- School of Psychology, Sichuan Normal University, Chengdu, China
Although previous research has demonstrated that parent–adolescent relationships have a significant effect on adolescent Internet Addiction (IA), the mechanisms underlying these associations and parental differences in these effects have received insufficient attention. We investigated the mediating role of Perceived Social Support and Dual System of Self-Control (DSSC) in the relationship between Father-Adolescent Relationships/Mother-Adolescent Relationships (FAR/MAR) and adolescent IA, as well as the differences in the effects of FAR and MAR. A cross-sectional survey of 732 Chinese adolescents was conducted using the Adolescent Pathological Internet Use Scale, Parent–Adolescent Relationship Scale, Multidimensional Scale of Perceived Social Support, and Dual System of Self-Control Scale. Multiple linear regression analysis, Pearson correlation analysis and structural equation modeling were used. The results of structural modeling analysis showed that neither FAR nor MAR directly predicted adolescent IA. In contrast, FAR/MAR had an impact on adolescent IA mainly through the mediating effects of Perceived Social Support and Impulsive System. Furthermore, in the relationship between FAR/MAR and adolescent IA, the Impulsive System and Perceived Social Support both served as chain mediators, as did Perceived Social Support and the Reflective System. And more importantly, unlike FAR, MAR affects adolescent IA through the mediating effect of the Reflective System. Multiple linear regression showed that the regression coefficient of MAR on adolescent IA had stronger significance compared to FAR, MAR is deserving of more attention than FAR. These findings contribute to our understanding of the mechanisms underlying the association between FAR/MAR and adolescent IA and suggest that family relationship-focused training approaches are critical for suppressing adolescent IA. These interventions should be tailored to the unique circumstances of each family.
Introduction
Internet devices (e.g., computers, smartphones, and tablets) have become increasingly popular among adolescents in recent years, and an increasing number of adolescents are overusing Internet devices, which has resulted in Internet addiction (IA) becoming a widespread problem worldwide (Talis, 2022). IA has been defined differently by various researchers. In Goldberg (1996), coined the term “Internet Addiction Disorder (IAD)” to describe the effect of excessive Internet use on people’s daily lives in the absence of addictive substances. Then some researchers define IA as a behavioral disorder that is unrelated to addictive substances and is therefore a typical mental illness disorder (Young, 2004). Davis (2001) proposed a cognitive-behavioral model, defined IA as problematic Internet use (PIU), classified PIU into general and specific pathological Internet use, and developed the problematic Internet use scale (PIUS) to assess individuals’ irrational perceptions and usage behaviors. On the other hand, Young (1998) developed a questionnaire to assess IA behaviors based on the DSM-IV diagnostic criteria for pathological gambling; additionally, Li and Yang (2007) developed the Adolescent Pathological Internet Use Scale (APIUS) based on Davis’ PIU model. Although there is no universally accepted definition of IA, existing definitions and questionnaires place a premium on describing people’s emotional, attitude, and behavioral dependence on the Internet, as well as the negative consequences of this dependence on their lives.
Numerous studies have established that adolescents who suffer from IA have poorer mental health than adolescents who do not suffer from IA (Lauricella et al., 2014). IA demonstrated significant negative correlations with adolescents’ self-esteem (Fioravanti et al., 2012; Peng et al., 2019), psychological well-being (Jia et al., 2017), and peer aggression (Jia et al., 2018). And IA has been shown to impair academic performance, anxiety disorders, feelings of stress, depression, and aggression in adolescents (Ha and Hwang, 2014; Shek and Yu, 2016; Cerniglia et al., 2017; Wang C. Y. et al., 2017; Zhao et al., 2022), thereby inhibiting adolescents’ positive psychological development. More importantly, the prevalence of IA has remained high in adolescents over the last decade. The prevalence of IA among adolescents varies considerably across the globe, ranging from 26.8 percent in Hong Kong (Chung T. W. et al., 2019) to 14.7 percent in Taiwan (Lin et al., 2018), 1.5–8.2 percent in Europe and the United States (Kuss et al., 2014), 6.5 percent in Switzerland (World Health Organization, 2015), and 40.64 percent in mainland China (Bu et al., 2021). Thus far, the global situation for adolescents with IA has deteriorated, with prevalence rates ranging from 12.6 to 67.5% (Kuss et al., 2021). Why does the prevalence of IA vary by country or region among adolescents? Some researchers conducted a meta-analysis of the prevalence of IA in 31 countries or regions and discovered a significant correlation between the prevalence of IA and lower life satisfaction, increased air pollution, increased transportation commuting time, and lower national income (Cheng and Li, 2014). Cheng and Li also argue that as a country or region advances technologically, it may result in a sustained increase in the prevalence of IA. On the other hand, Blachnio et al. (2019) argue that the cultural presence of denial of one’s addiction and loss of control over one’s online time are significant manifestations of the IA epidemic (e.g., Taiwan).
Gaming content, social content, short video content (e.g., Tik Tok), and news and information on Internet devices are all intrinsically appealing and can provide instant feedback to users of all ages, making them happy and enjoyable (Peris et al., 2020). While any group has the potential to develop an addiction to the Internet, there are several reasons why adolescents are more likely to develop an addiction than other age groups: (1) More eager for attention: Compared to other age groups, adolescents are more eager for attention from others to satisfy their sense of belonging and self-expression (Kim and Kim, 2015), and, in comparison to real life, the Internet provides a platform for adolescents to demonstrate themselves, and they are more willing to establish and sustain social relationships on online platforms, making them more likely to generate IA (Seidman, 2013). (2) More impulsive: there is evidence that impulsivity increases from childhood to adolescence and then declines (Rosenbaum and Hartley, 2019), and that increased impulsivity in adolescents may be related to ventral striatum hyperresponsivity (Sherman et al., 2018). Comparing groups revealed that IA individuals were more impulsive than healthy controls (Li et al., 2021), and behavioral experiments also indicate that IA individuals are more focused on immediate gratification (Wang L. et al., 2017). These findings suggest that impulsivity is strongly associated with IA in adolescents. (3) More negative coping styles: Adolescents undergo rapid physical, emotional, intellectual, and life development and are susceptible to a variety of negative events such as peer pressure, academic stress, school bullying, emotional difficulties, and social discrimination (Kuss et al., 2013; Lin et al., 2018; Chung S. et al., 2019). Adolescents, on the other hand, often struggle to actively deal with these life frustrations, and instead turn to the Internet environment to vent and escape from real-world problems, eventually developing an unhealthy reliance on the Internet. As a result, adolescents have a higher risk of developing IA than other age groups (Anderson et al., 2017).
Parent–Adolescent Relationship and Adolescent Internet Addiction
Previous research has indicated that the primary causes of IA in adolescents are either individual factors (e.g., self-control, personality traits) or environmental factors (e.g., family economic environment, school environment) (Xu et al., 2012; Zhou et al., 2017). However, an increasing number of researchers have discovered that relationships between parents and adolescents in the family environment may be a significant influence factors for adolescent IA. Through a questionnaire study, Park et al. (2008) discovered significant differences in parent-child relationships between three groups: IA, addictive tendency, and non-addiction. Post hoc comparisons revealed that the parent–child relationship was significantly less positive in the addiction and addictive tendency groups compared to the non-addiction group. Additionally, Xu et al. (2014) examined the parent–child relationships and family environment of adolescent parents. They discovered that, while family socioeconomic status had no effect on adolescent IA prevalence, the quality of the parent-adolescent relationship had a significant negative effect on adolescent IA prevalence. In conclusion, while a positive parent-child relationship can help adolescents feel accepted and act as a protective factor against IA (Ahmadi and Saghafi, 2013), prolonged parent–child conflict can cause adolescents to perceive themselves as rejected and act as a risk factor for IA (Ko et al., 2015). Thus, strengthening the parent-child relationship can help adolescents develop adaptive functioning and reduce their risk of developing IA (Kapetanovic et al., 2019; Skinner et al., 2021). For instance, Liu et al. (2015) discovered that family group therapy can address adolescents’ psychological needs by enhancing parent-adolescent communication and facilitating parent–adolescent relationships, ultimately resulting in effective treatment for adolescent IA.
Recently, researchers have begun to investigate the mediating effects of parent–adolescent relationships on adolescent IA in order to better understand how parent–adolescent relationships affect IA. There is evidence that factors such as emotion regulation ability (Wang J. et al., 2018) and peer relationships (Ding et al., 2017) mediate the relationship between parent-adolescent relationships and adolescent IA. The Problem-Behavior Theory (PBT) aims to explain how parent-adolescent relationship further influences adolescent IA as a risk behavior by altering individual characteristics of adolescents (Jessor, 2014). PBT recognizes that adolescents’ problem behaviors (e.g., alcohol abuse, violence, and IA) are the result of the relationship of environmental and individual factors. Family is the primary environment in which adolescents live, and parenting, supervision, and behavioral control interact with adolescents’ traits, thinking, and emotions to influence the quality of parent-adolescent relationships (Mo et al., 2018; Chung T. W. et al., 2019; Martins et al., 2020). The quality of the parent–adolescent relationship may enhance adolescents’ perceptions of social support and self-control, thereby influencing their IA (Li et al., 2017; Shek et al., 2018). With the increasing prevalence of adolescent IA in recent years, the relationship between the parent-adolescent relationship and adolescent IA has also been explored to some extent. However, these previous studies made little distinction between father–adolescent relationships (FAR) and mother–adolescent relationships (MAR), and thus do not know whether FAR/MAR both influence adolescent IA in the same way. Previous empirical studies on FAR/MAR and adolescent IA have found that, while increased father control over adolescent behavior predicted a slower decline in adolescent IA, increased maternal psychological control predicted a faster decline in IA (Shek et al., 2018). Additionally, Xu et al. (2014) concluded that relationships between mothers and adolescents are more likely to influence adolescent IA. Therefore, the first aim of this study was to distinguish FAR from MAR to explore how the direct and mediating effects of FAR and MAR differ in influencing adolescent IA.
The Mediating Role of Perceived Social Support
Perceived Social Support is a subjective emotional experience, and the more support and understanding an individual receives from family, peers, teachers, and others, the stronger the subjective social support (Kang et al., 2018). Adolescents generally receive social support from members of their environment, such as family, school, and community, and thus their Perceived Social Support is classified into three categories: family support, peer support, and other support (Hyun et al., 2015; Wang Y. et al., 2017). Adolescence is a period of rapid physical and psychological development during which adolescents frequently exhibit defiance, emotional instability, and difficulties with adaptation. Relational Regulation Theory (RRT) suggests that Perceived Social Support is always associated with good and healthy emotions and behaviors. Perceived Social Support is a positive experience resulting from ordinary and emotionally consequential conversations and shared activities that can effectively regulate adolescents’ feelings, thoughts, and actions, buffer adolescents from stress, promote mental health, and avoid problematic behaviors (Lakey and Orehek, 2011). Numerous studies have discovered that adolescents with low Perceived Social Support have impaired emotional regulation and report feelings of loneliness (Wang J. et al., 2018), depression (Ren et al., 2018; Wang P. et al., 2018), and psychological distress (Ren et al., 2018; Zhang et al., 2018). Although no empirical study has examined the relationship between Perceived Social Support, FAR/MAR, and adolescent IA directly, some indirect evidence suggests that Perceived Social Support mediates these associations. On the one hand, FAR/MAR is the primary channel through which adolescents access Perceived Social Support (Shaheen et al., 2019), and both daily positive parent-adolescent communication and behavioral relationships help adolescents increase their Perceived Social Support (Taylor et al., 2015). On the other hand, existing studies have found that Perceived Social Support can significantly and negatively predict adolescent IA (Karaer and Akdemir, 2019). In a family setting, elevated Perceived Social Support can be effective in preventing IA in adolescents (Gunuc and Dogan, 2013). Thus, FAR/MAR is associated with Perceived Social Support, which in turn may be associated with adolescent IA.
The Mediating Role of Dual System of Self-Control
Hofmann et al. (2009) proposed the Dual-System of Self-Control Model, arguing that a complete model of self-control should include both the Impulsive and Reflective Systems. The Impulsive System is a relatively fast processing method that rarely requires cognitive processing or attentional resources. It is an automatic behavioral schema that individuals gradually form based on their previous behavioral patterns and long-term learning experiences. The reflective System, which processes information in the opposite direction of the impulsive system, is primarily responsible for restraining an individual’s tendency to react impulsively and automatically through the establishment of high-level goals for assessing, monitoring, and managing behavior, and its operation requires the involvement of individual volitional effort and attentional resources (Lieberman, 2007). The system confers a greater degree of flexibility and control over decision-making and behavior, overcoming impulsive responses elicited by stimuli or temptations. Individual self-control is achieved through the manipulation of executive functions, which enable individuals to make deliberate judgments and assessments that either inhibit or overwhelm impulsive behavior (Hofmann et al., 2009; Gillebaart, 2018).
Puberty has been shown to be strongly associated with impulsivity (Niv et al., 2012). In adolescents, a decrease in the thickness of the cerebral cortex in the area of value selection is indicative of impulsivity (Pehlivanova et al., 2018). Additionally, there is an upward trend in executive functions among adolescents as they transition from adolescence to adulthood (Friedman et al., 2016). In general, the relationship of impulsivity and executive function results in significantly lower self-control in adolescence than in adulthood (Meldrum et al., 2012; Oliva et al., 2019). Numerous studies have established a link between the DSSC, Parent–Adolescent Relationship, and IA. For instance, Niu et al. (2020) discovered a positive correlation between self-control and the Parent–Adolescent Relationship and a negative correlation between self-control and adolescent problematic internet use. Additionally, self-control mitigates the effect of the Parent–Adolescent Relationship on problematic adolescent internet use. However, no study has examined the mediating role of DSSC to our knowledge. On the one hand, the relationship between a parent and an adolescent has a significant impact on adolescents’ impulsivity and executive functioning (Fay-Stammbach et al., 2014; Bennett and Blissett, 2017). Adolescents’ self-control is highly dependent on the parent–adolescent relationship (Brody et al., 2005; Liu et al., 2019). On the other hand, increased impulsivity (Babakr et al., 2019; Zhang Y. et al., 2021), as well as a deficiency in executive function (Li et al., 2014; Fumero et al., 2018; Kuo et al., 2018), are also major contributors to IA in the adolescent population. Thus, FAR/MAR is associated with DSSC in adolescents, which may be associated with IA.
Additionally, Perceived Social Support has been demonstrated to have a significant positive predictive effect on adolescent self-control. According to the Dual-System Model of Self-Control, social support may reduce impulsivity and increase executive function activation in adolescents (Sims et al., 2011; Khoo and Yang, 2020). FAR and MAR may affect DSSC via Perceived Social Support, which in turn affects adolescent IA. As a result, Perceived Social Support and DSSC may function as a chain mediating mechanism in FAR/MAR and adolescent IA.
The Present Study
The purpose of this study was to examine how FAR/MAR affects adolescent IA. This study investigates the mediating effects of Perceived Social Support and DSSC on FAR/MAR and adolescent IA, as well as the differences in the effects of FAR and MAR on adolescent IA. To our knowledge, this is the first empirical study to examine the roles of FAR/MAR, Perceived Social Support, and DSSC in IA concurrently. The Hypothesis Model is illustrated in Figure 1. It is based on the RRT, PBT, and Dual-System of Self-Control Model (Hofmann et al., 2009; Lakey and Orehek, 2011; Jessor, 2014). We can hypothesize that (1) FAR/MAR has a significant negative predictive effect on adolescent IA; (2) Perceived Social Support mediates the effect between FAR/MAR and adolescent IA; (3) DSSC mediates the effect between FAR/MAR and adolescent IA; (4) Perceived Social Support and DSSC act as a chain mediator between FAR/MAR and adolescent IA; (5) The effect of MAR on adolescent IA was stronger compared to FAR.
Materials and Methods
Participants
A total of 976 Chinese adolescents were recruited from two public schools in Sichuan Province. Before beginning the study, we obtained informed consent from the adolescents, their guardians, and teachers. Adolescents could stop filling out the questions if they felt uncomfortable with them. A total of 732 valid questionnaires were returned after excluding non-completed questionnaires. The sample included 317 boys and 415 girls, ranging in age from 11 to 16 years (Mage = 13.83 years, SD = 1.20 years). Of these, 36 (4.9%) were 11-year-old students, 44 (6.0%) were 12-year-old students, 183 (25%) were 13-year-old students, 280 (38.3%) were 14-year-old students, 121 (16.5%) were 15-year-old students, and 68 (9.3%) were 16-year-old students. All students completed the questionnaire in a quiet classroom at the school.
Sample Size Determination
Considering the structural equation modeling approach used in this study, the sample size at the time of data analysis needed to meet the criterion of matching at least 10 participants for each free parameter (Bentler and Chou, 1987; Hu and Bentler, 1999). Because the Hypothesis Model contains 58 free parameters and the Correction Model contains 55 free parameters in this study, the minimum sample size should be greater than 580 participants. This study’s sample size was adequate.
Measurement
Parent–Adolescent Relationship Scale
The Parent–Adolescent Relationship Scale (PARS scale), developed by Buchanan et al. (1991), is widely used to assess parent-adolescent relationships. Parent–child relationships span a variety of dimensions, most notably attachment, parenting styles, parent–child communication, parent–child bonding, and parent–child conflict (Armstrong et al., 2018; River et al., 2022). The PARS used in this study focuses on parent–child communication and bonding and reflects the status of FAR and MAR by inquiring about adolescents’ communication and bonding with their fathers and mothers. The scale contains a total of 20 items and can be divided into two subscales: FAR (10 items, e.g., “Do you feel comfortable and natural when you express your emotions to your dad?”) and MAR (10 items, e.g., “When you want to talk to your mom, will she be willing to talk to you?”). Respondents were asked to rate the extent to which each item was true for them on a five-point scale (1 = strongly disagree, 5 = definitely applies). We summed items in each subscale, with higher scores indicating higher levels of parent–adolescent relationship. This scale has shown good reliability and validity in Chinese adolescents (Zhang et al., 2011). The Cronbach’s α coefficient in our study was 0.85 (FAR) and 0.89 (MAR).
Adolescent Pathological Internet Use Scale
The Adolescent Pathological Internet Use (APIU) scale developed by Li and Yang (2007) is widely used to assess IA. The scale contains a total of 38 items and can be divided into six subscales: salience (3 items, e.g., “Once I’m online, I don’t think about anything else”), tolerance (5 items, e.g., “I would rather hold back my bowel movements in order to stay online”), withdrawal symptoms (11 items, such as “When I can’t go online, I really want to know what’s happening online”), mood alteration (5 items, such as “When I’m depressed, going online can make me feel better “), social comfort (6 items, e.g., “I feel more comfortable when I communicate with others online”) and negative outcomes (8 items, e.g., “I sometimes skip class to go online “). Respondents were asked to rate the extent to which each item was true for them on a five-point scale (1 = never, 5 = always). We summed items in each subscale, with higher scores indicating higher levels of IA. This scale has shown good reliability and validity in Chinese adolescents (Liu et al., 2012). The Cronbach’s α coefficient in our study was 0.94.
Multidimensional Scale of Perceived Social Support
The Chinese version of the Multidimensional Scale of Perceived Social Support (MSPSS) is used to assess Perceived Social Support (Zimet et al., 1988). The scale contains a total of 12 items and can be divided into three subscales: family (four items, e.g., “My family can help me in a practical and concrete way”), friends (four items, such as “My friends can really help me”), and significant others (four items, such as “My teachers, relatives, and classmates are there for me when I have problems”). Respondents were asked to rate the extent to which each item was true for them on seven-point scale (1 = Very strongly disagree, 7 = Very strongly agree). We summed items in each subscale, with higher scores indicating higher levels of Perceived Social Support. This scale has shown good reliability and validity in Chinese adolescents (Wang L. et al., 2017). The Cronbach’s α coefficient in our study was 0.86.
Dual System of Self-Control Scale
The Chinese version of the Dual System of Self-Control (DSSC) scale is used to assess self-control ability, and it contains two subscales, impulse system and Reflective System (Xie et al., 2014). The impulse system subscale includes subscales: impulsive (six items, e.g., “I often do or say things without thinking”); easy distraction (three items, e.g., “I often feel unable to complete my tasks”); and delay gratification (three items, e.g., “I can’t save money for future purchases”). The Reflective System subscale includes two subscales: problem-solving (six items, e.g., “I will try everything to deal with this”) and future time view (three items, e.g., “I think we should plan our day in the morning”). Respondents were asked to rate the extent to which each item was true for them on five-point scale (1 = not at all true, 7 = very true). The higher the score on the impulse system subscale, the stronger the factors of impulsiveness, distraction, and delay gratification, and the weaker the self-control ability. The higher the score in the control system subscale, the more likely the problem is solved satisfactorily, the stronger the future time view, and the stronger the self-control. This scale has shown good reliability and validity in Chinese adolescents (Wang L. et al., 2017). The Cronbach’s α coefficient in our study was 0.82.
Data Analysis
Statistical analysis was performed using SPSS 23.0 software and Amos 26.0 software. It was divided into the following steps: (1) Data standardization and descriptive statistics (including the mean, standard deviation, Cronbach’s α, and correlation for each variable) were performed using SPSS software. (2) Multiple regression analysis using SPSS software was used to compare the effects of FAR and MAR on adolescent IA. (3) Structural equation modeling (SEM) was conducted to test the mediating role of preceived social support and DSSC between FAR/MAR and adolescent IA (with Maximum Likelihood estimation). Where the Chi-square to degrees of freedom ratio (x2/df) < 5, the comparative fit index (CFI) and Tucker-Lewis Index (TLI) indices were above 0.90, and the standardized root mean square residual (SRMR) and the root mean square error of approximation (RMSEA) were less than 0.08 show good model fit (Bentler and Chou, 1987). (4) Bias was corrected for by a bias-corrected non-parametric percentile bootstrap method with 5000 replicate samples using 95% confidence intervals (CI). Indirect effects were significant if the 95%CI did not include zero (Preacher and Hayes, 2008).
Normal Distribution and Multicollinearity
We used the Kolmogorov–Smirnov test to determine whether each variable was normally distributed on 732 valid samples, and the results indicated that each variable was normally distributed with a two-sided significance range of 0.058–0.18, implying that each variable was normally distributed. Additionally, the variance inflation factor (VIF) is a parameter that indicates the degree of cointegration in a multiple linear regression model, with tolerance equal to 1/VIF. If the VIF of the independent variables is greater than 5 and the tolerance value is greater than 0.2, the model is considered to have severe multicollinearity (Akinwande et al., 2015). The VIFs of the variables in this study ranged from 1.011 to 1.827; the tolerance ranged from 0.547 to 0.998, indicating that the model was not significantly multicollinear.
Item Parceling
The FAR and MAR are both one-dimensional instruments, and the questions are highly homogeneous. To avoid measurement error inflation of latent variables, which reduces the model’s fit, the factorial algorithm method was used in this study to package the FAR and MAR questions, and the ten FAR/MAR questions were combined into two questions each (Rogers and Schmitt, 2004). After factor analysis, the questions with the highest factor loadings were included as anchor items in the package, followed by the questions with the next highest factor loadings in reverse order according to the direction of the balance, and the score for each question combination after the package was equal to the average of the questions in the package. Due to the fact that the other questionnaires have their own dimensions, there is no reason to use the factorial algorithm method.
Control Variables
This study includes two demographic variables as control variables: age and gender (1 = male, 2 = female). The demographic control variables were chosen based on the findings of the study. To begin, adolescents may exhibit greater impulsivity than other age groups due to a more active nervous system, specifically the ventral striatum, during adolescence (Sherman et al., 2018; Rosenbaum and Hartley, 2019). Additionally, there is a non-linear increase in adolescents’ self-control throughout adolescence, which means that adolescents may have varying levels of self-control at various ages (Casey, 2015). Second, girls have greater self-control than boys among adolescents, which may be explained by the fact that girls are less impulsive during adolescence (Chapple and Johnson, 2007) and are better at contemplation and reflection (Burwell and Shirk, 2007). As a result of the preceding study, we used age and gender as control variables in our study and assigned them to the column of independent variables in multiple regression analysis. In SEM analysis, we assigned the two variables age and gender to the three latent variables: Impulsive System, Reflective System, and IA (Yang et al., 2010).
Results
Descriptive Statistics and Correlations
Table 1 contains the means, standard deviations, and correlation coefficients for each variable. The results indicated that Adolescent IA was significantly positively correlated with Impulsive System and negatively correlated with FAR/MAR, Perceived Social Support, and Reflective System; FAR/MAR was significantly positively correlated with Perceived Social Support, Reflective System, and Impulsive System; Perceived Social Support was significantly positively correlated with Reflective System and negatively correlated with Impulsive System; Impulsive System was significantly positively correlated with Reflective System; Impulsive System was significantly Additionally, the correlation coefficients between the main variables ranged between 0.34 and 0.55 in absolute value, and the significance coefficients between all variables were less than 0.001.
Multiple Linear Regression Analysis
Multiple linear regression analysis was used to compare the direct effects of FAR and MAR on adolescent IA while accounting for demographic and other primary variables. Clogg et al. (1995) contended that when variables are standardized to unify their scales, the magnitude of the effects of various independent variables on the dependent variable can be compared using regression coefficients. However, this comparison is not absolute; regression coefficients represent differences in the slopes of the various variables, which means that when the coefficient for variable an is greater than the coefficient for variable b, it indicates that changes in variable a have a greater effect on the dependent variable than changes in variable b. Therefore, after standardizing all variables, we used the demographic variables and the main variables including FAR/MAR as our independent variables and adolescent IA as the dependent variable.
The model’s results were summarized in Table 2. The fit of the model was satisfactory (F = 68.016, p < 0.001). The standardized R2 value was 0.397, indicating that the independent variables could account for 39.7 percent of the variance in the model IA. In addition, the results show that FAR had no effect on adolescent IA (β = –0.071, t = –1.88, p = 0.061), whereas MAR had a significant effect on adolescent IA (β = –0.108, t = –2.904, p = 0.003). In comparison to FAR, the regression coefficient of MAR on adolescent IA was larger and more significant. This suggests that mothers may exert a greater inhibitory effect on adolescent IA than fathers do in this model condition, a finding that is consistent with previous research (Xu et al., 2014; Shek et al., 2018). It is worth noting that, while the regression coefficients for MAR were greater than those for FAR, this does not imply that MAR had a greater effect on adolescent IA under any condition. More precisely, it means that for each unit increase in MAR, the propensity for adolescent IA may decrease by 0.108 units, when these independent variables are taken into account. Relatively, due to the non-significant regression coefficients for FAR, there may not be a significant change in adolescent IA propensity for each unit of FAR enhancement. Which of the following has a greater impact on adolescent IA: MAR or FAR? It may produce inconsistent results under different conditions, which may be influenced by how the parent-adolescent relationship is measured (Li et al., 2014; Kim and Kim, 2015), necessitating additional research.
Structural Equation Model Analysis
Due to the inconsistent range of scores across questionnaires, and to avoid impairing model fit by inflating the measurement error of the variables, this study transformed all variables into standardized variables with a mean of 0 and a standard deviation of 1 before conducting SEM analysis (Wang and Wang, 2019).
The test results of the Hypothesis Model are presented in Table 3 and Figure 2. where the regression coefficients of FAR on adolescent IA (β = –0.02, p > 0.05, 95% CI = [–0.12, 0.08]) and MAR on adolescent IA (β = –0.06, p > 0.05, 95% CI = [–0.15, 0.04]) were too small to be significant; the regression coefficients of FAR on Reflective System regression coefficient was small (β = 0.06, p > 0.05, 95% CI = [–0.05, 0.18]) with p-values greater than 0.05 and 95% CI including 0, all of which were non-significant paths. As a result, these paths have been eliminated from the Correction Model. The Correction Model’s test results are depicted in Figure 3.
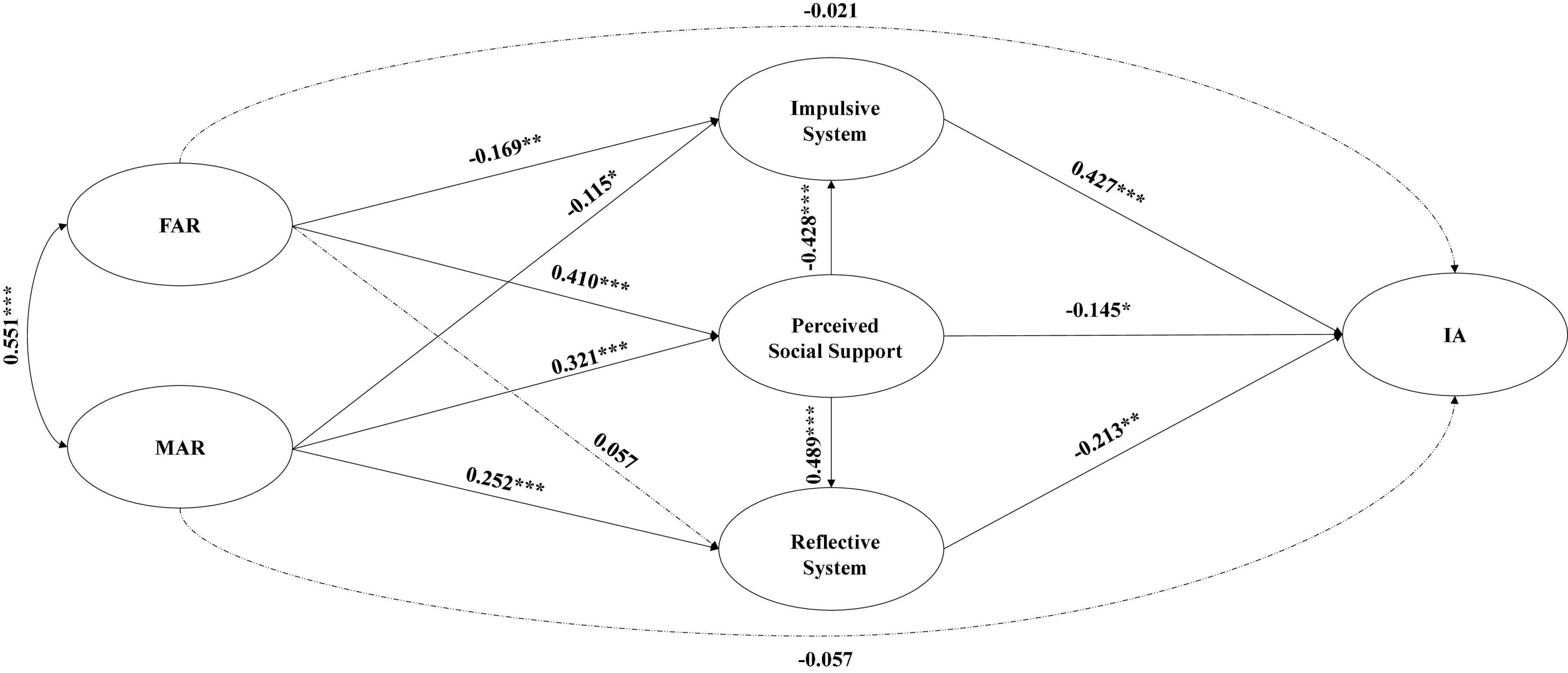
Figure 2. Hypothesis model. The dashed lines indicate paths with non-significant regression coefficients; control variables and precursor measures are not shown.
The difference in cardinality between the Hypothesis Model and Correction Model was x2 = 3.712, p < 0.05. As shown in Table 3, the Correction Model fit well and all criteria were met (Bentler and Chou, 1987). SEM and bootstrap analysis (5000 replicate samples) were used to validate the hypothesized mediated paths. As shown in Table 4, (1) in terms of total effect, both FAR and MAR had a significant negative predictive effect on adolescent IA [FAR: β = –0.271, 95% CI: (–0.342) – (–0.204); MAR: β = –0.276, 95% CI: (–0.347) – (–0.204)]. (2) In terms of direct effects, neither FAR (p > 0.05) nor MAR (p > 0.05) had a significant direct effect on adolescent IA, suggesting that FAR and MAR affect adolescent IA primarily through indirect effects (Preacher and Hayes, 2008). (3) In terms of indirect effects, the mediating effect of Perceived Social Support between FAR/MAR and adolescent IA was significant [FAR: β = –0.065, 95% CI: (–0.133) – (–0.004); MAR: β = –0.050, 95% CI: (–0.105) – (–0.004)]; Impulsive System had a significant mediating effect between FAR/MAR and adolescent IA [FAR: β = –0.075, 95% CI: (–0.129) – (–0.028); MAR: β = –0.054, 95% CI: (–0.102) – (–0.014)]; Reflective System had a significant mediating effect between MAR and adolescent IA [β = –0.070, 95% CI: (–0.143) – (–0.023)]; Perceived Social Support and Impulsive System had a significant chain mediating effect between FAR/MAR and adolescent IA [FAR: β = –0.077, 95% CI: (–0.119) – (–0.050); MAR: β = –0.060, 95% CI: (–0.093) – (–0.039)]; the chain mediated effect of Perceived Social Support and Reflective System between FAR/MAR and adolescent IA effects were significant [FAR: β = –0.054, 95% CI: (–0.104) – (–0.024); MAR: β = –0.042, 95% CI: (–0.087) – (–0.017)]. In summary, several of this study’s hypotheses were validated.
Discussion
Direct Relationship Between Father–Adolescent Relationships/Mother–Adolescent Relationships and Adolescent Internet Addiction
The purpose of this study was to examine the mechanism of the effect of FAR/MAR on adolescent IA and to compare the effect of FAR and MAR. In terms of the direct relationship between FAR/MAR and adolescent IA, both FAR and MAR demonstrated a significant negative correlation with IA, i.e., the stronger the FAR and MAR, the less likely adolescents were to develop IA, which was also consistent with prior research (Xu et al., 2014). However, inconsistent with existing research (Wang W. et al., 2018), the test results of the Hypothesis Model suggest that FAR and MAR do not have a direct effect on adolescent IA. One reason for this could be that this study did not directly analyze the parent-adolescent relationship as a separate variable when constructing the SEM for adolescent IA, but instead separated it into FAR and MAR to compare parent differences, weakening the direct effects of FAR and MAR on adolescent IA overall (Wang W. et al., 2018). Another reason could be that the FAR/MAR effect on adolescent IA in the multiple regression model is directed, whereas the effect of FAR/MAR on adolescent IA in the SEM contains indirect effects, and the coefficients of these mediating effects would somewhat attenuate the magnitude of the direct effects if they were larger (Iacobucci et al., 2007; Wang and Wang, 2019). Furthermore, Preacher and Hayes (2008) argued that the lack of a significant direct effect does not mean that FAR and MAR have no effect on IA, but rather that they influence adolescent IA primarily through mediating effects.
Mediating Relations Between Father–Adolescent Relationships/Mother–Adolescent Relationships and Adolescent Internet Addiction
To elucidate the relationship between FAR/MAR and adolescent IA, a chain-mediated model was developed in this study. We discovered that Perceived Social Support and DSSC play a significant role in the association between FAR/MAR and adolescent IA. Reduced FAR/MAR specifically reduces adolescents’ Perceived Social Support, increasing their risk of IA. According to the deficiency-compensation theory, the primary reason adolescents become addicted to the Internet world is their inability to obtain emotional fulfillment in the real world. As a result, adolescents develop an increasing reliance on Internet socialization, Internet gaming, and Internet entertainment activities to compensate for unmet emotional needs in real life, which gradually results in IA (Gao and Chen, 2006). Thus, a lack of Perceived Social Support as a result of a decrease in FAR or MAR may be a significant factor in adolescent IA. Additionally, the lower FAR/MAR found in this study may increase the risk of IA by activating the Impulsive System and enhancing impulsivity in adolescents. Previous research has established a strong link between adolescence and impulsivity (Niv et al., 2012), and impulsivity in adolescence is primarily due to two physiological and environmental factors. With age, physiologically induced impulsivity decreases (Friedman et al., 2016; Pehlivanova et al., 2018). However, PBT suggests that problems in the living environment, particularly family problems, may play a significant role in adolescent impulsivity and ultimately lead to IA. A significant manifestation of family problems is the deterioration of the parent-adolescent relationship. Adolescents are prone to conflict with their parents, impairing their FAR or MAR (Chaplin et al., 2012). As a result of declining FAR or MAR, parental discipline and management of adolescents become more difficult, promoting impulsivity and ultimately increasing the risk of IA (Ding et al., 2017). In contrast, effective FAR and MAR can assist parents in communicating with their adolescents and reducing impulsivity, thereby suppressing adolescent IA (Liu et al., 2012; Martins et al., 2020).
More importantly, this study discovered that FAR/MAR enhanced adolescents’ Perceived Social Support, which in turn inhibited the Impulsive System’s activation and promoted the Reflective System’s activation, ultimately promoting adolescents’ self-control and lowering their risk of IA. According to the Dual-System Model of Self-Control, the impulsivity and Reflective Systems work in tandem to determine adolescents’ motivation for self-control. FAR/MAR can significantly improve adolescents’ perceptions of parental support (Karaer and Akdemir, 2019), thereby mitigating adolescents’ conflict with their parents and inhibiting impulsivity (Hamama and Ronen-Shenhav, 2012). Furthermore, increased parental support enables parents to carry out normal monitoring and parenting functions for their adolescents, reinforcing adolescents’ self-monitoring functions (Pilcher and Bryant, 2016). Thus, the processes contribute to the Reflective System’s dominance in the DSSC, which ultimately exhibits greater self-control (Zhang R. et al., 2021). In conclusion, this study demonstrates the importance of emphasizing both Perceived Social Support and DSSC when attempting to reduce the risk of IA in adolescents via FAR/MAR. Furthermore, if parents, teachers, or adolescents themselves wish to reduce the risk of adolescent IA via FAR/MAR, particular attention should be paid to the combined role of Perceived Social Support and DSSC.
Differences Among Father–Adolescent Relationships, Mother–Adolescent Relationships to Adolescent Internet Addiction
The most significant and intriguing findings in this study suggest that the Reflective System mediates the relationship between MAR and adolescent IA, but that FAR does not affect adolescent IA via the Reflective System. i.e., MAR can enhance the activation of the adolescent Reflective System, thereby promoting self-monitoring and reflection and lowering the risk of IA in adolescents, whereas FAR does not. The current findings cast doubt on the hypothesis that the main method of preventing IA in adolescents is to enhance parent-adolescent relationship and that FAR acts similarly to MAR (Wang W. et al., 2018). In opposition to that, the current study suggests that MAR may play a more prominent role in promoting adolescent self-reflection and monitoring functions necessary for preventing IA. The presence of MAR is critical for the development of adolescent self-regulation, i.e., MAR facilitates adolescent self-monitoring, assessment, modification, and inhibition of their behavior or emotions more than parent-adolescent relationships or parenting styles do (Moilanen et al., 2010). Self-reflection and monitoring functions, on the other hand, enable adolescents to keep a watch on and monitor their Internet use, effectively lowering the risk of IA among adolescents (Leménager et al., 2016; Wang et al., 2021).
Additionally, we discovered that low FAR was associated with adolescent IA, but the association was less significant than the association with MAR, which is consistent with previous research (Xu et al., 2014). This finding may imply that MAR has a more pronounced and representative effect on adolescent IA than FAR. According to parental investment theory, fathers and mothers contribute differently to adolescent development. Mothers are primarily responsible for preventing adolescent emotional and behavioral development, whereas fathers are primarily responsible for ensuring the family’s proper functioning (Bjorklund and Kipp, 1996). Although FAR and MAR are essentially the same relationship and both are used to reflect the emotional cohesion between father/mother and adolescent (Russell and Saebel, 1997), parental involvement in parenting styles contributes to the FAR/differential MAR’s effects on adolescent IA. The differences in Perceived Social Support and DSSC elicited by FAR/MAR resulted in FAR/MAR having a different effect on adolescent IA. In diverse cultural contexts, a low FAR is more likely to trigger emotional and behavioral problems in adolescents than a high MAR (Baker, 2017; Pitsoane and Gasa, 2018). The primary reason for this may be that fathers exert less behavioral control over adolescents than mothers (Shek et al., 2018). Through their thoughts and emotions, mothers are more likely to convince adolescents that “IA is unacceptable behavior,” and good MAR facilitates this process of change. As a result, the presence of MAR is more important than the presence of FAR in promoting Reflective System activation and preventing IA in adolescents. When combined with the direct and indirect associations between FAR/MAR and adolescent IA found in this study, these findings suggest that FAR and MAR exert distinct effects on adolescent IA and that MAR is critical for reducing the risk of adolescent IA. From a cultural standpoint, the majority of Chinese adolescents regard fathers as serious and cognitive figures. Fathers frequently impose numerous behavioral restrictions on adolescents to teach them what they are not allowed to do, including restrictions on the use of Internet devices (Li and Lamb, 2013). This parental restraint contributes to adolescents’ reflection and monitoring of Internet device use, thereby lowering their risk of IA. However, when communication between fathers and adolescents breaks down for a variety of reasons, resulting in low FAR (Russell and Saebel, 1997; Pitsoane and Gasa, 2018), adolescents may become liberated from their fathers’ restraint, and FAR loses its ability to influence adolescents’ IA via the Reflective System.
Additional research is needed in the future to elucidate additional mechanisms underlying the effects of FAR/MAR on adolescent IA and to compare the effects of FAR and MAR. Mothers are more likely to influence adolescents’ perceptions of “IA as unacceptable behavior” through their thoughts and emotions, and a positive MAR aids in this process of change. As a result, the presence of MAR is more important than FAR in promoting Reflective System activation and preventing IA in adolescents. Taken together with the direct and indirect associations between FAR/MAR and adolescent IA found in this study, these findings suggest that FAR and MAR exert distinct effects on adolescent IA and that MAR is critical for reducing the risk of adolescent IA. Additional research is required in the future to elucidate additional mechanisms underlying the effects of FAR/MAR on adolescent IA and to compare the effects of FAR and MAR.
Implications for Practical Services
To our knowledge, this is the first study to examine the mediating role of perceived and congregational support, as well as DSSC, in the relationship between FAR/MAR and adolescent IA, by comparing the effects of FAR and MAR on adolescent IA and contributing to our understanding of adolescent IA.
From a practical standpoint, our findings may contribute to the development of practical prevention and intervention strategies for reducing IA in adolescents. To begin, family intervention programs for adolescents with IA should be expanded to increase adolescents’ Perceived Social Support through FAR/MAR promotion. And, on this basis, adolescents should be guided toward developing self-reflective and monitoring abilities to rein in their impulsivity and thus reduce their risk of IA (Gunuc and Dogan, 2013). Second, and perhaps most importantly, different intervention plans should be developed for families with varying FAR/MAR statuses in various situations. For families with low FAR but a high MAR, priority can be given to fostering FAR to compensate for the perceived lack of social support and to promoting self-control to prevent adolescent IA (Liu et al., 2015). Priority should be given to fostering MAR in families with both low FAR and MAR, as MAR plays a critical role in inhibiting adolescent IA. Thirdly, the discovery that Perceived Social Support has a negative predictive effect on the Impulsive System while having a positive predictive effect on the Reflective System provides critical practice insights. To prevent and intervene with IA in adolescents, families, schools, and communities can establish a strong social support system (Shaheen et al., 2019). This system can help adolescents receive more social support, which can help reduce Impulsive System activation and promote the development of the Reflective System, which can help adolescents achieve self-control of IA.
Limitations of This Study
This study is not without limitations. To begin, this study’s participants were concentrated in early adolescence. IA was more prevalent in early adolescence than in late adolescence (Moilanen et al., 2010). This means that the study’s findings may be slightly skewed and may not accurately reflect the general adolescent population. Second, this study focused primarily on the effect of perceived family support on adolescents’ IA. However, social support from school and community settings is critical in suppressing adolescent IA, and the family, school, and community all influence adolescent IA in different ways (Liu et al., 2015; Shaheen et al., 2019). As a result, additional research is required in the future to examine the effects of social support on adolescent IA in a variety of contexts. Third, only the cases of Perceived Social Support, Impulsivity System, and Reflective System as variable conditions were considered when comparing the differences in effects between FAR and MAR. MAR and FAR may also have opposite predictive effects on adolescent IA in other circumstances. As a result, the explanation for the causal relationship between FAR/MAR and adolescent IA becomes weaker. Future research should consider a longitudinal design that incorporates additional data on adolescent Internet use in order to reach more conclusive conclusions. Fourthly, future research could focus on family-centered approaches to adolescent IA interventions. Adolescents today face a complex and changing world filled with Internet device temptations, and not every adolescent is able to successfully avoid or overcome IA. Positive parental attitudes toward adolescents can contribute to the development of positive parent–adolescent relationships, which can significantly reduce or eliminate adolescent IA. Once adolescents develop an Internet addiction, parents should attempt to minimize the adolescent’s Internet identity. Parents’ love and trust motivate their children to overcome IA, and parents’ and children’s collaborative efforts are critical in assisting their children in overcoming IA.
Data Availability Statement
The raw data supporting the conclusions of this article will be made available by the authors, without undue reservation.
Ethics Statement
The studies involving human participants were reviewed and approved by Ethics Committee of Sichuan Normal University. Written informed consent to participate in this study was provided by the participants’ legal guardian/next of kin.
Author Contributions
HQ: significant contribution to the design and writing of the study. QK: significant contribution to the writing and data collection and analysis. CB: significant contribution to the design and data collection aspects of the study. All authors: contributed to the article and approved the submitted version.
Funding
This work was supported by Sichuan Applied Psychology Research Center (CSXL-21101).
Conflict of Interest
The authors declare that the research was conducted in the absence of any commercial or financial relationships that could be construed as a potential conflict of interest.
Publisher’s Note
All claims expressed in this article are solely those of the authors and do not necessarily represent those of their affiliated organizations, or those of the publisher, the editors and the reviewers. Any product that may be evaluated in this article, or claim that may be made by its manufacturer, is not guaranteed or endorsed by the publisher.
References
Ahmadi, K., and Saghafi, A. (2013). Psychosocial profile of Iranian adolescents’ Internet addiction. Cyberpsychol. Behav. Soc. Network. 16, 543–548. doi: 10.1089/cyber.2012.0237
Akinwande, M. O., Dikko, H. G., and Samson, A. (2015). Variance inflation factor: as a condition for the inclusion of suppressor variable (s) in regression analysis. Open J. Stat. 5:754. doi: 10.4236/ojs.2015.57075
Anderson, E. L., Steen, E., and Stavropoulos, V. (2017). Internet use and problematic internet use: a systematic review of longitudinal research trends in adolescence and emergent adulthood. Int. J. Adolesc. Youth 22, 430–454. doi: 10.1080/02673843.2016.1227716
Armstrong, E., Eggins, E., Reid, N., Harnett, P., and Dawe, S. (2018). Parenting interventions for incarcerated parents to improve parenting knowledge and skills, parent well-being, and quality of the parent–child relationship: a systematic review and meta-analysis. J. Exp. Criminol. 14, 279–317. doi: 10.1007/s11292-017-9290-6
Babakr, Z. H., Majeed, K., Mohamedamin, P., and Kakamad, K. (2019). Internet Addiction in kurdistan university students: prevalence and association with self-control. Eur. J. Edu. Res. 8, 867–873. doi: 10.12973/eu-jer.8.3.867
Baker, C. E. (2017). Father-son relationships in ethnically diverse families: Links to boys’ cognitive and social emotional development in preschool. J. Child Family Stud. 26, 2335–2345. doi: 10.1007/s10826-017-0743-3
Bennett, C., and Blissett, J. (2017). Parental monitoring may protect impulsive children from overeating. Pediatric Obes. 12, 414–421. doi: 10.1111/ijpo.12159
Bentler, P. M., and Chou, C. P. (1987). Practical issues in structural modeling. Sociol. Methods Res. 16, 78–117. doi: 10.1177/0049124187016001004
Bjorklund, D. F., and Kipp, K. (1996). Parental investment theory and gender differences in the evolution of inhibition mechanisms. Psychol. Bull. 120, 163–188. doi: 10.1037/0033-2909.120.2.163
Blachnio, A., Przepiorka, A., Gorbaniuk, O., Benvenuti, M., Ciobanu, A. M., Senol-Durak, E., et al. (2019). Cultural correlates of internet addiction. Cyberpsychol. Behav. Soc. Network. 22, 258–263. doi: 10.1089/cyber.2018.0667
Brody, G. H., McBride Murry, V., McNair, L., Chen, Y. F., Gibbons, F. X., Gerrard, M., et al. (2005). Linking changes in parenting to parent–child relationship quality and youth self-control: the strong african american families program. J. Res. Adolesc. 15, 47–69.
Bu, H., Chi, X., and Qu, D. (2021). Prevalence and predictors of the persistence and incidence of adolescent internet addiction in Mainland China: a two-year longitudinal study. Addict. Behav. 122:107039. doi: 10.1016/j.addbeh.2021.107039
Buchanan, C. M., Maccoby, E. E., and Dornbusch, S. M. (1991). Caught between parents: Adolescents’ experience in divorced homes. Child Dev. 62, 1008–1029. doi: 10.1111/j.1467-8624
Burwell, R. A., and Shirk, S. R. (2007). Subtypes of rumination in adolescence: Associations between brooding, reflection, depressive symptoms, and coping. J. Clin. Child Adolesc. Psychol. 36, 56–65. doi: 10.1080/15374410709336568
Casey, B. J. (2015). Beyond simple models of self-control to circuit-based accounts of adolescent behavior. Ann. Rev. Psychol. 66, 295–319. doi: 10.1146/annurev-psych-010814-015156
Cerniglia, L., Zoratto, F., Cimino, S., Laviola, G., Ammaniti, M., and Adriani, W. (2017). Internet addiction in adolescence: neurobiological, psychosocial and clinical issues. Neurosci. Biobehav. Rev. 76, 174–184. doi: 10.1016/j.neubiorev.2016.12.024
Chaplin, T. M., Sinha, R., Simmons, J. A., Healy, S. M., Mayes, L. C., Hommer, R. E., et al. (2012). Parent–adolescent conflict interactions and adolescent alcohol use. Addict. Behav. 37, 605–612. doi: 10.1016/j.addbeh.2012.01.004
Chapple, C. L., and Johnson, K. A. (2007). Gender differences in impulsivity. Youth viol. Juven. Just. 5, 221–234. doi: 10.1177/1541204007301286
Cheng, C., and Li, A. Y. L. (2014). Internet addiction prevalence and quality of (real) life: A meta-analysis of 31 nations across seven world regions. Cyberpsychol. Behav. Soc. Network. 17, 755–760. doi: 10.1089/cyber.2014.0317
Chung, S., Lee, J., and Lee, H. K. (2019). Personal factors, internet characteristics, and environmental factors contributing to adolescent internet addiction: a public health perspective. Int. J. Environ. Res. Public Health 16:4635. doi: 10.3390/ijerph16234635
Chung, T. W., Sum, S. M., and Chan, M. W. (2019). Adolescent internet addiction in Hong Kong: prevalence, psychosocial correlates, and prevention. J. Adolesc. Health 64, S34–S43.
Clogg, C. C., Petkova, E., and Haritou, A. (1995). Statistical methods for comparing regression coefficients between models. Am. J. Sociol. 100, 1261–1293. doi: 10.1086/230638
Davis, R. A. (2001). A cognitive-behavioral model of pathological Internet use. Comp. Human Behav. 17, 187–195. doi: 10.1016/S0747-5632(00)00041-8
Ding, Q., Li, D., Zhou, Y., Dong, H., and Luo, J. (2017). Perceived parental monitoring and adolescent internet addiction: a moderated mediation model. Addict. Behav. 74, 48–54. doi: 10.1016/j.addbeh.2017.05.033
Fay-Stammbach, T., Hawes, D. J., and Meredith, P. (2014). Parenting influences on executive function in early childhood: a review. Child Dev. Persp. 8, 258–264. doi: 10.1111/cdep.12095
Fioravanti, G., Dèttore, D., and Casale, S. (2012). Adolescent Internet addiction: testing the association between self-esteem, the perception of Internet attributes, and preference for online social interactions. Cyberpsychol. Behav. Soc. Network. 15, 318–323. doi: 10.1089/cyber.2011.0358
Friedman, N. P., Miyake, A., Altamirano, L. J., Corley, R. P., Young, S. E., Rhea, S. A., et al. (2016). Stability and change in executive function abilities from late adolescence to early adulthood: a longitudinal twin study. Dev. Psychol. 52:326. doi: 10.1037/dev0000075
Fumero, A., Marrero, R. J., Voltes, D., and Penate, W. (2018). Personal and social factors involved in internet addiction among adolescents: a meta-analysis. Comp. Human Behav. 86, 387–400. doi: 10.1016/j.chb.2018.05.005
Gao, W., and Chen, Z. (2006). A study on psychopathology and psychotherapy of Internet addiction. Adv. Psychol. Sci. 14:596.
Gillebaart, M. (2018). The ‘operational’definition of self-control. Front. Psychol. 9:1231. doi: 10.3389/fpsyg.2018.01231
Gunuc, S., and Dogan, A. (2013). The relationships between Turkish adolescents’ Internet addiction, their Perceived Social Support and family activities. Comp. Human Behav. 29, 2197–2207. doi: 10.1016/j.chb.2013.04.011
Ha, Y. M., and Hwang, W. J. (2014). Gender differences in internet addiction associated with psychological health indicators among adolescents using a national web-based survey. Int. J. Ment. Health Addict. 12, 660–669. doi: 10.1007/s11469-014-9500-7
Hamama, L., and Ronen-Shenhav, A. (2012). Self-control, social support, and aggression among adolescents in divorced and two-parent families. Child. Youth Serv. Rev. 34, 1042–1049. doi: 10.1016/j.childyouth.2012.02.009
Hofmann, W., Friese, M., and Strack, F. (2009). Impulse and self-control from a dual-systems perspective. Persp. Psychol. Sci. 4, 162–176. doi: 10.1111/j.1745-6924.2009
Hu, L. T., and Bentler, P. M. (1999). Cutoff criteria for fit indexes in covariance structure analysis: conventional criteria versus new alternatives. Struct. Equat. Model. Multidiscipl. J. 6, 1–55. doi: 10.1080/10705519909540118
Hyun, G. J., Han, D. H., Lee, Y. S., Kang, K. D., Yoo, S. K., Chung, U. S., et al. (2015). Risk factors associated with online game addiction: a hierarchical model. Comp. Human Behav. 48, 706–713. doi: 10.1016/j.chb.2015.02.008
Iacobucci, D., Saldanha, N., and Deng, X. (2007). A meditation on mediation: evidence that structural equations models perform better than regressions. J. Cons. Psychol. 17, 139–153.
Jessor, R. (2014). “Chapter 23: Problem behavior theory. A halfcentury of research on adolescent behavior and development,” in The developmental science of adolescence: history through autobiography, eds R. M. Lerner, A. C. Petersen, R. K. Silbereisen, and J. Brooks-Gunn (New York, NY: Psychology Press), 239–256.
Jia, J., Li, D., Li, X., Zhou, Y., Wang, Y., and Sun, W. (2017). Psychological security and deviant peer affiliation as mediators between teacher-student relationship and adolescent Internet addiction. Comp. Human Behav. 73, 345–352. doi: 10.1016/j.chb.2017.03.063
Jia, J., Li, D., Li, X., Zhou, Y., Wang, Y., Sun, W., et al. (2018). Peer victimization and adolescent Internet addiction: the mediating role of psychological security and the moderating role of teacher-student relationships. Comp. Human Behav. 85, 116–124. doi: 10.1016/j.chb.2018.03.042
Kang, H. W., Park, M., and Wallace, J. P. (2018). The impact of perceived social support, loneliness, and physical activity on quality of life in South Korean older adults. J. Sport Health Sci. 7, 237–244. doi: 10.1016/j.jshs.2016.05.003
Kapetanovic, S., Skoog, T., Bohlin, M., and Gerdner, A. (2019). Aspects of the parent–adolescent relationship and associations with adolescent risk behaviors over time. J. Family Psychol. 33:1. doi: 10.1037/fam0000436
Karaer, Y., and Akdemir, D. (2019). Parenting styles, Perceived Social Support and emotion regulation in adolescents with internet addiction. Comprehen. Psychiatry 92, 22–27. doi: 10.1016/j.comppsych.2019.03.003
Khoo, S. S., and Yang, H. (2020). Social media use improves executive functions in middle-aged and older adults: a structural equation modeling analysis. Comp. Human Behav. 111:106388. doi: 10.1016/j.chb.2020.106388
Kim, K., and Kim, K. (2015). Internet game addiction, parental attachment, and parenting of adolescents in South Korea. J. Child Adolesc. Subst. Abuse 24, 366–371. doi: 10.1080/1067828X.2013.872063
Ko, C. H., Wang, P. W., Liu, T. L., Yen, C. F., Chen, C. S., and Yen, J. Y. (2015). Bidirectional associations between family factors and I nternet addiction among adolescents in a prospective investigation. Psychiatry. Clin. Neurosci. 69, 192–200. doi: 10.1111/pcn.12204
Kuo, S. Y., Chen, Y. T., Chang, Y. K., Lee, P. H., Liu, M. J., and Chen, S. R. (2018). Influence of internet addiction on executive function and learning attention in Taiwanese school-aged children. Persp. Psychiatric Care 54, 495–500. doi: 10.1111/ppc.12254
Kuss, D. J., Griffiths, M. D., Karila, L., and Billieux, J. (2014). Internet addiction: a systematic review of epidemiological research for the last decade. Curr. Pharm. Des. 20, 4026–4052. doi: 10.2174/13816128113199990617
Kuss, D. J., Kristensen, A. M., and Lopez-Fernandez, O. (2021). Internet addictions outside of Europe: a systematic literature review. Comp. Human Behav. 115:106621. doi: 10.1016/j.chb.2020.106621
Kuss, D. J., Van Rooij, A. J., Shorter, G. W., Griffiths, M. D., and van de Mheen, D. (2013). Internet addiction in adolescents: prevalence and risk factors. Comp. Human Behav. 29, 1987–1996. doi: 10.1016/j.chb.2013.04.002
Lakey, B., and Orehek, E. (2011). Relational regulation theory: a new approach to explain the link between Perceived Social Support and mental health. Psychol. Rev. 118:482. doi: 10.1037/a0023477
Lauricella, A. R., Cingel, D. P., Blackwell, C., Wartella, E., and Conway, A. (2014). The mobile generation: youth and adolescent ownership and use of new media. Commun. Res. Rep. 31, 357–364. doi: 10.1080/08824096.2014.963221
Leménager, T., Dieter, J., Hill, H., Hoffmann, S., Reinhard, I., Beutel, M., et al. (2016). Exploring the neural basis of avatar identification in pathological Internet gamers and of self-reflection in pathological social network users. J. Behav. Addict. 5, 485–499. doi: 10.1556/2006.5.2016.048
Li, C., Dang, J., Zhang, X., Zhang, Q., and Guo, J. (2014). Internet addiction among Chinese adolescents: the effect of parental behavior and self-control. Comp. Human Behav. 41, 1–7. doi: 10.1016/j.chb.2014.09.001
Li, L., and Yang, Y. (2007). The development and validation of adolescent pathological Internet use scale. Acta Psychologica Sinica 39:688.
Li, S., Ren, P., Chiu, M. M., Wang, C., and Lei, H. (2021). The relationship between self-control and internet addiction among students: a meta-analysis. Front. Psychol. 12:755. doi: 10.3389/fpsyg.2021.735755
Li, X. N., Li, Z. Y., and Zhang, L. (2017). Relationships between social support and aggression of adolescents: the chain mediating roles of self-esteem and self-control. Psychol. Dev. Edu. 33, 240–248. doi: 10.16187/j.cnki.issn1001-4918.2017.02.13
Li, X., and Lamb, M. E. (2013). “Fathers in Chinese culture,” in Fathers in cultural context, eds D. W. Shwalb, B. J. Shwalb, and M. E. Lamb (New York, NY: Routledge), 15–41.
Lieberman, M. D. (2007). “The X- and C-Systems: The Neural Basis of Automatic and Controlled Social Cognition,” in Social neuroscience: Integrating biological and psychological explanations of social behavior, eds E. Harmon-Jones and P. Winkielman (New York, NY: The Guilford Press), 290–315.
Lin, M. P., Wu, J. Y. W., You, J., Hu, W. H., and Yen, C. F. (2018). Prevalence of internet addiction and its risk and protective factors in a representative sample of senior high school students in Taiwan. J. Adolesc. 62, 38–46. doi: 10.1016/j.adolescence.2017.11.004
Liu, L., Wang, N., and Tian, L. (2019). The parent-adolescent relationship and risk-taking behaviors among Chinese adolescents: the moderating role of self-control. Front. Psychol. 10:542. doi: 10.3389/fpsyg.2019.00542
Liu, Q. X., Fang, X. Y., Deng, L. Y., and Zhang, J. T. (2012). Parent–adolescent communication, parental Internet use and Internet-specific norms and pathological Internet use among Chinese adolescents. Comp. Human Behav. 28, 1269–1275. doi: 10.1016/j.chb.2012.02.010
Liu, Q. X., Fang, X. Y., Yan, N., Zhou, Z. K., Yuan, X. J., Lan, J., et al. (2015). Multi-family group therapy for adolescent Internet addiction: exploring the underlying mechanisms. Addict. Behav. 42, 1–8. doi: 10.1016/j.addbeh.2014.10.021
Martins, M. V., Formiga, A., Santos, C., Sousa, D., Resende, C., Campos, R., et al. (2020). Adolescent internet addiction–role of parental control and adolescent behaviours. Int. J. Pediat. Adolesc. Med. 7, 116–120. doi: 10.1016/j.ijpam.2019.12.003Get
Meldrum, R. C., Young, J. T., and Weerman, F. M. (2012). Changes in self-control during adolescence: investigating the influence of the adolescent peer network. J. Crimin. Just. 40, 452–462. doi: 10.1016/j.jcrimjus.2012.07.002
Mo, P. K., Chan, V. W., Chan, S. W., and Lau, J. T. (2018). The role of social support on emotion dysregulation and Internet addiction among Chinese adolescents: a structural equation model. Addict. Behav. 82, 86–93. doi: 10.1016/j.addbeh.2018.01.027
Moilanen, K. L., Shaw, D. S., and Fitzpatrick, A. (2010). Self-regulation in early adolescence: Relations with mother–son relationship quality and maternal regulatory support and antagonism. J. Youth .Adolesc. 39, 1357–1367. doi: 10.1007/s10964-009-9485-x
Niu, G., Yao, L., Wu, L., Tian, Y., Xu, L., and Sun, X. (2020). Parental phubbing and adolescent problematic mobile phone use: the role of parent-child relationship and self-control. Child. Youth Serv. Rev. 116:105247. doi: 10.1016/j.childyouth.2020.105247
Niv, S., Tuvblad, C., Raine, A., Wang, P., and Baker, L. A. (2012). Heritability and longitudinal stability of impulsivity in adolescence. Behav. Genet. 42, 378–392. doi: 10.1007/s10519-011-9518-6
Oliva, A., Antolín-Suárez, L., and Rodríguez-Meirinhos, A. (2019). Uncovering the link between self-control, age, and psychological maladjustment among Spanish adolescents and young adults. Psychosoc. Interv. 28, 49–55. doi: 10.5093/pi2019a1
Park, S. K., Kim, J. Y., and Cho, C. B. (2008). Prevalence of Internet addiction and correlations with family factors among South Korean adolescents. Adolescence 43:172.
Pehlivanova, M., Wolf, D. H., Sotiras, A., Kaczkurkin, A. N., Moore, T. M., Ciric, R., et al. (2018). Diminished cortical thickness is associated with impulsive choice in adolescence. J. Neurosci. 38, 2471–2481. doi: 10.1523/JNEUROSCI.2200-17.2018
Peng, W., Li, D., Li, D., Jia, J., Wang, Y., and Sun, W. (2019). School disconnectedness and Adolescent Internet Addiction: mediation by self-esteem and moderation by emotional intelligence. Comp. Human Behav. 98, 111–121. doi: 10.1016/j.chb.2019.04.011
Peris, M., DelaBarrera, U., Schoeps, K., and Montoya-Castilla, I. (2020). Psychological risk factors that predict social networking and internet addiction in adolescents. Int. J. Environ. Res. Public Health 17:4598. doi: 10.3390/ijerph17124598
Pilcher, J. J., and Bryant, S. A. (2016). Implications of social support as a self-control resource. Front. Behav. Neurosci. 10:228. doi: 10.3389/fnbeh.2016.00228
Pitsoane, E. M., and Gasa, V. G. (2018). The role of father-son relationship in behavioural and emotional development of adolescent boys. Gender Behav. 16, 10748–10757.
Preacher, K. J., and Hayes, A. F. (2008). Asymptotic and resampling strategies for assessing and comparing indirect effects in multiple mediator models. Behav. Res. Methods 40, 879–891. doi: 10.3758/BRM.40.3.879
Ren, P., Qin, X., Zhang, Y., and Zhang, R. (2018). Is social support a cause or consequence of depression? A longitudinal study of adolescents. Front. Psychol. 9:1634. doi: 10.3389/fpsyg.2018.01634
River, L. M., O’Reilly Treter, M., Rhoades, G. K., and Narayan, A. J. (2022). Parent–child relationship quality in the family of origin and later romantic relationship functioning: a systematic review. Family Proc. 61, 259–277. doi: 10.1111/famp.12650
Rogers, W. M., and Schmitt, N. (2004). Parameter recovery and model fit using multidimensional composites: a comparison of four empirical parceling algorithms. Multivar. Behav. Res. 39, 379–412. doi: 10.1207/S15327906MBR3903_1
Rosenbaum, G. M., and Hartley, C. A. (2019). Developmental perspectives on risky and impulsive choice. Philosoph. Trans. Royal Soc. B 374:133. doi: 10.1098/rstb.2018.0133
Russell, A., and Saebel, J. (1997). Mother–son, mother–daughter, father–son, and father–daughter: are they distinct relationships? Dev. Rev. 17, 111–147. doi: 10.1006/drev.1996.0431
Seidman, G. (2013). Self-presentation and belonging on Facebook: how personality influences social media use and motivations. Pers. Individ. Differ. 54, 402–407. doi: 10.1016/j.paid.2012.10.009
Shaheen, A. M., Hamdan, K. M., Albqoor, M., Othman, A. K., Amre, H. M., and Hazeem, M. N. A. (2019). Perceived Social Support from family and friends and bullying victimization among adolescents. Child. Youth Serv. Rev. 107:104503. doi: 10.1016/j.childyouth.2019.104503
Shek, D. T., and Yu, L. (2016). Adolescent internet addiction in Hong Kong: prevalence, change, and correlates. J. Pediatric Adolesc. Gynecol. 29, S22–S30. doi: 10.1016/j.jpag.2015.10.005
Shek, D. T., Zhu, X., and Ma, C. (2018). The influence of parental control and parent-child relational qualities on adolescent internet addiction: a 3-year longitudinal study in Hong Kong. Front. Psychol. 9:642. doi: 10.3389/fpsyg.2018.00642
Sherman, L., Steinberg, L., and Chein, J. (2018). Connecting brain responsivity and real-world risk taking: Strengths and limitations of current methodological approaches. Dev. Cogn. Neurosci. 33, 27–41. doi: 10.1016/j.dcn.2017.05.007
Sims, R. C., Levy, S. A., Mwendwa, D. T., Callender, C. O., and Campbell, A. L. (2011). The influence of functional social support on executive functioning in middle-aged African Americans. Aging Neuropsychol. Cogn. 18, 414–431. doi: 10.1080/13825585.2011.567325
Skinner, A. T., Godwin, J., Alampay, L. P., Lansford, J. E., Bacchini, D., Bornstein, M. H., et al. (2021). Parent–adolescent relationship quality as a moderator of links between COVID-19 disruption and reported changes in mothers’ and young adults’ adjustment in five countries. Dev. Psychol. 57:1236. doi: 10.1037/dev0001236
Talis, G. (2022). Internet Addiction in Substance and Non-Substance Related Addictions. Cham: Springer, 99–107. doi: 10.1007/978-3-030-84834-7_7
Taylor, Z. E., Conger, R. D., Robins, R. W., and Widaman, K. F. (2015). Parenting practices and perceived social support: longitudinal relations with the social competence of Mexican-origin children. J. Latin. Psychol. 3:193. doi: 10.1037/lat0000038
Wang, C. Y., Wu, Y. C., Su, C. H., Lin, P. C., Ko, C. H., and Yen, J. Y. (2017). Association between Internet gaming disorder and generalized anxiety disorder. J. Behav. Addict. 6, 564–571. doi: 10.1556/2006.6.2017.088
Wang, C., Zhang, Z., Che, L., Wu, Y., Qian, H., and Guo, X. (2021). The gray matter volume in superior frontal gyrus mediates the impact of reflection on emotion in Internet gaming addicts. Psychiatry Res. Neuroimag. 310:111269. doi: 10.1016/j.pscychresns.2021.111269
Wang, J., and Wang, X. (2019). Structural equation modeling: Applications using Mplus. New York, NY: John Wiley & Sons, doi: 10.1002/9781118356258
Wang, J., Mann, F., Lloyd-Evans, B., Ma, R., and Johnson, S. (2018). Associations between loneliness and Perceived Social Support and outcomes of mental health problems: a systematic review. BMC Psychiatry 18:1736. doi: 10.1186/s12888-018-1736-5
Wang, L., Tao, T., Fan, C., Gao, W., and Wei, C. (2017). The association between Internet addiction and both impulsivity and effortful control and its variation with age. Addict. Res. Theory 25, 83–90. doi: 10.1080/16066359.2016.1206082
Wang, P., Lei, L., Wang, X., Nie, J., Chu, X., and Jin, S. (2018). The exacerbating role of Perceived Social Support and the “buffering” role of depression in the relation between sensation seeking and adolescent smartphone addiction. Pers. Ind. Diff. 130, 129–134. doi: 10.1016/j.paid.2018.04.009
Wang, W., Li, D., Li, X., Wang, Y., Sun, W., Zhao, L., et al. (2018). Parent-adolescent relationship and adolescent internet addiction: a moderated mediation model. Addict. Behav. 84, 171–177. doi: 10.1016/j.addbeh.2018.04.015
Wang, Y., Wan, Q., Huang, Z., Huang, L., and Kong, F. (2017). Psychometric properties of multi-dimensional scale of Perceived Social Support in Chinese parents of children with cerebral palsy. Front. Psychol. 8:2020. doi: 10.3389/fpsyg.2017.02020
World Health Organization (2015). Public health implications of excessive use of the internet, computers, smartphones and similar electronic devices: Meeting report, Main Meeting Hall, Foundation for Promotion of Cancer Research, National Cancer Research Centre. Tokyo: World Health Organization.
Xie, D. J., Wang, L. G., Tao, T., Fan, C. L., and Gao, W. B. (2014). Validity and reliability of the chinese version of the dual-mode of self-control scale for adolescents. Chin. Ment. Health J. 28, 386–391. SUN: ZXWS.0.2014-05-016 doi: CNKI:
Xu, J., Shen, L. X., Yan, C. H., Hu, H., Yang, F., Wang, L., et al. (2012). Personal characteristics related to the risk of adolescent internet addiction: a survey in Shanghai, China. BMC Public Health 12:1106. doi: 10.1186/1471-2458-12-1106
Xu, J., Shen, L. X., Yan, C. H., Hu, H., Yang, F., Wang, L., et al. (2014). Parent-adolescent interaction and risk of adolescent internet addiction: a population-based study in Shanghai. BMC Psychiatry 14:112. doi: 10.1186/1471-244X-14-112
Yang, C., Nay, S., and Hoyle, R. H. (2010). Three approaches to using lengthy ordinal scales in structural equation models: parceling, latent scoring, and shortening scales. Appl. Psychol. Measur. 34, 122–142. doi: 10.1177/0146621609338592
Young, K. S. (1998). Internet addiction: the emergence of a new clinical disorder. Cyberpsychol. Behav. 1, 237–244. doi: 10.1089/cpb.1998.1.237
Young, K. S. (2004). Internet addiction: a new clinical phenomenon and its consequences. Am. Behav. Sci. 48, 402–415. doi: 10.1177/0002764204270278
Zhang, J., Liu, Q., Deng, Y., Fang, X., Liu, Z., and Lan, J. (2011). Parents-adolescent’s relations and adolescent’s internet addiciton: the mediaiton effect of loneliness. Psychol. Dev. Edu. 6, 641–647. doi: 10.16187/j.cnki.issn1001-4918.2011.06.003
Zhang, M., Zhang, J., Zhang, F., Zhang, L., and Feng, D. (2018). Prevalence of psychological distress and the effects of resilience and Perceived Social Support among Chinese college students: does gender make a difference? Psychiatry Res. 267, 409–413. doi: 10.1016/j.psychres.2018.06.038
Zhang, R., Qiu, Z., Li, Y., Liu, L., and Zhi, S. (2021). Teacher support, peer support, and externalizing problems among left-behind children in rural China: sequential mediation by self-esteem and self-control. Child. Youth Serv. Rev. 121:105824. doi: 10.1016/j.childyouth.2020.105824
Zhang, Y., Liu, Z., and Zhao, Y. (2021). Impulsivity, social support and depression are associated with latent profiles of internet addiction among male college freshmen. Front. Psychiatry 12:914. doi: 10.3389/fpsyt.2021.642914
Zhao, Q., Huang, Y., and Li, C. (2022). Does adolescents’ Internet addiction trigger depressive symptoms and aggressive behavior, or vice versa? The moderating roles of peer relationships and gender. Comp. Human Behav. 129:107143. doi: 10.1016/j.chb.2021.107143
Zhou, Y., Li, D., Li, X., Wang, Y., and Zhao, L. (2017). Big five personality and adolescent Internet addiction: the mediating2 role of coping style. Addict. Behav. 64, 42–48. doi: 10.1016/j.addbeh.2016.08.009
Keywords: adolescents, parent–adolescent relationship, internet addiction, perceived social support, dual system of self-control
Citation: Qi H, Kang Q and Bi C (2022) How Does the Parent–Adolescent Relationship Affect Adolescent Internet Addiction? Parents’ Distinctive Influences. Front. Psychol. 13:886168. doi: 10.3389/fpsyg.2022.886168
Received: 28 February 2022; Accepted: 11 May 2022;
Published: 07 June 2022.
Edited by:
Lenka Sokolová, Comenius University in Bratislava, SlovakiaReviewed by:
Meyran Boniel-Nissim, Kinneret College, IsraelKatarina Krizova, Comenius University in Bratislava, Slovakia
Copyright © 2022 Qi, Kang and Bi. This is an open-access article distributed under the terms of the Creative Commons Attribution License (CC BY). The use, distribution or reproduction in other forums is permitted, provided the original author(s) and the copyright owner(s) are credited and that the original publication in this journal is cited, in accordance with accepted academic practice. No use, distribution or reproduction is permitted which does not comply with these terms.
*Correspondence: Cuihua Bi, cuihuabi1215@163.com
†These authors share first authorship