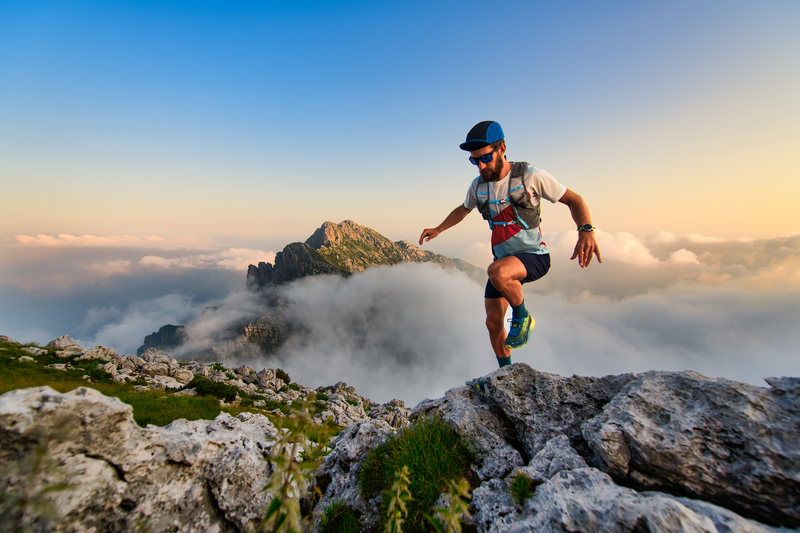
94% of researchers rate our articles as excellent or good
Learn more about the work of our research integrity team to safeguard the quality of each article we publish.
Find out more
ORIGINAL RESEARCH article
Front. Psychol. , 23 December 2022
Sec. Cognition
Volume 13 - 2022 | https://doi.org/10.3389/fpsyg.2022.1076735
This article is part of the Research Topic Brain Health: Risk, Resilience and Reserve View all 7 articles
Daily driving is a multi-faceted, real-world, behavioral measure of cognitive functioning requiring multiple cognitive domains working synergistically to complete this instrumental activity of daily living. As the global population of older adult continues to grow, motor vehicle crashes become more frequent among this demographic. Cognitive reserve (CR) is the brain’s adaptability or functional robustness despite damage, while brain reserve (BR) refers the structural, neuroanatomical resources. This study examined whether CR and BR predicted changes in adverse driving behaviors in cognitively normal older adults. Cognitively normal older adults (Clinical Dementia Rating 0) were enrolled from longitudinal studies at the Knight Alzheimer’s Disease Research Center at Washington University. Participants (n = 186) were ≥65 years of age, required to have Magnetic Resonance Imaging (MRI) data, neuropsychological testing data, and at least one full year of naturalistic driving data prior to the beginning of COVID-19 lockdown in the United States (March 2020) as measured by Driving Real World In-vehicle Evaluation System (DRIVES). Findings suggest numerous changes in driving behaviors over time were predicted by increased hippocampal and whole brain atrophy, as well as lower CR scores as proxied by the Wide Range Achievement Test 4. These changes indicate that those with lower BR and CR are more likely to reduce their driving exposure and limit trips as they age and may be more likely to avoid highways where speeding and aggressive maneuvers frequently occur.
Motor vehicle crashes are the eight leading causes of death globally, across all age groups (Rosen et al., 2022). In the United States, motor vehicle crashes are the second leading cause of death from unintentional injuries among adults aged 65+ (Rosen et al., 2022). Driving is the ultimate expression of functioning requiring multiple domains working concertedly (sensory, motor, visual, cognitive processes) to complete this complex instrumental activity of daily living (IADL; Babulal, 2022). The risk of a crash, decline in health, or family concerns may influence the decision to retire from driving (Campbell et al., 1993; O’neill et al., 2000). Driving retirement may reduce the risk of crashes and resulting fatalities; however, this creates an elevated risk of psychosocial sequalae such as depression, isolation, and admission to institutional care (Freeman et al., 2006; Edwards et al., 2009; Qin et al., 2020). To reduce the risk of motor vehicle crash fatalities without increasing driving retirement, some older adults may begin to regulate driving behaviors to maintain their independence. This may include trip reduction, driving at specific times, or avoiding highways/high-traffic roads. Due to an anticipated growth of older adult drivers over the next three decades, it is more important to consider options to preserve driver safety and monitor decline in driving behavior (Pollack, 2005).
Cognitive reserve (CR) and brain reserve (BR) are promising constructs posited to explain why differences in aging outcomes persist in the presence of cognitive decline or neurological diseases like dementia. CR is considered to be the breadth of memory and thinking abilities, including flexibility and adaptability of attentional processes (conscious and subconscious) commonly subserving daily function (i.e., work, play, and leisure; Stern et al., 2020). Conversely, BR refers to physical and neuroanatomical structures of the brain (Stern et al., 2020). More simply, CR can be conceptualized as the brain’s software, while BR is the hardware, both functioning synergistically to support human function. Since BR can be quantitatively measured via brain volume or neuronal count, it is often simpler to compare BR values than CR in aging studies. CR measurement relies on common proxies (e.g., education, literacy, and occupational attainment) that evaluates sociobehavioral components which may contribute to individual reserve (Tucker and Stern, 2011; Stern et al., 2020). However, these proxies are often conducted via collateral source or self-report and may not allow for the objective investigation of CR’s impact on complex tasks such as driving.
Driving provides a novel medium to examine the breadth and depth of cognitive functioning. In a study examining IADL and cognition, better scores on timed measures were associated with increased scores on the Mattis Dementia Rating Scale-Second Edition, while increased awareness of self-reported IADL performance was also associated with higher levels of CR (Suchy et al., 2011). Older adults with high CR seem to have an increased awareness of their deficits and can implement compensatory strategies early, which may be effective during more serious decline (Suchy et al., 2011). A recent study from Milan, Italy assessed healthy, community-residing older adults’ fitness to drive and found that higher CR (education as proxy) was associated with improved driving skills such as processing speed and decision speed when assessing cognitively normal older adults’ fitness to drive (Balzarotti et al., 2021). Another study that investigated age-related decline on driving via a driving simulator demonstrated that older adults with higher scores on a cognitive battery assessing logical reasoning, spatial visualization, processing speed, executive control, and perceptual-motor skills were able to alter their speed and time-to-stop (headway) more quickly (Andrews and Westerman, 2012). One proposed explanation is that older adults may strategically compensate, selecting driving conditions and parameters that reduce task demands, such as reducing night driving or avoiding heavy traffic areas. Since awareness of deficits is associated with CR level, performance in daily living tasks may also be impacted by CR. However, generalizability to daily driving behavior is limited since the extant literature assessed how simulated driving and road testing are impacted by cognitive reserve. There is a significant gap in the literature that examines complex human function (i.e., IADLs) and CR/BR beyond prototypical neuropsychological tasks. Additionally, prior studies have sought to determine differences in cognitive function that may contribute to adverse driving behaviors among cognitively normal older adults with and without preclinical Alzheimer’s disease and found no significant difference (Roe et al., 2017a,b). Furthermore, research has investigated associations between several cognitive substrates and driving decline in relation to driving behavior and have found unreliable relationships (Aschenbrenner et al., 2022). Therefore, the present study seeks to fill a gap in literature that is necessary to better understand if CR and BR constructs contribute to changes of the complex IADL of driving. The present study chose not to propose mechanisms that could impact driving ability since naturalistic driving is a novel and highly variable task that is measured in real time with unpredictable events that influence driver response.
Recent advances in telematics and global position systems (GPS) technology have produced affordable and unobtrusive dataloggers that allow tracking of individual vehicles and the acquisition of daily driving data via velocity, spatial, and temporal characteristics (Babulal et al., 2016). In this study, we investigate the relationship between CR, vis-a-vie a reading fluency task and longitudinal naturalistic driving behaviors measured via an in-vehicle data logger. We also investigate the relationship between daily driving and BR evaluated by hippocampal and whole brain volume quantified via magnetic resonance imaging (MRI). We hypothesize that older drivers with lower CR and BR at baseline will demonstrate less self-regulation (e.g., lower miles driven) and have an increase in adverse driving behavior over time, such as over speeding and hard braking.
Participants were enrolled in longitudinal aging and driving studies at the Knight Alzheimer’s Disease Research Center (ADRC) at Washington University School of Medicine. Participants included in this study were at least 65 years of age or older, were cognitively normal at baseline as evaluated by a 0 on the Clinical Dementia Rating (CDR®; Morris, 1993), and did not progress upon subsequent follow-up assessment. Additionally, participants had their magnetic resonance imaging (MRI) within 2 years of enrollment in the longitudinal driving study. Participants self-reported driving at least once per week and had naturalistic driving data available for at least for one full year to account for seasonality and daylight time changes. Additionally, longitudinal methods were implemented to investigate intraindividual change over time as one continues to drive. All data were limited to 3/31/2020 to exclude the effects of pandemic guidelines (e.g., lockdown, restrictions, shelter-in-place) on driving behavior (Roe et al., 2021). Additionally, all participants either self-identified as white or Black for race.
A datalogger (Azuga G2 Tracking device) was installed into each participant’s vehicle’s onboard diagnostic port (OBDII). Driving behavior was continuously collected and for a given “trip,” defined as ignition start to ignition off, data collected includes the date, time, vehicle speed, latitude and longitude, and adverse events such as speeding, hard braking, and sudden acceleration. We have used the Driving Real-World In-Vehicle Evaluation System (DRIVES; Babulal et al., 2016, 2019; Roe et al., 2019) to examine various metrics, including the total number of trips, the average distance traveled, number of night trips taken, number of trips across multiple distances from their home, idle time, over speeding, hard braking, and sudden acceleration. Idle time reflects the amount of time a vehicle is started in park but not moving. Over speeding events are defined as a speed six MPH or more over the posted speed limit. Hard braking is defined as a sudden decrease in speed of eight MPH or more per second. Sudden acceleration defined as an increase in speed of eight MPH or more per second. Over speeding, hard braking, and speeding were conceptualized as adverse driving behavior.
The Word Reading subtest (the blue form) of the Wide Range Achievement Test 4 (WRAT 4) served as a proxy for cognitive reserve (Tucker and Stern, 2011; Barulli and Stern, 2013). The Word Reading subtest is administered annually during an office testing session. In the subtest, the participant is asked to read 55 words aloud to the administrator. The participant reads until they have incorrectly pronounced 10 words consecutively at which point the task is discontinued or have finished the list (Wilkinson and Robertson, 2006). WRAT 4 scores were compared via dichotomized groups with those performing amongst the top two thirds of the sample being considered high performers and those performing amongst the bottom third being considered poor performers. The WRAT Reading subtest has been utilized, validated, and commonly used as a proxy for CR amongst older adults in previous research (Siedlecki et al., 2009; Brickman et al., 2011; Baker et al., 2017).
An MRI was completed on a 3 T Tesla using a research imaging protocol that is based upon the Alzheimer’s Disease Neuroimaging Initiative (ADNI), which includes a high-resolution T1 MPRAGE for assessment of brain structures to produce normalized whole brain volume (WBV) and hippocampal volume (HV) measurements. Prior to analysis, HV was normalized to account for differences in head size. The procedure consisted of computing the mean intracranial volume (ICV) for the sample, and then conducting a regression analysis with ICV as the sole independent variable and participants’ HV (the sum of right and left HV) as the dependent variable. The β-weight was then used to compute participants’ normalized HV using the following equation: normalized HV = raw HV – [β-weight × (participant’s ICV – sample mean ICV); Buckner et al., 2004]. These elements are part of standard clinical brain MRIs. All MRIs were reviewed by board-certified neuroradiologists. WBV and HV were determined by the neuroradiologist based on standard clinical assessment. Details of the structural brain MRI and radiological assessment are available in prior publications (Koenig et al., 2021, 2022). WBV and HV were each assessed dichotomously, comparing the top two thirds with the bottom third where the lower group represented increased atrophy.
All participants were administered an annual comprehensive clinical and cognitive assessment. A trained clinician rated the participant for presence and severity of dementia symptoms using the CDR® (Morris, 1993). The cognitive battery consists of several assessments of cognitive functioning and included the following assessments: the free recall test from the Free and Cued Selective Reminding test (Grober et al., 1988) to measure episodic memory; Trail Making Parts A and B (Armitage, 1946) to measure processing speed, and Animal Naming (Tombaugh et al., 1999) to measure semantic fluency. Each cognitive test was z-scored to the baseline cognitive assessment, defined as the cognitive assessment nearest a participant’s enrollment into the driving study, and were averaged to form a composite score.
The reserve markers were dichotomized using the first tertile and the top two tertiles to classify participants into lower/higher risk groups, respectively. Descriptive statistics summarized key demographics variables and compared risk groups using independent t-test or χ2 test, McNemar’s test assessed the concordance of the three markers for classifying participants into lower/higher risk. Longitudinal data analyses assumed a linear relationship between aggregated monthly naturalistic driving variables and the markers. A random coefficients model (linear mixed model) was used to predict the average rate of change in the driving outcomes based on the groups adjusting for age (centered at the sample mean), education, and gender. This model allowed y-intercepts and slopes (monthly rate of change) to vary randomly between participants and fitted a separate regression line for each participant. The y-intercept generated from the linear mixed model estimated the mean of a driving variable at the beginning of data collection (baseline). The interaction between marker group and time was considered for testing for slope difference over time, and the model examined whether there was a difference at the y-intercept. Predicted means of the driving variables of each participant were obtained from the random coefficients model, and locally weighted scatter plot smoothing was applied to visualize the estimated y-intercept and slope change over time between groups. Sensitivity analysis was conducted to determine if accounting for education would impact the slope models. Akaike information criterion (AIC) as a model fit statistics was compared between the random coefficients model with and without including education. The random coefficients model including education yielded smaller AIC and thus was selected. All statistical analyses were two-tailed at a significance level of 0.05 and performed with SAS 9.4 (SAS Institute, Cary, NC).
A total of 186 older adults met inclusion criteria. On average, participants were in their early 70’s, well-educated, non-Hispanic white, and had similar sex distribution (Table 1). Longitudinal driving was present for over 4 years with a mean centering around 2 years. The WRAT was moderately correlated with CR (r = 0.52; p = <0.0001) but not the two measures of BR (HV [r = −0.07; p = 0.3009] WBV [r = 0.02; p = 0.7264]). There was a moderate correlation between CR and BR (r = 0.61; p = <0.0001). There were no statistically significant differences between males and females found for the WRAT (p = 0.7385), HV (p = 0.3155), and WBV (p = 0.6774). Two-sample t-test showed that no significant differences across age for high and low performers on the WRAT (p = 0.9367). However, a statistically significant difference was found across age between those with lower and higher values of HV (p < 0.0001) and WBV (p < 0.0001). Significant differences were found for education level between high and low performers on the WRAT (p < 0.0001), as well as those with low and high values of HV (p = 0.0258). Lastly, statistically significant differences for cognitive composite score were found for those with low and high values of only WBV (p = 0.0146) but not for WRAT 4 (p = 0.0844) or HV (p = 0.1493). The random coefficient models were conducted in which numerous slope models for driving metrics were found to be statistically significant (Table 2).
Table 1. Baseline demographics (N = 186)*.
Lower HV and WBV were found to predict increased idle time across follow-up (p = 0.0005; p < 0.0001), compared to those without atrophy (Figure 1). Additionally, lower performers on the WRAT 4 were also found to have increased idle time (p = 0.0012) compared to high performers. Participants with lower HV and WBV experienced a significant decrease in over speeding at a greater rate of change compared to those without HV or normalized WBV atrophy (p = 0.0035; p = 0.0003). Poor performers on the WRAT 4 also showed a significant decrease in over speeding compared to high performers (p = 0.0035). Poor performers on the WRAT 4 showed a statistically significant decrease in hard braking over time (p = 0.0180), while WRAT 4 high performers showed little to no change in hard braking behavior over time. Comparatively, decreased HV and WBV were found to predict significant decreases in adverse driving behaviors over time (p = 0.0016; p < 0.0001). Those with decreased HV and WBV show a greater change longitudinally. The same was found for poor performers on the WRAT 4 (p = 0.0024).
Figure 1. Longitudinal change in adverse driving outcomes across groups where x-axis represents the number of months (0–50) and y-axis represents the predicted mean in driving outcome (A–I).
While both high and low performers across HV and WBV showed a decrease in trips taken during the day, those with an absence of atrophy showed a steeper decline in daytime trips over time (p < 0.0001; p < 0.0001; Figure 2). For WRAT 4 high performers, a steeper decline in daytime trips overtime was also found (p < 0.0001). Conversely, those with increased atrophy in HV and WBV showed a steeper increase in nighttime trips over time (p = 0.0003; p = 0.0002) compared to those with lower levels of atrophy. A steeper increase in nighttime trips overtime was also found (p = 0.0004) for poor performers on the WRAT 4. Although both groups across HV and WBV showed an increase in trips taken daily with variability in start and end time, those with increased atrophy show a more significant increase in variability (p = 0.0455; p = 0.0481). For poor performers on the WRAT 4, a steeper increase in variability of start and end time of trips was found (p = 0.0429).
Figure 2. Longitudinal changed in driving frequency outcomes across groups where x-axis represents the number of months (0–50) and y-axis represents the predicted mean in driving outcome (A–I).
We investigated the relationship between CR performance as proxied by the WRAT 4 and naturalistic driving behavior, assessing a complex IADL as measured via an in-vehicle data logger. We also investigated the relationship between BR evaluated by HV and WBV and daily driving. Numerous changes in daily driving behaviors over an average of 2.15 years were predicted by decreased HV and WBV, and lower CR scores proxied by the WRAT 4. Due to the lack of statistical significance on the cognitive composite with performance on the WRAT, these behavioral changes cannot be explained by cognitive performance resulting from subsequent impairments. Longitudinal changes in daily driving suggests that cognitively normal older adults regulate their driving over time. Those with lower BR and CR are more likely to restrict their driving behavior and adapt their daily behaviors such as trips made during the day, speeding, and hard braking as time progresses.
This finding runs contrary to our hypothesis which posited that lower CR and BR would demonstrate self-regulation and more adverse driving. Instead, our results suggest that older drivers with lower BR and CR are more likely to restrict their driving behaviors compared to their high performer counterparts as demonstrated in over adverse driving, speeding, and trips made during the day. However, low performers also show a higher number of trips taken over time regardless of time, as well as a significant increase in night driving. The increase in night driving may be a result of less traffic or congestion on their roads. With reduced drivers or traffic distractions during nighttime hours, drivers with decreased HV and WBV, and lower CR may feel more comfortable driving. In a recent study that assessed cognitive domain scores and daily driving behavior, individuals with lower attentional control drove less frequently and in smaller proximity to their home but drove more often in their routinely visited areas (Aschenbrenner et al., 2022). Therefore, if individuals perceived night driving as requiring fewer external stimuli, they may be more apt to drive during those times. Previous research investigated the relationship between attentional control and age-related atrophy. Researchers found evidence of decreased responsiveness of cortexes such as dorsolateral prefrontal and parietal regions that are associated with attentional control (Milham et al., 2002). These findings suggest that further investigation is needed to understand CR impact on attentional control and driving behavior changes as associations between cortical function, attentional control, and driving are shown to exist.
As we age, neuronal loss results in atrophy of areas like the hippocampus and cortical regions (O’banion et al., 1994; Morrison and Hof, 1997). Furthermore, results from this study support that BR and CR as proxied by scores on the WRAT 4 may be rather comparable as our results were nearly identical across reserve constructs. This suggests that BR may be more easily assessed through reading fluency tasks. This has been demonstrated in recent research investigating neural efficiency as a predictor of CR and WBV where it was found that CR and WBV can predict neural efficiency (Argiris et al., 2022). Additionally, past research has viewed CR as an index of neuroplasticity (Bartres-Faz and Arenaza-Urquijo, 2011). Due to the complexities of completing MRI in patient population with metal implants or claustrophobia, easily administered tasks of reading fluency may be more efficient.
Past CR and BR research have focused on specific diseases of aging such as Alzheimer’s disease; however longitudinal studies in these pathologies are limited (Groot et al., 2018; Soldan et al., 2020). For example, previous research has shown that individuals in the earliest stages of Alzheimer’s disease (CDR 0.5 and 1) can demonstrate impaired driving in attention-related tasks (Duchek et al., 1998; Stein and Dubinsky, 2011). However, this research was conducted utilizing driving simulators (Stein and Dubinsky, 2011). Additionally, Argiris et al. (2022) investigated behavioral tasks such as reaction time, a large component to daily driving behavior, in a small sample of mixed age groups (young adult and older adult) to identify a new proxy for CR and found that this was positively associated with cortical thickness. While these studies investigate CR from the lens of a simulated IADL, they are limited in their findings as they may not necessarily be generalizable to daily driving which is uncontrolled, dynamically changing, and highly variable. The literature examining CR and BR is unable to speak to possible change over time especially in a cognitively normal older adult sample (Soldan et al., 2020; McQuail et al., 2021). Our present study adds an additional layer to the limited literature by addressing both CR and BR in a cognitively normal sample investigating longitudinal change in a complex IADL with naturalistic data.
The current directions in CR/BR literature have advocated for investigation of more complex and specific proxies to better demonstrate the breadth of reserve (Nilsson and Lövdén, 2018; Stern et al., 2020). Prior research suggests that cognitive tasks are not often associated with BR evincing the need for more sensitive measurement (Neth et al., 2020). Our results indicate that cognitive tasks are not predictive of the difference between groups, with the exception of WBV, providing strong evidence that naturalistic IADLs may be more closely aligned as a suitable proxy for CR than previously used cognitive measures and educational proxies. Due to parallel results between CR and BR in longitudinal changes in driving behavior, future research may also begin to utilize naturalistic driving behavior as a proxy for both CR and BR. However, experts in the field suggest utilizing predictive interaction models between naturalistic driving behavior and brain pathology (Stern et al., 2020).
The results showing a more significant decline in adverse driving behaviors for those with decreased HV and WBV may support the mechanism behind the synaptic pruning theory. This theory suggests that more routine or local processes are completed with greater efficiency due to the elimination of redundant synapses (Stephan et al., 2012; Megias et al., 2018). The elimination of synapses that are redundant does not necessarily infer a lack of learned information but may suggest that more efficient and, quite possibly, stronger synapses are formed for routine or overlearned tasks such as driving. In this study, older drivers with lower brain volume were found to significantly self-regulate their driving behaviors over time which may be attributed to less, but stronger synapses formed due to synaptic pruning. Previous research has shown reduction in brain volume for experts in areas such as playing instruments (Vaguero et al., 2016) or performing ballet (Nigmatullina et al., 2015). The present study was unable to factor in driving history due to possible recall bias which may have further supported the synaptic pruning theory for driving as a highly learned behavior and IADL. Future research should investigate the impact of length of time and frequency of driving history on both CR/BR in relations to driving behaviors.
The present study examined CR and BR in two groups, investigating the low performers (bottom 1/3) with the high performers (top 2/3), however, several limitations exist. It may be important to consider individuals with a range/continuous CR, instead of a dichotomous group (high and low CR). Due to increased participant burden, cost, and MRI radiological considerations was only conducted at baseline. Future research should further investigate BR with additional detail by incorporating longitudinal MRI data. An increased sample size with more diverse representation (race, ethnicity, education) may assist in further generalizability to the larger population since our sample was majority highly educated, non-Hispanic white individuals. An adult’s experience/exposure with driving over many decades may influence the rate of decline over time which was not captured in this study. However, the intraindividual change captured over the data collection period would account for that experience since the older adults are in driving in their own vehicle and home environments. Future research should build on the limited body of literature regarding the area deprivation index (ADI) that is investigating environmental disadvantages across populations. Past work has shown that it may be easier to build and preserve CR in environments fostering physical, social, and cognitively beneficial activities (Vassilaki et al., 2022). Investigating the built and natural environment’s impact on driving and CR could further clarify the relationship between driving behavior changes and CR. Past research has also shown that maintenance of the functional networks associated with an individual’s ability to interpret and respond to external stimuli could preserve driving abilities overtime (Wisch et al., 2022). Additional work could investigate the relationship between CR and functional connectivity to better understand the maintenance of driving ability for the longevity of older adult drivers.
We sought to understand the relationship between reserve on changes in daily driving (an IADL) using a novel naturalistic methodology. The relationship between driving was explored via BR, through WBV and HV, and CR, as proxied by the WRAT 4. Older drivers with lower levels of CR and BR demonstrated an increase in idle time but reduced incidents of over speeding and adverse behavior. Additionally, these drivers demonstrated an increase in the variability of start and end time of their trips, but especially showed an increase in night trips. Their high performer CR and BR counterparts are more likely to restrict their driving during all times of the day. Driving remains a complex task that hundreds of millions of drivers safely complete on a daily basis. Changes in CR and BR may presage decline in driving and could be leveraged as screening to better characterize patterns in behavior among older drivers.
The raw data supporting the conclusions of this article will be made available by the authors, without undue reservation.
The studies involving human participants were reviewed and approved by Washington University Institutional Review Board. The patients/participants provided their written informed consent to participate in this study.
GB and SM designed the study and wrote the manuscript with contributions from all authors. LC performed the data analysis. All authors contributed to the article and approved the submitted version.
This study is supported by the National Institute of Health/National Institute on Aging (grant numbers: AG068183, AG067428, and AG056466) and the BrightFocus Foundation (A2021142S). DC declares that they received additional support from NIA, MoDOT, the Traffic Injury Research Foundation, Medscape, UpToDate, Hoffman La Roche, and GreenValley. The funders were not involved in the study design, collection, analysis, interpretation of data, the writing of this article, or the decision to submit it for publication.
We would like to thank the participants that participate in various DRIVES Lab studies. We would also like to thank all of the DRIVES Lab team members including staff and students that contribute to the development of our research.
The authors declare that the research was conducted in the absence of any commercial or financial relationships that could be construed as a potential conflict of interest.
All claims expressed in this article are solely those of the authors and do not necessarily represent those of their affiliated organizations, or those of the publisher, the editors and the reviewers. Any product that may be evaluated in this article, or claim that may be made by its manufacturer, is not guaranteed or endorsed by the publisher.
Andrews, E. C., and Westerman, S. J. (2012). Age differences in simulated driving performance: compensatory processes. Accid. Anal. Prev. 45, 660–668. doi: 10.1016/j.aap.2011.09.047
Argiris, G., Stern, Y., and Habeck, C. (2022). Neural similarity across task load relates to cognitive reserve and brain maintenance measures on the letter Sternberg task: A longitudinal study.
Armitage, S. G. (1946). An analysis of certain psychological tests used for the evaluation of brain injury. Psychol. Monogr. 60, 1–48. doi: 10.1037/h0093567
Aschenbrenner, A. J., Murphy, S. A., Doherty, J. M., Johnson, A. M., Bayat, S., Walker, A., et al. (2022). Neuropsychological correlates of changes in driving behavior among clinically healthy older adults. J. Gerontol.: Seri. B 77, 1769–1778. doi: 10.1093/geronb/gbac101
Babulal, G. M. (2022). Predicting driving decline and assessing crash risk in a globally aging population. Arq. Neuropsiquiatr. 80, 1–2. doi: 10.1590/0004-282x-anp-2022-e001
Babulal, G. M., Stout, S. H., Benzinger, T. L. S., Ott, B. R., Carr, D. B., Webb, M., et al. (2019). A naturalistic study of driving behavior in older adults and preclinical Alzheimer disease: a pilot study. J. Appl. Gerontol. 38, 277–289. doi: 10.1177/0733464817690679
Babulal, G. M., Traub, C. M., Webb, M., Stout, S. H., Addison, A., Carr, D. B., et al. (2016). Creating a driving profile for older adults using GPS devices and naturalistic driving methodology. F1000Res. 5:2376. doi: 10.12688/f1000research.9608.2
Baker, L. M., Laidlaw, D. H., Cabeen, R., Akbudak, E., Conturo, T. E., Correia, S., et al. (2017). Cognitive reserve moderates the relationship between neuropsychological performance and white matter fiber bundle length in healthy older adults. Brain Imaging Behav. 11, 632–639. doi: 10.1007/s11682-016-9540-7
Balzarotti, S., Biassoni, F., Confalonieri, F., Meinero, C. A., and Ciceri, M. R. (2021). Cognitive reserve and driving-related cognitive abilities in a sample of oldest old drivers undergoing assessment of fitness to drive. J. Appl. Gerontol. 40, 1758–1767. doi: 10.1177/0733464821994703
Bartres-Faz, D., and Arenaza-Urquijo, E. M. (2011). Structural and functional imaging correlates of cognitive and brain reserve hypotheses in healthy and pathological aging. Brain Topogr. 24, 340–357. doi: 10.1007/s10548-011-0195-9
Barulli, D., and Stern, Y. (2013). Efficiency, capacity, compensation, maintenance, plasticity: emerging concepts in cognitive reserve. Trends in cognitive sciences, 17, 502-509.Wilkinson, G. S. & Robertson, G. J. 2006. Wide range achievement test (WRAT4). Lutz, FL: Psychological Assessment Resources.
Brickman, A. M., Siedlecki, K. L., Muraskin, J., Manly, J. J., Luchsinger, J. A., Yeung, L. K., et al. (2011). White matter hyperintensities and cognition: testing the reserve hypothesis. Neurobiol. Aging 32, 1588–1598. doi: 10.1016/j.neurobiolaging.2009.10.013
Buckner, R. L., Head, D., Parker, J., Fotenos, A. F., Marcus, D., Morris, J. C., et al. (2004). A unified approach for morphometric and functional data analysis in young, old, and demented adults using automated atlas-based head size normalization: reliability and validation against manual measurement of total intracranial volume. NeuroImage 23, 724–738. doi: 10.1016/j.neuroimage.2004.06.018
Campbell, M. K., Bush, T. L., and Hale, W. E. (1993). Medical conditions associated with driving cessation in community-dwelling, ambulatory elders. J. Gerontol. 48, S230–S234. doi: 10.1093/geronj/48.4.S230
Duchek, J. M., Hunt, L., Ball, K., Buckles, V., and Morris, J. C. (1998). Attention and driving performance in Alzheimer's disease. J. Gerontol. Ser. B Psychol. Sci. Soc. Sci. 53, 130–141.
Edwards, J. D., Lunsman, M., Perkins, M., Rebok, G. W., and Roth, D. L. (2009). Driving cessation and health trajectories in older adults. J. Gerontol. Ser. A: Biomed. Sci. Med. Sci. 64, 1290–1295. doi: 10.1093/gerona/glp114
Freeman, E. E., Gange, S. J., Muñoz, B., and West, S. K. (2006). Driving status and risk of entry into long-term care in older adults. Am. J. Public Health 96, 1254–1259. doi: 10.2105/AJPH.2005.069146
Grober, E., Buschke, H., Crystal, H., Bang, S., and Dresner, R. (1988). Screening for dementia by memory testing. Neurology 38, 900–903. doi: 10.1212/WNL.38.6.900
Groot, C., Van Loenhoud, A. C., Barkhof, F., Van Berckel, B. N., Koene, T., Teunissen, C. C., et al. (2018). Differential effects of cognitive reserve and brain reserve on cognition in Alzheimer disease. Neurology 90, e149–e156. doi: 10.1212/WNL.0000000000004802
Koenig, L. N., Lamontagne, P., Glasser, M. F., Bateman, R., Holtzman, D., Yakushev, I., et al. (2022). Regional age-related atrophy after screening for preclinical Alzheimer disease. Neurobiol. Aging 109, 43–51. doi: 10.1016/j.neurobiolaging.2021.09.010
Koenig, L. N., Mccue, L. M., Grant, E., Massoumzadeh, P., Roe, C. M., Xiong, C., et al. (2021). Lack of association between acute stroke, post-stroke dementia, race, and beta-amyloid status. Neuroimage Clin. 29:102553. doi: 10.1016/j.nicl.2020.102553
Mcquail, J. A., Dunn, A. R., Stern, Y., Barnes, C. A., Kempermann, G., Rapp, P. R., et al. (2021). Cognitive reserve in model systems for mechanistic discovery: the importance of longitudinal studies. Front. Aging Neurosci. 12:607685. doi: 10.3389/fnagi.2020.607685
Megias, A., Petrova, D., Navas, J. F., Candido, A., Maldonado, A., and Catena, A. (2018). Neuroanatomical variations as a function of experience in a complex daily task: a VBM and DTI study on driving experience. Brain Imaging Behav. 12, 653–662. doi: 10.1007/s11682-017-9725-8
Milham, M. P., Erickson, K. I., Banich, M. T., Kramer, A. F., Webb, A., Wszalek, T., et al. (2002). Attentional control in the aging brain: insights from an fMRI study of the stroop task. Brain Cogn. 49, 277–296. doi: 10.1006/brcg.2001.1501
Morris, J. C. (1993). The clinical dementia rating (CDR): current version and scoring rules. Neurology 43, 2412–2414. doi: 10.1212/WNL.43.11.2412-a
Morrison, J. H., and Hof, P. R. (1997). Life and death of neurons in the aging brain. Science 278, 412–419. doi: 10.1126/science.278.5337.412
Neth, B. J., Graff-Radford, J., Mielke, M. M., Przybelski, S. A., Lesnick, T. G., Schwarz, C. G., et al. (2020). Relationship between risk factors and brain reserve in late middle age: implications for cognitive aging. Front. Aging Neurosci. 11:355. doi: 10.3389/fnagi.2019.00355
Nigmatullina, Y., Hellyer, P. J., Nachey, P., Sharp, D. J., and Seemungal, B. M. (2015). The neuroanatomical correlates of training-related perceptuo-reflex uncoupling in dancers. Cereb. Cortex 25, 554–562. doi: 10.1093/cercor/bht266
Nilsson, J., and Lövdén, M. (2018). Naming is not explaining: future directions for the “cognitive reserve” and “brain maintenance” theories. Alzheimers Res. Ther. 10, 1–7. doi: 10.1186/s13195-018-0365-z
O’banion, M. K., Coleman, P. D., and Callahan, L. M. (1994). Regional neuronal loss in aging and Alzheimer’s disease: a brief review. Semin. Neurol. 6, 307–314. doi: 10.1006/smns.1994.1039
O’neill, D., Bruce, I., Kirby, M., and Lawlor, B. (2000). Older drivers, driving practices and health issues. Clin. Gerontol. 22, 47–54.
Pollack, M. E. (2005). Intelligent technology for an aging population: the use of AI to assist elders with cognitive impairment. AI Mag. 26, 9–9. doi: 10.1609/aimag.v26i2.1810
Qin, W., Xiang, X., and Taylor, H. (2020). Driving cessation and social isolation in older adults. J. Aging Health 32, 962–971. doi: 10.1177/0898264319870400
Roe, C. M., Babulal, G. M., Head, D. M., Stout, S. H., Vernon, E. K., Ghoshal, N., et al. (2017a). Preclinical Alzheimer's disease and longitudinal driving decline. Alzheimer's Dementia: Trans. Res. Clin. Interv. 3, 74–82. doi: 10.1016/j.trci.2016.11.006
Roe, C. M., Barco, P. P., Head, D. M., Ghoshal, N., Selsor, N., Babulal, G. M., et al. (2017b). Amyloid imaging, cerebrospinal fluid biomarkers predict driving performance among cognitively normal individuals. Alzheimer Dis. Assoc. Disord. 31, 69–72. doi: 10.1097/WAD.0000000000000154
Roe, C. M., Rosnick, C. B., Colletta, A., and Babulal, G. M. (2021). Reaction to a pandemic: social distancing and driving among older adults during COVID-19. J. Appl. Gerontol. 40, 263–267. doi: 10.1177/0733464820966516
Roe, C. M., Stout, S. H., Rajasekar, G., Ances, B. M., Jones, J. M., Head, D., et al. (2019). A 2.5-year longitudinal assessment of naturalistic driving in preclinical Alzheimer's disease. J. Alzheimers Dis. 68, 1625–1633. doi: 10.3233/JAD-181242
Rosen, H. E., Bari, I., Paichadze, N., Peden, M., Khayesi, M., Monclús, J., and Hyder, A. A. (2022). Global road safety 2010–18: an analysis of global status reports. Injury.
Siedlecki, K. L., Stern, Y., Reuben, A., Sacco, R. L., Elkind, M. S., and Wright, C. B. (2009). Construct validity of cognitive reserve in a multiethnic cohort: the northern Manhattan study. J. Int. Neuropsychol. Soc. 15, 558–569. doi: 10.1017/S1355617709090857
Soldan, A., Pettigrew, C., and Albert, M. (2020). Cognitive reserve from the perspective of preclinical Alzheimer disease: 2020 update. Clin. Geriatr. Med. 36, 247–263. doi: 10.1016/j.cger.2019.11.006
Stein, A. C., and Dubinsky, R. M. (2011). Driving simulator performance in patients with possible and probable Alzheimer’s disease. Annals of advances in automotive medicine/annual scientific conference. Association for the Advancement of Automotive Medicine, 325.
Stephan, A. H., Barres, B. A., and Stevens, B. (2012). The complement system: an unexpected role in synaptic pruning during development and disease. Annu. Rev. Neurosci. 35, 369–389. doi: 10.1146/annurev-neuro-061010-113810
Stern, Y., Arenaza-Urquijo, E. M., Bartrés-Faz, D., Belleville, S., Cantilon, M., Chetelat, G., et al. (2020). Whitepaper: defining and investigating cognitive reserve, brain reserve, and brain maintenance. Alzheimers Dement. 16, 1305–1311. doi: 10.1016/j.jalz.2018.07.219
Suchy, Y., Kraybill, M. L., and Franchow, E. (2011). Instrumental activities of daily living among community-dwelling older adults: discrepancies between self-report and performance are mediated by cognitive reserve. J. Clin. Exp. Neuropsychol. 33, 92–100. doi: 10.1080/13803395.2010.493148
Tombaugh, T. N., Kozak, J., and Rees, L. (1999). Normative data stratified by age and education for two measures of verbal fluency: FAS and animal naming. Arch. Clin. Neuropsychol. 14, 167–177.
Tucker, A. M., and Stern, Y. (2011). Cognitive reserve in aging. Curr. Alzheimer Res. 8, 354–360. doi: 10.2174/156720511795745320
Vaguero, L., Hartmann, K., Ripolles, P., Rojo, N., Sierpowska, J., Francois, C., et al. (2016). Structural neuroplasticity in expert pianists depends on the age of musical training onset. NeuroImage 126, 106–119. doi: 10.1016/j.neuroimage.2015.11.008
Vassilaki, M., Aakre, J., Castillo, A., Chamberlain, A., Wilson, P., Kremers, W., Mielke, M., Geda, Y., Machulda, M., and Alhurani, R. (2022). Area deprivation index and progression to dementia (S2.007). AAN Enterprises.
Wilkinson, G. S., and Robertson, G. J. (2006). Wide range achievement test (WRAT4). Lutz, FL: Psychological Assessment Resources.
Keywords: cognitive reserve, brain reserve, driving (veh), aging, older (elderly) drivers
Citation: Murphy SA, Chen L, Doherty JM, Acharyya P, Riley N, Johnson AM, Walker A, Domash H, Jorgensen M, Bayat S, Carr DB, Ances BM and Babulal GM (2022) Cognitive and brain reserve predict decline in adverse driving behaviors among cognitively normal older adults. Front. Psychol. 13:1076735. doi: 10.3389/fpsyg.2022.1076735
Received: 24 October 2022; Accepted: 06 December 2022;
Published: 23 December 2022.
Edited by:
Mario Alfredo Parra, University of Strathclyde, United KingdomReviewed by:
Natalia Trujillo, University of Antioquia, ColombiaCopyright © 2022 Murphy, Chen, Doherty, Acharyya, Riley, Johnson, Walker, Domash, Jorgensen, Bayat, Carr, Ances and Babulal. This is an open-access article distributed under the terms of the Creative Commons Attribution License (CC BY). The use, distribution or reproduction in other forums is permitted, provided the original author(s) and the copyright owner(s) are credited and that the original publication in this journal is cited, in accordance with accepted academic practice. No use, distribution or reproduction is permitted which does not comply with these terms.
*Correspondence: Ganesh Babulal, YmFidWxhbGdAd3VzdGwuZWR1
Disclaimer: All claims expressed in this article are solely those of the authors and do not necessarily represent those of their affiliated organizations, or those of the publisher, the editors and the reviewers. Any product that may be evaluated in this article or claim that may be made by its manufacturer is not guaranteed or endorsed by the publisher.
Research integrity at Frontiers
Learn more about the work of our research integrity team to safeguard the quality of each article we publish.