- 1University Research Priority Program ‘Dynamics of Healthy Aging’, University of Zurich, Zurich, Switzerland
- 2Center for Gerontology, University of Zurich, Zurich, Switzerland
- 3Department of Psychology, University of Zurich, Zurich, Switzerland
- 4Institute of Geomatics, University of Applied Sciences and Arts Northwestern Switzerland, Muttenz, Switzerland
- 5Department of Geography, University of Zurich, Zurich, Switzerland
Prominent theories of aging emphasize the importance of resource allocation processes as a means to maintain functional ability, well-being and quality of life. Little is known about which activities and what activity patterns actually characterize the daily lives of healthy older adults in key domains of functioning, including the spatial, physical, social, and cognitive domains. This study aims to gain a comprehensive understanding of daily activities of community-dwelling older adults over an extended period of time and across a diverse range of activity domains, and to examine associations between daily activities, health and well-being at the within- and between-person levels. It also aims to examine contextual correlates of the relations between daily activities, health, and well-being. At its core, this ambulatory assessment (AA) study with a sample of 150 community-dwelling older adults aged 65 to 91 years measured spatial, physical, social, and cognitive activities across 30 days using a custom-built mobile sensor (“uTrail”), including GPS, accelerometer, and audio recording. In addition, during the first 15 days, self-reports of daily activities, psychological correlates, contexts, and cognitive performance in an ambulatory working memory task were assessed 7 times per day using smartphones. Surrounding the ambulatory assessment period, participants completed an initial baseline assessment including a telephone survey, web-based questionnaires, and a laboratory-based cognitive and physical testing session. They also participated in an intermediate laboratory session in the laboratory at half-time of the 30-day ambulatory assessment period, and finally returned to the laboratory for a posttest assessment. In sum, this is the first study which combines multi-domain activity sensing and self-report ambulatory assessment methods to observe daily life activities as indicators of functional ability in healthy older adults unfolding over an extended period (i.e., 1 month). It offers a unique opportunity to describe and understand the diverse individual real-life functional ability profiles characterizing later life.
1. Introduction
Daily activities have been proposed to play a pivotal role in health and well-being in numerous aging theories (Martin et al., 2015; Michel and Sadana, 2017). For example, the activity theory by Havighurst (1963) proposes that a key condition for older adults to obtain satisfaction and happiness is to maintain the activities of middle age as long as possible and to find substitutes for those activities they have to give up. Rowe and Kahn (1997) emphasized that successful aging is not only about the absence of disease or the maintenance of functional physical and cognitive capacities, but also about active engagement in social and productive activities. In promoting healthy and active aging, the World Health Organization (WHO) has also placed high emphasis on daily activities. Specifically, active aging is defined as “the process of optimizing opportunities for health, participation, and security for the enhancement of quality of life” (World Health Organization, 2002, p. 12). Moreover, the recent healthy aging model (World Health Organization, 2020) emphasizes the enablement of older adults to be mobile, have relationships, and contribute to society as a reflection of functional ability and, in turn, of healthy aging at many levels of functioning.
Studies that invited older adults to report their time use during the preceding day (i.e., “yesterday interview,” day reconstruction method) have shown that a typical day of many older adults consists of (1) self-maintenance activities [measured by Activities of Daily Living (ADLs), and Instrumental Activities of Daily Living (IADLs)], (2) resting (e.g., sleep), and (3) various physical, social, cognitive, and spatial activities (Horgas et al., 1998; Möwisch et al., 2022). This third type of activities has also been referred to as leisure activities or lifestyle activities, reflecting the idea that older adults make agentic choices in their leisure time, which, eventually, form their lifestyle (Jopp and Hertzog, 2010; Bielak, 2017). Lifespan developmental theory explicitly conceptualizes individuals as producers of their own development, flexibly adapting to biological and environmental opportunities and constraints (Baltes et al., 1998). In turn, examining how older adults allocate their time and energy to different activities, particularly to lifestyle activities, helps to understand how individuals “produce” their own developmental pathways and achieve the maintenance of their individual health and well-being in older age.
To embrace the advancement of mobile technology and to comprehensively examine daily activities, this study aims to obtain a comprehensive description and understanding of daily activities of community-dwelling older adults in relation to a wide range of within-person (i.e., intraindividual) and between-person (i.e., interindividual) differences in health and well-being by combining different ambulatory assessment methods (i.e., passive activity sensing that requires no input from the person and experience-sampling that involves self-reports). More specifically, this study developed a single custom-built sensor (i.e., the “uTrail”) that includes a Global Positioning System (GPS), an accelerometer, and audio recording for high-density continuous and simultaneous measurements throughout a full day without the need to recharge. The uTrail was used to observe 150 older adults over 30 days in 2018. In the first 2 weeks, a smartphone was used in addition to the uTrail to concurrently collect a wide range of data seven times per day, including self-reported daily activities, mood, stress, and context information as well as working memory performance. The combination of different sensors and self-reports provides a rich collection of both objective and subjective momentary information on diverse activities and their contextual correlates. In sum, this is the first study which combines multi-domain activity sensing and experience-sampling to observe healthy older adults over an extended daily life period (i.e., up to 1 month), providing a unique opportunity to better understand daily activities and their relation to health and well-being as indicators of functional ability in healthy aging.
1.1. Capturing daily activities, health and well-being in older age through the lens of ambulatory assessment
Decades of research have shown that more active engagement in daily activities is closely associated with older adults’ health and well-being (Hertzog et al., 2008; Adams et al., 2011). In recent years, ambulatory assessment methods have become more popular in assessing daily activities in older adults in psychological research (Brose and Ebner-Priemer, 2015; Conner and Mehl, 2015). Specifically, ambulatory self-report is the method with which individuals repeatedly provide responses to queries on their current or very recent behaviors and experiences (Conner and Mehl, 2015). Ambulatory self-report has been used to examine a wide range of psychological constructs in diverse age groups, including daily activities, functional health, and well-being. For example, activities (e.g., TV watching, social, physical activities) that were reported multiple times per day over days are associated with interindividual differences in baseline self-reported pain and physical health (Fingerman et al., 2021). At the intraindividual level, self-reported activities are also associated with concurrent emotions and cognitive performance (Pauly et al., 2017; Campbell et al., 2020). The ambulatory self-report method, prompting participants to report activities momentarily or shortly beforehand, has the advantage of minimizing retrospective recall bias on daily activities (Hatt et al., 2021). It also enables examination of interindividual differences in activity engagement as well as of intraindividual variations in daily activities over time and contexts (Hamaker, 2012). Most studies with ambulatory self-report have lasted over only 1 or 2 weeks. Although this may have reduced participant burden, it was probably insufficient to capture an extended view of the typical daily routine of an older adult and their agentic role in “producing” their own life.
Passive sensing technology (a different ambulatory assessment method), sharing the advantages of ambulatory self-report in minimizing retrospective recall bias and enabling analyses on inter- and intra-individual differences, overcomes the disadvantage of self-report by objectively and unobtrusively monitoring daily activities (Harari et al., 2017; Macdonald et al., in press). Audio recordings, for instance, have been used to observe spoken conversations, reflecting social and cognitive activities in daily life (Demiray et al., 2020). Time spent in conversations was associated with inter- and intra-individual differences in well-being across adulthood (Milek et al., 2018; Sun et al., 2019). Similarly, physical activities assessed by accelerometers have been shown to be associated with well-being and cognitive abilities on the inter- (Dillon et al., 2018; Burzynska et al., 2020) and intra-individual levels (Maher and Conroy, 2017) in samples of young, middle-aged, and older adults. Using a GPS tracking kit to assess older adults’ spatial activities, studies have examined time out-of-home in relation to inter- and intraindividual differences in well-being and physical and cognitive health (Kaspar et al., 2015; Petersen et al., 2015; Kamalyan et al., 2021).
Daily activities in aging research have been quantified often, if not primarily, by frequency and type (Bielak et al., 2019). Passive sensing technology offers a chance to largely enrich dimensions of daily activities in research, which would otherwise only be accessible through detailed interviews with participants. For example, audio recordings offer rich information on the nature of daily conversations (Mehl and Pennebaker, 2003). Specifically, conversation content, relating to reminiscence or future- and past-time orientation, have been shown to differ by age between young and older adults (Demiray et al., 2018; Haas et al., 2022) and by interindividual differences in well-being (Brianza and Demiray, 2019; Demiray et al., 2019). Language features extracted from audio recordings, such as use of complex language, have also been shown to be associated with interindividual differences in chronological age (Luo et al., 2019, 2020), executive functioning (Polsinelli et al., 2020), and working memory (Ferrario et al., 2022). Indicators of physical activities could be diverse, including volume (e.g., time spent on walking, step count), patterns (e.g., length of walking bouts), and variability of bout lengths, which were shown to relate to older adults’ cognitive health (Mc Ardle et al., 2020). Similarly, mobility has also been shown to be a multidimensional concept (much more complex than “time out-of-home”), whose relations with health and well-being require much more research to investigate (Wettstein et al., 2014; Fillekes et al., 2019a).
Furthermore, passive sensing technology offers rich data streams with which activity patterns involving temporal structures could be discovered. First, studies with ambulatory self-report methods assessing activities multiple times per day have shown that moments of activity engagement are associated with not only concurrent, but also subsequent emotions, fatigue, and cognitive performance with delayed time lags (Paolillo et al., 2018; Zhaoyang et al., 2021). With more closely-spaced sampling, studies using passive sensing technology have great potential to uncover time-lagged associations between activities and psychological outcomes. Second, closely-spaced data from sensing technology also enable research to uncover novel activity patterns in time series, such as sequential complexity (Paraschiv-Ionescu et al., 2012), temporal regularity (D’Mello and Gruber, 2021), and time-lagged patterns and trajectories (Kuppens and Verduyn, 2017). Additionally, large volumes of daily activities data from sensing technology enable big data analytics, such as using machine learning methods in predicting older adults’ health and well-being (Ferrario et al., 2020, 2022).
Thus far, most studies have used one type of technology to study a specific type of activity. Some have combined ambulatory self-report and one sensing technology (Sun et al., 2020; Haas et al., 2022). Very few of them have combined different sensing technologies together with ambulatory self-report to obtain contextualized multi-domain assessments. Nevertheless, in spite of its advantages, activities captured by sensing technology could sometimes require extra works on validating and analyzing, to establish their links with subjective psychological experience (Harari et al., 2017; Cornet and Holden, 2018). Thus, combining ambulatory self-report and different passive sensing technologies to simultaneously capture different types of activities and observe how they unfold and interact with each other in daily life for a longer period can extract more comprehensive information of daily activities and their relations to within- and between-person health variation.
1.2. Adding a layer: Contextual correlates of daily activities
One of the salient advantages of ambulatory assessment methods over single-occasion retrospective self-report measurements is the possibility to examine contextual factors that co-occur with an activity (Shiffman et al., 2008; Brose and Ebner-Priemer, 2015). Social and physical contexts have been conceptualized as environmental opportunities or barriers for activities participation, which subsequently influence health and well-being (Stine-Morrow et al., 2014; Wahl and Gerstorf, 2018). Ambulatory assessment methods, particularly sensing technology, capture large amounts of information on contextual situations that could be examined in relation to psychological variables (Harari et al., 2020; Lazarević et al., 2020).
Thus far, only a few ambulatory assessment studies have examined effects of contextual factors on associations between daily activities and health and well-being. For example, findings from a 14-day study with GPS and accelerometer assessment in older adults showed that, at the between-person level, time spent outdoors was significantly associated with steps-per-day, which were associated with baseline cardiorespiratory fitness, lower-extremity strength, and well-being (Harada et al., 2017). The authors proposed that their findings suggest outdoor contextual environments influence physical and psychological functioning through stimulating physical activities. Fingerman et al. (2021) showed that older adults who reported more baseline pain and lower physical functioning had a higher likelihood of visiting doctors, but this association was only significant when social encounters were present at the assessment points. Thus, the context of who is present in the moment would influence associations between activities and physical health. Luo et al. (2019, 2020) showed that, within persons, older adults produced equally complex grammatical structures when talking with the significant other as younger adults, but simpler grammar than younger adults when talking with strangers. Their findings suggest that being in familiar contexts, such as with emotionally close others, enables (and possibly motivates) older adults to produce more complex language. In sum, the above findings highlight the importance of contextual correlates on daily activities in various domains, including physical, cognitive, and social activities. Further examination of contextual effects on associations between daily activities and health and well-being in older age is desired to describe and understand the diversity of daily life pathways to functional health during adulthood and aging. With both self-report and objectively measured contexts of daily activities combined, understanding of daily activities would become much more comprehensive.
1.3. The current study
The Mobility, Activity, and Social Interactions Study (MOASIS) is an interdisciplinary study at the University of Zurich’s (UZH) Research Priority Program (URPP) “Dynamics of Healthy Aging” that aims to understand daily activity profiles of healthy older adults and examine intra- and interindividual associations between these profiles, health, and well-being. As such, it also aims to examine contextual determinants of associations between daily activities and health and well-being in older age.
We expect to observe diverse activity profiles in different healthy older adults and will examine associations between daily activities, health, and well-being. We will also examine effects of contextual factors on the associations between daily activities, health, and well-being at both the within-person and between-person levels. Furthermore, with the high-density sensor-based spatial and physical activity data, we expect to develop innovative measures and apply advanced statistical models to understand patterns of daily activities of healthy older adults. Figure 1, for instance, displays a visualization of one potential outcome of this study, in which a comprehensive representation of contextualized activities across multiple activity domains is linked over temporal and spatial contexts as one basis for the identification of the kinds of typical real-life activities of different individuals that unfold and how they can then be linked with different health and well-being parameters.
2. Methods and analysis
2.1. Recruitment and sample
Recruitment was carried out through the survey center of the URPP Dynamics of Healthy Aging using the internal participant database. Additional means of recruitment involved snowballing among registered participants as well as advertisements in local newspapers. Inclusion criteria for the study involved sufficient eyesight to operate the smartphone and uTrail, computer and internet access at home (assessed via self-report), absence of cognitive impairment as tested with a cognitive screening (Mini Mental State Examination Score ≥ 27), and being 65 years or older.
Eligible participants were contacted via phone or email and informed about the general goals and procedure of the study. Upon agreement, a package was sent to the participants containing an information brochure detailing the study background, assessment procedure and time requirements from the participants and compensation, as well as the informed consent form and a pre-stamped return envelope. Upon receipt of the informed consent, a technical research assistant called each participant to schedule all required study components and lab visits. A total of 161 participants enrolled in the study and 150 participants (aged 65–91 years) met all the inclusion criteria. Table 1 provides information on sociodemographic sample characteristics.
2.2. Procedure
As shown in Figure 2, the MOASIS design involved (I) a 3-part baseline assessment consisting of a telephone interview, a web-based questionnaire and a lab visit, (II) a 30-day ambulatory assessment and experience sampling phase, an intermediate laboratory session 2 weeks into this ambulatory testing period used for a brief recap and mobile device return and exchange, and (III) a posttest lab session at the very end of the full 30-day ambulatory assessment phase. This core assessment design will be repeated longitudinally after 5 years.
Part I: Baseline Assessment. The (a) telephone interview consisted of questionnaires primarily assessing mobility and leisure activities as well as depressive symptoms. These questionnaires, in part, proved during pilot testing to require more detailed instructions than deemed feasible in a web-based assessment. They were assessed over the phone by trained research assistants who directly entered responses into a websurvey platform. The telephone interview was scheduled to last about 45 min. Following the telephone interview, participants received a link to (b) an online survey implemented using SoSci Survey (Leiner, 2019) to be completed at home within 1 week. This survey included a wide range of questionnaires assessing demographic information, living circumstances in the home and the neighborhood important for mobility and physical, social, and cognitive activity, as well as trait ratings of constructs representing key facets of quality of life and psychological adaptation (see Table 2). We included three single items as attention controls throughout this long questionnaire to identify participants who may have just clicked through the items (e.g., “When reading this sentence, please check 2 on the rating scale.,” “Which of the following items resembles an apple most closely?”). One week after the telephone interview, participants attended a (c) lab-based introductory session at UZH during which they completed a psychometric cognitive test battery of a wide range of crystallized and fluid cognitive abilities as well as a physical performance test. Participants were also familiarized with the uTrail and the smartphone questionnaire and cognitive testing.
Part IIa: Ambulatory Assessment Phase. During this 30-day phase of daily real-life activity sensing and experience-sampling, participants wore the uTrail (Figure 3; Table 3) on their hip throughout their waking hours. They were asked to charge the device each night and there was a privacy button they could push when wanting to prevent audio recordings for the next 15 min. A red LED light indicated to participants when muting was in progress. Otherwise, participants could not and did not have to interact with the device. Activity data measured with the uTrail were stored directly on two SD cards on the device and downloaded manually at the end of Week 2 (i.e., during the intermediate session) and Week 4 (i.e., at posttest), respectively. The decision for local storage on the uTrail and manual download and against remote access and download was made for privacy reasons.
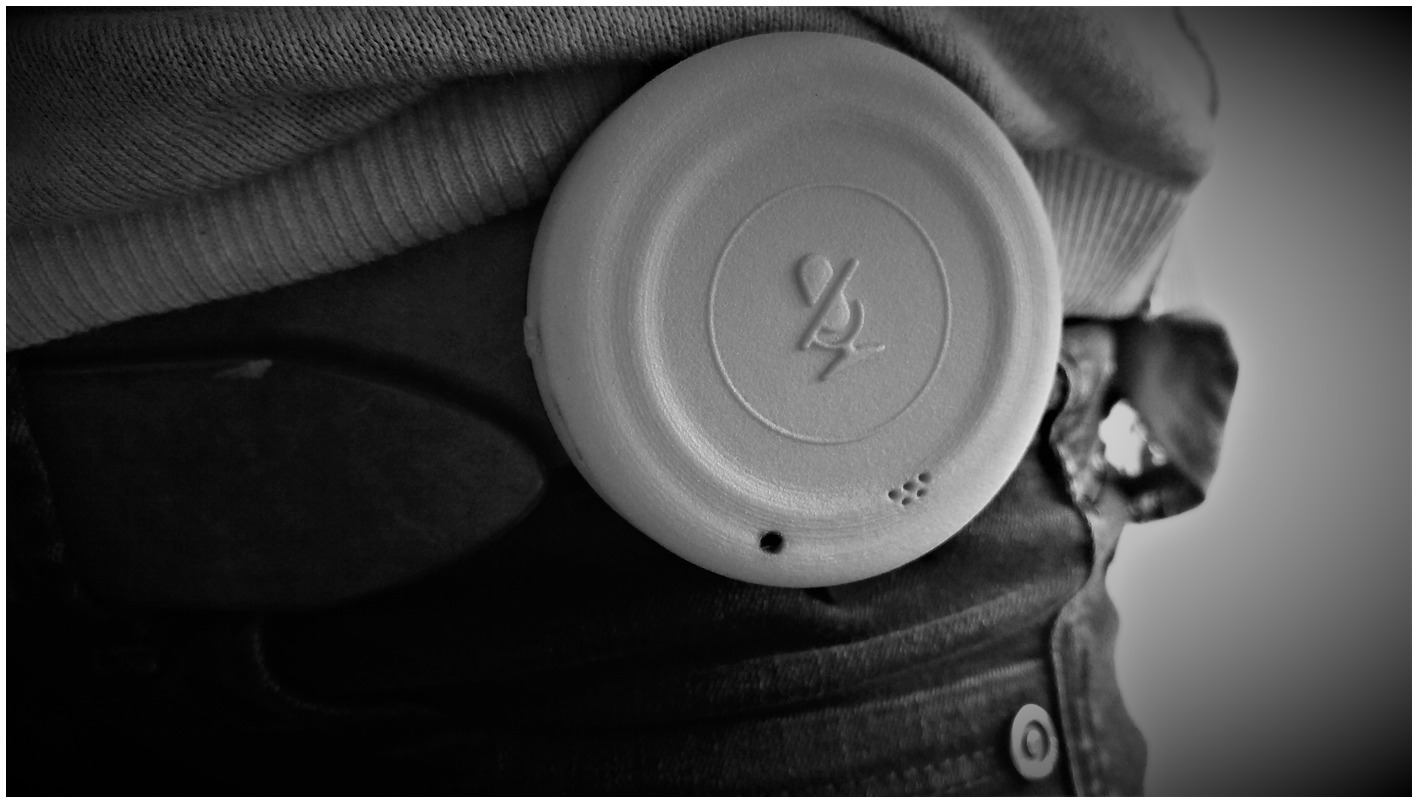
Figure 3. uTrail (diameter = 5.5 cm, depth = 2 cm, weight < 100 g). The uTrail has a clip to fix it on the waist (either at a belt or waistband). Photo taken by authors.
For the first 15 consecutive days of the ambulatory assessment phase (i.e., between baseline and intermediate session), participants also carried a smartphone with them and were prompted 7 times per day every 120 min with a random interval of plus or minus 0–15 min (e.g., roughly around 8:30, 10:35, 12:40, 14:45, 16:50, 18:55, and 21:00 h) to initiate a short experience-sampling and cognition test on the phone. During pilot testing, we found that this covered the waking time that our older individuals tended to report. At each prompt, participants responded to a short survey outlined in more detail below and in the end completed 2 trials of varying difficulty level of a working memory task, all of which on the smartphones provided for the study (see Figure 4 for an overview).
Participants’ compliance with the smartphone assessments was monitored online as data were transmitted to the server of the experience-sampling software used (MovisensXS). In case no experience-sampling and ambulatory cognition data collected on the smartphones were uploaded to the server, a student assistant checked in with a given participant via phone. Depending on the visible compliance with respect to the smartphone-based assessments, potential problems regarding both mobile devices were addressed and encouragement and appreciation for their participation was expressed.
Part IIb: Intermediate Session. As the uTrail can store activity data from its three sensors for up to 2 weeks, participants were invited back to the laboratory 2 weeks after the baseline lab session for data download and to return the study smartphones. Because data download and reinitialization of a uTrail device could take up to 4 h, we exchanged uTrail devices used in weeks 1–2 for a new device for each participant for the remaining second half of the ambulatory assessment period. Since the uTrails use standard commercial components that have been calibrated before deployment, inter-device variation in data accuracy is minimal, and hence device swapping is expected to have no effect on data quality. Participants also responded to a brief questionnaire on the computer regarding retrospective ratings of affect, mobility, physical activity, and mind wandering during the 2 weeks prior. Participants were also asked for a brief oral assessment of their experiences so far and then dismissed for the second half of the study.
Part III: Posttest Session. Thirty days after the laboratory baseline session, participants came back to the lab for a final session to return their uTrail and to complete web-based questionnaires assessing a selection of the person-level traits already measured at baseline as well as overall experience and feedback questions regarding the uTrail, the smartphone assessment, and the study in general. They also completed a slightly shortened cognitive test battery compared to the one administered at baseline. Participants could indicate whether they wanted to listen to any of their audio snippets and indicate possible audio files they wished to delete. They were then thanked and reimbursed with 150 CHF (equivalent to app. 160 $) for the entire study participation if they met compliance criteria for the ambulatory assessment phase (i.e., 80% or more of the smartphone surveys completed).
2.3. Measures
A battery of measures reflecting trait-like physical activities and spatial mobility, health, well-being, as well as contextual factors were collected in the baseline and posttest sessions to obtain (a) information on interindividual differences in those activity variables intensively assessed in daily life, (b) information on interindividual differences in various domains of psychological health to be investigated as antecedents, correlates, and consequences of activities carried out in daily life (emotional, cognitive, physical), and (c) information on individual environmental and other context characteristics likely to shape daily activity routines. Table 2 provides an overview of all measures and the time point or period throughout the study when they were collected.
2.3.1. Baseline and posttest: Self-report measures
Sociodemographics. Participants provided information on conventional sociodemographic measures such as sex, age, years of education and type of occupation, marital status, presence and number of children, and income.
Physical health. Physical health was assessed using the Short-Form Health Survey (SF-12; Lawton and Brody, 1969; Ware et al., 1996). The survey contains questions about emotional and physical health and was slightly extended for use in the current study to include additional questions about possible head injury during the preceding 2 years, number of illnesses using a list of 23 possible diagnoses (e.g., cancer, hypertension), changes in vision and hearing as well as limitations in activities of daily living. In addition, we collected information on body mass index, consumption of alcohol, and usage of any walking aid.
Living circumstances and mobility. Participants indicated their living situation in more general terms (e.g., living in an urban or rural area, in an apartment or house, on which floor they live) and also regarding more specific aspects that we deemed important for our focus on physical, spatial, and social activities (e.g., whether they own a car, how many neighbors they are in contact with regularly, which means of transport they typically use). Participants reported on their typical radius of mobility using the Life Space Questionnaire (LSQ; Stalvey et al., 1999), and also indicated their five favorite locations, what they typically do at these locations and how often they visit them (self-developed based on Iwarsson et al., 2004; Mollenkopf et al., 2004) in addition to mobility preferences. We used the Neighborhood Environment Walkablity Scale (NEWS; Saelens et al., 2003; Bödeker and Bucksch, 2011) to measure individuals’ neighborhood perception (e.g., types of residences, stores and facilities, access to services, streets, places for walking and cycling, surroundings, safety from traffic, safety from crime, satisfaction with neighborhood).
Physical and leisure activities. We assessed typical physical activity using the short form of the International Physical Activity Questionnaire (IPAQ; Craig et al., 2003). To account for the fact that some activities, such as gardening, hiking or skiing, are more typical in some seasons than in others, these questions were asked for the warm season and the cold season separately. To obtain a broader view on individuals’ common leisure activities beyond the physical domain, participants were also asked about their typical weekly activities and routines across a number of categories (i.e., crafting, games, cultural activities, social activities, TV activities, health care and acquisition of knowledge) using an adapted version of the Leisure Activity Questionnaire by Jopp and Hertzog (2010). Participants indicated how often and at which type of location they engaged in each of these activities over the past 12 months.
Subjective well-being, stress, and emotion regulation. We assessed several components of subjective well-being or lack thereof, including depressive symptoms (using the German Version of the CES-D scale, the General Depression Scale [ADS]; Riediger et al., 1998), life satisfaction (Satisfaction with Life Scale [SWLS]; E. D. Diener et al., 1985), and affect. We used a large pool of items to assess high and low arousal positive and negative affect, based on the Positive and Negative Affect Schedule (PANAS; Watson et al., 1988: upset, proud, hostile, irritable, expectant, afraid, attentive, active, strong, distressed, inspired, nervous, ashamed, guilty, interested, enthusiastic, scared, determined, alert), the Multidimensional Affect Questionnaire (MDBF; Steyer et al., 1997: content, rested, restless, bad, worn-out, composed, tired, great, uneasy, energetic, uncomfortable, relaxed, highly activated), and 7 additional items to match the full range of the daily life affect experience sampling items (without energy, balanced, sad, annoyed, angry, happy, concerned). Psychological well-being was assessed using the Ryff-Scales (Ryff, 1989). Participants’ trait emotion regulation was measured using the Emotion Regulation Questionnaire (ERQ; Gross and John, 2003; Abler and Kessler, 2009). We further measured psychological need satisfaction (Balanced Measure of Psychological Needs Scale; Sheldon and Hilpert, 2012), and obtained behavioral measures of emotional (facial) expressions to emotional film clips using software and film clip material from Carvalho et al. (2012).
Self and personality. We assessed several interindividual difference characteristics from the self and personality domains, including Big Five personality traits (Big Five Inventory-2; Danner et al., 2016), self-efficacy (General Self-Efficacy Scale; Schwarzer and Jerusalem, 1999), control beliefs (Midlife Development Inventory; Lachman and Weaver, 1998), and mindfulness (Mindful Attention and Awareness Scale; Michalak et al., 2008). Furthermore, we assessed participants’ time perspective using the Future Time Perspective Scale (Carstensen and Lang, 1996) and the Modified Balanced Time Perspective Scale (mBTPS; Vowinckel et al., 2017). In addition, participants rated their sense of purpose in life (German Purpose Scale; Scheier et al., 2006), self-esteem (Rosenberg Self-Esteem Scale; Rosenberg, 1965; Von Collani and Herzberg, 2003), and several aspects of subjective age (i.e., felt age, looked age, wanted age).
Social relations. We assessed participants’ contacts with family members and non-family members (Brunstein, 1999), satisfaction with family and friend relationships (Lindenberger et al., 2010), as well as perceived loneliness (UCLA Loneliness Scale; Russell et al., 1980). Relationship closeness was assessed with the Relationship Closeness Inventory (RCI; Berscheid et al., 1989), and with the Inclusion of Other in Self (IOS) Scale (Aron et al., 1992). Various aspects of social support (e.g., perceived social support, support in autonomy, received support, provided support, companionship, social distress, and health related social control) were assessed using the Berlin Social Support Scales (BSSS; Schwarzer and Schulz, 2003), and adaptations from other measures (Kasser and Ryan, 1999; Williams et al., 1999; Butterfield and Lewis, 2002; Cyranowski et al., 2013).
Metacognition. Participants’ metacognition was measured using the Metamemory in Adulthood Questionnaire (MIA; Dixon et al., 1988), the Cognitive Failures Questionnaire (Klumb, 1995), as well as the Thinking about Life Experience (TALE) Questionnaire (Bluck et al., 2005). Mind wandering was measured using two different scales (Carriere et al., 2008; Mrazek et al., 2011), complemented by the Daydreaming Frequency Scale (Giambra, 1989).
Technology use, experience and attitude. Given the use of mobile technological devices for activity tracking and experience sampling, we also assessed several aspects regarding technology. On a list of 12 items (e.g., TV, phone, computer, e-reader, smartphone), participants indicated which of these technological devices they owned and how often they used these, in particular computers, smartphones, and in addition the internet. Participants also answered more specific questions about their experience with, and attitude towards technology with a set of items adapted from Mollenkopf et al. (2000). Only at posttest, we further obtained feedback on participants’ experiences and perception of the technical quality and the handling of the uTrail sensor used in this study with a set of self-developed items. Examples of items are “During the past 4 weeks to what extent have you been aware of the device in your everyday life?,” “During the past 4 weeks to what extent was the use of the device easy, intuitive?” or “During the past 4 weeks to what extent did the device influence the behavior of the people in your environment?.” Participants also responded to questions about the extent to which the uTrail and the smartphone influenced their awareness of everyday life and their environment, and the extent to which they had carried the uTrail on themselves in daily life and reasons for why not.
2.3.2. Baseline and posttest: Cognitive ability and physical functioning tests
Cognitive abilities were assessed with lab-based performance tasks covering several crystallized and fluid intelligence markers. These included verbal knowledge [Mehrfachwahl-Wortschatz-Intelligenztest (MWT-B); Lehrl, 2005; spelling test from the Leistungsprüfsystem (LPS 1 & 2), Sturm et al., 1993], phonemic fluency [Leistungsprüfsystem (LPS6); Sturm et al., 1993], and semantic fluency [Regenburger Wortflüssigkeitstest (RWT); Aschenbrenner et al., 2000]. Further, we obtained interindividual differences in processing speed using four different tests [speed of perception test from Leistungsprüfsystem (LPS14); Sturm et al., 1993; digit-symbol-test from the HAWIE; von Aster and Neubauer, 2009; identical pictures test (IPS); French et al., 1963; and the trail making test (TMT), Reitan, 1992]. Working memory was assessed by the repeated numbers task forward and backwards (HAWIE; von Aster and Neubauer, 2009), a computerized 2-back task using the Test of Attentional Performance (TAP) software (Zimmermann and Fimm, 2002), and a numerical memory updating task executed on the study smartphones (Riediger et al., 2011) that is described in greater detail in the section on the ambulatory assessment of cognitive performance. Episodic memory was measured by the Verbal Learning and Memory Test (VLMT; Helmstaedter et al., 2001, a German version of Rey Auditory Verbal Learning Test (RAVLT) Rey, 1964). Spatial memory was measured by a computerized object-location task similar to the card game “Memory” (Rasch et al., 2007). Executive functioning was measured by using the difference score between version A and B of the TMT (Reitan, 1992). In addition to cognitive performance, we also assessed physical functioning with the Short Physical Performance Battery (SPPB; Guralnik et al., 1994), including performance tests for balance, walking speed, and leg strength, to obtain a baseline interindividual difference measure in these domains given our interest in capturing daily life mobility and physical activity.
2.3.3. Ambulatory assessment: Passive real-life activity sensing
The uTrail used in this study for the passive sensing of spatial, physical, and social activities is a custom-built tracking device. It was developed with the aim to (a) collect multi-domain activity data in a single device, (b) provide data density in each of the three sensor units appropriate for in-depth analysis in each activity domain, (c) be able to store all that data over a period of 2 weeks, (d) provide sufficient battery capacity for at least one full day of high-density sampling, and (e) be user friendly also for an older adult population with diverse technological experiences.
As outlined in Table 3, the uTrail features the following three sensor units: a GNSS (Global Navigation Satellite System) unit (colloquially called GPS); an IMU (Inertial Measurement Unit) consisting of an accelerometer and a magnetometer; and a MEMS (microelectro-mechanical systems) microphone. All sensor units are commercial off-the-shelf components. The sampling rates were selected to reflect those commonly used for the different sensors to derive as meaningful and accurate information as possible in each activity domain. The timestamp that is recorded in all three datasets allows to cross-link information from the different sensor units. Besides timestamp, the GPS data features the position (longitude, latitude), different accuracy values (i.e., number of available satellites, horizontal and vertical dilution of precision [VDOP, HDOP, respectively]), and Doppler-based instant speed. The uTrail device stores data internally in a binary format to make best use of the available storage capacity. When the data is downloaded it is extracted and saved automatically as a Comma Separated Values (csv) text file.
The GPS recordings can be used to obtain information about an individual’s mobility patterns and multiplace personal exposures (Dodge et al., 2009; Siła-Nowicka et al., 2016; Chaix, 2018). Over 20 corresponding indicators of daily spatial mobility have been developed and coded in the R statistics system in a preceding project using data recorded with smartphones but with the same sampling and accuracy specifications as in MOASIS (Fillekes et al., 2019b).
The IMU measures acceleration and the magnetic field intensity along three dimensions to capture information on physical activity intensity and activity types. In a related project, indicators of physical activity including physical activity intensity and levels, the major posture and transport-related motion activity types (sitting, standing, lying, walking, non-level walking, running and cycling), as well as step counts (Pham et al., 2018) have been developed and implemented in R, as documented in Allahbakhshi et al. (2020).
The audio data assessments were modeled after the Electronically Activated Recorder (EAR; Mehl et al., 2001) and consist of 50-s ambient sound snippets sampled every 18 min that contain human speech recordings of the participant as a sensor-based indicator of social interactions in daily life. Such data can then be analyzed regarding social behavior, using coding or transcription and automatic language processing approaches (e.g., Yordanova et al., 2019; Ferrario et al., 2020, 2022). Based on linguistic characteristics of the speech utterances, inferences on cognitive activity are also possible (e.g., Luo et al., 2019, 2020). As ground truth data for speaker identification, we obtained noise-free speech samples from each participant during the posttest session by asking participants to read a prepared brief statement out aloud which we recorded.
2.3.4. Ambulatory assessment: Experience sampling
The experience sampling was carried out on study smartphones (Motorola moto G4, Android 6.0) provided to participants and aimed to capture self-report information to complement the passive sensing carried out with the uTrail. The seven momentary surveys presented to participants each day during the experience sampling phase each contained a set of core items that were identical across prompts. In addition, some questions were only relevant in the morning (e.g., sleep quality during the preceding night, outlook on the day) or evening (e.g., review of daily stress experienced that day, subjective health). In the following, we provide an overview of the experience sampling variables available, and unless particularly mentioned, these were collected seven times per day. Figure 4 provides an overview of the diurnal flow of questions.
Sleep. In the first momentary survey in the morning, participants rated their daily sleep quality, the time they went to bed the night before and got up that morning, and how many hours they had slept, responding to single items each.
Situational evaluation. Situations can be evaluated along several dimensions to assess their psychological meaning (Rauthmann and Sherman, 2016). In an effort to include such contextual information into the real-life assessments, in addition to the related information collected using the GPS, IMU and audio data, each momentary survey included measurement of stress and uplifts, positive and negative affect, the geographical and social context, as well as the activity pursued including its intellectual and physical load. In the following paragraphs, the specific variables and their measurement are described in more detail.
Stress and uplifts. In the morning survey, participants were asked about anticipated stress and anticipated positive events for that same day (Scott et al., 2013). At each prompt, participants also indicated whether, since they got up in the morning or since the last prompt, they experienced something unpleasant or something pleasant (Almeida et al., 2002). For each positive response, they also indicated when during the roughly past two-hour time window this event took place or whether it was still ongoing (Wrzus et al., 2015).
A more detailed assessment of daily stress occurrence and severity was obtained at each daily evening prompt with an adapted 7-item version of the Daily Inventory of Stressful Events, including an extra item on health-related stressors (DISE; Almeida et al., 2002). Similar to the stressors, daily uplifts were assessed with five items regarding both occurrence and intensity, that directly mimicked the DISE questions, focusing on uplifts instead.
Subjective well-being. Emotional well-being in terms of momentary positive and negative affect intensity was measured at each of the seven momentary prompts using a select set of items also included in the baseline assessment, that were drawn from the PANAS-X (Watson et al., 1988; Grühn et al., 2010) and the momentary version of the Multidimensional Mood Questionnaire (Wilhelm and Schoebi, 2007) to cover both low and high arousal items from the emotional circumplex and those that have been shown to be sensitive to momentary fluctuations (Brose et al., 2020; content, unwell, restless, awake, without energy, nervous, relaxed, worried, happy, angry, sad, balanced, furious). In the evening, an additional single item measured daily life satisfaction, and personal need satisfaction was assessed with three items based on the balanced measure of psychological needs (BMPN; Sheldon and Hilpert, 2012) and the General Causality Orientation Scale (Deci and Ryan, 1985). The items asked about the connection to other people, feeling competent and whether one’s own actions were based on one’s own interests and values.
Pain and subjective health. Occurrence of pain was assessed both at each momentary assessment and reflecting back on the day each evening with single items. In addition, a single item asking about daily subjective health was included in the evening survey.
Emotion regulation. Emotion regulation was measured at every prompt by asking participants to report to which extent they had applied each of six emotion regulation strategies since waking up or since the last momentary assessment (Brans et al., 2013).
Social and environmental context. At each prompt, participants specified whether they were with another person or not. If others were present, the type of relation was assessed (e.g., partner, family, stranger). Participants were also asked in each momentary survey, which type of location they were in (e.g., at home, indoors, outdoors, at a private or a public place), and how often they visited that place.
Mobility and physical activity. Being mobile (vs. not) and type of transport mode was measured by asking participants at each prompt whether they were currently in transit or not and whether they had been moving since getting up or since the last prompt. Given a positive response, they further indicated the mode of passive or active transportation used (e.g., walking, riding a bike, taking a bus or car). In the evening, participants were asked how much time they had spent on high and moderate physical activities that day and the duration of those types of activities, using a shortened version of the IPAQ (Booth et al., 1996). In addition, we asked for ratings of the duration of active and passive locomotion, and whether individuals had been to atypical locations that day (i.e., those visited less than twice a month). They also specified their daily life space by indicating how far away from their home they had been during that day (Stalvey et al., 1999).
General activity. At each prompt participants indicated their current activity from a list of 13 options (e.g., social interaction, resting, doctor’s appointment, reading, or other) and how long they had been engaged in it (Wrzus et al., 2015). They also rated how physically and mentally exhausting the selected activity was.
Metacognition. Time focus was measured with a single item on the past, present or future focus of participants’ momentary thoughts (Demiray et al., 2016). To measure momentary mind wandering, participants were asked whether their thoughts were unrelated to what they were doing at the moment of the prompt (Carriere et al., 2013). In the evening assessment, future time perspective was measured with two items addressing the perception of time and the perception of possibilities as limited or infinite (Lang and Carstensen, 2002; Allemand et al., 2012).
Self-reported Compliance. In the evenings, we asked individuals whether they had been wearing the uTrail continuously during the day and for possible reasons for non-wearing time (e.g., activity in water, forgetfulness). To measure compliance regarding the questionnaires, participants indicated the extent to which they had just clicked through any of the questions during that day.
2.3.5. Ambulatory assessment: Cognitive test
As part of each momentary prompt, and after responding to all experience sampling questions, participants completed two trials of a numeric memory-updating task on their smartphone that has been shown to reliably assess numerical working memory performance in an ambulatory setting, including older adults (e.g., Riediger et al., 2011). The task involved a 2 × 2 grid with a total of four digits between 0 and 9. After starting the task via button press, the initial digits were randomly updated by an addition or subtraction between −8 and +8, which appeared consecutively in a random order in each of the four cells. Two consecutive updating operations did not appear in the same cell. Participants had to continuously update and remember the new resulting digit in each cell. After the final operation, participants had to fill in the final result in each of the four cells, correct their results if necessary, and then finish the task again via button press. They then received feedback on the number of correct cells in each trail (e.g., 3 out of 4 correct cells). We used the proportion correct across both trials as the momentary working memory indicator, with a possible range of values between 0 and 1, where higher scores indicated a greater number of correct answers and thus higher working memory performance. Daily working memory was calculated as the average of the momentary working memory scores across a day.
The momentary assessment at each prompt consisted of two trials: an easier task version based on Riediger et al. (2011) and a more difficult version based on Oberauer and Kliegl (2001) and Schmiedek et al. (2010). In the easier (vs. more difficult) version, presentation time was initially 6,000 ms (vs. 4,000 ms), and 3,500 ms (vs. 1,250 ms) at each of the following operations, the time between operations was 500 ms (vs. 250 ms), and there were 5 (vs. 8) operations in total. After the final operation, participants had to fill in the final result in each of the four cells by pressing the finish button, they then received feedback on the proportion of correct cells (e.g., 3 out of 4 correct cells).
2.3.6. Intermediate assessment
The goal of the intermediate assessment was to use the opportunity of participants returning after the first 2 weeks of the ambulatory assessment period to swap and drop off mobile sensor devices to ask a few questions retrospectively over those first 2 weeks to check for possible immediate changes in select constructs following the intensive experience-sampling period.
Subjective well-being. Participants rated the frequency of experiencing each of a number of positive and negative affects with regard to the preceding 2 weeks (rather than during the past year) that were identical to the ones used at baseline and posttest.
Mind wandering. To assess mind wandering and its temporal focus and emotional tone during the preceding 2 weeks, participants responded to a set of 11 items selected from the larger questionnaires used at baseline.
Smartphone handling. Participants answered questions about their experiences using the smartphone and with the cognition task (e.g., perceived difficulty of the task and user friendliness of the ESM application). They were also asked about the use of particular strategies to solve the cognition task (e.g., ignoring certain boxes, repeating the digits to oneself or making notes). Participants also indicated whether they considered the seven prompts per day to appropriately capture their daily experiences.
Working memory. Participants completed 2 trials of the working memory task on the study smartphones.
General feedback. Additionally, participants provided oral feedback about the past 2 weeks to the research staff, which was then written down in an open format.
2.4. Analytic approach and power analysis
We plan to primarily use mixed-effect models to examine between- and within-person associations between activity engagement, cognitive abilities/health/well-being, and contextual correlates. Mixed-effect models flexibly and simultaneously estimate associations at the levels of between-person and within-person, as well as across-level interactions, taking into account autocorrelated within-person errors of the repeatedly assessed variables (Bolger and Laurenceau, 2013). In order to determine the sample size of the main study, we used data from one of the pilot studies of 27 older adults completing daily mobile surveys over 30 days on a select set of similar questionnaires. Specifically, we conducted Monte Carlo simulations using the “simr” package in R based on these prior pilot data, referring to existing guidance (Bolger and Laurenceau, 2013; Green and MacLeod, 2016). More specifically, we extracted means and variances of example variables by estimating the pilot data through mixed-effects models in the “lme4” package in R (Bates et al., 2007). We then calculated standardized effect sizes (i.e., Cohen’s d) and variances (based on variance partitioning coefficients [VPCs]) from the mixed-effects models according to the guidance of Westfall et al. (2014). Finally, based on the extracted information, we applied Monte Carlo simulations (i.e., 1,000 times) to calculate the sample size of the main study.
For example, we estimated within-person association between the repeatedly assessed variable of life satisfaction (7-point scale: 0 = not at all to 6 = very much) and occurrence of positive events (0 = no, 1 = yes); and a moderator analysis with a baseline variable of loneliness (7-point scale: 0 = does not apply at all to 6 = applies very well). The repeatedly assessed variables of life satisfaction and occurrence of positive events had about 0.4 intraclass correlation coefficients (i.e., ICCs). The ICC scores suggest a medium-to-large degree of similarity among the repeated measurements within persons, which are similar to existing studies that have repeated assessments (Arend and Schäfer, 2019). We aimed to achieve power of 0.80 with an alpha 0.05 to detect small-to-medium size of fixed effects (Cohen’s d = 0.2 to 0.5; Westfall et al., 2014). Thus, we arrived at the seven prompts per day over 15 days in 150 older adults for the ambulatory assessment phase. We chose to extend the activity sensing by uTrail, involving less participant burden, for an additional 2 weeks to finally cover a full 4-week cycle in order to obtain information on possible week-to-week variation in some mobility and activity patterns.
3. Discussion and outlook
This is the first study which combines different ambulatory assessment methods and tools (ranging from passive activity sensing to self-reports and ambulatory cognition tests) to observe community-dwelling older adults’ spatial, physical, and social activities jointly and together with well-being, health, cognition, and contextual information over up to 1 month. We have obtained a high degree of compliance in the intensive experience-sampling phase that covered a period of 2 weeks. We obtained an average of 96.67 (SD = 13.89) of the possible 105 (i.e., 7 × 15 days) prompts. Responding to the feedback questions regarding usability and experiences with the uTrail, our sample reported, on average, moderate to high usability and very low levels of disturbance and influence on daily life routines (see Figure 5). In addition, on a range of 0 (“not typical”) to 4 (“very typical), the ambulatory assessment period was rated on average as “rather typical” for participants’ life in general (M = 3.03, SD = 0.88).
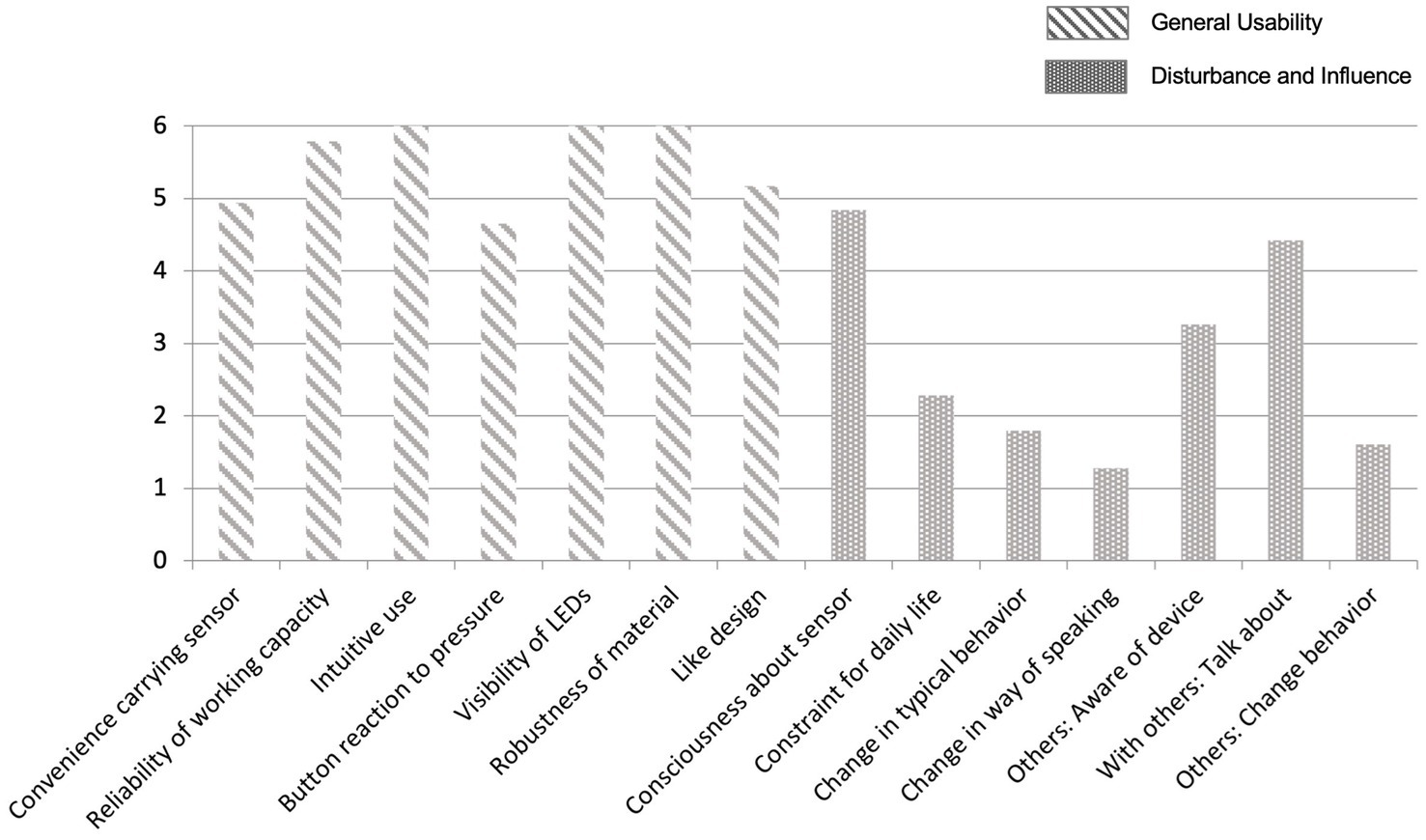
Figure 5. Evaluation of uTrail handling and experience by participants. Response scale ranged from 0 (does not apply at all) to 6 (applies very well).
This study offers unique opportunities for research into how healthy aging manifests itself in daily life activities across multiple key domains (i.e., mobility, physical activity, social interactions, cognitive activities). Sampling activity across multiple domains (and not just on physical activity, or spatial mobility) provides the added benefit of capturing a more complete picture of the ingredients of what characterizes inter- and intraindividual differences in behavioral and health-related patterns and profiles at different stages of the lifespan. It offers a unique opportunity to collect intensive objective evidence to understand daily activities across multiple domains that characterize healthy aging at the inter- and intra-individual levels. The inclusion of both passive and active sensing and self-report as well as performance data further provides a unique opportunity to establish associations between behavioral data in relation to subjective experience (Müller et al., 2020) and, potentially, to compare daily activities captured by passive sensing vs. self-report (i.e., experience sampling; Shiroma et al., 2015; Sun et al., 2019; Fillekes et al., 2019a).
Further, the 30-day long observation period of different sensing technologies offers unique opportunities to extract innovative indicators for different types of activities (Fillekes et al., 2019b; Demiray et al., 2020). The study design also enables analyses on complex time series data which is only possible with sufficient amount of data (Paraschiv-Ionescu et al., 2012; D’Mello and Gruber, 2021). These data provide a rich basis to apply a wide range of emerging analytical tools from longitudinal data analyses that address the various levels of analysis, to emerging approaches of multimodal data integration and machine learning. In particular, compared to prior studies that included only a handful of days of audio recordings (Yordanova et al., 2019; Ferrario et al., 2020, 2022), our study offers sufficient amount of data that is necessary to develop machine learning approaches that can help to automate key tasks in speech data analytics (i.e., speaker identification) for naturally occurring speech in daily real-life contexts (i.e., noisy data) and would, in turn, reduce time and personnel efforts required to (pre-)process the data.
Eventually, the first 30-day measurement period (as well as the accompanying baseline, intermediate and posttest assessments) will be repeated longitudinally to examine the interplay between daily lifestyle activity variability and long-term development. This follow-up (i.e., second burst assessment; Nesselroade, 1991), is planned for spring to summer 2023 and will include a re-assessment of the original MOASIS cohort, but in addition we aim to expand the sample by a new group of older adults with a greater range of mobility-related functional health and more diverse physical health profiles, and a young adult comparison group to examine age-related differences in activity antecedents, correlates, and outcomes over a larger portion of the adult lifespan.
With rich information of activities, health, and well-being in the current and the following bursts, our data can be used for global comparisons of healthy aging during The Decade of Healthy Aging (2021–2030) as long as the data are based on relatively unobtrusive measurement approaches. As a first effort of examining country- and thus context-specificity and comparability, we have begun to build a global network for a multi-country study on functional ability, in which region-specific modifications of the core MOASIS design are used in data collections in Hong Kong and Mexico City (World Health Organization, 2020). In sum, the MOASIS project offers data to facilitate innovative research to understand daily activities as a resource for older adults’ health and well-being.
4. Ethics and dissemination
The MOASIS project was conducted in compliance with the ethics regulations outlined in the Declaration of Helsinki. Participants received detailed information on the entire study procedure and all technical details in particular and signed an informed consent form in the recruitment process when agreeing to participate in the study. This study protocol was evaluated and approved by the Ethics Committee of the Faculty of Arts and Social Sciences at the University of Zurich (permission no. 17.2.4). Particular emphasis was put on ensuring privacy and data protection given the protocol involves the microlongitudinal and thus high-density collection of data from multiple sensors and sources for any given individual. All data are stored using numeric identifiers for anonymization on password-protected servers accessible only to authorized members of the research team.
Privacy protection particularly regarding the audio assessments were ensured through the following ways: (a) In the evening, the request to participants was to charge the uTrail, with the additional and explicitly outlined benefit of the uTrails being technically prevented from recording any data during the charging process. (b) In order to protect privacy during the day, participants had the possibility to activate a mute-button on the top of the device which stopped the audio sensor from recording for the following 15 min. A red LED light indicated to participants that muting was in progress. (c) The selected sampling rate ensured that only 2.5% of participants’ waking time, on average, was recorded with an average of three recordings (i.e., 2.5 min) per hour. (d) When returning the uTrail at the intermediate and posttest sessions, we asked whether prior to storage of the audio data on our servers, participants wished to screen individual audio files from a particular day and time in case of an incident or conversation they wanted to delete or at least check on. In that case, participants could listen to as many files as they wanted and then provided written consent for each of the two 2-week tracking periods, that we were allowed to download and store the audio and the other data on our storage using the numeric participant code as sole identifier. (e) We asked participants to proactively inform their social interaction partners about participation in the study and about the repeated audio recordings. We emphasized that our analytic focus was solely on the participant’s utterings, and that any transcripts and ratings focused exclusively on these and did not involve any speech utterances by non-participants.
Finally, dissemination of the study’s findings is planned within the scientific community and stakeholders from the general public and the policy sector (e.g., at the national and global level, such as Swiss Academy of Sciences, WHO).
Ethics statement
The studies involving human participants were reviewed and approved by Ethics Committee of the Faculty of Arts and Social Sciences at the University of Zurich. The patients/participants provided their written informed consent to participate in this study.
Author contributions
CR, RW, and MM are the principal investigators of the MOASIS study and acquired the funding. Together with PB, MK, and MF they conceived and designed the study. CR has the project management lead. Together with ML, MK, AS, and VG, CR has been coordinating the data collection campaigns. RW supervises and AS implements the uTrail development and quality assurance. CR and ML wrote the original draft of the manuscript. All authors contributed to the article and approved the submitted version.
Funding
The research reported in this paper was supported by the Velux Stiftung (project no. 917) and the University Research Priority Program (URPP) “Dynamics of Healthy Aging” at the University of Zurich.
Acknowledgments
We thank Brigitte Sonderegger and Corinne Boillat for the recruitment of participants, our student assistants for their invaluable contribution to data collection and above all, our participants for their time and willingness to take part in this research.
Conflict of interest
The authors declare that the research was conducted in the absence of any commercial or financial relationships that could be construed as a potential conflict of interest.
Publisher’s note
All claims expressed in this article are solely those of the authors and do not necessarily represent those of their affiliated organizations, or those of the publisher, the editors and the reviewers. Any product that may be evaluated in this article, or claim that may be made by its manufacturer, is not guaranteed or endorsed by the publisher.
References
Abler, B., and Kessler, H. (2009). Emotion regulation questionnaire–Eine deutschsprachige Fassung des ERQ von Gross und John. Diagnostica 55, 144–152. doi: 10.1026/0012-1924.55.3.144
Adams, K. B., Leibbrandt, S., and Moon, H. (2011). A critical review of the literature on social and leisure activity and wellbeing in later life. Ageing Soc. 31, 683–712. doi: 10.1017/S0144686X10001091
Allahbakhshi, H., Conrow, L., Naimi, B., and Weibel, R. (2020). Using accelerometer and GPS data for real-life physical activity type detection. Sensors 20:588. doi: 10.3390/s20030588
Allemand, M., Hill, P. L., Ghaemmaghami, P., and Martin, M. (2012). Forgivingness and subjective well-being in adulthood: the moderating role of future time perspective. J. Res. Pers. 46, 32–39. doi: 10.1016/j.jrp.2011.11.004
Almeida, D. M., Wethington, E., and Kessler, R. C. (2002). The daily inventory of stressful events: an interview-based approach for measuring daily stressors. Assessment 9, 41–55. doi: 10.1177/1073191102091006
Arend, M. G., and Schäfer, T. (2019). Statistical power in two-level models: a tutorial based on Monte Carlo simulation. Psychol. Methods 24, 1–19. doi: 10.1037/met0000195
Aron, A., Aron, E. N., and Smollan, D. (1992). Inclusion of other in the self scale and the structure of interpersonal closeness. J. Pers. Soc. Psychol. 63, 596–612. doi: 10.1037/0022-3514.63.4.596
Aschenbrenner, S., Tucha, O., and Lange, K. W. (2000). Regensburger Wortflüssigkeitstest. Göttingen: Hogrefe.
Baltes, P. B., Lindenberger, U., and Staudinger, U. M. (1998). “Life-span theory in developmental psychology,” in Handbook of child psychology: Theoretical models of human development. eds. W. Damon and R. M. Lerner (John Wiley & Sons Inc.), 1029–1143.
Bates, D., Sarkar, D., Bates, M. D., and Matrix, L. (2007). The lme4 package. R Package Version 2, 74.
Berscheid, E., Snyder, M., and Omoto, A. M. (1989). The relationship closeness inventory: assessing the closeness of interpersonal relationships. J. Pers. Soc. Psychol. 57, 792–807. doi: 10.1037/0022-3514.57.5.792
Bielak, A. A. (2017). Different perspectives on measuring lifestyle engagement: a comparison of activity measures and their relation with cognitive performance in older adults. Aging Neuropsychol. Cogn. 24, 435–452. doi: 10.1080/13825585.2016.1221378
Bielak, A. A., Mogle, J. A., and Sliwinski, M. J. (2019). Two sides of the same coin? Association of variety and frequency of activity with cognition. Psychol. Aging 34, 457–466. doi: 10.1037/pag0000350
Bluck, S., Alea, N., Habermas, T., and Rubin, D. C. (2005). A tale of three functions: the self–reported uses of autobiographical memory. Soc. Cogn. 23, 91–117. doi: 10.1521/soco.23.1.91.59198
Bödeker, M., and Bucksch, J. (2011). Deutschsprachige Fassung der Neighborhood Environmental Walkability Scale (NEWS)–Entwicklung und erste Hinweise zur Testgüte. Gesundheitswesen 73:A82. doi: 10.1055/s-0031-1283423
Bolger, N., and Laurenceau, J.-P. (2013). Intensive longitudinal methods: An introduction to diary and experience sampling research. New York, NY: Guilford Press.
Booth, M. L., Owen, N., Bauman, A. E., and Gore, C. J. (1996). Retest reliability of recall measures of leisure-time physical activity in Australian adults. Int. J. Epidemiol. 25, 153–159. doi: 10.1093/ije/25.1.153
Brans, K., Koval, P., Verduyn, P., Lim, Y. L., and Kuppens, P. (2013). The regulation of negative and positive affect in daily life. Emotion 13, 926–939. doi: 10.1037/a0032400
Brianza, E., and Demiray, B. (2019). Future time perspective and real-life utterances about the future in young and older adults. Gerontopsychology 32, 167–173. doi: 10.1024/1662-9647/a000216
Brose, A., and Ebner-Priemer, U. W. (2015). Ambulatory assessment in the research on aging: contemporary and future applications. Gerontology 61, 372–380. doi: 10.1159/000371707
Brose, A., Schmiedek, F., Gerstorf, D., and Voelkle, M. C. (2020). The measurement of within-person affect variation. Emotion 20, 677–699. doi: 10.1037/emo0000583
Brunstein, J. C. (1999). Persönliche Ziele und subjektives Wohlbefinden bei älteren Menschen. Zeitschrift für Differentielle und Diagnostische Psychologie 20, 58–71. doi: 10.1024/0170-1789.20.1.58
Burzynska, A. Z., Voss, M. W., Fanning, J., Salerno, E. A., Gothe, N. P., McAuley, E., et al. (2020). Sensor-measured sedentariness and physical activity are differentially related to fluid and crystallized abilities in aging. Psychol. Aging 35, 1154–1169. doi: 10.1037/pag0000580
Butterfield, R. M., and Lewis, M. A. (2002). Health-related social influence: a social ecological perspective on tactic use. J. Soc. Pers. Relat. 19, 505–526. doi: 10.1177/0265407502019004050
Campbell, L. M., Paolillo, E. W., Heaton, A., Tang, B., Depp, C. A., Granholm, E., et al. (2020). Daily activities related to Mobile cognitive performance in middle-aged and older adults: an ecological momentary cognitive assessment study. JMIR Mhealth Uhealth 8:e19579. doi: 10.2196/19579
Carriere, J. S., Cheyne, J. A., and Smilek, D. (2008). Everyday attention lapses and memory failures: the affective consequences of mindlessness. Conscious. Cogn. 17, 835–847. doi: 10.1016/j.concog.2007.04.008
Carriere, J. S., Seli, P., and Smilek, D. (2013). Wandering in both mind and body: individual differences in mind wandering and inattention predict fidgeting. Can. J. Exp. Psychol. Can. Psychol. Exp. 67, 19–31. doi: 10.1037/a0031438
Carvalho, S., Leite, J., Galdo-Álvarez, S., and Gonçalves, O. F. (2012). The emotional movie database (EMDB): a self-report and psychophysiological study. Appl. Psychophysiol. Biofeedback 37, 279–294. doi: 10.1007/s10484-012-9201-6
Chaix, B. (2018). Mobile sensing in environmental health and neighborhood research. Annu. Rev. Public Health 39, 367–384. doi: 10.1146/annurev-publhealth-040617-013731
Conner, T. S., and Mehl, M. R. (2015). Ambulatory assessment: methods for studying everyday life. Emerg. Trends Soc. Behav. Sci. Interdiscip. Search. Link. Resour. 2015, 1–15.
Cornet, V. P., and Holden, R. J. (2018). Systematic review of smartphone-based passive sensing for health and wellbeing. J. Biomed. Inform. 77, 120–132. doi: 10.1016/j.jbi.2017.12.008
Craig, C. L., Marshall, A. L., Sjöström, M., Bauman, A. E., Booth, M. L., Ainsworth, B. E., et al. (2003). International physical activity questionnaire: 12-country reliability and validity. Med. Sci. Sports Exerc. 35, 1381–1395. doi: 10.1249/01.MSS.0000078924.61453.FB
Cyranowski, J. M., Zill, N., Bode, R., Butt, Z., Kelly, M. A., Pilkonis, P. A., et al. (2013). Assessing social support, companionship, and distress: National Institute of health (NIH) toolbox adult social relationship scales. Health Psychol. 32, 293–301. doi: 10.1037/a0028586
D’Mello, S. K., and Gruber, J. (2021). Emotional regularity: associations with personality, psychological health, and occupational outcomes. Cognit. Emot. 35, 1460–1478. doi: 10.1080/02699931.2021.1968797
Danner, D., Rammstedt, B., Bluemke, M., Treiber, L., Berres, S., Soto, C. J., et al. (2016). Die Deutsche Version des Big Five Inventory 2 (BFI-2). Mannheim: GESIS - Leibniz-Institut für Sozialwissenschaften. doi: 10.6102/zis247
Deci, E. L., and Ryan, R. M. (1985). The general causality orientations scale: self-determination in personality. J. Res. Pers. 19, 109–134. doi: 10.1016/0092-6566(85)90023-6
Demiray, B., Luo, M., Tejeda-Padron, A., and Mehl, M. R. (2020). “Sounds of healthy aging: assessing everyday social and cognitive activity from ecologically sampled ambient audio data” in Personality and healthy aging in adulthood. eds. P. L. Hill and M. Allemand (Springer Nature Switzerland AG), 111–132.
Demiray, B., Martin, M., Hernández, N., Yavuz, G., and Arnrich, B. (2016). Within-person variability in the relation between mental time travel and mood: An experience sampling study in everyday life. Poster presented at the Conference on Mental Time Travel, Center on Autobiographical Memory Research, Aarhus, Denmark.
Demiray, B., Mehl, M. R., and Martin, M. (2018). Conversational time travel: Evidence of a retrospective bias in real life conversations. Front. Psychol. 9:2160. doi: 10.3389/fpsyg.2018.02160
Demiray, B., Mischler, M., and Martin, M. (2019). Reminiscence in everyday conversations: a naturalistic observation study of older adults. J. Gerontol. Ser. B 74, 745–755. doi: 10.1093/geronb/gbx141
Diener, E. D., Emmons, R. A., Larsen, R. J., and Griffin, S. (1985). The satisfaction with life scale. J. Pers. Assess. 49, 71–75. doi: 10.1207/s15327752jpa4901_13
Dillon, C. B., McMahon, E., O’Regan, G., and Perry, I. J. (2018). Associations between physical behaviour patterns and levels of depressive symptoms, anxiety and well-being in middle-aged adults: a cross-sectional study using isotemporal substitution models. BMJ Open 8:e018978. doi: 10.1136/bmjopen-2017-018978
Dixon, R. A., Hultsch, D. F., and Hertzog, C. (1988). The metamemory in adulthood (MIA) questionnaire. Psychopharmacol. Bull. 24, 671–688.
Dodge, S., Weibel, R., and Forootan, E. (2009). Revealing the physics of movement: comparing the similarity of movement characteristics of different types of moving objects. Comput. Environ. Urban. Syst. 33, 419–434. doi: 10.1016/j.compenvurbsys.2009.07.008
Ferrario, A., Demiray, B., Yordanova, K., Luo, M., and Martin, M. (2020). Social reminiscence in older adults’ everyday conversations: automated detection using natural language processing and machine learning. J. Med. Internet Res. 22:e19133. doi: 10.2196/19133
Ferrario, A., Luo, M., Polsinelli, A. J., Moseley, S. A., Mehl, M. R., Yordanova, K., et al. (2022). Predicting working memory in healthy older adults using real-life language and social context information: a machine learning approach. JMIR Aging 5:e28333. doi: 10.2196/28333
Fillekes, M. P., Giannouli, E., Kim, E.-K., Zijlstra, W., and Weibel, R. (2019a). Towards a comprehensive set of GPS-based indicators reflecting the multidimensional nature of daily mobility for applications in health and aging research. Int. J. Health Geogr. 18, 1–20. doi: 10.1186/s12942-019-0181-0
Fillekes, M. P., Röcke, C., Katana, M., and Weibel, R. (2019b). Self-reported versus GPS-derived indicators of daily mobility in a sample of healthy older adults. Soc. Sci. Med. 220, 193–202. doi: 10.1016/j.socscimed.2018.11.010
Fingerman, K. L., Ng, Y. T., Zhang, S., Britt, K., Colera, G., Birditt, K. S., et al. (2021). Living alone during COVID-19: social contact and emotional well-being among older adults. J. Gerontol. Ser. B 76, e116–e121. doi: 10.1093/geronb/gbaa200
French, J. W., Ekstrom, R. B., and Price, L. A. (1963). Manual for kit of reference tests for cognitive factors (revised 1963). Educational Testing Service Princeton NJ.
Giambra, L. M. (1989). Task-unrelated thought frequency as a function of age: a laboratory study. Psychol. Aging 4, 136–143. doi: 10.1037/0882-7974.4.2.136
Green, P., and MacLeod, C. J. (2016). SIMR: an R package for power analysis of generalized linear mixed models by simulation. Methods Ecol. Evol. 7, 493–498. doi: 10.1111/2041-210X.12504
Gross, J. J., and John, O. P. (2003). Individual differences in two emotion regulation processes: implications for affect, relationships, and well-being. J. Pers. Soc. Psychol. 85, 348–362. doi: 10.1037/0022-3514.85.2.348
Grühn, D., Kotter-Grühn, D., and Röcke, C. (2010). Discrete affects across the adult lifespan: Evidence for multidimensionality and multidiretionality of affective experiences in young, middle-aged and older adults. Journal of Research in Personality 44, 492–500. doi: 10.1016/j.jrp.2010.06.003
Guralnik, J. M., Simonsick, E. M., Ferrucci, L., Glynn, R. J., Berkman, L. F., Blazer, D. G., et al. (1994). A short physical performance battery assessing lower extremity function: association with self-reported disability and prediction of mortality and nursing home admission. J. Gerontol. 49, M85–M94. doi: 10.1093/geronj/49.2.M85
Haas, M., Mehl, M. R., Ballhausen, N., Zuber, S., Kliegel, M., and Hering, A. (2022). The sounds of memory: extending the age-prospective memory paradox to everyday behavior and conversations. J. Gerontol. Ser. B. 77, 695–703. doi: 10.1093/geronb/gbac012
Hamaker, E. L. (2012). “Why researchers should think" within-person": a paradigmatic rationale” in Handbook of research methods for studying daily life. eds. M. R. Mehl and T. S. Conner (New York, NY: The Guilford Press), 43–61.
Harada, K., Lee, S., Lee, S., Bae, S., Harada, K., Suzuki, T., et al. (2017). Objectively-measured outdoor time and physical and psychological function among older adults. Geriatr Gerontol Int 17, 1455–1462. doi: 10.1111/ggi.12895
Harari, G. M., Müller, S. R., Aung, M. S., and Rentfrow, P. J. (2017). Smartphone sensing methods for studying behavior in everyday life. Curr. Opin. Behav. Sci. 18, 83–90. doi: 10.1016/j.cobeha.2017.07.018
Harari, G. M., Vaid, S. S., Müller, S. R., Stachl, C., Marrero, Z., Schoedel, R., et al. (2020). Personality sensing for theory development and assessment in the digital age. Eur. J. Personal. 34, 649–669. doi: 10.1002/per.2273
Hatt, C. R., Brydges, C. R., Mogle, J. A., Sliwinski, M. J., and Bielak, A. A. M. (2021). Evaluating the consistency of subjective activity assessments and their relation to cognition in older adults. Geriatrics 6:74. doi: 10.3390/geriatrics6030074
Helmstaedter, C., Lendt, M., and Lux, S. (2001). VLMT: Verbaler Lern-und Merkfähigkeitstest: Beltz Test. Gött. Germany.
Hertzog, C., Kramer, A. F., Wilson, R. S., and Lindenberger, U. (2008). Enrichment effects on adult cognitive development: can the functional capacity of older adults be preserved and enhanced? Psychol. Sci. Public Interest 9, 1–65. doi: 10.1111/j.1539-6053.2009.01034.x
Horgas, A. L., Wilms, H.-U., and Baltes, M. M. (1998). Daily life in very old age: everyday activities as expression of successful living. The Gerontologist 38, 556–568. doi: 10.1093/geront/38.5.556
Iwarsson, S., Sixsmith, J., Oswald, F., Wahl, H.-W., Nygren, C., Sixsmith, A., et al. (2004). “The ENABLE-AGE Project: multi-dimensional methodology for European housing research,” in Housing Research Methodologies. eds. N. Wilkinson, and Y. Hurol (Mersin: Urban International), 70–90.
Jopp, D. S., and Hertzog, C. (2010). Assessing adult leisure activities: an extension of a self-report activity questionnaire. Psychol. Assess. 22, 108–120. doi: 10.1037/a0017662
Kamalyan, L., Yang, J.-A., Pope, C. N., Paolillo, E. W., Campbell, L. M., Tang, B., et al. (2021). Increased social interactions reduce the association between constricted life-space and lower daily happiness in older adults with and without HIV: a GPS and ecological momentary assessment study. Am. J. Geriatr. Psychiatry 29, 867–879. doi: 10.1016/j.jagp.2020.11.005
Kaspar, R., Oswald, F., Wahl, H.-W., Voss, E., and Wettstein, M. (2015). Daily mood and out-of-home mobility in older adults: does cognitive impairment matter? J. Appl. Gerontol. 34, 26–47. doi: 10.1177/0733464812466290
Kasser, V. G., and Ryan, R. M. (1999). The relation of psychological needs for autonomy and relatedness to vitality, well-being, and mortality in a nursing home 1. J. Appl. Soc. Psychol. 29, 935–954. doi: 10.1111/j.1559-1816.1999.tb00133.x
Klumb, P. L. (1995). Cognitive failures and performance differences: validation studies of a German version of the cognitive failures questionnaire. Ergonomics 38, 1456–1467. doi: 10.1080/00140139508925202
Kuppens, P., and Verduyn, P. (2017). Emotion dynamics. Curr. Opin. Psychol. 17, 22–26. doi: 10.1016/j.copsyc.2017.06.004
Lachman, M. E., and Weaver, S. L. (1998). Sociodemographic variations in the sense of control by domain: findings from the MacArthur studies of midlife. Psychol. Aging 13, 553–562. doi: 10.1037/0882-7974.13.4.553
Lang, F. R., and Carstensen, L. L. (2002). Time counts: future time perspective, goals, and social relationships. Psychol. Aging 17, 125–139. doi: 10.1037/0882-7974.17.1.125
Lawton, M. P., and Brody, E. M. (1969). Assessment of older people: self-maintaining and instrumental activities of daily living. The Gerontologist 9, 179–186. doi: 10.1093/geront/9.3_Part_1.179
Lazarević, L. B., Bjekić, J., Živanović, M., and Knežević, G. (2020). Ambulatory assessment of language use: evidence on the temporal stability of electronically activated recorder and stream of consciousness data. Behav. Res. Methods 52, 1817–1835. doi: 10.3758/s13428-020-01361-z
Lindenberger, U., Smith, J., Mayer, K. U., and Baltes, P. B. (Hrsg.) (2010). Die Berliner Altersstudie (3. Aufl.). Berlin: Akademie Verlag.
Luo, M., Debelak, R., Schneider, G., Martin, M., and Demiray, B. (2020). With a little help from familiar interlocutors: real-world language use in young and older adults. Aging Ment. Health 25, 2310–2319. doi: 10.1080/13607863.2020.1822288
Luo, M., Robbins, M. L., Martin, M., and Demiray, B. (2019). Real-life language use across different interlocutors: a naturalistic observation study of adults varying in age. Front. Psychol. 10:1412. doi: 10.3389/fpsyg.2019.01412
Macdonald, B., Becker, M., Martin, M., and Röcke, C. (in press). “Mobile sensing in aging research,” in Handbook on Mobile Sensing in Psychology, eds. M. Mehl, M. Eid, C. Wrzus, G. Harari, and U. Ebner-Priemer (New York, NY: Guilford)
Maher, J. P., and Conroy, D. E. (2017). Daily life satisfaction in older adults as a function of (in) activity. J. Gerontol. B Psychol. Sci. Soc. Sci. 72, 593–602. doi: 10.1093/geronb/gbv086
Martin, P., Kelly, N., Kahana, B., Kahana, E., Willcox, B. J., Willcox, D. C., et al. (2015). Defining successful aging: a tangible or elusive concept? The Gerontologist 55, 14–25. doi: 10.1093/geront/gnu044
Mc Ardle, R., Del Din, S., Donaghy, P., Galna, B., Thomas, A., and Rochester, L. (2020). Factors that influence habitual activity in mild cognitive impairment and dementia. Gerontology 66, 197–208. doi: 10.1159/000502288
Mehl, M. R., and Pennebaker, J. W. (2003). The sounds of social life: a psychometric analysis of students’ daily social environments and natural conversations. J. Pers. Soc. Psychol. 84, 857–870. doi: 10.1037/0022-3514.84.4.857
Mehl, M. R., Pennebaker, J. W., Crow, D. M., Dabbs, J., and Price, J. H. (2001). The Electronically Activated Recorder (EAR): a device for sampling naturalistic daily activities and conversations. Behavior research methods, instruments, & computers : a journal of the Psychonomic Society, Inc 33, 517–523. doi: 10.3758/bf03195410
Michalak, J., Heidenreich, T., Ströhle, G., and Nachtigall, C. (2008). Die deutsche version der mindful attention and awareness scale (maas) psychometrische befunde zu einem achtsamkeitsfragebogen. Z. Für Klin. Psychol. Psychother. 37, 200–208. doi: 10.1026/1616-3443.37.3.200
Michel, J.-P., and Sadana, R. (2017). “Healthy aging” concepts and measures. J. Am. Med. Dir. Assoc. 18, 460–464. doi: 10.1016/j.jamda.2017.03.008
Milek, A., Butler, E. A., Tackman, A. M., Kaplan, D. M., Raison, C. L., Sbarra, D. A., et al. (2018). “Eavesdropping on happiness” revisited: a pooled, multisample replication of the association between life satisfaction and observed daily conversation quantity and quality. Psychol. Sci. 29, 1451–1462. doi: 10.1177/0956797618774252
Mollenkopf, H., Marcellini, F., Ruoppila, I., Széman, Z., Tacken, M., and Wahl, H.-W. (2004). Social and behavioural science perspectives on out-of-home mobility in later life: findings from the European project MOBILATE. Eur. J. Ageing 1, 45–53. doi: 10.1007/s10433-004-0004-3
Mollenkopf, H., Meyer, S., Schulze, E., Wurm, S., and Friesdorf, W. (2000). Technik im Haushalt zur Unterstützung einer selbstbestimmten Lebensführung im Alter Das Forschungsprojekt sentha und erste Ergebnisse des Sozialwissenschaftlichen Teilprojekts. Z. Für Gerontol. Geriatr. 33, 155–168. doi: 10.1007/s003910070056
Möwisch, D., Brose, A., and Schmiedek, F. (2022). Active time use and well-being in older adulthood: results from a day reconstruction method study. Work Aging Retire. :waab030. doi: 10.1093/workar/waab030
Mrazek, M. D., Chin, J. M., Schmader, T., Hartson, K. A., Smallwood, J., and Schooler, J. W. (2011). Threatened to distraction: mind-wandering as a consequence of stereotype threat. J. Exp. Soc. Psychol. 47, 1243–1248. doi: 10.1016/j.jesp.2011.05.011
Müller, S. R., Peters, H., Matz, S. C., Wang, W., and Harari, G. M. (2020). Investigating the relationships between mobility behaviours and indicators of subjective well–being using smartphone–based experience sampling and GPS tracking. Eur. J. Personal. 34, 714–732. doi: 10.1002/per.2262
Nesselroade, J. R. (1991). “The warp and the woof of the developmental fabric,” in Visions of aesthetics, the environment and development: The legacy of Joachim Wohlwill, eds. R. M. Downs, L. S. Liben, and D. S. Palermo (Hillsdale, NJ: Erlbaum), 213–240.
Oberauer, K., and Kliegl, R. (2001). Beyond resources: formal models of complexity effects and age differences in working memory. Eur. J. Cogn. Psychol. 13, 187–215. doi: 10.1080/09541440042000278
Paolillo, E. W., Tang, B., Depp, C. A., Rooney, A. S., Vaida, F., Kaufmann, C. N., et al. (2018). Temporal associations between social activity and mood, fatigue, and pain in older adults with HIV: an ecological momentary assessment study. JMIR Ment. Health 5:e9802. doi: 10.2196/mental.9802
Paraschiv-Ionescu, A., Perruchoud, C., Buchser, E., and Aminian, K. (2012). Barcoding human physical activity to assess chronic pain conditions. PLoS One 7:e32239. doi: 10.1371/journal.pone.0032239
Pauly, T., Lay, J. C., Nater, U. M., Scott, S. B., and Hoppmann, C. A. (2017). How we experience being alone: age differences in affective and biological correlates of momentary solitude. Gerontology 63, 55–66. doi: 10.1159/000450608
Petersen, J., Austin, D., Mattek, N., and Kaye, J. (2015). Time out-of-home and cognitive, physical, and emotional wellbeing of older adults: a longitudinal mixed effects model. PLoS One 10:e0139643. doi: 10.1371/journal.pone.0139643
Pham, V. T., Nguyen, D. A., Dang, N. D., Pham, H. H., Tran, V. A., Sandrasegaran, K., et al. (2018). Highly accurate step counting at various walking states using low-cost inertial measurement unit support indoor positioning system. Sensors 18:3186. doi: 10.3390/s18103186
Polsinelli, A. J., Moseley, S. A., Grilli, M. D., Glisky, E. L., and Mehl, M. R. (2020). Natural, everyday language use provides a window into the integrity of older adults’ executive functioning. J. Gerontol. Ser. B 75, e215–e220. doi: 10.1093/geronb/gbaa055
Rasch, B., Büchel, C., Gais, S., and Born, J. (2007). Odor cues during slow-wave sleep prompt declarative memory consolidation. Science 315, 1426–1429. doi: 10.1126/science.1138581
Rauthmann, J. F., and Sherman, R. A. (2016). Ultra-brief measures for the Situational Eight DIAMONDS domains. Eur. J. Psychol. Assess. 32, 165–174. doi: 10.1027/1015-5759/a000245
Reitan, R. M. (1992). Trail making test: Manual for administration and scoring. Reitan Neuropsychology Laboratory, Length.
Rey Auditory Verbal Learning Test (RAVLT) Rey, A. (1964). “Rey Auditory Verbal Learning Test,” in L’examen de Clinique en Psychologie. Paris: Presses Universitaires de France.
Riediger, M., Linden, M., and Wilms, H.-U. (1998). Die deutsche Version der CES-D als Instrument der gerontologischen Forschung. Z. Für Klin. Psychol. Psychiatr. Psychother. 46, 344–364.
Riediger, M., Wrzus, C., Schmiedek, F., Wagner, G. G., and Lindenberger, U. (2011). Is seeking bad mood cognitively demanding? Contra-hedonic orientation and working-memory capacity in everyday life. Emotion 11, 656–665. doi: 10.1037/a0022756
Rosenberg, M. (1965). “Society and the adolescent self-image” in Society and the adolescent self-image. New Jersey: Princeton University Press.
Rowe, J. W., and Kahn, R. L. (1997). Successful aging. The Gerontologist 37, 433–440. doi: 10.1093/geront/37.4.433
Russell, D., Peplau, L. A., and Cutrona, C. E. (1980). The revised UCLA loneliness scale: concurrent and discriminant validity evidence. J. Pers. Soc. Psychol. 39, 472–480. doi: 10.1037/0022-3514.39.3.472
Ryff, C. D. (1989). Happiness is everything, or is it? Explorations on the meaning of psychological well-being. J. Pers. Soc. Psychol. 57, 1069–1081. doi: 10.1037/0022-3514.57.6.1069
Saelens, B. E., Sallis, J. F., Black, J. B., and Chen, D. (2003). Neighborhood-based differences in physical activity: an environment scale evaluation. Am. J. Public Health 93, 1552–1558. doi: 10.2105/AJPH.93.9.1552
Scheier, M. F., Wrosch, C., Baum, A., Cohen, S., Martire, L. M., Matthews, K. A., et al. (2006). The life engagement test: assessing purpose in life. J. Behav. Med. 29, 291–298. doi: 10.1007/s10865-005-9044-1
Schmiedek, F., Lövdén, M., and Lindenberger, U. (2010). Hundred days of cognitive training enhance broad cognitive abilities in adulthood: findings from the COGITO study. Front. Aging Neurosci. 2:27. doi: 10.3389/fnagi.2010.00027
Schwarzer, R., and Jerusalem, M. (1999). Skalen zur erfassung von Lehrer-und schülermerkmalen. in Dokumentation der psychometrischen Verfahren im Rahmen der Wissenschaftlichen Begleitung des Modellversuchs Selbstwirksame Schulen. Berlin: Freie Universität Berlin.
Schwarzer, R., and Schulz, U. (2003). Soziale Unterstützung bei der Krankheitsbewältigung: Die Berliner Social Support Skalen (BSSS). Diagnostica 49, 73–82. doi: 10.1026//0012-1924.49.2.73
Scott, S. B., Sliwinski, M. J., and Blanchard-Fields, F. (2013). Age differences in emotional responses to daily stress: the role of timing, severity, and global perceived stress. Psychol. Aging 28, 1076–1087. doi: 10.1037/a0034000
Sheldon, K. M., and Hilpert, J. C. (2012). The balanced measure of psychological needs (BMPN) scale: an alternative domain general measure of need satisfaction. Motiv. Emot. 36, 439–451. doi: 10.1007/s11031-012-9279-4
Shiffman, S., Stone, A. A., and Hufford, M. R. (2008). Ecological momentary assessment. Annu. Rev. Clin. Psychol. 4, 1–32. doi: 10.1146/annurev.clinpsy.3.022806.091415
Shiroma, E. J., Cook, N. R., Manson, J. E., Buring, J. E., Rimm, E. B., and Lee, I.-M. (2015). Comparison of self-reported and accelerometer-assessed physical activity in older women. PLoS One 10:e0145950. doi: 10.1371/journal.pone.0145950
Siła-Nowicka, K., Vandrol, J., Oshan, T., Long, J. A., Demšar, U., and Fotheringham, A. S. (2016). Analysis of human mobility patterns from GPS trajectories and contextual information. Int. J. Geogr. Inf. Sci. 30, 881–906. doi: 10.1080/13658816.2015.1100731
Stalvey, B. T., Owsley, C., Sloane, M. E., and Ball, K. (1999). The life space questionnaire: a measure of the extent of mobility of older adults. J. Appl. Gerontol. 18, 460–478. doi: 10.1177/073346489901800404
Steyer, R., Schwenkmezger, P., Notz, P., and Eid, M. (1997). Der Mehrdimensionale Befindlichkeitsfragebogen MDBF [Multidimensional mood questionnaire]. Gött. Ger. Hogrefe.
Stine-Morrow, E. A., Payne, B. R., Roberts, B. W., Kramer, A. F., Morrow, D. G., Payne, L., et al. (2014). Training versus engagement as paths to cognitive enrichment with aging. Psychol. Aging 29, 891–906. doi: 10.1037/a0038244
Sturm, W., Willmes, K., and Horn, W. (1993). Leistungsprufsystem fur 50-90jährige (LPS 50þ). Gött. Hogrefe.
Sun, J., Harris, K., and Vazire, S. (2019). Is well-being associated with the quantity and quality of social interactions? J. Pers. Soc. Psychol. 119, 1478–1496. doi: 10.1037/pspp0000272
Sun, J., Schwartz, H. A., Son, Y., Kern, M. L., and Vazire, S. (2020). The language of well-being: tracking fluctuations in emotion experience through everyday speech. J. Pers. Soc. Psychol. 118, 364–387. doi: 10.1037/pspp0000244
von Aster, M., and Neubauer, A. (2009). Wechsler-Intelligenztest für Erwachsene: WIE; Manual; Übersetzung und Adaptation der WAIS-III von David Wechsler. Pearson Assessment & Information.
Von Collani, G., and Herzberg, P. Y. (2003). Eine revidierte Fassung der deutschsprachigen Skala zum Selbstwertgefühl von Rosenberg. Z. Für Differ. Diagn. Psychol. 24:37. doi: 10.1024//0170-1789.24.1.3
Vowinckel, J. C., Westerhof, G. J., Bohlmeijer, E. T., and Webster, J. D. (2017). Flourishing in the now: initial validation of a present-eudaimonic time perspective scale. Time Soc. 26, 203–226. doi: 10.1177/0961463X15577277
Wahl, H.-W., and Gerstorf, D. (2018). A conceptual framework for studying COntext dynamics in aging (CODA). Dev. Rev. 50, 155–176. doi: 10.1016/j.dr.2018.09.003
Ware, J. E. Jr., Kosinski, M., and Keller, S. D. (1996). A 12-item short-form health survey: construction of scales and preliminary tests of reliability and validity. Med. Care 34, 220–233. doi: 10.1097/00005650-199603000-00003
Watson, D., Clark, L. A., and Tellegen, A. (1988). Development and validation of brief measures of positive and negative affect: the PANAS scales. J. Pers. Soc. Psychol. 54, 1063–1070. doi: 10.1037/0022-3514.54.6.1063
Westfall, J., Kenny, D. A., and Judd, C. M. (2014). Statistical power and optimal design in experiments in which samples of participants respond to samples of stimuli. J. Exp. Psychol. Gen. 143, 2020–2045. doi: 10.1037/xge0000014
Wettstein, M., Wahl, H.-W., and Diehl, M. K. (2014). A multidimensional view of out-of-home behaviors in cognitively unimpaired older adults: examining differential effects of socio-demographic, cognitive, and health-related predictors. Eur. J. Ageing 11, 141–153. doi: 10.1007/s10433-013-0292-6
Wilhelm, P., and Schoebi, D. (2007). Assessing mood in daily life. Eur. J. Psychol. Assess. 23, 258–267. doi: 10.1027/1015-5759.23.4.258
Williams, G. C., Cox, E. M., Kouides, R., and Deci, E. L. (1999). Presenting the facts about smoking to adolescents: effects of an autonomy-supportive style. Arch. Pediatr. Adolesc. Med. 153, 959–964. doi: 10.1001/archpedi.153.9.959
World Health Organization (2002). Active ageing: A policy framework. Geneva: World Health Organization.
World Health Organization (2020). Decade of healthy ageing: Baseline report. Geneva: World Health Organization.
Wrzus, C., Luong, G., Wagner, G. G., and Riediger, M. (2015). Can’t get it out of my head: age differences in affective responsiveness vary with preoccupation and elapsed time after daily hassles. Emotion 15, 257–269. doi: 10.1037/emo0000019
Yordanova, K. Y., Demiray, B., Mehl, M. R., and Martin, M. (2019). “Automatic detection of everyday social behaviours and environments from verbatim transcripts of daily conversations.“ in 2019 IEEE international conference on pervasive computing and communications PerCom (IEEE). pp. 1–10.
Zhaoyang, R., Scott, S. B., Martire, L. M., and Sliwinski, M. J. (2021). Daily social interactions related to daily performance on mobile cognitive tests among older adults. PLoS One 16:e0256583. doi: 10.1371/journal.pone.0256583
Keywords: mobility, physical activity, social interactions, cognitive activities, ambulatory assessment, functional ability, aging
Citation: Röcke C, Luo M, Bereuter P, Katana M, Fillekes M, Gehriger V, Sofios A, Martin M and Weibel R (2023) Charting everyday activities in later life: Study protocol of the mobility, activity, and social interactions study (MOASIS). Front. Psychol. 13:1011177. doi: 10.3389/fpsyg.2022.1011177
Edited by:
Razak M. Gyasi, African Population and Health Research Center (APHRC), KenyaReviewed by:
Carmen García-Peña, Instituto Nacional de Geriatría, MexicoJesus Favela, Center for Scientific Research and Higher Education in Ensenada (CICESE), Mexico
Copyright © 2023 Röcke, Luo, Bereuter, Katana, Fillekes, Gehriger, Sofios, Martin and Weibel. This is an open-access article distributed under the terms of the Creative Commons Attribution License (CC BY). The use, distribution or reproduction in other forums is permitted, provided the original author(s) and the copyright owner(s) are credited and that the original publication in this journal is cited, in accordance with accepted academic practice. No use, distribution or reproduction is permitted which does not comply with these terms.
*Correspondence: Christina Röcke, ✉ christina.roecke@uzh.ch