- Industrial Engineering Department, Diponegoro University, Semarang, Indonesia
This study has several purposes. First, identify indicators contributing to the performance of small and medium-sized enterprises (SMEs) that could be affected by the COVID-19. Second, formulate the framework to measure the level of vulnerability of SMEs. Third, assign the SMEs into several clusters. Data used in this research were collected through web-based closed questionnaires and short telephone interviews. This study used Content Validity Analysis, Analytical Hierarchy Process, Multi-Attribute Value Theory approach, K-means Clustering Analysis, and Discriminant Analysis for data processing. The data processing results indicated that the 44 valid indicators belonging to ten dimensions could be used to measure the level of vulnerability of SMEs whose performance was affected by the COVID-19 pandemic. The surveyed SMEs can be segmented into four clusters, namely resilient cluster, low vulnerability cluster, moderate vulnerability cluster, and high vulnerability cluster. Most of the surveyed SMEs belong to the moderate and high vulnerability clusters. The differences between the clusters were based on 16 indicators. These indicators include levels of supplier disruption and the SMEs’ market in which the SMEs operate or expect to operate. The results of this study help quantify how the pandemic could generate different levels of impact on each indicator that could depend on the business and what policymakers should consider as they contemplate the scale of the required intervention. Overall, this study contributes to the literature on the effects of the pandemic on SMEs by synthesizing the findings of studies on the impact of COVID-19 on SMEs. The study also determined the framework and the equation for measuring the level of SME vulnerability caused by the pandemic.
Introduction
The negative impact of the COVID-19 pandemic has been recorded in every aspect of life, with economic, political, social, and psychological implications (Bretas and Alon, 2020; Ratten, 2020). Although the main impact of this pandemic is on human health and human health perception (Akpan et al., 2020), Akbulaev et al. (2020) highlight the multi-faceted impact of the COVID-19 crisis. Besides human health and human health perception, the COVID-19 pandemic affects companies’ supply and demand and then forces them to operate in the new condition. During the pandemic, some industries have shown a certain level of resilience or even found a new operating niche and most small and medium-sized enterprises (SMEs) in several sectors found themselves in “new normal” operating environments (Gregurec et al., 2021). There is anxiety about how the pandemic and the government’s response to it (lockdowns, social distancing guidelines, etc.) will affect SMEs. This anxiety is significant since SMEs represent over 90% of all firms worldwide. This condition makes SMEs the backbone of the world economy in the formal and informal business sectors (Tannenbaum et al., 2020). SMEs constitute a significant part of the private sector in most developed and developing countries (Beck and Demirguc-Kunt, 2006). Despite their critical role, SMEs are the most threatened by the COVID-19 crisis, given their relatively vulnerable financial position (Ghosal and Ye, 2015; Doshi et al., 2018). Their vulnerability has triggered many government programs, including financial assistance, wage subsidies, and payment deferrals (OECD, 2020). Mainly, SMEs face many issues in managing their business, revenues, and finances (Shahbaz et al., 2020). The pandemic has significantly affected small companies due to their limited financial resources and business scale (Carruthers, 2020).
Given that Indonesian SMEs absorb approximately 97% of the total workforce in the economic sector, contributing about 61.41% to gross domestic product (GDP) in 2018 (Hidayati and Rachman, 2021), it is crucial to understand the negative impact of the COVID-19 pandemic on the GDP. Some researchers (such as Tairas, 2020; Saturwa et al., 2021) and government institutions present descriptive statistics explaining the impact of COVID-19 on Indonesian SMEs. Based on 1,332 complaints from SMEs in 18 provinces, the Indonesian Ministry of Cooperatives and Small and Medium Enterprises identified several issues facing SMEs during the COVID-19 pandemic. These issues include declining sales, difficulty obtaining raw materials, stagnant distribution caused by lockdown, difficulty securing capitalization, and low productivity caused by work hour restrictions (Kemenkop-UKM, 2020). About 917 SMEs (69%) experienced decreased sales turnover. Approximately 119 SMEs (9%) had difficulty distributing manufactured goods, and about 179 SMEs (13%) had difficulty accessing business capital. Moreover, approximately 50 SMEs (4%) experienced a drastic reduction in production that temporarily stopped production. Although the result of this study does not represent the overall conditions of SMEs in Indonesia, which consists of 59–62 million businesses unit, the results may indicate that SMEs in Indonesia experienced considerable pressure because of the pandemic.
Despite the attempts by several studies to explain the impact of the pandemic on Indonesian SME performance, it was not easy to find research that explicitly studied the significant indicators and framework for measuring the impact of the pandemic and then clustering the SMEs based on the level of the impacts. Accordingly, this study seeks to provide insights into the following research questions.
1. What indicators contribute to SME performance because of the COVID-19 pandemic?
2. How should the framework be formulated to measure SME vulnerability during the pandemic?
3. Should SMEs be assigned into clusters according to similar indicators reflecting the impact of the pandemic?
Based on these three research questions, our study contributes to the emerging body of literature on the performance management measurement of SMEs (such as Ng et al., 2020; Rojas-Lema et al., 2020; Sardi et al., 2020; Mendy, 2021; Ndzana et al., 2021) and the effects of the COVID-19 pandemic on SMEs (Al-Fadly, 2020; Bartik et al., 2020; Fabeil et al., 2020; Humphries et al., 2020; Lu et al., 2020; Lutfi et al., 2020; Omar et al., 2020; Ratnasingam et al., 2020; Shafi et al., 2020; Tairas, 2020; Dai et al., 2021; Nawaiseh, 2021; Saturwa et al., 2021). Basically, not only about the effect of the COVID-19 pandemic on the SMEs, the previous studies have been carried out on COVID-19 from different perspectives like digital learning during the emergence of COVID-19 virus (Hasan and Bao, 2020; Aditya, 2021; Chaturvedi et al., 2021; Deshpande and Mhatre, 2021; Smith et al., 2021), its impact on the economies of different countries (Ye et al., 2020; Ali et al., 2021; Bhattacharya and Banerjee, 2021; Cuschieri and Grech, 2021; Delbiso et al., 2021; Donnarumma and Pezzulo, 2021; Mahi et al., 2021; Prempeh, 2021; Roy et al., 2021), its role in the global health crisis (Ankrah et al., 2021; Chaturvedi et al., 2021; Chirisa et al., 2021; Donnarumma and Pezzulo, 2021; Hannam-Swain and Bailey, 2021; Prempeh, 2021; Sarfraz et al., 2021; Wang et al., 2021; Zhao and Zhou, 2021), the worst of all its impact on the mental wellbeing of people (Ciotti et al., 2020; Elmer et al., 2020; Filipova et al., 2020; Lee, 2020; Serafini et al., 2020; Adom et al., 2021; Chaturvedi et al., 2021; Coupet et al., 2021; Das and Bhattacharyya, 2021; Deshpande and Mhatre, 2021; Hannam-Swain and Bailey, 2021; Kareem, 2021; Li and Cao, 2021; Pandya and Lodha, 2021; Saha et al., 2021; Tonkin and Whitaker, 2021; Xiong et al., 2021), the role of online social media use to meet the social needs after the closure of physical social interactive places amid COVID-19 is critical (Haman, 2020; Jogezai et al., 2021; Yu et al., 2022). However, understanding the impact of COVID-19 on SME performance is critical. Then, our study is among the first to conduct a systematic survey on the impact of the COVID-19 pandemic on SMEs in a developing country, as many scholars only employed the qualitative survey or statistics descriptive to understand the impact of a pandemic. Overall, this research contributes to understanding those indicators most significantly affected by the pandemic. Indeed, the results of this study help quantify how the pandemic could generate different levels of impact on each indicator that could depend on the business and on what policymakers should consider as they contemplate the scale of the required intervention. They could then develop policy based on the impacted indicators, ensuring that policy measures are appropriately designed to meet the SME needs. Finally, by learning from the previous crisis, they could design an appropriate intervention to help SMEs survive, such as encouraging the SMEs to implement proactive sales to understand customer demand, building better customer and supplier relationships, and improving efficiency in resource utilization (Haluk Köksal and Özgül, 2007; Bourletidis and Triantafyllopoulos, 2014).
Literature review
Recently, the negative impact of the COVID-19 pandemic on SME performance became a topic of interest for many researchers. In detail, Table 1 shows the country and object of the study, the method, and the negative impact of the pandemic on SME’s performance were retrieved from each study.
According to Table 1, the impacts might vary according to the type of business activity. However, most authors concentrated on assessing the impact of COVID-19 on SMEs using descriptive statistics (Bartik et al., 2020; Humphries et al., 2020; Lu et al., 2020; Lutfi et al., 2020; Ratnasingam et al., 2020; Shafi et al., 2020; Abu Hatab et al., 2021; Dai et al., 2021). The negative impact of the COVID-19 pandemic on SMEs can be differentiated into six main categories: an employee-safe working environment (SWE), production process, markets and economic conditions, financial condition, public infrastructure, and political and regulatory environments.
(a) The pandemic has caused reduced labor productivity in an employee-SWE as employees cannot commute to work. It has also reduced the number of staff, reduced the number of working days, and increased work absenteeism as employees cannot return to work, or they refuse to work due to health concerns (Al-Fadly, 2020; Bartik et al., 2020; Humphries et al., 2020; Lu et al., 2020; Abu Hatab et al., 2021; Dai et al., 2021). In this case, employees are the heart of any organization, regardless of size or industry, which is why employee safety and well-being impact its short and long-term goals. As a result, the enterprise must maintain control over working conditions to provide a quality working environment for employees and increase a safe, positive atmosphere (Maamri et al., 2021).
Related to control over the working condition, according to Azizi et al. (2021), there are some practicing corporate social responsibility (CSR) in the context of human resource managers’ strategies for COVID-19 management, among others:
• Flexibility and employees’ virtual life cycle (such as flexibility of contracts, distance working, and working from home),
• Use innovative methods to support employees and maintain their health and welfare (such as helping and supporting employees by identifying where employees live; for an example, when employees have to cook for themselves),
• Use of staff safety measures and focusing on work conditions (such as the use of protective equipment, distribution of protective equipment to the employee at their discretion, observing of safety protocols by the employee, increasing the availability of testing health and safety of employees),
• Managers’ commitment (such as the obligation of administrative managers for supervision and monitoring during the working day in order to monitor the implementation of pandemic prevention measures, resolve disputes, obtain on-site feedback and take new measures),
• Selection and participation of the employee in decision-making (such as - creating an intimate atmosphere with employees and communicating with them by asking the question: (i) what factors cause your health to be good or bad here? (2) What factors can have a positive and not-so-positive effect on your health? (3) What factors can contribute to the health of the team/unit/employer?
• Making changes based on organization assessment and data (such as decision-making and continuous improvement of the safety, health, and well-being of employees by continuous monitoring carbon dioxide levels in indoor air, and the amount of fresh air in the workplace
Moreover, the company must meet the rights of its employees and society by preserving its workforce’s health and safety and improving working conditions (Ouffroukh et al., 2018). Occupational practitioners, healthcare advisers, and safety professionals should all be involved in developing adequate control measures, especially in the face of the new challenge of a pandemic situation (Maamri et al., 2021).
(b) In the production process, the pandemic has led to increased production costs, operational difficulties, permanent closure, operational disruption, underutilized or low production, deteriorating workflow and work environment (Al-Fadly, 2020; Humphries et al., 2020; Omar et al., 2020; Abu Hatab et al., 2021; Nawaiseh, 2021).
(c) In the market (on the demand side), the pandemic has led to decreased customer demand, sales, or orders, cancelation of export orders, and decreased sales turnover. However, on the supply side, the pandemic has led to difficulty in obtaining raw materials or services from suppliers; difficulty in supplier communications, increased cost of raw materials, logistical blocks, and supply chain disruptions (Al-Fadly, 2020; Fabeil et al., 2020; Lu et al., 2020; Lutfi et al., 2020; Mahajan, 2020; Omar et al., 2020; Ratnasingam et al., 2020; Shafi et al., 2020; Tairas, 2020; Abu Hatab et al., 2021; Dai et al., 2021; Saturwa et al., 2021). Supply-side is related to the entrepreneurial business network, a multifaceted network of business firms working together to achieve firm business objectives. (Eisenhardt and Martin, 2000; Ozcan and Eisenhardt, 2009). These objectives are typically operational and strategic, and business networks adapt them based on their role in the competitive environment in the market (Ford et al., 2014). There are two major categories of entrepreneurial business networks, namely business associations and business firm aggregations, which help SMEs become more dynamic, innovative, and competitive (Chung et al., 2015). An entrepreneurial business network is the socioeconomic business activity and a platform by which business executives and entrepreneurs meet to discuss available business network opportunities.
(d) Manufacturers are perpetually confronted with liquidity and profitability issues, and the COVID-19 pandemic has made them even more susceptible to economic shocks (Juergensen et al., 2020). During an economic storm, the manufacturing sector struggles with canceled orders, poor revenues, and falling stock prices (Handfield et al., 2020; Wuest et al., 2020; Tian et al., 2021). These market instabilities and unpredictability (Linton and Vakil, 2020; Paul and Chowdhury, 2020) cause panic in the industry, resulting in market anomalies and distorted supply-demand patterns (Khoo and Hock, 2020). So, to design an appropriate intervention, it is necessary to conduct a comprehensive assessment of the state of the businesses due to the new obstacles (Kapoor et al., 2021).
(e) In the financial condition of SMEs, the pandemic has led to decreased revenue, decreased profit, decreased financial liquidity, little cash on hand, reduced income, and cash flow risk or problems (Al-Fadly, 2020; Bartik et al., 2020; Fabeil et al., 2020; Lu et al., 2020; Omar et al., 2020; Ratnasingam et al., 2020; Shafi et al., 2020; Tairas, 2020; Nawaiseh, 2021). Generally, SMEs are financially fragile and smaller in size and resources; they are more vulnerable to the environmental crisis than their counterparts, i.e., large enterprises (Shafi et al., 2020). In this case, financial performance is an essential factor that may quantify economic pandemic effects on the companies’ function and existence. SMEs entrepreneurs more negatively perceived the financial performance of their companies during the pandemic as opposed to the period before the pandemic (Belas et al., 2021).
(f) In public infrastructure, the pandemic has led to a lack of transportation and un-operational services (Ratnasingam et al., 2020; Abu Hatab et al., 2021).
(g) In the political and regulatory environment, the pandemic has led to operational difficulties in businesses and the need for financial assistance (Al-Fadly, 2020; Abu Hatab et al., 2021).
Method of research
Study areas and sample of research
The object of research was SMEs in the Central Java Province. These SMEs were sampled to test the framework for measuring the impact of the COVID-19 pandemic on SME performance. There were 104 SMEs located in Semarang, Kudus, Jepara, Pati, Pekalongan, Magelang, Surakarta, Solo, and Cilacap that were sampled. Among the 104 sampled SMEs, 62.50% were in the furniture sector, 9.2% in the culinary sector, 2.88% in the handicraft sector, and 25% in the garment sector. Most of the sampled SMEs (102 SMEs or 98.08%) have fewer than 100 employees, and only two SMEs (1.92%) have over 100 employees.
This research employs non-probability convenient sampling to select the SMEs. The SMEs were selected from a community of individuals who were easy to reach or meet, such as friends, coworkers, classmates, and a WhatsApp group. The selection criteria also included their willingness to participate as respondents; they have been in operation for at least a year and were still operating throughout the COVID-19 pandemic. Although there are issues of generalizability in using the non-probability sample, developing research goals is scientifically warranted. Therefore, any sample coverage compromise is outweighed by the cost savings, convenience, target participant, and speed of response.
This study also used non-probability purposive sampling to choose respondents who completed the validation and analytic hierarchy process (AHP) questionnaire. These respondents should have sufficient knowledge of the impact of the pandemic on SME performance. This research comprised seven respondents who were willing to participate as the panel of experts. Those seven respondents are representatives from the Office of Cooperatives, Small and Medium Enterprises, Central Java Province (General Administration, Section Head of SME Production, Section Head of SME Financing, Division, Head of SME Program, Section Head of SME Marketing, Analyst of SME Financial Strategy, and an MSME consultant.
Variable and measurement items
Initially, this study identified 54 indicators for measuring the impact of COVID-19 on SME performance. These indicators were developed using previous research (related to the impact of the COVID-19 pandemic as perceived by SMEs) and the indicators from previous measurement frameworks issued by organizations, such as the International Trade Centre (ITC) (International Trade Centre, 2020), Enterprise Survey for Innovation and Entrepreneurship in China (ESIEC) (ESIEC, 2020), and the International Labour Organization (ILO) (International Labour Organization, 2020). Given the content validity results, the number of indicators used to assess the impact of COVID-19 on SME performance was reduced from 54 to 44. Accordingly, the validation questionnaire had to be completed by a panel of experts with knowledge of the indicators for measuring the COVID-19 impact on SME performance. The panel had to evaluate if indications were relevant for measuring a particular dimension, establishing the domain of interest, and determining field conditions. The Content Validity Index (CVI) value was then used to assess the indicators’ validity (Haas and Springer, 2020). The CVI value for each indication is calculated by dividing the number of experts who gave a rating of 3 (relevant) or 4 (very relevant) for that indicator by the total number of experts, that is, the proportion of experts who agreed on relevance. For example, an item with a CVI of 0.80 would be judged as “relevant” or “very relevant” by four out of five assessors (Lynn, 1986). Haas and Springer (2020) established widely referenced standards for what constitutes an appropriate CVI value regarding the number of experts. When there are five or fewer experts, they believe the CVI should be 1.00, meaning that all experts must agree that the indicator is valid.
Table 2 shows the results of the CVI computation for each indicator. Only indicators with a CVI of 0.867 or above were kept in this study. As seven experts were involved in the validation process, a CVI of 0.867 or above shows that the indicator is valid if no more than one expert disagreed with the indicator’s relevance. The following indicators were removed from the listed indicators for measuring the impact of the COVID-19 pandemic on SME performance: SWE5, SWE6, SWE7, SWE10, SWE11, SWE16, PPF1, EEM4, PUI2, and PAR4. So, after the content validation process, 44 indicators were used to measure the impact of the COVID-19. Then, the hierarchical structure of indicators for measuring the impact of the COVID-19 pandemic on SME performance can be seen in Figure 1 below.
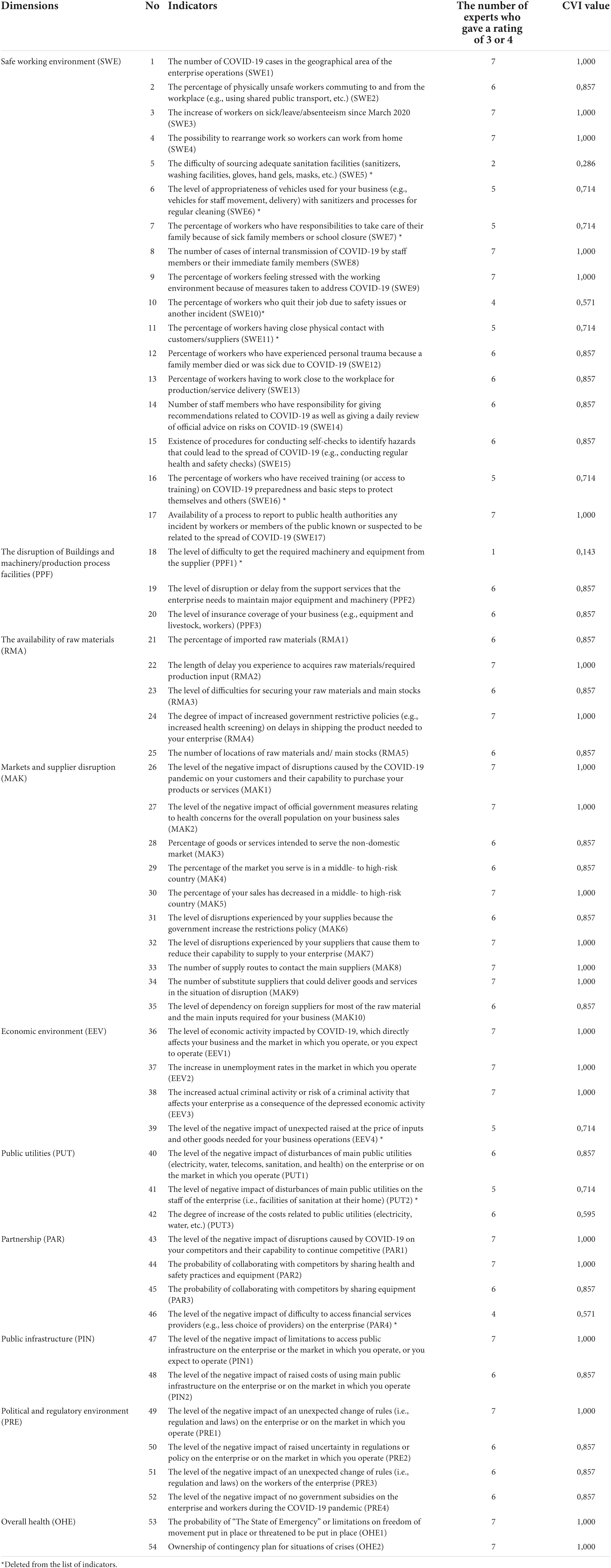
Table 2. The results of the content validity of the indicators for measuring the impact of COVID-19 on SME performance.
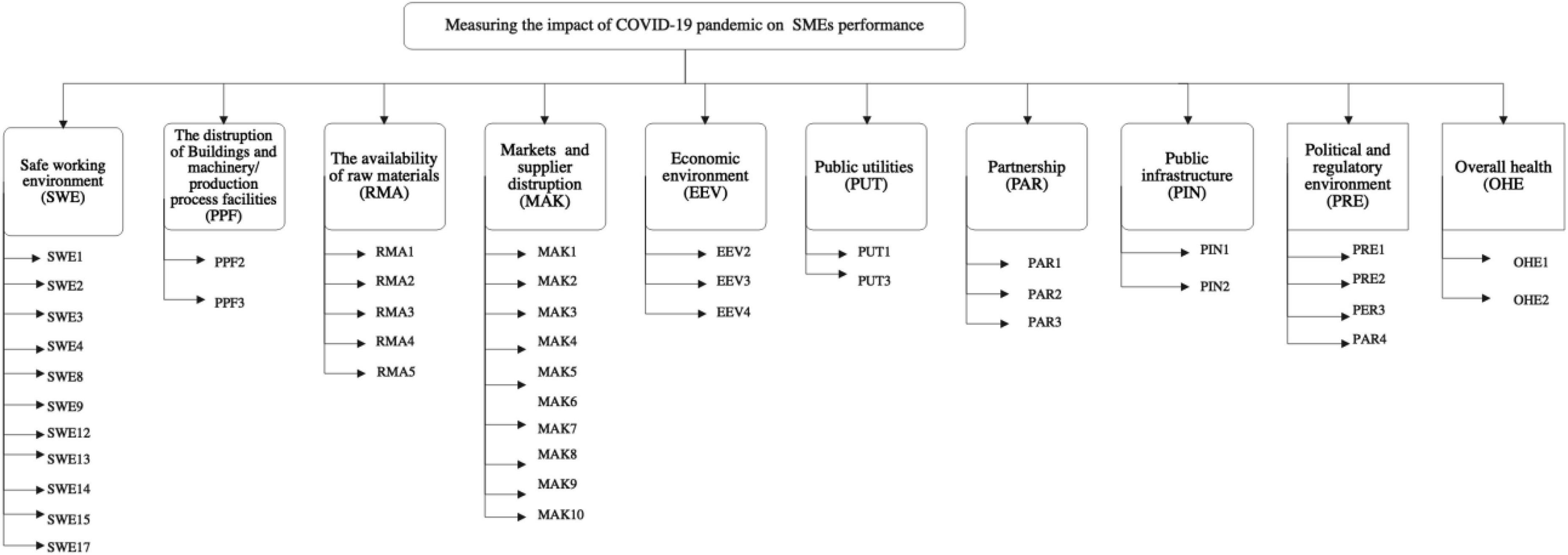
Figure 1. Hierarchical structure of indicators for measuring the impact of COVID-19 pandemic on SMEs performance.
Data collection procedure
Two sources of data, primary and secondary data, were used in this research. The primary data sources were the results of the completed web-based questionnaire and short telephone interviews with the SME owner and a representative from the Office of Cooperatives, Small and Medium Enterprises, Central Java Province. The purpose of the interview is to clarify the results of filling out the questionnaire by SMEs so that the respondents who fill out the questionnaire and those interviewed are the same SMEs. Then, the secondary data is intended to complete the documents (such as data related to sales, profit, etc.) that may be required based on the questionnaire results and interviews.
Three types of questionnaires were developed in this research. The first was a validation questionnaire with a 4-point Likert scale (1 = not relevant, 2 = slightly relevant, 3 = relevant, and 4 = very relevant) to assess the validity of the 54 indicators for measuring the impact of the COVID-19 pandemic on SME performance. Second, an AHP questionnaire with Saaty’s 9-point scale (Saaty, 1995) assessed the level of importance of each dimension and indicator. Third, a closed-ended questionnaire measured the current condition of each indicator. The third questionnaire also applied a 4-point Likert scale with different meanings, as detailed in Table 3. Both the second and third questionnaires only used valid indicators (44 indicators out of 54 indicators). All the questionnaires were web-based, developed, and produced using Google Forms. Google Forms is a cloud-based data management tool freely available on the Internet by Google Inc. It may be used and created by anybody using the Internet (Vasantha Raju and Harinarayana, 2016).
The seven URL copies of the first and second questionnaires were distributed to seven respondents through their emails, which consisted of the representative of the Office of Cooperatives, Small and Medium Enterprises, Central Java Province. In this case, the seven respondents should fill out a validation questionnaire (first questionnaire) by giving a value with a range of 1–4 on each of the indicators asked. Furthermore, approximately 2 weeks later, the seven respondents had to fill out the AHP questionnaire (second questionnaires) with a value of 1–9 to assess the importance level of each dimension and indicator. Then, the 125 URL copies of the third questionnaires were distributed to the SMEs through the owners’ emails or other social media accounts. In this case, the owners of SMEs should fill out the questionnaire by giving a value in the range of 1–4 according to the condition faced by the SMEs asked by each indicator. Although email distribution is thought to limit distribution to individuals with computers and email accounts, this was not the case in this study as the respondents’ profiles matched the survey requirements. There were also short telephone interviews with the representative of the Office of Cooperatives, Small and Medium Enterprises, Central Java Province, and the SME owners to further investigate the reasons for choosing a specific value or scale in the questionnaire.
Shortly, referring to Abbas et al. (2019), this study presents three phases of the data collection procedure. In the first step, this study selected the dimensions and indicators for assessing the impact of the COVID-19 pandemic on SMEs’ performance. In phase two, this study planned a pilot study to examine and understand the reliability of indicators and modified it accordingly if needed (first questionnaire or validation questionnaire). In the third and final phase, we conducted the survey and received data from respondents about the level of importance of each dimension and indicator (second questionnaire or AHP questionnaire). In the third phase, this study also conducted a survey and received data from respondents about the condition of each indicator perceived by the SMEs (third questionnaire). The three phases of the data collection procedure can be seen in Figure 2.
Data processing procedure
Several different methods were used to analyze the data. The data from the first type of questionnaire were examined using content validity analysis (Lynn, 1986). The data from the second type of questionnaire were analyzed using AHP (Saaty, 1995). The data from the third type of questionnaire were examined using several approaches, such as simple descriptive statistics (statistical mean and simple graphics display), Multi-Attribute Value Theory (MAVT) approach (Keeney et al., 1993; Riabacke et al., 2012; Bottero et al., 2016), K-means cluster analysis (Xu and Tian, 2015), and discriminant analysis (Alayande and Adekunle, 2015; Keskin et al., 2020).
Result and discussion
Result of data processing with analytic hierarchy process
In this study, the AHP questionnaire evaluated the level of importance of each dimension and indicator to measure the impact of the pandemic on SME performance. Data were initially processed using AHP with a disseminated pairwise questionnaire given to the panel of experts, consisting of seven respondents or decision-makers. Each of the seven decision-makers initially completed the pairwise comparison at dimension and indicator levels separately. Then, the geometric mean of an individual’s judgments of the level of importance of each dimension and indicator was used to obtain a single value for each dimension and indicator. The geometric mean is used to find a combination of the answer of all decision-makers. In this case, the geometric mean was used to avoid a biased attitude of decision-makers toward a particular importance level (Dyer et al., 1992). Finally, each dimension’s global priority weight, the indicator’s local priority weight, and the consistency index (CI) were computed using the base of the combined or single value of pairwise comparisons data.
At level dimensions, based on the rearranged priority weight in descending order, the ranks of dimensions are as follows: SWE (0,2578), overall health (OHE) (0.2412), availability of raw material (RMA) (0.1147), market and supplier disruption (MAK) (0.1131), political and regulatory environment (PRE) (0.0965), partnership (PAR) (0.0582), the disruption of building and machinery production process facilities (PPF) (0.0476), economic environment (EEV) (0.1131), public utilities (PUT) (0.0184), and public infrastructure (PIN) (0.0965). In detail, each indicator’s local and global priority weight can be seen in the Supplemetary Appendix.
In the case of a SWE, research conducted by Zhou et al. (2021) suggests non-pharmaceutical interventions (NPIs) to combine various measures, such as the suppression strategy (lockdown and restrictions) and mitigation model, to decrease the burden on health systems. NPIs are significantly practical and helpful in reducing the quick transmission of the deadliest diseases. However, implementing the social distancing strategy is incredibly effective and beneficial, more so than other NPIs, to contain the rapid spread of the coronavirus. As a result, two or more synchronous NPIs are more productive and valuable than a single NPI strategy. In addition, the literature indicated that NPIs help significantly contains the rapid spread of the COVID-19 transmission. Based on the above debate, social distancing and implementing two or more NPI strategies can significantly contain the quick spread among people. Therefore, these strategies should be the priority in the ongoing panic situation of COVID-19.
Result of data processing with multi-attribute value theory
Based on the local priority weight of each indicator and its measurement scale, the level of vulnerability of each SME during the pandemic can be assessed using the MAVT approach. Although MAVT includes different aggregation models, the simplest and most used one is the additive model (Belton and Stewart, 2002) as represented in the equation: V(a) = wi × vi(ai), where V(a) is the total value given to certain SMEs by considering all indicators simultaneously; wi is the weight assigned to reflect the importance of indicator i, and vi(ai) is the value reflecting certain SME performance on indicator i.
As seen in Figure 3, only 13 of 44 indicators have a good performance. These indicators have a mode value of 1 or 2. However, most of the indicators proposed in this research have a mode value of 3 or 4. Accordingly, most of the proposed indicators have poor performance during the pandemic. There are 12 indicators with a mode value of 4 (the worst condition), namely SWE4, SWE13, PPF3, MAK1, MAK2, MAK4, MAK5, PAR1, PAR2, and OHE1.
This study was only able to collect the results of filling out questionnaires from 104 SMEs from 125 SMEs or a response rate of 82.3%. So, based on this condition, the histogram and descriptive statistics of the total value given to each of the 104 SMEs, by considering all indicators simultaneously, can be seen in Figure 4. This total value indicates the level of vulnerability of SMEs during the COVID-19 pandemic. The maximum number of vulnerability values achieved by each of the 104 SMEs is 100, as we converted marks from 4 to 100. We divided the total value achieved by four and then multiplied it by 100. Regarding the scale used by each indicator: the higher the total value given to SMEs, the more vulnerable the SME during the COVID-19 pandemic.
The average value of the level of vulnerability is 63.6382, with a median of 64.9706, a mode of the smallest value) 54.59, and a standard deviation of 8.798. The value of the level of vulnerability has a positive kurtosis value, indicating a “heavy-tailed” distribution.
Result of data processing with cluster analysis
The K-means clustering algorithm segmented the level of vulnerability of SMEs because of the impact of the COVID-19 pandemic on SME performance. The values reflecting the SME performance for each of the 44 indicators were used as inputs in this algorithm. Referring to the value of the silhouette index (SI), the optimal number of segments equals 4 (four), meaning that four clusters have the highest SI value among other selected numbers of clusters (this study tried a different number of clusters, i.e., 2, 3, …, 6.). Xiong and Li (2013), Moulavi et al. (2014), and Tomasini et al. (2016) have compared SI with a set of other internal measures and proven it to be one of the most effective and generally applicable measures for clustering validity evaluation. The name of each cluster accords with ILO terminology (International Labour Organization, 2020), that is, resilient, low vulnerability, moderate vulnerability, and high vulnerability. Resilient means the SME is on the right path toward becoming more resilient, but some factors will reduce the SME’s vulnerability. Low vulnerability means the SME has increased preparedness, although it remains vulnerable. Moderate vulnerability means the SME has increased preparedness, but it remains vulnerable. High vulnerability means the SME was severely affected, which may cause long-term disruption should the situation deteriorate.
The average total vulnerability value of each cluster is shown in Figure 5 below. The SME profile in each cluster can be seen in Table 3, and the characteristics of the impact of the pandemic according to their dimensions in each cluster are shown in Table 4. Moreover, Figure 6 shows the radar chart comparing all segments according to the characteristics of the COVID-19 pandemic impact on each dimension. According to Figure 5, the average total value of vulnerability with the highest score is cluster 3 (69,4075), followed by cluster 4 (62.8176), cluster 2 (61,94815), and cluster 1 (50.0825). Thus cluster 1 is termed a resilient cluster. Cluster 2 is a low vulnerability cluster, and cluster 3 is a high vulnerability cluster. Cluster 4 is a moderate vulnerability cluster.
Referring to Table 3, cluster 1, or the resilient cluster, comprises 11 SMEs (10.58%) and SMEs in the furniture and garment sectors in a domestic market dominate. Most of the SMEs in cluster 1 have fewer than 15 employees. Cluster 2, or the low vulnerable cluster, comprises six SMEs (5.71%), SMEs in the furniture sector with a domestic market dominant. Like cluster 1, most SMEs in cluster 2 have fewer than 15 employees. Cluster 3, or the high vulnerability cluster, comprises 35 SMEs (33.65%), with domestic and overseas markets, where SMEs in the furniture sector dominate. Most of the SMEs in this cluster, about 77%, have over 30 employees. Cluster 4, or the moderate vulnerability cluster, comprises 52 SMEs (52%), where SMEs in the furniture sector dominate in domestic and overseas markets. The number of employees who worked in SMEs in cluster 4 varied significantly. About 32.69% of SMEs in cluster 4 have fewer than 15 employees, 15.38% of SMEs in cluster 4 have 15–30 employees, and 51.92% of SMEs in cluster 4 have more than 30 employees. As shown in Table 4 and Figure 6, cluster 3 has the highest impact in almost all dimensions of the clusters.
Result of data processing with discriminant analysis
After conducting the clustering analysis to obtain the number of parts, the discriminant analysis was performed to determine any significant differences between the parts. The analysis also built a predictive model for each segment, group, or case membership through identification, using the best independent variables or indicators to distinguish between two or more segments, groups, or cases. These independent variables form a distinctive discriminant function (Alayande and Adekunle, 2015; Keskin et al., 2020).
In this study, the discriminant analysis started with calculating Wilk’s lambda to test the equality of the groups’ means for the same variables or indicators. Wilk’s lambda value varies from 0 to 1, where 0 indicates the differences in the parts’ means, and one indicates the similarity of all the segment means. The smaller the lambda value, the more the variable contributes to the discriminant function (Poulsen and French, 2004; Balogun et al., 2014, 2015). The Wilk’s lambda test statistic is designed to determine the discriminant function that maximizes the quotient between the variation explained by the difference between the segment means and the variation within these segments. As shown in Table 5 below, the smallest value of Wilk’s lambda is the MAK 7 indicator (the level of disruptions experienced by your suppliers that causes the supplier to reduce supply capability to your enterprise). It implies that this indicator is the one that provides the most significant difference between the means of the segments.
The next step was to check the critical discriminating variables by entering a variable into the SPSS software or removing it from the SPSS software (see Figure 7 for the result). There were 16 indicators that could identify the differences between the four clusters. The 16 variables are PUT1, MAK10, OHE1, PRE2, EEV2, MAK8, SWE15, PIN1, RMA5, RMA1, MAK7, MAK2, PRE1, EEM1, and MAK9.
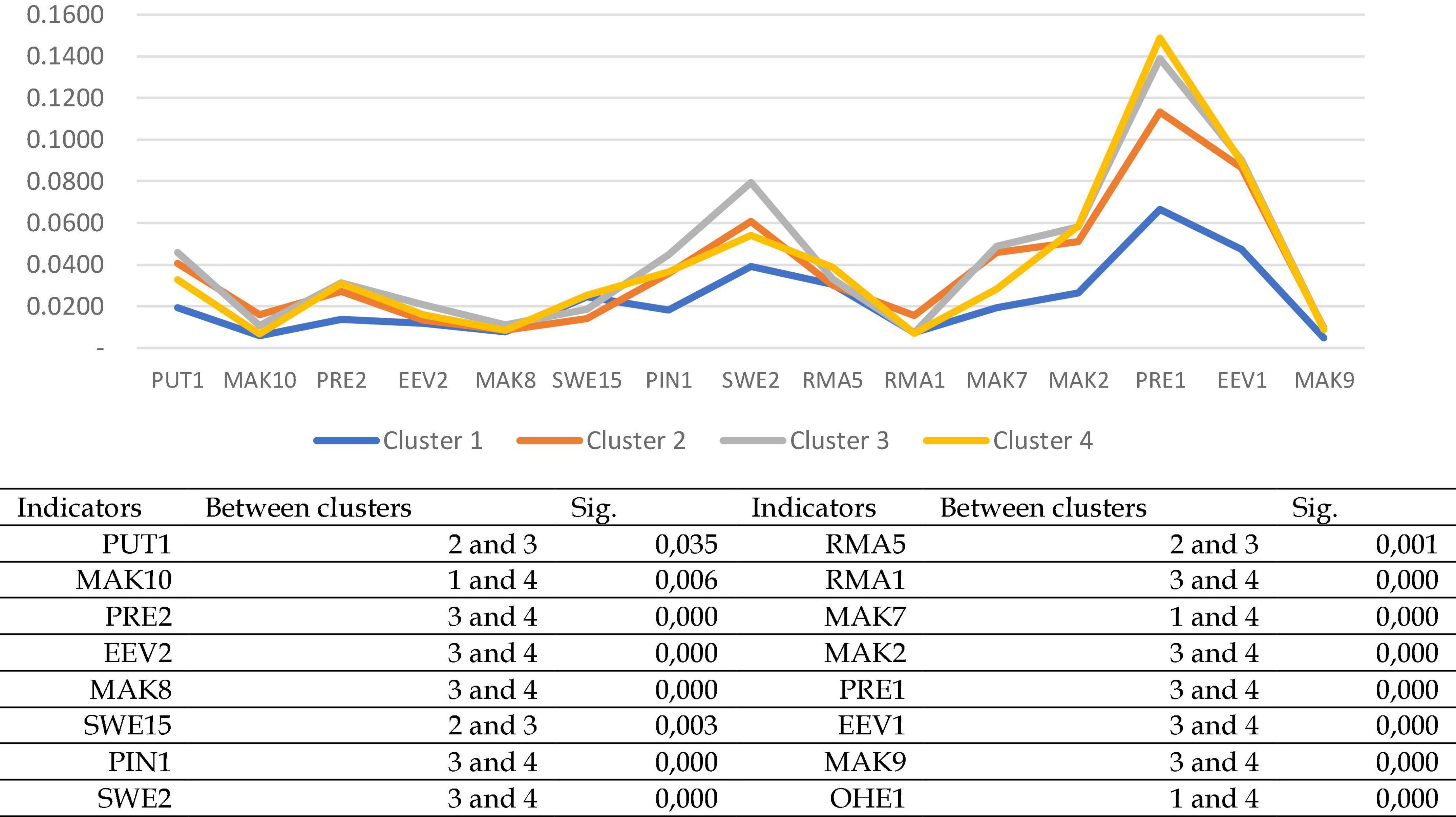
Figure 7. The result of checking the best indicators to identify four cluster segments in each function by entering or removing a variable.
Based on the indicators to identify the four clusters, the Fisher’s linear discriminant function (FLDF) was arranged using SPSS software to predict the location of SMEs in a particular cluster. This function (FLDF) is frequently used for discrimination, classification, and prediction purposes under the usual basic statistical assumptions of multivariate normality of the independent variables, equality of variance and covariance matrices, and the relative equality of group sample sizes. Moreover, Fisher’s linear discriminant analysis technique was used to fit a predictive equation based on the measured variables for classifying new individuals and re-classifying the original data to interpret the predictive equation and better understand the relationships among the variables (Dibal and Abraham, 2020). The results of the FLDF from the SPSS software can be seen in Table 6.
Based on Table 6, the equation for the level of vulnerability of cluster 1 is:
For example, the SME’s performance is as follows during the -19 pandemic:
• The percentage of physically unsafe workers commuting to and from the workplace is 0–10% (e.g., using shared public transport) (SWE2 = 4)
• Most of the areas already have procedures to conduct self-inspections to identify hazards that could lead to the spread of COVID-19 (e.g., conducting regular health and safety checks) (SWE15 = 2)
• The percentage of imported raw materials is less than 5% (RMA1 = 1)
• The negative impact of official government measures relating to health concerns for the overall population on your business sales caused sales of the product to decrease by more than 20% (MAK2 = 4)
• The level of disruptions experienced by suppliers caused 50% or more of the main suppliers to be unable to supply input to the enterprise (MAK7 = 4)
• The enterprise has two substitute suppliers that could deliver goods and services in case of disruption (MAK9 = 3)
• Less than 25% of critical inputs and raw materials need to come from foreign suppliers (MAK10 = 1)
• Effect of COVID-19 on economic activity has a high impact on the business or the markets you operate in, or you expect it to (EEV1 = 4)
• The unemployment rates in the markets in which you operate are 5% to 25% (EEV2 = 4)
• Restrictions to accessing public infrastructure have a significant impact on your business or the markets you operate in, or you expect it to (you have to close your operation) (PIN1 = 4)
• Increased costs of using key public infrastructure have a moderate impact on business or the markets you operate in, or you expect it to (you can operate at half of your capacity) (i.e., regulation and laws) on the enterprise or on the market in which you operate (PRE1 = 3)
• Increased uncertainty in the policy or regulatory environment has a significant impact on your business or the markets you operate, or you expect it to (you have to close your operation) (PRE2 = 4)
According to this information and equations 1–4, each cluster’s vulnerability level can be calculated. The calculation results indicated that the total value for the levels of vulnerability for cluster 1 to cluster 4 is 155.3039, 167.4348, 188.2879, and -48.7982, respectively. The calculation results indicated that the SMEs belonging to cluster 3 have high vulnerability as the value for the level of vulnerability for cluster 3 is higher than the others.
Conclusion, theoretical implication, and practical implication
Conclusion
This research conducted a quantitative analysis to answer the first three research questions. The results demonstrated how to design a framework to measure SME vulnerability in terms of its performance caused by the effects of the COVID-19 pandemic. The results also demonstrated how to segment the SMEs based on the performance of the indicators that were impacted by the COVID-19 pandemic. The measurement framework consisted of 44 indicators belonging to 10 dimensions. It was conducted using the MAVT approach, and the segmentation was conducted using the K-means clustering algorithm and discriminant analysis. The data on the impact of the pandemic on SME performance was collected through online web-based questionnaires completed by 104 SMEs. Those SMEs were located in several regions in the Central Java Province. The segmentation generated four clusters: resilient cluster, low vulnerability cluster, moderate vulnerability cluster, and high vulnerability cluster. Most of the surveyed SMEs fell into the moderate and high vulnerability clusters.
The differences between the clusters relied on 16 indicators. However, the differences between the low and moderate clusters relied on the ownership procedures for carrying out self-checks to identify hazards that could lead to the spread of the COVID-19 disease; the number of locations of suppliers or raw materials; and the level of impact of disruption of key public utilities on SME businesses. For comparison, most SMEs in the moderate vulnerability level already have procedures to conduct self-inspections. The market in which those SMEs operate was less affected by key public utility disruptions, and those SMEs have several suppliers in different locations. Consequently, if one of their suppliers cannot work because of policy restrictions or other operational reasons, the SMEs still have other suppliers to guarantee their production process. For example, the SMEs would have to use other suppliers who could deliver material if they could obtain material from their regular suppliers.
The differences between the moderate vulnerability cluster and the high vulnerability cluster rely on the levels of the impact of uncertainty in policy or regulatory processes; unexpected rule changes; official government measures relating to health concerns; limitation to public infrastructure access, and the level of impact the number of physically unsafe workers’ commuting to and from the workplace has on the SME business or on the market in which the SME operates. The differences between moderate and high vulnerability clusters also rely on the negative impact of unemployment on the SME’s market, the number of substitute suppliers, and the main supplier’s supply route. On average, SMEs in the high vulnerability cluster have a moderate impact because they decreased their sales by more than 20%. The raw material supply for production was disrupted as they used 25–50% less imported raw material. Indeed, the capability of SMEs and the market in which the SMEs operate to manage the sudden changes in government regulations and the ease of SMEs in getting raw materials for production became important factors that differentiated between being a resilient or a high vulnerability cluster.
Theoretical implication and practical implication
This research contributes both to theory and practice. Theoretically, this research contributes to the literature on multi-criteria decision making, performance management, and the effects of the COVID-19 pandemic on SMEs in four ways. First, this research synthesized the findings of the reviewed studies of the impact of the pandemic on SME performance by grouping them into six main themes consisting of various SME-related issues, such as employee- SWEs, production processes, markets, and economic conditions, financial conditions, public infrastructure, and the political and regulatory environment. Second, this research synthesized the findings of the reviewed studies on the pandemic’s impact on SMEs by developing a framework based on multi-criteria decision-making for measuring the level of SME vulnerability caused by the effect of the pandemic on their operations. Third, this research found how the framework can help cluster SMEs into four main clusters: resilient, low vulnerability, moderate vulnerability, and high vulnerability. Accordingly, this research attempts to improve the understanding of the indicators in question: which indicators help differentiate the impact of the COVID-19 pandemic and provide a guide for future studies in this area. Fourth, in addition to clustering the SMEs, this research determined the equation to assess which cluster an SME can be placed in based on its indicator performance. Fifth, in addition to summarizing this research’s knowledge of the COVID-19 pandemic and SMEs, it outlined how this knowledge was acquired (methodology) and in which contexts the knowledge applies. These findings can help shape decisions about methodology and context in future work. Finally, this research identified gaps in the study domain of the impact of the COVID-19 pandemic on SME performance and suggested unique research questions and opportunities for meaningful future research to fill those gaps.
Practically, the results of this study make recommendations for SMEs and the government. Using a measurement framework and the discriminant equation that resulted from this research, SMEs can understand their level of vulnerability in terms of specific impacts of the COVID-19 pandemic. Thus, SMEs can adapt to the new situation quickly and reduce damage. However, one of the most important conclusions of the current research is that supplier and market disruptions can make SMEs more vulnerable. To reduce SME vulnerability during the COVID-19 pandemic, the SME management or owner should proactively and informatively communicate with their suppliers and customers.
The SMEs should also have a contingency plan to buy from numerous suppliers, changing from foreign to domestic suppliers. It is suggested that SMEs propose innovative marketing by adopting digital media and using the Internet for business operations. They should also manage customer loyalty as the pandemic has negatively affected business sales. By embracing digital media or the Internet, SMEs can present their products effectively despite customers being unable to visit their business premises. Consumers can have a thorough understanding of what products are available and which products they want. Consumers can communicate online with sellers about products without restraint during the pandemic. Furthermore, digital media enables users to display buyer testimonies, record the number of visitors, and make specific offers to consumers. These activities have proven effective in creating sales, attracting new customers, and maintaining SME performance.
Moreover, to manage customer loyalty, SMEs should offer more reasonable prices given high unemployment levels or other changes in regulations that could affect their markets. The SME should also consider designing alternatives for customers in restricted areas, such as providing collection and delivery services. The SME management or owner should maintain long-term relationships with customers, which are vital to maintaining sales. Strengthening customer relationships may help SMEs to maintain their performance.
It is recommended that the government consider the current situation and the level of SME vulnerability and reduce tax and other costs. Given their vulnerability, the government should offer SMEs a stimulus package to avoid enterprise closures and bankruptcies. The government should also assist SMEs in distributing their products or using SME services. The sudden changes in government regulations can affect SMEs or the markets they operate in or expect to operate. The most common type of assistance received by SMEs was the distribution of goods.
Limitation and future research
Finally, we acknowledge some limitations of our study concerning the sample and employed techniques, which future research should address. First, this study was limited geographically. The sample in our study included a small number of cases, limited to only SMEs in several regions in the Central Java Province. Second, we only measured the impact of the COVID-19 pandemic on general SMEs and did not focus on particular sectors or industries. The inclusion of specific sectors or industries may identify a specific impact on how that sector or industry will be more severely affected than others. This limitation may also reveal an opportunity to compare resilient and vulnerable SMEs in different sectors or industries, thus generating a more reliable response. Future research could benefit from replicating our work in similar and dissimilar contexts: in each sector, business activity and size, and a wider geographical area could be considered, including a nationwide study. Such research could allow for more accurate measurements of the pandemic’s impact on each indicator’s performance based on sector or industry.
Future studies could adopt a complementary regression approach (OLS and PLS) and the ISM approach regarding the quantitative analysis techniques. In this case, the OLS and PLS estimations could determine the relationship between one or more explanatory variables (such as a SWE, the readiness of building and machinery or production process facilities, the availability of raw material), and the SME financial performance during the pandemic. Finally, the ISM approach could help us articulate the mental models of the relationship between the indicators used in this research.
Data availability statement
The original contributions presented in this study are included in the article/Supplementary material, further inquiries can be directed to the corresponding author.
Ethics statement
The study protocol was reviewed and approved by the Review Board of Industrial Engineering Department, Diponegoro University. Written informed consent from the participants was not required to participate in this study in accordance with the national legislation and the institutional requirements.
Author contributions
AS, NP, and AB conceived and designed the study. FP participated in the acquisition of data. AS, NP, and FP analyzed the data, gave advice on methodology, and drafted the manuscript. AS and AB revised the manuscript. AS was the guarantor of this work and had full access to all the data in the study and takes responsibility for its integrity and the accuracy of the data analysis. All authors read and approved the final manuscript.
Funding
The authors would like to thank the Rector of Diponegoro University and Head of Institute for Research and Head Community and for funding this research under the “International Scientific Publication”, budget year 2021.
Conflict of interest
The authors declare that the research was conducted in the absence of any commercial or financial relationships that could be construed as a potential conflict of interest.
Publisher’s note
All claims expressed in this article are solely those of the authors and do not necessarily represent those of their affiliated organizations, or those of the publisher, the editors and the reviewers. Any product that may be evaluated in this article, or claim that may be made by its manufacturer, is not guaranteed or endorsed by the publisher.
Supplementary material
The Supplementary Material for this article can be found online at: https://www.frontiersin.org/articles/10.3389/fpsyg.2022.927628/full#supplementary-material
References
Abbas, J., Raza, S., Nurunnabi, M., Minai, M. S., and Bano, S. (2019). The impact of entrepreneurial business networks on firms’ performance through a mediating role of dynamic capabilities. Sustainability 11:3006. doi: 10.3390/su11113006
Abu Hatab, A., Lagerkvist, C. J., and Esmat, A. (2021). Risk perception and determinants in small- and medium-sized agri-food enterprises amidst the COVID-19 pandemic: Evidence from Egypt. Agribusiness 20, 187–212. doi: 10.1002/agr.21676
Aditya, D. S. (2021). Embarking Digital Learning Due to COVID-19: Are Teachers Ready? J. Technol. Sci. Educ. 11, 104–116. doi: 10.3926/jotse.1109
Adom, D., Mensah, J. A., and Osei, M. (2021). The psychological distress and mental health disorders from COVID-19 stigmatization in Ghana. Soc. Sci. Hum. Open 4:100186. doi: 10.1016/j.ssaho.2021.100186
Akbulaev, N., Mammadov, I., and Aliyev, V. (2020). Economic impact of COVID-19. SSRN Electron. J. 164, 113–126. doi: 10.2139/ssrn.3649813
Akpan, I. J., Soopramanien, D., and Kwak, D. H. (2020). Cutting-edge technologies for small business and innovation in the era of COVID-19 global health pandemic. J. Small Bus. Entrepreneurship 32, 1–11. doi: 10.1080/08276331.2020.1799294
Alayande, S., and Adekunle, B. (2015). An overview and application of discriminant analysis in data analysis. IOSR J. Math. 11, 12–15.
Al-Fadly, A. (2020). Impact of covid-19 on SMEs and employment. Entrepreneurship Sustain. Issues 8, 629–648. doi: 10.9770/jesi.2020.8.2(38)
Ali, M., Gasca, V., Schrier, R., Pensa, M., Brockman, A., Olson, D. P., et al. (2021). Social determinants and COVID-19 in a community health center cohort. J. Immigr. Minor. Health 24, 10–17. doi: 10.1007/s10903-021-01320-6
Ankrah, D. A., Agyei-Holmes, A., and Boakye, A. A. (2021). Ghana’s rice value chain resilience in the context of COVID-19. Soc. Sci. Hum. Open 4:100210. doi: 10.1016/j.ssaho.2021.100210
Azizi, M. R., Atlasi, R., Ziapour, A., Abbas, J., and Naemi, R. (2021). Innovative human resource management strategies during the COVID-19 pandemic: A systematic narrative review approach. Heliyon 7:e07233. doi: 10.1016/j.heliyon.2021.e07233
Balogun, O. S., Akingbade, T. J., and Oguntunde, P. E. (2015). An assessment of the performance of discriminant analysis and the logistic regression methods in classification of mode of delivery of an expectant mother. J. Math. Theory Model. 5, 147–154.
Balogun, O. S., Balogun, M. A., Abdulkadir, S. S., and Jibasen, D. (2014). A comparison of the performance of discriminant analysis and the logistic regression methods in classification of drug offenders in Kwara State. Int. J. Adv. Res. 2, 280–286.
Bartik, A. W., Bertrand, M., Cullen, Z., Glaeser, E. L., Luca, M., and Stanton, C. (2020). The impact of COVID-19 on small business outcomes and expectations. Proc. Natl. Acad. Sci. U. S. A. 117, 17656–17666. doi: 10.1073/pnas.2006991117
Beck, T., and Demirguc-Kunt, A. (2006). Small and medium-size enterprises: Access to finance as a growth constraint. J. Bank. Financ. 30, 2931–2943. doi: 10.1016/j.jbankfin.2006.05.009
Belas, J., Gavurova, B., Dvorsky, J., Cepel, M., and Durana, P. (2021). The impact of the COVID-19 pandemic on selected areas of a management system in SMEs. Econ. Res. 35, 1–24. doi: 10.1080/1331677X.2021.2004187
Belton, V., and Stewart, T. J. (2002). Multiple Criteria Decision Analysis: An Integrated Approach. Boston: Kluwer Academic Press. doi: 10.1007/978-1-4615-1495-4
Bhattacharya, M., and Banerjee, P. (2021). COVID-19: Indices of economic and health vulnerability for the Indian states. Soc. Sci. Hum. Open 4:100157. doi: 10.1016/j.ssaho.2021.100157
Bottero, M., Mondini, G., and Oppio, A. (2016). Decision support systems for evaluating urban regeneration. Procedia Soc. Behav. Sci. 223, 923–928. doi: 10.1016/j.sbspro.2016.05.319
Bourletidis, K., and Triantafyllopoulos, Y. (2014). SMEs survival in time of crisis: Strategies, tactics and commercial success stories. Procedia Soc. Behav. Sci. 148, 639–644. doi: 10.1016/j.sbspro.2014.07.092
Bretas, V. P. G., and Alon, I. (2020). The impact of COVID-19 on franchising in emerging markets: An example from Brazil. Glob. Bus. Organ. Excell. 39, 6–16. doi: 10.1002/joe.22053
Carruthers, P. (2020). How coronavirus can kill small businesses: COVID-19. Pers. Financ. 2020, 8–10.
Chaturvedi, K., Vishwakarma, D. K., and Singh, N. (2021). COVID-19 and its impact on education, social life and mental health of students: A survey. Child. Youth Serv. Rev. 121:105866. doi: 10.1016/j.childyouth.2020.105866
Chirisa, I., Mavhima, B., Nyevera, T., Chigudu, A., Makochekanwa, A., Matai, J., et al. (2021). The impact and implications of COVID-19: Reflections on the Zimbabwean society. Soc. Sci. Hum. Open 4:100183. doi: 10.1016/j.ssaho.2021.100183
Chung, H. F., Yang, Z., and Huang, P. H. (2015). How does organizational learning matter in strategic business performance? The contingency role of guanxi networking. J. Bus. Res. 68, 1216–1224. doi: 10.1016/j.jbusres.2014.11.016
Ciotti, M., Ciccozzi, M., Terrinoni, A., Jiang, W. C., Wang, C. B., and Bernardini, S. (2020). The COVID-19 pandemic. Crit. Rev. Clin. Lab. Sci. 57, 365–388. doi: 10.1080/10408363.2020.1783198
Coupet, S., Nicolas, G., Louder, C. N., and Meyer, M. (2021). When public health messages become stressful: Managing chronic disease during COVID-19. Soc. Sci. Hum. Open 4:100150. doi: 10.1016/j.ssaho.2021.100150
Cuschieri, S., and Grech, V. (2021). Protecting our vulnerable in the midst of the COVID-19 pandemic: Lessons learnt from Malta. Public Health 198, 270–272. doi: 10.1016/j.puhe.2021.07.043
Dai, R., Feng, H., Hu, J., Jin, Q., Li, H., Wang, R., et al. (2021). The impact of COVID-19 on small and medium-sized enterprises (SMEs): Evidence from two-wave phone surveys in China. China Econ. Rev. 67:101607. doi: 10.1016/j.chieco.2021.101607
Das, M., and Bhattacharyya, A. (2021). Social distanciation through COVID-19: A narrative analysis of Indian Peri-Urban Elderly. Soc. Sci. Hum. Open 4:100139. doi: 10.1016/j.ssaho.2021.100139
Delbiso, T. D., Kotecho, M. G., and Asfaw, F. M. (2021). Effects of COVID-19 imposed school closure on school feeding program in Addis Ababa. Ethiopia. Soc. Sci. Hum. Open 4:100185. doi: 10.1016/j.ssaho.2021.100185
Deshpande, D., and Mhatre, C. K. (2021). A study of impact of online education on mental health and academic performance of children of project affected people studying at undergraduate level in navi mumbai. Rev. Geint. 11, 3866–3875. doi: 10.47059/revistageintec.v11i4.2412
Dibal, P. N., and Abraham, A. C. (2020). On the application of linear discriminant function to evaluate data on diabetic patients at the University of Port Harcourt Teaching Hospital, Rivers, Nigeria. Am. J. Theor. Appl. Statist. 9:53. doi: 10.11648/j.ajtas.20200903.14
Donnarumma, F., and Pezzulo, G. (2021). Moral decisions in the age of COVID-19: Your choices really matter. Soc. Sci. Hum. Open 4:100149. doi: 10.1016/j.ssaho.2021.100149
Doshi, H., Kumar, P., and Yerramilli, V. (2018). Uncertainty, capital investment, and risk management. Manag. Sci. 4, 5769–5786. doi: 10.1287/mnsc.2017.2815
Dyer, R. F., Forman, E. H., and Mustafa, M. A. (1992). Decision support for media selection using the analytic hierarchy process. J. Adv. 21, 59–72. doi: 10.1080/00913367.1992.10673360
Eisenhardt, K. M., and Martin, J. A. (2000). Dynamic capabilities: What are they? Strat. Manag. J. 21, 1105–1121. doi: 10.1002/1097-0266(200010/11)21:10/11<1105::AID-SMJ133>3.0.CO;2-E
Elmer, T., Mepham, K., and Stadtfeld, C. (2020). Students under lockdown: Comparisons of students’ social networks and mental health before and during the COVID-19 crisis in Switzerland. PLoS One 15:e0236337. doi: 10.1371/journal.pone.0236337
ESIEC (2020). ESIEC2020 questionnaire on the resilience of micro, small and Medium Enterprises under the new coronavirus outbreak (COVID-19). Available online at: https://www.cgdev.org/sites/default/files/coronavirus-SME-survey-instrument-english.pdf (accessed January 15, 2022).
Fabeil, N. F., Pazim, K. H., and Langgat, J. (2020). The impact of Covid-19 pandemic crisis on micro-enterprises: Entrepreneurs’ perspective on business continuity and recovery strategy. J. Econ. Bus. 3, 837–844. doi: 10.31014/aior.1992.03.02.241
Filipova, T., Kopsieker, L., Gerritsen, E., Bodin, E., Brzezinski, B., and Ramirez- Rubio, O. (2020). Mental Health and the Environment: How European Policies Can Better Reflect the Impact of Environmental Degradation On People’s Mental Health and Well-Being. Available online at: https://ieep.eu/uploads/articles/attachments/2bfb2051-b305-4338-9770-ae8071320b1a/Mental%20health%20and%20the%20environment.pdf?v=63775265428 (accessed on Jan 15, 2022).
Ford, D., Gadde, L. E., Hakansson, H., Lundgren, A., Snehota, I., Turnbull, P., et al. (2014). Industrial marketing and purchasing rogup. Managing Business Relationships. Hoboken, NJ: Wiley.
Ghosal, V., and Ye, Y. (2015). Uncertainty and the employment dynamics of small and large businesses. Small Bus. Econ. 44, 529–558. doi: 10.1007/s11187-014-9614-0
Gregurec, I., Tomičić Furjan, M., and Tomičić-pupek, K. (2021). The impact of covid-19 on sustainable business models in SMEs [El impacto de covid-19 en modelos de negocio sontenibles en PYMES]. Sustainability 13:1098. doi: 10.3390/su13031098
Haluk Köksal, M., and Özgül, E. (2007). The relationship between marketing strategies and performance in an economic crisis. Mark. Int. Plan. 25, 326–342. doi: 10.1108/02634500710754574
Haman, M. (2020). The use of Twitter by state leaders and its impact on the public during the COVID-19 pandemic. Heliyon 6:e05540. doi: 10.1016/j.heliyon.2020.e05540
Handfield, R. B., Graham, G., and Burns, L. (2020). Coronavirus, tariffs, trade wars and supply chain evolutionary design. Int. J. Oper. Prod. Manag. 40, 1649–1660. doi: 10.1108/IJOPM-03-2020-0171
Hannam-Swain, S., and Bailey, C. (2021). Considering Covid-19: Autoethnographic reflections on working practices in a time of crisis by two disabled UK academics. Soc. Sci. Hum. Open 4:100145. doi: 10.1016/j.ssaho.2021.100145
Hasan, N., and Bao, Y. (2020). Impact of “e-Learning crack-up” perception on psychological distress among college students during COVID-19 pandemic: A mediating role of “fear of academic year loss”. Child. Youth Serv. Rev. 118:105355. doi: 10.1016/j.childyouth.2020.105355
Hidayati, R., and Rachman, N. M. (2021). Indonesian Government Policy and SMEs business strategy during the Covid-19 pandemic. Niagawan 10, 1–9. doi: 10.24114/niaga.v10i1.21813
Humphries, J. E., Neilson, C., Ulyssea, G., Haven, N., Humphries, J. E., and Neilson, C. (2020). The evolving impacts of COVID-19 on small businesses since the CARES Act, Cowles Foundation Discussion Papers 2230. New Haven: Cowles Foundation for Research in Economics, Yale University. doi: 10.2139/ssrn.3584745
International Labour Organization (2020). The Six-Step COVID-19 Business Continuity Plan for SMEs. Geneva: International Labour Organization.
International Trade Centre (2020). Covid-19 Business Impact Survey. Available online at: https://itcsurvey.intracen.org/fs.aspx?surveyid=30d16e769054375b886a8b0f1f93f41 (accessed December 15, 2021).
Jogezai, N. A., Baloch, F. A., Jaffar, M., Shah, T., Khilji, G. K., and Bashir, S. (2021). Teachers’ attitudes towards social media (SM) use in online learning amid the COVID-19 pandemic: The effects of SM use by teachers and religious scholars during physical distancing. Heliyon 7:e06781. doi: 10.1016/j.heliyon.2021.e06781
Juergensen, J. Guimón, J., and Narula, R. (2020). European SMEs amidst the COVID-19 crisis: Assessing impact and policy responses. J. Ind. Bus. Econ. 47, 499–510. doi: 10.1007/s40812-020-00169-4
Kapoor, K., Bigdeli, A. Z., Dwivedi, Y. K., and Raman, R. (2021). How is COVID-19 altering the manufacturing landscape? A literature review of imminent challenges and management interventions. Ann. Oper. Res. [Epub ahead of print]. doi: 10.1007/s10479-021-04397-2
Kareem, B. (2021). Do global pandemics disrupt or seed transformations in cities? A systematic review of evidence. Soc. Sci. Hum. Open 4:100138. doi: 10.1016/j.ssaho.2021.100138
Keeney, R. L., Raiffa, H., and Meyer, R. F. (1993). Decisions with Multiple Objectives: Preferences and Value Trade-offs. Cambridge: Cambridge university press. doi: 10.1017/CBO9781139174084
Kemenkop-UKM (2020). Identifikasi KUMKM yang Terdampak Pandemi Covid-19. Available online at: https://kemenkopukm.go.id/read/kemkop-dan-ukm-identifikasi-kumkm-yang-terdampak-pandemi-covid-19 (accessed December 15, 2021).
Keskin, A., Dincer, B., and Dincer, C. (2020). Exploring the impact of sustainability on corporate financial performance using discriminant analysis. Sustainability 12:2346. doi: 10.3390/su12062346
Khoo, T. L., and Hock, O. Y. (2020). Configure-to-Order (CTO) production through lean initiatives: Manufacturing delivery in COVID-19 pandemic milieu. Eur. J. Mol. Clin. Med. 7, 494–505.
Lee, J. (2020). Mental health effects of school closures during COVID-19. Lancet Child Adolesc. Health 4:421. doi: 10.1016/S2352-4642(20)30109-7
Li, H., and Cao, Y. (2021). Facing the pandemic in the dark: Psychopathic personality traits and life history strategies during COVID-19 lockdown period in different areas of China. Curr. Psychol. 1–9. [Epub ahead of print]. doi: 10.1007/s12144-021-01549-2
Linton, T., and Vakil, B. (2020). Coronavirus is proving we need more resilient supply chains. Boston: Harvard Business Review.
Lu, Y., Wu, J., Peng, J., and Lu, L. (2020). The perceived impact of the Covid-19 epidemic: Evidence from a sample of 4807 SMEs in Sichuan Province. China. Environ. Hazards 19, 323–340. doi: 10.1080/17477891.2020.1763902
Lutfi, M., Buntuang, P. C. D., Kornelius, Y., and Erdiyansyah Hasanuddin, B. (2020). The impact of social distancing policy on Small and Medium-sized Enterprises (SMEs) in Indonesia. Probl. Perspect. Manag. 18, 492–503. doi: 10.21511/ppm.18(3).2020.40
Lynn, M. R. (1986). Determination and quantification of content validity. Nurs. Res. 35, 382–385. doi: 10.1097/00006199-198611000-00017
Maamri, N., Chaib, R., and Benidir, M. (2021). Covi-19 safety measures in companies for employee well-being and sustainability. Int. J. Recent Adv. Multidisciplinary Res. 8, 6687–6693.
Mahajan, Y. (2020). Study of impact of coronavirus pandemic on small and Medium Enterprises (SME’s) in India. GIS Sci. J. 7, 1–6.
Mahi, M., Mobin, M. A., Habib, M., and Akter, S. (2021). A bibliometric analysis of pandemic and epidemic studies in economics: Future agenda for COVID-19 research. Soc. Sci. Hum. Open 4:100165. doi: 10.1016/j.ssaho.2021.100165
Mendy, J. (2021). Performance management problem of four small and medium-sized enterprises (SMEs): Towards a performance resolution. J. Small Bus. Enterp. Dev. 28, 690–710. doi: 10.1108/JSBED-06-2019-0201
Moulavi, D., Jaskowiak, P. A., Campello, R. J. G. B., Zimek, A., and Sander, J. (2014). “Density-based clustering validation,” in Proceeding of 14th SIAM International Conference on Data Mining, (Philadelphia, PA: SIAM), 839–847. doi: 10.1137/1.9781611973440.96
Nawaiseh, H. (2021). The effect of COVID-19 pandemic on SMEs management in Jordan. J. Contemp. Issues Bus. Govern. 7, 20–29.
Ndzana, M., Cyrille, O., Mvogo, G., and Bedzeme, T. (2021). Innovation and small and medium enterprises’ performance in Cameroon. J. Small Bus. Enterp. Dev. 28, 724–743. doi: 10.1108/JSBED-06-2020-0188
Ng, H. S., Kee, D. M. H., and Ramayah, T. (2020). Examining the mediating role of innovativeness in the link between core competencies and SME performance. J. Small Bus. Enterp. Dev. 27, 103–129. doi: 10.1108/JSBED-12-2018-0379
OECD (2020). Coronavirus (COVID-19): SME Policy Responses. Available online at: https://www.oecd.org/coronavirus/policy-responses/coronavirusCOVID-19-sme-policy-responses-04440101 (accessed on 2 Jul, 2021).
Omar, A. R. C., Ishak, S., and Jusoh, M. A. (2020). The impact of Covid-19 movement control order on SMEs’ businesses and survival strategies. Malays. J. Soc. Space 16, 139–150. doi: 10.17576/geo-2020-1602-11
Ouffroukh, L. A., Chaib, R., Ion, V., and Khochmane, L. (2018). Analysis of risk and the strengthening of the safety technical barriers: Application of Skikda (Algeria) oil refining complex. World J. Eng. 15, 99–109. doi: 10.1108/WJE-02-2017-0031
Ozcan, P., and Eisenhardt, K. M. (2009). Origin of alliance portfolios: Entrepreneurs, network strategies, and firm performance. Acad. Manag. J. 52, 246–279. doi: 10.5465/amj.2009.37308021
Pandya, A., and Lodha, P. (2021). Mental Health of College Students Amidst COVID-19: Implications for Reopening of Colleges and Universities. Indian J. Psychol. Med. 43, 274–275. doi: 10.1177/02537176211005622
Paul, S. K., and Chowdhury, P. (2020). Strategies for managing the impacts of disruptions during COVID-19: An example of toilet paper. Glob. J. Flex. Syst. Manag. 21, 283–293. doi: 10.1007/s40171-020-00248-4
Poulsen, J., and French, A. (2004). Discriminant Function Analysis (DA). California: State University, California.
Prempeh, C. (2021). Religion and the state in an episodic moment of COVID-19 in Ghana. Soc. Sci. Hum. Open 4:100141. doi: 10.1016/j.ssaho.2021.100141
Ratnasingam, J., Khoo, A., Jegathesan, N., Wei, L. C., Latib, H. A., Thanasegaran, G., et al. (2020). How are Small and Medium Enterprises in Malaysia’s furniture industry coping with COVID-19 pandemic? Early evidences from a survey and recommendations for policymakers. BioResources 15, 5951–5964. doi: 10.15376/biores.15.3.5951-5964
Ratten, V. (2020). Coronavirus and international business: An entrepreneurial ecosystem perspective. Thunderbird Int. Bus. Rev. 62, 629–634. doi: 10.1002/tie.22161
Riabacke, M., Danielson, M., and Ekenberg, L. (2012). State-of-the-art prescriptive criteria weight elicitation. Adv. Decis. Sci. 2012, 1–24. doi: 10.1155/2012/276584
Rojas-Lema, X., Alfaro-Saiz, J. J., Rodríguez-Rodríguez, R., and Verdecho, M. J. (2020). Performance measurement in SMEs: Systematic literature review and research directions. Total Qual. Manag. Bus. Excell. 32, 15–16. doi: 10.1080/14783363.2020.1774357
Roy, S., Dutta, R., and Ghosh, P. (2021). Identifying key indicators of job loss trends during COVID-19 and beyond. Soc. Sci. Hum. Open 4:100163. doi: 10.1016/j.ssaho.2021.100163
Saha, A., Dutta, A., and Sifat, R. I. (2021). The mental impact of digital divide due to COVID-19 pandemic induced emergency online learning at undergraduate level: Evidence from undergraduate students from Dhaka City. J. Affect. Disord. 294, 170–179. doi: 10.1016/j.jad.2021.07.045
Sardi, A., Sorano, E., Ferraris, A., and Garengo, P. (2020). Evolutionary paths of performance measurement and management system: The longitudinal case study of a leading SME. Meas. Bus. Excell. 24, 495–510. doi: 10.1108/MBE-01-2020-0016
Sarfraz, M., Mohsin, M., Naseem, S., and Kumar, A. (2021). Modeling the relationship between carbon emissions and environmental sustainability during COVID-19: A new evidence from asymmetric ARDL cointegration approach. Environ. Dev. Sustainabil. 23, 16208–16226. doi: 10.1007/s10668-021-01324-0
Saturwa, H. N., Suharno, S., and Ahmad, A. A. (2021). The impact of Covid-19 pandemic on MSMEs. Jurnal Ekonomi dan Bisnis 24, 65–82. doi: 10.24914/jeb.v24i1.3905
Serafini, G., Parmigiani, B., Amerio, A., Aguglia, A., Sher, L., and Amore, M. (2020). The psychological impact of COVID-19 on the mental health in the general population. Int. J. Med. 113, 531–535. doi: 10.1093/qjmed/hcaa201
Shafi, M., Liu, J., and Ren, W. (2020). Impact of COVID-19 pandemic on micro, small, and medium-sized Enterprises operating in Pakistan. Res. Glob. 2:100018. doi: 10.1016/j.resglo.2020.100018
Shahbaz, M., Bilal, M., Moiz, A., Zubair, S., and Iqbal, H. M. N. (2020). Food safety and COVID-19: Precautionary measures to limit the spread of coronavirus at food service and retail sector. J. Pure Appl. Microbiol. 14, 749–756. doi: 10.22207/JPAM.14.SPL1.12
Smith, J., Guimond, F. A., Bergeron, J., St-Amand, J., Fitzpatrick, C., and Gagnon, M. (2021). Changes in students’ achievement motivation in the context of the COVID-19 pandemic: A function of extraversion/introversion? Educ. Sci. 11, 30–38. doi: 10.3390/educsci11010030
Tairas, D. R. (2020). Covid-19 pandemic and MSMEs: Impact and mitigation. Jurnal Ekonomi Indonesia 9, 67–80. doi: 10.52813/jei.v9i1.48
Tannenbaum, C. R., Boyle, R. J., and Tandon, V. (2020). Small businesses and oil market shocked by COVID-19, but don’t rush reopening. Advisor Perspectives. Available online at: https://www.advisorperspectives.com/commentaries/2020/04/24/small-businesses-and-oil-market-shocked-by-covid-19-but-dont-rush-reopening (accessed December 15, 2021).
Tian, J., Coreynen, W., Matthyssens, P., and Shen, L. (2021). Platform-based servitization and business model adaptation by established manufacturers. Technovation (in press). doi: 10.1016/j.technovation.2021.102222
Tomasini, C., Emmendorfer, L., Borges, E. N., and Machado, K. (2016). “A methodology for selecting the most suitable cluster validation internal indices,” in Proceedings of the 31st Annual ACM Symposium on Applied Computing, (New York, NY: ACM), 901–903. doi: 10.1145/2851613.2851885
Tonkin, A., and Whitaker, J. (2021). Play and playfulness for health and wellbeing: A panacea for mitigating the impact of coronavirus (COVID 19). Soc. Sci. Hum. Open 4:100142. doi: 10.1016/j.ssaho.2021.100142
Vasantha Raju, N., and Harinarayana, N. S. (2016). “Online survey tools: A case study of Google Forms,” in National conference on scientific, computational & information research trends in engineering, GSSS-IETW, Mysore.
Wang, C., Wang, D., Abbas, J., Duan, K., and Mubeen, R. (2021). Global financial crisis, smart lockdown strategies, and the COVID-19 spillover impacts: A global perspective implications from Southeast Asia. Front. Psychiatry 12:643783. doi: 10.3389/fpsyt.2021.643783
Wuest, T., Romero, D., Cavuoto, L. A., and Megahed, F. M. (2020). Empowering the workforce in post-COVID- 19 smart manufacturing systems. Smart Sustain. Manuf. Syst. 4, 1–5. doi: 10.1520/SSMS20200043
Xiong, H., and Li, Z. (2013). “Clustering validation measures,” in Data Clustering: Algorithms and Application, eds C. C. Aggrawal and C. K. Reddy (Boca Raton, USA: Chapman and Hall/CRC), 571–605. doi: 10.1201/9781315373515-23
Xiong, P., Ming, W. K., Zhang, C., Bai, J., Luo, C., Cao, W., et al. (2021). Factors influencing mental health among Chinese medical and non-medical students in the early stage of the COVID-19 pandemic. Front. Public Health 9:603331. doi: 10.3389/fpubh.2021.603331
Xu, D., and Tian, Y. (2015). A comprehensive survey of clustering algorithms. Ann. Data Sci. 2, 165–193. doi: 10.1007/s40745-015-0040-1
Ye, Z., Yang, X., Zeng, C., Wang, Y., Shen, Z., Li, X., et al. (2020). Resilience, social support, and coping as mediators between COVID-19-related stressful experiences and acute stress disorder among college students in China. Appl. Psychol. 12, 1074–1094. doi: 10.1111/aphw.12211
Yu, S., Abbas, J., Draghici, A., Negulescu, O. H., and Ain, N. U. (2022). Social media application as a new paradigm for business communication: The role of COVID-19 knowledge, social distancing, and preventive attitudes. Front. Psychol. 13:903082. doi: 10.3389/fpsyg.2022.903082
Zhao, N., and Zhou, G. (2021). COVID-19 stress and addictive social media use (SMU): Mediating role of active use and social media flow. Front. Psychiatry 12:635546. doi: 10.3389/fpsyt.2021.635546
Keywords: COVID-19 pandemic, multi-attribute value theory approach, K-means cluster analysis, discriminant analysis, SMEs, vulnerability
Citation: Susanty A, Puspitasari NB, Bakhtiar A and Prasetya F (2022) Assessing the impact of the COVID-19 pandemic on small and medium-sized enterprises performance. Front. Psychol. 13:927628. doi: 10.3389/fpsyg.2022.927628
Received: 24 April 2022; Accepted: 03 August 2022;
Published: 11 October 2022.
Edited by:
Eijaz Khan, Melbourne Institute of Technology, AustraliaReviewed by:
Jaffar Abbas, Shanghai Jiao Tong University, ChinaEduardo Moraes Sarmento, Lusophone University of Humanities and Technologies, Portugal
Copyright © 2022 Susanty, Puspitasari, Bakhtiar and Prasetya. This is an open-access article distributed under the terms of the Creative Commons Attribution License (CC BY). The use, distribution or reproduction in other forums is permitted, provided the original author(s) and the copyright owner(s) are credited and that the original publication in this journal is cited, in accordance with accepted academic practice. No use, distribution or reproduction is permitted which does not comply with these terms.
*Correspondence: Aries Susanty, YXJpZXNzdXNhbnR5QGdtYWlsLmNvbQ==