- 1Department of Human-Artificial Intelligence Interaction, Sungkyunkwan University, Seoul, South Korea
- 2Department of Interaction Science, Sungkyunkwan University, Seoul, South Korea
The mobile game “Immortal Conquest,” created by NetEase Games, caused a dramatic user dissatisfaction event after an introduction of a sudden and uninvited “pay-to-win” update. As a result, many players filed grievances against NetEase in a court. The official game website issued three apologies, with mix results, to mitigate the crisis. The goal of the present study is to understand user feedback content from the perspective of Situational Crisis Communication Theory through semantic network analysis and sentiment analysis to explore how an enterprise’s crisis communication strategy affects users’ attitudes. First, our results demonstrate that the diminishing crisis communication strategies (excuse and justification) do not change players’ negative attitudes. It was not a failure because it successfully alleviated the players’ legal complaints and refocused their attention on the game itself. Second, the rebuild (apology & compensation) strategy was effective because it significantly increased the percentage of positive emotions and regenerated expectations for the game. The litigation crisis was identified within gamer communications with respect to Chinese gaming companies for the first time. Nevertheless, this does not indicate an increase in overall legal awareness among the larger Chinese population. It may only reflect greater legal awareness among Chinese online gamers. Fourth, gamers emphasized that they and enterprises should be equally involved when communicating with each other. Finally, in-game paid items should be reasonably priced, otherwise, they will drive users to competitors.
Introduction
With the outbreak of COVID-19, China has paid unprecedented attention to crisis communication via social media (Chen et al., 2020), prioritizing it as a tool for crisis communication in the face of major public health emergencies (Su et al., 2021). Moreover, the epidemic has prompted people to switch from offline communication to online or remote communication when dealing with disputes related to products or enterprises (Paska, 2021). Therefore, enterprises have to do so (Obrenovic et al., 2020; Flemming et al., 2021).
Enterprises use crisis communication on social media to maintain their reputation and market position (Utz et al., 2013; Bratu, 2019). Therefore, enterprises must evaluate whether crisis communication strategies have the expected impact on users. In 2021, academic studies on social media crisis communication mainly focused on communication strategies (Triantafillidou and Yannas, 2020; Wang et al., 2021) and information transmission modes (Mirbabaie et al., 2021; Puyod and Charoensukmongkol, 2021). However, there were few studies on whether crisis communication strategies of enterprises can affect users’ attitudes (Stieglitz et al., 2015; Mirbabaie and Zapatka, 2017).
User’s attitude refers to the individual’s proclivity to appraise the goal. For example, the studies of users’ attitudes toward vaccines (Gao et al., 2021), bitcoin (Mnif et al., 2021), social apps (Kocakoç and Özkan, 2022), the liberating movement of COVID-19 (Haupt et al., 2021), and other fields have proved the effectiveness of using social media content to explore user attitudes. Therefore, based on social media content, this study attempts to evaluate the influence of crisis communication tactics on user perceptions.
In the study of the attitude of users in crisis communication, Cho et al. (2021) used SNA to examine how racial concerns were portrayed in Twitter posts posted in English and Chinese, particularly how social media users blamed those responsible for the current crisis. Based on SCCT, Park and Park (2020) examined the public’s opinion on Samsung’s crisis communication strategy through SNA. These studies suggest that SNA can identify users’ attitudes or perceptions on social media. Therefore, this study first uses SNA to explore how an enterprise’s crisis communication strategy affects users’ attitudes.
RQ1. What were the reactions of users after NetEase issued three statements?
Although SNA plays an essential role in inferring the word clusters or framework, it is not easy to measure public emotions quantitatively. Therefore, to address the limitations of SNA, Stieglitz and Krüger (2011) analyzed tweets related to the 2010 Toyota crisis using Linguistic Inquiry and Word Count to categorize sentiment, allowing them to observe the changing trend of positive and negative public sentiments with the release of official declarations. Furthermore, Stieglitz et al. (2018) examined Volkswagen’s exhaust emission scandal and analyzed the mood and content of each period of that scandal. The study reported that Volkswagen’s tweets failed to soothe the negative emotions of consumers. These studies show that sentiment analysis has a noticeable effect on sentiment judgment. Therefore, sentiment analysis is used in the second step of this study to measure the impact of enterprises’ crisis communication strategies on users.
RQ2. How did users’ feelings change after NetEase released each statement?
To sum up, this study is based on the Situational Crisis Communication Theory (SCCT) and the crisis event that “Immortal Conquest,” a game subordinated to NetEase Games, encountered in the Chinese market in 2020. Furthermore, this paper uses Semantic Network Analysis (SNA) and sentiment analysis to explore how enterprises’ social media crisis communication strategies affect users’ attitudes.
We first used the SCCT model to classify the crisis communication strategies adopted by NetEase and then used SNA to analyze user comment texts after the release of official statements to understand the connection between critical issues in the crisis. Finally, sentiment analysis was used to judge the positivity and negativity of these texts. Since NetEase officially issued three statements, the changes of text content and emotional polarity in the comments are the keys to evaluating whether crisis communication strategies impact users’ attitudes.
Literature Review
Case Background
This paper analyzes the impact of enterprises’ crisis communication strategies on users’ attitudes in the aftermath of user complaints regarding the game Immortal Conquest, a free-to-play strategy war game developed and operated by the Chinese company NetEase. On May 27th, 2020, the company pushed a controversial update to the game, which implemented deeper integration of a Treasure system that allowed players to enhance their game experience using microtransactions. Many players voiced their concerns before the update, citing the likelihood of a vast “pay-to-win” trend that would not be limited to cosmetic upgrades. Indeed, the update put corporate income over the users’ experience, making it nearly impossible to compete in a fair setting. After the update, players amplified their voices of dissatisfaction on social media. Gamers arranged boycotts in several forms, and some users sued the company in the Court of Hangzhou. NetEase released three apology statements (see Table 1) on the official Immortal Conquest page on Sina Weibo, a Chinese microblogging site, attempting to control the damage.
Crisis Communication
Crisis communication refers to the continuous behavior and process of crisis resolution and crisis avoidance through communication (Seeger, 2006). It is regarded as an effective way to reduce the impact of a crisis on companies and to enhance user welfare. If a company handles it correctly, it can turn a crisis into an opportunity that the company can take advantage of (Ma et al., 2020). On the contrary, a lack of crisis communication can amplify the crisis causing severe damage or even a collapse of the organization.
Crisis communication is critical in crisis management. According to academic study, crisis communication theories primarily focus on the development of solutions, which aim to reduce the responsibility of organizations or individuals and help them tide over difficulties. For example, according to the image repair theory (Benoit, 2015), after the crisis, the main communication strategies of organizations include denial strategy, evading responsibility, reducing offensiveness, corrective action, and mortification. SCCT, which is based on attribution theory in social psychology and places defense tactics in stakeholder attribution, is another important theory in this subject (Coombs, 2007a). First, based on the public’s perception of crisis attribution responsibility, SCCT divides crisis into victim cluster, accidental cluster, and intentional cluster. Furthermore, the crisis response strategy is divided into deny strategy, diminishing strategy, and rebuild strategy; Finally, the crisis situation is matched with the coping strategy. According to SCCT, the most effective crisis communication strategy matches an organization’s rhetorical skills to the reputational threat (Coombs and Holladay, 2002).
In a word, crisis communication is studied to help enterprises maximize the probability of reaching a turning point in their crises and minimize the negative impact on consumers.
Semantic Network Analysis
Semantic network analysis is a method for representing knowledge in graphs in an organized manner. In a semantic network, information is represented as nodes connected by a set of marked directed lines that represent relationships between nodes (Kang et al., 2017). The assumption behind SNA is a specific connection between the words or concepts that frequently co-occur in the text, along with statistical indicators that can measure these connections. As a result, SNA enables the extraction of important ideas by recognizing emergent clusters of concepts rather than evaluating the frequency of solitary words (Kang et al., 2017; Golob et al., 2018). In this way, we can enhance our understanding of the text of social media users’ comments.
Therefore, with the rapid development of social media, SNA has become one of the commonly used case analysis methods of crisis communication in academia (Schultz et al., 2012; Park et al., 2019). In crisis communication, researchers and practitioners use SNA to infer the cluster composition of words. Then, according to their relevance, the terms are classified into different clusters. Finally, we can extensively explore social media users’ attitudes toward those responsible for the crisis and their main concerns (Scheufele, 1999).
For example, Tang et al. (2018) performed an SNA of people’s comments on Twitter during the measles epidemic of 2015. They found news updates, public health, vaccine, and political clusters and identified different adaptive crisis communications in different stages of the crisis based on these frameworks. Similarly, with the help of SNA, Park et al. (2019) identified that the content framework of tourism organizations dynamically changes in different stages of the crisis. Therefore, we also apply the feature of SNA in the exploration of this case.
Sentiment Analysis
Sentiment analysis is the process of evaluating, processing, inducing, and reasoning about subjective writings that have emotional hue (Zhao et al., 2020). For example, the Internet produces considerable user engaged, valuable information about people, happenings, goods, and so on. These remarks reflect people’s multiple emotional hues and inclinations, such as joy, rage, grief, criticism, admiration, and so on. As a result, potential users may read these subjective remarks to gain a better understanding of public opinion on a specific incident or item (Kim et al., 2021; Park et al., 2021).
As of 2021, there are two kinds of sentiment analysis methods: sentiment analysis based on lexicon and machine learning (Dhaoui et al., 2017). The lexicon-based sentiment analysis primarily creates a set of sentimental lexicons and rules, performs paragraph deconstruction, parsing, calculates sentimental value, and then utilizes the sentimental value as the foundation of the text’s sentimental tendency (Li and Wang, 2021). Most sentiment analyses based on machine learning translate this challenge into a classification working. The target sentiment is divided into two groups for the purpose of judging sentiment polarity: positive and negative. After manually labeling the training text, a supervised classification procedure is carried out (Bijlstra et al., 2010). For example, machine learning based on large-scale corpus is more common.
Compared with lexicon-based sentiment analysis, machine learning pays less attention to whether the text contains specific sentiment words and relies more on what categories the text is labeled in the training set. The result of machine learning depends on the corpus’s size and the accuracy of corpus annotation. In practice, sentiment analysis based on lexicons performs better in short texts, such as Facebook (Ortigosa et al., 2014), Twitter (Saif et al., 2016), and Sina Weibo (Xue et al., 2014). Meanwhile, the long text is better suited to machine learning. Therefore, this study uses sentiment analysis based on the lexicon.
Sentiment analysis has a good application effect in crisis communication, as it directly presents the two sides of social media users’ comments in the form of scores. For example, Bygstad and Presthus (2013) used Facebook to study customer communication cases of two Scandinavian airlines during the volcanic-ash crisis of April 2010. Their research showed that comparative sentiment analysis of two subjects was adequate to observe the differences in crisis communication strategies.
Sentiment analysis can also evaluate the effect of official crisis communication strategies. For example, Mishra and Sharma (2019) investigate the brand crisis experienced by Nestle’s instant noodle brand Maggi, as well as the extent to which a health-related problem might influence customer response on Facebook for a strong and trusted brand.
Sentiment analysis shows a unique understanding of public attitudes toward crisis communication strategies. Based on Twitter, Kaur et al. (2021) explored the impact of crisis communication strategies of Indian leaders in public health events after the outbreak of COVID-19 on the public’s emotions, which can be divided into 10 types, including anger, expectation, disgust, and fear. Similarly, based on Twitter, Tian et al. (2021) divided sentiments into eight categories, including arousal and valence, and examined the crisis of United Airlines’ in 2017. The authors found that social media sentiment analysis can help identify public responses and help enterprises adopt appropriate communication strategies. Therefore, we also applied the feature of Sentiment analysis to explore this case.
Situational Crisis Communication Theory
Based on the attribution theory, Coombs created SCCT in 2007, and it has emerged as the most widely used and authoritative Crisis Communication and Management Theory. SCCT says that organizations’ crisis communication strategies should be based on the nature of the crisis. SCCT has three main components: organizational crisis, crisis response strategy, and matching crisis situation and response strategy (Coombs, 2007b).
Some studies have classified communication strategies adopted by organizations using crisis response strategies based on SCCT (Park and Park, 2020) into four types: deny crisis response strategies, diminishing crisis response strategies, rebuild crisis response strategies, bolstering crisis response strategies (Coombs, 2007b). As a result, the same work is done in this study, SCCT is used to classify NetEase’s crisis communication tactics.
Deny crisis response strategies mainly include following: (a) Attack the accuser: crisis managers directly attack those who claim that the organization has problems. (b) Denial: the crisis manager asserts that there is no crisis. (c) Scapegoat: Other people or organizations are really responsible and insist that there is no crisis.
Diminishing crisis response strategies have two main options: (d) Excuse means to let the public believe that they have no intention to harm. (e) Justification provides rationalized explanations for the occurrence of crisis and reduces the perceived degree of crisis.
Rebuild crisis Response Strategies include following: (f) Compensation means to compensate the victim in the form of material or money. (g) Apology means admitting responsibility and making a frank apology to earn the victim’s or the public’s understanding.
Bolstering crisis response strategies focus on strengthening their positive image in various ways, including following: (h) Reminder: reminding the public of good experiences in the past, or (i) Ingratiation: pleasing stakeholders; (j) Victimage: conveying the message that they are also victims.
We qualitatively examined the crisis response strategies NetEase employed in social media from June 6, 2020, right after the incident, to June 9, 2020, as shown in Table 1.
Materials and Methods
Figure 1 shows the data processing process: how we collected, processed, and analyzed data. We pre-processed the data before answering the RQ: (1) used the Web Scraper to crawl data, (2) used Excel to clean the data, and (3) used Jieba for word segmentation.
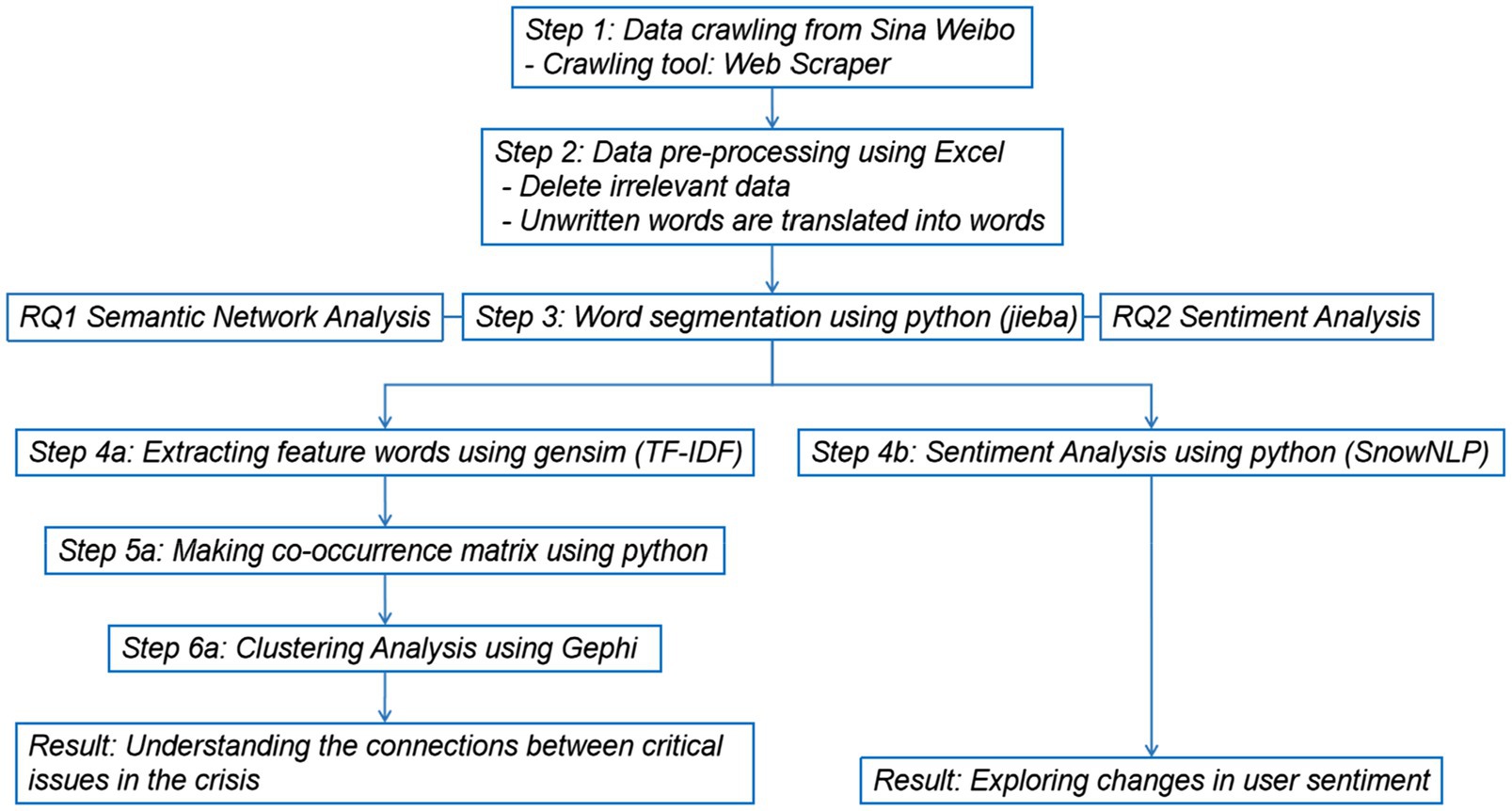
Figure 1. Research method for determining whether NetEase’s crisis communications were successful by combining SNA and sentiment analysis. TF-IDF, term frequency–inverse document frequency; Jieba, a Python package for word segmentation in the Chinese language.
SNA was used in RQ1, and the specific process can be divided into following steps: (4a) used Term Frequency–Inverse Document Frequency (TF-IDF) to extract feature words; (5a) obtained a co-occurrence matrix based on feature words; (6a) used Gephi to conduct clustering analysis.
Sentiment lexicon, a type of sentiment analysis, is used in RQ2. Sentiment analysis is divided into machine learning and sentiment lexicon, and the latter is adopted in this study.
Data Crawling
NetEase issued three apology statements via their official Sina Weibo account, eliciting various user responses. To collect these data, we used a web scraper plug-in in Google Chrome, enabling the browser to collect all comments by mimicking human mouse actions. Because the time interval between the three apology announcements was very short and this study only focused on the words under the official account, after data cleaning, we collected 2,066 responses to the first, 912 to the second, and 878 to the third statements (3,856 statements total).
Data Pre-processing
First, we eliminated data not relevant to this study (Che, 2021). As shown in Figure 2, we were only interested in the content of the text (in the blue box), and the rest of the data were deleted, such as posts ID, time of release, etc. Then, using MS Excel, non-essential columns were removed, including timestamps and user nicknames.
Sina Weibo automatically replaces abusive words with emoticons. Hence, we replaced those and user-added emoticons and symbols with emotion-colored textual statements to neutralize the non-textual data as much as possible.
Word Segmentation
Finally, we used the Jieba python package for word segmentation in Chinese. Jieba supports three modes of segmentation:
Precise mode cuts sentence precisely into slices suitable for text analysis.
Omnibus mode allows for the faster scanning of words in a sentence.
Search engine mode splits longer sentences into smaller ones to improve the recall rate for search engine segmentation.
After several comparisons, the precise mode was selected for this study because the omnibus mode does not resolve ambiguity and the search engine mode is more suitable for search engine analysis. In contrast, the precise mode is the most suitable for text analysis.
Data Analysis (RQ1 & RQ2)
This study combines SNA and sentiment analysis to measure whether the enterprise’s crisis communication strategy has the expected impact on users’ attitudes. First, SNA was used to conduct a content analysis on comments; then, sentiment analysis was used to calculate the emotional polarity of the comments. For example, suppose the proportion of negative emotions in the comments was much higher than positive ones. In this case, the high-frequency words and cluster analyses generated by SNA would not be positive.
SNA
Term Frequency-Inverse Document Frequency
We generated a “one-dimensional co-occurrence matrix” using the 50 most important keywords and estimated modularity to gauge the quality of the communities by calculating the term frequency-inverse document frequency (TF-IDF). The TF-IDF method is a statistical analytic method for determining the relative relevance of keywords in document sets or corpora. For example, if a word is somewhat uncommon yet appears frequently in a certain article, it will most likely represent the qualities of the piece.
We generated three datasets of comments (one for each apology statement) and used TF-IDF to extract the most relevant terms from each set. The relevance of a term was related to how many times it appeared in a comment and inversely proportional to how many times it appeared in a corpus. As a result, this computation approach effectively eliminated the effects of common terms on keywords while also improving the association between keywords and articles (Che et al., 2021).
Clustering Analysis Using Gephi Through Community Detection Algorithm
We constructed an undirected graph to study the high TF-IDF words in gamer comments. The nodes in the graphs represent common TF-IDF keywords, and the edges indicate the co-occurrence relationship between two nodes. Thus, the nodes’ size reflects the TF-IDF size, and the thickness of the edges reflects the frequency with which the two nodes appear together.
Community detection, a popular method in social network analysis, was utilized to further display nodes and edges. The Louvain algorithm is a modular community finding algorithm. Technique is effective in detecting hierarchical community structures. The detection of communities is accomplished by optimizing the modularity of the overall community network. The degree of tightness inside a community is described by modularity. Because the high degree of modularization reflects the excellent quality division, this study repeated the modular calculation many times to achieve the maximum results. The following is the calculation formula for modularization:
where is the proportion of two vertices of the community edge in community . The term after the formula indicates the proportion of at least one vertex of the edge in community . In a highly modularized network, the connections between nodes are dense, and the connections between nodes in different modules are sparse. A modularity score close to zero indicates that the fraction of edges among communities is no better than random and that close to one indicates that the network community structure is as strong as it can be (Chen et al., 2014).
Sentiment Analysis
The emotional tendency can be regarded as a tendency of inner evaluation and liking of the subject to the subjective existence of an object. Two aspects measure it: the direction of emotional inclination and the degree of an emotional preference. As of 2021, sentiment analysis approaches are classified into two types: those based on sentiment lexicons and on machine learning, such as machine learning based on large-scale corpora. The former requires a well-annotated emotional vocabulary, whereas the latter requires a large number of manually annotated corpora as a training set to achieve sentiment classification by extracting text features and building classifiers.
SnowNLP is a popular Python package in Chinese sentiment lexicon analysis in recent 2 years (Huang et al., 2021). Relevant studies have proven that SnowNLP is highly feasible and accurate (Chang et al., 2018; Tseng et al., 2018). It calculates a score per sentence and returns a value between zero and one, and it is used for sentiment analysis to determine the emotional polarity of comments. A score closer to one indicates a positive emotion, whereas a score closer to zero indicates a more negative emotion, and middling scores indicate neutral emotions. Sentiment analysis was used in this study to measure the impact of enterprises’ crisis communication strategies on users.
Results
Term Frequency-Inverse Document Frequency
In this study, we used the Gensim library in Python to calculate the TF-IDF. Figure 3 shows the top 50 words in word cloud form. Table 2 shows the top 10 words.
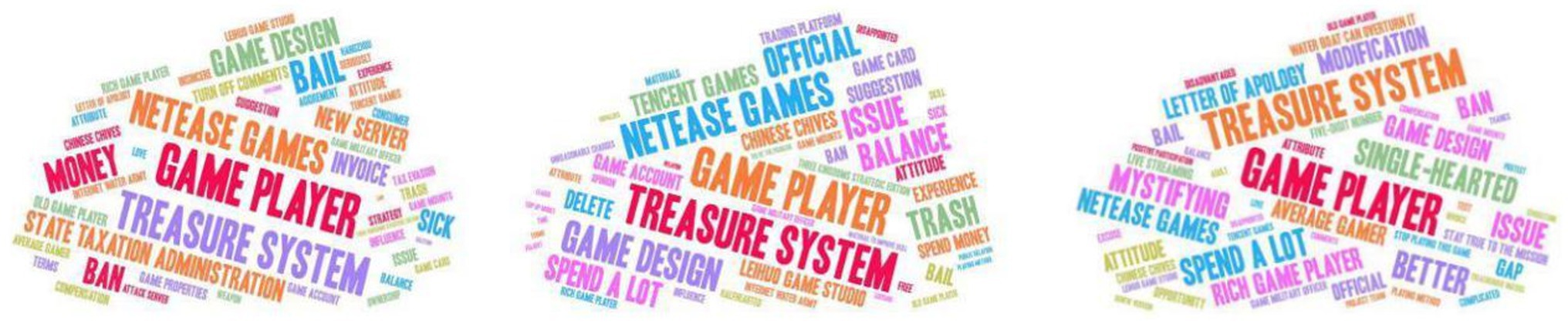
Figure 3. Term frequency–inverse document frequency word clouds of responses to the apology announcements.
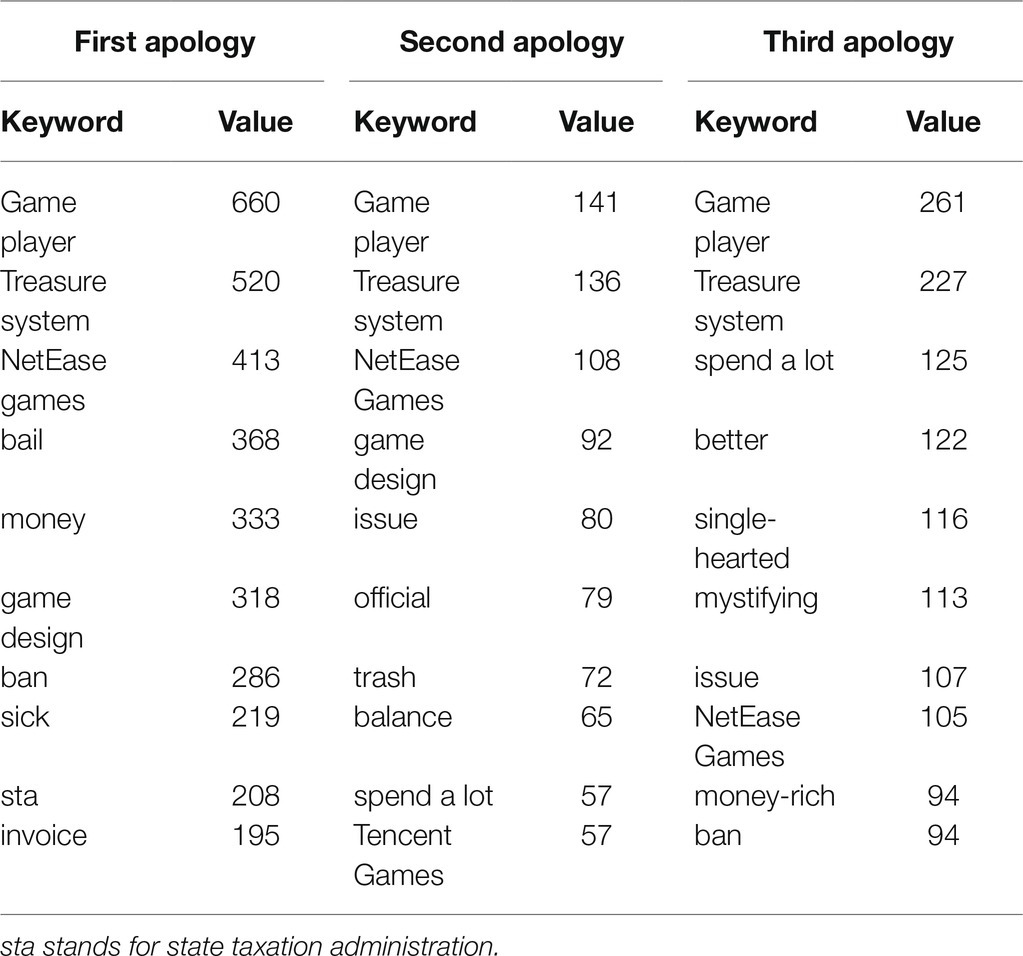
Table 2. Term frequency–inverse document frequency scores of responses to the apology announcements.
We can see from Figure 3 and Table 2 that Game player and Treasure system are consistently the most critical core words in the three reviews. Secondly, each comment data set is represented by specific terms, such as bail, money, and sta in the first data set; Issue, official, and trash in the second dataset; Better, single-hearted, and mystifying in the third dataset.
Clustering Analysis Using Gephi Through Community Detection Algorithm
In this study, GePhi0.9.2 was used to retrieve word clusters through a community detection algorithm and visualization technique. The layout algorithm selected was “Fruchterman Reingold,” for which the sizes of nodes and labels are determined according to their degree of centrality. The larger the degree of centrality, the larger the nodes and labels. In addition, the co-occurrence frequency determines the connection thickness between nodes. This study used the modularity function of Gephi, which has a built-in Louvain algorithm. Finally, nodes of the same color represent the same community.
Table 3 shows the percentages of each cluster for the three communications. Finally, we manually assigned the specific cluster description based on the text, as shown in Table 4.
First Apology Statement
As the cluster in Figure 4 shows, the green network accounted for 60% of the total comments, and it describes players’ dissatisfaction with punishments for game users and requirement for bail money to unlock accounts. Players further expressed their protest plans through various actions. “Sick,” “trash,” and “attitude” refer to the players’ attitude toward IC. “New servers” and “attack servers” refer to groups of players threatening or planning to attack the game’s servers, and “closed comments” refer to IC removing negative user comments.
The purple network (28%) describes how players felt about the “Treasure system,” with the keywords “game cards” and “balance” referring to the game’s new version disrupting the game’s content and balance, respectively.
The brown network (12%) refers to players who considered IC’s actions to have seriously violated the law. For example, players asked the State Taxation Administration to investigate IC because IC refused to issue receipts for the bail that IC asked players to pay.
Second Apology Statement
In the cluster shown in Figure 5, the brown network accounted for 28% of comments. Although not the most crucial cluster, this cluster has the most central node. From “Game player,” “Treasure system,” and “delete,” we can see that the Game players asked IC to remove the Treasure system.
The green network accounted for 38% of comments, showing players’ dissatisfaction with IC’s handling, including words like “handle,” “attitude,” “fine,” “half-hearted,” and “protest.” The purple network (34%) describes players who feel the Treasure system breaks the game’s balance and are dissatisfied with the game’s design and designers.
Third Apology Statement
As Figure 6 shows, the brown networks account for 40% of the comments. Players say the Treasure system breaks the game’s balance, widens the gap between money-rich and time-rich players, and makes it harder for ordinary players to enjoy the game.
The green network (54%) showed that Game players still expressed dissatisfaction with how the IC handled the problem. “Water can overturn a boat” is a Chinese saying that suggested IC to re-examine its relationship with its users.
In the purple network (6%), we found users advising IC to use this opportunity to improve its products, services, and relationships with customers.
Sentiment Dictionary Analysis
We further trained the data on the Jieba dictionary, manually adding 250 positive and 250 negative texts.
Overall Sentiment Proportion and Change Trend
As shown in Figure 7 and Table 5, the proportions of positive emotions corresponding to the first, second, and third apologies was 31.96, 32.85, and 40.58%, respectively. Conversely, the proportions of negative emotions to the first, second, and third apology statements were 68.04, 67.15, and 59.42%, respectively. Thus, overall, the proportion of positive comments gradually increased, and there was almost no change between the second and the first; the third apology showed an apparent upward trend.
The second official announcement did not work well as it did not address the players’ demands, whereas the third official announcement addressed some problems.
Sentiment Proportion and Change Trend in the Unit of Score Interval
This study divided the range (0–1) into five smaller intervals to observe the changes in sentiment values at smaller gaps.
As shown in Table 6, the emotional scores of the first apology were 46.61, 13.89, 14.67, 08.52, and 16.31%, respectively. The emotional scores of the second were 42.76, 16.24, 14.58, 11.73, and 14.70%, respectively. The emotional scores for the third were 35.99, 16.97, 13.90, 14.12, and 19.02%, respectively.
As shown in Figure 7, the first two statements of NetEase were dominated by words that reflected negative community responses. The negative emotions accounted for a high proportion of sentiment, for which explanations and excuses were near 50%. However, the positive sentiment significantly increased after the avoidance of explanatory words in the third statement.
Discussion
RQ1: What Were the Reactions of Users After NetEase Issued Three Statements?
To have a more intuitive understanding of the changes in the content discussed by users, we made the topic change diagram.
As shown in Figure 8, we found that the clusters (represented by the green line) spanned three review datasets, implying that IC’s three-time strategy (excuse, justification, and apology & compensation) did not resolve players’ persistent dissatisfaction with the way IC handled the incident.
The purple line showing an upward trend demonstrates that gamers were paying more attention to game content. This meant that IC’s third strategy (apology & compensation) may gradually directed the player’s attention to the game itself. No matter how much IC tried to explain the purpose of the Treasure system, the Treasure system was still perceived as unbalanced.
The legal action clusters (represented by the red line) appeared only in the first dataset. This indicates that the diminishing (justification) communication strategy adopted by IC in the second apology successfully reduced or diverted the attention of Game players from creating a legal issue.
The clusters represented by the blue line appeared only in the third dataset. This reveals that the rebuild (apology & compensation) crisis communication strategy adopted by IC in the third apology positively impacted users’ attitudes. IC succeeded in making the Game players hope for the future of IC after the third apology.
RQ2: How Did Users’ Feelings Change After NetEase Released Each Statement?
As shown in Figure 7, the overall negative emotions (0 ≤ score ≤ 0.5) in the first two datasets barely changed (68.04% ≈ 67.15%), which may indicate that the diminishing (justification) crisis communication strategies adopted by IC in the second announcement was ineffective. However, Figure 9 shows that extreme negative sentiment (0 ≤ score ≤ 0.2) decreased slightly (46.62% → 42.82%), whereas milder negative sentiment (0.2 < score ≤ 0.4) increased slightly (13.92% → 16.27%). This result shows that although the Diminishing (justification) communication strategy adopted by IC for the second announcement was not effective, it still alleviated the more extreme expressions and appeals of Game players.
After IC proposed specific compensation measures in the third apology, the overall positive sentiment (0.5 < score ≤ 1.0) increased significantly (32.85% → 40.58%), indicating that IC’s communication strategy in the third apology (Rebuild (apology & compensation) crisis communication strategy) might have a positive impact on player attitudes.
Overall, the diminishing crisis communication strategies (excuse and justification) did not change players’ negative attitudes, so they were not successful. But it was not a failure because it successfully defused the player’s legal complaint and focused the player’s attention on the game itself. Finally, the rebuild (Apology & Compensation) strategy was the most successful strategy because it significantly increased the percentage of positive emotions and regenerated expectations for IC.
Notable Findings
For the first time, the litigation crisis was identified within gamer communications with respect to Chinese gaming companies.
“请你对我们所有违法行为做出解释,包括所谓的保释金.”
Translation: “Please account for all our violations, including the so-called bail money.”
Nevertheless, this does not indicate an increase in overall legal awareness among the larger Chinese population. It may only reflect greater legal awareness among Chinese online gamers. For example, Ma et al. found that Chinese people’s legal awareness of food safety was weak (Ma et al., 2020). Perhaps Chinese people have different legal awareness in various fields; this requires further research and observation.
In all three comments, “attitude” was at the forefront of the TF-IDF high-frequency word sets. By returning to the original text, we found that Game players believed that NetEase had a poor attitude toward their crisis communication process and that users were not treated well, as shown below:
“就这?这就是你们的态度?就拖呗,避重就轻呗.”
Translation: “Is this it? Is that your attitude? Just procrastinate, avoid the most important things.”
Game players desired “an equal relationship between game companies and their players.” However, NetEase failed to balance the relationship between the enterprise and its users, resulting in the phrase “Tencent Games” to appear in numerous comments. Tencent Games is NetEase’s biggest competitor in the Chinese market. Bolton et al. demonstrated that consumers would compare their earnings with corporate profits in a market environment and oppose unfair systems. If the pricing looks greedy and results in higher profits for the company than in consumer satisfaction, they may choose to avoid the product and instead buy products from competitors (Bolton et al., 2003).
Conclusion
This research considers the crisis events of NetEase Games’ game “Immortal Conquest” in the Chinese market in 2020 as the research object. Based on the SCCT, we used SNA and sentiment analysis to understand user feedback content and discussed how the corporate crisis communication strategy affects users’ attitudes.
First, our results demonstrate that the diminishing crisis communication (excuse and justification) strategies did not change players’ negative attitudes. Still, it was not a failure because it successfully defused the player’s legal complaint and focused the player’s attention on the game itself.
Second, the rebuild (apology & compensation) strategy was effective because it significantly increased the percentage of positive emotions and regenerated expectations for the game.
Third, the litigation crisis was identified within gamer communications with respect to Chinese gaming companies for the first time. Nevertheless, this does not indicate an increase in overall legal awareness among the larger Chinese population. It may only reflect greater legal awareness among Chinese online gamers. This requires further research and observation.
Fourth, gamers emphasize that they and enterprises should be equal when communicating, because we found that NetEase failed to balance the relationship between the enterprise and its users, and Game players believed that NetEase had a poor attitude toward their crisis communication process and that users were not treated well.
Fifth, as the data indicated in-game paid items should be reasonably priced. Otherwise, they will drive users to competitors.
Finally, this study suggests that after the outbreak of a crisis, the online game enterprises in crisis communication can use SNA first to understand the needs of users and the connection between critical issues in the crisis. Secondly, sentiment analysis is used to assess the effectiveness of the communication strategy, according to the validity used to adjust their communication strategy, finally used to achieve the goal of appeasing the crisis.
Limitations and Future Research
First, given that users’ emotions and demands can vary depending on age or gender, it may be meaningful to consider the age and gender of users when evaluating the crisis communication process.
Second, future studies need to evaluate the process of crisis communication in other countries and cultures using sentiment analysis and SNA.
Third, we replaced visual emoticons with words. While we minimized the impact of substitution, the effect of this behavior on the text is unmeasured. Therefore, we should strengthen the processing of visual emoticons in the future word processing of Sina Weibo (Sion, 2019).
Fourth, the relationship between social media content (Taylor et al., 2020), users’ emotions and demands, and mobile game addiction in terms of semantic network and sentiment analysis has not been covered (Mircică, 2020; Pop et al., 2021).
Finally, the relationship between social media crisis communications and visual content-sharing applications in terms of semantic network and sentiment analysis has not been covered (Bratu, 2019; Adams et al., 2020).
Data Availability Statement
The original contributions presented in the study are included in the article/supplementary material, and further inquiries can be directed to the corresponding author.
Author Contributions
SC: conceptualization, research design, implementation, writing, and editing. DN: conceptualization, writing, and editing. PK and SZ: writing and editing. JK: conceptualization, research design, writing, and editing. All authors contributed to the article and approved the submitted version.
Funding
This work was supported by a National Research Foundation of Korea (NRF) grant funded by the Korean government (NRF-2020R1A2C1014957).
Conflict of Interest
The authors declare that the research was conducted in the absence of any commercial or financial relationships that could be construed as a potential conflict of interest.
Publisher’s Note
All claims expressed in this article are solely those of the authors and do not necessarily represent those of their affiliated organizations, or those of the publisher, the editors and the reviewers. Any product that may be evaluated in this article, or claim that may be made by its manufacturer, is not guaranteed or endorsed by the publisher.
References
Adams, C., Grecu, I., Grecu, G., and Balica, R. (2020). Technology-related behaviors and attitudes: compulsive smartphone usage, stress, and social anxiety. Rev. Contemp. Philosop. 19, 71–77. doi: 10.22381/RCP1920207
Benoit, W. L. (2015). “Image restoration theory,” in The International Encyclopedia of Communication. ed. W. Donsbach (United States: Wiley Online Library).
Bijlstra, G., Holland, R. W., and Wigboldus, D. H. J. (2010). The social face of emotion recognition: evaluations versus stereotypes. J. Exp. Soc. Psychol. 46, 657–663. doi: 10.1016/j.jesp.2010.03.006
Bolton, L. E., Warlop, L., and Alba, J. W. (2003). Consumer perceptions of price (un) fairness. J. Consum. Res. 29, 474–491. doi: 10.1086/346244
Bratu, S. (2019). Can social media influencers shape corporate brand reputation? Online followers’ trust, value creation, and purchase intentions. Rev. Contemp. Philosop. 18, 157–163. doi: 10.22381/RCP18201910
Bygstad, B., and Presthus, W. (2013). Social media as CRM? How two airline companies used Facebook during the “Ash Crisis” in 2010. Scand. J. Inf. Syst. 25, 1–22.
Chang, W., Xu, Z., Zhou, S., and Cao, W. (2018). Research on detection methods based on Doc2vec abnormal comments. Futur. Gener. Comput. Syst. 86, 656–662. doi: 10.1016/j.future.2018.04.059
Che, S. (2021). “A comparative analysis of attention to facial recognition payment between China and South Korea: a news analysis using Latent Dirichlet allocation.” in International Conference on Human-Computer Interaction ; July 3 2021 (Cham: Springer), 75–82.
Che, S., Nan, D., Kamphuis, P., Jin, X., and Kim, J. H. (2021). “A cluster analysis of Lotte young plaza using semantic network analysis method.” Paper presented at the 2021 15th International Conference on Ubiquitous Information Management and Communication (IMCOM) ; January 4–6, 2021 (Seoul, Korea), 1–6.
Chen, M., Kuzmin, K., and Szymanski, B. K. (2014). Community detection via maximization of modularity and its variants. IEEE Trans. Comput. Soc. Sys. 1, 46–65. doi: 10.1109/TCSS.2014.2307458
Chen, Q., Min, C., Zhang, W., Wang, G., Ma, X., and Evans, R. (2020). Unpacking the black box: how to promote citizen engagement through government social media during the COVID-19 crisis. Comput. Hum. Behav. 110:106380. doi: 10.1016/j.chb.2020.106380
Cho, M., Xiong, Y., and Boatwright, B. (2021). Through the lens of ethnicity: semantic network and thematic analyses of united airlines’ dragging crisis. Public Relat. Rev. 47:102006. doi: 10.1016/j.pubrev.2020.102006
Coombs, W. T. (2007a). Attribution theory as a guide for post-crisis communication research. Public Relat. Rev. 33, 135–139. doi: 10.1016/j.pubrev.2006.11.016
Coombs, W. T. (2007b). Protecting organization reputations during a crisis: the development and application of situational crisis communication theory. Corp. Reput. Rev. 10, 163–176. doi: 10.1057/palgrave.crr.1550049
Coombs, W. T., and Holladay, S. J. (2002). Helping crisis managers protect reputational assets: initial tests of the situational crisis communication theory. Manag. Commun. Q. 16, 165–186. doi: 10.1177/089331802237233
Dhaoui, C., Webster, C. M., and Tan, L. P. (2017). Social media sentiment analysis: lexicon versus machine learning. J. Consum. Mark. 34, 480–488. doi: 10.1108/JCM-03-2017-2141
Flemming, G., Jonas, H., Kroll, W., Michl, J., and Tetzel, M. H. (2021). Keeping up the dialog: understanding the use of social media by German mobility companies during the Covid-19 pandemic. SSOAR 75399, 39–68.
Gao, H., Guo, D., Wu, J., Zhao, Q., and Li, L. (2021). Changes of the public attitudes of china to domestic covid-19 vaccination after the vaccines were approved: a semantic network and sentiment analysis based on Sina Weibo texts. Front. Public Health 9:723015 doi: 10.3389/fpubh.2021.723015
Golob, U., Turkel, S., Kronegger, L., and Uzunoglu, E. (2018). Uncovering CSR meaning networks: a cross-national comparison of Turkey and Slovenia. Public Relat. Rev. 44, 433–443. doi: 10.1016/j.pubrev.2018.05.003
Haupt, M. R., Jinich-Diamant, A., Li, J., Nali, M., and Mackey, T. K. (2021). Characterizing twitter user topics and communication network dynamics of the “liberate” movement during COVID-19 using unsupervised machine learning and social network analysis. Soc. Network. Media 21:100114. doi: 10.1016/j.osnem.2020.100114
Huang, M., Wen, S., Jiang, M., and Yao, Y. (2021). “LDA topic Mining of Light Food Customer Reviews on the Meituan platform.” in Paper presented at the International Conference on Data Mining and Big Data ; October 30, 2021 (Springer, Singapore), 108–121.
Kang, G. J., Ewing-Nelson, S. R., Mackey, L., Schlitt, J. T., Marathe, A., Abbas, K. M., et al. (2017). Semantic network analysis of vaccine sentiment in online social media. Vaccine 35, 3621–3638. doi: 10.1016/j.vaccine.2017.05.052
Kaur, M., Verma, R., and Otoo, F. N. K. (2021). Emotions in leader’s crisis communication: twitter sentiment analysis during COVID-19 outbreak. J. Hum. Behav. Soc. Environ. 31, 362–372. doi: 10.1080/10911359.2020.1829239
Kim, J. H., Nan, D., Kim, Y., and Park, M. H. (2021). Computing the user experience via big data analysis: a case of Uber services. CMC 67, 2819–2829. doi: 10.32604/cmc.2021.014922
Kocakoç, I. D., and Özkan, P. (2022). “Clubhouse experience: sentiment analysis of an alternative platform From the eyes of classic social media users,” in Data Mining Approaches for Big Data and Sentiment Analysis in Social Media. United States: IGI Global, 244–264.
Li, Z., and Wang, X. (2021). Research on sentiment analysis model due to COVID-19 using social media. J. Phys. Conf. Series 1856:012064. doi: 10.1088/1742-6596/1856/1/012064
Ma, C.-C., Chen, H. S., and Chang, H. P. (2020). Crisis response and supervision system for food security: a comparative analysis between mainland China and Taiwan. Sustainability 12:3045. doi: 10.3390/su12073045
Mirbabaie, M., Stieglitz, S., and Brünker, F. (2021). Dynamics of convergence behaviour in social media crisis communication–a complexity perspective. Inf. Technol. People. doi: 10.1108/ITP-10-2019-0537 [Epub ahead of print]
Mirbabaie, M., and Zapatka, E. (2017). “Sensemaking in social media crisis communication–A case study on the Brussels bombings in 2016.” in Proceedings of the 25th European Conference on Information Systems (ECIS) ; June 5–10, 2017 (Guimarães, Portugal), 2169–2186.
Mircică, N. (2020). Restoring public trust in digital platform operations: machine learning algorithmic structuring of social media content. Rev. Contemp. Philosop. 19, 85–91. doi: 10.22381/RCP1920209
Mishra, M. S., and Sharma, R. W. (2019). Brand crisis-sentiment analysis of user-generated comments about@ Maggi on Facebook. Corp. Reput. Rev. 22, 48–60. doi: 10.1057/s41299-018-0057-4
Mnif, E., Lacombe, I., and Jarboui, A. (2021). Users’ perception toward Bitcoin green with big data analytics. Soc. Bus. Rev. 16, 592–615. doi: 10.1108/SBR-02-2021-0016
Obrenovic, B., du, J., Godinic, D., Tsoy, D., Khan, M. A. S., and Jakhongirov, I. (2020). Sustaining enterprise operations and productivity during the COVID-19 pandemic: “enterprise effectiveness and sustainability model”. Sustainability 12:5981. doi: 10.3390/su12155981
Ortigosa, A., Martín, J. M., and Carro, R. M. (2014). Sentiment analysis in Facebook and its application to e-learning. Comput. Hum. Behav. 31, 527–541. doi: 10.1016/j.chb.2013.05.024
Park, D., Kim, W. G., and Choi, S. (2019). Application of social media analytics in tourism crisis communication. Curr. Issue Tour. 22, 1810–1824. doi: 10.1080/13683500.2018.1504900
Park, G., Nan, D., Park, E., Kim, K. J., Han, J., and del Pobil, A. P. (2021). “Computers as social actors? Examining how users perceive and interact with virtual influencers on social media.” in Paper presented at the 2021 15th International Conference on Ubiquitous Information Management and Communication (IMCOM) ; January 4–6, 2021 (Seoul, Korea), 1–6.
Park, S., and Park, H. W. (2020). A webometric network analysis of electronic word of mouth (eWOM) characteristics and machine learning approach to consumer comments during a crisis. El Profesional de la Informacion 29:16. doi: 10.3145/epi.2020.sep.16
Paska, I. (2021). Bits and pieces: experiences of social reality in the midst of the Covid-19 pandemic. Media. Res. 10, 2789–2802. doi: 10.46640/imr.10.18.1
Pop, R.-A., Săplăcan, Z., Dabija, D. C., and Alt, M. A. (2021). The impact of social media influencers on travel decisions: the role of trust in consumer decision journey. Curr. Issue Tour. 1–21. doi: 10.1080/13683500.2021.1895729
Puyod, J. V., and Charoensukmongkol, P. (2021). Interacting effect of social media crisis communication and organizational citizenship behavior on employees’ resistance to change during the COVID-19 crisis: evidence from university employees in the Philippines. Asia Pac. Soc. Sci. Rev. 21.
Saif, H., He, Y., Fernandez, M., and Alani, H. (2016). Contextual semantics for sentiment analysis of twitter. Inf. Process. Manag. 52, 5–19. doi: 10.1016/j.ipm.2015.01.005
Scheufele, D. A. (1999). Framing as a theory of media effects. J. Commun. 49, 103–122. doi: 10.1111/j.1460-2466.1999.tb02784.x
Schultz, F., Kleinnijenhuis, J., Oegema, D., Utz, S., and van Atteveldt, W. (2012). Strategic framing in the BP crisis: a semantic network analysis of associative frames. Public Relat. Rev. 38, 97–107. doi: 10.1016/j.pubrev.2011.08.003
Seeger, M. W. (2006). Best practices in crisis communication: an expert panel process. J. Appl. Commun. Res. 34, 232–244. doi: 10.1080/00909880600769944
Sion, G. (2019). Self-portraits in social media: means of communicating emotion through visual content-sharing applications. Linguis. Philosop. Invest. 18, 133–139. doi: 10.22381/LPI1820199
Stieglitz, S., Bruns, A., and Kruger, N. (2015). “Enterprise-related crisis communication on twitter.” in Paper Presented at the Smart Enterprise Engineering: Proceedings of the 12th International Conference on Wirtschaftsinformatik (WI 2015) ; March 4-6, 2015; Universitat Osnabruck, Germany, 133–139.
Stieglitz, S., and Krüger, N. (2011). “Analysis of sentiments in corporate twitter communication – a case study on an issue of Toyota.” in ACIS 2011 Proceedings. Vol. 29.
Stieglitz, S., Mirbabaie, M., and Potthoff, T. (2018). “Crisis communication on twitter during a global crisis of Volkswagen-The case of “Dieselgate”.” in Paper Presented at the Proceedings of the 51st Hawaii International Conference on System Sciences.
Su, Z., McDonnell, D., Wen, J., Kozak, M., Abbas, J., Šegalo, S., et al. (2021). Mental health consequences of COVID-19 media coverage: the need for effective crisis communication practices. Glob. Health 17, 1–8. doi: 10.1186/s12992-020-00654-4
Tang, L., Bie, B., and Zhi, D. (2018). Tweeting about measles during stages of an outbreak: a semantic network approach to the framing of an emerging infectious disease. Am. J. Infect. Control 46, 1375–1380. doi: 10.1016/j.ajic.2018.05.019
Taylor, P., Kral, P., Vrbka, J., and Gregova, E. (2020). Problematic smartphone use, social anxiety symptom severity, and technology-related behaviors and attitudes. Analysis Metaphysics 19, 73–79. doi: 10.22381/AM1920208
Tian, X., He, W., and Wang, F. K. (2021). Applying sentiment analytics to examine social media crises: a case study of united Airline’s crisis in 2017. Data Technol. Appl. doi: 10.1108/DTA-09-2018-0087 [Epub ahead of print]
Triantafillidou, A., and Yannas, P. (2020). Social media crisis communication in racially charged crises: exploring the effects of social media and image restoration strategies. Comput. Hum. Behav. 106:106269. doi: 10.1016/j.chb.2020.106269
Tseng, C.-W., Chou, J. J., and Tsai, Y. C. (2018). Text mining analysis of teaching evaluation questionnaires for the selection of outstanding teaching faculty members. IEEE Access 6, 72870–72879. doi: 10.1109/ACCESS.2018.2878478
Utz, S., Schultz, F., and Glocka, S. (2013). Crisis communication online: how medium, crisis type and emotions affected public reactions in the Fukushima Daiichi nuclear disaster. Public Relat. Rev. 39, 40–46. doi: 10.1016/j.pubrev.2012.09.010
Wang, L., Schuetz, C. G., and Cai, D. (2021). Choosing response strategies in social media crisis communication: an evolutionary game theory perspective. Inf. Manag. 58:103371. doi: 10.1016/j.im.2020.103371
Xue, B., Fu, C., and Shaobin, Z. (2014). “A study on sentiment computing and classification of sina weibo with word2vec.” Paper presented at the 2014 IEEE International Congress on Big Data ; July 2, 2014 (Anchorage, AK, USA), 358–363.
Keywords: mobile game, crisis communication, semantic network analysis, sentiment analysis, Sina Weibo
Citation: Che S, Nan D, Kamphuis P, Zhang S and Kim JH (2022) Examining Crisis Communication Using Semantic Network and Sentiment Analysis: A Case Study on NetEase Games. Front. Psychol. 13:823415. doi: 10.3389/fpsyg.2022.823415
Edited by:
Kostas Karpouzis, Panteion University, GreeceCopyright © 2022 Che, Nan, Kamphuis, Zhang and Kim. This is an open-access article distributed under the terms of the Creative Commons Attribution License (CC BY). The use, distribution or reproduction in other forums is permitted, provided the original author(s) and the copyright owner(s) are credited and that the original publication in this journal is cited, in accordance with accepted academic practice. No use, distribution or reproduction is permitted which does not comply with these terms.
*Correspondence: Jang Hyun Kim, YWxvaGFraW1Ac2trdS5lZHU=