- 1College of Physical Education and Sports Science, Beijing Normal University, Beijing, China
- 2College of Biological Sciences and Technology, Beijing Forestry University, Beijing, China
- 3School of Statistics, Beijing Normal University, Beijing, China
- 4Zhongguancun Foreign Language School, Beijing, China
Purpose: In football, attacking has seen evolving for decades and attacking pattern detection is an important topic in this sport. The purpose of this study was to identify the general and threatening attacking patterns of different playing styles in world top football matches, which represented the latest evolvement of soccer attacking.
Methods: Attacking sequence data of the top three teams from 21 matches in the 2018 World Cup were collected. The three teams were classified into two playing styles according to a previous study, France was a direct-play team, and Croatia and Belgium were possession-play teams. The football field was divided into 12 zones and Markov transition matrix-based zone models were applied to assess the attacking pattern in the 21 matches. Both descriptive analysis and simulative analysis were conducted using this model.
Results: The results revealed that (1) flanker attacks were frequently taken among all three teams, and possession playing teams (Croatia and Belgium) played more often than direct playing teams (France) in their center of the midfield zone and (2) forward passes across/through zones toward the middle of attacking quarter (A1/4) have a positive impact of creating a chance of a goal.
Conclusion: Using Markov transition matrix, general and threatening attacking patterns were found. The combination of possession play and counterattack was a new trend that emerged in the 2018 World Cup. These findings can help coaches to develop corresponding strategies when facing opponents of different playing styles.
Introduction
For team sports such as football, the overall strength of the team is not equal to the linear accumulation of the athletic abilities of all of the players on the pitch. The team’s performance is influenced by many factors; thus, the traditional descriptive statistical method can neither provide global information about the game (Glazier, 2010) nor convert a single variable into tactical information (Pfeiffer and Perl, 2006). Therefore, some studies have used nonlinear models to evaluate the performance of collective events (such as ball games). Lames (2006) believes that football matches are complex systems. Therefore, the concept of the relative phase is introduced into the game analysis to analyze football matches by establishing a nonlinear model (Lames, 2006). Afterward, decision tree technology (Lin, 2011), the Apriori algorithm (Pan, 2010; Liu and Hohmann, 2013a) and its improved version (Tianbiao and Andreas, 2016), and the sequential pattern mining algorithm (Liu et al., 2018) are used to analyze the set-piece tactics and attack patterns in football matches. Moreover, by applying social network analysis methods, Cao et al. (2019) analyzed the group combination of players in the football game, and Wu et al. (2020) and Yu et al. (2020) analyzed the importance of player positions in the match and the performance of foreign players. These studies analyzed the relationship between players by constructing a passing network and used network parameters to evaluate the performance of the players. The lines connecting the nodes in these passing networks represent passing activities.
Passing is one of the most important behaviors in the football field. The team organizes the offense by passing the ball, thus creating scoring opportunities. Current research on passing has mainly focused on counting the number of passes in the game and evaluating the relationship between various passes and winning (Shafizadeh et al., 2013; Liu et al., 2016, 2019). However, simple quantitative research cannot describe complex passing behaviors (Rein et al., 2017), nor can it support the research of passing decision-making. In particular, it is difficult to help coaches and players to apply any behavioral data in practice (Rein et al., 2017). Due to the characteristics of using technical and tactical behaviors in different areas of the football ground, as well as the different pressures given by opponents (Chen et al., 2015), it is particularly important to add position and field information when studying passing, technical, and tactical indicators (Fournier-Viger et al., 2019; Li and Zhang, 2019).
For each team, the passing and the use of the court area have their own characteristics. Camerino et al. (2012) studied the playing style of FC Barcelona and identified two different attacking patterns (T-Patterns), which contained different areas and passing routines. Moreover, Yi et al. (2019) observed that the technical and tactical performance and running of teams of different playing styles are different; in addition, by using technical and tactical indicators, it is possible to distinguish teams of different game styles (Lago-Peñas et al., 2017). Traditionally, it is believed that there are two typical playing styles (possession play and direct play; Hughes and Franks, 2005; Kempe et al., 2014) during offending. Later, combining with defending styles, researchers developed this playing style theory and more styles were identified (Fernandez-Navarro et al., 2016; Lago-Peñas et al., 2017; Castellano and Pic, 2019). The difference in the playing style is related to culture, football philosophy, and the skill levels of players. Ball possession is one of the most commonly used indicators to distinguish these two styles of play (Hewitt et al., 2016), which also influences the technical and physical performances of teams and players (Bradley et al., 2013; Liu et al., 2021). Additionally, situational variables also play an important role and affect playing styles (Fernandez-Navarro et al., 2018).
As a vital algorithm for the mathematical simulation of performance diagnoses, the Markov chain model has been applied to diagnostic analysis of net sports, such as table tennis (Zhang, 2003; Pfeiffer et al., 2010; Wenninger and Lames, 2016), tennis (Lames, 1991), and volleyball (Miskin et al., 2010; Hileno et al., 2020). In invasion games, the Markov chain state transition matrix can be used to describe and diagnose important passes in football (Liu and Hohmann, 2013b; Liu, 2014) or important connections in frisbee (Lam et al., 2021). Although there have been other important pattern detection technologies in the research of football (Liu and Hohmann, 2013a; Sarmento et al., 2014b), such as T-pattern (Borrie et al., 2002; Camerino et al., 2012; Pic Aguilar, 2017), these studies only aimed to describe general attacking pattern and did not offer a simulative way to discover threatening patterns. Moreover, most of the previous studies in this field did not include different playing styles in the analysis (Hirotsu and Wright, 2002; Wright and Hirotsu, 2003). Therefore, this study considers the attack sequences of the top three teams in the 2018 World Cup (the champion France, the second place Croatian, and the third place Belgium teams) as the research objects and explores the offensive routes and covering areas of the world’s top national teams with different playing styles in the World Cup.
Materials and methods
Samples
As shown in Table 1, this study recorded a total of 13,666 passing events in 21 games (7 games per team) of the top three teams (France, Croatia, and Belgium) in the 2018 World Cup in Russia. The dataset contained the time, area, and player of each pass. In the 21 recorded games, there were 10 games against possession-play opponents and 11 games against direct-play opponents. According to the division of Yi et al. (2019), among the top three teams, France was a direct-play team, and Croatian and Belgian teams exhibited possession-play styles. Among the opponents in the competition, Denmark, Russia, Iceland, Nigeria, Panama, Tunisia, Uruguay, and Peru were direct-play teams, whereas England, Argentina, Brazil, Japan, and Australia were possession-play teams.
Division of football field
The World Cup football field is based on FIFA standards, with a length of 105 m and a width of 68 m. According to a previous study (Pfeiffer et al., 2006), the football field is divided into four fields according to the front field, center front field, center backfield, and backfield. Each field is divided into three areas: left, center, and right areas, with a total of 12 areas (Figure 1A). Moreover, the division of the field is divided according to the grass stripes. Each half of the World Cup venue has 10 horizontal grass stripes; therefore, each field section contains 5 horizontal grass stripes (Figure 1B).
Data collection
All of the game videos were collected from the World Cup 2018 homepage of CCTV (China Central Television), which holds exclusive media rights to FIFA World Cup 2018. Two experienced observers observed the game video and recorded the passing information in the game according to the division of the field. To verify the reliability of the data, two matches were randomly selected; furthermore, a Cohen’s Kappa test was run for the two sets of data, and = 0.61. According to Landis and Koch (1977) and Fleiss et al. (2013), the data have good consistency (Substantial) and can be used in research.
Game observation model
Based on previous studies (Liu and Hohmann, 2013b; Liu, 2014), during the game, each area that the ball passes through is regarded as a state, the process consisting of passing is regarded as a ball control sequence (chain), and each ball control sequence (Chain) is composed of several intermediate states (zones). The sequence of possession (chain) is initiated with the possession of the ball (specifically, the starting state) and ends with the loss of possession (Figure 2; Liu and Hohmann, 2013b). The absorbing state is defined as whether a scoring opportunity is created when there is a loss of possession of the ball (TC = opportunity is created; NTC = opportunity is not created; Tianbiao and Andreas, 2016).
Statistical model and data processing
Constructing the game state transition matrix
Passing between different zones is regarded as the transition between the game states. A two-dimensional state transition probability matrix can be constructed through the state transition probability. Each element in the matrix is a positive percentage number that is not greater than 100%, and the sum of any row elements in the matrix is 100%. Table 2 shows an example of state transition probability matrix from one match. Therefore, besides calculating general descriptive results of the passing paths, according to Lames (1991), the state transition probability matrix of the game can be used to calculate the probability of creating a scoring opportunity through a Markov chain (Liu and Hohmann, 2013b).
Markov chain
In this study, zone was used as the research variable. In the game, players pass the ball from one zone to the next. This study regards the ball’s trajectory as a random system, and the zone where the ball is located at a certain moment is defined as a state.
Let be the state of the ball at time n; then, according to the Markov chain formula (Ching and Ng, 2006):
It can be expressed as follows: at the nth time, the ball is in zone j, and at the (n + 1)th time, the probability of the ball passing to zone k is the probability of the transition from state j to state k.
In the game, the state of the ball (the zone where the ball is located) at a certain time in the future is only related to the current state (zone) and has nothing to do with its previous state (zone), which is consistent with Markov chain’s “no aftereffect.” In addition, the time parameter of the possession chain is a discrete process, which can be represented by a sequence of random variables. The value of n is 0, 1, 2 …, then is called a Markov chain. When supposing that the random process is ; if it satisfies any integer , any , its state transition probability is (Ching and Ng, 2006):
Subsequently, for the transition matrix M containing the absorbing state, the Markov chain whose initial state row vector is S, after n rounds of iterations, reaches its final state Sfinal as:
where n is a sufficiently large positive integer, and the probability of a transition state (zone state) in Sfinal is close to 0. In this study, the probability of the absorbing state represents the probability of creating a scoring opportunity (TC) and the probability of failing to create a scoring opportunity (NTC).
Calculating competitive relevance (performance relevance)
After calculating the probability of creating a scoring opportunity, a new state transition probability is calculated according to a deflection formula (Lames, 1991)
where is the transition probability, is the transition probability after the change, the constant , (Lames, 1991; Pfeiffer, 2005). In this process, every element (transition probability) in the initial matrix will be modified. To ensure that the sum of the rows of the modified matrix would still be 1, other cells in the same row in the matrix are calculated by using the compensation formula (Liu and Hohmann, 2013b)
Afterward, the Markov chain model is used to calculate the probability of creating a scoring opportunity for the newly obtained matrix, and this new probability is compared with the result of the initial matrix calculation to determine the impact of changes between the states of the game (changes between the zones = passing) on the creation of scoring opportunities.
Data processing
First, the descriptive statistics of the passes between the different zones derived from the state transition matrix were calculated. The chi-square test was used to compare the general passing data of the top three teams in the 2018 World Cup against different opponents (n possession vs. possession = 6; n possession vs. direct = 8; n direct vs. possession = 4; n direct to direct = 3). Afterward, the Markov chain transition matrix was used to simulate and calculate the influence of the change of state in the game on the creation of scoring opportunities. The significance level was set to . According to Cohen (1988), the effect size is , and the threshold is ; in this study, the value was 4. Data were processed by using SPSS (ver. 26, IBM, Chicago, United States) and Python (ver. 3.8), and graphical visualization was performed by using the online drawing tool draw.io (v. 14.8.4).
Results
Descriptive analysis of the passing paths of the top three teams in the 2018 world cup
Figure 3 shows the descriptive analysis of the passing paths of the top three teams in the 2018 World Cup. The depth of the color of the field zones represents the frequency of technical and tactical activities in the area, and the thickness of the arrow represents the closeness of the connection between the areas. The top three teams rarely passed the ball across regions (with only a few long passes being observed), and all of the teams focused on using the wing area to organize their offenses. However, the differences in the passing and active areas of the different playing style teams were significant (Possession play vs. direct play, ). Moreover, Croatian and Belgium teams, which exhibit possession playing styles, tended to use their own midfield zones (LDM, ZDM, and RDM) to organize their offenses, whereas France, which is a direct-play style team, tended to directly form an offense through their own backside zones (LA and RA). Furthermore, the differences in the passing and active areas of the same style of play teams against opponents of different styles were also significant (possession play vs. different opponents, ; direct play vs. different opponents, ).
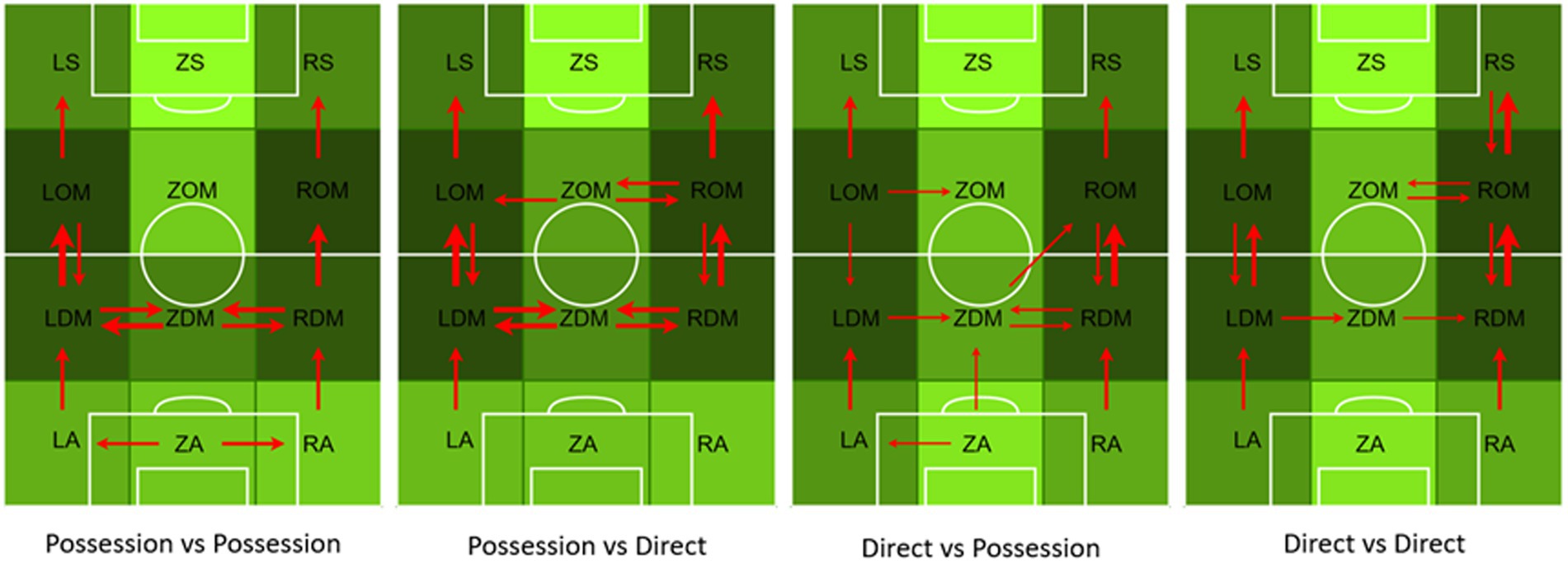
Figure 3. Descriptive analysis of actions zones and passing paths among top three teams in the World Cup 2018.
Diagnostic analysis of the passing paths of the top three teams in the 2018 World Cup
Table 3; Figure 4 illustrate the diagnostic analysis of the passing paths of the top three teams in the 2018 World Cup. For the top three teams, regardless of whether Croatia and Belgium teams focused on possession, or France focused on direct play, the diagnostic analysis observed that cross-regional passes (long passes) to the forward center (ZS) and to the side of the opponent’s half (LS and RS) have a positive effect on the creation of scoring opportunities. Especially for the direct playing style of France, when facing the same direct playing opponent, increasing side-way attack can increase its chance of scoring.
Discussion
The aim of this study was to analyze the paths and covering zones in the offensive sequence of high-level football games. The results found that (1) the top three teams in the 2018 World Cup tended to form offenses in the wing area, and possession-play teams (Croatia and Belgium) had more passes in their own midfielder zones than direct-play teams (France); and (2) cross-regional forward passes, especially passes toward the forward middle zone, had a particularly important positive impact on creating scoring opportunities.
From the offensive mode of the top three teams in the 2018 World Cup, the flanker areas are still effective offensive areas, which is also consistent with the studies on previous international football tournaments (Xue et al., 2015; Yamada and Hayashi, 2015; Mitrotasios et al., 2022). As attacks through middle zones will encounter greater defensive resistance, the side-way attack is a more effective offensive method (Grehaigne et al., 2002; Diana et al., 2017), which can disrupt the opponent’s defensive balance and increase the probability of a successful offense (Fernandez-Navarro et al., 2016). However, this study found that teams with different playing styles used the field areas differently. The possession playing Croatia and Belgium exhibited more lateral passes in the midfield than direct playing France, whereas France made more use of side areas on its own backcourt. For the top three teams in the 2018 World Cup, the difference in the use of covering areas and paths may be due to teams of different playing styles actively adopting their own style of play. Evidence indicates that stronger teams dominated ball possession against their opponents and shows that more stable patterns of play independently of the evolving score-line (Lago, 2009; Lago-Peñas and Dellal, 2010). Previous studies also have shown that both FC Barcelona and Manchester United (in the 2011 UEFA Champions League Finale) were possession-play teams (Sarmento et al., 2014a), although Manchester United was not as strong as FC Barcelona in that match, the players still had been trying and insisting on passing and controlling in the midfield area in the Finale (Liu and Hohmann, 2013a). During a football game, the team with the higher ball possession rate tends to be stronger (Hughes and Franks, 2005); therefore, they are also able to maintain possession of the ball in the midfield area (Casal et al., 2017) and have a higher possession rate in the opponent’s half and the 35-m area of the frontcourt (the attacking 1/3; this does not consider match status). In addition, the center of the formation (the centroid) in the game will also move forward accordingly (Clemente et al., 2013). In contrast, direct-play (counterattack) teams have a relatively low ball possession rate in the game, especially in the midfield. Therefore, direct playing teams are more inclined to use the wing to organize and launch offenses, especially from the sidelines of their own backcourt. This finding is also consistent with the research of Yi et al. (2019).
This study also found that for teams with both styles of play, passing from the side toward the forward center of the field (the ZS area) can better help in creating scoring opportunities. Therefore, the possession playing style teams can appropriately increase the cross-area long pass with the target of the front middle. Research on the European Cup and World Cup has shown that teams do not easily change the playing style in the game (Casal et al., 2017); however, teams that flexibly combine possession play and direct play in different situations can achieve better results (Yi et al., 2019). These findings also support the results of our study. During a football game, researchers have long known that the middle area of the frontcourt is an important area that is used to create scoring opportunities (Worthington, 1975; Hughes, 1990; Xue et al., 2015); thus, the early research of Pollard and Reep (1997) proposed that the ball should be introduced into this area as soon as possible to form a shot. This theory has considerably affected England and Norway teams (Larson, 2001). An early study (Grehaigne et al., 2002) showed that the area most likely to be intercepted and counterattacked by the opponent in the offense is the opponent’s middle area of the backcourt (ZOM, for the attacking team, the middle of the forecourt); however, the opponent’s penalty area (which is located in the frontcourt for the attacking team) is not the area where the defenders are most likely to steal the ball and counterattack (Grehaigne et al., 2002). Therefore, after combining the data of Bate (1988) and Dufour (1993), the target area of a cross-area long pass (the counterattack) should be the area between the goal area and the penalty area (around the penalty point). However, it should be noted that the abovementioned studies were conducted several decades ago. With the development of football, high position press and formation forward pressure tactics have gradually become a trend. Therefore, the target area of the pass in a quick counterattack and a positional attack is also different. The target area of a long pass for a quick counterattack and a positional attack should be combined with the area where the opponent’s formation is located; generally, the target is the area between the opponent’s central defender and the goalkeeper (Larson, 2001), which likely represents the attacking team’s middle front. Therefore, in a quick counterattack, the target area may be slightly far from the defender’s penalty area, whereas in a positional attack, the target area may be closer to the defender’s goal area.
Furthermore, pattern analysis is always an interesting topic in sports, which provides coaches and players with important information and helps to win the match. Previous studies also introduced pattern detection using T-pattern (Pic Aguilar, 2017) or Polar coordinates (Castañer et al., 2016; Maneiro Dios and Amatria Jiménez, 2018) or mixed method (Castañer et al., 2017) which are already sophisticated methods (Magnusson, 2019). Comparing with these studies, the current study used Markov Chain transition matrix model to describe and diagnose zone patterns of World Cup top teams, combining with style of play, it offers a new perspective to solve such problems. A difference between current method and T-pattern is that Markov Chain transition matrix method is based on event-sequence and not temporal sensitive for the temporal distances between events. It is worth noting in the future study to add some temporal factors.
The limitation of this study was that only the data of three teams were used. The current sample size between teams of two playing styles is not balanced enough and may not fully reflect the general situation of the two styles of play, but the characteristics of the top teams in the 2018 World Cup were still representative and showed the latest development trend of playing style and attacking pattern in football games. Through this study, coaches can understand different styles of play and development trends of football, as well as combine the data from this study with other studies, to make proper tactical responses in future games. In future research, situational factors (such as different playing styles, team strengths, match statuses, and match time) can be incorporated to analyze the changes in team play.
Conclusion
This study analyzed and diagnosed the offensive patterns of the top three teams in the 2018 World Cup based on the Markov chain transition matrix. By constructing a transfer matrix, we demonstrated the methods that the top teams with different playing styles used in their offenses. Both playing style teams mainly used the wing area in their offense. Furthermore, the possession playing team organized more passes in the midfield, whereas the direct-play teams made more use of the side zones of their backcourt. In addition, the combination of possession play and counterattack was a new trend that emerged in the 2018 World Cup.
Although the possession playing style has prevailed in the past decade, the success of the French national team in the 2018 World Cup and the failure of Germany and Spain (who were in pursuit of pass and control tactics) have caused researchers to re-examine the game style. The efficiency of offense in the game has become increasingly important. Furthermore, possession-play football and direct play football are not incompatible, and offensive efficiency and scoring are ways to achieve game success.
Data availability statement
The raw data supporting the conclusions of this article will be made available by the authors, without undue reservation.
Ethics statement
Ethical review and approval was not required for the study on human participants in accordance with the local legislation and institutional requirements. Written informed consent for participation was not required for this study in accordance with the national legislation and the institutional requirements.
Author contributions
TL conceptualized the study and wrote the original draft preparation. TL and CZ contributed to the methodology. XS, LZ, and CZ contributed to data collection and visualization. TL, JZ, and LY reviewed and edited the manuscript. All authors contributed to the article and approved the submitted version.
Funding
This study was supported by the National Social Science Fund of China (18CTY011).
Acknowledgments
The authors would like to thank Prof. Dr. Martin Lames and Frederic Rothe for the support to this study.
Conflict of interest
The authors declare that the research was conducted in the absence of any commercial or financial relationships that could be construed as a potential conflict of interest.
Publisher’s note
All claims expressed in this article are solely those of the authors and do not necessarily represent those of their affiliated organizations, or those of the publisher, the editors and the reviewers. Any product that may be evaluated in this article, or claim that may be made by its manufacturer, is not guaranteed or endorsed by the publisher.
References
Bate, R. (1988). “Football chance: tactics and strategy,” in Science and football: Proceedings of the first world congress of science and football Liverpool, 13–17th April 1987. eds. T. Reilly, A. Lees, K. Davids, and W. J. Murphy (New York: Routledge) 293
Borrie, A., Jonsson, G. K., and Magnusson, M. S. (2002). Temporal pattern analysis and its applicability in sport: an explanation and exemplar data. J. Sports Sci. 20, 845–852. doi: 10.1080/026404102320675675
Bradley, P. S., Lago-Peñas, C., Rey, E., and Gomez Diaz, A. (2013). The effect of high and low percentage ball possession on physical and technical profiles in English FA premier league soccer matches. J. Sports Sci. 31, 1261–1270. doi: 10.1080/02640414.2013.786185
Camerino, O. F., Chaverri, J., Anguera, M. T., and Jonsson, G. K. (2012). Dynamics of the game in soccer: detection of T-patterns. Eur. J. Sport Sci. 12, 216–224. doi: 10.1080/17461391.2011.566362
Cao, X., Li, X., Fu, Y., Wu, H., Liu, T., and Liang, Y. (2019). Effect of home and away matches of Chinese super league on team performance based on the complex network method. Sport Sci. Res. 40, 22–28.
Casal, C. A., Maneiro, R., Ardá, T., Marí, F. J., and Losada, J. L. (2017). Possession zone as a performance indicator in football. The game of the best teams. Front. Psychol. 8:1176. doi: 10.3389/fpsyg.2017.01176
Castañer, M., Barreira, D., Camerino, O., Anguera, M. T., Canton, A., and Hileno, R. (2016). Goal scoring in soccer: a polar coordinate analysis of motor skills used by Lionel Messi. Front. Psychol. 7:806. doi: 10.3389/fpsyg.2016.00806
Castañer, M., Barreira, D., Camerino, O., Anguera, M. T., Fernandes, T., and Hileno, R. (2017). Mastery in goal scoring, T-pattern detection, and polar coordinate analysis of motor skills used by Lionel Messi and Cristiano Ronaldo. Front. Psychol. 8:741. doi: 10.3389/fpsyg.2017.00741
Castellano, J., and Pic, M. (2019). Identification and preference of game styles in LaLiga associated with match outcomes. Int. J. Environ. Res. Public Health 16:5090. doi: 10.3390/ijerph16245090
Chen, X., Li, C., and Huang, Z. (2015). Analysis of Opponent's technique and tactic in professional football match. J. Beijing Sport Univ. 38, 120–127. doi: 10.19582/j.cnki.11-3785/g8.2015.12.019
Ching, W.-K., and Ng, M. K. (2006). Markov chains: Models, algorithms and applications/Wai-Ki Ching, Michael K. Ng. New York, N.Y., Great Britain: Springer.
Clemente, F. M., Couceiro, M. S., Martins, F. M. L., Mendes, R., and Figueiredo, A. J. (2013). Measuring tactical behaviour using technological metrics: case study of a football game. Int. J. Sports Sci. Coach. 8, 723–739. doi: 10.1260/1747-9541.8.4.723
Cohen, J. (Ed.) (1988). Chi-square tests for goodness of ft and contingency tables. in Statistical power analysis for the behavioral sciences. 2nd Edn. (New York: Lawrence Erlbaum Associates), 215–271.
Diana, B., Zurloni, V., Elia, M., Cavalera, C. M., Jonsson, G. K., and Anguera, M. T. (2017). How game location affects soccer performance: T-pattern analysis of attack actions in home and away matches. Front. Psychol. 8:1415. doi: 10.3389/fpsyg.2017.01415
Dufour, W. (1993). “Computer-assisted scouting in soccer,” in Science and football II: Proceedings of the second world congress of science and football, Eindhoven, Netherlands, 22nd-25th may 1991/edited by T. Reilly, J. Clarys and A. Stibbe. eds. T. Reilly, J. P. Clarys, and A. Stibbe (London: E. & F.N. Spon), 160–166.
Fernandez-Navarro, J., Fradua, L., Zubillaga, A., Ford, P. R., and McRobert, A. P. (2016). Attacking and defensive styles of play in soccer: analysis of Spanish and English elite teams. J. Sports Sci. 34, 2195–2204. doi: 10.1080/02640414.2016.1169309
Fernandez-Navarro, J., Fradua, L., Zubillaga, A., and McRobert, A. P. (2018). Influence of contextual variables on styles of play in soccer. Int. J. Perform. Anal. Sport 18, 423–436. doi: 10.1080/24748668.2018.1479925
Fleiss, J. L., Levin, B., and Paik, M. C. (2013). Statistical methods for rates and proportions. Hoboken, NJ: Wiley.
Fournier-Viger, P., Liu, T., and Chun-Wei Lin, J. (2019). “Football pass prediction using player locations,” in Machine learning and data mining for sports analytics: 5th international workshop, MLSA 2018, co-located with ECML/PKDD 2018, Dublin, Ireland, September 10, 2018, proceedings, ed. U. Brefeld, J. Davis, J. Haarenvan, and A. Zimmermann (Cham, Switzerland: Springer), 152–158.
Glazier, P. S. (2010). Game, set and match? Substantive issues and future directions in performance analysis. Sports Med. 40, 625–634. doi: 10.2165/11534970-000000000-00000
Grehaigne, J., Marchal, D., and Duprat, E. (2002). “Regaining possession of the ball in the defensive area in soccer,” in Science and football IV. eds. W. Spinks, T. Reilly, and A. Murphy (London: Routledge).
Hewitt, A., Greenham, G., and Norton, K. (2016). Game style in soccer: what is it and can we quantify it? Int. J. Perform. Anal. Sport 16, 355–372. doi: 10.1080/24748668.2016.11868892
Hileno, R., Arasanz, M., and García-De-Alcaraz, A. (2020). The sequencing of game complexes in Women's volleyball. Front. Psychol. 11:739. doi: 10.3389/fpsyg.2020.00739
Hirotsu, N., and Wright, M. (2002). Using a Markov process model of an association football match to determine the optimal timing of substitution and tactical decisions. J. Oper. Res. Soc. 53, 88–96. doi: 10.1057/palgrave/jors/2601254
Hughes, M., and Franks, I. (2005). Analysis of passing sequences, shots and goals in soccer. J. Sports Sci. 23, 509–514. doi: 10.1080/02640410410001716779
Kempe, M., Vogelbein, M., Memmert, D., and Nopp, S. (2014). Possession vs. direct play: evaluating tactical behavior in elite soccer. Int. J. Sports Sci. 4, 35–41. doi: 10.5923/s.sports.201401.05
Lago, C. (2009). The influence of match location, quality of opposition, and match status on possession strategies in professional association football. J. Sports Sci. 27, 1463–1469. doi: 10.1080/02640410903131681
Lago-Peñas, C., and Dellal, A. (2010). Ball possession strategies in elite soccer according to the evolution of the match-score: the influence of situational variables. J. Hum. Kinet. 25, 93–100. doi: 10.2478/v10078-010-0036-z
Lago-Peñas, C., Gómez-Ruano, M., and Yang, G. (2017). Styles of play in professional soccer: an approach of the Chinese soccer super league. Int. J. Perform. Anal. Sport 17, 1073–1084. doi: 10.1080/24748668.2018.1431857
Lam, H., Kolbinger, O., Lames, M., and Russomanno, T. G. (2021). State transition modeling in ultimate Frisbee: adaptation of a promising method for performance analysis in invasion sports. Front. Psychol. 12:664511. doi: 10.3389/fpsyg.2021.664511
Lames, M. (1991). Leistungsdiagnostik durch Computersimulation: Ein Beitrag zur Theorie der Sportspiele am Beispiel Tennis. Frankfurt/Main: Harri Deutsch.
Lames, M. (2006). Modelling the interaction in game sports – relative phase and moving correlations. J. Sports Sci. Med. 5, 556–560.
Landis, J. R., and Koch, G. G. (1977). The measurement of observer agreement for categorical data. Biometrics 33:159. doi: 10.2307/2529310
Larson, O. (2001). Charles Reep: a major influence on British and Norwegian football. Soccer Soc. 2, 58–78. doi: 10.1080/714004854
Li, H., and Zhang, Z. (2019). “Predicting the receivers of football passes,” in Machine learning and data mining for sports analytics: 5th international workshop, MLSA 2018, co-located with ECML/PKDD 2018, Dublin, Ireland, September 10, 2018, proceedings/Ulf Brefeld, ed. U. Brefeld, J. Davis, J. Haarenvan, and A. Zimmermann (Cham, Switzerland: Springer), 167–177.
Lin, J. (2011). “The data Mining in the Attacking Tactics of the opposing-half corner kick of the football match,”. Master’s dissertation. Shanghai Sport University.
Liu, T. (2014). “Systematische Spielbeobachtung im internationalen Leistungsfußball,”. Doctoral thesis. Kulturwissenschaftlichen Fakultät, University of Bayreuth.
Liu, T., Fournier-Viger, P., and Hohmann, A. (2018). “Using diagnostic analysis to discover offensive patterns in a football game,” in Recent developments in data science and business analytics: Proceedings of the International Conference on Data Science and Business Analytics (ICDSBA-2017). eds. M. Tavana and S. Patnaik (Cham, Switzerland: Springer), 381–386.
Liu, T., García-De-Alcaraz, A., Zhang, L., and Zhang, Y. (2019). Exploring home advantage and quality of opposition interactions in the Chinese football super league. Int. J. Perform. Anal. Sport 19, 289–301. doi: 10.1080/24748668.2019.1600907
Liu, T., and Hohmann, A. (2013a). Applying data mining to analyze the different styles of offense between Manchester United and FC Barcelona in the European champions league final. Int. J. Sports Sci. Eng. 7, 67–78.
Liu, T., and Hohmann, A. (2013b). Applying the Markov chain theory to analyze the attacking actions between FC Barcelona and Manchester United in the European champions league finale. Int. J. Sports Sci. Eng. 7, 79–86.
Liu, H., Hopkins, W. G., and Gómez, M.-A. (2016). Modelling relationships between match events and match outcome in elite football. Eur. J. Sport Sci. 16, 516–525. doi: 10.1080/17461391.2015.1042527
Liu, T., Yang, L., Chen, H., and García-De-Alcaraz, A. (2021). Impact of possession and player position on physical and technical-tactical performance indicators in the Chinese football super league. Front. Psychol. 12:722200. doi: 10.3389/fpsyg.2021.722200
Magnusson, M. S. (2019). T-pattern detection and analysis (TPA) with THEMETM: a mixed methods approach. Front. Psychol. 10:2663. doi: 10.3389/fpsyg.2019.02663
Maneiro Dios, R., and Amatria Jiménez, M. (2018). Polar coordinate analysis of relationships with teammates, areas of the pitch, and dynamic play in soccer: a study of Xabi Alonso. Front. Psychol. 9:389. doi: 10.3389/fpsyg.2018.00389
Miskin, M. A., Fellingham, G. W., and Florence, L. W. (2010). Skill importance in Women's volleyball. J. Quant. Analy. Sports 6. doi: 10.2202/1559-0410.1234
Mitrotasios, M., Kubayi, A., Armatas, V., and Larkin, P. (2022). Analysis of crossing opportunities at the 2018 FIFA world cup. Montenegr. J. Sports Sci. Med. 11, 43–52. doi: 10.26773/mjssm.220305
Pan, C. (2010). Appliance of Apriori algorithm on technical-tactics analysis of football. Comput. Knowl. Technol. 6, 8835–8837.
Pfeiffer, M. (2005). Leistungsdiagnostik im Nachwuchstraining der Sportspiele [performance diagnosis in the game sport training of young athletes]. Köln: Sport und Buch Strauß.
Pfeiffer, M., Hohmann, A., and Bührer, M. (2006). “Computersimulation zur Bestimmung der Leistungsrelevanz taktischer Verhaltensweisen bei der FIFA WM 2006” in Zukunft der Sportspiele: fördern, fordern, forschen. eds. M. Raab, A. Arnold, K. Gärtner, J. Köppen, C. Lempertz, and N. Tielemann, et al. (Flensburg: University Press), 195–198.
Pfeiffer, M., and Perl, J. (2006). Analysis of tactical structures in team handball by means of artificial neural networks. Int. J. Comput. Sci. Sport 5, 4–14.
Pfeiffer, M., Zhang, H., and Hohmann, A. (2010). A Markov chain model of elite table tennis competition. Int. J. Sports Sci. Coach. 5, 205–222. doi: 10.1260/1747-9541.5.2.205
Pic Aguilar, M. (2017). Temporal consistencies in two champion teams of European football? (¿Regularidades temporales en dos campeones del fútbol europeo?). Retos. 34, 94–99. doi: 10.47197/retos.v0i34.58805
Pollard, R., and Reep, C. (1997). Measuring the effectiveness of playing strategies at soccer. J. R. Stat. Soc. Series D 46, 541–550. doi: 10.1111/1467-9884.00108
Rein, R., Raabe, D., and Memmert, D. (2017). "which pass is better?" novel approaches to assess passing effectiveness in elite soccer. Hum. Mov. Sci. 55, 172–181. doi: 10.1016/j.humov.2017.07.010
Sarmento, H., Anguera, M. T., Campanio, J., Rui, R., and Leito, J. (2014a). Manchester UNITED, INTERNAZIONALE MILANO and fc Barcelona – WHATS different? Sport Mont. J. 12, 50–56.
Sarmento, H., Anguera, M. T., Pereira, A., Marques, A., Campaniço, J., and Leitão, J. (2014b). Patterns of play in the counterattack of elite football teams – a mixed method approach. Int. J. Perform. Anal. Sport 14, 411–427. doi: 10.1080/24748668.2014.11868731
Shafizadeh, M., Taylor, M., and Peñas, C. L. (2013). Performance consistency of international soccer teams in euro 2012: a time series analysis. J. Hum. Kinet. 38, 213–226. doi: 10.2478/hukin-2013-0061
Tianbiao, L., and Andreas, H. (2016). “Apriori-based diagnostical analysis of passings in the football game,” in 2016 IEEE International Conference on Big Data Analysis (ICBDA) (IEEE), 1–4.
Wenninger, S., and Lames, M. (2016). Performance analysis in table tennis – stochastic simulation by numerical derivation. Int. J. Comput. Sci. Sport 15, 22–36. doi: 10.1515/ijcss-2016-0002
Wright, M., and Hirotsu, N. (2003). The professional foul in football: tactics and deterrents. J. Oper. Res. Soc. 54, 213–221. doi: 10.1057/palgrave.jors.2601506
Wu, Y., Xia, Z., Wu, T., Yi, Q., Yu, R., and Wang, J. (2020). Characteristics and optimization of core local network: big data analysis of football matches. Chaos Solitons Fractals 138:110136. doi: 10.1016/j.chaos.2020.110136
Xue, J., Liu, T., Li, Y., He, C., and Cao, W. (2015). Basic characteristics and law of goal in modern football game: a case study on the goals from 14th to 20th FIFA world cup games. J. Beijing Sport Univ. 38, 125–129. doi: 10.19582/j.cnki.11-3785/g8.2015.08.019
Yamada, H., and Hayashi, Y. (2015). Characteristics of goal-scoring crosses in international soccer tournaments. Football Sci. 12, 24–32.
Yi, Q., Gómez, M. A., Wang, L., Huang, G., Zhang, H., and Liu, H. (2019). Technical and physical match performance of teams in the 2018 FIFA world cup: effects of two different playing styles. J. Sports Sci. 37, 2569–2577. doi: 10.1080/02640414.2019.1648120
Yu, Q., Gai, Y., Gong, B., Gómez, M.-Á., and Cui, Y. (2020). Using passing network measures to determine the performance difference between foreign and domestic outfielder players in Chinese football super league. Int. J. Sports Sci. Coach. 15, 398–404. doi: 10.1177/1747954120905726
Keywords: soccer, Markov chain, stochastic process, playing behaviors, playing style, offensive sequence, zone, performance analysis
Citation: Liu T, Zhou C, Shuai X, Zhang L, Zhou J and Yang L (2022) Influence of different playing styles among the top three teams on action zones in the World Cup in 2018 using a Markov state transition matrix. Front. Psychol. 13:1038733. doi: 10.3389/fpsyg.2022.1038733
Edited by:
Miguel-Angel Gomez-Ruano, Polytechnic University of Madrid, SpainReviewed by:
Marek Konefał, University School of Physical Education in Wroclaw, PolandMiguel Pic, South Ural State University, Russia
Copyright © 2022 Liu, Zhou, Shuai, Zhang, Zhou and Yang. This is an open-access article distributed under the terms of the Creative Commons Attribution License (CC BY). The use, distribution or reproduction in other forums is permitted, provided the original author(s) and the copyright owner(s) are credited and that the original publication in this journal is cited, in accordance with accepted academic practice. No use, distribution or reproduction is permitted which does not comply with these terms.
*Correspondence: Tianbiao Liu, bHRiQGJudS5lZHUuY24=