- The Auditory Cognition Lab, Department of Communication Sciences and Disorders, University of Haifa, Haifa, Israel
Older adults with age-related hearing loss exhibit substantial individual differences in speech perception in adverse listening conditions. We propose that the ability to rapidly adapt to changes in the auditory environment (i.e., perceptual learning) is among the processes contributing to these individual differences, in addition to the cognitive and sensory processes that were explored in the past. Seventy older adults with age-related hearing loss participated in this study. We assessed the relative contribution of hearing acuity, cognitive factors (working memory, vocabulary, and selective attention), rapid perceptual learning of time-compressed speech, and hearing aid use to the perception of speech presented at a natural fast rate (fast speech), speech embedded in babble noise (speech in noise), and competing speech (dichotic listening). Speech perception was modeled as a function of the other variables. For fast speech, age [odds ratio (OR) = 0.79], hearing acuity (OR = 0.62), pre-learning (baseline) perception of time-compressed speech (OR = 1.47), and rapid perceptual learning (OR = 1.36) were all significant predictors. For speech in noise, only hearing and pre-learning perception of time-compressed speech were significant predictors (OR = 0.51 and OR = 1.53, respectively). Consistent with previous findings, the severity of hearing loss and auditory processing (as captured by pre-learning perception of time-compressed speech) was strong contributors to individual differences in fast speech and speech in noise perception. Furthermore, older adults with good rapid perceptual learning can use this capacity to partially offset the effects of age and hearing loss on the perception of speech presented at fast conversational rates. Our results highlight the potential contribution of dynamic processes to speech perception.
Introduction
Aging is often accompanied by sensorineural hearing loss (presbycusis) and poor speech perception in daily listening environments (Committee on Hearing, Bioacoustics and Biomechanics (CHABA), 1988; Humes, 1996; Morrell et al., 1996; Pichora-Fuller, 1997; Gordon-Salant and Fitzgibbons, 2001; Dubno et al., 2008), especially under adverse listening conditions (e.g., in the presence of fast speech or competing noise; Pichora-Fuller and Singh, 2006; Schneider et al., 2010). There is tremendous variability in degraded speech perception among older adults. This variability is associated with sensory and cognitive factors (Committee on Hearing, Bioacoustics and Biomechanics (CHABA), 1988; Souza, 2016), as well as with individual differences in perceptual learning for speech (Karawani et al., 2017; Manheim et al., 2018; Rotman et al., 2020b). Hearing aids are the most common rehabilitation for speech perception difficulties in older adults with age-related hearing loss (Souza, 2016). However, like their non-hearing aid using peers, older adults who use hearing aids also vary widely on measures of speech perception. We hypothesize that the same factors that account for individual differences in degraded speech processing in adults with presbycusis are likely responsible for some of the variability in speech perception performance observed among hearing aid users. Therefore, the overall aim of the current study is to assess the relative contribution of sensory (i.e., hearing acuity) and cognitive factors (working memory, vocabulary, and selective attention), rapid perceptual learning, and the use of hearing aids to the identification of different types of degraded speech among older adults. We used three speech tasks—fast speech, speech in babble noise, and competing speech—which represent different challenges that can be encountered in daily listening situations, and which are known to pose difficulties for older adults with hearing loss (for review see Humes et al., 2012). The effects of cognitive factors, learning, and hearing aids might differ across these different conditions. Whereas the challenges associated with fast speech result from source degradation (speaking rapidly changes the temporal and spectral characteristics of speech, Koreman, 2006), the challenges associated with speech in babble noise and competing speech are associated with the listening environment (transmission degradation according to the terminology proposed by Mattys et al., 2012).
Speech Perception in Age-related Hearing Loss
Age-related hearing loss is a primary contributor to speech perception difficulties in older adults (e.g., Humes and Roberts, 1990; Jerger et al., 1991; Humes, 2002). Individuals with age-related sensorineural hearing loss often require favorable signal to noise ratios to recognize speech due to elevated hearing thresholds (Killion, 1997). Reduced audibility (e.g., Gates and Mills, 2005), impaired temporal synchrony (e.g., Hopkins and Moore, 2007, 2011), and broadening of auditory filters (e.g., Peters and Moore, 1992; Leek and Summers, 1993) have also been suggested to account for the connection between age-related hearing loss and reduced perception of speech in noisy environments. Overall, it is estimated that these sensory factors account for 50–90% of individual differences in speech perception (for review, see Humes and Dubno, 2010).
When listening to connected speech (i.e., utterances longer than one word such as sentences or longer units of speech) older adults with age-related hearing loss often have perceptual difficulties with rapid speech rates (e.g., Tun, 1998; Gordon-Salant and Fitzgibbons, 2001; Wingfeld et al., 2006), in the presence of background noise (e.g., Pichora-Fuller et al., 1995; Helfer and Freyman, 2008) or in the presence of competing speech in dichotic listening situations (e.g., Jerger et al., 1994, 1995; Roup et al., 2006). However, auditory factors might be insufficient to explain individual differences in these situations because listeners with identical audiograms can have vastly different speech perception abilities (Luterman et al., 1966; Phillips et al., 2000; Schneider and Pichora-Fuller, 2001; Pichora-Fuller and Souza, 2003). Even when matched for audiological factors, older adults often find it more difficult than their young counterparts to perceive and comprehend speech in adverse listening situations (Gordon-Salant and Fitzgibbons, 1993; Needleman and Crandell, 1995).
The contribution of audiometric thresholds to speech perception tends to be larger in relatively easy conditions (e.g., identifying words in a quiet background) than in more challenging ones (e.g., with temporally distorted speech and speech in noise; Gordon-Salant and Fitzgibbons, 1993, 2001; Humes, 2007). Furthermore, whereas audiometric factors typically allow reasonably accurate predictions of speech in quiet, using auditory thresholds often leads to over estimation of performance of speech in noise (Dubno et al., 1984; Schum et al., 1991; Hargus and Gordon-Salant, 1995). Thus, once a task becomes more demanding, additional factors are needed to explain performance, as explained below.
Cognitive Abilities and Speech Perception in Older Adults
Current models of speech recognition like the Ease of Language Understanding (ELU) model (Rönnberg et al., 2013) highlight the significance of cognitive factors for the processing of speech in ecological listening. The ELU model suggests that when the speech signal is degraded, for example, by competing noise or due to hearing loss, the automatic encoding of incoming speech may fail to match long-held representations within an individual’s mental lexicon. When such failure occurs, explicit processing of the signal becomes necessary to achieve speech understanding. This is done by utilization of previous experience and context, as well as recruitment of linguistic knowledge and more domain-general cognitive resources (e.g., working memory and attention) to support listening (Pichora-Fuller et al., 1995; Akeroyd, 2008). By this account, individual differences in cognitive or linguistic functions are expected to contribute to individual differences in the explicit processes required for recognition under adverse conditions.
Consistent with the ELU model, studies suggest that individual differences in cognition are associated with individual differences in the processing of speech under adverse listening conditions (e.g., Salthouse, 1994, 1996). For example, cognitive speed of processing contributes to the perception of both time-compressed speech (a form of rapid speech; Wingfield et al., 1985; Wingfield, 1996; Dias et al., 2019) and speech in noise (Tun and Wingfield, 1999). Working memory and attention (specifically, divided attention and selective listening) are also associated with perception of time-compressed speech (Tun et al., 1992; Vaughan et al., 2006) and speech in noise (Pichora-Fuller et al., 1995; Tun and Wingfield, 1999; Schneider et al., 2002, 2007; Tun et al., 2002). For dichotic speech, declines in attention are also related to performance declines (McDowd and Shaw, 2000; Rogers, 2000). Linguistic context can also positively contribute to speech perception (for review, see Burke and Shafto, 2008). Larger vocabulary in older adults and improved ability to utilize contextual cues facilitate speech perception in adverse listening conditions (Pichora-Fuller et al., 1995; Verhaeghen, 2003; Sheldon et al., 2008; Ben-David et al., 2015; Signoret and Ruder, 2019).
However, it is probably the combination of sensory and cognitive factors that affect speech perception of older adults (e.g., Cherry, 1953; Humes et al., 2006; Bronkhorst, 2015). If listeners possess a finite amount of information-processing resources (Kahneman, 1973), and if hearing-impaired older adults have to divert some of them to the normally automatic process of auditory encoding, then fewer resources will be available for subsequent higher-level processing (Rabbitt, 1990; Pichora-Fuller, 2003a). In addition, the interplay between sensory and cognitive factors can change in different listening conditions, but studies on the contribution of cognition to individual differences in speech perception in older adults often focused on a single task, making it hard to determine if either the contribution of cognition or the cognitive/sensory interplay changes across speech tasks. Whether the use of hearing aids changes, this interplay is also unknown.
Rapid Perceptual Learning Accounts for Variance in Speech Perception in Older Adults
Rapid perceptual learning also relates to the variability in perception of speech under challenging conditions (Peelle and Wingfield, 2005; Golomb et al., 2007; Manheim et al., 2018; Banai and Lavie, 2020; Rotman et al., 2020b). Rapid perceptual learning, defined as the ability to rapidly adapt to changes in one’s environment, occurs under many adverse or sub-optimal conditions (Samuel and Kraljic, 2009). Perceptual learning is observed in old age, but it appears to be slower or reduced (Schneider and Pichora-Fuller, 2001; Forstmann et al., 2011; Lu et al., 2011) and more specific (Peelle and Wingfield, 2005) than in young adults (for a recent review, see Bieber and Gordon-Salant, 2021). Age-related hearing loss might have a further negative effect on learning. For example, older adults with preserved hearing exhibit poorer rapid learning of time-compressed speech compared to young adults, but better rapid learning than older adults with age-related hearing loss (Manheim et al., 2018). Relevant to the current study, across a range of speech tasks, rapid learning was documented in older adults with different levels of hearing (Peelle and Wingfield, 2005; Karawani et al., 2017; Manheim et al., 2018).
Perceptual learning and speech perception are related in the sense that learning contributes to future perception (Ahissar et al., 2009; Samuel and Kraljic, 2009; Banai and Lavie, 2021). However, recent studies suggest that the links could go beyond what could be expected from associations across different speech tasks (Banai and Lavie, 2021). Recent studies on perceptual learning (with both visual and speech materials) suggest that a general learning factor across learning tasks could serve as an individual capacity that supports performance across a range of scenarios (Yang et al., 2020; Dale et al., 2021; Heffner and Myers, 2021). Consistent with this view, we observed that individual differences in rapid perceptual learning of one type of speech (e.g., time-compressed speech) are consistently related to individual differences in speech perception under different adverse conditions (speech in noise and fast speech; Karawani et al., 2017;Manheim et al., 2018; Rotman et al., 2020b). Speech perception and rapid learning have also been found to be associated even when learning is assessed under conditions designed to offset the effects of age and hearing loss on speech perception (Manheim et al., 2018; Rotman et al., 2020b). We hypothesize that individuals who retain good rapid perceptual learning despite aging and hearing loss, can offset some of their negative impacts through rapid online learning (Banai and Lavie, 2021). To further explore this hypothesis, we now focus on the unique contribution of rapid perceptual learning to other challenging listening conditions, after accounting for sensory and cognitive factors and for the use of hearing aids.
Hearing Aid Use
For older adults with hearing loss, hearing aids are the most widely used rehabilitation devices. While hearing aids are unlikely to fully compensate for the auditory processing deficits of individuals with hearing impairment, they amplify sounds to improve audibility (Souza, 2016) and incorporate multiple algorithms intended to improve communication in adverse listening conditions (Neher et al., 2014). However, the perceptual results of using hearing aids depend not only on hearing aid technology but also on the factors described above. Moreover, long-term acclimatization induced benefits may further improve speech perception in some individuals. The effects reported in the literature include improved speech perception in noise, reduced distractibility to background noise, and reduced listening effort (Gatehouse, 1992; Munro and Lutman, 2003; Lavie et al., 2015; Habicht et al., 2016; Dawes and Munro, 2017; Lavie et al., 2021). However, other studies have failed to demonstrate improved identification of degraded speech (speech in noise, Dawes et al., 2013, 2014a,b; fast speech, Rotman et al., 2020a) in new or experienced hearing aid users. Thus, even though there are some indications for perceptual gains after months or years of hearing aid use, the effects of hearing aids on higher-level language processes in complex listening conditions are not well understood.
Unsurprisingly, most studies seeking to explain individual differences in aided speech perception have identified differences in hearing thresholds as the main source of variance (e.g., Tun and Wingfield, 1999; Humes, 2007). However, after controlling for the effects of audibility and age, working memory span score was correlated with both aided and unaided perception of speech in noise (Lunner, 2003), and the benefit from hearing aid algorithms (i.e., fast acting compression) was positively associated with cognitive skill (Lunner, 2003; Gatehouse et al., 2006; Cox and Xu, 2010; Souza et al., 2015).
Research Questions and Hypotheses
According to the literature review above, the interplay between the perception of different types of degraded speech, multiple cognitive factors, and perceptual learning is not sufficiently understood. It is also unclear whether the use of hearing aids changes the interplay among the different factors or results in plastic changes in speech perception (see Lavie et al., 2021 for a systematic review). The present study was designed to address these issues by investigating the contribution of hearing, cognition, rapid perceptual learning, and the contribution of long-term hearing aid use to three indices of speech perception: fast speech, speech in babble noise, and dichotic speech.
If, as explained above, perceptual learning is a capacity that can support other processes, such as “online” speech perception, rapid perceptual learning should explain unique variance in the perception of different types of distorted speech in addition to the known contributions of other sensory and cognitive factors. To this end, we use rapid learning of time-compressed speech as an index of learning for two reasons. First, the work reviewed above suggests that with this task, rapid learning rates are maintained even in older adults with hearing loss. Second, most listeners have no experience with this form of accelerated speech, and initial performance can be quite poor, making it easy to observe learning. Additionally, we hypothesize that the same factors that account for individual differences in degraded speech processing in adults with presbycusis also play a role when it comes to the effects of hearing aids, but the current literature (see Kalluri et al., 2019; Lavie et al., 2021 for recent reviews) makes it hard to draw more specific hypotheses, and therefore, in this regard, this is an exploratory study.
Materials and Methods
Participants
A total of 95 potential participants were recruited via hearing clinics, retirement communities, and community centers. Potential participants were screened based on the following inclusion criteria: (1) age 65 and above; (2) bilateral, adult-onset, symmetric, sensory hearing loss of 30–70 dB, with flat or moderately sloping audiograms, and suprathreshold word recognition scores of ≥60% and air-bone gaps ≤15 dB; (3) no known neurological or psychiatric diagnoses; (4) normal or corrected-to-normal vision; (5) high proficiency in Hebrew; (6) normal cognitive status [a score of 24 or higher on the Mini-Mental State Examination (MMSE; Folstein et al., 1975)]; and (7) hearing aid use: we targeted only non-users (no prior experience with hearing aids and no plans to acquire hearing aids during the period of the study) and experienced hearing aid users [at least 6 months of bilateral hearing aid use; hearing aids were digital, with at least 16 amplification channels, at least four compression channels, noise reduction and anti-feedback algorithms, and wireless (ear to ear) processing]. Participants received modest monetary compensation for their participation and signed written informed consent forms. All aspects of the study were approved by the ethics committee of the Faculty of Social Welfare and Health Sciences at the University of Haifa (permit 362/18).
Twenty-two recruits failed to meet inclusion criteria and were excluded from the study: 12 for having insufficient hearing loss, five for having more severe hearing loss or low suprathreshold word recognition scores, two for asymmetric hearing loss, two for having insufficient experience with hearing aids [in the experienced hearing aid group (see below)], and one for reporting additional motor issues that could have influenced their responses on some of the tasks (e.g., block design and flanker). Three additional participants completed the first experimental session only (see experimental design below), and their data were thus excluded from all analyses.
The final study sample included 70 participants (23 males and 47 females) who met all inclusion criteria: 35 older adults with hearing loss (OHI) who did not use hearing aids and 35 older hearing-impaired adults who were experienced hearing aid users (OHI-HA). The two groups had similar ages, MMSE and cognitive scores, but hearing aid users had poorer hearing, somewhat poorer suprathreshold word recognition scores and somewhat higher education (see Figure 1; Table 1). Hearing thresholds were considered in our statistical modeling; the differences in word recognition (corresponding to 1–2 words difference) and education were considered negligible. Based on a power analysis on the data of our previous study (Rotman et al., 2020b), no effect for hearing aid use was expected even if we increased our sample size to 400 (200 in each group) participants, which was unrealistic. In contrast, a sample of 40 participants (20 in each group) was deemed sufficient to replicate the perceptual, learning, and cognitive effects reported by Rotman et al. (2020a) with a statistical power of 0.8. Power calculation was performed using the simr package (Green et al., 2016) in R.
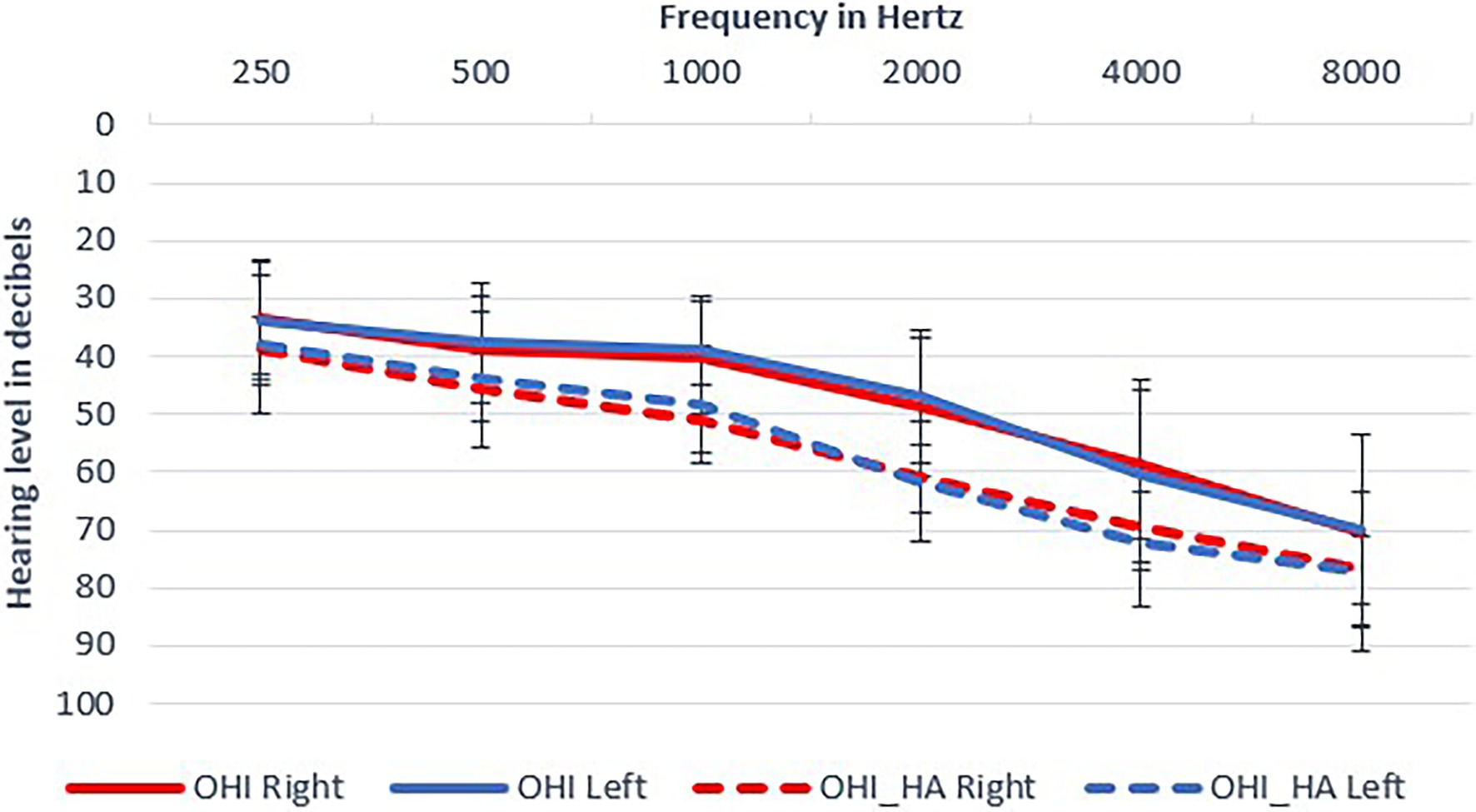
Figure 1. Mean audiograms of participants. Mean thresholds and standard deviations are shown: older hearing-impaired adults (OHI) in full lines; older hearing-impaired adults who use hearing aids (OHI-HA) in dashed lines.
Procedure
Testing was comprised of two sessions conducted 7–14 days apart at a hearing clinic or at the participants’ home, based on each participant’s preference (see Figure 2). Except for the audiometric assessments in the clinic (see below), all other testing was conducted in a quiet room in the clinic or in the participants’ homes. In session I, potential participants were screened based on the inclusion criteria. Participants who met the inclusion criteria underwent assessments of rapid perceptual learning of time-compressed speech, perception of fast speech, and speech in noise and dichotic word identification. Session II included cognitive assessments and another assessment of time-compressed speech learning, data from which are not reported here. All testing was conducted by two clinical audiologists experienced in working with hearing-impaired patients and therefore accustomed to speaking loudly and clearly.
Task
Screening Assessments
Demographic Questionnaire
A questionnaire regarding education, handedness, lifestyle, and general health was used in the current study. The participants completed the questionnaire before completing further assessments.
Cognitive and Hebrew Screening
Participants were screened with a the MMSE (Folstein et al., 1975), with a cutoff score of 24 as an inclusion criterion. Proficiency in Hebrew was evaluated using a short screening with a series of questions and commands in Hebrew. To participate in the study, one had to complete this screening with a perfect score (see Lavie, 2011).
Audiological Assessments
A full pure-tone and speech audiometry (suprathreshold word recognition) was conducted in an acoustic booth, using the MAICO audiometer (model MA42) or at the participants’ home with Inventis Cello and Piccolo portable audiometers and Silenta Supermax supra-aural headphones. Most comfortable levels (MCL) for speech were also assessed. The audiograms were classified based on Duthey (2013), with four frequencies pure-tone average (0.5, 1, 2, and 4 KHz) ≥ 30 dB as a criterion of hearing loss. Participants with up-to-date (≤6 months) audiograms were not evaluated again.
Speech Perception and Learning
Stimuli
Stimuli were 80 simple sentences in Hebrew, five to six words long, with a common subject-verb-object grammatical structure (adapted from Prior and Bentin, 2006). All sentences were recorded in a sound attenuating booth using a built-in MacBook Air microphone, sampled at 44 KHz and saved in WAV format. The root-mean-square levels of the recorded sentences were normalized using Audacity audio software version 2.2.0. Sentences were recorded by four native Hebrew speakers (three females and one male). Speaker 1 (female) recorded 10 different sentences at her natural fast rate (M = 183 words/min, SD = 17); speaker 2 (female) recorded 10 sentences at her natural fast rate (M = 210 words/min, SD = 21) and 10 sentences at her normal, unhurried rate (M = 111 words/min, SD = 27); speaker 3 (male) recorded 10 sentences at a normal rate of 88 words/min (SD = 10.30); and speaker 4 (female) recorded 40 sentences at a normal rate of 102 words/min (SD = 12.68). To minimize the effects of sentence familiarity on performance, there was no sentence repetition within or across conditions. In addition, Speaker 4 also recorded a list containing 25 pairs of monosyllabic words, adapted from the Hebrew PB-50 test (Lavie et al., 2015) for the dichotic word identification task (see below).
Presentation and Scoring
Speech materials were presented through Meze 99 classics headphones to both ears as follows: (1) unaided to the OHI group and (2) aided for the OHI-HA group (i.e., headphones were placed while participants wore their hearing aids). Stimuli were presented at each listener’s preferred level. To determine this level, a pre-recorded short passage was played and listeners determined their preferred listening level. Because some of the participants were tested at home, and others in several rooms in the clinic, achieving constant acoustic settings for sound field presentation of the speech stimuli was impossible. Thus, we decided to test all participants with headphones and play the stimuli from the computers (in line with Rotman et al., 2020b). In the OHI-HA group, the testers verified that the hearing aids were working properly at the beginning of each session. After listening to each test stimulus (sentences or dichotic word pairs), participants were asked to repeat what they had heard, and the experimenter transcribed their replies. Each stimulus was presented only once, and no feedback was provided. Performance was scored off-line. For the rapid learning, fast speech and speech in noise tasks, all words, including function words, were counted for scoring. Scoring of the dichotic listening task is described below. Unless otherwise noted, the proportion of correctly recognized words/sentence was computed and used for statistical modeling, although for visualization proportion was averaged across sentences.
Rapid Perceptual Learning (Session I)
Ten sentences (recorded by Speaker 2) were presented as time-compressed speech. Time-compressed speech was chosen because learning with this form of speech was previously documented in older adults within and across sessions (e.g., Peelle and Wingfield, 2005; Golomb et al., 2007; Manheim et al., 2018; Rotman et al., 2020b). In addition, most older listeners have no experience with this type of artificially accelerated speech, making it useful in studying the correlations between learning and the recognition of other forms of degraded speech (e.g., naturally fast speech and speech in noise). Following earlier work (Rotman et al., 2020b), sentences were compressed to 45–50% of their original length (45% for participants with PTA of 26–47 dB and 50% for PTA ≥ 48 dB) in Matlab, using a pitch preserving algorithm (WSOLA, Verhelst and Roelands, 1993). Speech rates were adjusted based on hearing threshold to minimize the effects of hearing on the estimate of rapid learning.
Baseline recognition of time-compressed speech was defined as the proportion of correctly identified words in the two first sentences. Learning of time-compressed speech was defined as the rate of improvement in recognition over time. It was quantified as the linear slopes of the learning curves over an additional eight time-compressed speech sentences (for further details see Rotman et al., 2020b).
Speech Perception
Speech perception was evaluated using the following tasks:
Fast Speech
Twenty sentences (10 sentences recorded by Speaker 1 and 10 sentences recorded by Speaker 2).
Speech Recognition in Noise
Twenty sentences were presented (10 sentences recorded by Speaker 3 and 10 sentences recorded by Speaker 4). All sentences were embedded in a 4-talker babble noise with a fixed SNR level of +3 dB.
Dichotic Word Identification
Following previous research (Lavie et al., 2013, 2015), we used a list of 25 pairs of monosyllabic words, adapted from the Hebrew PB-50 test. One word of each pair was presented to the right ear while the other word was presented simultaneously to the left ear, and participants were required to repeat both words in whichever order they chose. For statistical analysis, the number of correctly repeated words in each ear was counted and two indices of dichotic listening were calculated as: the sum (= dominant ear score + non-dominant ear score) and the difference between the ears (= dominant ear - non-dominant ear).
Cognitive Assessments
A battery of cognitive assessments was used to evaluate cognitive status and identify characteristics that might influence participants’ performance on the experimental tasks. This battery was administered at a comfortable auditory level that was defined by each participant to negate potential confounding effects of audibility on performance. The following subtests from the Wechsler Adult Intelligence Scale-Third Edition in Hebrew (WAIS-III; Wechsler, 1997) were used as: vocabulary (semantic knowledge), digit span (working memory), and block design (non-verbal reasoning).
All subtests were administrated and scored according to the test manual. Raw scores were converted to standardized scores.
Attention
Two tests were used as: (1) Flanker test (Eriksen and Eriksen, 1974). A computerized version of the well-validated Flanker test was used as a measure of inhibition and selective attention. The target stimulus was an arrow-head heading right or left, embedded in the middle of a row of five arrow-heads or other stimuli. Participants were asked to note the direction of a central arrow, which was flanked by arrows pointing in the same direction (congruent trials) or the opposite direction (incongruent trials) or non-arrow stimuli (neutral trials). Reaction time and accuracy were measured. The “flanker cost” for each participant was used for statistical analyses. The cost was calculated as the mean logRT.
(RT = reaction time in ms) of the correct responses in the incongruent trials divided by the mean log RT of the correct responses in the neutral trials. A higher flanker cost (>1) means poorer selective attention. (2) Trail making test (Reitan, 1958). Attention switching control was tested in two test conditions: in condition A, participants were asked to draw lines to connect circled numbers in a numerical sequence (i.e., 1-2-3) as rapidly as possible. In condition B, participants were asked to draw lines to connect circled numbers and letters in an alternating numeric and alphabetic sequence (i.e., 1-A-2-B) as rapidly as possible. Response speed was measured by a stopwatch.
Results
Descriptive Statistics
As shown in Table 2, hearing aid users had somewhat higher vocabulary scores than the non-hearing aid group, and this was considered in the statistical analyses reported below. In both groups, there was large between-participant variance across all speech and learning tasks (the raw data and analysis code can be found at https://osf.io/sreq4).
As shown in Table 3, rapid perceptual learning of time-compressed speech was positively correlated with identification of fast speech and speech in noise, and negatively correlated with hearing thresholds. In addition, and as expected from the literature, speech perception was correlated with specific cognitive indices. Rapid learning of time-compressed speech also correlated with some of the cognitive measures.
Modeling Speech Perception As a Function of Age, Hearing, Cognition, Rapid Perceptual Learning, and Hearing Aid Use
The contribution of hearing and cognition to recognition accuracy in the speech tasks was studied in the past. Therefore, our modeling here focused on the unique additional contributions of perceptual learning and hearing aid use. To this end, modeling was performed in stages: hearing and cognition were modeled first, followed by learning, and then hearing aid use. With this approach, if a later model fits the data significantly better than a previous one (with a model comparison), the predictor entered at the later stage has a unique contribution to speech recognition when all other included variables are considered. Within a given model, the coefficient of each predictor reflects its contribution while all other predictors in the model are kept constant. Since there were repeated measures for the fast speech and speech in noise, a series of generalized linear mixed models was run using the lme4 package in R (Bates et al., 2014). Single trial fast speech and speech in noise scores served as the dependent variables, and age, hearing, cognition, rapid perceptual learning, and hearing aid use served as the independent variables (i.e., the predictors). Given the number of predictors relative to sample size, and to avoid overloading the models, block design and trail making were excluded from the analysis; likewise, interactions were not modeled. The random effects structure consisted of random intercepts for both participant and sentence; predictors were standardized (z-scored) prior to modeling. Following earlier work, and due to dealing with proportion scores, binomial regressions with a logit link function (logistic regressions) were used (Rotman et al., 2020b).
Five models were constructed for fast speech and for speech in noise, starting with a model that included only the random effects (Model 0). Thereafter, each subsequent model added one additional predictor over the previous model(s), with the models building upon one another sequentially (e.g., model 1 = Model 0 + variable 1; Model 2 = Model 1 + variable 2; and Model 3 = Model 2 + variable 3). Model 1 included background variables of the participants as predictors, which included as: age, hearing, vocabulary, working memory, and attention. Model 2 included baseline recognition of time-compressed speech; Model 3 added the rapid perceptual learning slope; and Model 4 added hearing aid use (rated on a nominal scale—yes/no). To isolate the unique contribution of each additional variable, these four increasingly complex models were compared using likelihood ratio tests with the R ANOVA function.
Note that in general, correlations between the different predictors were not high (the highest Pearson correlations were r = 0.43 between vocabulary and working memory, r = −0.38 between hearing and learning, and r = 0.35 between learning and baseline recognition of TCS), suggesting that multicollinearity is not a serious concern. Likewise, all Variance Inflation Factors (VIF) were low (< 2), as reported below for the best fitting models.
Recognition of Fast Speech
The inclusion of the background variables in the model resulted in a better fit to the data than the model that included the random effects only. However, the addition of baseline recognition of time-compressed speech and rapid learning both improved the fits significantly, suggesting that rapid learning had a significantly unique contribution to the recognition of fast speech, beyond that of other variables. Hearing aids had no additional effect (see Table 4).
In the best fitting model (model 3), age, hearing, baseline recognition of time-compressed speech, and learning were all significant predictors of fast speech recognition (see Table 5 which also includes model 1 with only background variables). Hearing was the strongest negative predictor (largest beta in absolute value, see Table 5) of fast speech recognition followed by age, indicating that fast speech recognition was poorer in individuals with more severe hearing loss and in older individuals. Baseline recognition of time-compressed speech and rapid learning were both positive predictors, suggesting that for a given age/hearing loss, listeners who maintained better perception and learning of time-compressed speech also maintained more accurate recognition of fast speech, regardless of hearing aid use (see Figure 3). Variance Inflation Factors for the best fitting model were 1.27 for age, 1.91 for hearing, 1.41 for vocabulary, 1.55 for working memory, 1.06 for attention, 1.31 for baseline recognition of TCS, and 1.46 for learning.
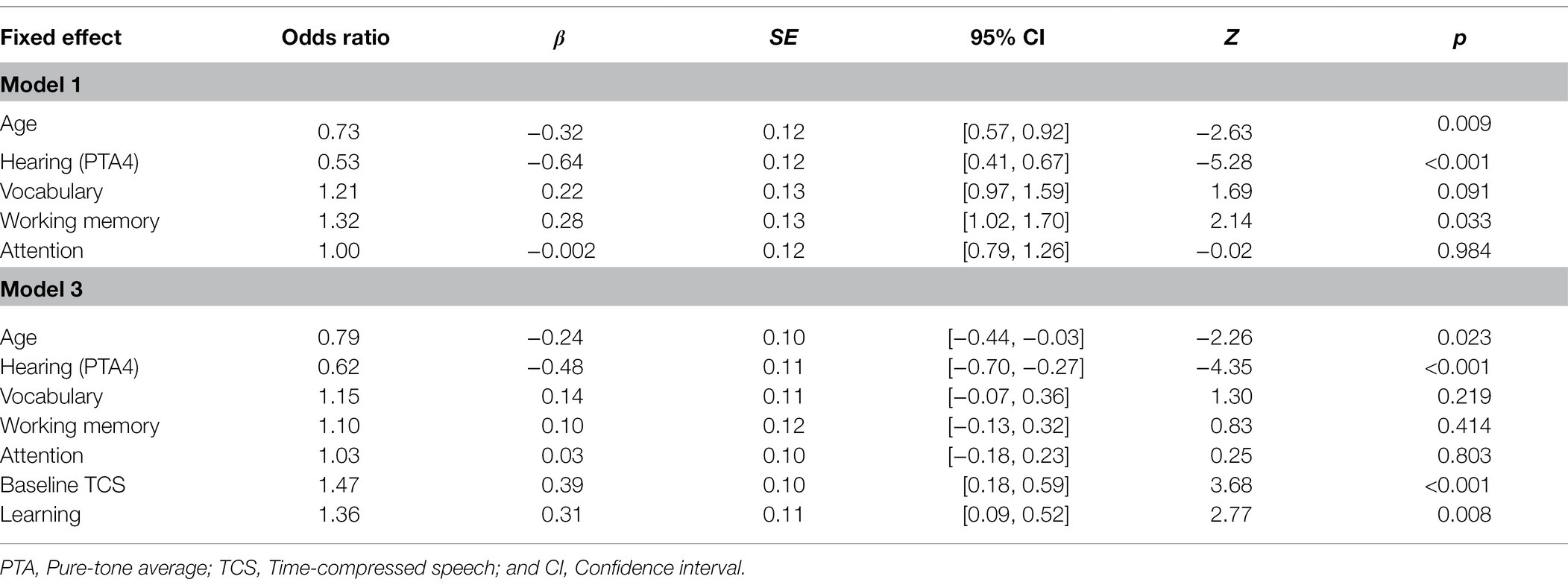
Table 5. Results of generalized linear mixed-model for fast speech recognition as a function of the background variables (Model 1) and as a function of age, hearing, cognition, baseline recognition of time-compressed speech, and rapid perceptual learning as fixed effects (Model 3).
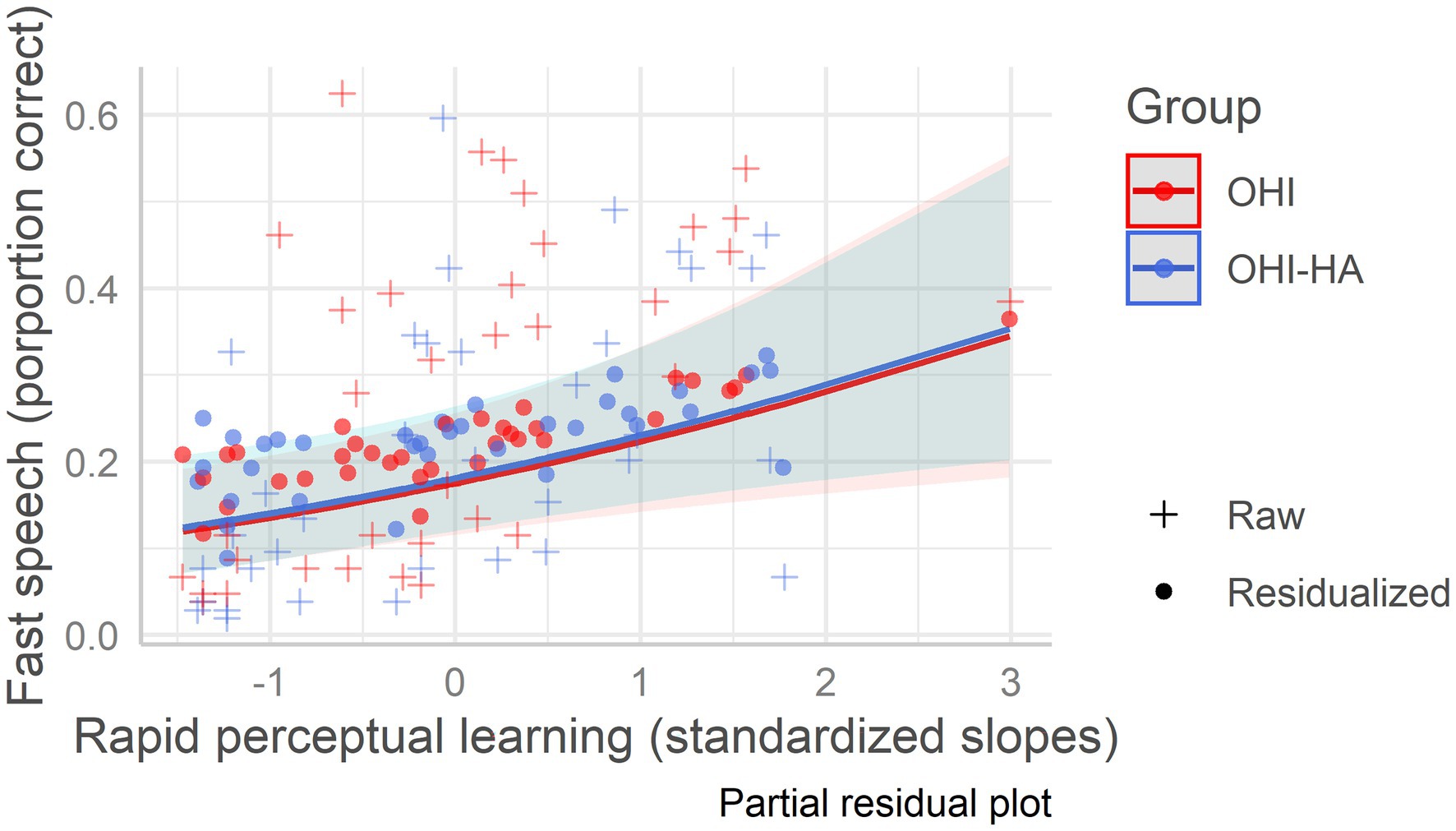
Figure 3. Fast speech recognition as a function of rapid learning among older hearing-impaired adults and older hearing-impaired adults who use hearing aids. Older hearing-impaired adults (OHI) in red; older hearing-impaired adults who use hearing aids (OHI-HA) in blue. The y-axis indicates the correct perception percentage of fast speech, and the x-axis indicates the standardized rapid perceptual learning slope. The dots (residualized aggregate scores) mark the predicted scores; their deviation from the regression line indicates prediction error, while the pluses mark the raw/true scores. The shaded areas are the confidence intervals.
Recognition of Speech in Noise
The inclusion of the background variables in the model resulted in a better fit to the data than the model that included the random effects only (Table 6). However, baseline recognition of time-compressed speech improved the fits significantly (see Table 6), suggesting that time-compressed speech perception had a significant unique contribution to the recognition of speech in noise, beyond that of other variables. Hearing aids had no additional effect.
In the best fitting model (see Table 7 which also includes model 1 with only background variables), hearing was the strongest predictor (i.e., largest beta in absolute value) of speech in noise recognition, followed by baseline recognition of time-compressed speech. Neither rapid learning nor hearing aid use further improved the fit (see Figure 4). Thus, lower hearing thresholds and more accurate time-compressed speech recognition were associated with better recognition of speech in noise. Variance Inflation Factors for the best fitting model were 1.29 for age, 1.97 for hearing, 1.45 for vocabulary, 1.55 for working memory, 1.06 for attention, 1.33 for baseline recognition of TCS, and 1.53 for learning.
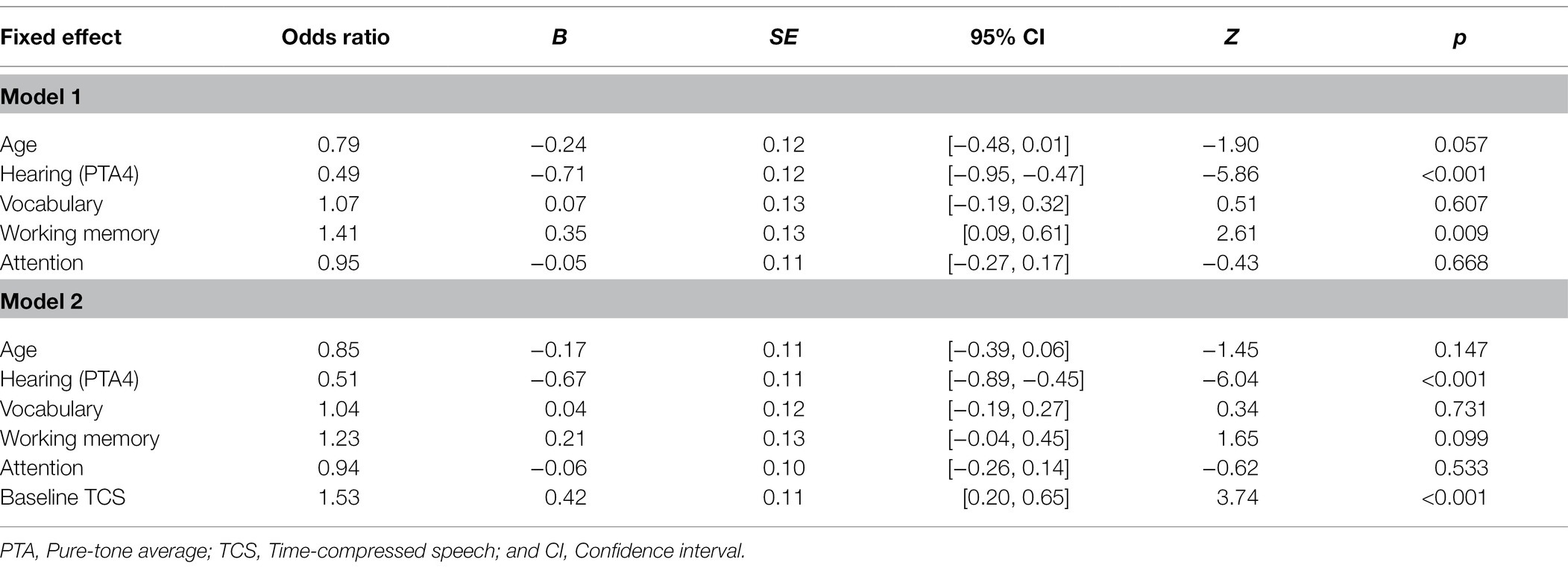
Table 7. Results of generalizedlinear mixed-effects model for speech in noise recognition as a function of the background variables (Model 1) and as a function of age, hearing, cognition, and baseline recognition of time-compressed speech as fixed effects (Model 2).
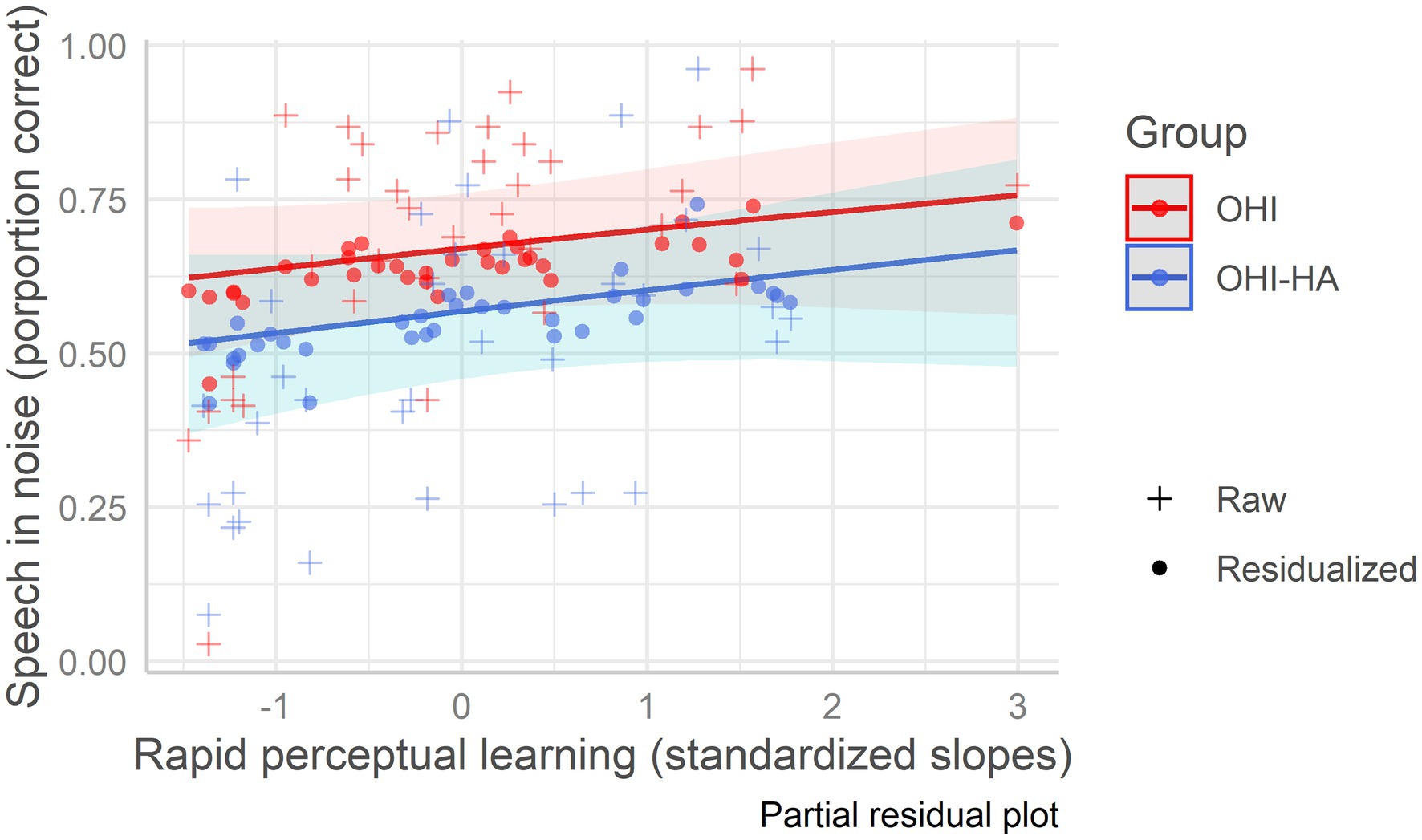
Figure 4. Speech in noise recognition as a function of rapid learning among older hearing-impaired adults and older hearing-impaired adults who use hearing aids. Older hearing-impaired adults (OHI) in red; older hearing-impaired adults who use hearing aids (OHI-HA) in blue. The y-axis indicates the correct perception percentage of speech in noise, and the x-axis indicates the standardized rapid perceptual learning slope. The dots (residualized aggregate scores) mark the predicted scores; their deviation from the regression line indicates prediction error, while the pluses mark the raw/true scores. The shaded areas are the confidence intervals.
Dichotic Word Identification
Since there were no repeated measures (i.e., there was only one score for each participant), linear regression analyses were used. Four models were constructed for the dichotic listening task in two different ways: once with the dichotic sum serving as the dependent variable and once with the dichotic gap serving as the dependent variable. The models all included age, hearing, vocabulary, working memory, and attention as predictors. Thereafter, as with the models mentioned above, each subsequent model added one additional variable, with the models building upon one another sequentially. Model 2 included baseline recognition of time-compressed speech; Model 3 added the rapid learning slope; and Model 4 added hearing aids. To isolate the unique contribution of each additional variable, these four successively complex models were compared using an ANOVA Table for Comparison of Nested Model tests.
Dichotic Sum as the Dependent Variable. For the dichotic listening task, with dichotic sum serving as the dependent variable, model comparisons showed that there were no contributions of variables/effects that did not appear in the first model (see Table 8).
Dichotic Gap As the Dependent Variable
For the dichotic listening task, with dichotic gap serving as the dependent variable, model comparisons showed that there were no contributions of variables/effects that did not appear in the first model (see Table 9).
Discussion
We assessed the relative contribution of hearing acuity, cognitive factors, and rapid perceptual learning to the identification of fast speech, speech in noise, and dichotic speech in older adults with hearing loss. Hearing acuity and time-compressed speech perception uniquely contributed to the perception of both fast speech and speech in noise. Rapid perceptual learning was a significant predictor of fast speech perception even after accounting for age, hearing, and cognition. Hearing aid use had no effect on any of the speech tasks. Our findings suggest that in older adults, good rapid perceptual learning can partially offset the effects of age and hearing loss on the perception of fast speech, but not on the perception of speech in noise or dichotic speech. Determining if this is due to inherent differences between the different speech tasks or due to other differences (e.g., overall level of difficulty) requires further investigation. Furthermore, the finding that time-compressed speech recognition is strongly associated with the perception of speech in noise suggests a potential link between the perception of these two types of challenging speech.
In the present study, hearing acuity was the strongest predictor of both fast speech and speech in noise perception. This finding is consistent with previous work on speech perception in older adults (e.g., Frisina and Frisina, 1997; Janse, 2009; Humes and Dubno, 2010). For example, Janse (2009) investigated the relative contributions of auditory and cognitive factors to fast speech perception in older adults. While hearing acuity, reading rate, and visual speed of processing were all significant predictors, hearing acuity was the strongest one. Similarly, for speech in noise among new and experienced hearing aid users, hearing loss was repeatedly identified as the primary and best predictor for unaided performance (Humes, 2002). Our study extends this finding to the perception of fast speech among hearing aid users.
An interesting outcome of the current study is that the initial performance of time-compressed speech remained the second strongest predictor of perception of both fast speech and speech in noise. These findings are in line with previous results regarding the perception of fast speech (Manheim et al., 2018; Rotman et al., 2020b) and extend them to speech in noise. Although fast speech is harder to recognize than time-compressed speech at similar rates, performance is correlated between these two tasks, and temporal processing is likely involved in the perception of both (Janse, 2004; Gordon-Salant et al., 2014). Indeed, the increased difficulties older adults have in processing distorted speech are thought to result in part from age-related declines in temporal processing (e.g., Pichora-Fuller and Singh, 2006; Anderson et al., 2011; Füllgrabe et al., 2015). Temporal cues within both the temporal envelope of the speech signal and its fine structure convey information that influences lexical, syntactic, and phonemic processing and these can support speech perception across a range of conditions (Kidd et al., 1984; Nelson and Freyman, 1987; Festen and Plomp, 1990; Rosen, 1992). Fast speech recognition can thus be affected by the temporal resolution of phonetic information and by linguistic context, suggesting that both low-level and high-level processes can independently contribute to the processing of temporally distorted speech (Gordon-Salant and Fitzgibbons, 2001; Pichora-Fuller, 2003b; Gordon-Salant et al., 2014).
As for the association between time-compressed speech and speech in noise recognition, loss of synchrony in aging auditory systems may disrupt the fine structure cues that important for recognizing speech in noise (Schneider and Pichora-Fuller, 2001). The fine structure of speech, in particular its harmonic structure, enables listeners to attend to a target speech source or to distinguish competing speech or noise sources, especially when they are spectrally similar to the target signal (Moore, 2008, 2011). Similarly, binaural advantage for detecting and identifying speech presented in a noisy background relies on the ability of the binaural system to process interaural, minimal timing differences (Levitt and Rabiner, 1967). If the perception of temporal fine structure affects both identification of speech in the presence of competing noise and fast speech, it is perhaps unsurprising that perception of time-compressed speech accounts for some of the individual differences in the perception of speech in noise. Indeed, speech reception threshold in fluctuating noise and susceptibility to time compression are highly correlated among normal-hearing and hearing-impaired older adults (Versfeld and Dreschler, 2002).
Our results indicate that the association across speech tasks is not limited to tasks that share obvious sensory characteristics. This suggests that common speech perception processes could underlie performance variability across a range of listening challenges in older adults with different levels of hearing. Consistent with this view, research on speech recognition under adverse listening conditions has shown relationships across different conditions (e.g., Borrie et al., 2017; Carbonell, 2017). For example, Carbonell (2017) found that performance was correlated across noise-vocoded, time-compressed, and speech in babble noise tasks, and regression models that predicted performance on one task based on performance of the other two also showed a strong relationship. Nevertheless, it is hard to determine whether these findings reflect common underlying processing. Furthermore, in some studies, correlations across speech conditions were more limited (Bent et al., 2016; McLaughlin et al., 2018). Bent et al. (2016) studied intelligibility under different types of signal adversity and showed that English-speaking listeners who were good at understanding non-native (Spanish) accent were also good at understanding a regional dialect (Irish English) and disordered speech (ataxic dysarthria). These results indicated that, rather than possessing a general speech skill, listeners may possess specific cue sensitivities and/or favor perceptual strategies that allow them to be successful with particular types of listening adversity. Therefore, at present, it is hard to determine whether differences between different speech conditions stem from differences in the requirements they pose on underlying auditory mechanisms, from differences in listening effort or from methodological issues. For example, in the current study and with similar tasks, recognition of fast speech was poorer than that of speech in babble, but using different fast talkers or a more challenging SNR could have changed this pattern. Further studies with conditions matched for accuracy might shed further light on this issue if listening effort is tracked and compared across conditions. As for older adults with hearing impairment, both general speech skills and specific cue sensitivities/perceptual strategies decline with aging. Further research is needed to understand individual differences in those declines, which could help shed light on the varying degrees of benefit from current rehabilitative strategies.
In contrast to previous work in older adults (e.g., Salthouse, 1994, 1996; Pichora-Fuller et al., 1995; Humes, 2007; Rotman et al., 2020b), in the present study, cognitive abilities (working memory, vocabulary, and selective attention) were not significant predictors of performance on any of the speech perception tasks. This suggests that the relationship between cognition and speech perception is not straightforward. Indeed, Akeroyd (2008) found inconsistencies across studies both when the speech and the cognitive tasks varied across studies, and also when the assessed cognitive domain (e.g., working memory) was constant and only the speech task differed. However, task and stimulus related factors do not provide a sufficient account for the discrepancies across studies, because in the current study, we used the same time-compressed, fast speech and cognitive tasks as in a previous study from our lab in which we did find an association between fast speech recognition and vocabulary (Rotman et al., 2020b). A recent review by Dryden et al. (2017) highlighted that not only do measures of speech in noise perception and cognitive tasks vary greatly across published studies, but research participant samples vary widely as well and can include any combination of young and old listeners with or without hearing loss, tested under aided or unaided listening conditions. Consistent with this view, in the current study, effect sizes (expressed in odd ratios) were similar to those observed in our previous study. Furthermore, based on our previous data (Rotman et al., 2020b), statistical power was adequate. On the other hand, hearing levels were more variable and this increased variability may have contributed to the lack of significant effects.
The current finding that hearing aid use had no effect on degraded speech perception is consistent with that of Rotman et al. (2020b). However, this finding contradicts previous research showing improved speech perception following hearing aid use (Gatehouse, 1992; Munro and Lutman, 2003; Lavie et al., 2015; Habicht et al., 2016; Dawes and Munro, 2017; Wright and Gagné, 2020). One potential explanation for this could be that in our study, the average hearing loss (PTA) in hearing aid users was approximately 10 dB more severe than in non-users (see Table 1). This greater severity of hearing loss could have masked a hearing aid induced effect despite the inclusion of PTAs in statistical modeling. Methodological differences, including: timing and duration of hearing aid use (e.g., Gatehouse, 1992; Munro and Lutman, 2003), variability of outcome measures (e.g., Larson et al., 2000; Humes et al., 2001), and lack of baseline tests before starting to use the hearing aids (e.g., Vogelzang et al., 2021), can also account for the discrepancy between studies. The above differences highlight the need for further research on speech processing among hearing aid users. For example, future studies should include an unaided condition for the group with hearing aids and an aided condition for the group without hearing aids. This could test differences between the effects of hearing aid use and the effects of amplification during testing, without using hearing aids between test sessions.
Data Availability Statement
The data from this study is available at Open Science Foundation; https://osf.io/sreq4.
Ethics Statement
This study involves human participants. It was reviewed and approved by the ethics committee of the Faculty of Social Welfare and Health Sciences, University of Haifa. Protocol 362/18 participants provided their written informed consent to participate in this study.
Author Contributions
LL and KB designed the study, prepared the study materials, and edited the manuscript. LS recruited study participants, collected and analyzed the data, and wrote the manuscript with oversight and conceptual guidance from KB and LL. All authors approved the submitted version.
Funding
This study was supported by the Israel Science Foundation (grant number 206/18).
Conflict of Interest
The authors declare that the research was conducted in the absence of any commercial or financial relationships that could be construed as a potential conflict of interest.
Publisher’s Note
All claims expressed in this article are solely those of the authors and do not necessarily represent those of their affiliated organizations, or those of the publisher, the editors and the reviewers. Any product that may be evaluated in this article, or claim that may be made by its manufacturer, is not guaranteed or endorsed by the publisher.
Acknowledgments
The authors wish to thank Mor Ben-Dor for her help in the data collection.
References
Ahissar, M., Nahum, M., Nelken, I., and Hochstein, S. (2009). Reverse hierarchies and sensory learning. Philos. Trans. R. Soc. Lond. B Biol. Sci. 364, 285–299. doi: 10.1098/rstb.2008.0253
Akeroyd, M. A. (2008). Are individual differences in speech reception related to individual differences in cognitive ability? A survey of twenty experimental studies with normal and hearing-impaired adults. Int. J. Audiol. 47(Suppl. 2), S53–S71. doi: 10.1080/14992020802301142
Anderson, S., Parbery-Clark, A., Yi, H. G., and Kraus, N. (2011). A neural basis of speech-in-noise perception in older adults. Ear Hear. 32, 750–757. doi: 10.1097/AUD.0b013e31822229d3
Banai, K., and Lavie, L. (2020). Perceptual learning and speech perception: A new hypothesis. Proceedings of the International Symposium on Auditory and Audiological Research, 7, 53–60.
Banai, K., and Lavie, L. (2021). Rapid perceptual learning and individual differences in speech perception: The good, the bad, and the sad. Audit. Percept. Cognition 3, 201–211. doi: 10.1080/25742442.2021.1909400
Bates, D., Maechler, M., Bolker, B., and Walker, S. (2014). Lme4: linear mixed-effects models using Eigen and S4. R package version 1, 1–7.
Ben-David, B. M., Erel, H., Goy, H., and Schneider, B. A. (2015). “Older is always better”: age-related differences in vocabulary scores across 16 years. Psychol. Aging 30, 856–862. doi: 10.1037/pag0000051
Bent, T., Baese-Berk, M., Borrie, S. A., and McKee, M. (2016). Individual differences in the perception of regional, nonnative, and disordered speech varieties. J. Acoust. Soc. Am. 140, 3775–3786. doi: 10.1121/1.4966677
Bieber, R. E., and Gordon-Salant, S. (2021). Improving older adults' understanding of challenging speech: auditory training, rapid adaptation and perceptual learning. Hear. Res. 402, 1–68. doi: 10.1016/j.heares.2020.108054
Borrie, S. A., Baese-Berk, M., Van Engen, K., and Bent, T. A. (2017). A relationship between processing speech in noise and dysarthric speech. J. Acoust. Soc. Am. 141, 4660–4667. doi: 10.1121/1.4986746
Bronkhorst, A. W. (2015). The cocktail-party phenomenon revisited: early processing and selection of multi-talker speech. Atten. Percept. Psychophys. 77, 1465–1487. doi: 10.3758/s13414-015-0882-9
Burke, D. M., and Shafto, M. A. (2008). “Language and aging,” in The Handbook of Aging and Cognition. eds. F. I. M. Craik and T. A. Salthouse. 3rd Edn. (New York: Psychology Press), 373–443.
Carbonell, K. M. (2017). Reliability of individual differences in degraded speech perception. J. Acoust. Soc. Am. 142, EL461–EL466. doi: 10.1121/1.5010148
Cherry, E. C. (1953). Some experiments on the recognition of speech, with one and two ears. J. Acoust. Soc. Am. 25, 975–979. doi: 10.1121/1.1907229
Committee on Hearing, Bioacoustics and Biomechanics (CHABA) (1988). Working group on speech understanding, Speech understanding and aging. J. Acoust. Soc. Am. 83, 859–895. doi: 10.1121/1.395965
Cox, R. M., and Xu, J. (2010). Short and long compression release times: speech understanding, real world preferences, and association with cognitive ability. J. Am. Acad. Audiol. 21, 121–138. doi: 10.3766/jaaa.21.2.6
Dale, G., Cochrane, A., and Green, C. S. (2021). Individual difference predictors of learning and generalization in perceptual learning. Atten. Percept. Psychophys. 83, 2241–2255. doi: 10.3758/s13414-021-02268-3
Dawes, P., and Munro, K. J. (2017). Auditory distraction and acclimatization to hearing aids. Ear Hearing 38, 174–183. doi: 10.1097/AUD.0000000000000366
Dawes, P., Munro, K. J., Kalluri, S., and Edwards, B. (2013). Unilateral and bilateral hearing aids, spatial release from masking and auditory acclimatization. J. Acoust. Soc. Am. 134, 596–606. doi: 10.1121/1.4807783
Dawes, P., Munro, K. J., Kalluri, S., and Edwards, B. (2014a). Auditory acclimatization and hearing aids: late auditory evoked potentials and speech recognition following unilateral and bilateral amplification. J. Acoust. Soc. Am. 135, 3560–3569. doi: 10.1121/1.4874629
Dawes, P., Munro, K. J., Kalluri, S., and Edwards, B. (2014b). Acclimatization to hearing aids. Ear Hear. 35, 203–212. doi: 10.1097/AUD.0b013e3182a8eda4
Dias, J. W., McClaskey, C. M., and Harris, K. C. (2019). Time-compressed speech identification is predicted by auditory neural processing, Perceptuomotor speed, and executive functioning in younger and older listeners. J. Assoc. Res. Otolaryngol. 20, 73–88. doi: 10.1007/s10162-018-00703-1
Dryden, A., Allen, H. A., Henshaw, H., and Heinrich, A. (2017). The association Between cognitive performance and speech-in-noise perception for adult listeners: A systematic literature review and meta-analysis. Trends Hear. 21:44675. doi: 10.1177/2331216517744675
Dubno, J. R., Ahlstrom, J. B., and Horwitz, A. R. (2008). Binaural advantage for younger and older adults with normal hearing. J. Speech Lang. Hear. Res. 51, 539–556. doi: 10.1044/1092-4388(2008/039)
Dubno, J. R., Dirks, D. D., and Morgan, D. E. (1984). Effects of age and mild hearing loss on speech recognition in noise. J. Acoust. Soc. Am. 76, 87–96. doi: 10.1121/1.391011
Eriksen, B. A., and Eriksen, C. W. (1974). Effects of noise letters upon the identification of a target letter in a nonsearch task. Percept. Psychophys. 16, 143–149. doi: 10.3758/BF03203267
Festen, J. M., and Plomp, R. (1990). Effects of fluctuating noise and interfering speech on the speech-reception threshold for impaired and normal hearing. J. Acoustic. Soc. Am. 88, 1725–1736. doi: 10.1121/1.400247
Folstein, M., Folstein, S., and McHugh, P. (1975). "mini-mental state": a practical method for grading the cognitive state of patients for the clinician. J. Psychiatr. Res. 12, 189–198. doi: 10.1016/0022-3956(75)90026-6
Forstmann, B. U., Tittgemeyer, M., Wagenmakers, E. J., Derrfuss, J., Imperati, D., and Brown, S. (2011). The speed-accuracy tradeoff in the elderly brain: A structural model-based approach. J. Neurosci. 31, 17242–17249. doi: 10.1523/JNEUROSCI.0309-11.2011
Frisina, D. R., and Frisina, R. D. (1997). Speech recognition in noise and presbycusis: relations to possible neural mechanisms. Hear. Res. 106, 95–104. doi: 10.1016/s0378-5955(97)00006-3
Füllgrabe, C., Moore, B. C. J., and Stone, M. A. (2015). Age-group differences in speech identification despite matched audiometrically normal hearing: contributions from auditory temporal processing and cognition. Front. Aging Neurosci. 6, 1–25. doi: 10.3389/fnagi.2014.00347
Gatehouse, S. (1992). The time course and magnitude of perceptual acclimatization to frequency responses: evidence from monaural fitting of hearing aids. J. Acoust. Soc. Am. 92, 1258–1268. doi: 10.1121/1.403921
Gatehouse, S., Naylor, G., and Elberling, C. (2006). Linear and nonlinear hearing aid fittings – 2. Patterns of candidature. Int. J. Audiol. 45, 153–171. doi: 10.1080/14992020500429484
Gates, G. A., and Mills, J. H. (2005). Presbycusis. Lancet 366, 1111–1120. doi: 10.1016/S0140-6736(05)67423-5
Golomb, J. D., Peelle, J. E., and Wingfield, A. (2007). Effects of stimulus variability and adult aging on adaptation to time-compressed speech. J. Acoust. Soc. Am. 121, 1701–1708. doi: 10.1121/1.2436635
Gordon-Salant, S., and Fitzgibbons, P. J. (1993). Temporal factors and speech recognition performance in young and elderly listeners. J. Speech Hear. Res. 36, 1276–1285. doi: 10.1121/1.2436635
Gordon-Salant, S., and Fitzgibbons, P. J. (2001). Sources of age-related recognition difficulty for time-compressed speech. J. Speech Lang. Hear. Res. 44, 709–719. doi: 10.1044/1092-4388(2001/056)
Gordon-Salant, S., Zion, D. J., and Espy-Wilson, C. (2014). Recognition of time-compressed speech does not predict recognition of natural fast-rate speech by older listeners. J. Acoust. Soc. Am. 136, EL268–EL274. doi: 10.1121/1.4895014
Green, P., MacLeod, C. J., and Nakagawa, S. (2016). SIMR: an R package for power analysis of generalized linear mixed models by simulation. Methods Ecol. Evol. 7, 493–498. doi: 10.1111/2041-210X.12504
Habicht, J., Kollmeier, B., and Neher, T. (2016). Are experienced hearing aid users faster at grasping the meaning of a sentence than inexperienced users? An eye-tracking study. Trend. Hear. 20, 1–13. doi: 10.1177/2331216516660966
Hargus, S. E., and Gordon-Salant, S. (1995). Accuracy of speech intelligibility index predictions for noise-masked young listeners with normal hearing and for elderly listeners with hearing impairment. J. Speech Hear. Res. 38, 234–243. doi: 10.1044/jshr.3801.234
Heffner, C. C., and Myers, E. B. (2021). Individual differences in phonetic plasticity Across native and nonnative contexts. J. Speech Lang. Hear. 64, 3720–3733. doi: 10.1044/2021_JSLHR-21-00004
Helfer, K. S., and Freyman, R. L. (2008). Aging and speech-on-speech masking. Ear Hear. 29, 87–98. doi: 10.1097/AUD.0b013e31815d638b
Hopkins, K., and Moore, B. C. (2007). Moderate cochlear hearing loss leads to a reduced ability to use temporal fine structure information. J. Acoust. Soc. Am. 122, 1055–1068. doi: 10.1121/1.2749457
Hopkins, K., and Moore, B. C. (2011). The effects of age and cochlear hearing loss on temporal fine structure sensitivity, frequency selectivity, and speech reception in noise. J. Acoust. Soc. Am. 130, 334–349. doi: 10.1121/1.3585848
Humes, L. E. (2002). Factors underlying the speech-recognition performance of elderly hearing-aid wearers. J. Acoust. Soc. Am. 112, 1112–1132. doi: 10.1121/1.1499132
Humes, L. E. (2007). The contributions of audibility and cognitive factors to the benefit provided by amplified speech to older adults. J. Am. Acad. Audiol. 18, 590–603. doi: 10.3766/jaaa.18.7.6
Humes, L. E., and Dubno, J. R. (2010). “Factors affecting speech understanding in older adults,” in The Aging Auditory System. Vol. 34. eds. S. Gordon-Salant, R. D. Frisina, A. N. Popper, and R. R. Fay (New York: Springer), 167–210.
Humes, L. E., Dubno, J. R., Gordon-Salant, S., Lister, J. J., Cacace, A. T., Cruickshanks, K. J., et al. (2012). Central presbycusis: a review and evaluation of the evidence. J. Am. Acad. Audiol. 23, 635–666. doi: 10.3766/jaaa.23.8.5
Humes, L. E., Garner, C. B., Wilson, D. L., and Barlow, N. N. (2001). Hearing-aid outcome measured following one month of hearing aid use by the elderly. J. Speech Lang. Hear. Res. 44, 469–486. doi: 10.1044/1092-4388(2001/037)
Humes, L. E., Lee, J. H., and Coughlin, M. P. (2006). Auditory measures of selective and divided attention in young and older adults using single-talker competition. J. Acoust. Soc. Am. 120, 2926–2937. doi: 10.1121/1.2354070
Humes, L. E., and Roberts, L. (1990). Speech-recognition difficulties of the hearing-impaired elderly: the contributions of audibility. J. Speech Hear. Res. 33, 726–735. doi: 10.1044/jshr.3304.726
Janse, E. (2004). Word perception in fast speech: artificially time-compressed vs. naturally produced fast speech. Speech Comm. 42, 155–173. doi: 10.1016/j.specom.2003.07.001
Janse, E. (2009). Processing of fast speech by elderly listeners. J. Acoust. Soc. Am. 125, 2361–2373. doi: 10.1121/1.3082117
Jerger, J., Alford, B., Lew, H., Rivera, V., and Chmiel, R. (1995). Dichotic listening, event-related potentials, and interhemispheric transfer in the elderly. Ear Hear. 16, 482–498. doi: 10.1097/00003446-199510000-00005
Jerger, J., Chmiel, R., Allen, J., and Wilson, A. (1994). Effects of age and gender on dichotic sentence identification. Ear Hear. 15, 274–286. doi: 10.1097/00003446-199408000-00002
Jerger, J., Jerger, S., and Pirozzolo, F. (1991). Correlational analysis of speech audiometric scores, hearing loss, age, and cognitive abilities in the elderly. Ear Hear. 12, 103–109. doi: 10.1097/00003446-199104000-00004
Kalluri, S., Ahmann, B., and Munro, K. J. (2019). A systematic narrative synthesis of acute amplification-induced improvements in cognitive ability in hearing-impaired adults. Int. J. Audiol. 58, 455–463. doi: 10.1080/14992027.2019.1594414
Karawani, H., Lavie, L., and Banai, K. (2017). Short-term auditory learning in older and younger adults. Proceedings of the International Symposium on Auditory and Audiological Research, 6, 1–8.
Kidd, G. Jr., Mason, C. R., and Feth, L. L. (1984). Temporal integration of forward masking in listeners having sensorineural hearing loss. J. Acoust. Soc. Am. 75, 937–944. doi: 10.1121/1.390558
Koreman, J. (2006). Perceived speech rate: the effects of articulation rate and speaking style in spontaneous speech. J. Acoust. Soc. Am. 119, 582–596. doi: 10.1121/1.2133436
Larson, V. D., Williams, D. W., Henderson, W. G., Luethke, L. E., Beck, L. B., Noffsinger, D., et al. (2000). Efficacy of 3 commonly used hearing aid circuits: A crossover trial. JAMA 284, 1806–1813. doi: 10.1001/jama.284.14.1806
Lavie, L. (2011). Plasticity of the Auditory System in the Elderly Following Hearing Aids Usage. Doctoral Dissertation, University of Haifa, Israel.
Lavie, L., Attias, J., and Karni, A. (2013). Semi-structured listening experience (listening training) in hearing aid fitting: influence on dichotic listening. Am. J. Audiol. 22, 347–350. doi: 10.1044/1059-0889(2013/12-0083)
Lavie, L., Banai, K., Karni, A., and Attias, J. (2015). Hearing aid-induced plasticity in the auditory system of older adults: evidence from speech perception. J. Speech Lang. Hear. Res. 58, 1601–1610. doi: 10.1044/2015_JSLHR-H-14-0225
Lavie, L., Banai, K., and Shechter Shvartzman, L. (2021). Plastic changes in speech perception in hearing-impaired older adults following hearing aid use: a systematic review. Int. J. Audiol., 1–9. doi: 10.1080/14992027.2021.2014073
Leek, M. R., and Summers, V. (1993). Auditory filter shapes of normal- hearing and hearing- impaired listeners in continuous broadband noise. J. Acoust. Soc. Am. 94, 3127–3137. doi: 10.1121/1.407218
Levitt, H., and Rabiner, L. R. (1967). Binaural release from masking for speech and gain in intelligibility. J. Acoust. Soc. Am. 42, 601–608. doi: 10.1121/1.1910629
Lu, P. H., Lee, G. J., Raven, E. P., Tingus, K., Khoo, T., Thompson, P. M., et al. (2011). Age-related slowing in cognitive processing speed is associated with myelin integrity in a very healthy elderly sample. J. Clin. Exp. Neuropsychol. 33, 1059–1068. doi: 10.1080/13803395.2011.595397
Lunner, T. (2003). Cognitive function in relation to hearing aid use. Int. J. Audiol. 42, 49–58. doi: 10.3109/14992020309074624
Luterman, D. M., Welsh, O. L., and Melrose, J. (1966). Responses of aged males to time-altered speech stimuli. J. Speech Hear. Res. 9, 226–230. doi: 10.1044/jshr.0902.226
Manheim, M., Lavie, L., and Banai, K. (2018). Age, hearing, and the perceptual learning of rapid speech. Trend. Hear. 22, 1–18. doi: 10.1177/2331216518778651
Mattys, S. L., Davis, M. H., Bradlow, A. R., and Scott, S. K. (2012). Speech recognition in adverse conditions: A review. Lang. Cogni. Proces. 27, 953–978. doi: 10.1080/01690965.2012.705006
McDowd, J. M., and Shaw, R. J. (2000). “Attention and aging: A functional perspective,” in Handbook of Aging and Cognition. eds. F. I. M. Craik and T. A. Salthouse. 2nd ed (New York: Erlbaum), 221–292.
McLaughlin, D. J., Baese-Berk, M. M., Bent, T., Borrie, S. A., and Van Engen, K. J. (2018). Coping with adversity: individual differences in the perception of noisy and accented speech. Atten. Percept. Psychophys. 80, 1559–1570. doi: 10.3758/s13414-018-1537-4
Moore, B. C. (2008). The role of temporal fine structure processing in pitch perception, masking, and speech perception for normal-hearing and hearing-impaired people. J. Assoc. Res. Otolaryngol. 9, 399–406. doi: 10.1007/s10162-008-0143-x
Moore, B. C. J. (2011). The importance of temporal fine structure for the intelligibility of speech in complex backgrounds. Proceedings of the International symposium on Auditory and Audiological Research, 3, 21–32.
Morrell, C. H., Gordon-Salant, S., Pearson, J. D., Brant, L. J., and Fozard, J. L. (1996). Age- and gender-specific reference ranges for hearing level and longitudinal changes in hearing level. J. Acoust. Soc. Am. 100, 1949–1967. doi: 10.1121/1.417906
Munro, K. J., and Lutman, M. E. (2003). The effect of speech presentation level on measurement of auditory acclimatization to amplified speech. J. Acoust. Soc. Am. 114, 484–495. doi: 10.1121/1.1577556
Needleman, A. R., and Crandell, C. C. (1995). Speech recognition in noise by hearing-impaired and noise-masked normal-hearing listeners. J. Am. Acad. Audiol. 6, 414–424.
Neher, T., Grimm, G., and Hohmann, V. (2014). Perceptual consequences of different signal changes due to binaural noise reduction: do hearing loss and working memory capacity play a role? Ear Hear. 35, e213–e227. doi: 10.1097/AUD.0000000000000054
Nelson, D. A., and Freyman, R. L. (1987). Temporal resolution in sensorineural hearing-impaired listeners. J. Acoust. Soc. Am. 81, 709–720. doi: 10.1121/1.395131
Peelle, J. E., and Wingfield, A. (2005). Dissociations in perceptual learning revealed by adult age differences in adaptation to time-compressed speech. J. Exp. Psychol. Hum. Percept. Perform. 31, 1315–1330. doi: 10.1037/0096-1523.31.6.1315
Peters, R. W., and Moore, B. C. (1992). Auditory filter shapes at low center frequencies in young and elderly hearing- impaired subjects. J. Acoust. Soc. Am. 91, 256–266. doi: 10.1121/1.402769
Phillips, S. L., Gordon-Salant, S., Fitzgibbons, P. J., and Yeni-Komshian, G. (2000). Frequency and temporal resolution in elderly listeners with good and poor word recognition. J. Speech Lang. Hear. Res. 43, 217–228. doi: 10.1044/jslhr.4301.217
Pichora-Fuller, M. K. (1997). Language comprehension in older adults. J. Speech Lan. Pathol. Audiol. 21, 125–142.
Pichora-Fuller, M. K. (2003a). Cognitive aging and auditory information processing. Int. J. Audiol. 42(Suppl. 1):26. doi: 10.3109/14992020309074641
Pichora-Fuller, M. K. (2003b). Processing speed and timing in aging adults: psychoacoustics, speech perception, and comprehension. Int. J. Audiol. 42(Suppl. 2), S59–S67. doi: 10.3109/14992020309074625
Pichora-Fuller, M. K., Schneider, B. A., and Daneman, M. (1995). How young and old adults listen to and remember speech in noise. J. Acoust. Soc. Am. 97, 593–608. doi: 10.1121/1.412282
Pichora-Fuller, M. K., and Singh, G. (2006). Effects of age on auditory and cognitive processing implications for hearing aid fitting and audiologic rehabilitation. Trends Amplif. 10, 29–59. doi: 10.1177/108471380601000103
Pichora-Fuller, M. K., and Souza, P. E. (2003). Effects of aging on auditory processing of speech. Int. J. Audiol. 42:(Suppl. 2), 11. doi: 10.3109/14992020309074638
Prior, A., and Bentin, S. (2006). Differential integration efforts of mandatory and optional sentence constituents. Psychophysiology 43, 440–449. doi: 10.1111/j.1469-8986.2006.00426.x
Rabbitt, P. M. A. (1990). Mild hearing loss can cause apparent memory failures which increase with age and reduce with IQ. Acta Otolaryngologica (Suppl. 111), 167–176. doi: 10.3109/00016489109127274
Reitan, R. M. (1958). Validity of the trail making test as an indicator of organic brain damage. Percept. Mot. Skills 8, 271–276. doi: 10.2466/pms.1958.8.3.271
Rogers, W. A. (2000). “Attention and aging,” in Cognitive Aging: A primer. eds. D. C. Park and N. Schwarz (New York: Psychology Press), 57–73.
Rönnberg, J., Lunner, T., Zekveld, A., Sörqvist, P., Danielsson, H., Lyxell, B., et al. (2013). The ease of language understanding (ELU) model: theoretical, empirical, and clinical advances. Front. Syst. Neurosci. 7, 1–17. doi: 10.3389/fnsys.2013.00031
Rosen, S. (1992). Temporal information in speech: acoustic, auditory and linguistic aspects. Philos. Trans. R. Soc. Lond. Ser. B Biol. Sci. 336, 367–373. doi: 10.1098/rstb.1992.0070
Rotman, T., Lavie, L., and Banai, K. (2020a). Rapid perceptual learning of time-compressed speech and the perception of natural fast speech in older adults with presbycusis. Proceedings of the International symposium on Auditory and Audiological Research, 7, 93–100.
Rotman, T., Lavie, L., and Banai, K. (2020b). Rapid perceptual learning: a potential source of individual differences in speech perception under adverse conditions? Trend. Hear. 24, 1–16. doi: 10.1177/2331216520930541
Roup, C. M., Wiley, T. L., and Wilson, R. H. (2006). Dichotic word recognition in young and older adults. J. Am. Acad. Audiol. 54, 292–297. doi: 10.1044/1092-4388(2010/09-0230)
Salthouse, T. A. (1994). The aging of working memory. Neuropsychology 8, 535–543. doi: 10.1037/0894-4105.8.4.535
Salthouse, T. A. (1996). The processing-speed theory of adult age differences in cognition. Psychol. Rev. 103, 403–428. doi: 10.1037/0033-295X.103.3.403
Samuel, A. G., and Kraljic, T. (2009). Perceptual learning for speech. Atten. Percept. Psychophys. 71, 1207–1218. doi: 10.3758/APP.71.6.1207
Schneider, B. A., Daneman, M., and Pichora-Fuller, M. K. (2002). Listening in aging adults: from discourse comprehension to psychoacoustics. Can. J. Exp. Psychol. 56, 139–152. doi: 10.1037/h0087392
Schneider, B. A., Li, L., and Daneman, M. (2007). How competing speech interferes with speech comprehension in everyday listening situations. J. Am. Acad. Audiol. 18, 559–572. doi: 10.3766/jaaa.18.7.4
Schneider, B. A., and Pichora-Fuller, M. K. (2001). Age-related changes in temporal processing: implications for listening comprehension. Semin. Hear. 22, 227–240. doi: 10.1177/108471380601000103
Schneider, B. A., Pichora-Fuller, K., and Daneman, M. (2010). “Effects of senescent changes in audition and cognition on spoken language comprehension,” in The Aging Auditory System. eds. S. Gordon-Salant, R. D. Frisina, R. R. Fay, and A. N. Popper (New York: Springer International Publishing), 167–210.
Schum, D. J., Matthews, L. J., and Lee, F. S. (1991). Actual and predicted word-recognition performance of elderly hearing-impaired listeners. J. Speech Lang. Hear. Res. 34, 636–642. doi: 10.1044/jshr.3403.636
Sheldon, S., Pichora-Fuller, M. K., and Schneider, B. A. (2008). Priming and sentence context support listening to noise-vocoded speech by younger and older adults. J. Acoust. Soc. Am. 123, 489–499. doi: 10.1121/1.2783762
Signoret, C., and Ruder, M. (2019). Hearing impairment and perceived clarity of predictable speech. Ear Hear. 40, 1140–1148. doi: 10.1097/AUD.0000000000000689
Souza, P. (2016). “Speech perception and hearing aids,” in Hearing Aids. eds. G. R. Popelka, B. C. J. Moore, R. R. Fay, and A. N. Popper (New York: Springer International Publishing), 151–180.
Souza, P., Arehart, K., and Neher, T. (2015). Working memory and hearing aid processing: literature findings, future directions, and clinical applications. Front. Psychol. 6, 1–12. doi: 10.3389/fpsyg.2015.01894
Tun, P. A. (1998). Fast noisy speech: age differences in processing rapid speech with background noise. Psychol. Aging 13, 424–434. doi: 10.1037/0882-7974.13.3.424
Tun, P. A., O'Kane, G., and Wingfield, A. (2002). Distraction by competing speech in young and older adult listeners. Psychol. Aging 17, 453–467. doi: 10.1037/0882-7974.17.3.453
Tun, P. A., and Wingfield, A. (1999). One voice too many: adult age differences in language processing with different types of distracting sounds. J. Gerontol. Psychol. Sci. 54, P317–P327. doi: 10.1093/geronb/54B.5.P317
Tun, P. A., Wingfield, A., Stine, E. A., and Mecsas, C. (1992). Rapid speech processing and divided attention: processing rate versus processing resources as an explanation of age effects. Psychol. Aging 7, 546–550. doi: 10.1037//0882-7974.7.4.546
Vaughan, N., Storzbach, D., and Furukawa, I. (2006). Sequencing versus nonsequencing working memory in understanding of rapid speech by older listeners. J. Am. Acad. Audiol. 17, 506–518. doi: 10.3766/jaaa.17.7.6
Verhaeghen, P. (2003). Aging and vocabulary score: a meta-analysis. Psychol. Aging 18, 332–339. doi: 10.1037/0882-7974.18.2.332
Verhelst, W., and Roelands, M. (1993). An overlap-add technique based on waveform similarity (Wsola) for high quality time-scale modification of speech [paper presentation]. IEEE International Conference on Acoustics, Speech, and Signal Processing (ICASSP), Minneapolis, MN, United States.
Versfeld, N. J., and Dreschler, W. A. (2002). The relationship between the intelligibility of time-compressed speech and speech in noise in young and elderly listeners. J. Acoust. Soc. Am. 111, 401–408. doi: 10.1121/1.1426376
Vogelzang, M., Thiel, C. M., Rosemann, S., Rieger, J. W., and Ruigendijk, E. (2021). Effects of age-related hearing loss and hearing aid experience on sentence processing. Sci. Rep. 11, 5994–5914. doi: 10.1038/s41598-021-85349-5
Wechsler, D. (1997). WAIS-3: Wechsler Adult Intelligence Scale: Administration and Scoring Manual. Psychological Corporation.
Wingfeld, A., McCoy, S. L., Peelle, J. E., Tun, P. A., and Cox, C. L. (2006). Effects of adult aging and hearing loss on comprehension of rapid speech varying in syntactic complexity. J. Am. Acad. Audiol. 17, 487–497. doi: 10.3766/jaaa.17.7.4
Wingfield, A. (1996). Cognitive factors in auditory performance: context, speed of processing, and constraints of memory. J. Am. Acad. Audiol. 7, 175–182.
Wingfield, A., Poon, L. W., Lombardi, L., and Lowe, D. (1985). Speed of processing in Normal aging: effects of speech rate, linguistic structure, and processing time. J. Gerontol. 40, 579–585. doi: 10.1093/geronj/40.5.579
Wright, D., and Gagné, J. P. (2020). Acclimatization to hearing aids by older adults. Ear Hear. 42, 193–205. doi: 10.1097/AUD.0000000000000913
Keywords: perceptual learning, degraded speech, hearing aids, aging, age-related hearing loss
Citation: Shechter Shvartzman L, Lavie L and Banai K (2022) Speech Perception in Older Adults: An Interplay of Hearing, Cognition, and Learning? Front. Psychol. 13:816864. doi: 10.3389/fpsyg.2022.816864
Edited by:
Leah Fostick, Ariel University, IsraelReviewed by:
Yang Zhang, University of Minnesota Health Twin Cities, United StatesCarine Signoret, Linköping University, Sweden
Copyright © 2022 Shechter Shvartzman, Lavie and Banai. This is an open-access article distributed under the terms of the Creative Commons Attribution License (CC BY). The use, distribution or reproduction in other forums is permitted, provided the original author(s) and the copyright owner(s) are credited and that the original publication in this journal is cited, in accordance with accepted academic practice. No use, distribution or reproduction is permitted which does not comply with these terms.
*Correspondence: Liat Shechter Shvartzman, bGlhdC5zaGVjaHRlci44NkBnbWFpbC5jb20=; Limor Lavie, bGxhdmllQHdlbGZhcmUuaGFpZmEuYWMuaWw=; Karen Banai, a2JhbmFpQHJlc2VhcmNoLmhhaWZhLmFjLmls