- 1School of Foreign Languages, Zhejiang Gongshang University, Hangzhou, China
- 2School of Foreign Studies, Hunan First Normal University, Changsha, China
The coronavirus disease 2019 (COVID-19) pandemic has influenced all aspects significantly, and an estimated 1.5 billion students across the globe have been forced to keep up with online courses at home. Many recent empirical studies reported the prevalence of mental health problems among students caused by remote learning during the COVID-19 pandemic, but a few studies aggregated these results. Therefore, to strengthen statistical power, the article aimed to examine the prevalence of anxiety, depression, and stress among remote learning students during the COVID-19 pandemic via a meta-analysis. A total of 36 original articles have been selected from five academic databases, including Web of Science, PubMed, Scopus, EBSCO, and Google Scholar, covering 78,674 participants in 19 nations, and yielding 60 effect sizes (22 for anxiety, 17 for depression, and 21 for stress) based on the random effects model via Comprehensive Meta-Analysis (CMA) software. The results showed that the prevalence of anxiety, depression, and stress among remote learning students during the COVID-19 pandemic was as high as 58, 50, and 71%, respectively. Besides, the moderator analysis found that (1) the prevalence of anxiety and depression among students in higher education was significantly higher than that of students in elementary education. (2) an increasing number of medical students and students in emergency remote learning context suffered from mental stress than their non-medical and traditional distance learning counterparts. Thus, the COVID-19 pandemic triggers concerns related to physical health and mental disorders, especially for remote online learning students. The current situation should be brought to the forefront by educators to develop psychological interventions for relieving students’ anxiety, depression, and stress during the pandemic period.
1. Introduction
In December 2019, a cluster of pneumonia cases caused by a newly discovered coronavirus was reported in Wuhan, China. The disease was named coronavirus disease 2019 (COVID-19), which first spread across China and then across 216 nations, areas, or territories worldwide (Dutta et al., 2020). Facing the rapid spread of the disease, the World Health Organization (WHO) declared the COVID-19 outbreak a Public Health Emergency of International Concern on 30 January 2020 and a pandemic on 12 March 2020 (WHO, 2020). According to the statistics reported by United Nations Educational, Scientific and Cultural Organization (UNESCO), schools and universities have been closed in around 190 countries across the world to prevent and control the spread of the COVID-19 pandemic, which leads to an estimated 1.5 billion students of all ages having been forced to stay at home and keep up with lessons online (WHO, 2022).
Online education, including online teaching and learning, refers to a new style that involves no in-person communication but digital online interaction between students and instructors (Tunc and Toprak, 2022). Effective online education requires careful planning and preparation not only for course content but also for providing support for different types of digital interactions (Fuchs, 2022). However, the outbreak of the COVID-19 pandemic broke the traditional model of online learning. A new concept, emergency remote learning was put forward and refers to a temporary switch of instruction delivery via an alternate delivery mode because of crisis circumstances (Tunc and Toprak, 2022). One of the major primary misalignments between online education and emergency remote education is that the latter is usually improvised with little time to plan and prepare due to time constraints (Ferri et al., 2020; Fuchs, 2022).
Although online education possesses distinct advantages, such as saving commuting time and flexibility to choose, disadvantages such as online study potentially influencing academic performance and the lack of practical knowledge have also been found (Ferri et al., 2020; Kotrikadze and Zharkova, 2021). Apart from the negative effect exerted on the study itself, distance learning also causes many mental problems, such as online anxiety (anxiety aroused from acquiring knowledge through the use of the internet; Warner et al., 2020) and technostress (stress aroused from the prolonged exposure of information-communication-technologies; Torales et al., 2022). After the outbreak of the COVID-19 pandemic, many scholars across the globe have paid closer attention to the mental health of students who have undergone (emergency) online learning. For example, in China, the prevalence of symptoms of anxiety, depression, and stress was observed in 32.9, 31.9, and 14.6%, respectively, of university medical students (Chang et al., 2021), and 25.13, 29.95, and 75.89% of university students from different majors suffered from anxiety, depression, and insomnia symptoms, respectively (Zhang et al., 2022). In Poland, a total of 56 and 58% of the students from the Opole University of Technology were characterized by depression and stress symptoms, and even 18% of the participants had suicidal thoughts (Rutkowska et al., 2022). Despite numerous empirical studies focusing on the detection rate of mental problems among remote learning students during the COVID-19 pandemic, a few studies integrated the results of these multiple studies that tend to address the same question. Therefore, to aggregate data resulting in a more substantial statistical power than any individual study, the study aimed to examine the prevalence of anxiety, depression, and stress among remote learning students during the COVID-19 pandemic via a meta-analysis.
2. Methods
This study aimed to explore the prevalence of anxiety, depression, and stress among remote learning students during the COVID-19 pandemic. To achieve this aim, previous studies were searched first and screened based on the inclusion and exclusion criteria. Then, data were extracted and coded from selected empirical studies. Statistical analyses including testing and correcting publication bias, selecting a model, and processing data were carried out as the last procedure. The methods of the study are introduced in subsections.
2.1. Literature searching and screening
The present meta-analysis concentrated on the prevalence of anxiety, depression, and stress among remote learning students during the COVID-19 pandemic. The first step in conducting this study is searching and screening the previous studies on this well-specific subject. Academic databases, including Web of Science, PubMed, Scopus, EBSCO, and Google Scholar, were used to search related literature systematically. To identify the articles, search terms (i.e., COVID-19, Coronavirus, Online learning, emergency online learning, remote learning, distance learning, e-learning, mental health, anxiety, depression, and stress) and all the possible combinations of these keywords were input in the search bar with the following string: (“COVID-19” OR “Coronavirus”) AND (“Online learning” OR “emergency online learning” OR “remote learning” OR “distance learning” OR “e-learning”) AND (“mental health” OR “anxiety” OR “depression” OR “stress”). The final search date of this study was 1 November 2022.
The criteria for screening resulting articles were as follows: (1) studies that were published during the COVID-19 pandemic; (2) studies that were empirical research; (3) studies that reported the detection rate of anxiety, depression, or stress among remote learning students during the COVID-19 pandemic; (4) studies that reported the accurate sample size; and (5) studies that specified whether students had undergone online learning or emergency online learning. After applying these inclusion criteria, a total of 36 studies, yielding 22 anxiety effect sizes, 17 depression effect sizes, and 21 stress effect sizes, were selected in this meta-analysis (see flow diagram of Figure 1).
2.2. Data extraction and coding
Selected studies were coded, and data were extracted according to the following variables: author, publication year, country, learning phrase, students’ major, whether attending emergency online learning, measurement, sample size, and prevalence rate. Tables 1–3 show the summary coding of included studies on anxiety, depression, and stress.
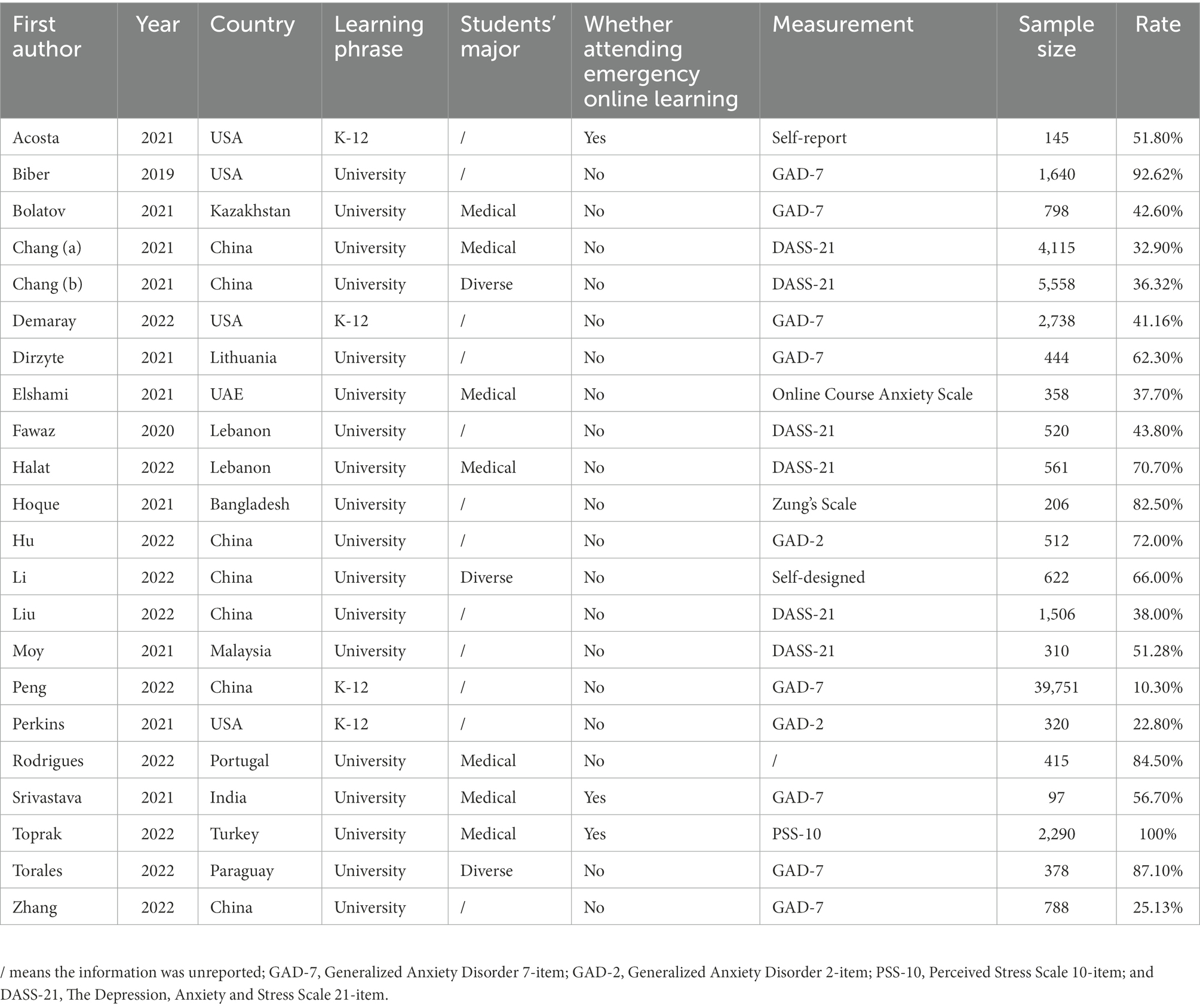
Table 1. Summary coding of included anxiety studies (arranged in the alphabetical order of the first author).
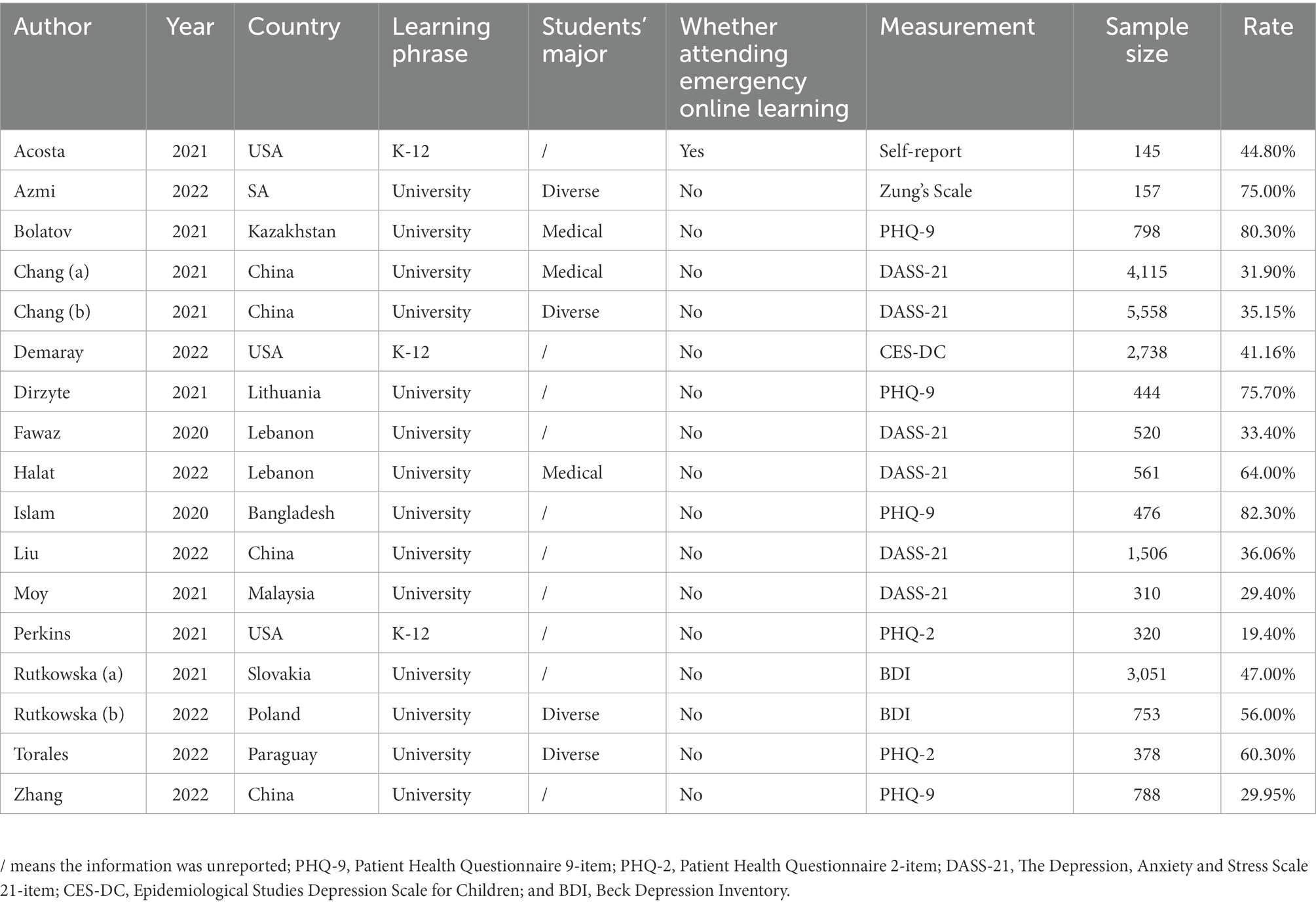
Table 2. Summary coding of included depression studies (arranged in alphabetical order of the first author).
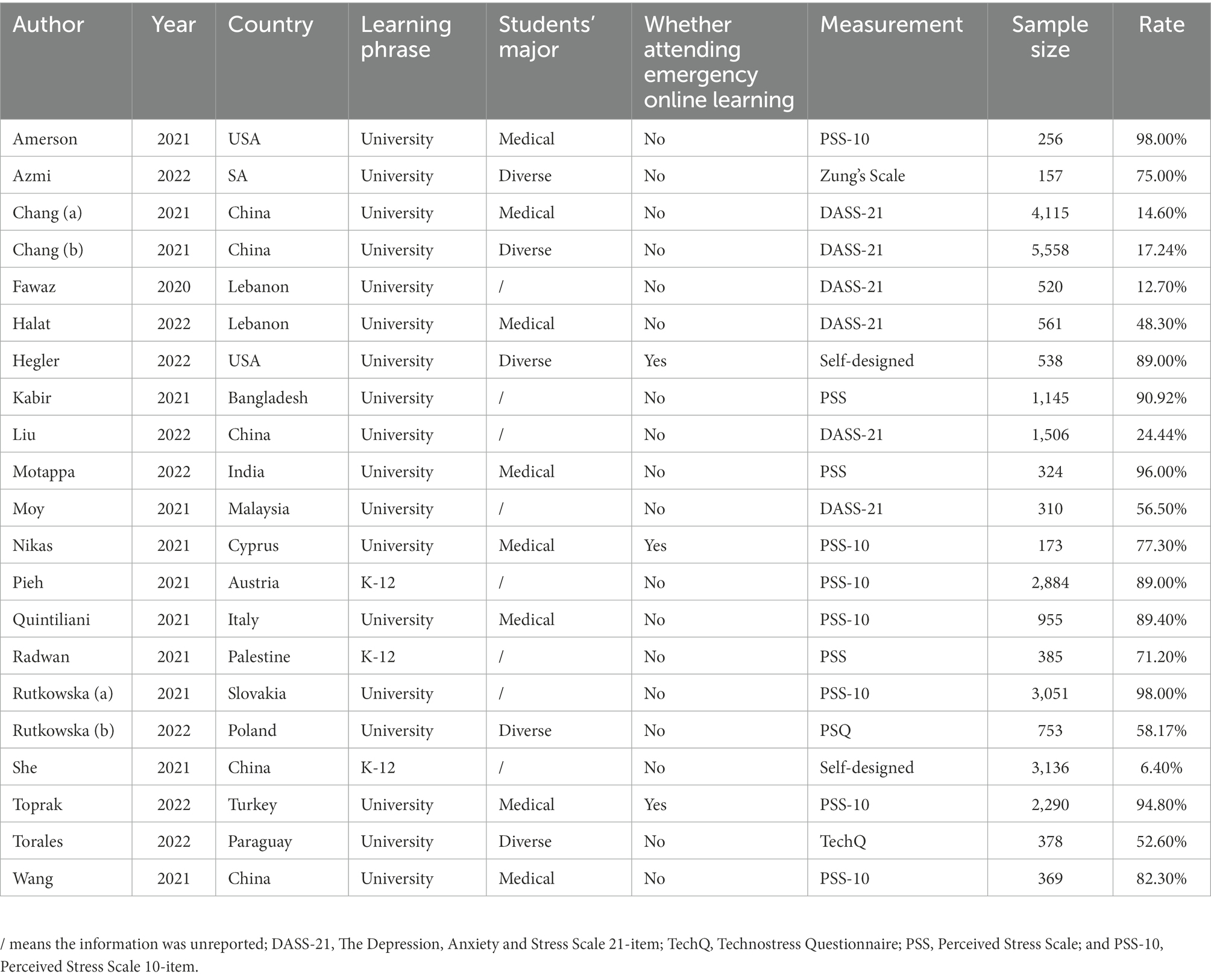
Table 3. Summary coding of included stress studies (arranged in alphabetical order of the first author).
2.3. Statistical analyses
Testing publication bias, selecting a model, and processing data are indispensable to statistical analysis before conducting meta-analysis. In terms of publication bias, it indicates that studies with more significant findings are more likely to be published in a scientific journal, so it is a severe issue that can undermine the reliability and validity of results in a meta-analysis (Begg and Berlin, 1988; Lin and Chu, 2017). One method to test publication bias is observing the asymmetry of the funnel plot. However, due to the lack of precision, multiple statistical tests have been put forward to examine publication bias accurately, such as Egger’s regression test (Egger et al., 1997) and Begg’s rank test (Begg and Mazumdar, 1994). If publication bias is detected, methods can be applied to correct bias, including the trim-and-fill method (Duval and Tweedie, 2000) or Rosenthal’s failsafe N (Rosenthal, 1979). In this study, Egger’s regression test was chosen to be the method applied to assess whether publication bias exists in this meta-analysis.
As regards model selection, two statistical models have been modified in the meta-analysis: the fixed effects model and the random effects model (Hedges and Vevea, 1998). The former fixed effects model treats the effect size parameters as fixed but unknown constants to be estimated, while the latter random effects model treats the effect size parameters as though they were a random sample from the population of effect parameters (Rosenthal and Rubin, 1982; DerSimonian and Laird, 1986). To decide which model (fixed effects model vs. random effects model) should be applied, measuring heterogeneity through the Q test and I2 confidence interval (CI) are crucial evaluation criteria. If the Q test shows significant or I2 results higher than 75%, it indicates that high heterogeneity exists, and a random effects model should be selected; otherwise, a fixed effects model should be chosen (Huedo-Medina et al., 2006).
This meta-analysis was processed and performed using the Comprehensive Meta-Analysis (CMA) software. To aggregate the overall prevalence rate, the software first transformed input ratio data into logit data through the formula , and then, converted logit data back to ratio data by the formula (Card, 2012). There were two ways to analyze moderating effect in this meta-analysis: (1) When moderating variables were continuous, meta-regression was used to test whether the results were significant and (2) when moderating variables were categorical; the subgroup analysis was used to test whether the results were significant. In the subgroup analysis, to ensure the representativeness of the studies under certain moderating variables, the number of effect sizes under the same moderating variable should be no less than 3 (Zhang et al., 2021).
3. Results
The results of the study are presented in the following subsections. The Results section starts with description of the characteristic of the study sample, followed by an investigation and measurement of heterogeneity and publication bias. The Results section ends in displaying the combined effect, sensitivity, and moderator analysis of the present meta-analysis.
3.1. Study sample characteristics
Overall, 60 total effect sizes from 36 different studies were extracted, and 78,674 participants were included in this meta-analysis. Specifically, anxiety included 22 studies and 64,072 participants, generating 22 effect sizes; depression comprised 17 studies and 22,618 participants, yielding 17 effect sizes; stress incorporated 21 studies and 29,364 participants, generating 21 effect sizes. These 36 studies were conducted from 19 nations (i.e., Austria, Bangladesh, China, Cyprus, India, Italy, Kazakhstan, Lebanon, Lithuania, Malaysia, Palestine, Paraguay, Poland, Portugal, Saudi Arabia, Slovakia, Turkey, United Arab Emirates, and the United States of America), covering both developed and developing countries from Asia, Europe, North America, and South America.
3.2. Investigating heterogeneity and publication bias
Investigating heterogeneity is the critical step to judge whether the fixed or random effects model should be selected in this meta-analysis. Table 4 shows that all Q tests were significant, and I2 results exceeded 75% in anxiety (p = 0.000, I2 = 99.784), depression (p = 0.000, I2 = 98.955), and stress (p = 0.000, I2 = 99.797) studies, indicating that the random effects model should be chosen as the statistical model in this study (Huedo-Medina et al., 2006).
In addition, Egger’s regression test is indispensable for examining the publication bias in this study. As shown in Table 4, the value of p showed significance in anxiety (p < 0.001), depression (p = 0.04), and stress (p = 0.04), with publication bias manifesting in any of the three clinical symptoms. Therefore, the trim-and-fill method, one of the most popular means, was opted for correcting publication bias (Shi and Lin, 2019). Trim-and-fill analysis was completed in the software Stata. The analysis showed that “no trimming performed; data unchanged,” indicating that the results were robust and that no literature were needed to be added to the present study. The funnel plots assessing publication bias of anxiety, depression, and stress are shown in Figures 2–4 in sequence.
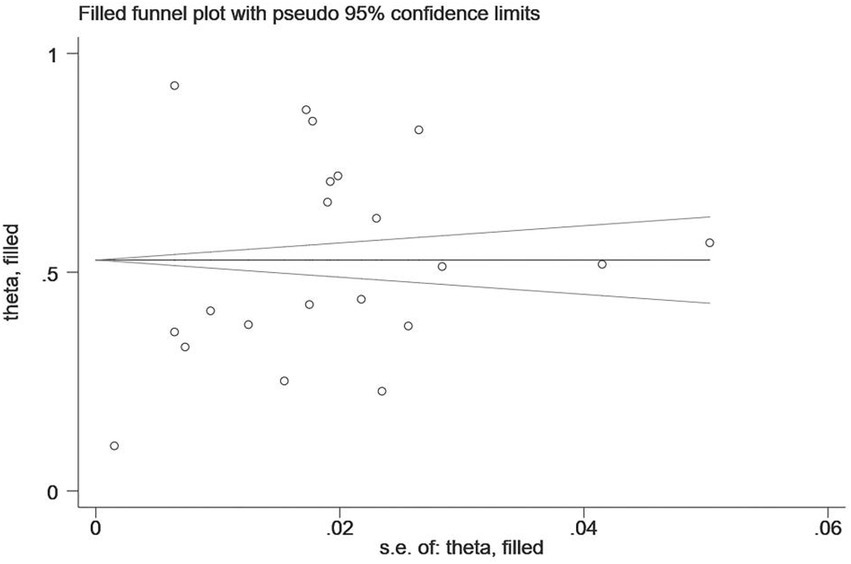
Figure 2. A funnel plot assessing publication bias of the prevalence of anxiety among remote learning students during the COVID-19 pandemic.
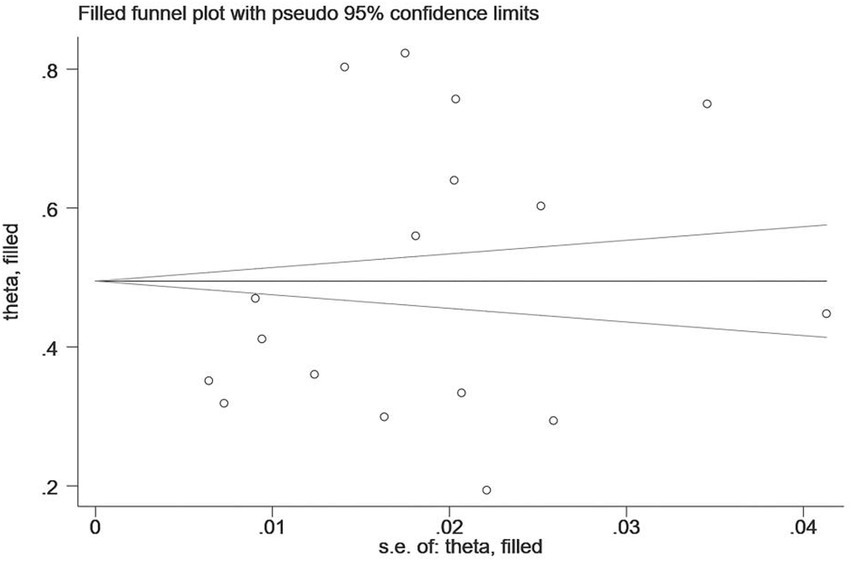
Figure 3. A funnel plot assessing publication bias of the prevalence of depression among remote learning students during the COVID-19 pandemic.
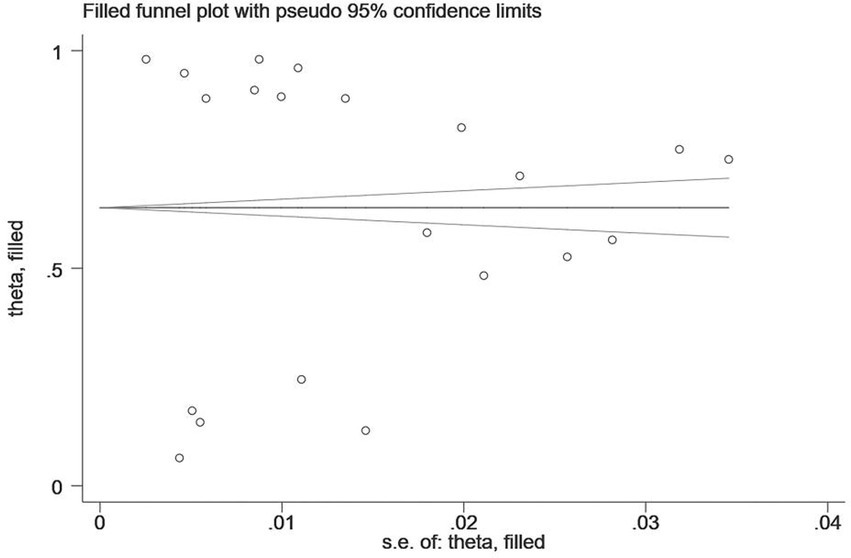
Figure 4. A funnel plot assessing publication bias of the prevalence of stress among remote learning students during the COVID-19 pandemic.
3.3. Combined effect, sensitivity, and moderator analysis
Based on the random effects model, the prevalence of the mental problem is shown in Table 5: (1) the prevalence of anxiety among remote learning students during the COVID-19 pandemic was 58% [95% confidence interval (CI) = [0.45, 0.70]]; (2) the prevalence of depression among remote learning students during the COVID-19 pandemic was 50% (95% CI = [0.43, 0.57]); and (3) the prevalence of stress among remote learning students during the COVID-19 pandemic was 71% (95% CI = [0.52, 0.84]).
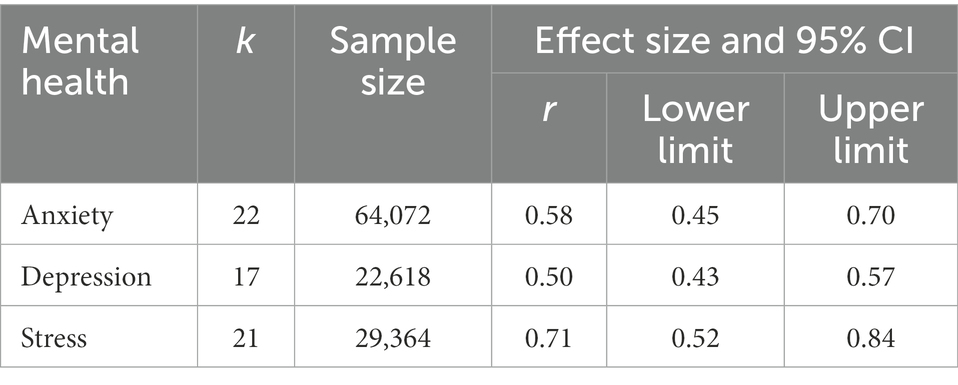
Table 5. The prevalence of anxiety, depression, and stress among remote learning students during the COVID-19 pandemic.
Sensitivity analysis was also applied to examine the robustness of the results. After a random individual study was removed from the input data, the prevalence of anxiety fluctuated between 54.0 and 60.0%, depression between 47.4 and 51.9%, and stress between 69.5 and 73.3%. There is little difference between the finding before and after sensitivity analysis, which indicates that the results are robust and stable.
In terms of moderator analysis, several variables were set as moderator types to assess whether a third variable causes a significant difference. In terms of anxiety (see Table 6), the results showed that (1) the moderating effect of publication year was nonsignificant (b = −0.13, 95% CI = [−0.75, 0.50]), which indicates that the prevalence of anxiety hardly changed with time lapse; (2) the moderating effect of whether attending emergency online learning (p = 0.09), development level (p = 0.63), and students’ major (p = 0.92) were all not significant; and (3) the moderating effect of educational level (p = 0.03) was significant. Prevalence of the anxiety of university students (63%) was more than two times that of the k-12 students (29%).
As regards depression (see Table 7), the results showed that (1) the moderating effect of publication year was non-significant (b = −0.13, 95% CI = [−0.75, 0.49]), which also indicates that the prevalence of depression hardly changed over time; (2) the moderating effect of development level (p = 0.40) and students’ major (p = 0.92) were both not significant; and (3) the moderating effect of educational level (p = 0.04) was significant. The prevalence of depression in university students (53%) was much higher than that of k-12 students (34%).
For the third mental health problem, stress (see Table 8), the results showed that (1) the moderating effect of publication year was not significant (b = 0.63, 95% CI = [−0.80, 2.06]), which also indicates that the prevalence of stress scarcely variates with time; (2) the moderating effect of educational level (p = 0.59) and students’ major (p = 0.25) was both not significant; and (3) the moderating effect of whether attending emergency online learning (p = 0.03) and development level (p = 0.00) was significant. The stress prevalence of students under emergency online learning contexts (89%) was much higher than that of students under traditional online learning contexts (67%). In addition, the stress prevalence of students in a developed country (93%) was more than 1.5 times higher than their counterparts in a developing country (56%).
4. Discussion
Based on the above findings, we have discussed below the possible explanation of these outcomes (the combined and moderating effect of anxiety, depression, and stress prevalence), combining it with related prior literature. In addition, limitations are discussed for guiding follow-up studies in the future.
4.1. Prevalence of anxiety, depression, and stress
The present study is the first meta-analysis on the prevalence of anxiety, depression, and stress among remote learning students during the COVID-19 pandemic. It synthesized the evidence on the empirical studies associated with online learning and mental health problems, such as anxiety, depression, and stress during the COVID-19 pandemic period. After a rigorous literature search and screening, a total of 36 studies with 78,674 participants from 19 nations were included and generated 60 effect sizes (22 for anxiety, 17 for depression, and 21 for stress). This meta-analysis showed that the prevalence of anxiety, depression, and stress among remote learning students during the COVID-19 pandemic was high, 58, 50, and 71%, respectively.
Comparing the current result with previous analogous meta-analysis, one study found that the prevalence of anxiety, depression, and stress among remote learning students during the COVID-19 pandemic is significantly higher than that of the general population during the pandemic, students during the pandemic, and students before the pandemic. More specifically, Salari et al. (2020) found that the general population’s prevalence of anxiety, depression, and stress during the COVID-19 pandemic was 31.9, 33.7, and 29.6% via meta-analysis. In addition, Wang et al. (2021) conducted a meta-analysis study and concluded that the prevalence of anxiety, depression, and stress among general college students during the pandemic period was 29, 37, and 23%. As regards the meta-analysis before the outbreak of COVID-19, the prevalence of stress, anxiety, and depression among students was 32.9, 30.6, and 49.9%, respectively (Pacheco et al., 2017). All these meta-analyses indicate that many more remote learning students suffer from anxiety, depression, and stress than the general population and students during and before pandemic.
Based on the mentioned data comparison, student groups, online learning, and the COVID-19 pandemic are three factors that lead to a higher prevalence of anxiety, depression, and stress. First of all, children and young people nowadays are facing unprecedented challenges that are unacquainted with previous generations’ cognition, and it is exactly the time in one’s life that confronts the peak risk of suffering from mental health problems (Claveirole and Gaughan, 2011; Cunningham and Duffy, 2019). According to a study conducted by the American College Health Association (2014) based on 79,266 participants, students reported feeling anxious, depressed, hopeless, psychologically exhausted, and even considered suicide and self-harm. The stressors they are exposed to are not limited to academic pressure but social, financial, and lifestyle factors (Cunningham and Duffy, 2019). Therefore, the current result conforms to the previous finding that students are more vulnerable and prone to mental illness. Second, using technology may also be detrimental to mental health, though electronic devices have long been applied as everyday tools for people from all walks of life including students (Zeeni et al., 2018). Computer-related anxiety is not an up-to-date concept but was put forward decades ago, which refers to a non-rational anticipation of dread brought on by the notion of using the computer (Brosnan, 1998). It further derives from another concept, internet-related anxiety, including four aspects of specific anxiety caused by the internet: (1) internet terminology anxiety, (2) net search anxiety, (3) internet time delay anxiety, and (4) a general fear of Internet failure (Presno, 1998). Under the learning context, students who took online lessons reported anxiety, especially at the beginning of the courses (DeVaney, 2010). Apart from anxiety, stress is another mental health problem that resulted from the use of technology. Utilizing new information-communication-technologies (ICTs), such as laptops, mobile phones, and virtual education, can result in stress, which is referred to as “technostress” in modern parlance (Torales et al., 2022). Some researchers found that the most common technostress consequences are anxiety and depression (Chiappetta, 2017; Lin et al., 2017). Hence, it is understandable that online learning can trigger negative emotions, which is consistent with previous research (Zembylas, 2008). Last but not least, the COVID-19 pandemic is a non-negligible element that has exacerbated mental health problems, especially for students and young people (Meda et al., 2021; Kauhanen et al., 2022). A systematic review conducted by Meda et al. (2021) found that 87% of previous studies showed increased anxiety, depression, and mental distress among children and young people after the outbreak of the COVID-19 pandemic. Potential explanations for this phenomenon are numerous, including but not limited to remote learning (Almeida et al., 2021), increased social media use (Ellis et al., 2020), more stringent COVID-19 policies (Aknin et al., 2022), social isolation, worries about being infected, and restrained leisure activities (Meda et al., 2021). Thus, taking present and previous studies into consideration, student groups, online learning, and the COVID-19 pandemic are three factors that have given rise to the prevalence of anxiety, depression, and stress.
4.2. Moderating factors of anxiety, depression, and stress
In the moderator analysis, several variables are set as moderators in the current meta-analysis, including publication year, whether attending emergency online learning, educational level, development level, and students’ major. It is the first study to explore the moderating effect of study publication year on the prevalence of anxiety, depression, and stress among remote learning students during the COVID-19 pandemic. This meta-analysis concluded that the moderating effect of publication year was not significant, which also indicates that the prevalence of anxiety, depression, and stress scarcely variates with time after the outbreak of the COVID-19 pandemic. The result shows a different trend from that before the pandemic. Before the start of the COVID-19 pandemic, studies found that the level of anxiety and depression among adolescents increased substantially from 1997 to 2017 in China and from 2011 to 2018 in America (Twenge, 2020; Xin et al., 2020). The same result was also shown in the study focused on university students, which found that the rate of depression among American college students also increased from 2007 to 2017 (Lipson et al., 2019). The reason behind such difference may be hidden by the fact that the time duration after the pandemic is merely 3 years (from the end of 2019 until now), which does not have a prolonged chronological root as previous studies.
Whether attending emergency online learning is also an innovative variable studied in this meta-analysis. The result showed that the moderating effect of whether attending emergency online learning was significant for the prevalence of stress (the stress prevalence of students under the emergency online learning context was significantly higher than that of students under the traditional online learning context), but we failed to find the moderating effect in the prevalence of anxiety and depression among online learning students during the COVID-19 pandemic. Compared to traditional online education, the emergency pattern is usually a temporary shift to another delivery mode due to crisis circumstances (Ferri et al., 2020; Hodges et al., 2020; Fuchs, 2022). For this reason, emergency online teaching confronts various distinct challenges and obstacles from traditional online study, including restrained planning and preparation time, lack of faculty professional training, and unfamiliar access to a technological support system (O’Keefe et al., 2020). Thus, emergency remote education could exert a series of negative effects, such as anxiety, depression, distress, fear, and worry (El-Sakran et al., 2022). In the present study, the significant difference between traditional and emergency online learning in students’ perception of stress was identified on comparison with previous studies. However, the reason for showing no difference in online students’ perception of anxiety and depression may be aroused by the insufficient sample of related studies.
Educational level is the third moderator variable in this meta-analysis. The result showed that the moderating effect of the educational level was significant for the prevalence of anxiety and depression among e-learning students during the COVID-19 pandemic. The anxiety and depression prevalence of university students was significantly higher than that of the k-12 students. This result partly conforms to the finding in another meta-analysis, which found that people with a higher level of education experienced a higher level of anxiety, depression, and stress (Salari et al., 2020). There are two potential explanations. On the one hand, people with a higher educational level possess higher self-awareness in terms of their health (Zhang and Ma, 2020). On the other hand, the stressors confronting people with different educational levels are disparate. University students are exposed not only to academic pressure but more importantly to prolonged unemployment, along with financial insecurity and undermining self-esteem, which are all contributors to the increased rate of mental health problems such as anxiety and depression (Islam et al., 2020), which should not be taken into consideration for k-12 students. Nevertheless, no significant difference showed between tertiary and elementary students in the prevalence of stress during the COVID-19 pandemic online learning. A possible reason for the difference of the present study from the previous study is that the subjects of the present study are remote learning students while the subject in the other study is the general population (Salari et al., 2020).
As regards the development level, the result showed that the moderating effect of development level was significant for the prevalence of stress among e-learning students during the COVID-19 pandemic. The stress prevalence of students in a developed country was more than 1.5 times higher than their counterparts in a developing country. The current result is exactly opposite to the finding among the general population, which found that people in underdeveloped and developing countries suffer from more psychological problems (Salari et al., 2020). It is primarily because underdeveloped nations are associated with poorer treatment conditions, community inefficiency, and many other infectious diseases (Moghanibashi-Mansourieh, 2020; Salari et al., 2020; Sigdel et al., 2020). However, narrowing down the participants to students only, similar findings have been reported in China, which found the prevalence of sleep problems and suicide attempts among university students in eastern China (a relatively developed region) is higher than their counterparts in central and northeast of China (a relative developing region; Chen et al., 2022). A possible explanation may have originated from the actuality that students suffer from greater peer pressure in places with rich educational and economic resources due to better enrollment quality and more intense social competition (Liu et al., 2019; Chen et al., 2022). Hence, the prevalence of stress for distance learning students in developed countries was found to be higher than their counterparts in developing countries.
The last moderator variable in this meta-analysis is students’ major, which shows no significant difference between students in medical and other diverse majors in the prevalence rate of anxiety, depression, and stress during the COVID-19 online study period. This result is in discordance with the previous study. Reviewing preceding research comparing mental health between medical and non-medical students, there are two contrary findings before and after pandemic. Before the outbreak of the COVID-19 pandemic, more medical and non-medical students reported worse mental health status due to perceiving greater academic pressure (Al-Dabal et al., 2010). However, the result reversed after the spread of COVID-19 since medical students showed fewer mental health symptoms such as anxiety, depression, and stress than non-medical students, because they gained richer medical knowledge and had a higher sense of awareness (Xiong et al., 2021). Some scholars assumed that online study posed numerous challenges to medical education, since hands-on experience is of vital importance for medical students (Patra et al., 2021; Nikas et al., 2022), whereas the result of this meta-analysis did not indicate lacking hands-on experience leads to more mental problems among medical students. It is possibly because greater academic stress and richer medical professional knowledge cancel out, so there is no higher prevalence level of anxiety, depression, and stress among online medical students during the pandemic period.
4.3. Limitations and future study
Despite this study being a pioneering work exploring the prevalence of anxiety, depression, and stress among remote learning students during the COVID-19 pandemic, several limitations exist in the present meta-analysis. First, the number of studies on emergency remote learning is insufficient: only three for anxiety and stress and one for depression, which leads to the moderating effect of whether attending emergency remote learning on the prevalence of depression cannot be examined. Therefore, more searches under emergency remote learning can be collected to be further analyzed in the future. Second, there are only two categories for students’ major variables: medical and diverse. Students in different majors confront their own specific challenges that other disciplines cannot notice. Thus, scholars should also pay attention to students’ mental health in other majors, such as business, literature, art, and science. Third, only published articles were collected in this study. It is relatively difficult to avoid publication bias in meta-analysis since it is a secondary analysis based on abundant original literature. However, including unpublished articles could lower bias. Fourth, the current research is yet to cover all types of mental health problems. Only anxiety, depression, and stress were regarded as representative indicators reflecting remote learning students’ mental status; therefore, many psychological disorders, such as distress and self-injury, can catch attention in the future. Fifth, all original studies in the current meta-analysis were periodic, which could only reflect participants’ mental health over a period of time. However, psychological status changes as time goes on. Therefore, future follow-up studies could be conducted to explore the overall psychological impact of the COVID-19 pandemic on students in multiple disciplines over a more forward-looking period.
5. Conclusion
Over the past 3 years, the COVID-19 pandemic has triggered psychological problems afflicting people worldwide. This study conducted a meta-analysis based on 36 original articles and 78,674 participants across 19 nations and found that the prevalence of anxiety, depression, and stress among remote learning students during the COVID-19 pandemic was as high as 58, 50, and 71%, respectively. The prevalence rate of anxiety and depression among students in higher education was significantly higher than that of students in elementary education. Besides, significantly more medical students and students in emergency remote learning contexts suffer from mental stress than their non-medical and traditional distance learning counterparts.
Therefore, instructors, schools, and governments should take notice of the current situation and figure out ways to collaboratively help online learning students relieve anxiety, depression, and stress against the backdrop of the COVID-19 pandemic. Instructors, as the group with the closest connections to students, could alleviate students’ negative emotions by designing flexible coursework and test-taking, having organized online courses, and showing care about students’ mental health (Mohammed et al., 2021; Busch et al., 2022). From the schools’ perspective, professional programs for reducing students’ mental disorders, such as anxiety, depression, and stress, could be implemented. Besides, as a determining factor in students’ experience, the schools should shoulder the responsibility to provide school counseling services for faculty, students, and their family members (Karaman et al., 2021). In terms of governments, more macroscopic measurements can be implemented, including enhancing digital infrastructure and enriching e-content and e-resources, especially for students in less developed areas (Adnan and Anwar, 2020). Under the joint effort of instructors, schools, and governments, it is assumed that the symptoms of anxiety, depression, and stress can be relieved among remote learning students.
Data availability statement
The raw data supporting the conclusions of this article will be made available by the authors, without undue reservation.
Author contributions
TX is responsible for putting forward ideas, collecting and analyzing data, and drafting the paper. HW is responsible for supervising the whole process and revising the paper. All authors contributed to the article and approved the submitted version.
Conflict of interest
The authors declare that the research was conducted in the absence of any commercial or financial relationships that could be construed as a potential conflict of interest.
Publisher’s note
All claims expressed in this article are solely those of the authors and do not necessarily represent those of their affiliated organizations, or those of the publisher, the editors and the reviewers. Any product that may be evaluated in this article, or claim that may be made by its manufacturer, is not guaranteed or endorsed by the publisher.
References
Adnan, M., and Anwar, K. (2020). Online learning amid the COVID-19 pandemic: students perspectives. J. Pedagog. Res. 1, 45–51. doi: 10.33902/jpsp.2020261309
Aknin, L., Andretti, B., Goldszmidt, R., Helliwell, J., Petherick, A., De Neve, J., et al. (2022). Policy stringency and mental health during the COVID-19 pandemic: a longitudinal analysis of data from 15 countries. Lancet Public Health 7, e417–e426. doi: 10.1016/S2468-2667(22)00060-3
Al-Dabal, B. K., Koura, M. R., Rasheed, P., Al-Sowielem, L., and Makki, S. M. (2010). A comparative study of perceived stress among female medical and non-medical university students in Dammam, Saudi Arabia. Sultan Qaboos Univ. Med. J. 10, 231–240.
Almeida, M., Challa, M., Ribeiro, M., Harrison, A. M., and Castro, M. C. (2021). Editorial perspective: the mental health impact of school closures during the COVID-19 pandemic. J. Child Psychol. Psychiatry 63, 608–612. doi: 10.1111/jcpp.13535
American College Health Association (2014). Reference group executive summary, Spring 2014. Available at: http://www.Acha-Ncha.org/Docs/ACHA-NCHA-II_ReferenceGroup_ExecutiveSummary_Spring2014.pdf
Begg, C. B., and Berlin, J. A. (1988). Publication bias: a problem in interpreting medical data. J. Roy. Statis. Soc. Ser. A. 151, 419–463. doi: 10.2307/2982993
Begg, C. B., and Mazumdar, M. (1994). Operating characteristics of a rank correlation test for publication bias. Biometrics 50, 1088–11101. doi: 10.2307/2533446
Brosnan, M. J. (1998). Technophobia: The Psychological Impact of Information Technology. London:Routledge.
Busch, C. A., Mohammed, T. F., Nadile, E. M., and Cooper, K. M. (2022). Aspects of online college science courses that alleviate and exacerbate undergraduate depression. PLoS One 17:e0269201. doi: 10.1371/journal.pone.0269201
Chang, W., Shi, L., Zhang, L., Jin, Y., and Yu, J. (2021). The mental health status and associated factors among medical students engaged in online learning at home during the pandemic: a cross-sectional study from China. Front. Psychol. 12:755503. doi: 10.3389/fpsyt.2021.755503
Chen, Y., Zhang, Y., and Yu, G. (2022). Prevalence of mental health problems among college students in mainland China from 2010 to 2020: a meta-analysis. Adv. Psychol. Sci. 30, 991–1004. doi: 10.3724/sp.j.1042.2022.00991
Chiappetta, M. (2017). The technostress: definition, symptoms and risk prevention. Sens. Sci. 4, 358–361. doi: 10.14616/sands-2017-1-358361
Claveirole, A., and Gaughan, M. (2011). Understanding Children and Young People’s Mental Health. Chichester, West Sussex, Angleterre: John Wiley & Sons.
Cunningham, S., and Duffy, A. (2019). Investing in our future: importance of postsecondary student mental Health Research. Can. J. Psychiatr. 64, 79–81. doi: 10.1177/0706743718819491
DerSimonian, R., and Laird, N. (1986). Meta-analysis in clinical trials. Control. Clin. Trials 7, 177–188. doi: 10.1016/0197-2456(86)90046-2
DeVaney, T. A. (2010). Anxiety and attitude of graduate students in on-campus vs. online statistics courses. J. Stat. Educ. 18, 1–15. doi: 10.1080/10691898.2010.11889472
Dutta, J., Goswami, S., and Mitra, A. (2020). “Introduction to coronaviruses and COVID-19,” in COVID-19 and Emerging Environmental Trends eds. ByJoystu Dutta, Srijan Goswami, Abhijit Mitra (Boca Raton, Fl: CRC Press).
Duval, S., and Tweedie, R. (2000). Trim and fill: a simple funnel-plot-based method of testing and adjusting for publication bias in meta-analysis. Biometrics 56, 455–463. doi: 10.1111/j.0006-341x.2000.00455.x
Egger, M., Smith, G. D., Schneider, M., and Minder, C. (1997). Bias in meta-analysis detected by a simple, graphical test. BMJ 315, 629–634. doi: 10.1136/bmj.315.7109.629
Ellis, W. E., Dumas, T. M., and Forbes, L. M. (2020). Physically isolated but socially connected: psychological adjustment and stress among adolescents during the initial COVID-19 crisis. Can. J. Behav. Sci. 52, 177–187. doi: 10.1037/cbs0000215
El-Sakran, A., Salman, R., and Alzaatreh, A. (2022). Impacts of emergency remote teaching on college students amid COVID-19 in the UAE. Int. J. Environ. Res. Public Health 19:2979. doi: 10.3390/ijerph19052979
Ferri, F., Grifoni, P., and Guzzo, T. (2020). Online learning and emergency remote teaching: opportunities and challenges in emergency situations. For. Soc. 10:86. doi: 10.3390/soc10040086
Fuchs, K. (2022). The difference between emergency remote teaching and e-learning. Front. Educ. 7:921332. doi: 10.3389/feduc.2022.921332
Hedges, L. V., and Vevea, J. L. (1998). Fixed- and random-effects models in meta-analysis. Psychol. Methods 3, 486–504. doi: 10.1037/1082-989x.3.4.486
Hodges, C., Moore, S., Lockee, B., Trust, T., and Bond, A. (2020). The difference between emergency remote teaching and online learning. Er.educause.edu. Available at: https://er.educause.edu/articles/2020/3/the-difference-between-emergency-remote-teaching-and-online-learning
Huedo-Medina, T. B., Sánchez-Meca, J., Marín-Martínez, F., and Botella, J. (2006). Assessing heterogeneity in meta-analysis: Q statistic or I2 index? Psychol. Methods 11, 193–206. doi: 10.1037/1082-989x.11.2.193
Islam, M. A., Barna, S. D., Raihan, H., Khan, M. N. A., and Hossain, M. T. (2020). Depression and anxiety among university students during the COVID-19 pandemic in Bangladesh: a web-based cross-sectional survey. PLoS One 15:e0238162. doi: 10.1371/journal.pone.0238162
Karaman, M. A., Eşici, H., Tomar, İ. H., and Aliyev, R. (2021). COVID-19: are school counseling services ready? Students’ psychological symptoms, school counselors’ views, and solutions. Front. Psychol. 12:647740. doi: 10.3389/fpsyg.2021.647740
Kauhanen, L., Wan Mohd Yunus, W. M. A., Lempinen, L., Peltonen, K., Gyllenberg, D., Mishina, K., et al. (2022). A systematic review of the mental health changes of children and young people before and during the COVID-19 pandemic. Eur. Child Adolesc. Psychiatry 1–19. doi: 10.1007/s00787-022-02060-0
Kotrikadze, E. V., and Zharkova, L. I. (2021). Advantages and disadvantages of distance learning in universities. Propósit. Represent. 9, 1–7. doi: 10.20511/pyr2021.v9nspe3.1184
Lin, L., and Chu, H. (2017). Quantifying publication bias in meta-analysis. Biometrics 74, 785–794. doi: 10.1111/biom.12817
Lin, J. S., Lee, Y. I., Jin, Y., and Gilbreath, B. (2017). Personality traits, motivations, and emotional consequences of social personality traits, motivations, and emotional consequences of social media usage. Cyberpsychol. Behav. Soc. Netw. 20, 615–623. doi: 10.1089/cyber.2017.0043
Lipson, S. K., Lattie, E. G., and Eisenberg, D. (2019). Increased rates of mental health service utilization by U.S. college students: 10-year population-level trends (2007–2017). Psychiatr. Serv. 70, 60–63. doi: 10.1176/appi.ps.201800332
Liu, J., Lin, S., Wang, Y., and Ma, L. (2019). A multidimensional comparative analysis of the academic performance of college students coming from Western China: an empirical study based on students from N college of B university. Chongqing High. Educ. Res. 7, 12–29. doi: 10.15998/j.cnki.issn1673-8012.2019.04.002
Meda, N., Pardini, S., Slongo, I., Bodini, L., Zordan, M. A., Rigobello, P., et al. (2021). Students’ mental health problems before, during, and after COVID-19 lockdown in Italy. J. Psychiatr. Res. 134, 69–77. doi: 10.1016/j.jpsychires.2020.12.045
Moghanibashi-Mansourieh, A. (2020). Assessing the anxiety level of Iranian general population during COVID-19 outbreak. Asian J. Psychiatr. 51:102076. doi: 10.1016/j.ajp.2020.102076
Mohammed, T. F., Nadile, E. M., Busch, C. A., Brister, D., Brownell, S. E., Claiborne, C. T., et al. (2021). Aspects of large-enrollment online college science courses that exacerbate and alleviate student anxiety. CBE Life Sci. Educ. 69, 1–23. doi: 10.1187/cbe.21-05-0132
Nikas, I. P., Lamnisos, D., Meletiou-Mavrotheris, M., Themistocleous, S. C., Pieridi, C., Mytilinaios, D. G., et al. (2022). Shift to emergency remote preclinical medical education amidst the Covid-19 pandemic: a single-institution study. Anat. Sci. Educ. 15, 27–41. doi: 10.1002/ase.2159
O’Keefe, L., Rafferty, J., Gunder, A., and Vignare, K. (2020). Delivering high-quality instruction online in response to COVID-19 faculty playbook faculty playbook. Available at: http://olc-wordpress-assets.s3.amazonaws.com/uploads/2020/05/Faculty-Playbook_Final-1.pdf
Pacheco, J. P., Giacomin, H. T., Tam, W. W., Ribeiro, T. B., Arab, C., Bezerra, I. M., et al. (2017). Mental health problems among medical students in Brazil: a systematic review and meta-analysis. Brazi. J. Psychiatry 39, 369–378. doi: 10.1590/1516-4446-2017-2223
Patra, A., Chaudhary, P., and Ravi, K. S. (2021). Adverse impact of Covid-19 on anatomical sciences teachers of India and proposed ways to handle this predicament. Anat. Sci. Educ. 14, 163–165. doi: 10.1002/ase.2052
Presno, C. (1998). Taking the byte out of internet anxiety: instructional techniques that reduce computer/internet anxiety in the classroom. J. Educ. Comput. Res. 18, 147–161. doi: 10.2190/uy72-5tg8-0lt5-au4l
Rosenthal, R. (1979). The file drawer problem and tolerance for null results. Psychol. Bull. 86, 638–641. doi: 10.1037/0033-2909.86.3.638
Rosenthal, R., and Rubin, D. B. (1982). Comparing effect sizes of independent studies. Psychol. Bull. 92, 500–504. doi: 10.1037/0033-2909.92.2.500
Rutkowska, A., Cieślik, B., Tomaszczyk, A., and Szczepańska-Gieracha, J. (2022). Mental health conditions among E-learning students during the COVID-19 pandemic. Front. Public Health 10:871934. doi: 10.3389/fpubh.2022.871934
Salari, N., Hosseinian-Far, A., Jalali, R., Vaisi-Raygani, A., Rasoulpoor, S., Mohammadi, M., et al. (2020). Prevalence of stress, anxiety, depression among the general population during the COVID-19 pandemic: a systematic review and meta-analysis. Glob. Health 16, 57–61. doi: 10.1186/s12992-020-00589-w
Shi, L., and Lin, L. (2019). The trim-and-fill method for publication bias. Medicine 98:e15987. doi: 10.1097/md.0000000000015987
Sigdel, A., Bista, A., Bhattarai, N., Poon, B., Giri, G., and Marqusee, H. (2020). Depression, anxiety and depression-anxiety comorbidity amid COVID-19 pandemic: an online survey conducted during lockdown in Nepal. MedRxiv [Preprint]. doi: 10.1101/2020.04.30.20086926
Torales, J., Torres-Romero, A. D., Di Giuseppe, M. F., Rolón-Méndez, E. R., Martínez-López, P. L., Heinichen-Mansfeld, K. V., et al. (2022). Technostress, anxiety, and depression among university students: a report from Paraguay. Int. J. Soc. Psychiatry 68, 1063–1070. doi: 10.1177/00207640221099416
Tunc, S., and Toprak, M. (2022). COVID-19 pandemic and emergency remote education practices: effects on dentistry students. Niger. J. Clin. Pract. 25, 621–629. doi: 10.4103/njcp.njcp_1564_21
Twenge, J. M. (2020). Why increases in adolescent depression may be linked to the technological environment. Curr. Opin. Psychol. 32, 89–94. doi: 10.1016/j.copsyc.2019.06.036
Wang, C., Wen, W., Zhang, H., Ni, J., Jiang, J., Cheng, Y., Zhou, M., Ye, L., Feng, Z., Ge, Z., Luo, H., Wang, M., Zhang, X., and Liu, W. (2021). Anxiety, depression, and stress prevalence among college students during the COVID-19 pandemic: a systematic review and meta-analysis. J. Am. Coll. Heal. 1–8. doi: 10.1080/07448481.2021.1960849 (Epub ahead of print).
Warner, D. O., Nolan, M., Garcia-Marcinkiewicz, A., Schultz, C., Warner, M. A., Schroeder, D. R., et al. (2020). Adaptive instruction and learner interactivity in online learning: a randomized trial. Adv. Health Sci. Educ. 25, 95–109. doi: 10.1007/s10459-019-09907-3
WHO (2020). WHO director-General’s opening remarks at the Mission briefing on COVID-19. Available at: Https://Www.who.int/Dg/Speeches/Detail/Who-Director-General-s-Opening-Remarks-At-The-Mission-Briefing-On-Covid-19
WHO (2022). Learning never stops—Tell UNESCO how you are coping with COVID-19 school closures |UNESCO. Available at: www.unesco.org; https://www.unesco.org/en/articles/learning-never-stops-tell-unesco-how-you-are-coping-covid-19-school-closures
Xin, S., Wang, Y., and Sheng, L. (2020). Impact of social changes and birth cohort on anxiety in adolescents in mainland China (1992–2017): a cross-temporal meta-analysis. Child Youth Serv. Rev. 116:105159. doi: 10.1016/j.childyouth.2020.105159
Xiong, P., Ming, W., Zhang, C., Bai, J., Luo, C., Cao, W., et al. (2021). Factors influencing mental health among chinese medical and non-medical students in the early stage of the COVID-19 pandemic. Front. Public Health 9:603331. doi: 10.3389/fpubh.2021.603331
Zeeni, N., Doumit, R., Abi Kharma, J., and Sanchez-Ruiz, M.-J. (2018). Media, technology use, and attitudes: associations with physical and mental well-being in youth with implications for evidence-based practice. Worldviews Evid.-Based Nurs. 15, 304–312. doi: 10.1111/wvn.12298
Zembylas, M. (2008). Adult learners’ emotions in online learning. Dist. Educ. 29, 71–87. doi: 10.1080/01587910802004852
Zhang, C., Hao, J., Liu, Y., Cui, J., and Yu, H. (2022). Associations between online learning, smartphone addiction problems, and psychological symptoms in Chinese college students after the COVID-19 pandemic. Front. Public Health 10:881074. doi: 10.3389/fpubh.2022.881074
Zhang, Y., Li, S., and Yu, G. (2021). The relationship between social media use and fear of missing out: a meta-analysis. Acta Psychol. Sin. 53, 273–290. doi: 10.3724/SP.J.1041.2021.00273
Keywords: COVID-19, prevalence, mental health, anxiety, depression, stress, remote learning students, meta-analysis
Citation: Xu T and Wang H (2023) High prevalence of anxiety, depression, and stress among remote learning students during the COVID-19 pandemic: Evidence from a meta-analysis. Front. Psychol. 13:1103925. doi: 10.3389/fpsyg.2022.1103925
Edited by:
Barbara Masluk, University of Zaragoza, SpainReviewed by:
Brahmi Mohsen, University of Sfax, TunisiaXihui Chen, Zhejiang University of Technology, China
Copyright © 2023 Xu and Wang. This is an open-access article distributed under the terms of the Creative Commons Attribution License (CC BY). The use, distribution or reproduction in other forums is permitted, provided the original author(s) and the copyright owner(s) are credited and that the original publication in this journal is cited, in accordance with accepted academic practice. No use, distribution or reproduction is permitted which does not comply with these terms.
*Correspondence: Huang Wang, ✉ Z3did2FuZ2h1YW5nQGhuZm51LmVkdS5jbg==