- International College of Chinese Studies, Fujian Normal University, Fuzhou, China
In the information age, online teaching has become an essential field of educational development. The work aims to analyze the factors influencing learning intention of Overseas Students (OSs) during the Coronavirus Disease 2019 (COVID-19). The work adopts the method of Unified Theory of Acceptance and Use of Technology (UTAUT), and implements the influencing factor model of open source software online Chinese learning (OCL). Specifically, the OSs of five colleges and universities in Fuzhou are taken as the research objects. The data is collected through questionnaire survey (QS) and Spss25.0 to analyze the reliability and validity of the data, and Amos23.0 is used to validate the model. The results present that autonomy and self-discipline have become the necessary factors affecting open source software OCL, and personal interests and hobbies are the main factors affecting open source software OCL. Meantime, perceived interest, self-learning management, effort expectation, community influence, performance expectation, and promotion conditions have a significant positive impact on the willingness to promote Chinese learning, and their influence degree increases in turn. The conclusion can provide a novel online teaching and learning strategy for open source software under the COVID-19 situation.
Introduction
Due to the ongoing Corona Virus 2019 (COVID-19) pandemic, Online Teaching and Learning (OTL) has become a general trend in most countries across the world (Spitzer et al., 2021). Back in early 2020, the COVID-19 outbreak has hindered many students from going to school for normal educational courses. To that end, the Chinese Ministry of Education (MoE) has issued some policy packages to encourage OTL, including “Classes suspended but learning continues.” Overnight, OTL has gone viral in China, with many effective measures being put forward (Agyeiwaah et al., 2021; Al-Nasa'h et al., 2021). As of February 2020, the MoE has organized 22 OTL platforms to open free Online Courses (OLC)s for Chinese College Students (CCSs). Remarkably, the “Chinese University MOOC” website provides nearly 8,000 high-quality OLCs. To help CCSs learn and study normally during the COVID-19 pandemic, Chinese higher institutions have dedicated immensely to high-quality OLC development tailored to CCSs' practical needs. Statistics show that China has the most vigorous OTL implementation during the COVID-19 crisis, so OTL has seen unprecedented popularity and technical maturity. However, whether to adhere to OTL during and after school suspension remains investigated.
The introduction of OLC has revolutionized the traditional teaching model, the success and failure of which largely depends on students' and teachers' skills and dexterity to manipulate OTL-oriented Applications (APPs) (Jacqmin, 2021; Muflih et al., 2021). Meanwhile, OTL requires novel teaching methods and proactive learning attitudes, which run throughout the whole OTL process (Maqableh and Alia, 2021). To date, there has been extensive research on OTL. Some put forward the hypothesis of influencing factors of College Students' willingness to use mobile OTL platform based on User-Perceived Value (UPV) theory and verified the hypothesis by Questionnaire Survey (QS) and Structural Equation Modeling (SEM). The results show that the designed QS has good reliability and validity. Meanwhile, the network's exploratory learning and good reputation positively impact college students' perception of the Ease of Use (EoU) and usefulness of the mobile OTL platform. Perceived usefulness has a significant direct and positive impact on their willingness to use the online education platform.
Willingness To Learn (WTL), a key factor determining the results of Chinese learning, can arouse learning activities and learning behaviors (Catalano et al., 2021; Liu et al., 2021). At present, there are few studies on WTL, and they are basically the summary of the education reform experience of education suppliers. Therefore, there is a lack of empirical investigation on the online education demanders' [namely Overseas Student (OS)] Chinese WTL. With the push of the Belt and Road initiative, OSs are increasing. Thus, Chinese teaching has become critical for OSs. Thereupon, the present work studies the online Chinese WTL in five universities in Fuzhou and collects and discusses the factors controlling the OSs' Chinese WTL, which provides a reference for developing Chinese OTL strategies for OSs under the COVID-19 pandemic.To sum up, with regard to the analysis of foreign students' Online Chinese learning (OCL) intention, the advantage of the current studies is that a large number of learning data of foreign students can be collected through questionnaire survey and SEM to model and analyze the degree of learning intention. However, the disadvantage of these studies is that the effect of empirical test is poor. Therefore, the model needs to be further improved and optimized.
WTL of five universities in Fuzhou is discussed. The main innovation is to collect and discuss the important factors affecting foreign students' Chinese learning intention. First, the background of the COVID-19 and the traditional teaching mode of OCL are explored. Through the study of OCL strategies and characteristics, the factors of satisfaction of OCL for foreign students are modeled, and the OCL-oriented operating system is analyzed as a model. Through the design and distribution of questionnaires, the reliability of the sample is tested and the results of the model fitting are obtained. The results reveal that the standardized coefficients of Overseas Students' Facilitating Conditions (OSFC), Overseas Students' Self-learning Management (OSSM), and Overseas Students' Perceived Interest (OSPI) for Overseas students' willingness to learn Chinese Online (OSLW) are 0.156, 0.254, and 0.289, respectively. The work provides a specific experimental reference for the development of open source software under the leadership of the COVID-19 outbreak and the promotion of OCL efficiency.
Characteristics of OTL
Different teaching methods and learning modes have different characteristics. In particular, the traditional class teaching system has been proposed to meet the needs of large-scale talent training. Still, the new University Geography Education Resource Bank provides a novel approach to breaking the barriers of economic and geography education resources. Meanwhile, with the advent of the learning society, e-learning has gained more momentum, and significant changes have taken place in teaching modes and even classroom time allocations (Moura et al., 2021; Yu, 2022). Additionally, the popularity of the Internet also has a significant impact on learners' physical, mental, and family conditions, rendering some unique characteristics for OTL.
During the COVID-19 pandemic, OTL is still based on classes, teaching materials, and timetables. However, the teaching location is not fixed due to the geographic separations, the teaching content will be reduced, and the interaction between teachers and students will be weakened. The result is it alters the platform on which the OLC depends, the concept of teaching basis, students' participation, and sense of experience (Binali et al., 2021; Thepwongsa et al., 2021). Generally speaking, under the background of the COVID-19 pandemic, the OTL features a traditional classroom, MOOC, and mixed teaching. At the same time, OTL's characteristics might differ due to the audiences and teaching motives. Therefore, there is a need to consider OTL beyond single traditional teaching, online learning, or mixed teaching modes but comprehensively analyze its characteristics. In other words, to explore the factors of online learners' WTL, there is a need for educators to consider both the universality and particularity of OTL.
Online Chinese Learning (OCL)-Oriented Learning Satisfaction (LS) Factor Modeling
Based on the Unified Theory of Acceptance and Use of Technology (UTAUT), this section implements the OCL-oriented OS WTL factor model, as shown in Figure 1.
Generally, UTAUT is believed to have the highest power in understanding and explaining Behavioral Intention (BI) and usage behavior, but it has some limitations. Thus, this section does not employ UTAUT to model the OS WTL factors directly but adds new variables concerning the COVID-19 pandemic and the OS characteristics. In so doing, OS WTL for OCL can be better explained during the COVID-19 pandemic by introducing two independent variables: Self-Learning Management (SLM) and Perceived Interest (PI). For example, studies have shown that learners with strong SLM abilities have stronger online WTL (Theobald, 2021). Meanwhile, others believe that SLM ability directly affects online learning, and consciousness and self-discipline are also important (Hong et al., 2021). More precisely, PI refers to the learners' pleasantness during online learning, and the flexibility and interactivity of online learning can better stimulate students' learning interest (Apostolou, 2021; Nuutila et al., 2021; Oppermann and Lazarides, 2021). Additionally, most OSs lack learning motivation for Chinese and might have difficulty completing the whole course. Thus, the interest of the course is crucial to stimulate learning motivation.
Research Hypothesis
Firstly, in the UTAUT model, performance expectation refers to the extent to which users believe that technology can help them improve their work performance (Erjavec and Manfreda, 2021). It is the main factor affecting users' online learning. The current work believes that when OSs feel that online learning can improve their Chinese level, their WTL gets stronger. Secondly, effort expectation in UTAUT is user perception of how convenient technological usage is (Andrews et al., 2021). Many studies reveal that effort expectation directly or indirectly impacts online learning BI. The present work holds that when learners can use online learning more efficiently and skillfully, they have a stronger WTL. Thirdly, community influence in UTAUT is the users' perception surrounding groups' attitudes toward a technology (Jadil et al., 2021). Relevant studies have uncovered that community influence directly or indirectly impacts online learners' Willingness To Use (WTU) (Akinnuwesi et al., 2021). This study contends that external factors, such as school fellows, teachers, and parents, significantly impact online learners' WTU. Fourthly, facilitating conditions in UTAUT are objective factors making technology easier (Bu et al., 2021). Users who feel objective conditions are easy to meet will exert higher BI (Ayuning Budi et al., 2021). Thus, if the online learners feel objective conditions for using OTL platforms are easy to meet, their WTU will be stronger (Ye et al., 2020). On the other hand, SLM is an individual's perception of the degree of self-discipline and autonomous learning. Particularly, since online learning features the “three separations” characteristics, learners are required to have better SLM ability. Research shows that a lack of SLM ability will impact the use of OTL platforms (Vinette and Bilodeau, 2021). The present work argues that students with solid SLM ability have a strong online WTL. Meanwhile, the higher the PI is, the stronger learners' WTU to use OTL platforms. Lastly, BI is the degree to which OSs are willing, intend, or use OTL platforms again.
Based on the above analysis, the research hypotheses are put forward in Table 1. After the questionnaire survey, the fitting evaluation model is implemented by using the collected data, and these hypotheses are experimentally verified:
Based on the above analysis, Table 1 proposes the research hypotheses:
As Table 1 shows, H1 is the positive impact of OSPE on OSTL, H2 is the positive impact of OSEE on OSTL, H3 is the positive impact of OSCI on OSTL, H4 is the positive impact of OSFC on OSTL, H5 is the positive impact of OSSM on OSTL, H6 is the positive impact of OSTL on OCL, H7 is the positive impact of OSLB on OCL. The hypothesis is verified by the fitting evaluation model, and the estimation result is the hypothesis that has passed the test.
Design of Questionnaire Survey (QS)
Next, this section collects data through QS to verify the proposed model. Before QS design, the existing scales are sorted out to determine the measurement items of each dimension based on the situation of the present work. Meanwhile, experts are invited to discuss and modify the QS items. Then, the designed QS is pre-tested and adjusted accordingly. Finally, the experiment designs an OSs' OCL-oriented WTL influencing factors QS.
The QS comprises a personal information survey and an influencing factors survey. The options adopt Likert level 5 items: 1-disagree and 5-fully agree. The theoretical model measures the eight variables: OSPE, OSEE, OSCI, OSFC, OSLM, OSPI, OSLW, and the OSLB. Table 2 lists the set measurement items for the specific variables:
QS Distribution and Recovery
This section recruits OSs from five higher institutions in Fuzhou as research subjects because these students have experience of the COVID-19 epidemic and online learning. Thus, sample selection is representative. Then, the Questionnaire Star generates the electronic and word versions of the QS, distributed online. Totally, 500 QSs are distributed, with 100 pieces for each school, and 480 QSs are recovered. After removing invalid QS, such as incomplete answers and inconsistent answers, the 438 pieces are effective, with an effective rate of 91.25%. Meanwhile, there are 29 measurement items, and the effective QSs are over 290, so SEM can analyze the data.
Result Analysis
Descriptive Statistics
Subsequently, for a more intuitive understanding of the QS, Figure 2 statistically analyzes the samples' demographic features, such as gender distribution and duration of study abroad.
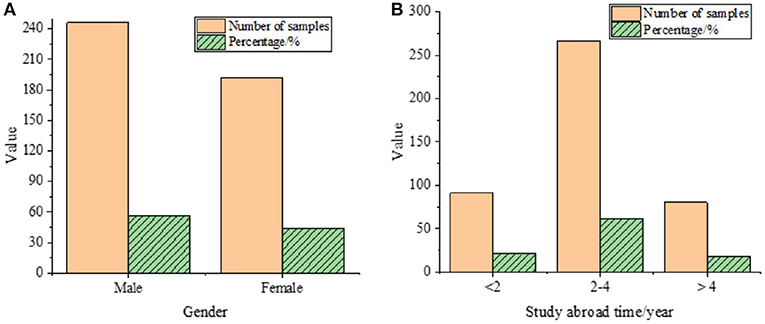
Figure 2. Descriptive statistics of sample demographic features. (A) Gender, (B) Duration of study abroad.
As depicted in Figure 2, according to the basic statistics description of 438 respondents, respondents studying abroad for 2-4 years account for the most, 60.9%. In terms of gender distribution, males and females account for 56% and 44%, respectively, relatively uniform.
Reliability Test of Questionnaire
For the reliability of questionnaire samples, Cronbach's alpha coefficient needs to be used for reliability evaluation, as shown in Figure 3.
Sample Reliability Analysis
The sample reliability analysis employs Cronbach's Alpha coefficient for evaluation, as outlined in Figure 3.
Figure 3 suggests that the Cronbach's α coefficients of OSPE, OSEE, OSCI, OSFC, and OSSM are 0.902, 0.884, 0.878, 0.891, and 0.876, respectively. The Cronbach's α coefficients of OSPI, OSLW, and OSLB are 0.801, 0.789, and 0.808. Overall, the α coefficients of these eight dimensions of Cronbach are greater than the standard value of 0.7. Therefore, the collected QS data has good internal reliability and can be further studied.
Sample Validity Analysis of Samples
Questionnaire Validity Test
Then, Amos 23.0 is used to extract Composite Reliability (CR) and Average Variance (AVE) to analyze the convergence validity of the questionnaire. The measurement results are shown in Figure 4.
As Figure 4 presents, CR of OSPE, OSEE, OSCI, OSFC, OSSM, OSPI, OSLW, and OSLB is greater than 0.7. AVE is greater than 0.5, indicating that the measurement scale has good internal consistency. These eight potential variables obtained relatively high changes from the corresponding observed variables. Therefore, the correlation is relatively high, indicating that the validity of the questionnaire has been tested.
In addition to testing the CR and AVE, it is also necessary to test the Discriminant Validity (DV). Specifically, DV refers to the degree of difference between potential variables. Table 3 lists the DV results of the design scale.
As detailed in Table 3, the diagonal values are greater than their corresponding rows and columns, which shows that the measurement scale has good DV. In conclusion, the reliability and validity of the designed measurement scale are relatively good, based on which the subsequent analysis can be carried out.
SEM Test
Model Fitting Evaluation
SEM is introduced into SPSS, and the parameters are estimated by the maximum likelihood estimation method. Table 4 and Figure 5 show standardized estimation results and non-standardized estimation results, respectively.
Figure 5 shows the standardized path coefficient structure of the initial model. In the model, the management, expectation, and community influence of foreign students are comprehensively analyzed, and a unified initial parameter model is implemented through the standardization of coefficients. Additionally, for the fitting index between the standardized fitting result of the model and the initial model, the data arrangement is shown in Table 4.
Table 4 manifests the fitting index of the initial model.
As displayed in Table 4, among all fitting indexes, CMIN/DF is less than 0.3, RMR is less than 0.5, RMSEA is less than 0.08, and the values of other fitting indexes are greater than 0.9. Thus, the fitting indexes have reached the ideal value, and there is no need to modify the model.
Path Analysis
Figure 6 analyzes the path of the modified model using Amos 23.0. OSPE->OSLW, OSEE->OSLW, OSCI->OSLW, OSFC->OSLW, OSSM->OSLW, OSPI->OSLW represent the impact degree of OSPE, OSEE, OSCI, OSFC, OSSM, and OSPI on OSLW. And OSLW->OSLB suggests the impact degree of OSLW on OSLB.
As indicated in Figure 6, when P <0.001, the standardization coefficients of the impact of OSPE, OSEE, OSCI, OSFC, OSSM, and OSPI on OSLW for OCL is 0.203, 0.224, 0.211, 0.156, 0.254, and 0.289, respectively. The standardized coefficient of the impact of OSLW for OCL on OSLB is 0.457. Therefore, research hypotheses hold.
Research Hypothesis Test
Table 5 reveals the test result of the proposed hypotheses.
According to Table 5, all hypotheses (H1, H2, H3, H4, H5, H6, and H7) proposed have been well verified. OSPE, OSEE, OSCI, OSFC, OSSM, and OSPI all have a direct positive impact on OSLW.
According to the research and analysis, OSPE, OSEE, OSCI, OSSM, and OSPI have a significant positive impact on OSLW for OCL and indirectly affect OSLB through OSLW. The results show that OSs can improve their WTL for OCL by improving OSPE. Additionally, OSs feel that the lower the difficulty of OCL is, the stronger their WTL is. The OSFC has a positive impact on OSLW and OSLB for OCL. At present, learners' OSFC perception is relatively low, which may be because they used to learn Chinese offline, which is now hindered by the impact of the COVID-19 epidemic. Therefore, OSs have not fully understood OCL and have little perception of the facilitating conditions. Moreover, OSs' online learning might get distracted because they might have difficulties in understanding, so autonomy and self-discipline have become important factors affecting OSs' OCL. Personal interests and hobbies are the main factors affecting OSs' OCL.
Conclusion
The work adopts the empirical research method based on online WTL research and specific situations. Meanwhile, the work implements the influencing factor model of online education for foreign students, determines six main factors, and compiles a questionnaire. Finally, the initial model is empirically tested according to the sample data. The empirical results show that the Cronbach α coefficients of OSPI, OSLW and OSLB are 0.801, 0.789, and 0.808, respectively. CR is greater than 0.7 and AVE is greater than 0.5, indicating that the measurement scale has good internal consistency. In addition, the standardization coefficients of OSFC, OSSM, and OSPI on OSLW are 0.156, 0.254 and 0.289 respectively, and the standardization coefficient of OCL and OSLW on OSLB is 0.457. Therefore, the research hypothesis holds. It verifies the four core elements of the model and the impact of new factors on the degree of learning intention, and the applicability of the proposed model. Moreover, it enriches the research on variables in the theory of learning intention, and expands the application of the model in OTL. This study provides a reference basis for formulating OTL strategies for foreign students' Chinese curriculum. However, it also has some limitations. Although the work is mainly aimed at the theoretical model based on OSs, due to the diversity and complexity of learners, different learners have differences in cognitive level and learning style. Thus, in the future research, the individual characteristics of learners should be emphasized and the explanatory power of the model should be improved through the specific analysis of individual characteristics. In addition, COVID-19 education has entered a rapid development stage due to the restriction of teaching places. In the follow-up research, the broad development prospects of international students' online education should be focused on.
Data Availability Statement
The original contributions presented in the study are included in the article/supplementary material, further inquiries can be directed to the corresponding author/s.
Author Contributions
XZ: editing, data curation and writing—original draft preparation.
Conflict of Interest
The author declares that the research was conducted in the absence of any commercial or financial relationships that could be construed as a potential conflict of interest.
Publisher's Note
All claims expressed in this article are solely those of the authors and do not necessarily represent those of their affiliated organizations, or those of the publisher, the editors and the reviewers. Any product that may be evaluated in this article, or claim that may be made by its manufacturer, is not guaranteed or endorsed by the publisher.
References
Agyeiwaah, E., Badu Baiden, F., Gamor, E., and Hsu, F.-C. (2021). Determining the attributes that influence students' online learning satisfaction during the COVID-19 pandemic. J. Hospital. Leisure Sport Tour. Educ. 21, 100364. doi: 10.1016/j.jhlste.2021.100364
Akinnuwesi, B. A., Uzoka, F.-M. E., Fashoto, S. G., Mbunge, E., Odumabo, A., Amusa, O. O., et al. (2021). A Modified UTAUT model for the acceptance and use of digital technology for tackling COVID-19. Sustain. Operat. Comput. 12, 1. doi: 10.1016/j.susoc.2021.12.001
Al-Nasa'h, M., Al-Tarawneh, L., Abu Awwad, F. M., and Ahmad, I. (2021). Estimating students' online learning satisfaction during COVID-19: a discriminant analysis. Heliyon 7, e08544. doi: 10.1016/j.heliyon.2021.e08544
Andrews, J. E., Ward, H., and Yoon, J. (2021). UTAUT as a model for understanding intention to adopt ai and related technologies among librarians. J. Acad. Librarianship 47, 102437. doi: 10.1016/j.acalib.2021.102437
Apostolou, M (2021). Involuntary singlehood and its causes: the effects of flirting capacity, mating effort, choosiness and capacity to perceive signals of interest. Personal. Individ. Differen. 176, 110782. doi: 10.1016/j.paid.2021.110782
Ayuning Budi, N. F., Adnan, H. R., Firmansyah, F., Hidayanto, A. N., Kurnia, S., and Purwandari, B. (2021). Why do people want to use location-based application for emergency situations? the extension of UTAUT perspectives. Technol. Soc. 65, 101480. doi: 10.1016/j.techsoc.2020.101480
Binali, T., Tsai, C.-C., and Chang, H.-Y. (2021). University students' profiles of online learning and their relation to online metacognitive regulation and internet-specific epistemic justification. Comput. Educ. 175, 104315. doi: 10.1016/j.compedu.2021.104315
Bu, F., Wang, N., Jiang, B., and Jiang, Q. (2021). Motivating information system engineers' acceptance of Privacy by Design in China: an extended UTAUT model. Int. J. Inform. Manage. 60, 102358. doi: 10.1016/j.ijinfomgt.2021.102358
Catalano, A. S., Jimmieson, N. L., and Knight, A. T. (2021). Building better teams by identifying conservation professionals willing to learn from failure. Biologic. Conserv. 256, 109069. doi: 10.1016/j.biocon.2021.109069
Erjavec, J., and Manfreda, A. (2021). Online shopping adoption during COVID-19 and social isolation: extending the UTAUT model with herd behavior. J. Retail. Consum. Serv. 21, 102867. doi: 10.1016/j.jretconser.2021.102867
Hong, J.-C., Lee, Y.-F., and Ye, J.-H. (2021). Procrastination predicts online self-regulated learning and online learning ineffectiveness during the coronavirus lockdown. Personal. Individ. Differen. 174, 110673. doi: 10.1016/j.paid.2021.110673
Jacqmin, J (2021). Why are some massive open online courses more open than others? Technovation 21, 102395. doi: 10.1016/j.technovation.2021.102395
Jadil, Y., Rana, N. P., and Dwivedi, Y. K. (2021). A meta-analysis of the UTAUT model in the mobile banking literature: the moderating role of sample size and culture. J. Bus. Res. 132, 354–372. doi: 10.1016/j.jbusres.2021.04.052
Liu, M., Zuo, J., Tao, Y., Zhao, L., Wu, S., Feng, L., et al. (2021). Influencing factors of learning sustained attention for nursing students in online settings: a structural equation model. Nurse Educ. Today 21, 105248. doi: 10.1016/j.nedt.2021.105248
Maqableh, M., and Alia, M. (2021). Evaluation online learning of undergraduate students under lockdown amidst COVID-19 Pandemic: the online learning experience and students' satisfaction. Child. Youth Serv. Rev. 128, 106160. doi: 10.1016/j.childyouth.2021.106160
Moura, J., Estrela, M., Almeida, A. M., Ferraz, I., Figueiras, A., Roque, F., et al. (2021). A usability study of pharmacists' perceptions toward an online course for respiratory infections and antibiotic use. Procedia Comput. Sci. 181, 269–276. doi: 10.1016/j.procs.2021.01.146
Muflih, S., Abuhammad, S., Al-Azzam, S., Alzoubi, K. H., Muflih, M., and Karasneh, R. (2021). Online learning for undergraduate health professional education during COVID-19: Jordanian medical students' attitudes and perceptions. Heliyon 7, e08031. doi: 10.1016/j.heliyon.2021.e08031
Nuutila, K., Tapola, A., Tuominen, H., Molnár, G., and Niemivirta, M. (2021). Mutual relationships between the levels of and changes in interest, self-efficacy, and perceived difficulty during task engagement. Learn. Individ. Differen. 92, 102090. doi: 10.1016/j.lindif.2021.102090
Oppermann, E., and Lazarides, R. (2021). Elementary school teachers' self-efficacy, student-perceived support and students' mathematics interest. Teach. Teach. Educ. 103, 103351. doi: 10.1016/j.tate.2021.103351
Spitzer, M. W. H., Gutsfeld, R., Wirzberger, M., and Moeller, K. (2021). Evaluating students' engagement with an online learning environment during and after COVID-19 related school closures: a survival analysis approach. Trends Neurosci. Educ. 25, 100168. doi: 10.1016/j.tine.2021.100168
Theobald, M (2021). Self-regulated learning training programs enhance university students' academic performance, self-regulated learning strategies, and motivation: a meta-analysis. Contemporary Educ. Psychol. 66, 101976. doi: 10.1016/j.cedpsych.2021.101976
Thepwongsa, I., Sripa, P., Muthukumar, R., Jenwitheesuk, K., Virasiri, S., and Nonjui, P. (2021). The effects of a newly established online learning management system: the perspectives of Thai medical students in a public medical school. Heliyon 7, e08182. doi: 10.1016/j.heliyon.2021.e08182
Vinette, B., and Bilodeau, K. (2021). Progression of self-management learning experiences of young adults following an allogeneic hematopoietic stem cell transplantation: A qualitative study. Eur. J. Oncol. Nurs. 52, 101951. doi: 10.1016/j.ejon.2021.101951
Ye, J., Zheng, J., and Yi, F. (2020). A study on users' willingness to accept mobility as a service based on UTAUT model. Technologic. Forecast. Soc. Change 157, 120066. doi: 10.1016/j.techfore.2020.120066
Keywords: online learning, willingness to learn, influencing factors, structural equation modeling, COVID-19 pandemic
Citation: Zou X (2022) Exploration on the Influencing Factors of Overseas Students' Online Chinese Learning Intention During the Epidemic Period. Front. Psychol. 13:907965. doi: 10.3389/fpsyg.2022.907965
Received: 30 March 2022; Accepted: 02 May 2022;
Published: 25 May 2022.
Edited by:
Deepak Kumar Jain, Chongqing University of Posts and Telecommunications, ChinaReviewed by:
Ming Zhao, Central South University, ChinaJiansong Fang, Guangdong Baiyun University, China
Gongxing Yan, Chongqing Vocational Institute of Engineering, China
Copyright © 2022 Zou. This is an open-access article distributed under the terms of the Creative Commons Attribution License (CC BY). The use, distribution or reproduction in other forums is permitted, provided the original author(s) and the copyright owner(s) are credited and that the original publication in this journal is cited, in accordance with accepted academic practice. No use, distribution or reproduction is permitted which does not comply with these terms.
*Correspondence: Xiaoyu Zou, c3l6aG91QGZqbnUuZWR1LmNu