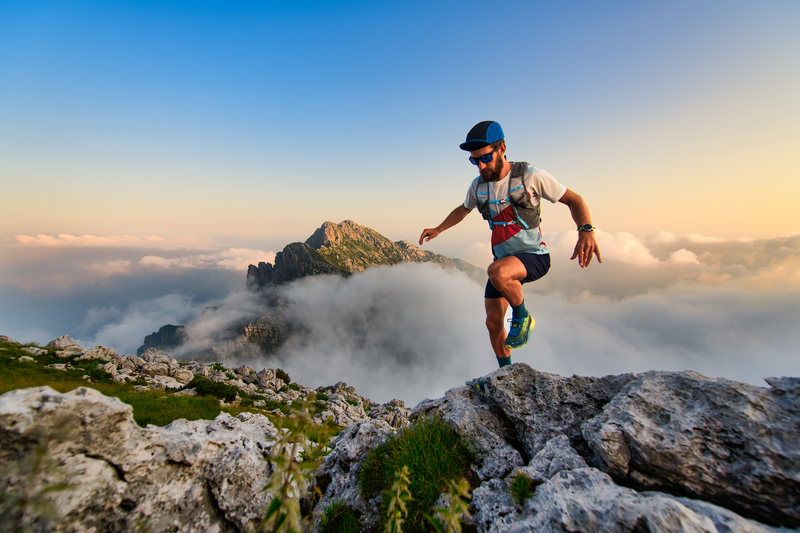
94% of researchers rate our articles as excellent or good
Learn more about the work of our research integrity team to safeguard the quality of each article we publish.
Find out more
ORIGINAL RESEARCH article
Front. Psychol. , 04 January 2023
Sec. Human-Media Interaction
Volume 13 - 2022 | https://doi.org/10.3389/fpsyg.2022.1011883
Introduction: Designing artificial intelligence (AI) to support health and wellbeing is an important and broad challenge for technologists, designers, and policymakers. Drawing upon theories of AI and cybernetics, this article offers a design framework for designing intelligent systems to optimize human wellbeing. We focus on the production of wellbeing information feedback loops in complex community settings, and discuss the case study of My Wellness Check, an intelligent system designed to support the mental health and wellbeing needs of university students and staff during the COVID-19 pandemic.
Methods: The basis for our discussion is the community-led design of My Wellness Check, an intelligent system that supported the mental health and wellbeing needs of university students and staff during the COVID-19 pandemic. Our system was designed to create an intelligent feedback loop to assess community wellbeing needs and to inform community action. This article provides an overview of our longitudinal assessment of students and staff wellbeing (n = 20,311) across two years of the COVID-19 pandemic.
Results: We further share the results of a controlled experiment (n = 1,719) demonstrating the enhanced sensitivity and user experience of our context-sensitive wellbeing assessment.
Discussion: Our approach to designing “AI for community wellbeing,” may generalize to the systematic improvement of human wellbeing in other human-computer systems for large-scale governance (e.g., schools, businesses, NGOs, platforms). The two main contributions are: 1) showcasing a simple way to draw from AI theory to produce more intelligent human systems, and 2) introducing a human-centered, community-led approach that may be beneficial to the field of AI.
As the use of Artificial Intelligence (AI) continues to advance, researchers are increasingly exploring its potential to support and enhance mental health and wellbeing. With its ability to analyze large amounts of data and make complex decisions, AI has the potential to provide valuable insights and support to individuals and communities seeking to improve their mental health and wellbeing (D’Alfonso, 2020). In this paper, rather than viewing “AI for wellbeing” as a specialized interest in the mental health community, we argue that all ethical AI systems should have the implicit objective of enhancing human wellbeing. This is in line with the Institute of Electrical and Electronics Engineers standards review on ethical design: “by aligning the creation of [AI] with the values of its users and society we can prioritize the increase of human wellbeing as our metric for progress in the algorithmic age” (Shahriari and Shahriari, 2017).
While ‘AI for Wellbeing’ often refers to the use of AI tools, such as chatbots, to support mental health and wellbeing, this article focuses on using theories developed by AI researchers to better understand how large-scale systems can be designed to enhance and support wellbeing outcomes.
For example, the European Commission defines AI Systems as “systems that display intelligent behavior by analyzing their environment and taking actions—with some degree of autonomy—to achieve specific goals” (2019, p. 1).
More specifically, a popular AI textbook defines the field of artificial intelligence as the “study and design of intelligent agents” where an agent is anything that can be viewed as perceiving its environment through sensors and acting upon that environment through actuators (Russell and Norvig, 2022). For such an agent to be considered intelligent, it must possess the “ability to select an action that is expected to maximize [a] performance measure…an agent that is assigned an explicit “goal function” is considered more intelligent if it consistently takes actions that successfully maximize its programmed goal function” (Russell and Norvig, 2022, p. 58). Based on these criteria, AI systems must possess the ability to sense their environment, act on their environment, measure an explicit goal state in the environment (i.e., a performance measure or objective function), and use sense data to choose actions likely to improve that performance measure—see Box 1 for a proposed framework based on these theoretical propositions. In brief, this theory suggests that it may be inevitable that future wellbeing-aligned AI systems will necessarily need mechanisms for assessing human wellbeing.
BOX 1 A framework for designing AI Systems, based on a definition of AI focused on the “ability to select an action that is expected to maximize [a] performance measure” (Russell and Norvig, 2022).
This paper provides a demonstration of developing context-sensitive wellbeing assessments that may inform the design of future AI system assessments of wellbeing through community-led design. I.e., we present the case study of My Wellness Check: an intelligent system that measures human wellbeing in order to optimize and support the needs of university students and staff during the COVID-19 pandemic. Designed in collaboration with students, staff, and mental health professionals, the My Wellness Check system provided a governing feedback loop capable of assessing community wellbeing needs and informing community action. Based on theoretically-derived factors of wellbeing as well as factors defined by community participants, My Wellness Check produced real-time insights into community wellbeing that were used to inform actions at various levels of the university, from top administrators to individual students. We share data from a longitudinal deployment of My Wellness Check to nearly 30,000 students and staff across 2 years of the COVID-19 pandemic. To evaluate our system, we share the results of a controlled experiment comparing our community-led wellbeing assessment to other wellbeing assessments. This shows that our community-led designs generated greater predictive value and a significantly better user experience. While our results cannot serve as proof of efficacy for the performance of our entire system, it does show the benefits of our community-led design process.
Schools, businesses, NGOs, social platforms and other large-scale governing systems may wish to systematically improve the wellbeing of the people they serve. Our work aims to provide insights that can generalize to these different contexts. Rather than designing a fully autonomous system (for example, a chatbot to help provide students with mental health recommendations), we focused on introducing an intelligent feedback loop to an existing sociotechnical system.
In the first part of this article, we provide an overview of the concept of “designing AI for Wellbeing” and review some related efforts. We then discuss several methods and ideas popular within the field of human-centered design, such as participatory design, community-led design, systems thinking, and cybernetic thinking to address some of the challenges of designing AI for Wellbeing.
In the second part of the paper, we describe the specific context and the design of My Wellness Check. We then present data from multiple assessments of wellbeing over the period of the pandemic. Following a description of the design of the system, we present the design and implementation of a controlled experiment to evaluate our context-sensitive assessment. Following the presentation of the results of this experiment, we then reflect upon our design framework and suggest opportunities for future research in the design of AI systems for Community Wellbeing.
There is a small, but growing, body of work on the use of AI for wellbeing or mental health, much of which focuses on the use of AI for health monitoring and personalized health advice. Often these services are delivered through the use of virtual agents, chatbot, wearables and other IoT technologies (see review by Shah and Chircu, 2018). According to D’Alfonso (2020) the three main applications of AI in mental health are: (1) personal sensing or digital phenotyping; (2) natural language processing of clinical texts and social media content; and (3) chatbots, while another review found opportunities for AI in mental health mainly related to self-tracking and AI assisted data analysis (Graham et al., 2019).
A 2020 Designing Interactive Systems (DIS) workshop on wellbeing offered the following summary of the field: “Most human-computer interaction (HCI) work on the exploration and support of mental wellbeing involves mobiles, sensors, and various on-line systems which focus on tracking users” (Sas et al., 2020). This reflects a focus on user-centered solutions for wellbeing, where wellbeing is conceptualized as the concern of an individual person.
In this paper, we present an alternative design objective: to support the wellbeing of a community of people. The wellbeing of a community can be understood as a multidimensional set of values, including economic, social, and environmental values, that impact people in a community (see review by Shah and Chircu, 2018). One advantage of this approach is that it does not require tracking individuals over time, which poses more risks from a data privacy and security perspective. Individual tracking, when it reveals deficits in wellbeing, may be damaging to individual self-image and produce feelings of guilt or disappointment (Chan et al., 2018).
A 2019 review of HCI technologies for wellbeing proposes the following: “We argue for an ethical responsibility for researchers to design more innovative mental health technologies that leverage less the tracked data and more its understanding, reflection, and actionability for positive behavior change.” (Sanches et al., 2019). By focusing on wellbeing at a community level (namely, the students and staff at a campus university), we can avoid data tracking issues and include diverse stakeholders that can assist with understanding the wellbeing data, reflecting upon it and formulating approaches for positive action.
As part of our community-focus, our work centers around two components of the system: assessments of community wellbeing needs and the design of interventions that target those needs. We are inspired by cybernetic theory to design our system to produce a wellbeing feedback loop that supports both top-down and bottom-up processes.This approach lends itself to our participatory and community-led design methods It also stands in contrast to the objective of developing an autonomous system that uses a black-box, algorithmic approach to intervene in the community. Finally, we use an iterative, longitudinal design approach that emphasizes improvements in the assessment of wellbeing and the processes taken to transform those assessments into action.
Cybernetics has seen a resurgence of interest due to the increasing popularity of artificial intelligence and machine learning (Pangaro, 2017, 2021), and can be seen as its conceptual predecessor (Figure 1). Partially explaining this interest is the common (mis)conception that the purpose of artificial intelligence is to replace human intelligence with computational intelligence—also called “AI thinking” (van der Maden et al., 2022). As artificial intelligence does typically focus primarily on computational systems, cybernetics offers a conceptual framework for understanding the design of systems that are capable of purposeful (intelligent) behavior—regardless of whether the systems involved are natural, artificial, or a mix of the two.
Figure 1. Representation of cybernetics as the conceptual predecessor of AI used with permission from Lomas J. D. et al. (2021).
Cybernetics can be described as the interdisciplinary study of the design of governing systems, both human and machine, that use sensors and actuators to achieve a goal. The word cybernetics comes from the Greek word κῠβερνήτης (kubernḗtēs), which means “steersman” or “governor” (note that the verb “to govern” also comes from this Greek root). Cybernetics has been used to help design everything from robots to organizations. It has also been used to study human cognition and social interactions.
Box 2: Wellbeing theory.
Conceptualizing Wellbeing
According to the World Health Organization, wellbeing is “a state of complete physical, mental, and social well-being, and not merely the absence of disease or infirmity” (World Health Organization, 1946). In other words, wellbeing is more than just being physically healthy—it also includes being mentally and emotionally healthy and feeling like you belong to and are supported by a community.
The academic literature consists of many ways to conceptualize and operationalize wellbeing. Some common dimensions of wellbeing include physical health, mental health, emotional health, social health, and spiritual health. While there is agreement among scholars, a strong consensus on the definition of the concept of wellbeing seems absent (Dodge et al., 2012). Academics criticize the field on the basis that definitions are heavily dependent on the cultural background of the researcher and the application area of the research (Alexandrova, 2012). Considering the conceptual dissensus, a review by Cooke et al. (2016) identifies four main areas of wellbeing literature which will be used as a framework in this paper.
Hedonic models of wellbeing focus on both pleasure and happiness. This field is pioneered by Ed Diener’s tripartite model of subjective wellbeing (Diener et al., 1985, 1999), which considers satisfaction with life, the absence of negative emotions, and the presence of positive emotions, as vital components of wellbeing. This perspective typically considers all three of these constructs when assessing wellbeing, although many researchers focus primarily on Diener’s “Life Satisfaction Score” (Cooke et al., 2016; Linton et al., 2016). Eudaimonic models of wellbeing offer research that tries to account for more than the pleasure of a satisfied life. For example, Ryff’s six-factor model of psychological wellbeing focuses on self-acceptance, positive relations with others, autonomy, environmental mastery, purpose in life, and personal growth (Ryff and Keyes, 1995; Ryff and Singer, 2006). Further, Martin Seligman’s wellbeing theory encompasses both perspectives (hedonic and eudaimonic) stating that wellbeing (or flourishing) can be conceptualized in terms of positive emotion, engagement, meaning, positive relationships, and accomplishment (PERMA) (Seligman, 2011). A third category of wellbeing research focuses on quality of life (QoL). Cooke et al. (2016) note that while this term is often used interchangeably with wellbeing, it should be seen as a separate category because research on QoL generally conceptualizes wellbeing to encompass models of physical, psychological, and social functions. It is often associated with wellbeing towards or during end of life and living with a disability. A common assessment instrument is the Quality of Life Inventory developed by Frisch et al. (1992). Lastly, Cooke et al. (2016) describe a fourth category that is called wellness. They note that wellness approaches are often rooted in counseling and tend to be broader and less clearly defined and not necessarily associated with assessment instruments. Rather, wellness practitioners focus on a holistic lifestyle that can include many areas of health and functioning including spiritual health.
Community WellbeingWellbeing is often understood as centered around individual experiences. However, wellbeing for a person is also dependent on a “set of interlocking issues and constraints and embedded in a dynamic social context.” (Phillips and Wong, 2017). In this vein, Musikanski and colleagues consider community wellbeing to be defined as encompassing the domains of community, culture, economy-standard of living (which includes housing, food, transportation and information and communication technology), education, environment, government, health, psychological well-being, subjective well-being and affect, time balance and work (2020, p.41). In line with this categorization, community wellbeing has been operationalized to encompass similar categories (vander Weele, 2019; vander Weele et al., 2020).
A cybernetic system is a system where feedback loops are used to control the behavior of the system. At its simplest, a cybernetic system consists of just three parts: a controller, a sensor, and an effector or actuator. In a simple home thermostat, for example, the sensor is the temperature sensor, the effector is a switch that turns on a heater, and the controller is a mechanism that compares the sensor to a point set by the user. If the sensor value is below the set point, the controller turns on the heater, see Figure 2. If the sensor value is above the set point, the controller turns off the heater. More complex “smart thermostats” may have additional sensors (e.g., humidity, occupancy, etc.), and effectors (e.g., air conditioner, fan, etc.), and the controller may use a more sophisticated algorithm to determine when to turn devices on and off.
Figure 2. The schematic on the left (1) is an abstraction of a typical cybernetic system (adapted from Dubberly and Pangaro, 2007). The schematic on the right (2) shows a typical example of a cybernetic system, a thermostat.
Cybernetic systems are not restricted to simple devices like thermostats, however. Cybernetic systems can be found in living organisms (e.g., the feedback loops that control blood sugar levels), in social systems (e.g., the feedback loops that govern the interactions between people), and in artificial intelligence systems (e.g., the feedback loops that allow a robot to learn from its mistakes).
Cybernetics is closely related to the field of artificial intelligence. Both fields are concerned with the design of adaptive systems. A typical example is reinforcement learning, which is a machine learning method that uses rewards or punishment to train an agent to perceive and interpret its environment and take actions, see Figure 3. The fields also differ in their typical focus. For instance, cybernetics tends to be more concerned with understanding or designing feedback mechanisms that allow a system to govern its behavior, whereas artificial intelligence is more concerned with the design of algorithms that allow a system to learn from or adapt to its environment. Second, cybernetics tends to be more concerned with the design of natural systems (i.e., designing human governing systems), while artificial intelligence is more concerned with the design of computational systems.
Figure 3. A schematic of a typical reinforcement learning algorithm (adapted from Sutton and Barto, 2018).
Practically speaking, cybernetics offers a viewpoint for designing intelligent systems for governance that include both computers and people (e.g., Krippendorff, 2007, 2021; Glanville, 2009; Sweeting, 2016; Dubberly and Pangaro, 2019). Replacing human intelligence with computational automation is often not desirable, largely due to the special capacities of human interactions. Instead, there is a need to design systems, both natural and computational, that work together to create more intelligent behavior (i.e., more able to achieve goals in an uncertain environment). Cybernetics provides a means for conceptually uniting humans and artificial systems While keeping “humans in the loop” is a key design objective for many AI researchers, it is common for people to view artificial intelligence as an autonomous system that does not rely on human participation. It is as though, if human intelligence is still participating in the system, then the AI is not finished. Cybernetics may therefore offer a viewpoint for designing artificial intelligence in complex human systems where there is no desire to replace human intelligence with computational automation. This seemed especially apt in the context of supporting university administrators in supporting the wellbeing needs of their community during the COVID-19 pandemic.
It can be challenging to conceptualize the design of an AI system that makes such extensive use of human information processing and action. To conceptualize how an AI system can be designed in the context of a larger human system, we look to systems-thinking (e.g., Arnold and Wade, 2015) and cybernetic approaches (e.g., Wiener, 1961; von Foerster, 2003; Krippendorff, 2007). These perspectives point to how artificial systems may be designed to leverage human systems that are already functioning in a community, rather than trying to do everything autonomously. The cybernetic approach helps simplify the algorithmic design problem by focusing on a core process: generating a feedback loop between assessments of wellbeing and actions taken to enhance wellbeing. Furthermore, the cybernetics approach frees us from having to automate all processes into computational processes; we can design intelligence into a complex-sociotechnical system without having to make an entirely autonomous AI agent. Furthermore, we will show that such a system can be implemented rapidly and, over time, can be improved through iterative design, community feedback, and appropriate automation.
To summarize, based on theories of artificial intelligence and cybernetics, we sought to create an intelligent feedback loop capable of promoting community wellbeing. Figure 4 below visualizes the components that were involved in the feedback loops in our context. The design of the ability to sense the state of the system will be discussed next after which the process of defining the action space and processor (which we have explained are necessary for a cybernetic feedback loop) will be described.
Figure 4. A schematic that visualizes the different components that were involved in the feedback loops in our context.
The COVID-19 pandemic has been a major shock to societies around the world, with far-reaching consequences for mental health and wellbeing. In particular, the COVID-19 pandemic appears to have measurably reduced rates of human wellbeing around the world (Brodeur et al., 2021). The pandemic has also sparked interest in the role of large organizations in supporting wellbeing as an explicit criterion of organizational success. Universities, for instance, aim for a variety of organizational metrics of success (e.g., high graduation rates, large numbers of applicants, sustainability metrics, etc.). Increasingly, universities are recognizing student and staff wellbeing as explicit institutional priorities (Burns et al., 2020; Lomas D. et al., 2021).
During the onset of the COVID-19 pandemic in early 2020, the university administration asked us how they could best support the wellbeing of their staff and students who were now locked inside their homes due to government restrictions. This led to a two-year, iterative, community-led design process of a system that helped support mental-health and wellbeing. In this section we will address parts of that process that may translate to other contexts. The generalizability lies in the parts that have emerged from our cybernetic process—such as the context-sensitive assessment—and translate conceptually, not factually.
The most essential requirement of our system was to create the ability to sense wellbeing needs in the community. This “sensor” would be at the heart of any future AI system or cybernetic system for community wellbeing. Therefore, our primary goal was to design a wellbeing assessment instrument that was sensitive to the needs of our specific context—and capable of informing and motivating appropriate actions in response.
To develop our sensor, we did not choose one particular theory of human wellbeing (discussed in Box 2), but rather took a syncretic approach and drew from multiple theoretical traditions (e.g., Diener et al., 1999; Ryff and Singer, 2006; Seligman, 2011). This was justified because the goal of our assessment differed from the typical goal of conventional psychological approaches to measuring human wellbeing (discussed in Box 3), which is to create an accurate and theoretically valid measurement instrument. Instead, our goal was to create an actionable assessment: an assessment purposefully created to help inform and motivate concrete actions in the community to promote wellbeing.
Box 3: Wellbeing assessment.
Measuring Wellbeing
In a recent review of 99 self-report assessments of wellbeing in adults (Linton et al., 2016), the authors note that there are a vast range of instruments based on different fundamental theories. In their review, they suggest that two of the most influential theories are subjective wellbeing from Diener et al. (1985, 1999) and psychological wellbeing from Ryff and Keyes (1995). They conclude that different instruments may be suitable depending upon the needs of the context. This sentiment is echoed in another recent review of 42 instruments (Cooke et al., 2016).
Despite the lack of convergence in academia, a recent McKinsey report on wellbeing in Europe states that “a consensus is nevertheless emerging on how best to measure well-being. Researchers now tend to ask a basic question: “Overall, how satisfied are you with your life nowadays?” (Allas et al., 2020). This question, based on Diener’s life satisfaction measure, is appealing because it is short and because it allows for comparison between populations and over time. However, this measure does not specifically provide information about what is wrong or what might help.
Domain-specific Wellbeing
While life satisfaction scores provide an excellent means for comparison, an assessment of wellbeing may be intended to inform useful actions to support improved wellbeing. For instance, measures of employee satisfaction are typically undertaken with the goal of improving employee satisfaction. Because of this, wellbeing assessments should ideally be sensitive to the needs of a particular domain. In a primary school setting, for instance, bullying may have a significant effect on a student’s wellbeing; in a company setting, work-life balance may have a significant effect on employee wellbeing. In both cases, a domain-specific measure can be more useful for informing actions that may help improve wellbeing in the specific context.
When efforts are made to assess wellbeing in specific domains, like work or school, we refer to this as a domain-specific wellbeing assessment (e.g., Renshaw et al., 2014; Renshaw and Bolognino, 2014; Gregory et al., 2019). For instance, the College Student Subjective Wellbeing Scale (CSSWQ) has been designed to assess a combination of relevant components for college students which the researchers refer to as “covitality” (Renshaw et al., 2014). The different components include Satisfaction with Academics, Academic Grit, School Connectedness, Academic Self-Efficacy and College Gratitude.
COVID-19 and student wellbeing
The COVID-19 pandemic and lockdowns had a significant impact on subjective wellbeing around the world (e.g., Aucejo et al., 2020; White and Van Der Boor, 2020; De Pue et al., 2021; Hu et al., 2021; Khan et al., 2021). Common topics studied include anxiety, loneliness, psychological stress, and post-traumatic stress disorder (Xiong et al., 2020). These effects may be especially amplified in university students as many tend to live in small housing, away from their families, and experience financial instability. Aside from that, students were expected to complete their educational goals as if it were a normal situation despite the many factors restricting them (e.g., internet connection, lack of jobs, family challenges) (Crawford, 2020). According to the literature, students suffered from decreased motivation (Tan, 2020), hopelessness (Pretorius, 2021), and depression (Fawaz and Samaha, 2021). In response, some publications suggest that mindsets should be changed: for instance, grit and gratitude (Bono et al., 2020) or optimism (Genç and Arslan, 2021) are offered as approaches to improve wellbeing and cope with the pandemic. These recommendations, however, do not directly indicate how communities or organizations might respond to improving student wellbeing.
Box 4: Context-sensitivity, actionability, and assessment experience.
Context-sensitivity
Socially disruptive events, such as a pandemic, can trigger changes in human values and their prioritization in society (Daher et al., 2017; Klenk and Duijf, 2021). Due to isolation and lockdowns during COVID-19, there seemed to be many factors that were previously not considered as critical to wellbeing. For instance, the experience of one’s home working-environment—factors such as ‘Wi-Fi quality’ or ‘a dedicated work desk’ are generally not considered by wellbeing assessment instruments. Yet, in the context of COVID-19, these factors became relevant to the wellbeing experience of community members. It is a general challenge for design research to identify the various mechanics that affect wellbeing (Fokkinga et al., 2020). Therefore, we needed a method that could identify important new factors—to identify if we were asking the right questions to the right people. This method should help identify what factors are currently actively impacting wellbeing in a manner that can point to where interventions should and can be designed.
Actionability
“Off-the-shelf” measures of wellbeing, mainly found in psychological literature (as discussed in the previous section), are oftentimes constructed primarily for validity and reliability—not actionability. What we define as actionability is the usefulness of a measure for informing helpful actions. For example, the Satisfaction with Life Scale (SWLS; Pavot and Diener, 2009) has been proven to be a strong cross-cultural measure of a person’s wellbeing, but it is not designed to indicate how to improve wellbeing within a specific context. To illustrate, imagine you are an administrator aiming to improve the wellbeing of your members in your organization, knowing that the average member in your community has an SWLS score of 21, and a PANAS score of 26, does not immediately inform you on where you might take actions to improve these scores. The scores must be related to contextual factors in order to be meaningful. On the other hand, in the domain of universities, the College Student Subjective Wellbeing Questionnaire (CSSWQ; Renshaw and Bolognino, 2014) may be more actionable than a general measure (such as the SWLS) due to the granularity of its questions. But, despite this granularity, the questions do still not directly point to opportunities for taking action. Current measures of wellbeing may be reliable and valid and yet the information provided by these measures may not be sufficiently concrete that communities might use to take action to support improved wellbeing. Note that it is not specifically items that provide more actionable information, it is the assessment instrument as a whole, combining “off-the-shelf” measures with contextualized items. Hence the term context-sensitive assessment—not measure—of wellbeing.
Assessment Experience
The assessment experience is important for two basic reasons. First, a positive experience can lead to improved participant engagement and data quality (Stocké and Langfeldt, 2004; Baumgartner et al., 2021). Second, the experience of assessing wellbeing has the potential to offer an intervention in and of itself. Namely, reviewing different facets of one’s own life has the potential to lead to constructive change and experiences of improved wellbeing. While this second rationale for improving the wellbeing assessment experience was not quantitatively evaluated in this study, it was a driving motivation for the design of My Wellness Check.
Popular measures of wellbeing often focus on generalization. That is, they seek to validate a measure that can be used for comparing multiple contexts. Diener’s single item life satisfaction measure is a good example: on a scale of 0–10, how satisfied are you with your life as a whole? This measure (and its many variants) has been extremely useful for comparing wellbeing in different contexts. This measure is “actionable” insofar as a low score shows that something should be done. However, attributing the low score to specific causes is problematic—making it difficult to take specific actions. For this reason, we sought to devise new measures of wellbeing that were highly specific to the context of the community we sought to serve. We anticipated that a context-sensitive assessment would be more actionable (because it deals with specifics) as well as being more sensitive to the needs of the community.
Therefore, we used factorized models of wellbeing as an organizing principle to help identify concrete and specific questions that could support community action. Figure 5 shows how various theoretical factors underpinning wellbeing may manifest within our context. For instance, many different models of human wellbeing recognize Material Wellbeing as an important factor of wellbeing (e.g., Sirgy, 2018). However, what material wellbeing means is likely to differ from one context to the next. In the context of wellbeing during COVID-19, for instance, we asked about the ergonomic quality of home workspaces—which can be seen as a causal indicator (Wong and Law, 1999)—as part of an effort to assess the influence of the home working environment on wellbeing. According to Mackenzie et al. (2011), it is specifically these sorts of causal indicators that belong of survey instruments.
Figure 5. A selection of images depicting the appearance of the survey experience. The first screen shows a rating item about life satisfaction, the second, a checkbox item about physical wellbeing, and the third item shows a free text item about their additional wellbeing needs.
Across all iterations, the My Wellness Check assessment considered a diverse range of indicators for community wellbeing: academic experience, anxiety, autonomy, behavior, belongingness, competence, coping strategies, COVID-19 measures, depression, drugs and alcohol, exercise, expected university support, finances, home working environment, life satisfaction, loneliness, mood, motivation, nutrition, optimism, overall physical health, personal growth, purposefulness, remote education, sleep, study performance and subjective mood. Additionally, various surveys were consulted in constructing the assessment items, including “The Warwick-Edinburgh Mental Wellbeing Scale (WEMWBS)” (Tennant et al., 2007), “PERMA-profiler” (Butler and Kern, 2016), “Satisfaction with life Scale (SWLS-5)” (Diener et al., 1985; Pavot and Diener, 2009), “Harmony in Life Scale (HILS-3)” (Kjell and Diener, 2020), “World Health Organization (WHO-5)” (Topp et al., 2015), “Psychological Wellbeing Scale (PWB)” (Ryff and Keyes, 1995; Ryff and Singer, 2006), “Wisconsin Longitudinal Study (WLS)” (Piliavin and Siegl, 2007), “Midlife in the United States (MIDUS)” (Radler and Ryff, 2010), “National Survey of Families and Households (NSFH II)” (Springer and Hauser, 2006), “College Student Subjective Wellbeing Questionnaire (CSSWQ)” (Renshaw and Bolognino, 2014), and “Student WPQ” (Williams et al., 2017).
The design of our community wellbeing assessment combined traditional psychological methods for survey development (Boateng et al., 2018) with a variety of human-centered design approaches, particularly community-led design methods (Costanza-Chock, 2020). By community-led design, we specifically mean that we involved community members and community leaders in the informal design and informal evaluation of the assessment instrument (see Lomas and van der Maden, 2021). Rather than approaching this in a strictly systematic manner (typical of psychological survey development), we encouraged various levels of university leadership to “weigh in” on the types of questions to be asked (typically in response to a proposed concrete example). By engaging with community leaders, we were able to create a greater sense of investment and enthusiasm for the implementation of the assessment. Additionally, there were three concepts central to the development of the instrument: context-sensitivity, actionability, and assessment experience—see Box 4.
To balance out the needs of community leaders with the needs of the community at large, we also put significant effort into gather diverse perspectives across the many iterations of the survey. At first, we used informal, semi-structured interviews with about 15 students and 7 staff members to gather perspectives on current needs and ideas regarding the academic experience and overall community wellbeing. These interviews were focused on identifying concrete and specific indicators associated with different theoretical factors of wellbeing. Together with the priorities of university leadership, these community interviews helped inform the focus of our initial set of survey questions. Once an initial survey experience was developed, a sample of about 40 diverse students were asked to complete the entire survey over video chat. While offering informed consent and promising anonymity, we encouraged participants to comment aloud on individual survey items and give critical feedback. All content data was discarded for privacy reasons. However, this observational method was helpful for improving the relevance of the questions, reducing ambiguity about the meaning of questions and generally ensuring that important topics were not overlooked. Over the course of this development (which took approximately 4 weeks), many subtle iterations to the survey were made to ensure appropriate pacing and sequencing.
This iterative design and survey development continued even into the subsequent deployments of the survey, discussed in the following section. An example of the mobile user interface is presented in Figure 5; this shows the effort taken to create a motivating and positive survey experience. It also shows the tight integration of quantitative data collection procedures with opportunities to gather the voice of respondents in free text boxes.
After the initial deployments of the survey, statistical data about individual items made it possible to identify items that well predicted our central measure Life Satisfaction and items that did not. Furthermore, following the survey, all respondents were offered the opportunity to leave critical feedback about improving the survey. This strong focus on community-led iteration was hypothesized to create a better survey experience and to produce a more sensitive sensor of community wellbeing. As will be discussed, these hypotheses were tested through a controlled experiment.
This section presents an overview of the deployment of our assessment of community wellbeing to 27,270 students and 6,347 staff members staff members (total university population during the last iteration, November 2021). Separate surveys were designed for students and staff. The number of participants and a summary of each iteration can be found in Table 1.
Table 1. An overview of the different iterations of wellbeing assessment conducted at Delft University of Technology.
All students and staff received an email in both Dutch and English that invited them to participate in the study. The email contained a link that led them to an online version of the survey that could either be completed on a tablet, phone, or desktop. The welcome text of the assessment provided participants with information about the anonymity of their data (the limitations to guaranteeing their anonymity will be addressed in the discussion), the fact that the assessment was compliant with GDPR standards, and thus provided them with enough information to give their informed consent. All data were anonymized.
For reasons of space, we focus on our longitudinal data on student results. Table 2 presents student wellbeing data over time, based on rating-type questions (i.e., requesting a rating on a scale from 0 to 10). Table 3 shares data listing the percentage of students selecting that they agree with a specific statement (checkbox items).
Table 2. An overview of data gathered in five iterations through scaled items about student wellbeing at Delft University of Technology.
In addition to statistical measures, we also designed the survey to support the collection of written text that represented the “voice” of students and staff. Open-ended questions were important for assessing community needs and also to gather possible actions that the university might take to help. These questions included: “What contributes to your sense of belonging and community at Delft University of Technology?;” “What aspects are you missing?” and; “Do you have ideas on how Delft University of Technology might help support student motivation?”
Open-ended questions were useful for eliciting concrete statements of student needs as well as serving as a source for specific ideas for the improvement of wellbeing. The quantitative data was useful for showing patterns across different university populations (e.g., could see the degree of physical health issues in international students v. local students). Written responses were often a source of specific ideas for organizational improvement. For instance: “I really miss the in-between coffee chats with fellow students and the company. I want to see people”; “I really hope that hybrid learning can continue and that I can finish my master’s degree while being in my home country.”; “Organize silent discos with circles on the ground so that you can dance just in your own space!”; “I’m so happy to be back on campus, the facilities are awesome. However, it is hard to find a quiet place where I can talk in videocalls.” and; Because of [COVID-19], some know each other very well and others do not. It’s hard to join a group, especially if you do not know anyone at all. The university could help organize meeting groups for international students.”
The previous section focused on the design of a sensor for community wellbeing. The purpose of this sensor was not just to generate a measure, but to serve in a cybernetic feedback loop that could motivate subsequent community actions—actions that could help contribute to improvements in community wellbeing.
Therefore, there were two core tasks required in designing for community action: 1) identify possible actions that could plausibly improve community wellbeing and; 2) motivate community actors to take appropriate actions. In practice, we made an effort to combine these together: when community actors were engaged in a process to help them identify useful actions for improving wellbeing, this was a key motivation for their subsequent actions.
Following each survey iteration, we held community-led design workshops with approximately 20 to 40 diverse online participants. Workshop participants included students elected to the student council, staff counselors (including psychologists and employees involved in mental health coaching), deans, upper administrators, the vice-rector, and various other students and staff all from across the university. Prior to each workshop, each participant was given several hundred written responses to review. With the instruction to identify unique needs and their ideas for how to help. At the workshop, small groups synthesized and discussed these lists of needs and ideas. Following a whole group discussion about the “doability” and “urgency” of different ideas, the lists of needs and ideas were compiled together for presentation to university upper administration. The aim was to analyze qualitative data to inform the communities about the wellbeing needs emerging from the survey and to collect ideas for improvement. To maximize the potential for action, we took special care to involve administrative decision-makers in reading and reviewing survey responses. Below, Table 4 highlights some of the ideas and the institutions that may be able to act in accordance with them.
Table 4. Many examples of ideas for action coupled with a potential actor (i.e., ‘a cybernetic processor’) and their respective areas of need as communicated back to the community.
Motivating community action also occurred through the presentation of data to various stakeholders. For instance, following data collection events, data presentations were made to the executive board of the university and to the board of education. Several policies can be directly linked to the results of our analyses. For instance, the university organized a ‘Wellbeing Week’ with various activities related to the outcomes of the report (i.e., supporting sleep, exercise and socializing). More concretely, as we found that the home working environment was a strong predictor of wellbeing, the university funded a program to provide ergonomic chairs and desks. A subtler example came from the impact of many students expressing that they’d appreciate a more human communication approach—e.g., the dean sending out emails asking students how they were doing, in a very personal manner. This finding encouraged the university to provide guidance on altering the tone of voice in official emails. Next to administrative changes, action was also taken from a community perspective. For example, many PhD students that started in times of corona expressed they missed the opportunity to meet people and have “spontaneous social contact.” This inspired a program called ‘PhD Speed Dating’ where PhDs were assigned to a random person on zoom so they could chat and expand their social network.
Beyond these top-down policies, data were also used to motivate bottom-up community responses. Infographics were designed (see Figure 6) to communicate results to educators, staff and students at the university. These materials did not just incorporate quantitative survey data, but also the qualitative “voice” of students. Educators were invited to take these results in consideration when designing courses, lectures, and interactions with students. One responded: “When something resonates with me and I empathize with it, I feel the urgency to act and implement improvements in my practice.”
Figure 6. This figure shows parts of the infographic that was shared with all educators within the university, outlining both the quantitative but particularly the qualitative data. The infographic was shared in a university-wide newsletter and was accompanied by a short summary of the study and the message that this infographic could help inspire educators in creating an educational environment that supports wellbeing.
When measures against COVID-19 allowed it, community workshops were also organized in person. A community of researchers and designers were engaged in a workshop inspired by the World Café format, which is based on the belief that people within an organization, if put in a social environment open to dialogue and exchange, can find solutions even to complicated issues (Löhr et al., 2020).
We promoted and designed initiatives enabling the student community to take action and create impact on itself as well. All answers to the question “What daily routines are working well for you?” were collected and analyzed. This resulted in several visuals, which were shared in episodes once a week by study associations in their social media accounts, see Figure 7. The four episodes covered important student topics that emerged from the survey responses’ analysis itself and consisted of first-person sentences about positive routines. The goal was to inspire students with routines that worked for their peers, hence having a higher chance to work for them as well. Other bottom-up results include student projects focused on wellbeing. One student, for instance, created a recommendation system to help students optimize their living situation on a budget and promoted this to thousands of students.
Figure 7. This figure shows a selection of the ‘It works for me!” wellbeing campaign launched in cooperation with local student associations. The goal was to gather routines or rituals from the results of the survey that students use to promote their wellbeing and share them with their peers.
A quantitative evaluation of our overall system remains challenging—for instance, it would be largely infeasible to conduct a controlled experiment involving multiple communities. For this reason, we have sought to quantitatively evaluate parts of the system. In this next section, we share the results of a controlled experiment conducted to compare our wellbeing assessment to other wellbeing assessments that were developed using standard psychological methods (Boateng et al., 2018). Because our community-led design methods so actively engaged diverse stakeholders in our community, there is a possibility that it may have led to reduced measurement efficacy. We chose the Warwick-Edinburgh Mental Wellbeing Scale (WEMWBS) and the College Student Subjective Wellbeing Questionnaire (CSSWQ) because they are widely used wellbeing assessment instruments and suitable in the context of our survey intervention. That is, we could not expect students to complete a full Positive and Negative Assessment Scale (PANAS)—to point to another widely used assessment instrument. It would have been too burdensome and distinct from the survey experience they were used to after four iterations (i.e., MWC).
In comparison to existing and validated assessments, we aimed to test the following two hypotheses. We predicted that our context-sensitive assessment of wellbeing would achieve:
1. improved prediction of life satisfaction (a core measure of human wellbeing)
2. improved measures of user experience
These hypotheses were tested by randomly assigning samples of the research population to answer one of three questionnaires (WEMWBS, CSSWQ, MWC) after which their evaluation of their experience had been compared. This controlled experiment was conducted during the fourth iteration of the student survey, June 2021, see Table 5.
Table 5. An overview of the different iterations of wellbeing assessment conducted at Delft University of Technology.
2,062 student participants were randomly assigned to different versions of the questionnaire: 12.5 percent of all participants would receive the WEMWBS, 12.5 percent would receive CSSWQ and the remainder would receive My Wellness Check (75 percent). These proportions were chosen because we have conducted our study in a real-world setting, meaning that the objective of the survey had to remain true to the initial goal—gathering data on student and staff wellbeing during COVID-19 to inform institutional action. The WEMWBS and CSSWQ were chosen because they are frequently used and validated measures of global and domain-specific wellbeing.
Prior to beginning the experimental questions, all participants answered a common question about their life satisfaction. Following the experimental questions, participants were asked to complete seven questions about their questionnaire experience based on Stocké and Langfeldt (2004) and Baumgartner et al. (2021).
Table 6 shows that our context-sensitive assessment improved the overall sensitivity of the assessment and enhanced the survey experience for participants. To calculate sensitivity, we used a regression model to predict individual Life Satisfaction scores using the responses to questions from the three surveys. My Wellness Check (MWC) produced a higher R2 (a measure of predictive fit) than the WEMWBS or CSSWQ. Including all MWC items in the model produces an R2 of 0.75 while restricting the model to only the checkbox items (not the 0–10 scale questions) still produced an R2 of 0.53, exceeding the R2 of a model with all items in the WEMWBS (R2 = 0.51) and a model with all items in the CSSWQ (R2 = 0.42). Then, to compare participant ratings of the survey experience, a MANOVA showed a significant positive difference (p < 0.0001) between My Wellness Check (MWC) and WEMWBS and CSSWQ across all items listed in Table 6. The sole exception was that MWC was significantly more exhausting (p < 0.0001), see Table 6. This shows that participants taking the MWC survey found the experience to be of significantly greater value, significantly more engaging, significantly more worthwhile and significantly more fun. All statistical tests were conducted using JMP 16.
The aim of this article is to demonstrate an approach to designing systems for improving community wellbeing. Based on a proposed framework for designing AI systems, we highlight the value of cybernetic theory when designing intelligent systems that involve complex human communities—in the sense that AI theory helps us to understand that feedback loops are a key feature of complex systems, and that involving humans in the design of feedback loops is necessary to create intelligent systems.
Based on this theoretical background, we share a case study in which we design an intelligent feedback loop to promote university student and staff wellbeing during COVID-19. Our work focuses on the use of community-led and human-centered design activities to produce “sensors” of wellbeing (a context-sensitive wellbeing assessment), “actuators” of wellbeing (a space of action that can be taken by different stakeholders in our community), and “processors” of wellbeing (which enable the transformation of sensor data into action). In our case study, we describe the longitudinal fluctuation of community wellbeing over 2 years of the COVID-19 pandemic and explain the range of actions taken in response. To evaluate our efforts, we also share the results of a controlled experiment which indicate that our wellbeing assessment has improved sensitivity to wellbeing and provides an improved user experience in comparison to other “off-the-shelf” wellbeing assessment instruments. Our work demonstrates that community-led and human-centered design approaches may aid in the development of systems that can enhance and support wellbeing. Below, is a general schematic of our framework. Note that these steps apply to any complex system be they predominantly artificial or humane.
The remainder of our discussion shares a vision for describing how our “AI for Wellbeing” approach might generalize to other complex systems, including online social media systems and national governments. We then discuss several important limitations to this approach. Finally, we reflect on the relative merits of “cybernetic thinking” in the design of systems that seek to integrate human and machine intelligence.
The case study in this article is specific to the context of our own university during the COVID-19 pandemic. The approaches and methods may be generally applicable to other universities or organizations that seek to prioritize community wellbeing. Beyond this, our framework and methods show promise for guiding the design of wellbeing feedback loops within other complex sociotechnical systems. In other words, our approach is not necessarily a blueprint for the next “COVID-22” but rather a way to understand how systems can deal with novel or urgent phenomena that affect the global society at large.
For instance, approaches taken here may offer insights for the integration of human wellbeing into the optimization of contemporary social media platforms like Facebook. To provide context, the CEO of Meta said: “we feel a responsibility to make sure our services aren’t just fun to use, but also good for people’s wellbeing” (2018). This statement introduced a new “wellbeing” metric called Meaningful Social Interactions (MSI). Three years later, however, the ‘Facebook Files’ (Zuckerberg, 2018; Hagey and Horwitz, 2021) showed that there are still many aspects of social media services that harm user wellbeing. Our work demonstrates a system design approach and community-led design methods for human wellbeing feedback loops that may be useful in the design of social media services and other sociotechnical systems. For instance, Facebook’s MSI metric could be refined and expanded with wellbeing data collected through the community-led design methods and system design approach described in this article.
Our work may also generalize to societal governance, in general. During the COVID-19 pandemic, wellbeing in Europe fell to its lowest level in 40 years (Allas et al., 2020). Wellbeing is often not explicitly valued in discussions of economic growth and decline. However, McKinsey and Co proposed a model of the monetary value of wellbeing by considering how much additional income a person would need to receive in order to raise their wellbeing by a desired amount. With this model, McKinsey estimated that wellbeing losses during the COVID-19 pandemic cost more than three times as much as the economic losses (i.e., reduction in GDP).
Increasingly, national governments are shifting from a single-minded focus on economic growth and turning to a more integrated ‘wellbeing economy’ focus (Fioramonti et al., 2022). Since the country of Bhutan changed its constitution in 2008 (Ura et al., 2012) to focus on “Gross National Happiness,” the idea of wellbeing-based governance has become an intense topic of research. The Organization for Economic Co-operation and Development (OECD) promotes and maintains a measure of country-wide happiness and wellbeing that is used for ranking and policy purposes (Mizobuchi, 2014). Clearly, there are moves to make citizen wellbeing a more explicit measure of government success.
Here, we wish to communicate a design vision for governance for wellbeing that is focused on the experience of citizens. What do we want it to feel like to have governments or even smaller organizations work to maximize the wellbeing of their people? After all, there are always risks that come from focusing too much on optimizing a single metric (Rambur et al., 2013; Stray, 2020; Thomas and Uminsky, 2020). We turn to metaphor to communicate our design vision (Hekkert and van Dijk, 2011). An AI-based optimization of human wellbeing may feel unnerving, as though we have put a machine in charge of running society. Instead, our vision for optimizing societal wellbeing aims to feel more like a deliberative democratic process. Perhaps governments could use systematic wellbeing assessments as a participatory ritual (akin to voting day) to make it easier to “listen to the voices of the people.” Then, we envision that the collective review of citizen needs and wants could feel more like deliberative, “town hall” democracy: a messy, time-consuming but intensely social process of figuring out what do people need? and what actions can be taken to help? Our case study shows the potential for using human-centered and community-led methods to optimize wellbeing in organizations large and small; the above design vision aims to communicate how “AI for Wellbeing” might be extended to “governance for wellbeing” in a humanistic manner.
The goal of an “AI for Wellbeing” system is to improve human wellbeing. Designing such a system requires, foremost, measurements of human wellbeing. But it also requires the ability to take actions in response to measurements. In an ideal world, the actions taken in response to wellbeing assessments would be (1) observable, (2) theoretically grounded (or to have a known mechanism of action and some predicted effect), and (3) empirically evaluated. However, in the case of a university during the COVID-19 pandemic, these criteria were not met. It was very difficult to know precisely what actions community members took in response to the assessment data. Further, few actions had a clearly defined theoretical model of how they were likely to impact wellbeing. Finally, none of the actions taken were evaluated statistically. Indeed, even if some actions were evaluated, there is little to suggest that they would have had the same effect at another point in time. As a result, it was difficult to evaluate the efficacy of our overall system. In other words, whether the human-centered activities conducted were the best best of all possible actions is indeterminable nor was it verifiable whether our approach was the optimal approach.
While we cannot make causal claims about the benefits of a wellbeing feedback loop, it may be possible to observe the functioning of our system like a prototypical cybernetic system, a thermostat. In a thermostat, a heater will stay on until the temperature reaches a desired range. In our case, once wellbeing returned to a range deemed “normal,” the system goal had been reached and the university was able to shift resources to “business as usual.” The community motivation for promoting wellbeing is analogous to the heater in this analogy. When wellbeing fell below a certain level, the university community was motivated to take a wide variety of actions. When wellbeing rose above an acceptable level, the motivation to focus on wellbeing was diminished. Like a thermostat, My Wellness Check turned up the motivation for action while the assessed need was high and reduced the motivation when the assessed need was low.
Designing this in a real-world university setting involved more than creating technologies, developing surveys, and executing human-centered design methods. It also required a messy, informal and unscientific political engagement by ourselves, as researchers and designers. This engagement was essential for getting buy-in and participation from multiple university stakeholders. Yet, through the dozens of meetings necessary to implement this system, we were able to leverage the community expertise of, for instance, psychological counselors, student advisors, human resource personnel, student council members and administrative leaders. This process is vastly more involved than simply “keeping humans in the loop” within a technical system. This limitation (or feature) will be relevant to the design of other intelligent systems for improving wellbeing within large-complex social environments: messy, democratic political processes may be required in addition to software development and user interface design. This creates new opportunities and demands for the appropriate role of human-centered designers in large, complex socio-technical systems.
In this section, informed by our case study, we discuss our perspective on the design of AI systems applied within complex human systems. In the field of human-centered design, “design thinking” is a process for creative problem solving that is often used in the design industry (Pressman, 2019). In parallel, “AI thinking” can be described as a process for computational problem solving that is often used in the AI industry; in rough strokes, “if there is a problem, AI may be the solution.” However, many real-world problems are far more complex than AI algorithms can handle, particularly when AI is conceived as a fully autonomous agent. These real-world problems might include emotional engagement with other human participants or negotiating values or ethics. As a result, “AI thinking” has the potential to result in negative outcomes when it focuses AI designers on the production of fully autonomous systems that replace human intelligence with computational intelligence. A narrowed focus on algorithmic competence can result in the design of disembodied AI systems that fail to respect or leverage existing human capabilities in real-world systems (Gillespie, 2014; Krippendorff, 2021; Pangaro, 2021). For instance, “AI thinking” has produced product offerings promising to use complex data to provide medical diagnoses or educational recommendations. These offerings often fail because human doctors understandably distrust a “black box” diagnosis, just as teachers tend to distrust a “black box” curriculum selection (London, 2019; Wang et al., 2020). Instead, systems work better when they are not designed to be fully autonomous, but rather designed to provide services that can couple with existing workflows in an “unremarkable” manner (Yang et al., 2019).
“Human-centered AI” offers the opportunity to design AI systems designed to work in concert with humans, not just to replace them. Humans will remain better at understanding people and responding to their emotional needs for the foreseeable future. Complex ethical or value-laden decisions will continue to require human stakeholders to negotiate. AI design methods need to consider the limitations of AI systems and design AI systems capable of working in concert with humans and existing organizations—supporting humans in their decision-making, rather than seeking to replace them. If AI systems are to be used by humans, AI systems need to be designed to meet the needs of human users.
However, we suggest that there are important conceptual issues that emerge from trying to strictly distinguish between artificial intelligence and human intelligence. Many artificial processes, rules or algorithms exist within organizations that are designed to produce intelligent outcomes. Should the artificial design of intelligent human processes be considered artificial intelligence? If artificial intelligence is defined as “the artificial design of intelligent processes,” then this means that artificial intelligence does not require computational algorithms. Instead, algorithms might be merely written down and executed by humans. For example, “mastery learning” is an educational method involving a simple algorithm: if students demonstrate mastery on a topic test, they can proceed to the next topic, otherwise they are to continue to learn and master the topic at hand (Bloom, 1973). Mastery learning can be supported by computers, but it can also be implemented as a non-computational cybernetic feedback loop (e.g., just with teachers and paper tests).
Artificial intelligence could be defined as any kind of intelligent information process that is artificially designed—whether the process uses silicon microprocessors. This would broaden the scope of artificial intelligence to include all kinds of governing systems, not just those that rely on advanced computers. Consider the example of an autopilot; in the context of a self-driving car or even in a modern airplane, autopilot is certainly classified as a type of artificial intelligence. However, the first autopilot for an airplane was a mechanical system and was invented in 1912. If we take AI to mean “intelligent process that is artificially designed,” then the implication is that there is a great deal of AI that does not involve computers. This could have far-reaching implications for how we think about AI systems and their impact on society.
For the sake of convention, some may wish to adhere to a popular conception of artificial intelligence that might be described as “an autonomous algorithmic system that uses advanced computational techniques to accomplish non-trivial goals in a manner that precludes human intelligence.” In this case, the artificial design of intelligent processes that do not meet this definition might be termed “intelligent system design,” rather than “artificial intelligence.” Importantly, even non-computationally focused work may still contribute to the field of artificial intelligence, particularly when it demonstrates the application of artificial intelligence theory and methods to the design of intelligent systems.
In comparison to Design Thinking or AI Thinking, “Cybernetic Thinking” describes the design of intelligent systems, where the intelligence in the system relies on sensor/actuator feedback loops (van der Maden et al., 2022). By focusing on the design of information feedback loops, where a system’s performance is used to modify the system’s behavior, Cybernetic Thinking can be applied to the design of any goal-driven system, whether it is computational or not. For example, cybernetic thinking might be used in the design of educational systems (as in the description of mastery learning, above) or in employee performance reviews, where indicators of employee performance are used to modify ongoing performance. Cybernetic thinking may be valuable because it focuses on the dynamics of whole systems (including humans and machines), rather than naively focusing on popular computational algorithms. In our case, we found cybernetic thinking to be invaluable in the design of feedback loops to promote community wellbeing.
Wellbeing is not just an individual concern, but a community and a societal concern. By designing a system to assess and support community wellbeing in the times of COVID-19, we have demonstrated how to systematically prioritize wellbeing as an explicit objective within large, complex social systems. Our work makes the following key contributions:
First, based on theories of artificial intelligence and cybernetics, we contribute an approach to designing feedback loops to support human wellbeing at a community scale. This approach is highly relevant to sociotechnical systems that have large numbers of individuals. In the context of our case study, we are working in a very large university with over 30,000 students and staff.
Second, we contribute a specific case study applied to the context of COVID-19. This case study provides practical examples of the application of our approach, such as the community-led design of online surveys to generate valuable feedback in the form of wellbeing data from our university community. This feedback is then fed back to the community in the form of qualitative assessments of needs and a summary statistics providing visual representations of how wellbeing changed over time and across sub-communities (e.g., academic and non-academic staff).
Third, we contribute an approach for using human wellbeing data to inform sociotechnical system design. We use our wellbeing data to generate insights and recommendations for improvements to our university’s COVID-19 response. For example, we provide action recommendations to particular stakeholders in the university. This is an important contribution as it provides a concrete example of how wellbeing data can be used to improve sociotechnical systems.
Finally, the result of adopting a cybernetic framework and using human-centered and community-led design methods, is the development of a novel context-sensitive wellbeing assessment. To evaluate our instrument, we conducted a controlled experiment: in comparison to other validated wellbeing assessment instruments, we found that our context-sensitive wellbeing assessment was more highly rated by participants and also demonstrated stronger predictive validity. We also present qualitative evidence showing that our assessment yields more “actionable” data for motivating institutional and community action.
In our approach to designing interactive systems to support wellbeing, we have shifted from a focus on individual user needs to designing for communities and institutions. We have also shifted our thinking from designing a static product to designing an intelligent product-service system—a system designed to operate as a cybernetic loop within a large and complex socio-technical system. Finally, our mindset shifted as we accepted that we were not the experts leading the design so much as facilitators of a community-led design process. These shifts may be subtle, but they represented an enormous leap from our initial perspectives on applying HCI, design and AI methods to create tools to support wellbeing during COVID-19. Our argument is that any future work on aligning AI systems with values like wellbeing and democracy will benefit from a similar process as the one presented in this paper.
The original contributions presented in the study are included in the article/supplementary material, further inquiries can be directed to the corresponding author.
The studies involving human participants were reviewed and approved by HREC TU Delft. The patients/participants provided their written informed consent to participate in this study.
The research is primarily conducted by WM and DL who also contributed most of the writing with guidance and insight from PH. All authors contributed to the article and approved the submitted version.
The authors declare that the research was conducted in the absence of any commercial or financial relationships that could be construed as a potential conflict of interest.
All claims expressed in this article are solely those of the authors and do not necessarily represent those of their affiliated organizations, or those of the publisher, the editors and the reviewers. Any product that may be evaluated in this article, or claim that may be made by its manufacturer, is not guaranteed or endorsed by the publisher.
Alexandrova, A. (2012). Well-being as an object of science. Philos. Sci. 79, 678–689. doi: 10.1086/667870
Allas, T., Chinn, D., Sjatil, P.E., and Zimmerman, W., 2020. Well-being in Europe: Addressing the high cost of COVID-19 on life satisfaction. Mckinsey and Company.
Arnold, R. D., and Wade, J. P. (2015). A definition of systems thinking: a systems approach. Proc. Comput. Sci. 44, 669–678. doi: 10.1016/j.procs.2015.03.050
Aucejo, E. M., French, J., Ugalde Araya, M. P., and Zafar, B. (2020). The impact of COVID-19 on student experiences and expectations: evidence from a survey. J. Public Econ. 191, 104271–104271. doi: 10.1016/j.jpubeco.2020.104271
Baumgartner, J., Ruettgers, N., Hasler, A., Sonderegger, A., and Sauer, J. (2021). Questionnaire experience and the hybrid system usability scale: using a novel concept to evaluate a new instrument. Int. J. Hum. Comput. Stud. 147, 102575–102575. doi: 10.1016/j.ijhcs.2020.102575
Bloom, B. S. (1973). Recent developments in mastery learning. Educ. Psychol. 10, 53–57. doi: 10.1080/00461527309529091
Boateng, G. O., Neilands, T. B., Frongillo, E. A., Melgar-Quiñonez, H. R., and Young, S. L. (2018). Best practices for developing and validating scales for health, social, and Behavioral research: a primer. Front. Public Health 6:149. doi: 10.3389/fpubh.2018.00149
Bono, G., Reil, K., and Hescox, J. (2020). Stress and wellbeing in urban college students in the U.S. during the COVID-19 pandemic: can grit and gratitude help? Int. J. Wellbeing 10, 39–57. doi: 10.5502/ijw.v10i3.1331
Brodeur, A., Clark, A. E., Fleche, S., and Powdthavee, N. (2021). COVID-19, lockdowns and well-being: Evidence from Google trends. J. Public Econ. 193, 104346–104346. doi: 10.1016/j.jpubeco.2020.104346
Burns, D., Dagnall, N., and Holt, M. (2020). Assessing the impact of the COVID-19 pandemic on student wellbeing at universities in the United Kingdom: A conceptual analysis. Front. Educ. 5:5. doi: 10.3389/feduc.2020.582882
Butler, J., and Kern, M. L. (2016). The PERMA-profiler: a brief multidimensional measure of flourishing. Int. J. Wellbeing 6, 1–48. doi: 10.5502/ijw.v6i3.526
Carter, K., Banks, S., Armstrong, A., Kindon, S., and Burkett, I. (2013). Issues of disclosure and intrusion: ethical challenges for a community researcher. Ethics Soc. Welf. 7, 92–100. doi: 10.1080/17496535.2013.769344
Chan, L., Swain, V. D., Kelley, C., de Barbaro, K., Abowd, G. D., and Wilcox, L. (2018). Students’ experiences with ecological momentary assessment tools to report on emotional well-being. Proc. ACM Interact. Mob. Wearable Ubiquitous Technol. 2, 1–20. doi: 10.1145/3191735
Cloutier, S., Ehlenz, M., and Afinowich, R. (2019). Cultivating community wellbeing: guiding principles for research and practice. Int. J. Community Well-Being 2, 277–299. doi: 10.1007/s42413-019-00033-x
Coburn, A., and Gormally, S. (2020). Defining well-being in community development from the ground up: a case study of participant and practitioner perspectives. Commun. Dev. J. 55, 237–257. doi: 10.1093/cdj/bsy048
Cooke, P. J., Melchert, T. P., and Connor, K. (2016). Measuring well-being: a review of instruments. Couns. Psychol. 44, 730–757. doi: 10.1177/0011000016633507
Costanza-Chock, S., 2020. Design justice: Community-led practices to build the worlds we need. The MIT Press.
Crawford, D.N., 2020. Supporting student wellbeing during COVID-19: Tips from regional and remote Australia.
Daher, M., Carré, P. D., Jaramillo, A., Olivares, H., and Tomicic, A. (2017). Experience and meaning in qualitative research: a conceptual review and a methodological device proposal. Forum Qual. Sozialforschung 18. doi: 10.17169/fqs-18.3.2696
De Pue, S., Gillebert, C., Dierckx, E., Vanderhasselt, M.-A., De Raedt, R., and Van den Bussche, E. (2021). The impact of the COVID-19 pandemic on wellbeing and cognitive functioning of older adults. Sci. Rep. 11:4636. doi: 10.1038/s41598-021-84127-7
Diener, E., Emmons, R. A., Larsen, R., and Griffin, S. (1985). The satisfaction with life scale. J. Pers. Assess. 49, 71–75. doi: 10.23943/princeton/9780691188959.003.0002
Diener, E., Suh, E. M., Lucas, R. E., and Smith, H. L. (1999). Subjective well-being: three decades of progress. Psychol. Bull. 125, 276–302. doi: 10.1037/0033-2909.125.2.276
Dodge, R., Daly, A., Huyton, J., and Sanders, L. (2012). The challenge of defining wellbeing. Int. J. Wellbeing 2, 222–235. doi: 10.5502/ijw.v2i3.4
Dooris, M., Farrier, A., and Froggett, L. (2018). Wellbeing: the challenge of ‘operationalising’ an holistic concept within a reductionist public health programme. Perspect. Public Health 138, 93–99. doi: 10.1177/1757913917711204
Dubberly, H., and Pangaro, P. (2007). Cybernetics and service-craft: language for behavior-focused design. Kybernetes 36, 1301–1317. doi: 10.1108/03684920710827319
Dubberly, H., and Pangaro, P. (2019). Cybernetics and design: conversations for action. Des. Res. Found., 85–99. doi: 10.1007/978-3-030-18557-2_4
European Commission, 2019. A definition of artificial intelligence: Main capabilities and scientific disciplines|shaping Europe’s digital future [WWW document]. Available at: https://digital-strategy.ec.europa.eu/en/library/definition-artificial-intelligence-main-capabilities-and-scientific-disciplines [Accessed September 11, 2022].
Fawaz, M., and Samaha, A. (2021). E-learning: depression, anxiety, and stress symptomatology among Lebanese university students during COVID-19 quarantine. Nurs. Forum 56, 52–57. doi: 10.1111/nuf.12521
Fioramonti, L., Coscieme, L., Costanza, R., Kubiszewski, I., Trebeck, K., Wallis, S., et al. (2022). Wellbeing economy: an effective paradigm to mainstream post-growth policies? Ecol. Econ. 192, 107261–107261. doi: 10.1016/j.ecolecon.2021.107261
Fokkinga, S. F., Desmet, P. M. A., and Hekkert, P. (2020). Impact-centered design: Introducing an integrated framework of the psychological and behavioral effects of design. Int. J. Design 14, 97–116.
Frisch, M. B., Cornell, J., Villanueva, M., and Retzlaff, P. J. (1992). Clinical validation of the quality of life inventory. A measure of life satisfaction for use in treatment planning and outcome assessment. Psychol. Assess. 4:92. doi: 10.1037/1040-3590.4.1.92
Genç, E., and Arslan, G. (2021). Optimism and dispositional hope to promote college students’ subjective well-being in the context of the COVID-19 pandemic. J. Posit. Sch. Psychol. 5, 87–96. doi: 10.47602/jpsp.v5i2.255
Gillespie, T. (2014). “The relevance of algorithms” in Media Technologies. eds. T. Gillespie, P. J. Boczkowski, and K. A. Foot (Cambridge, Massachusetts: The MIT Press), 167–194.
Graham, S., Depp, C., Lee, E. E., Nebeker, C., Tu, X., Kim, H.-C., et al. (2019). Artificial intelligence for mental health and mental illnesses: an overview. Curr. Psychiatry Rep. 21:116. doi: 10.1007/s11920-019-1094-0
Gregory, T., Engelhardt, D., Lewkowicz, A., Luddy, S., Guhn, M., Gadermann, A., et al. (2019). Validity of the middle years development instrument for population monitoring of student wellbeing in Australian school children. Child Indic. Res. 12, 873–899. doi: 10.1007/s12187-018-9562-3
Hagey, K., and Horwitz, J. (2021). Facebook tried to make its platform a healthier place. It got angrier instead. Wall Str. J, 1–16.
Hekkert, P., and van Dijk, M., 2011. Vision in design - a guidebook for innovators. BIS Publishers, Amsterdam.
Hu, C., Chen, C., and Dong, X.-P. (2021). Impact of COVID-19 pandemic on patients with neurodegenerative diseases. Front. Aging Neurosci. 13:664965. doi: 10.3389/fnagi.2021.664965
Khan, I., Shah, D., and Shah, S. S. (2021). COVID-19 pandemic and its positive impacts on environment: an updated review. Int. J. Environ. Sci. Technol. 18, 521–530. doi: 10.1007/s13762-020-03021-3
Kjell, O. N. E., and Diener, E., 2020. Abbreviated Three-Item Versions of the Satisfaction with Life Scale and the Harmony in Life Scale Yield as Strong Psychometric Properties as the Original Scales. Journal of Personality Assessment, 0, 1–2. doi: 10.1080/00223891.2020.1737093
Klenk, M., and Duijf, H. (2021). Ethics of digital contact tracing and COVID-19: who is (not) free to go? Ethics Inf. Technol. 23, 69–77. doi: 10.1007/s10676-020-09544-0
Krippendorff, K. (2007). The cybernetics of design and the design of cybernetics. Kybernetes 36, 1381–1392. doi: 10.1108/03684920710827364
Krippendorff, K., 2021. From uncritical design to critical examinations of its systemic consequences. RSD Symp. Available at: https://rsdsymposium.org/professor-dr-klaus-krippendorff/ [Accessed August 14, 2022].
Kross, E., Verduyn, P., Sheppes, G., Costello, C. K., Jonides, J., and Ybarra, O. (2021). Social media and well-being: pitfalls, Progress, and next steps. Trends Cogn. Sci. 25, 55–66. doi: 10.1016/j.tics.2020.10.005
Linton, M. J., Dieppe, P., and Medina-Lara, A. (2016). Review of 99 self-report measures for assessing well-being in adults: exploring dimensions of well-being and developments over time. BMJ Open 6. doi: 10.1136/bmjopen-2015-010641
Löhr, K., Weinhardt, M., and Sieber, S. (2020). The “world Café” as a participatory method for collecting qualitative data. Int J Qual Methods 19:1609406920916976. doi: 10.1177/1609406920916976
Lomas, D., Matzat, U., Pei, L., Rouwenhorst, C., van der Maden, W., Stevens, T., Den Brok, P., and Klaassen, R., (2021). The impact of COVID-19 on university teaching and learning: Evidence for the central importance of student and staff well-being.
Lomas, J. D., Patel, N., and Forlizzi, J. (2021). Designing data-informed intelligent systems to create positive impact. Relat. Syst. Des. Think. Symposium :10.
Lomas, D., and van der Maden, W., (2021). My wellness check designing a student and staff wellbeing feedback loop to inform university policy and governance.
London, A. J. (2019). Artificial intelligence and black-box medical decisions: accuracy versus explainability. Hast. Cent. Rep. 49, 15–21. doi: 10.1002/hast.973
Mackenzie, S. B., Podsakoff, P. M., and Podsakoff, N. P. (2011). Linked references are available on JSTOR for this article: construct measurement and validation procedures in MIS and Behavioral research: integrating new and existing Techniques1. MIS Q. 35, 293–334. doi: 10.2307/23044045
Mccrea, R., Walton, A., and Leonard, R. (2015). Developing a model of community wellbeing and resilience in response to change. Soc. Indic. Res. 129. doi: 10.1007/s11205-015-1099-y
Mizobuchi, H. (2014). Measuring world better life frontier: a composite indicator for OECD better life index. Soc. Indic. Res. 118, 987–1007. doi: 10.1007/s11205-013-0457-x
Norman, D. A., and Stappers, P. J. (2015). DesignX: complex sociotechnical systems. She Ji 1, 83–106. doi: 10.1016/j.sheji.2016.01.002
Pangaro, P., 2017. Cybernetics as Phoenix: Why ashes, what new life, conversations. Cybernetics: State of the Art.
Pangaro, P., 2021. #NewMacy 2021: responding to pandemics of “Today’s AI”. Design+Conversation. Available at: https://pangaro.com/designconversation/2021/08/newmacy-in-2021-pandemics-ai/ [Accessed May 4, 2022].
Phillips, R., and Wong, C. (2017). “Handbook of community well-being research” in International Handbooks of Quality-of-Life (Netherlands, Dordrecht: Springer).
Pavot, W., and Diener, E. (2009). “Review of the satisfaction with life scale. in Assessing well-being. Springer, Dordrecht, 101–117.
Piliavin, J. A., and Siegl, E. (2007). Health benefits of volunteering in the Wisconsin longitudinal study. J. Health Soc. Behav. 48, 450–464. doi: 10.1177/002214650704800408
Pressman, A. (2019). Design thinking: A guide to creative problem solving for everyone, New York: Routledge.
Pretorius, T. (2021). Depression among health care students in the time of COVID-19: the mediating role of resilience in the hopelessness–depression relationship. South Afr. J. Psychol. 51, 269–278. doi: 10.1177/0081246321994452
Radler, B. T., and Ryff, C. D. (2010). Who participates? Accounting for longitudinal retention in the MIDUS National Study of health and well-being. J. Aging Health 22, 307–331. doi: 10.1177/0898264309358617
Rambur, B., Vallett, C., Cohen, J. A., and Tarule, J. M. (2013). Metric-driven harm: an exploration of unintended consequences of performance measurement. Appl. Nurs. Res. 26, 269–272. doi: 10.1016/j.apnr.2013.09.001
Renshaw, T. L., and Bolognino, S. J. (2014). The college student subjective wellbeing questionnaire: a brief, multidimensional measure of undergraduate’ s covitality. J. Happ. Stud.: Interdis. Forum Sub. Well-Being 17, 463–484. doi: 10.1007/s10902-014-9606-4
Renshaw, T.L., Long, A.C.J., and Cook, C.R., 2014. Assessing adolescents’ Positive psychological functioning at school: Development and validation of the student subjective wellbeing questionnaire 29.
Russell, S.J., and Norvig, P., 2022. Artificial intelligence: A modern approach, fourth edition, global Pearson series in artificial intelligence. Pearson, Harlow.
Ryff, C. D., and Keyes, C. L. M. (1995). The structure of psychological well-being revisited. J. Pers. Soc. Psychol. 69, 719–727. doi: 10.1037/0022-3514.69.4.719
Ryff, C. D., and Singer, B. H. (2006). Best news yet on the six-factor model of well-being. Soc. Sci. Res. 35, 1103–1119. doi: 10.1016/j.ssresearch.2006.01.002
Sanches, P., Janson, A., Karpashevich, P., Nadal, C., Qu, C., Daudén Roquet, C., Umair, M., Windlin, C., Doherty, G., Höök, K., and Sas, C., 2019. “HCI and affective health: Taking stock of a decade of studies and charting future research directions.” in Proceedings of the 2019 CHI conference on human factors in computing systems, CHI’19. Association for Computing Machinery: New York, NY, USA. pp. 1–17.
Sas, C., Höök, K., Doherty, G., Sanches, P., Leufkens, T., and Westerink, J. (2020). “Mental Wellbeing: Future Agenda Drawing from Design, HCI and Big Data” in Companion publication of the 2020 ACM designing interactive systems conference (Eindhoven Netherlands: ACM), 425–428.
Seligman, M. E. P. (2011). Flourish: a visionary new understanding of happiness and well-being. Policy 1st Ed. Policy. 14–29.
Shah, R, and Chircu, A. (2018). IOT and ai in healthcare: A systematic literature review. Issues in Information Systems, 19.
Shahriari, K., and Shahriari, M. (2017). IEEE standard review - ethically aligned design: a vision for prioritizing human wellbeing with artificial intelligence and autonomous systems. Int. Humanit. Technol. Conf. 2017, 197–201. doi: 10.1109/IHTC.2017.8058187
Shaukat, K., Iqbal, F., Alam, T. M., Aujla, G. K., Devnath, L., Khan, A. G., et al. (2020). The impact of artificial intelligence and robotics on the future employment opportunities. Trends Comp. Sci. Inform. Tech. 5, 050–054.
Sirgy, M. J. (2018). The psychology of material well-being. Appl. Res. Qual. Life 13, 273–301. doi: 10.1007/s11482-017-9590-z
Springer, K. W., and Hauser, R. M. (2006). An assessment of the construct validity of Ryff’s scales of psychological well-being: method, mode, and measurement effects. Soc. Sci. Res. 35, 1080–1102. doi: 10.1016/j.ssresearch.2005.07.004
Stocké, V., and Langfeldt, B. (2004). Effects of survey experience on respondents’ attitudes towards surveys. Bull. Méthodologie Sociol. 81, 5–32. doi: 10.1177/075910630408100103
Sutton, R.S., and Barto, A.G., (2018). Reinforcement learning, second edition: An Introduction. MIT Press. Well-Being 3, 443–463. doi:doi: 10.1007/s42413-020-00086-3
Sweeting, B. (2016). Design research as a variety of second-order cybernetic practice. Constr. Found. 11, 572–579. doi: 10.1142/9789813226265_0035
Tan, C. (2020). The impact of COVID-19 on student motivation, community of inquiry and learning performance. Asian Educ. Dev. Stud. 10, 308–321. doi: 10.1108/AEDS-05-2020-0084
Tennant, R., Hiller, L., Fishwick, R., Platt, S., Joseph, S., Weich, S., et al. (2007). The Warwick-Dinburgh mental well-being scale (WEMWBS): development and UK validation. Health Qual. Life Outcomes 5, 1–13. doi: 10.1186/1477-7525-5-63
Thomas, R. L., and Uminsky, D. (2020). Reliance on metrics is a fundamental challenge for AI. Ethics Data Sci. Conf.
Topp, C. W., Østergaard, S. D., Søndergaard, S., and Bech, P. (2015). The WHO-5 well-being index: a systematic review of the literature. Psychother. Psychosom. 84, 167–176. doi: 10.1159/000376585
van der Maden, W., Lomas, J. D., and Hekkert, P. (2022). Design for wellbeing during Covid-19: a cybernetic perspective on data feedback loops in complex socIotechnical systems. DRS2022: Bilbao, 25.
Vander Weele, T. J. (2019). Measures of community well-being: a template. Int. J. Community Well-Being 2, 253–275. doi: 10.1007/s42413-019-00036-8
Vander Weele, T. J., Trudel-Fitzgerald, C., Allin, P., Farrelly, C., Fletcher, G., Frederick, D. E., et al. (2020). Current recommendations on the selection of measures for well-being. Prev. Med. 133:106004. doi: 10.1016/j.ypmed.2020.106004
von Foerster, H. (2003). Cybernetics of cybernetics. Underst. Underst., 283–286. doi: 10.1007/0-387-21722-3_13
Wang, F., Kaushal, R., and Khullar, D. (2020). Should health care demand interpretable artificial intelligence or accept “black box” medicine?. Annals of internal medicine 179, 59–60.
White, R. G., and Van Der Boor, C. (2020). Impact of the COVID-19 pandemic and initial period of lockdown on the mental health and well-being of adults in the UK. BJPsych. Open 6:e90. doi: 10.1192/bjo.2020.79
Wiener, N. (1961). Cybernetics or control and communication in the animal and the machine, New York: MIT Press.
Williams, G. M., Pendlebury, H., Thomas, K., and Smith, A. P. (2017). The student well-being process questionnaire (student WPQ). Psychology 08, 1748–1761. doi: 10.4236/psych.2017.811115
Wong, C. S., and Law, K. S. (1999). Multidimensional constructs in structural equation analysis: an illustration using the job perception and job satisfaction constructs. J. Manag. 25, 143–160. doi: 10.1177/014920639902500202
Xiong, J., Lipsitz, O., Nasri, F., Lui, L. M. W., Gill, H., Phan, L., et al. (2020). Impact of COVID-19 pandemic on mental health in the general population: a systematic review. J. Affect. Disord. 277, 55–64. doi: 10.1016/j.jad.2020.08.001
Yang, Q., Steinfeld, A., and Zimmerman, J., 2019. “Unremarkable AI: Fitting intelligent decision support into critical, clinical decision-making processes.” in Proceedings of the 2019 CHI conference on human factors in computing systems. ACM, Glasgow Scotland Uk. pp. 1–11.
Zuckerberg, M., 2018. Untitled. Facebook. https://www.facebook.com/zuck/posts/10104413015393571 (accessed December 1, 2022).
Keywords: human-centered design, community wellbeing, artificial intelligence, cybernetics, wellbeing economy, feedback loop, human values
Citation: van der Maden W, Lomas D and Hekkert P (2023) A framework for designing AI systems that support community wellbeing. Front. Psychol. 13:1011883. doi: 10.3389/fpsyg.2022.1011883
Received: 04 August 2022; Accepted: 23 November 2022;
Published: 04 January 2023.
Edited by:
Simon D'Alfonso, The University of Melbourne, AustraliaReviewed by:
Galena Pisoni, University of Nice Sophia Antipolis, FranceCopyright © 2023 van der Maden, Lomas and Hekkert. This is an open-access article distributed under the terms of the Creative Commons Attribution License (CC BY). The use, distribution or reproduction in other forums is permitted, provided the original author(s) and the copyright owner(s) are credited and that the original publication in this journal is cited, in accordance with accepted academic practice. No use, distribution or reproduction is permitted which does not comply with these terms.
*Correspondence: Willem van der Maden, dy5sLmEudmFuZGVybWFkZW5AdHVkZWxmdC5ubA==
Disclaimer: All claims expressed in this article are solely those of the authors and do not necessarily represent those of their affiliated organizations, or those of the publisher, the editors and the reviewers. Any product that may be evaluated in this article or claim that may be made by its manufacturer is not guaranteed or endorsed by the publisher.
Research integrity at Frontiers
Learn more about the work of our research integrity team to safeguard the quality of each article we publish.