child Adolesc. Psychiatry 47, 642–651. doi: 10.1097/CHI.0b013e31816bffb7
Gotham, K., Risi, S., Pickles, A., and Lord, C. (2007). The Autism Diagnostic Observation Schedule: revised algorithms for improved diagnostic validity. J. Autism Dev. Disord. 37, 613–627. doi: 10.1007/s10803-006-0280-1
Gottfredson, N. C., Cole, V. T., Giordano, M. L., Bauer, D. J., Hussong, A. M., and Ennett, S. T. (2019). Simplifying the implementation of modern scale scoring methods with an automated R package: automated moderated nonlinear factor analysis (aMNLFA). Addict. Behav. 94, 65–73. doi: 10.1016/j.addbeh.2018.10.031
Grzadzinski, R., Di Martino, A., Brady, E., Mairena, M. A., O’Neale, M., Petkova, E., et al. (2011). Examining autistic traits in children with ADHD: does the autism spectrum extend to ADHD? J. Autism Dev. Disord. 41, 1178–1191. doi: 10.1007/s10803-010-1135-3
Harrison, A. J., Long, K. A., Tommet, D. C., and Jones, R. N. (2017). Examining the role of race, ethnicity, and gender on social and behavioral ratings within the Autism Diagnostic Observation Schedule. J. Autism Dev. Disord. 47, 2770–2782. doi: 10.1007/s10803-017-3176-3
Havdahl, K. A., Hus Bal, V., Huerta, M., Pickles, A., Øyen, A.-S., Stoltenberg, C., et al. (2016). Multidimensional influences on autism symptom measures: Implications for use in etiological research. J. Am. Acad. Child Adolesc. Psychiatry 55, 1054–1063.e3. doi: 10.1016/j.jaac.2016.09.490
Hepburn, S. L., and Moody, E. J. (2011). Diagnosing autism in individuals with known genetic syndromes: clinical considerations and implications for intervention. Int. Rev. Res. Dev. Disabil. 40, 229–259. doi: 10.1016/B978-0-12-374478-4.00009-5
Hoch, J., Spofford, L., Dimian, A., Tervo, R., MacLean, W. E., and Symons, F. J. (2016). A direct comparison of self-injurious and stereotyped motor behavior between preschool-aged children with and without developmental delays. J. Pediatr. Psychol. 41, 566–572. doi: 10.1093/jpepsy/jsv102
Hu, L., and Bentler, P. M. (1999). Cutoff criteria for fit indexes in covariance structure analysis: conventional criteria versus new alternatives. Struct. Equ. Model. 6, 1–55. doi: 10.1080/10705519909540118
Huerta, M., Bishop, S. L., Duncan, A., Hus, V., and Lord, C. (2012). Application of DSM-5 criteria for autism spectrum disorder to three samples of children with DSM-IV diagnoses of pervasive developmental disorders. Am. J. Psychiatry 169, 1056–1064. doi: 10.1176/appi.ajp.2012.12020276
Hus, V., Bishop, S., Gotham, K., Huerta, M., and Lord, C. (2013). Factors influencing scores on the social responsiveness scale. J. Child Psychol. Psychiatry 54, 216–224. doi: 10.1111/j.1469-7610.2012.02589.x
Kalb, L. G., Singh, V., Hong, J. S., Holingue, C., Ludwig, N. N., Pfeiffer, D., et al. (2022). Analysis of race and sex bias in the Autism Diagnostic Observation Schedule (ADOS-2). JAMA Pediatr. 5:e229498. doi: 10.1001/jamanetworkopen.2022.9498
King, B. H., de Lacy, N., and Siegel, M. (2014). Psychiatric assessment of severe presentations in autism spectrum disorders and intellectual disability. Child Adolesc. Psychiatr. Clin. North Am. 23, 1–14. doi: 10.1016/j.chc.2013.07.001
Kim, S. H., and Lord, C. (2012). Combining information from multiple sources for the diagnosis of autism spectrum disorders for toddlers and young preschoolers from 12 to 47 months of age. J. Child Psychol. Psychiatry 53, 143–151. doi: 10.1111/j.1469-7610.2011.02458.x
Kuhfeld, M., and Sturm, A. (2018). An examination of the precision of the Autism Diagnostic Observation Schedule using item response theory. Psychol. Assess. 30, 656–668. doi: 10.1037/pas0000512
Lord, C., and Bishop, S. L. (2021). Let’s Be Clear That “Autism Spectrum Disorder Symptoms”. Are Not Always Related to Autism Spectrum Disorder. Am. J. Psychiatry 178, 680–682. doi: 10.1176/appi.ajp.2021.21060578
Lord, C., Risi, S., Lambrecht, L., Cook, E. H., Leventhal, B. L., DiLavore, P. C., et al. (2000). The Autism Diagnostic Observation Schedule—Generic: a Standard Measure of Social and Communication Deficits Associated with the Spectrum of Autism. J. Autism Dev. Disord. 30, 205–223. doi: 10.1023/A:1005592401947
Lord, C., Rutter, M., and Le Couteur, A. (1994). Autism Diagnostic Interview-Revised: a revised version of a diagnostic interview for caregivers of individuals with possible pervasive developmental disorders. J. Autism Dev. Disord. 24, 659–685. doi: 10.1007/BF02172145
Lord, C., Rutter, M., DiLavore, P., Risi, S., Gotham, K., and Bishop, S. L. (2012). Autism Diagnostic Observation Schedule, Second Edition (ADOS-2) Manual (Part I): Modules 1-4. Torrance, CA: Western Psychological Services.
Mazurek, M. O., Baker-Ericzén, M., and Kanne, S. M. (2019). Brief Report: Calculation and convergent and divergent validity of a new ADOS-2 expressive language score. Am. J. Intellect. Dev. Disabil. 124, 438–449. doi: 10.1352/1944-7558-124.5.438
Meade, A. W. (2010). A taxonomy of effect size measures for the differential functioning of items and scales. J. Appl. Psychol. 95, 728–743. doi: 10.1037/a0018966
Moss, J., and Howlin, P. (2009). Autism spectrum disorders in genetic syndromes: implications for diagnosis, intervention and understanding the wider autism spectrum disorder population. J. Intellect. Disabil. Res. 53, 852–873. doi: 10.1111/j.1365-2788.2009.01197.x
Moss, J., Oliver, C., Arron, K., Burbidge, C., and Berg, K. (2009). The prevalence and phenomenology of repetitive behavior in genetic syndromes. J. Autism Dev. Disord. 39, 572–588. doi: 10.1007/s10803-008-0655-6
Richards, C., Jones, C., Groves, L., Moss, J., and Oliver, C. (2015). Prevalence of autism spectrum disorder phenomenology in genetic disorders: a systematic review and meta-analysis. Lancet Psychiatry 2, 909–916. doi: 10.1016/S2215-0366(15)00376-4
Risi, S., Lord, C., Gotham, K., Corsello, C., Chrysler, C., Szatmari, P., et al. (2006). Combining information from multiple sources in the diagnosis of autism spectrum disorders. J. Am. Acad. Child. Adolesc. Psychiatry 45, 1094–1103. doi: 10.1097/01.chi.0000227880.42780.0e
Roid, G., and Sampers, J. (2004). Merrill-Palmer Revised Scales of Development. Wood Dale, IL: Stoelting Co.
Ronkin, E., Tully, E. C., Branum-Martin, L., Cohen, L. L., Hall, C., Dilly, L., et al. (2021). Sex differences in social communication behaviors in toddlers with suspected autism spectrum disorder as assessed by the ADOS-2 toddler module. Autism [Epub ahead of print]. doi: 10.1177/13623613211047070
Rutter, M., Le Couteur, A., and Lord, C. (2005). Autism Diagnostic Interview-Revised. Los Angeles, CA: Western Psychological Services.
Schopler, E., Reichler, R. J., and Renner, B. R. (1988). The Childhood Autism Rating Scale (CARS). Los Angeles, CA: Western Psychological Services.
Schopler, E., Van Bourgondien, M. E., Wellman, G. J., and Love, S. R. (2010). The Childhood Autism Rating Scale, Second Edition (CARS 2). Los Angeles, CA: Western Psychological Services.
Sheldrick, R. C., Schlichting, L. E., Berger, B., Clyne, A., Ni, P., Perrin, E. C., et al. (2019). Establishing new norms for developmental milestones. Pediatrics 144:e20190374. doi: 10.1542/peds.2019-0374
Stevanovic, D., Costanzo, F., Fucà, E., Valeri, G., Vicari, S., Robins, D. L., et al. (2021). Measurement invariance of the Childhood Autism Rating Scale (CARS) across six countries. Autism Res. 14, 2544–2554. doi: 10.1002/aur.2586
Sturm, A., Kuhfeld, M., Kasari, C., and McCracken, J. T. (2017). Development and validation of an item response theory-based Social Responsiveness Scale short form. J. Child Psychol. Psychiatry 58, 1053–1061. doi: 10.1111/jcpp.12731
Teresi, J. A., and Fleishman, J. A. (2007). Differential item functioning and health assessment. Qual. Life Res. 16, 33–42. doi: 10.1007/s11136-007-9184-6
Thurm, A., Farmer, C., Salzman, E., Lord, C., and Bishop, S. (2019). State of the Field: differentiating intellectual disability from autism spectrum disorder. Front. Psychiatry 10:526. doi: 10.3389/fpsyt.2019.00526
Widaman, K. F., and Reise, S. P. (1997). “Exploring the measurement invariance of psychological instruments: Applications in the substance use domain,” in The Science of Prevention: Methodological Advances from Alcohol and Substance Abuse Research, eds K. J. Bryant, M. Windle, and S. G. West (Washington, DC: American Psychological Association), 281–324. doi: 10.1037/10222-009
Wolff, J. J., Bodfish, J. W., Hazlett, H. C., Lightbody, A. A., Reiss, A. L., and Piven, J. (2012). Evidence of a distinct behavioral phenotype in young boys with Fragile X Syndrome and Autism. J. Am. Acad. Child Adolesc. Psychiatry 51, 1324–1332. doi: 10.1016/j.jaac.2012.09.001
Keywords: autism symptoms, measurement invariance, language level, non-verbal mental age, ADOS
Citation: Zheng S, Kaat A, Farmer C, Thurm A, Burrows CA, Kanne S, Georgiades S, Esler A, Lord C, Takahashi N, Nowell KP, Will E, Roberts J and Bishop SL (2022) Bias in measurement of autism symptoms by spoken language level and non-verbal mental age in minimally verbal children with neurodevelopmental disorders. Front. Psychol. 13:927847. doi: 10.3389/fpsyg.2022.927847
Received: 25 April 2022; Accepted: 11 July 2022;
Published: 29 July 2022.
Edited by:
Lénia Carvalhais, Infante D. Henrique Portucalense University, PortugalReviewed by:
Zachary J. Williams, Vanderbilt University, United StatesCourtney Venker, Michigan State University, United States
Meagan Talbott, University of California, Davis, United States
Copyright © 2022 Zheng, Kaat, Farmer, Thurm, Burrows, Kanne, Georgiades, Esler, Lord, Takahashi, Nowell, Will, Roberts and Bishop. This is an open-access article distributed under the terms of the Creative Commons Attribution License (CC BY). The use, distribution or reproduction in other forums is permitted, provided the original author(s) and the copyright owner(s) are credited and that the original publication in this journal is cited, in accordance with accepted academic practice. No use, distribution or reproduction is permitted which does not comply with these terms.
*Correspondence: Shuting Zheng, c2h1dGluZy56aGVuZ0B1Y3NmLmVkdQ==