- 1University of Denver, Denver, CO, United States
- 2University of North Texas, Denton, TX, United States
- 3University of Georgia, Athens, GA, United States
In creativity research, ideational flexibility, the ability to generate ideas by shifting between concepts, has long been the focus of investigation. However, psychometric work to develop measurement procedures for flexibility has generally lagged behind other creativity-relevant constructs such as fluency and originality. Here, we build from extant research to theoretically posit, and then empirically validate, a text-mining based method for measuring flexibility in verbal divergent thinking (DT) responses. The empirical validation of this method is accomplished in two studies. In the first study, we use the verbal form of the Torrance Test of Creative Thinking (TTCT) to demonstrate that our novel flexibility scoring method strongly and positively correlates with traditionally used TTCT flexibility scores. In the second study, we conduct a confirmatory factor analysis using the Alternate Uses Task to show reliability and construct validity of our text-mining based flexibility scoring. In addition, we also examine the relationship between personality facets and flexibility of ideas to provide criterion validity of our scoring methodology. Given the psychometric evidence presented here and the practicality of automated scores, we recommend adopting this new method which provides a less labor-intensive and less costly objective measurement of flexibility.
Introduction
For the last century, psychologists have emphasized the importance of cognitive flexibility in creative thinking. Flexibility of thought is a metacognitive process related to shifting in thinking and alternating between controlled and spontaneous cognitive processes (Yu et al., 2019; Preiss, 2022). Shifting between different processes is central to creative idea generation and has been compared to mindful mind wandering (Martindale, 1999; Preiss and Cosmelli, 2017; Murray et al., 2021). Flexibility does not just provide plasticity of cognition to allow for creative performance, but it also supports the consolidation of knowledge which affords one to achieve the highest potential of learning (Kroes and Fernández, 2012; Beckmann, 2014).
Creativity studies have provided empirical links between individuals’ creative outcomes and the flexibility of their thinking (Zabelina and Robinson, 2010; De Dreu et al., 2011; Kenett and Faust, 2019) showing that individuals who are capable of thinking in a flexible way may be more likely to produce highly creative ideas and products (Hass, 2017; Acar et al., 2019; Kenett and Faust, 2019; Zhang et al., 2020). Indeed, genuine scientific and artistic achievement has been associated with flexibility of cognition (Zabelina and Robinson, 2010; Zabelina et al., 2019; Griffith, 2022) and flexibility of the lexical network (Kenett et al., 2016, 2018; Cosgrove et al., 2021). Additionally, flexibility has also been used to differentiate between gifted and non-gifted children in performance areas such as writing, crafts, art, and public presentation (Shore, 2000; Keleş and Yazgan, 2022).
Research on flexibility also supports fundamental cognitive and developmental inferences, including the idea that exposure to novel and unexpected experiences through diverse life events may increase cognitive switching (Ritter et al., 2012; Chen et al., 2022). Indeed, openness to experiences has been found to be a good predictor of creativity (Feist, 1998; Abraham, 2018; Runco and Pritzker, 2020). This personality characteristic is a domain of the five-factor model of personality (McCrae and John, 1992; Goldberg, 1993; John et al., 2008) and is defined as curiosity, imaginativeness, creativity, trying new things, and unconventional thinking (John and Srivastava, 1999). The five factors of the model include openness (to experiences), conscientiousness, extraversion, agreeableness, and neuroticism. Costa and McCrae (1995) found that openness is positively associated with creativity while conscientiousness is negatively associated. Feist (1998) also found a similar effect using a meta-analysis of studies focusing on artists and scientists. Several other studies have confirmed the relationship between openness and extraversion with divergent thinking performance (Carson et al., 2003; Dumas et al., 2020a; Asquith et al., 2022).
Assessing flexibility of idea generation
Divergent thinking tasks have been widely used to evaluate domain general creative ability (Plucker et al., 2004; Silvia et al., 2008; Karwowski et al., 2021). In DT tasks, a prompt or a specific problem is presented requiring ideas to be generated by way of exploring many possible solutions (Runco and Acar, 2012; Reiter-Palmon et al., 2019; Kanlı, 2020). Verbal DT tasks contain open-ended prompts such as unusual uses for common objects (e.g., brick, bottle, table), instances or examples of common descriptors (e.g., loud, bright, and cold), similarities between common concepts (e.g., milk and meat), and consequences of imaginary events (e.g., clouds had strings).
The DT idea generation process is thought to be free-flowing, non-linear where many possible solutions are explored in a random manner (Kenett et al., 2018; Gray et al., 2019). Because DT often leads to solutions that are unique and original DT has been associated with creativity (Guilford, 1962; Acar et al., 2019; Peterson and Pattie, 2022). DT is an estimator of creative potential and not a measure of creativity (Runco, 1993; Runco, 2010; Carruthers and MacLean, 2019). It reflects the process of idea generation and its product in an easily measurable format (Milgram, 1991; Runco, 1993; Acar and Runco, 2012; Reiter-Palmon et al., 2019).
Flexibility is a dimension of DT with high theoretical importance, although it is only measured in a minority of published work in the creativity research literature. Flexibility is generally conceptualized in two major ways in contemporary literature (Acar and Runco, 2017). The more traditional and historical definition addresses the grouping of products into categories (Guilford, 1968; Mastria et al., 2021). In this context, flexibility is operationalized as the number of unique categories to which ideas belong (Guilford, 1975; Runco, 1986a; Runco and Okuda, 1991; Runco, 2013; Johnson et al., 2021). An alternate view of flexibility has focused on the shifting or switching in the process of ideation (Torrance, 1974; Yu et al., 2019; Preiss, 2022).
The importance of flexibility is in its ability to differentiate the creative quality of participant responses above and beyond originality (Nijstad et al., 2010; Kenett et al., 2018; Forthmann et al., 2019; Reiter-Palmon et al., 2019). For instance, when asking participants to create unusual uses to the prompt of “bottle,” the answers of “decoration” and “vase” would be considered to be in the same semantic category as they are using the bottle in somewhat similar ways. Therefore, each individual idea might be considered to have a certain degree of originality, but those two responses together would not exhibit high flexibility. However, the answer of “musical instrument” to the same prompt (i.e., bottle) is in a different semantic category as it relates to a different use all together, therefore resulting in a higher flexibility score (see Figure 1). The quantification of flexibility, therefore, is a methodological choice that can account for the close relations between responses in the same cluster and ensures that participants who generate ideas that are truly divergent from one another are captured in the scoring method (Acar and Runco, 2019).
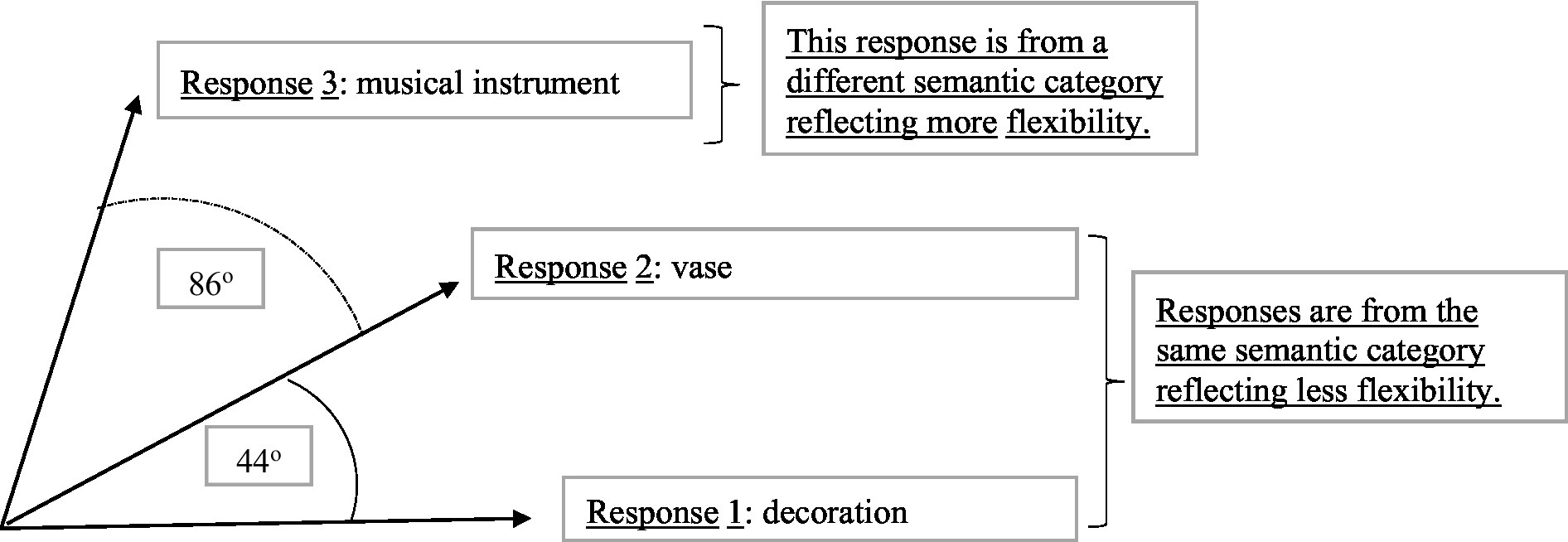
Figure 1. Measuring flexibility as a distance between consecutive responses. These responses are alternate uses of a bottle scored using GLoVe text-mining system.
Scoring idea generation
Together, originality and flexibility quantify the creative quality of participant responses to a DT task. As such, their scoring has historically been less objective and more time consuming compared to the scoring of fluency, which refers to more quantitative aspects of idea generation (i.e., the total count of ideas). Several scoring methods exist for flexibility, and each of those scoring methods presents unique advantages and weaknesses (Silvia et al., 2008; Barbot et al., 2019; Reiter-Palmon et al., 2019). Uniqueness scoring is one of the most widely used methods to evaluate the quality of idea generation in DT tasks (Silvia et al., 2008). Using this approach, a point is given for the use of a word from a category that has not occurred anywhere else within the analytic sample (Acar and Runco, 2019). As this method relies on the characteristics of a given sample for its scoring, the results are necessarily highly sample-dependent (Forthmann et al., 2019).
Also commonly used in the extant literature (e.g., Silvia, 2011), subjective scoring overcomes the sample dependency issues of uniqueness scoring. This type of scoring relies on trained human judges to rate the uniqueness of an idea in relation to each other to assess flexibility. Subjective scoring eliminates the need for the construction of a reference sample as the comparison and instead, answers are scored in relation to the human rater’s own experience. This scoring method is dependent on the raters’ perception of creativity and can produce inconsistent and biased results (Silvia et al., 2009; Primi et al., 2019; Reiter-Palmon et al., 2019). Hass and Beaty (2018) found that variances in scores obtained from 80 raters differed based on the task, such as for AUT the variance in ratings was 27% while in the case of consequences was 60%, and items, 10% in the case of AUT. For this reason, multiple raters are always needed in his method in order to ascertain inter-rater reliability and to understand the way in which idiosyncrasies among the raters are influencing the scoring (Kaufman et al., 2008; Guo et al., 2019). Forthmann et al. (2017) examined rater agreement scoring DT tasks and found that rater disagreement was higher when “be creative” instructions were used compared to standard instructions. They also found that rater disagreement was higher for less complex compared to more complex ideas across the different instructions.
Determining the cluster size when scoring flexibility also presents an additional problem in this scoring method (Hass, 2017). As cluster sizes are set arbitrarily therefore different scores are obtained when clusters are set to be wide, such as including all animals, or narrow, such as only including mammals. The size of the category or class during flexibility scoring is directly related to another phenomenon observed in creativity scoring termed the fluency bias (Plucker et al., 2004; Benedek et al., 2012; Forthmann et al., 2020). It has been observed that participants who generate a greater number of ideas are more likely to receive a higher originality score in comparison to those who generate fewer ideas, in part because more original ideas tend to arise later in the ideation process (Beaty and Silvia, 2012; Gonçalves and Cash, 2021). Flexibility suffers from the same confound as fluency in which a greater number of ideas generated increases the likelihood of those ideas belonging to different conceptual or semantic categories. Although research on the phenomenon is sparse in the context of flexibility, Acar et al. (2022), in a recent meta-analytic review, found a strong correlation between fluency and flexibility (r = 0.79) and concluded that fluency bias in the context of flexibility is even more profound than in the case of originality. Several summarization methods may function as solutions to the quantity-quality confound, such as using average quality (i.e., mean originality or mean flexibility), or only counting the most creative idea a participant produces (i.e., maximum originality or maximum flexibility), have been suggested (Runco and Acar, 2012; Acar and Runco, 2019; Reiter-Palmon et al., 2019). Therefore, in this study, we employed both average and maximum scoring methods to account for the effect of fluency bias (Table 1 lists all scoring methods used in this study).
Text mining-based scoring for idea generation
In addition to the clear sample dependency issues, confounds with the quantity of ideas, and human rater-biases that have plagued the scoring of creative quality dimensions (i.e., originality and flexibility) in the past, these traditional scoring methods are also highly labor intensive and costly. As verbal DT measures are designed to collect textual answers to open-ended prompts, each answer must be carefully read and scored by multiple human raters (Silvia, 2011; Reiter-Palmon et al., 2019). However, recent advances in machine learning have enabled creativity researchers to use associative network-based and text-mining based methods to assess creative ideations. Semantic network (SN) based methods rely on machine learning analysis which estimates relationships among words or concepts determined by their association within natural language. Words with close semantic association form clusters or neighborhoods which in turn form association networks (Rapp and Samuel, 2002; Pranoto and Afrilita, 2019). Acar and Runco (2014) were the first to examine the application of semantic network based methods in creativity research using the alternative uses task (AUT). They found that individuals with higher creative attitudes generated responses forming a more remote cluster compared to less creative individuals. Beketayev and Runco (2016) were the first to examine flexibility using semantic network based machine learning methods. They found a strong correlation between the number of categories into which responses fell and traditional uniqueness flexibility scores. Kenett et al. (2018) examined semantic networks of high creative individuals which demonstrated stronger links, therefore exhibiting more flexibility compared to low creative individuals. Focused on cognitive aspects of aging, Cosgrove et al. (2021) found diminished flexibility of the semantic networks of older adults compared to younger adults. SN based methods are useful for visualization and exploration of the relationships between responses. However, it lacks the functional ability to produce scores for individual responses, therefore, limiting practical applications.
Text-mining based methods are capable of denoting similarities between words numerically. There are several open accesses, web-based tools available for text-mining scoring to researchers through the Pennsylvania State University (Beaty and Johnson, 2021; http://semdis.wlu.psu.edu) or through the University of Denver (Organisciak and Dumas, 2020; https://openscoring.du.edu). To emulate natural language, text-mining methods collect a large number of textural documents or corpora (Cai et al., 2018). Forster and Dunbar (2009) were the first researchers to apply this method to creativity measurement, specifically to score divergent thinking tasks. They found a significant correlation between human-rated originality scores and originality scores obtained through text-mining on the Alternative Uses Test (AUT). Soon after that first study, Heinen and Johnson (2018) found that text-mining based creativity scores significantly correlated with subjective ratings of participants’ answers on a 20 and a 60-prompt creativity test. Dumas and Dunbar (2014) were the first to assess the construct reliability of text-mining based originality scores, and later examined the reliability of text-mining based originality scores even when controlling for fluency. To emulate natural language, text-mining methods collect a large number of textural documents or corpora. The matrix consists of rows and columns representing each word and each document, respectively (Cai et al., 2018). Words are then weighted in the matrix based on co-occurrences such that words that occur less often have greater weight, or emphasis in the model, and words occurring more often (i.e., common words) have less. Responses are represented as the vector of their summed and weighted words in semantic space. Semantic similarity between terms can be calculated as the cosine of the angle between their vectors (Oniani, 2020; Dumas et al., 2020a).
A proposed text-mining based measure of flexibility (OCS-flex)
Semantic distances measured between consecutive responses provide information about their semantic relationship. Small distances between words indicate semantic relatedness while large distances demonstrate unrelatedness expressions (Forthmann et al., 2019; Acar et al., 2021). Related words can be reasonably assumed to originate from the same semantic category while unrelated ideas are generated from different semantic categories, therefore, reflecting flexibility of thought (Beaty et al., 2021; Johnson et al., 2021).
Our proposed scoring method is based on this characteristic of flexibility, namely the relation between the semantic distance between the adjacent responses produced for the same prompt and the category to which these ideas belong. This relationship is numerically denoted in text-mining as the cosine of the angle between word vectors (Deerwester et al., 1990). For example, in Figure 1, the first response “decoration” given to the prompt “bottle” in an AUT exercise is in the same semantic category as the second response “vase.” This close semantic association is denoted by the cosine between the angles of the two responses (44o). However, the third response “musical instrument” is in a different semantic category from the first two, denoted by the larger cosine between their angles (86o).
In the current study, we created response pairs from the list of responses for each participant to assess flexibility of thinking. Similarly, to Johnson et al. (2021) who in their study examined metacognitive processes and operationalized idea diversity as the semantic distance between two responses, our first-word pair contained the first response and the second response. The second pair we created contained the second and third responses; the third pair contained the third and fourth responses, and so on (Figure 2). Next in our process of generating flexibility scores, semantic distance between the response pairs were calculated via a text-mining model, which becomes the flexibility score for those consecutive responses. We preserved the order in which the responses were given as creative idea generation is a process based on semantic search (Figure 2). This sequence of generated ideas holds important information about the participant’s ideation (Gray et al., 2019), indicating a temporal relationship among successive responses (Acar and Runco, 2014; Acar et al., 2019; Beaty et al., 2021; Olson et al., 2021).
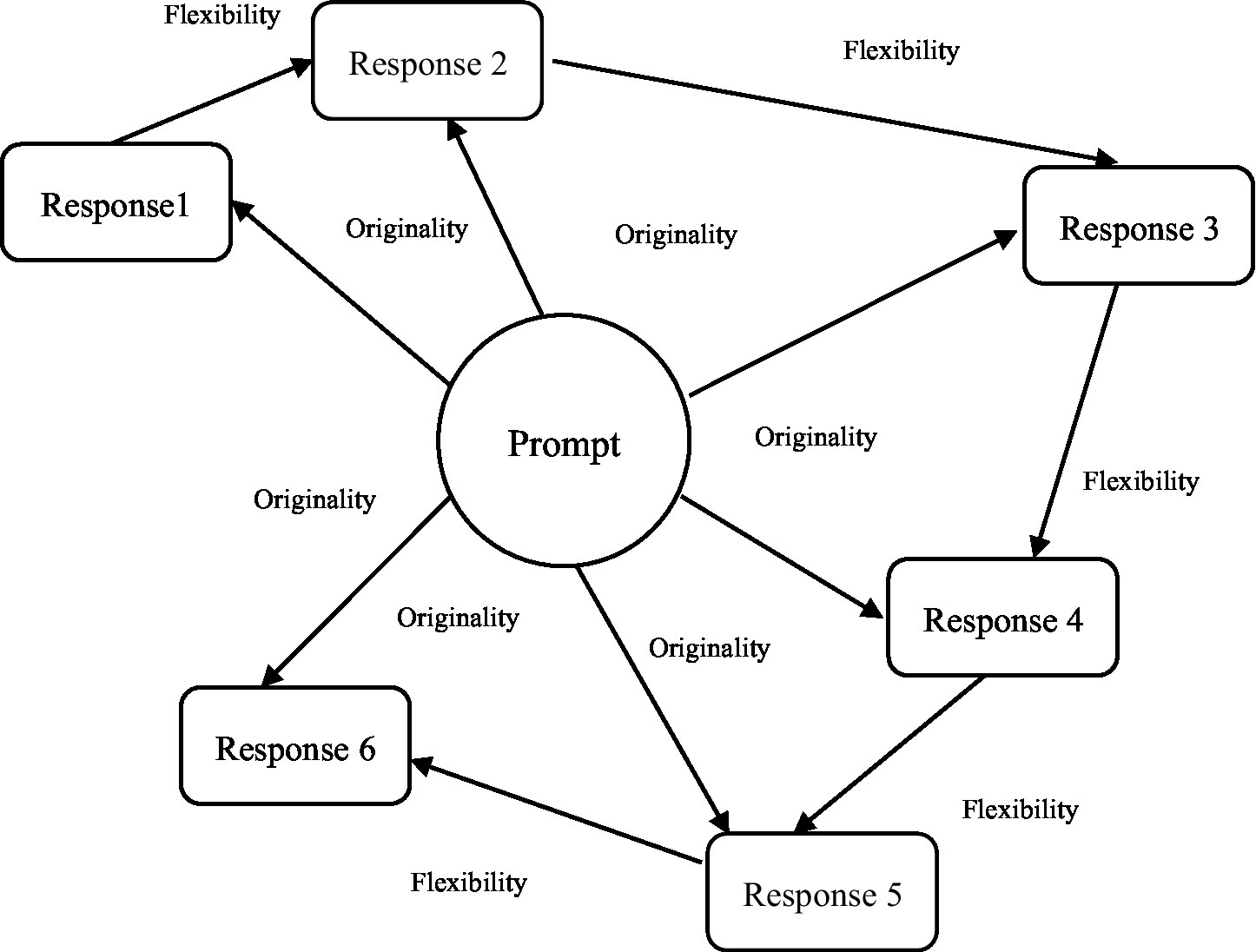
Figure 2. Flexibility as semantic distances between consecutive answers. The differing lengths of the arrows are meant to conceptually depict the varying semantic distance between prompt and responses for originality and between responses for flexibility. The model is based on Acar and Runco (2014).
To validate this scoring methodology, we used the GLoVe text-mining system to calculate semantic distance of adjacent responses (Pennington et al., 2014). GLoVe has been shown to mimic human raters in DT tasks and is currently considered superior to many other existing text-mining systems based on recent methodological investigations (Dumas et al., 2020b; Acar et al., 2021; Beaty and Johnson, 2021). We also plan to follow suggestions of Forthmann et al. (2019) to correct for multiword answers by stoplisting, which removes very commonly used words (e.g., the, and, as) from scoring. Term weighting was applied to enable incorporation of the frequency and importance of occurrences of each term. Averages of the weighted vectors were used to generate one vector representing multiword responses. As vector length does hold information about the detailedness of the response (Manning et al., 2008), some of this information could potentially be lost as a result of average weighting (Deerwester et al., 1990), however this methodology has been used successfully by many creativity researchers (Silvia et al., 2009; Dumas and Dunbar, 2014; Harbinson and Haarmann, 2014; Dumas and Strickland, 2018; Acar et al., 2021).
Goals of current study
To examine the validity of our conceptualization and proposed measurement procedure for flexibility (OCS-Flex), obtained from the https://openscoring.du.edu/about site which is based on the GLoVe text-mining system, we conducted two different studies using DT tasks measuring creative ideation. In the first study, we focused on the convergent and discriminant validity of OCS-Flex. To do this, we correlated OCS-Flex with the established flexibility scoring method (Pub-Flex) of the verbal form of the Torrance Test of Creative Thinking (TTCT; Torrance, 1998), as well as TTCT fluency (Pub-Flu) and originality scores (Pub-Orig; the different scoring methods used in this study are listed in Table 1).
In the second study, DT was assessed with the AUT. These data were used to examine both the internal and external validity of OCS-Flex from a latent variable perspective. Following a factor analysis methodology, we calculated both composite and optimally-weighted reliability indices for the OCS-Flex model and correlated the resulting latent OCS-Flex scores to OCS-Flu, and OCS-Orig. Finally, we examined correlates of OCS-Flex to personality dimensions that have been previously shown to predict creativity (scoring methods used in the study are listed in Table 1).
Based on these aims the following research questions are posited and addressed:
1. What is the relation of the OCS-Flex to TTCT Pub-Orig and Pub-Flu scores and OCS-Orig and OCS-Flu score (discriminant validity)?
2. What is the relation of the OCS-Flex scoring to TTCT Pub-Flex scores (convergent validity)?
3. How well does the OCS-Flex scoring method fit into the theoretical framework of DT suggesting that items measure one latent construct (construct validity)?
4. How well can latent models capture the relationship between the three main dimensions of flexibility, originality, and fluency (construct validity)?
5. How well do scores from the OCS-Flex align with creative personality characteristics (criterion validity)?
Study 1
Methodology
The first study reanalyzed data from Acar et al. (2021). In the original study, two tasks of the Torrance Test of Creative Thinking-Verbal (TTCT), the Unusual Uses Test (UUT), and Just Suppose Test (JST), were scored for originality using three different text-mining systems. The authors compared the text-mining scores to snapshot and TTCT publisher-generated scoring. They found that all text-mining scores correlated strongly with snapshot and TTCT publisher-generated scoring, but the GLoVe text-mining system scores replicated established scoring methods most accurately. Acar et al. (2021) did not examine the flexibility of participants within this dataset, and the present analysis is therefore entirely novel. Scores generated for the current study are archived and can be accessed on Zenodo (LINK: DOI 10.5281/zenodo.6323425).
Participants
This dataset contained information from 224 participants; 101 of whom completed Form A and 123 of whom completed Form B of the TTCT Verbal (Torrance, 1998). Participants were undergraduate freshman students at a large public university in the Northeast United States. The average age of the sample was 18.20 (SD = 1.31) of which 26.7% of the participants were male, 48.9% female, and 24.4% did not report gender.
Measure and scoring
The TTCT Verbal (Torrance, 1998) contains six activities. In this study, data from only two of the activities, the JST and the UUT, were analyzed. The UUT solicits a list of differing uses for an everyday object. Participants had 5 min to list uses for Cardboard Boxes on Form A and Tin Cans on Form B. The JST presents a hypothetical situation and participants are prompted to list possible consequences of the event. On Form A, participants are asked what would happen if “.. the clouds had strings attached to them..” and on Form B if “..all we could see of people would be their feet..” Participants were given 5 min for each of the above activities to be completed. The answer booklets were scored by Scholastic Testing Service (STS), the publisher of the TTCT, for fluency (Pub-Flu), originality (Pub-Orig), flexibility (Pub-Flex) and follows the process described in the TTCT manual.1 STS raters are highly trained whose performance is measured against expert raters using an intraclass correlation of 0.90 or higher (Torrance, 1974). The reported scores were used in the study without any alteration. In addition, OCS-Flex scores were produced. To reflect the conventional summation scoring used in the TTCT publisher-generated scores, we calculated a sum score for both OCS-Flex and OCS-Orig. To overcome a potential fluency confound, mean scores were created for every participant by summing the OCS-Flex scores for each word pair and dividing it by OCS-Flu scores on each task separately (i.e., to indicate the average flexibility per item on the measure). Maximum OCS-Flex scores were also generated by choosing the individual response with the highest OCS-Flex score for each participant on each task. All responses were scored using the freely available web-based tool by Organisciak and Dumas (2020; https://openscoring.du.edu/about).
Results
Results of the item-level descriptive statistics for each scoring methodology, listed in Table 1, are displayed in Table 2. In the first study, we correlated OCS-Flex (mean, maximum, and sum), OCS-Orig (mean, maximum, and sum), and Pub scores (flexibility, fluency, and originality). As some of the items violated normality, we used Spearman’s Rho.
Relations among publisher-generated (pub) and text-mining flexibility (OCS-flex) scores
TTCT Pub-Flex scores correlated significantly with all OCS-Flex scores supporting reliability of the proposed scoring methods (Table 3). By far, the strongest correlation was observed between sum OCS-Flex scoring and Pub-Flex scores on both the UUT (ρ = 0.72, p < 0.01, 95% CI [0.63, 0.79]) and JST (ρ = 0.70, p < 0.01, 95% CI [0.60, 0.78]). As publisher scores are calculated by adding all scores together, the strong relationship between Pub scores and sum OCS-Flex scores is reasonably expected.
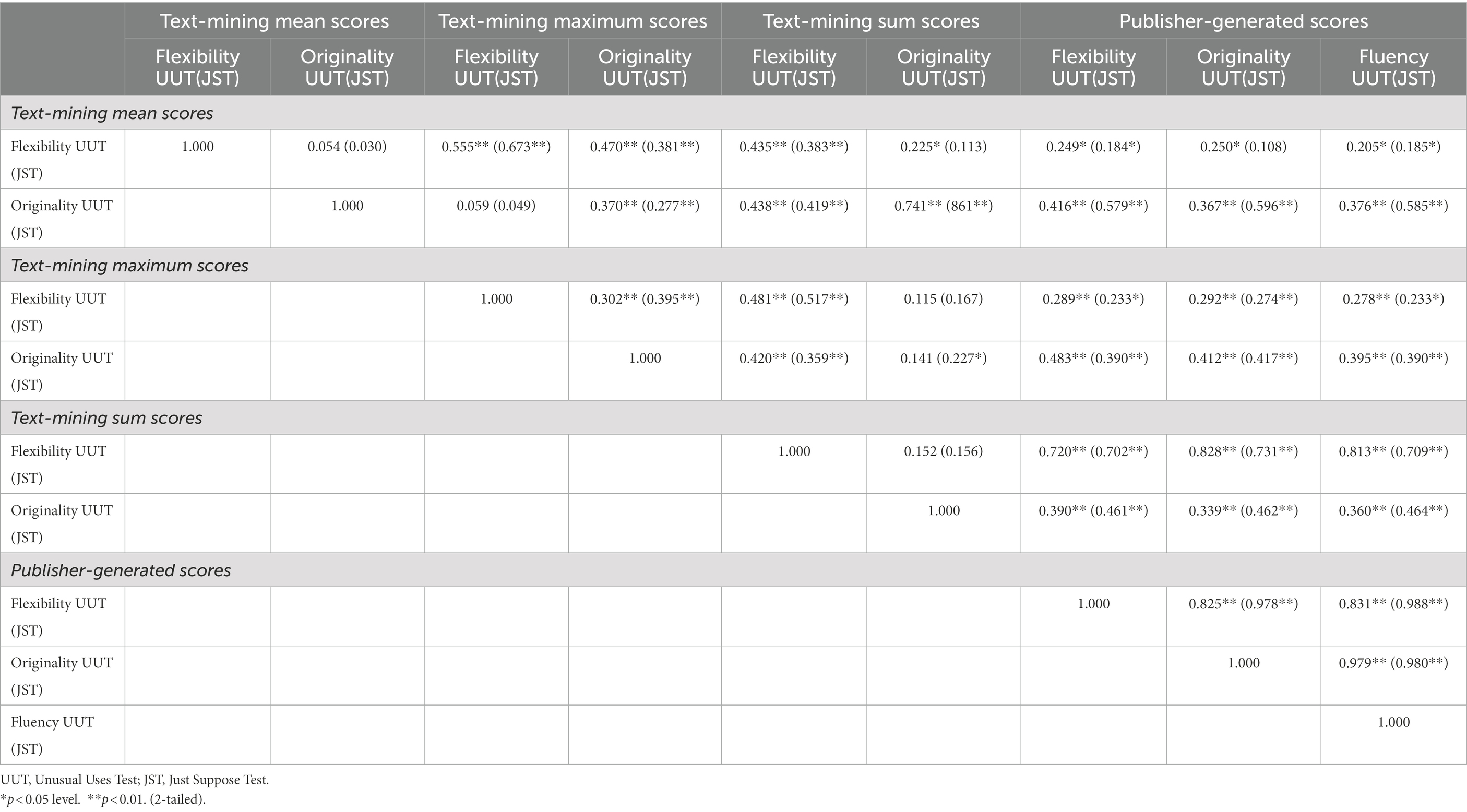
Table 3. Correlations of mean and maximum flexibility and originality scores with publisher-generated scores of the TTCT verbal.
Text-mining scores on the JST showed a consistently weaker although still significant correlation with publisher scores. The magnitude of differences in correlations between the UUT and the JST activities possibly depict the difficulty of scoring the more elaborate and complex JST. These findings are also in line with the study by Landreneau and Halpin (1978) reporting that the average flexibility, fluency, and originality scores on the JST were consistently lower on the TTCT compared to the UUT. Silvia (2011) also found that UUT achieved the highest reliability while JST had the poorest reliability using latent modeling techniques. Finally, in the study by Acar et al. (2021), the inter-rater reliability of the JST using the TTCT was lower compared to the UUT.
Relations among flexibility, originality, fluency scores
Publisher-generated (Pub) scores
Correlations of Pub-Orig, Pub-Flu, and Pub-Flex revealed very strong relations among the dimensions which ranged from 0.83 to 0.99. Activity-specific correlations between the TTCT Pub scores (flexibility, fluency, and originality) ranged between 0.83 and 0.98 on the UUT and between 0.98 and 0.99 on the JST (Table 3).
Text-mining (OCS) scores
The correlation between OCS-Orig and OCS-Flex for mean and the sum scoring was not significant on both tasks (UUT and JST; Table 3). However, when using maximum scoring the relation was significant although weak for both the UUT (ρ = 0.30, p < 0.01, 95% CI [0.18, 0.41]) and JST (ρ = 0.40, p < 0.01, 95% CI [0.28, 0.50]). Fisher’s transformation revealed that this correlation was significantly smaller than correlations between Pub-Flex and Pun-Orig scores for both UUT (z = 9.05, p < 0.05) and JST (z = 19.25, p < 0.05).
The sum OCS-Flex scores correlated the strongest with OCS-Flu scores for both the UUT (ρ = 0.81, p < 0.01, 95% CI [0.76, 0.85]) and JST (ρ = 0.71, p < 0.01, 95% CI [0.64, 0.77]). This scoring, similarly, Pub scores, is calculated by adding all flexibility scores together and therefore provides no correction for the confounding effects of fluency. Mean and maximum OCS-Flex scores correlated weaker although significantly with OCS-Flu (Table 3). Fisher’s transformation revealed that both maximum (zuut = 9.52, p < 0.05; zjst = 10.23, p < 0.05) and mean scoring (zuut = 10.34, p < 0.05; zjst = 10.56, p < 0.05) correlated significantly weaker with OCS-Flu than Pub-Flex scores. These results suggest that both mean and maximum OCS-Flex scoring provided differentiation from both OCS-Orig and OCS-Flu, therefore, outperforming Pub scores.
Study 2
Methodology
For the second study, we re-analyzed data collected by Dumas et al. (2020a). The data is archived and can be accessed on Zenodo (LINK: https://zenodo.org/record/3899579#.X3M81KSlaQ; DOI:10.5281/zenodo.3899578). Dumas et al. (2020a) used text-mining to examine originality on the AUT. The study compared originality of three different groups of participants (professional actors, student actors and non-actor controls), however they did not consider the flexibility of these participants, and therefore the present analysis is entirely novel.
Participants
In the second study, we used a dataset that included information from 296 participants across three groups: non-acting adults (n = 92), undergraduate acting and theater major students (n = 100), and professional actors (n = 104). Sixty-three percent of the sample were female, with an average age of 28 (SD = 6.40). Eighty-six percent of the participants were white, 6% African American, 5% Latinx, and 5% Asian. All individuals were financially compensated for their participation.
Measures and scoring
The Big Five Aspects Scale (DeYoung et al., 2007) contains 100 items and measures characteristics mapped onto the Big Five personality traits including: Neuroticism, Agreeableness, Conscientiousness, Extraversion, and Openness (Goldberg, 1990). These aspects are further subdivided into two facets each: the Neuroticism attribute contains Volatility and Withdrawal; the Agreeableness trait comprises Compassion and Politeness; the Conscientiousness characteristic measures Industriousness and Orderliness; the Extraversion attribute contains Enthusiasm and Assertiveness; and the Openness aspect is divided into Intellect and Openness. The 10 facets displayed strong internal consistency reliability (ranging between 0.85 to 0.95; Dumas et al., 2020a,b).
The AUT is one of the oldest and most widely used assessments in creativity research (Guilford, 1967; Silvia et al., 2008). This task is very similar to the UUT as it asks participants to generate different uses for everyday object. In this version of the AUT, participants were asked to generate as many original or unusual uses as they could to 10 everyday objects: book, fork, table, hammer, pants, bottle, brick, tire, shovel, and shoe. The time limit on this measure was 2 min per object. The measure was scored along four dimensions: fluency, originality, and flexibility.
To score the items in this study, the same freeware system was used as in Study 1 (Organisciak and Dumas, 2020; https://openscoring.du.edu/about). OCS-Flu was assessed as the count or number of responses each participant provided to each of the items. Mean OCS-Flu was measured as the average fluency across all tasks. The average was calculated as the sum of all OCS-Flu scores across all 10 items divided by the number of items (10). Maximum OCS-Flu indicates the highest fluency score achieved among the 10 tasks. OCS-Orig was calculated as the semantic distance between the prompt and each response (Dumas et al., 2020a,b). For mean OCS-Orig, we summed the scores and divided each by fluency on each task. Maximum OCS-Orig denotes the highest scores on each of the 10 tasks. Internal consistency reliability for OCS-Orig mean and OCS-Orig maximum scores were 0.85 and 0.86, respectively (Dumas et al., 2020a,b). Similar to the first study, word pairs were created to measure semantic distance and mean, and maximum flexibility scores were generated for each of the 10 AUT items.
Results
Item-level descriptive statistics for each measurement methodology employed in the study (Table 1) are displayed in Table 2. Many of the items displayed higher than acceptable skewness on both mean OCS-Flex and maximum OCS-Flex scoring. To account for the non-normal distribution, Spearman’s Rho correlations were used.
Reliability and validity of text-mining flexibility (OCS-flex) scores
Reliability of the AUT across 10 items was evaluated using Cronbach’s alpha (Cronbach, 1951), Hancock’s H (Hancock and Mueller, 2001), and omega of McDonald (1999). Taken together, these three indices encompass the theoretical range of the score reliability. Both OCS-Flex scoring methods achieved good reliability on all reliability indices (Table 4).
Construct validity of these 10 AUT items was assessed via confirmatory factor analysis (CFA) with Mplus 8.4 software (Muthén and Muthén, 2007). As normality was violated for many of the AUT items, robust maximum likelihood estimations with Santorra-Bentler corrections were used to model the data. To evaluate our OCS-Flex mean and maximum models (Figure 3), we used conventional cutoff values to assess model fit (Hu and Bentler, 1999). Although these fixed cutoffs for fit indices have been widely used, their generalizability is limited as values are based on one specific confirmatory factor analysis model therefore, results should be interpreted in conjunction with reliability indices and factor loadings. Although, specific model cutoffs can be calculated using the Shiny app by McNeish and Wolf (2020) it is only accurate for models estimated using maximum likelihood.
Chi-square goodness of fit statistics, for both methods, were not significant although root mean square error of approximation (RMSEA) was below the conventional cut off of 0.06 while the comparative fit index (CFI) and the Tucker-Lewis index (TLI) were above the conventional suggested cut-off value of 0.90 and the standardized root mean square residual (SRMR) were below the conventional cut-off of 0.08 (Table 4). Although all indices suggested a good fit for both of our models, not all items load equally well onto the OCS-Flex factor. The item “book” had to lowest loading for both mean (0.39) and maximum (0.34) onto OCS-Flex suggesting that this item might not measure flexibility well.
Relations among text-mining flexibility (OCS-flex), originality (OCS-Orig), fluency (OCS-flu) scores
To further examine relations among the three most often used dimensions of DT (fluency, originality, and flexibility) we constructed CFA models with OCS-Flex, OCS-Orig, and OCS-Flu correlated latent factors. A model was fitted with scores for mean OCS-Flex, mean OCS-Orig, and OCS-Flu and another for maximum OCS-Flex, maximum OCS-Orig, and OCS-Flu. The three correlated factors each contained 10 items (Figures 3, 4). It is reasonable to assume that the 10 items on the AUT share variance across the factors. Therefore, each item was correlated with the same item loading on the other two factors. No cross-loading or correlations between items on the same factor was allowed as variances across items should be accounted for by the factor onto which they load. These strong a-prior specifications resulted in models with good reliability (Cronbach’s alpha, Hancock’s H, and McDonald’s Omega) with the exception of the acceptable fit for the OCS-Flex dimension in the maximum model (Table 4). Chi-square goodness of fit statistics was significant for both models while RMSEA, comparative fit index (CFI) and the Tucker-Lewis index (TLI), SRMR all displayed a good fit for both methods (Hu and Bentler, 1999; Table 4).
The correlation between the OCS-Flex and OCS-Orig factors in the mean model (0.79) and between OCS-Flex and OCS-Flu factors in the maximum model (0.63) was both strong suggesting a persistent relationship between these factors. Although items loaded on the OCS-Flu factor well, item loading varied across the OCS-Flex and OCS-Origi factors for both models. Similar to the single factor model, the item “book” loaded the weakest on both OCS-Flex and OCS-Orig on both mean and maximum models (Figures 3, 4).
Criterion validity
To further expand on the examination of criterion validity, scores of mean OCS-Flex and maximum OCS-Flex were correlated with 10 facets of personality associated with the Big Five model (i.e., openness, intellect, enthusiasm, assertiveness, industriousness, orderliness, compassion, politeness, volatility and withdrawal; DeYoung et al., 2016). Mean OCS-Flex significantly positively correlated with openness (r(296) = 0.15, p = 0.047, 95% CI [0.04, 0.26]) while maximum OCS-Flex scores positively correlated with the enthusiasm facet of the extraversion factor (r(296) = 0.17, p = 0.003, 95% CI [0.06, 0.28]).
Discussion
In this study, we proposed and psychometrically validated an automated text-mining based approach to score flexibility on DT tasks. Based on our evaluation of these methods, the following key findings have emerged.
Text-mining based flexibility (OCS-flex) scores closely correlate with TTCT Publisher scoring (pub)
Due to its extensively studied validity, the TTCT publisher-generated scores were used to validate OCS-Flex scores in our first Study (Chase, 1985; Treffinger, 1985; Torrance, 1998; Cramond et al., 2005; Kim, 2006; Acar et al., 2022). All proposed OCS-Flex scores (mean, maximum, sum) correlated significantly with Pub-Flex scores providing proof of the validity of our scoring method. Publisher-generated scores are calculated as the sum across games of the TTC verbal, therefore, it was not surprising that our sum OCS-Flex score correlated the strongest with publisher scores (Table 3).
There were very high inter-correlations among the TTCT Pub-Flex, Pub-Flu, and Pub-Orig scores ranging between 0.83 and 0.99. The strong correlations among the DT dimensions are not unique to our current study and the lack of differentiation among dimensions on the TTCT has been long written about in the field (Mansfield et al., 1978; Clapham, 1998; Mouchiroud and Lubart, 2001; Kim, 2006; Silvia et al., 2008; Zeng et al., 2011; Grajzel et al., 2022). Due to this strong correlation between Pub-Orig and Pub-Flex scoring, we were not able to establish discriminant validity of our OCS-Flex scoring method. However, for both the sum and mean text-mining scoring, the correlation between OCS-Orig and OCS-Flex was not significant suggesting that these scoring methods allow for better differentiation between dimensions. Even in the case of maximum scoring, the correlation between OCS-Flex and OCS-Orig was significantly smaller than the correlation between Pub scoring of the same dimensions.
The correlation of OCS-Flex and OCS-Orig with OCS-Flu was the strongest for sum scores. This is of no surprise as this scoring method does not provide any correction for fluency confound. The reduced correlations between OCS-Flu scores and mean and maximum scoring suggest that at least some fluency control has been implemented using these scoring methods. These results suggest that all OCS scoring methods proposed in Study 1 (mean, maximum, and sum) allow for differentiation among the three dimensions of originality, fluency, and flexibility.
Text-mining based flexibility (OCS) scores demonstrated high reliability and construct validity
To evaluate construct reliability and construct validity of OCS-Flex, two CFA models were constructed. The first model included all mean OCS scores for all 10 items of the AUT while the second model contained all maximum OCS scores. Both models displayed a good fit to the data as indicated by model fit indices, internal reliability indices (Cronbach’s alpha, coefficient H and Omega; Table 4), and factor loadings (displayed in Figure 5). However, some of the item loadings were lower (e.g., book), suggesting that not all items measure the concept of flexibility equally well and highlighting the need for more latent measurement modeling in this area of research.
Text-mining based flexibility (OCS) scores showed valid relations with external variables
To examine the relationship between OCS-Flex, OCS-Orig, and OCS-Flu scores two further latent models were constructed (Figures 3, 4) These models, one including mean OCS-Flex, mean OCS-Orig, and OCS-Flu and the other containing maximum OCS-Flex, maximum OCS-Orig, and OCS-Flu displayed good to acceptable fit based on reliability indices (Cronbach’s alpha, coefficient H and Omega), fit indices and factor loadings (Table 4). There was a strong correlation between OCS-Flex mean and OCS-Orig mean latent factors. This strong relationship between originality and flexibility has been observed previously and could be accounted for by the theoretical relationship between the two dimensions (Runco, 1986b; Acar and Runco, 2014; Acar et al., 2022). The correlation observed between maximum OCS-Flex and OCS-Flu factors suggests that some of the fluency confound still remained using this scoring method.
To further expand on the examination of criterion validity, mean OCS-Flex and maximum OCS-Flex scores were correlated with personality characteristics of openness, intellect, enthusiasm, assertiveness, industriousness, orderliness, compassion, politeness, volatility, and withdrawal. Mean OCS-Flex was significantly positively correlated with openness to experiences. Openness has been long thought of as one of the main personality characteristics of creative individuals suggesting that open-minded and curious participants generate more creative responses (McCrae, 1987; King et al., 1996; Dollinger et al., 2004; Hagtvedt et al., 2019). Maximum OCS-Flex scores positively correlated with enthusiasm. Grohman et al. (2017) found students’ creative behavior was correlated with teacher reported enthusiasm. Passion or enthusiasm is also closely related to intrinsic motivation (Amabile and Fisher, 2000; Moeller et al., 2015) which has been implicated in creative idea generation (Paulus and Brown, 2007; Dumas et al., 2020a; Gu et al., 2020).
Limitations and future directions
Despite the strong results supporting the two OCS-Flex scoring methods (mean and maximum) presented in this study, the specific foci of the current work necessarily imply that many questions regarding flexibility scoring are still awaiting examination. Although the psychometric findings we reported here suggest that these scores are ready to be deployed in research, we also put forth a number of future directions in the measurement of flexibility that may be worth considering.
Targeted focus on verbal measures
Verbal DT tasks are the most often utilized measures assessing creative potential (Hass, 2017; Reiter-Palmon et al., 2019; Acar et al., 2020). Therefore, we focused our attention on verbal tasks only, examining data gathered with two of the most popular assessments (TTCT and researcher-created AUTs; Mouchiroud and Lubart, 2001; Cho et al., 2010; Cortes et al., 2019). Of course, the application of text-mining methods here also necessitates the use of verbal or written data, because the process fundamentally relies on the words participants use to respond to the task. So, it remains to be explored how figural DT scores might be related to text-mining verbal scores and specifically to flexibility using the mean and maximum scoring method explored in this article (Cropley and Marrone, 2022).
Modeling time between responses
There has been an emerging interest in recent years exploring the relation between time spent on tasks and flexibility of responses. Beketayev and Runco (2016) found a positive correlation between latency (i.e., the time between responses to a DT task) and flexibility which diminished after 178 s. Acar and Runco (2017) found that category switching was denoted by an approximate increase of 5 s spent on a given response. Said-Metwaly et al. (2020) conducted a meta-analytic study and found that longer time allowed on task increased flexibility of responses. Data used in this study did not allow for the examination of the length of time per idea generated. Therefore, future research should focus on exploring the relationship between latency and text-mining based flexibility scores.
Semantic vs. categorical flexibility
Vector based semantic methods, such as the one used in this study, allow for quantitative expressions of semantic distance therefore providing an automatic and valid way to measure DT (Forster and Dunbar, 2009; Beketayev and Runco, 2016; Forthmann et al., 2019; Dumas et al., 2020b; Acar et al., 2021). Text-mining methods however also present certain restrictions to measuring flexibility. The vector-based method we used here was only able to model semantic distances between two adjacent ideas (and then average those distances across all idea pairs), and therefore categories or clusters of ideas that could be sorted into conceptual groups were not detected (Kenett, 2019). For this reason, we propose that flexibility could be examined from multiple perspectives by combining LSA-based text-mining methods and associative-based semantic network models (Kenett, 2019; Kumar et al., 2020). This is especially important as although LSA-based text-mining methods have been successfully used in many DT studies, others have cautioned of their limited ability to capture the wide complexity of the semantic systems (Hutchison et al., 2008). Moreover, by not accounting for the clustering of the responses in our current methodology, flexibility can be overestimated. Therefore, future research should also examine the bias possibly embedded in this proposed scoring method due to the use of consecutive pairs of responses to calculate flexibility.
Conclusion
Flexible thinking patterns are a hallmark of creative cognition (Benedek et al., 2014; Chrysikou, 2018; Gube and Lajoie, 2020; Huang et al., 2020). Flexibility however is less often assessed in DT tasks compared to other dimensions such as fluency and originality (Reiter-Palmon et al., 2019). Flexibility provides a unique and important measure that can differentiate the creative quality above and beyond originality related to the metacognitive process of switching (Nijstad et al., 2010; Kenett et al., 2018; Forthmann et al., 2019).
In this study, we proposed an automated scoring for flexibility which provides an alternative, reliable, valid, and practical way to measure flexibility on DT tasks. We provided evidence that our scoring method aligns with existing standards employed by researchers for scoring flexibility (i.e., number of categories on TTCT responses) and that this method is able to mimic relationships between dimensions (fluency, originality) of DT. Latent structure of this flexibility scoring followed theoretically agreed-upon dimensionality and captured real-life differences in personality among participants. This methodology enables the more extensive use of this dimension and provides a less labor intensive and costly measurement of flexibility. Our proposed scoring methodology does not suffer from the sample dependence issues of uniqueness scoring or from rater subjectivity in subjective scoring, therefore, proving a scoring methodology that is comparable across studies and easy to implement. Given the psychometric evidence presented here and the practicality of automated scores, we recommend adopting this new method. Surely, further investigations are needed to examine additional evidence of validity.
Data availability statement
The original contributions presented in the study are included in the article/supplementary material, further inquiries can be directed to the corresponding author.
Author contributions
All authors listed have made a substantial, direct, and intellectual contribution to the work, and approved it for publication.
Conflict of interest
The authors declare that the research was conducted in the absence of any commercial or financial relationships that could be construed as a potential conflict of interest.
Publisher’s note
All claims expressed in this article are solely those of the authors and do not necessarily represent those of their affiliated organizations, or those of the publisher, the editors and the reviewers. Any product that may be evaluated in this article, or claim that may be made by its manufacturer, is not guaranteed or endorsed by the publisher.
Footnotes
References
Acar, S., Abdulla, A., Ahmed, M., Runco, M. A., and Beketayev, K. (2019). Latency as a predictor of originality in divergent thinking. Think. Skills Creat. 33:100574. doi: 10.1016/J.TSC.2019.100574
Acar, S., Berthuiaume, K., Grajzel, K., Dumas, D., and Flemister, T. (2021). Applying automated originality scoring to the verbal form of Torrance tests of creative thinking. Gifted Child Quarterly. Manuscript submitted for publication.
Acar, S., Ogurlu, U., and Zorychta, A. (2022). Exploration of discriminant validity in divergent thinking tasks: a meta-analysis. Psychol. Aesthet. Creat. Arts. Advance online publication. doi: 10.1037/aca0000469
Acar, S., and Runco, M. A. (2012). “Creative abilities: Divergent thinking,” in Handbook of Organizational Creativity. Academic Press, 115–139.
Acar, S., and Runco, M. A. (2014). Assessing associative distance among ideas elicited by tests of divergent thinking. Creat. Res. J. 26, 229–238. doi: 10.1080/10400419.2014.901095
Acar, S., and Runco, M. A. (2017). Latency predicts category switch in divergent thinking. Psychol. Aesthet. Creat. Arts 11, 43–51. doi: 10.1037/aca0000091
Acar, S., and Runco, M. A. (2019). Divergent thinking: new methods, recent research, and extended theory. Psychol. Aesthet. Creat. Arts 13, 153–158. doi: 10.1037/aca0000231
Acar, S., Runco, M. A., and Ogurlu, U. (2019). The moderating influence of idea sequence: a reanalysis of the relationship between category switch and latency. Personal. Individ. Differ. 142, 214–217. doi: 10.1016/j.paid.2018.06.013
Acar, S., Runco, M. A., and Park, H. (2020). What should people be told when they take a divergent thinking test? A meta-analytic review of explicit instructions for divergent thinking. Psychol. Aesthet. Creat. Arts 14, 39–49. doi: 10.1037/aca0000256
Amabile, T. M., and Fisher, C. M. (2000). “Stimulate creativity by fueling passion,” in Handbook of Principles of Organizational Behavior. 2nd Edition. ed. E. Locke (West Sussex, UK: John Wiley & Sons), 481–497.
Asquith, S. L., Wang, X., Quintana, D. S., and Abraham, A. (2022). Predictors of creativity in young people: using frequentist and Bayesian approaches in estimating the importance of individual and contextual factors. Psychol. Aesthet. Creat. Arts 16, 209–220. doi: 10.1037/aca0000322
Barbot, B., Hass, R. W., and Reiter-Palmon, R. (2019). Creativity assessment in psychological research: (re)setting the standards. Psychol. Aesthet. Creat. Arts 13, 233–240. doi: 10.1037/aca0000233
Beaty, R. E., and Johnson, D. R. (2021). Automating creativity assessment with SemDis: an open platform for computing semantic distance. Behav. Res. Methods 53, 757–780. doi: 10.31234/osf.io/nwvps
Beaty, R. E., and Silvia, P. J. (2012). Why do ideas get more creative across time? An executive interpretation of the serial order effect in divergent thinking tasks. Psychol. Aesthet. Creat. Arts 6, 309–319. doi: 10.1037/a0029171
Beaty, R. E., Zeitlen, D. C., Baker, B. S., and Kenett, Y. N. (2021). Forward flow and creative thought: assessing associative cognition and its role in divergent thinking. Think. Skills Creat. 41:100859. doi: 10.1016/j.tsc.2021.100859
Beckmann, J. F. (2014). The umbrella that is too wide and yet too small: why dynamic testing has still not delivered on the promise that was never made. J. Cogn. Educ. Psychol. 13, 308–323. doi: 10.1891/1945-8959.13.3.308
Beketayev, K., and Runco, M. A. (2016). Scoring divergent thinking test by computer with a semantic-based algorithm. Eur. J. Psychol. 12, 210–220. doi: 10.5964/ejop.v12i2.1127
Benedek, M., Jauk, E., Sommer, M., Arendasy, M., and Neubauer, A. C. (2014). Intelligence, creativity, and cognitive control: the common and differential involvement of executive functions in intelligence and creativity. Intelligence 46, 73–83. doi: 10.1016/j.intell.2014.05.007
Benedek, M., Franz, F., Heene, M., and Neubauer, A. C. (2012). Differential effects of cognitive inhibition and intelligence on creativity. Pers. Individ. Differ. 53, 480–485. doi: 10.1016/j.paid.2012.04.014
Cai, Z., Graesser, A. C., Windsor, L. C., Cheng, Q., Shaffer, D. W., and Hu, X. (2018). Impact of corpus size and dimensionality of LSA spaces from ikWipedia articles on AutoTutor answer evaluation. [Paper presentation]. 11th International Conference on Educational Data Mining, Raleigh, NC, United States.
Carruthers, L., and MacLean, R. (2019). “The dynamic definition of creativity: implications for creativity assessment,” in Dynamic Perspectives on Creativity: New Directions for Theory, Research, and Practice in Education. eds. R. A. Beghetto and G. Emanuele Corazza (Cham, Switzerland: Springer), 207–223.
Carson, S. H., Peterson, J. B., and Higgins, D. M. (2003). Decreased latent inhibition is associated with increased creative achievement in high-functioning individuals. J. Pers. Soc. Psychol. 85, 499–506. doi: 10.1037/0022-3514.85.3.499
Chase, C. I. (1985). “Review of the Torrance tests of creative thinking” in The Ninth Mental Measurements Yearbook. ed. J. V. Mitchell Jr. (Lincoln, NE: Buros Institute of Mental Measurements), 1631–1632.
Chen, X., He, J., and Fan, X. (2022). Relationships between openness to experience, cognitive flexibility, self-esteem, and creativity among bilingual college students in the US. Int. J. Biling. Educ. Biling. 25, 342–354. doi: 10.1080/13670050.2019.1688247
Cho, S. H., Nijenhuis, J. T., Vianen, A. E., Kim, H., and Lee, K. H. (2010). The relationship between diverse components of intelligence and creativity. J. Creat. Behav. 44, 125–137. doi: 10.3389/fpsyg.2017.00254
Chrysikou, E. G. (2018). “The costs and benefits of cognitive control for creativity” in The Cambridge Handbook of the Neuroscience of Creativity. eds. R. E. Jung and O. Vartanian (Cambridge, UK: Cambridge University Press), 299–317.
Clapham, M. M. (1998). Structure of figural forms A and B of the torrance tests of creative thinking. Educ. Psychol. Meas. 58, 275–283. doi: 10.1177/0013164498058002010
Cortes, R. A., Weinberger, A. B., Daker, R. J., and Green, A. E. (2019). Re-examining prominent measures of divergent and convergent creativity. Curr. Opin. Behav. Sci. 27, 90–93. doi: 10.1016/j.cobeha.2018.09.017
Cosgrove, A. L., Kenett, Y. N., Beaty, R. E., and Diaz, M. T. (2021). Quantifying flexibility in thought: the resiliency of semantic networks differs across the lifespan. Cognition 211:104631. doi: 10.1016/j.cognition.2021.104631
Costa, P. T., and McCrae, R. R. (1995). Solid ground in the wetlands of personality: a reply to block. Psychol. Bull. 117, 216–220. doi: 10.1037/0033-2909.117.2.216
Cramond, B., Matthews-Morgan, J., Bandalos, D., and Zuo, L. (2005). A report on the 40-year follow-up of the Torrance tests of creative thinking. Gift. Child Q. 49, 283–291. doi: 10.1177/001698620504900402
Cronbach, L. J. (1951). Coefficient alpha and the internal structure of tests. Psychometrika 16, 297–334. doi: 10.1007/BF02310555
Cropley, D. H., and Marrone, R. L. (2022). Automated scoring of figural creativity using a convolutional neural network. Psychol. Aesthet. Creat. Arts. Advance online publication. doi: 10.1037/aca0000510
De Dreu, C. K. W., Nijstad, B. A., and Baas, M. (2011). Behavioral activation links to creativity because of increased cognitive flexibility. Soc. Psychol. Personal. Sci. 2, 72–80. doi: 10.1177/1948550610381789
Deerwester, S., Dumais, S. T., Furnas, G. W., Landauer, T. K., and Harshman, R. (1990). Indexing by latent semantic analysis. J. Am. Soc. Inf. Sci. 41, 391–407. doi: 10.1002/(SICI)10974571(199009)41:6_391::AID-ASI13.0.CO
DeYoung, C. G., Carey, B. E., Krueger, R. F., and Ross, S. R. (2016). Ten aspects of the big five in the personality inventory for DSM-5. Pers. Disord. 7, 113–123. doi: 10.1037/per0000170
DeYoung, C. G., Quilty, L. C., and Peterson, J. B. (2007). Between facets and domains: 10 aspects of the big five. J. Pers. Soc. Psychol. 93, 880–896. doi: 10.1037/0022-3514.93.5.880
Dollinger, S. J., Urban, K. K., and James, T. A. (2004). Creativity and openness: further validation of two creative product measures. Creat. Res. J. 16, 35–47. doi: 10.1207/s15326934crj1601_4
Dumas, D., Doherty, M., and Organisciak, P. (2020a). The psychology of professional and student actors: creativity, personality, and motivation. PLoS One 15:e0240728. doi: 10.1371/journal.pone.0240728
Dumas, D., and Dunbar, K. N. (2014). Understanding fluency and originality: a latent variable perspective. Think. Skills Creat. 14, 56–67. doi: 10.1016/j.tsc.2014.09.003
Dumas, D., Organisciak, P., and Doherty, M. (2020b). Measuring divergent thinking originality with human raters and text-mining models: a psychological comparison of methods. Psychol. Aesthet. Creat. Arts 15, 645–663. doi: 10.1037/aca0000319
Dumas, D. G., and Strickland, A. L. (2018). From book to bludgeon: a closer look at unsolicited malevolent responses on the alternate uses task. Creat. Res. J. 30, 439–450. doi: 10.1080/10400419.2018.1535790
Feist, G. J. (1998). A meta-analysis of personality in scientific and artistic creativity. Personal. Soc. Psychol. Rev. 2, 290–309. doi: 10.1207/s15327957pspr0204_5
Forster, E. A., and Dunbar, K. N. (2009). Creativity evaluation through latent semantic analysis. In N. A. Taatgen and H. Rijnvan (Eds.), Proceedings of the 31th Annual Conference of the Cognitive Science Society (pp. 602–607). Austin: Cognitive Science Society.
Forthmann, B., Bürkner, P. C., Szardenings, C., Benedek, M., and Holling, H. (2019). A new perspective on the multidimensionality of divergent thinking tasks. Front. Psychol. 10:985. doi: 10.3389/fpsyg.2019.00985
Forthmann, B., Holling, H., Zandi, N., Gerwig, A., Çelik, P., Storme, M., et al. (2017). Missing creativity: the effect of cognitive workload on rater (dis-)agreement in subjective divergent-thinking scores. Think. Skills Creat. 23, 129–139. doi: 10.1016/j.tsc.2016.12.005
Forthmann, B., Oyebade, O., Ojo, A., Günther, F., and Holling, H. (2019). Application of latent semantic analysis to divergent thinking is biased by elaboration. J. Creat. Behav. 53, 559–575. doi: 10.1002/jocb.240
Forthmann, B., Szardenings, C., and Holling, H. (2020). Understanding the confounding effect of fluency in divergent thinking scores: revisiting average scores to quantify artifactual correlation. Psychol. Aesthet. Creat. Arts 14, 94–112. doi: 10.1037/aca0000196
Goldberg, L. R. (1990). An alternative "description of personality": the big-five factor structure. J. Pers. Soc. Psychol. 59, 1216–1229. doi: 10.1037/0022-3514.59.6.1216
Goldberg, L. R. (1993). The structure of phenotypic personality traits. Am. Psychol. 48, 26–34. doi: 10.1037/0003-066X.48.1.26
Gonçalves, M., and Cash, P. (2021). The life cycle of creative ideas: towards a dual-process theory of ideation. Des. Stud. 72:100988. doi: 10.1016/j.destud.2020.100988
Grajzel, K., Dumas, D., and Acar, S. (2022). Are the verbal TTCT forms actually interchangeable? J. Creat. Behav. 56, 629–646. doi: 10.1002/jocb.556
Gray, K., Anderson, S., Chen, E. E., Kelly, J. M., Christian, M. S., Patrick, J., et al. (2019). “Forward flow”: a new measure to quantify free thought and predict creativity. Am. Psychol. 74, 539–554. doi: 10.1037/amp0000391
Griffith, J. (2022). Flexibility, creativity, and visual storytelling: an interview with graphic novelist and voices from the middle cover artist Sophie Escabasse. Voices from the Middle 29, 10–13.
Grohman, M. G., Ivcevic, Z., Silvia, P., and Kaufman, S. B. (2017). The role of passion and persistence in creativity. Psychol. Aesthet. Creat. Arts 11, 376–385. doi: 10.1037/aca0000121
Gu, C., Han, M., Li, C., Bie, Z., Tan, Y., Xue, Y., et al. (2020). The effect of environmental cues and motivation on creative idea generation. Creat. Innov. Manag. 29, 581–596. doi: 10.1111/caim.12403
Gube, M., and Lajoie, S. (2020). Adaptive expertise and creative thinking: a synthetic review and implications for practice. Think. Skills Creat. 35:100630. doi: 10.1016/j.tsc.2020.100630
Guilford, J. P. (1968). Intelligence has three facets. There are numerous intellectual abilities, but they fall neatly into a rational system. Science 160, 615–620. doi: 10.1126/science.160.3828.615
Guilford, J. P. (1975). Varieties of creative giftedness, their measurement and development. Gift. Child Q. 19, 107–121. doi: 10.1177/001698627501900216
Guo, J., Ge, Y., and Pang, W. (2019). The underlying cognitive mechanisms of the rater effect in creativity assessment: the mediating role of perceived semantic distance. Think. Skills Creat. 33:100572. doi: 10.1016/j.tsc.2019.100572
Hagtvedt, L. P., Dossinger, K., Harrison, S. H., and Huang, L. (2019). Curiosity made the cat more creative: specific curiosity as a driver of creativity. Organ. Behav. Hum. Decis. Process. 150, 1–13. doi: 10.1016/j.obhdp.2018.10.007
Hancock, G. R., and Mueller, R. O. (2001). Rethinking construct reliability within latent variable systems. In R. Cudeck, S. Toitdu, and D. Sörbom (Eds.), Structural Equation Modeling: Present and Future – A Festschrift in Honor of Karl Jöreskog (pp. 195–216). Lincolnwood, IL: Scientific Software International.
Harbinson, J., and Haarman, H. (2014). “Automated scoring of originality using semantic representations.” in Proceedings of the Annual Meeting of the Cognitive Science Society, 36. Retrieved from https://escholarship.org/uc/item/17t264nk
Hass, R. W. (2017). Tracking the dynamics of divergent thinking via semantic distance: analytic methods and theoretical implications. Mem. Cogn. 45, 233–244. doi: 10.3758/s13421-016-0659-y
Hass, R. W., and Beaty, R. E. (2018). Use or consequences: Probing the cognitive difference between two measures of divergent thinking. Front. Psychol. 9:2327. doi: 10.3389/fpsyg.2018.02327
Heinen, D. J. P., and Johnson, D. R. (2018). Semantic distance: an automated measure of creativity that is novel and appropriate. Psychol. Aesthet. Creat. Arts 12, 144–156. doi: 10.1037/aca0000125
Hu, L., and Bentler, P. M. (1999). Cutoff criteria for fit indexes in covariance structure analysis: conventional criteria versus new alternatives. Struct. Equ. Model. 6, 1–55. doi: 10.1080/10705519909540118
Huang, N., Chang, Y., and Chou, C. (2020). Effects of creative thinking, psychomotor skills, and creative self-efficacy on engineering design creativity. Think. Skills Creat. 37:100695. doi: 10.1016/j.tsc.2020.100695
Hutchison, K. A., Balota, D. A., Cortese, M. J., and Watson, J. M. (2008). Predicting semantic priming at the item level. Q. J. Exp. Psychol. 61, 1036–1066. doi: 10.1080/17470210701438111
John, O. P., Naumann, L. P., and Soto, C. J. (2008). “Paradigm shift to the integrative big five trait taxonomy: history, measurement, and conceptual issues” in Handbook of Personality: Theory and Research. eds. O. P. John, R. W. Robins, and L. A. Pervin (New York, NY: Guilford), 114–158.
John, O. P., and Srivastava, S. (1999). “The big five trait taxonomy: history, measurement, and theoretical perspectives” in Handbook of Personality: Theory and Research. eds. L. A. Pervin and O. P. John (New York, NY: Guilford Press), 102–138.
Johnson, D. R., Cuthbert, A. S., and Tynan, M. E. (2021). The neglect of idea diversity in creative idea generation and evaluation. Psychol. Aesthet. Creat. Arts 15, 125–135. doi: 10.1037/aca0000235
Kanlı, E. (2020). “Assessment of creativity: theories and methods.” in Creativity-A Force to Innovation. IntechOpen.
Karwowski, M., Jankowska, D. M., Brzeski, A., Czerwonka, M., Gajda, A., Lebuda, I., et al. (2021). Delving into creativity and learning. In V. P. Glăveanu, I. J. Ness, and C. Saint Laurentde (Eds.), Creative Learning in Digital and Virtual Environments: Opportunities and Challenges of Technology-Enabled Learning and Creativity. New York, NY: Routledge/Taylor & Francis Group, 7–29.
Kaufman, J. C., Baer, J., Cole, J. C., and Sexton, J. D. (2008). A comparison of expert and nonexpert raters using the consensual assessment technique. Creat. Res. J. 20, 171–178. doi: 10.1080/10400410802059929
Keleş, T., and Yazgan, Y. (2022). Indicators of gifted students’ strategic flexibility in non-routine problem solving. Int. J. Math. Educ. Sci. Technol. 53, 2797–2818. doi: 10.1080/0020739X.2022.2105760
Kenett, Y. N. (2019). What can quantitative measures of semantic distance tell us about creativity? Curr. Opin. Behav. Sci. 27, 11–16. doi: 10.1016/j.cobeha.2018.08.010
Kenett, Y. N., Beaty, R. E., Silvia, P. J., Anaki, D., and Faust, M. (2016). Structure and flexibility: investigating the relation between the structure of the mental lexicon, fluid intelligence, and creative achievement. Psychol. Aesthet. Creat. Arts 10, 377–388. doi: 10.1037/aca0000056
Kenett, Y. N., and Faust, M. (2019). A semantic network cartography of the creative mind. Trends Cogn. Sci. 23, 271–274. doi: 10.1016/j.tics.2019.01.007
Kenett, Y. N., Lvey, O., Kenett, D. Y., Stanley, E. H., Faust, M., and Halvin, S. (2018). Flexibility of thought in high creativity individuals presented by percolation analysis. Proc. Natl. Acad. Sci. U. S. A. 115, 867–872. doi: 10.1073/pnas.1717362115
Kim, K. H. (2006). Can we trust creativity tests? A review of the Torrance tests of creative thinking (TTCT). Creat. Res. J. 18, 3–14. doi: 10.1207/s15326934crj1801_2
King, L. A., McKee Walker, L., and Broyles, S. J. (1996). Creativity and the five-factor model. J. Res. Pers. 30, 189–203. doi: 10.1006/jrpe.1996.0013
Kroes, M. C., and Fernández, G. (2012). Dynamic neural systems enable adaptive, flexible memories. Neurosci. Biobehav. Rev. 36, 1646–1666. doi: 10.1016/j.neubiorev.2012.02.014
Kumar, A. A., Balota, D. A., and Steyvers, M. (2020). Distant connectivity and multiple-step priming in large-scale semantic networks. J. Exp. Psychol. Learn. Mem. Cogn. 46, 2261–2276. doi: 10.1037/xlm0000793
Landreneau, E., and Halpin, G. (1978). The influence of modeling on Children’s creative performance. J. Educ. Res. 71, 137–139. doi: 10.1080/00220671.1978.10885055
Manning, C. D., Raghavan, P., and Schutze, H. (2008). Introduction to Information Retrieval. Cambridge, UK: Cambridge University Press.
Mansfield, R. S., Busse, T. V., and Krepelka, E. J. (1978). The effectiveness of creativity training. Rev. Educ. Res. 48, 517–536. doi: 10.3102/00346543048004517
Martindale, C. (1999). “Biological bases of creativity” in Handbook of Creativity. ed. R. Sternberg (Cambridge: University Press), 137–152.
Mastria, S., Agnoli, S., Zanon, M., Acar, S., Runco, M. A., and Corazza, G. E. (2021). Clustering and switching in divergent thinking: neurophysiological correlates underlying flexibility during idea generation. Neuropsychologia 158:107890. doi: 10.1016/j.neuropsychologia.2021.107890
McCrae, R. R. (1987). Creativity, divergent thinking, and openness to experience. J. Pers. Soc. Psychol. 52, 1258–1265. doi: 10.1037/0022-3514.52.6.1258
McCrae, R. R., and John, O. P. (1992). An introduction to the five-factor model and its applications. J. Pers. 60, 175–215. doi: 10.1111/j.1467-6494.1992.tb00970.x
McNeish, D., and Wolf, M. G. (2020). Dynamic fit index cutoffs for confirmatory factor analysis models.
Milgram, R. M. (ed.) (1991). Counseling gifted and talented children: A guide for teachers, counselors, and parents. Ablex Publishing.
Moeller, J., Eccles, J. S., Salmela-Aro, K., Dietrich, J., Schneider, B., and Grassinger, R. (2015). “Passion and motivation” in International Encyclopedia of Social and Behavioral Sciences. Vol. 17. ed. J. D. Wright. 2nd ed (Amsterdam, Netherland: Elsevier Scientific Publ. Co.), 570–576.
Mouchiroud, C., &, Lubart, T. (2001). Children's original thinking: an empirical examination of alternative measures derived from divergent thinking tasks. J. Genet. Psychol., 162, 382–401. doi: 10.1080/00221320109597491
Murray, S., Liang, N., Brosowsky, N., and Seli, P. (2021). What are the benefits of mind wandering to creativity? Psychology of aesthetics, creativity, and the arts. Advance online publication.
Muthén, L. K., and Muthén, B. O. (2007). Mplus User’s Guide. 6th Edn. Los Angeles, CA: Muthén & Muthén.
Nijstad, B. A., De Dreu, C. K., Rietzschel, E. F., and Bass, M. (2010). The dual pathway to creativity model: creativity ideation as a function of flexibility and persistence. Eur. Rev. Soc. Psychol. 21, 34–77. doi: 10.1080/10463281003765323
Olson, J. A., Nahasb, J., Chmoulevitchb, D., Cropperc, S. J., and Webbc, M. E. (2021). Naming unrelated words predicts creativity. Proc. Natl. Acad. Sci. U. S. A. 118, 1–6. doi: 10.1073/pnas.2022340118
Oniani, D. (2020). Cosine similarity and its applications in the domains of artificial intelligence.
Organisciak, P., and Dumas, D. (2020). Open Creativity Scoring [Computer Software]. Denver, CO: University of Denver.
Paulus, P. B., and Brown, V. R. (2007). Toward more creative and innovative group idea generation: a cognitive-social-motivational perspective of brainstorming. Soc. Personal. Psychol. Compass 1, 248–265. doi: 10.1111/j.1751-9004.2007.00006.x
Pennington, J., Socher, R., and Manning, C. (2014). “Glove: global vectors for word representation.” in Proceedings of the 2014 Conference on Empirical Methods in Natural Language Processing (Association for Computational Linguistics), 1532–1543.
Peterson, D. R., and Pattie, M. W. (2022). Think outside and inside the box: The role of dual-pathway divergent thinking in creative idea generation. Creat. Res. J. 1–19. doi: 10.1080/10400419.2022.2110738
Plucker, J. A., Beghetto, R. A., and Dow, G. T. (2004). Why isn’t creativity more important to educational psychologist? Potentials, pitfalls and future directions in creativity research. Educ. Psychol. 39, 83–96. doi: 10.1207/s15326985ep3902_1
Pranoto, B. E., and Afrilita, L. K. (2019). The organization of words in mental lexicon: evidence from word association test. Teknosastik 16, 26–33. doi: 10.33365/ts.v16i1.130
Preiss, D. D. (2022). Metacognition, mind wandering, and cognitive flexibility: understanding creativity. J. Intell. 10:69. doi: 10.3390/jintelligence10030069
Preiss, D. D., and Cosmelli, D. (2017). “Mind wandering, creative writing, and the self” in The Creative Self: Effect of Beliefs, Self-efficacy, Mindset, and Identity. eds. M. Karwowski and J. C. Kaufman (Cambridge, MA: Elsevier Academic Press), 301–313.
Primi, R., Silvia, P. J., Jauk, E., and Benedek, M. (2019). Applying many-facet Rasch modeling in the assessment of creativity. Psychol. Aesthet. Creat. Arts 13, 176–186. doi: 10.1037/aca0000230
Rapp, D. N., and Samuel, A. G. (2002). A reason to rhyme: Phonological and semantic influences on lexical access. J. Exp. Psychol. Learn. Mem. Cogn. 28, 564–571. doi: 10.1037/0278-7393.28.3.564
Reiter-Palmon, R., Forthmann, B., and Bardot, B. (2019). Scoring divergent thinking tests: a review and systematic framework. Psychol. Aesthet. Creat. Arts 13, 144–152. doi: 10.1037/aca0000227
Ritter, S. M., Damian, R. I., Simonton, D. K., van Baaren, R. B., Strick, M., Derks, J., et al. (2012). Diversifying experiences enhance cognitive flexibility. J. Exp. Soc. Psychol. 48, 961–964. doi: 10.1016/j.jesp.2012.02.009
Runco, M. A. (1986a). Divergent thinking and creative performance in gifted and nongifted children. Educ. Psychol. Meas. 46, 375–384. doi: 10.1177/001316448604600211R
Runco, M. A. (1986b). Divergent thinking, creativity, and giftedness. J. Psychol. 120, 345–352. doi: 10.1080/00223980.1986.9712632
Runco, M. A. (1993). Divergent thinking, creativity, and giftedness. Gift Child Q. 37, 16–22. doi: 10.1177/001698629303700103
Runco, M. A. (2010). “Divergent thinking, creativity, and ideation,” in The Cambridge Handbook of Creativity. eds. J. C. Kaufman and R. J. Sternberg (Cambridge University Press), 413–446.
Runco, M. A. (2013). Is there a creativity crisis? Presentation to the Mississippi Association for Gifted Children, Hattiesburg, MS.
Runco, M. A., and Acar, S. (2012). Divergent thinking as an indicator of creative potential. Creat. Res. J. 24, 66–75. doi: 10.1080/10400419.2012.652929
Runco, M. A., and Okuda, S. M. (1991). The instructional enhancement of the flexibility and originality scores of divergent thinking tests. Appl. Cogn. Psychol. 5, 435–441. doi: 10.1002/acp.2350050505
Runco, M. A., and Pritzker, S. R. (2020). Encyclopedia of Creativity. Cambridge, MA: Academic Press.
Said-Metwaly, S., Fernández-Castilla, B., Kyndt, E., and Van den Noortgate, W. (2020). Testing conditions and creative performance: meta-analyses of the impact of time limits and instructions. Psychol. Aesthet. Creat. Arts 14, 15–38. doi: 10.1037/aca0000244
Shore, B. M. (2000). “Metacognition and flexibility: qualitative differences in how gifted children think” in Talents Unfolding: Cognition and Development. eds. R. C. Friedman and B. M. Shore (Washington, D.C.: American Psychological Association), 167–187.
Silvia, P. J. (2011). Subjective scoring of divergent thinking: examining the reliability of unusual uses, instances, and consequences tasks. Think. Skills Creat. 6, 24–30. doi: 10.1016/j.tsc.2010.06.001
Silvia, P. J., Martin, C., and Nusbaum, E. C. (2009). A snapshot of creativity: evaluating a quick and simple method for assessing divergent thinking. Think. Skills Creat. 4, 79–85. doi: 10.1016/j.tsc.2009.06.005
Silvia, P. J., Winterstein, B. P., Willse, J. T., Barona, C. M., Cram, J. T., Hess, K. I., et al. (2008). Assessing creativity with divergent thinking tasks: exploring the reliability and validity of new subjective scoring methods. Psychol. Aesthet. Creat. Arts 2, 68–85. doi: 10.1037/1931-3896.2.2.68
Torrance, E. P. (1974). The Torrance Tests of Creative Thinking. Norms-technical Manual. Research Edition. Verbal Tests Forms A and B. Figural Tests, Forms A and B. Princeton, NJ: Personnel Press.
Torrance, P. E. (1998). Torrance Tests of Creative Thinking. Bensenville, IL: Scholastic Testing Services, Inc.
Treffinger, D. J. (1985). “Review of the Torrance tests of creative thinking” in The Ninth Mental Measurements Yearbook. ed. J. V. Mitchell Jr. (Lincoln, NE: Buros Institute of Mental Measurements), 1632–1634.
Yu, C., Beckmann, J. F., and Birney, D. P. (2019). Cognitive flexibility as a meta-competency. Stud. Psychol. 40, 563–584. doi: 10.1080/02109395.2019.1656463
Zabelina, D. L., Friedman, N. P., and Andrews-Hanna, J. (2019). Unity and diversity of executive functions in creativity. Conscious. Cogn. 68, 47–56. doi: 10.1016/j.concog.2018.12.005
Zabelina, D. L., and Robinson, M. D. (2010). Creativity as flexible cognitive control. Psychol. Aesthet. Creat. Arts 4, 136–143. doi: 10.1037/a0017379
Zeng, L., Proctor, R. W., and Salvedy, G. (2011). Can traditional divergent thinking tests be trusted in measuring and predicting real-world creativity? Creat. Res. J. 23, 24–37. doi: 10.1080/10400419.2011.545713
Keywords: divergent thinking, flexibility, text-mining, psychometrics, creativity
Citation: Grajzel K, Acar S, Dumas D, Organisciak P and Berthiaume K (2023) Measuring flexibility: A text-mining approach. Front. Psychol. 13:1093343. doi: 10.3389/fpsyg.2022.1093343
Edited by:
Rogelio Puente-Diaz, Anahuac University of North Mexico, MexicoReviewed by:
Soo Eun Chae, Gangneung–Wonju National University, Republic of KoreaHaijun Duan, Shaanxi Normal University, China
Copyright © 2023 Grajzel, Acar, Dumas, Organisciak and Berthiaume. This is an open-access article distributed under the terms of the Creative Commons Attribution License (CC BY). The use, distribution or reproduction in other forums is permitted, provided the original author(s) and the copyright owner(s) are credited and that the original publication in this journal is cited, in accordance with accepted academic practice. No use, distribution or reproduction is permitted which does not comply with these terms.
*Correspondence: Katalin Grajzel, ✉ katalin.grajzel@du.edu