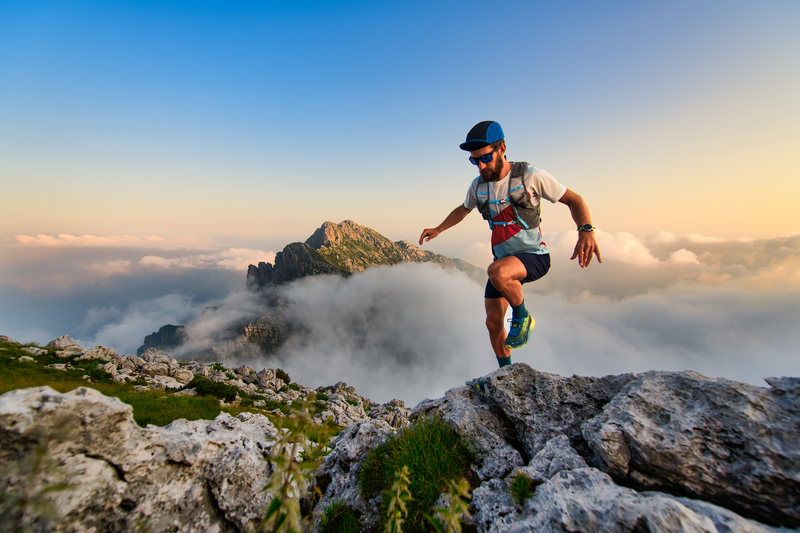
94% of researchers rate our articles as excellent or good
Learn more about the work of our research integrity team to safeguard the quality of each article we publish.
Find out more
ORIGINAL RESEARCH article
Front. Psychol. , 30 December 2021
Sec. Educational Psychology
Volume 12 - 2021 | https://doi.org/10.3389/fpsyg.2021.828027
A correction has been applied to this article in:
Corrigendum: Optimal experience in adult learning: Conception and validation of the flow in education scale (EduFlow-2)
While the formulation of Mihaly Csikszentmihalyi's theory of flow, including the experience dimensions, has remained stable since its introduction in 1975, its dedicated measurement tools, research methodologies, and fields of application, have evolved considerably. Among these, education stands out as one of the most active. In recent years, researchers have examined flow in the context of other theoretical constructs such as motivation. The resulting work in the field of education has led to the development of a new model for understanding flow experience in education, specifically dedicated to adult learning. As a result of both a meticulous analysis of existing models and consideration of more recent developments, a new flow scale has thus been developed. The aim of this study is therefore twofold: to validate the new flow measurement scale dedicated to the educational environment, EduFlow-2, and to test a new theoretical model. Students taking a course (N = 6,596), some on-site and others in a MOOC, participated. Several scales were administered online at the end of the participants' course during the 2017 academic year. The factor structure of EduFlow-2 was tested using Exploratory Structural Equation Modeling. Several models were tested. The model with a second-order factor best fit the data. We tested the invariance of the flow scale measure for gender and for the type of training (MOOC/on-site). We were able to show that the flow scale is invariant of the modalities of these two variables. Results revealed good psychometric qualities for the scale, making it suitable for both on-site and distance learning. The analysis also revealed significant relationships with the classic variables of motivation, self-efficacy, learning climate, and life satisfaction. Furthermore, all four dimensions of the model were found to be adequate and consistent with the underlying theoretical arguments. In the end, this new, short flow scale and the theoretical model were demonstrated to be promising for future studies in the field of education.
In January 1999, Ken Sheldon, Barbara Frederickson, Kevin Rathunde, and Mihaly Csikszentmihalyi came together for what was called the Akumal 1 Meeting in Akumal, Mexico, and produced the Positive Psychology Manifesto, which they revised during the Akumal 2 Meeting in January 2000. In the original version of the Manifesto, the authors laid the first foundations of this emerging field: The scientific study of optimal human functioning is to discover and to promote the factors that allow individuals and communities to thrive. In the revised version of the Manifesto, (Sheldon et al., 2000) stated that education is the first of the 6 core applications of positive psychology: “Improving child education by making greater use of intrinsic motivation, positive affect, and creativity within schools” (Sheldon et al., 2000, p. 1). However, we argue that this intention can be extended to lifelong learning, since the 4th core application mentioned in the Manifesto regarding working life is “Improving work satisfaction across the lifespan by helping people to find authentic involvement, experience states of flow, and make genuine contributions in their work” (Sheldon et al., 2000, p. 1). It is therefore not surprising that the volume Applications of Flow in Human Development and Education has a particularly important place in the Collected Works of Mihaly Csikszentmihalyi (Csikszentmihalyi, 2014a,b,c).
Flow theory is one of the most significant theories of contemporary psychology. Csikszentmihalyi's pioneering work began with his examinations of creativity during his doctoral research as early as 1965 (Csikszentmihalyi, 1996, 2014a). He expanded his inquiry to the psychological determinants of subjective experience, then later to what constitutes a good life, finally focusing on flow (Csikszentmihalyi, 1975, 1990, 2014b). For more than a quarter of a century, his work grew in influence beyond a small community of researchers. Martin Seligman, during his term as president of the American Psychological Association (APA) (1998–2000), emphasized the importance of Csikszentmihalyi's work, notably by describing him as “the world leader in positive psychology research.” In the year 2000, Csikszentmihalyi received Brain Channel's Thinker of the Year Award.
Since 1990, his book Flow: The Psychology of Optimal Experience, published in 1990, has been translated into 23 languages. Csikszentmihalyi has probably become one of the most-cited psychologists in a variety of fields, including psychology, sports, education, arts, management, video games, online learning, and many others.
With the aim of describing the growing interest in flow research in the new millennium, the European Flow-Researchers Network (EFRN) produced a scoping review (Peifer et al., 2021) following the steps proposed by Arksey and O'Malley (2005). The review included 258 quantitative and qualitative empirical studies published between 2000 and 2016. This review allowed us to correlate the themes identified in the review with the potential applications identified in the Positive Psychology Manifesto (Sheldon et al., 2000). The analysis classified “education” as the main topic, with 28.8% of the empirical studies published between 2000 and 2016 dedicated to this field of inquiry. Other topics representing at least 10% of published studies included arts/leisure (22.0%), health/psychotherapy/physiology (14.7 %), professional activities (11.6 %), physical activities/sport (11.0%). The works dedicated to education focused on pupils and education at the primary school level (8.2%), the secondary school/college level (20.4%), the continuing education level (69.4%), and teachers themselves (2.0%). Only 21.3% of education studies dealt with continuing vocational training/lifelong learning (vs. formal education, 78.7%).
Many researchers have also examined the link between flow experience and a wide range of motivational indicators: (a) engagement (e.g., Shernoff et al., 2003; Steele and Fullagar, 2009; Valenzuela and Codina, 2014; Mesurado et al., 2016), (b) goal orientation (e.g., Schüler et al., 2010; Oertig et al., 2014), (c) achievement motives (e.g., Engeser and Rheinberg, 2008; Busch et al., 2013), (d) interest (e.g., Bressler and Bodzin, 2013; Bachen et al., 2016), and (e) volition (e.g., Schattke, 2011). This is not surprising because many authors (e.g., Schüler et al., 2010; Schattke, 2011; Bassi and Delle Fave, 2012; Fulmer and Tulis, 2016) consider flow experience to represent a state of optimal motivation (Deci and Ryan, 2008; Heutte, 2019).
Flow in education has often been studied in combination with other theories. Many studies have examined the connection between flow and intrinsic motivation (Schüler et al., 2010; Keller et al., 2011; Valenzuela and Codina, 2014; Meyer et al., 2016). Flow is, in fact, often conceptualized as a theory of intrinsic motivation (Engeser and Rheinberg, 2008); however, studies have not yet reached consensus on whether or not intrinsic motivation is a necessary condition for flow in all contexts, or how the intrinsic motivation contributes to the dimensions of flow. The primary purpose of a meta-analysis of 28 studies (Fong et al., 2015) was to examine the relationship between Challenge-Skill Balance and flow, but the analysis also considered Challenge-Skill Balance and its possible relationship with intrinsic motivation. In the former, the correlation was moderate. In the latter, it was weaker still. Challenge-Skill Balance, combined with Clear Goals and Sense of Control, however, was a strong contributor to flow. Although the relationship between intrinsic motivation in the experience of flow and Deci and Ryan's (2008). Self-determination Theory, a prominent theory of intrinsic motivation, is not yet clearly established, Schattke (2011) did find that raising children in an environment that promotes self-determination helps them to engage in activities that will enhance flow experience.
Other studies on motivation and flow are linked to Social Cognitive Theory (SCT, Bandura, 2001). Results linking self-efficacy with flow frequency and higher levels of challenge and skill also show that self-efficacy predicts flow over time (Rodriguez-Sanchez et al., 2011; Heutte et al., 2016a). High levels of efficacy beliefs have a positive impact on flow experiences in academic settings (Salanova et al., 2006; Bassi et al., 2007; Heutte et al., 2016a). Various aspects of Bandura's (1986) self-regulation learning model were also shown to exert a significant and positive effect on flow state (Lee and LaRose, 2007; Rodriguez-Sanchez et al., 2011; Chen and Sun, 2016). Higher congruence between one's implicit motives and self-attributed motives is associated with better self-regulation, goal attainment and flow (Rheinberg and Engeser, 2012). Some studies highlight collective (or social) motivational conditions of flow and demonstrate that collective efficacy beliefs predict collective flow over time (Salanova et al., 2014).
To conclude this literature review, we can observe that various concepts raised by Deci and Ryan's SDT or Bandura's SCT seem to be excellent predictors of flow antecedents in educational contexts. Therefore, flow theory offers promising complementary perspectives for shedding more light on the psychological determinants of commitment and persistence in adult training and continuing education. It is for all these reasons that the perspectives concerning autotelic experience (well-being provided by the activity itself, rather than external rewards) in a lifelong learning context, although currently very little explored, seem particularly promising (Heutte, 2020).
Engeser et al. (2021) argued that the definition of flow has changed very little since Csikszentmihalyi's (1975/2000) original formulation in 1975, and that there is strong agreement among researchers on the definition itself. Yet, they pointed out that there is a certain level of disagreement among researchers regarding how flow should be measured: “Indeed, over the past 35 years, researchers have kept developing and validating new measurement tools for flow, and modifying and re-validating established ones, which indicates that a gold measurement standard for flow has yet to be achieved” (Moneta, 2021; pp. 31–32).
This apparent paradox is not uncommon in the history of psychology and can be understood by recognizing that the path from the theoretical definition to the operationalization of a construct goes through the intermediate process of modeling. Thus, various tools have long been used to study flow in educational contexts (Table 1). However, to our knowledge, before the development of the first Flow in Education Model (Heutte et al., 2014a), there was no short multidimensional scale designed specifically for education.
The Flow State Scale-2 (FSS-2) (Jackson and Eklund, 2002), measuring the nine conceptual dimensions of flow, is one of the most widely-used scales for studying optimal experience. However, Heutte et al. (2014b) were able to show that in an educational context, not all of the dimensions postulated by this scale were systematically captured by learners in self-report questionnaires. Thus, other studies, notably in French schools (Fenouillet et al., 2014) and in a MOOC (Massive Open Online Course), have made it possible to postulate a 4-dimensional structure (Heutte et al., 2016b).
The purpose of this study is, therefore, to propose the validation of a new scale dedicated to the educational environment for measuring flow. In accordance with the validation standards, several studies were carried out with a population of students at the INSPE (Institut National Supérieur du Professorat et de l'Education) of Lille (a Teacher Training Institute in the North of France) and in the Project Management MOOC (MOOC GdP) developed by a team of volunteers, startups and Centrale Lille (an engineering Grande École in North of France) in order to obtain an experimental version. Then, a series of analyses were carried out to explore its factorial structure and to confirm it. The construct validation was completed in a study of concurrent validity and correlates.
The sample (N = 6,596) had an age of 30.01 (Max = 69, Min = 18, SD = 9.41). Men (n = 2,947) and women (n = 3,225) (gender data missing for 397) were students at the INSPE of Lille (n = 3,232) and students enrolled in a MOOC (n = 4,264).
Multiple measures were used in the course of this study:
• EduFlow-2 (12 items) with four subscales including Cognitive control (FlowD1, three items), Immersion and Time transformation (FlowD2, three items), Loss of self-consciousness (FlowD3, three items) and Autotelic experience—well-being provided by the activity (FlowD4, three items).
• The Generalized self-efficacy scale (10 items) in academic activities (adapted from Schwarzer and Jerusalem, 1995; French of GSES, Heutte, 2011).
• Learning Climate Questionnaire [6-item French version of LCQ, Leroy et al., 2013; adapted from Williams and Deci (1996)].
• Satisfaction with Life Scale (5-item French version of SWLS, adapted from Diener et al., 1985; Blais et al., 1989).
• French Adult Education Motivation Scale (FAEMS, Fenouillet et al., 2015), with 24 items in six subscales, including Intrinsic Motivation to know (IMk, four items), four types of extrinsic motivation [i.e., integrated (MEinteg, four items), identified (MEident, four items), introjected (MEinteg, four items) and external (MEext, four items), and amotivation (AM, four items)].
During the phase of the study conducted at the INSPE of Lille, students were given a survey asking them to evaluate the quality of training, teaching, and administration [in French “évaluation de la qualité des formations, des enseignements et du fonctionnement” (EQFEF)] (Heutte and Ghouch, 2018). The procedure was described in a specific document: the EQFEF charter available on the Institute's website. At the time of administrative registration, in September 2016, information regarding the gender and age of all students was collected. At the end of the second semester of the academic year, in June 2017, the GSES, LCQ, SWLS and EduFlow-2 scales were completed. Consent of the participants was obtained at the beginning of the survey, after informing all students that they should read the EQFEF charter and that participation in the study was voluntary.
One week before the start of the MOOC in September 2016, information about the gender, age and status of the participants (full-time student or working person) was collected. At the end of the fourth week of the MOOC, the FAEMS and EduFlow-2 scales were completed.
All questionnaires were administered online on a server hosted and secured by the University of Lille (LimeSurvey Version 2.50+ Build 160620).
Descriptive statistics were performed with R 4.0.4 (package Psych 2.09) and Jamovi 1.2.27. Confirmatory analyses and structural equation modeling were performed with Mplus version 8.5.
To validate the factor structure of the EduFlow-2 scale, we used Exploratory Structural Equation Modeling (ESEM) as advocated by various authors (Asparouhov and Muthén, 2009; Marsh et al., 2013, 2014). To clearly establish the factor structure, while it is acceptable for items to be weakly correlated with all factors (λ <0.4), it is essential that the correlation is stronger with the factor that represents the underlying psychological construct.
Model fit was tested with the following indicators and their recommended cut-off values (Browne and Cudeck, 1992; Kahn, 2006; Worthington and Whittaker, 2006; Hooper et al., 2008): a comparative fit index (CFI) and the Tucker Lewis index (TLI) above.90; a Root Mean Squared Error of Approximation (RMSEA) below.08, with an upper confidence interval which should not exceed.08 (Hu and Bentler, 1999); a Standardized Root Mean Squared Residual (SRMR) below.08; a chi-square to degree of freedom ratio preferably under 3.
Indicators fit are all above the expected threshold even if the χ2 remains significant (χ2(24) = 227.072, p < 0.001; CFI = 0.99; TLI = 0.99; RMSEA = 0.036 [CI 90% 0.032 0.041]; SRMR = 0.007). Furthermore, the results of the correlations between factors and items are broadly in line with our expectations. No item correlated with more than one factor above 0.40 (Table 2). The three D1 items all have correlations above 0.40 on the first factor. It can, therefore, be stated that factor D1 represents cognitive control. The three D2 items have correlations above 0.40 only on the second factor, so this factor represents immersion. The three D3 items all correlate above 0.80 on factor 3, so it can be stated that this factor represents lack of concern about the self. Finally, the D4 items all have correlations above 0.70 on the fourth factor, so it can be stated that this factor represents autotelic experience (well-being provided by the activity itself). Finally, the internal consistency of each dimension is good since the omega coefficient is >0.70 for each of the four dimensions (Table 2).
Table 3 allows us to evaluate the relationships between the different dimensions of flow measured by our scale and other psychological dimensions measured by other scales. Overall, the correlations are in the expected direction. The SEGS (Self-efficacy in academic activities) LCQ (Learning Climate) and LSWS (Satisfaction with Life) scales all have positive and significant correlations with the four dimensions of the EduFlow-2 scale (Table 3). It also appears that the different dimensions of the French Adult Education Motivation Scale (FAEMS) do not show the same correlations with the EduFlow-2 dimensions. As expected, amotivation is negatively correlated with all EduFlow-2 dimensions. The two forms of controlled motivation, external regulation and introjected regulation, are also more weakly-correlated than are the three forms of self-determined motivation (identified regulation, integrated regulation and intrinsic motivation).
We also note that, although very weak, there are negative correlations between the EduFlow-2 dimension D3, Loss of self-consciousness, and identified regulation (r = −0.06, p < 0.001) and integrated regulation (r = −0.09, p < 0.001). These correlations, which run counter to our expectations, can be explained by the learning context in which these measures were taken, which does not favor social relations and therefore this dimension of flow.
We tested different relationships between the four dimensions of our scale that we identified earlier. The fit indicators of these models are compared to the fit of the measurement model (Figure 1) which is presented in Table 4.
With regard to the criteria stated above, the three models presented a correct fit to the data. However, if we compare the models with each other, it appears that the models do not all have the same fit indicators.
The flow condition and state model (Figure 2) is based on Kawabata and Mallett's (2011) model, which distinguishes flow conditions from flow state. From this perspective this model states that Flow D1 (Cognitive Control), which is considered a necessary precondition for flow in education, will have an effect on FlowD2 (Immersion and Time Transformation), FlowD3 (Loss of self-consciousness), and FlowD4 (Autotelic Experience), which together create the state of flow. As we can see (Table 4), this model fits the data but it is the one that deviates most from the measurement model.
The second order model (Figure 3) postulates the existence of a second order factor which would correspond to a general flow factor in education. Again this model fits the data correctly. However, the measurement model fits better than the other two. We can also see that the model with a second order factor is a better fit than the flow condition and state model (Table 4).
In order to assess whether responses of the scale differ by sample and differ by gender, we first tested the measurement invariance for these variables (Van de Schoot et al., 2012). In order to test the measurement invariance, we first tested the configurational invariance that allows us to establish the baseline measure for all groups. We then tested for metric invariance by constraining the coefficients of the latent factors to be equivalent across groups. We then proceeded to constrain the intercepts in addition to the coefficients of the latent factors, which corresponds to scalar invariance.
To test invariance, it was necessary to compare the different forms of invariance (configurational, metric, scalar) with each other. However, comparison methods based on the χ2 are sensitive to the sample size (Chen, 2007), so we relied on the difference between the fit indicators. Cheung and Rensvold (2002) recommended using a ΔCFI value >0.01 as a threshold for asserting a significant drop in fit between models. Chen (2007) also recommended using the ΔCFI, ΔRMSEA and ΔSRMR. To take these different recommendations into account, we considered the following thresholds for estimating measurement invariance, ΔCFI ≤ 0.01, ΔRMSEA ≤ 0.015 and ΔSRMR ≤ 0.03 for metric invariance and ΔCFI ≤ 0.01, ΔRMSEA ≤ 0.015 and ΔSRMR ≤ 0.01 for scalar invariance.
Table 5 summarizes the set of fit indicators for the configural, metric and scalar invariance for measurement invariance for the educational flow scale with respect to the sample (student/MOOC). As we can see, all the comparison indicators allow us to say that there is measurement invariance between flow in the MOOC and in the classroom. The same observation can be made when comparing men and women. The flow is invariant between men and women (Table 6).
At their core, the results reveal satisfactory psychometric qualities. Our results show that it was possible to identify a model that fist the data with an internal consistency in line with psychometric standards. Furthermore, the examination of the invariance of the measure confirmed that the scale could be used both in the context of a MOOC and in the classroom, as well as and regardless of gender. These different results attest to good psychometric qualities that will allow the scale to be used in future studies, both in classrooms and in MOOCs, and for both women and men. In other words, the model (Figure 2, Tables 2, 4–6) underpins the EduFlow-2 Scale construct. The EduFlow-2 Scale has three main advantages:
• It suits flow measurement in various educational contexts;
• It is a short tool, reducing respondent burden;
• It highlights the difference between four dimensions of flow that are strongly and significantly related to many psychological determinants of motivation and volition in learning contexts, such as self-efficacy in academic activities; Learning Climate; Satisfaction with Life scale; self-determination, including Intrinsic Motivation (Table 3).
Beyond validating the scale itself, the results focused our attention on the role played by Loss of self-consciousness (D3) in the development of the self and in the development of relationships with others, an intriguing relationship discussed in the following section.
While Loss of self-consciousness (D3) was strongly correlated with self-efficacy in Academic Activities and with Satisfaction with Life, and was negatively correlated with Amotivation, correlations with several motivation scales were weak, a finding that is not surprising given the mixed literature on both the influence of intrinsic motivation during flow and on the role that context plays in contributing to motivation during flow. Since Loss of self-consciousness has not been the focus of extensive empirical study, there remain under-explored issues relevant both to the EduFlow-2 and to flow research more generally.
A close reading of Csikszentmihalyi's theoretical discussions (Csikszentmihalyi, 1975, 1990, 1993), reveals his vision of not a single factor, but of a multidimensional and dynamic variable, potentially one contributing to the development of the Self and even to one's relationships with others. He enumerates several ways (Csikszentmihalyi, 1975), from the concrete and personal to the abstract and social, that the variable can be described: loss of ego, self-forgetfulness, transcendence of individuality, and fusion with the world (p. 42). Existing scales that measure individual flow (e.g., Jackson and Eklund, 2002; Heutte et al., 2016b) operationalize the variable as one that is concrete and personal. Psychic energy is so consumed by a challenging activity that no bits of attention remain for considering how an outside Other might be evaluating you.
Fidelity in measuring Loss of self-consciousness will improve following research that better delineates the dimension from Merging of Action and Awareness. While Loss of self-consciousness can happen without the outside Other, Csikszentmihalyi's descriptions sometimes blur the two dimensions, describing Loss of self-consciousness in Japanese motorcycle gang members who report feeling as if they are “one flesh” with other cyclists during a run (p. 63); surgeons who describe becoming “a single organism, moved by the same purpose” (p. 65), in a networked connection with others in the operating room; and climbers who develop a sense of kinship “between fingers and rock, between the frail body and the context of stone, sky, and wind” (Csikszentmihalyi, 1990, p. 64). Becoming part of a system greater than oneself elevates Loss of self-consciousness well beyond the concrete and personal.
Csikszentmihalyi's abstract and social view of Loss of self-consciousness—as transcendence of individuality and fusion with the world—advances the power of the construct radically beyond not feeling judged by the Other to feeling integrated with all Others. The perspective bears thematic similarity to Erikson's (1980) argument that adulthood brings the critical developmental crisis of developing Generativity (“establishing and guiding the next generation”) (p. 103) vs. Stagnation. Csikszentmihalyi's bold claim is that the Loss of self-consciousness grows more sophisticated as one's complexity increases. Flow theory holds that complexity increases incrementally each time a person experiences flow.
Flow measurement has established (Procci et al., 2012) that context and variations in activities can play a significant role in correlations between factors. Heutte et al. (2014b) noted that several dimensions are not experienced at measurable levels by learners in formal educational settings. For example, because students in a MOOC work with limited interaction, and often independently, there is little opportunity for a Loss of self-consciousness since there is no Other to judge them while doing their work. In contrast, high school students actively engaged in experiential learning environments do report a Loss of self-consciousness (Gute and Csikszentmihalyi, 2018).
Csikszentmihalyi's bold and optimistic theoretical foundation for Loss of self-consciousness has set the stage for necessary and significant empirical research that will greatly aid the measurement of flow in learning, particularly of adult learners. As a next step, we recommend that Loss of self-consciousness should become a focus in additional research, in order to build on this study by investigating the experience in a range of learning contexts.
EduFlow-2 is a short multidimensional scale specifically designed for education and for allowing repeated measures over time. Self-reporting measures offer several fundamental strengths in measuring the latent variable of interest: e.g. high construct validity, ease of interpretation, inexpensive and relatively quick administration (Kline, 1993). For these reasons, self-reports are often used as the gold standard for the latent variable under investigation, flow in this context. Yet, next to these strengths, self-reports are also prone to some fundamental limitations. Self-reporting requires participants to remember and assess their own emotional/motivational state, which depends on the person's emotional intelligence. Pirsoul et al. (2019) claimed that participants cannot capture their unconscious emotions. Self-reporting scores may also be biased because of the way in which the question is specified, or the way in which the answering options are formulated. Although Pekrun (2020) argued that self-report is indispensable for a nuanced assessment of mental states, he stressed that self-reports can be influenced by social desirability and can also be influenced by culture, an effect that is mainly attributed to differences in semantic understanding.
Next to the aforementioned fundamental limitations, self-reports are also prone to certain more practical limitations. For instance, the traditional approach of using self-reports to directly measure latent variables has a limited temporal resolution, as it is not possible to survey participants on a permanent basis. It is also not possible to automate self-reports, as the involvements of participants in self-reports is obviously necessary. Furthermore, when self-reports are collected during learning, participants' activities need to be interrupted, which can affect natural behavior and learning.
The combination of these practical limitations, together with the emergence of new monitoring technologies, has motivated innovative research projects to explore the possibility of directly measuring manifest variables that could serve as proxies for the latent variable of interest.
Several new technologies enable capture of new types of data: behavior within online learning environments or MOOCs can be tracked by logging clickstream data (e.g., Coussement et al., 2020), images can be analyzed by applying computer vision techniques on video-recordings (e.g., Raca et al., 2014; Vanneste et al., 2021), psycho-physiological data can be measured via wearable devices (e.g., Vanneste et al., 2020), and eye-measures can be monitored by eye-tracking and pupillometry (Van Acker et al., 2020).
These new tools not only provide opportunities to measure emotional/affective engagement (including flow), in real-time, they also creates opportunities to investigate optimal learning environments (Shernoff and Csikszentmihalyi, 2009). While MOOCs are remarkable environments for the development and validation of measurement tools from a methodological standpoint, they are not well-suited for the study of the social dimensions of learning. In these massive multi-learner online environments, learners are very often massively alone. MOOCs are therefore not the most suitable environments to study the optimal learning environment from the social-conative perspective (Heutte, 2017, 2019, 2021). In future research, we hope to investigate flow as one of the important factors within authentic learning settings—ecosystems in which learning happens in interaction with the content, the tools, the peers, the space, and the teacher. We aim to go beyond current work by defining and assessing quality of teaching and learning in terms of measurable multimodal indicators based on behavior, log data, eye tracking data, audio-visual data and sensor data from activities within the learning spaces. This means that we will not only focus on learning analytics, but also on modeling the skills developed by the learners using educational software. It has been stressed in the current educational literature (e.g., Prieto et al., 2016; Martinez-Maldonado et al., 2021) that we should broaden the scope of analytics, modeling not only the learners' interactions with digital tools, but also anything that may happen in this specific ecosystem called a classroom or learning space (Dillenbourg, 2021).
Our results revealed good psychometric qualities of the EduFlow-2 scale make it suitable for both on-site and distance learning. They also revealed significant relationships with the classic variables of motivation, self-efficacy, learning climate, and life satisfaction. Furthermore, all four dimensions of the model were found to be adequate and consistent with the underlying theoretical arguments. In the end, this new short, multidimensional flow scale and the theoretical model behind it will thus be very useful for the study of optimal learning experience in many contexts, in particular for longitudinal researchers wishing to study the interaction effects between flow and other theoretical concepts that may support lifelong learning.
The raw data supporting the conclusions of this article will be made available by the authors, without undue reservation.
Ethical review and approval was not required for the study on human participants in accordance with the local legislation and institutional requirements. The patients/participants provided their written informed consent to participate in this study.
JH, FF, CM-K, MC, and RB contributed to the conception and design of the study. RB organized the database. FF carried out the statistical analysis. JH, FF, CM-K, and MC wrote the first draft of the manuscript. GG, AR, RB, and DG wrote sections of the manuscript. All authors contributed to the revision of the manuscript, read, and approved the submitted version.
This research is dedicated to the memory of Mihaly Csikszentmihalyi, who provided inspiration and collaboration in the development of this study.
This project was supported by the French Government through the Programme Investissement d'Avenir (I-SITE ULNE / ANR-16-IDEX-0004 ULNE) managed by the Agence Nationale de la Recherche.
The authors declare that the research was conducted in the absence of any commercial or financial relationships that could be construed as a potential conflict of interest.
All claims expressed in this article are solely those of the authors and do not necessarily represent those of their affiliated organizations, or those of the publisher, the editors and the reviewers. Any product that may be evaluated in this article, or claim that may be made by its manufacturer, is not guaranteed or endorsed by the publisher.
The Supplementary Material for this article can be found online at: https://www.frontiersin.org/articles/10.3389/fpsyg.2021.828027/full#supplementary-material
Arksey, H., and O'Malley, L. (2005). Scoping studies: towards a methodological framework. Int. J. Soc. Res. Methodol. 8, 19–32. doi: 10.1080/1364557032000119616
Asparouhov, T., and Muthén, B. (2009). Exploratory structural equation modeling. Struct. Equ. Modeling. 16(3), 397–438. doi: 10.1080/10705510903008204
Bachen, C. M., Hernández-Ramos, P., Raphael, C., and Waldron, A. (2016). How do presence, flow, and character identification affect players' empathy and interest in learning from a serious computer game? Comput. Hum. Behav. 64, 77–87. doi: 10.1016/j.chb.2016.06.043
Bakker, A. D.. (2008). The work-related flow inventory: Construction and initial validation of the WOLF. J. Vocat. Behav. 72, 400–414. doi: 10.1016/j.jvb.2007.11.007
Bandura, A.. (2001). Social cognitive theory: An Agentic Perspective. Annu. Rev. Psychol. 52, 1–26. doi: 10.1146/annurev.psych.52.1.1
Bassi, M., and Delle Fave, A. (2012). Optimal experience among teachers: new insights into the work paradox. J. Psychol. 146, 533–557. doi: 10.1080/00223980.2012.656156
Bassi, M., Steca, P., Delle Fave, A., and Caprara, G. V. (2007). Academic self-efficacy beliefs and quality of experience in learning. J. Youth Adolesc. 36, 301–312. doi: 10.1007/s10964-006-9069-y
Blais, M. R., Vallerand, R. J., Pelletier, L. G., and Brière, N. M. (1989). L'échelle de satisfaction de vie: Validation canadienne-française du “Satisfaction with Life Scale.” Can. J. Behav. Sci. 21, 210. doi: 10.1037/h0079854
Bressler, D. M., and Bodzin, A. M. (2013). A mixed methods assessment of students' flow experiences during a mobile augmented reality science game. J. Comput. Assist. Learn. 29, 505–517. doi: 10.1111/jcal.12008
Browne, M. W., and Cudeck, R. (1992). Alternative Ways of Assessing Model Fit. Sociol Methods Res. 21, 230–258. doi: 10.1177/0049124192021002005
Busch, H., Hofer, J., Chasiotis, A., and Campus, D. (2013). The achievement flow motive as an element of the autotelic personality: predicting educational attainment in three cultures. Eur. J. Psychol. Educ. 28, 239–254. doi: 10.1007/s10212-012-0112-y
Chen, F. F.. (2007). Sensitivity of goodness of fit indexes to lack of measurement invariance. Struct. Equ. Model. 14, 464–504. doi: 10.1080/10705510701301834
Chen, L. X., and Sun, C. T. (2016). Self-regulation influence on game play flow state. Comput. Hum. Behav. 54, 341–350. doi: 10.1016/j.chb.2015.08.020
Cheung, G. W., and Rensvold, R. B. (2002). Evaluating goodness-of-fit indexes for testing measurement invariance. Struct. Equ. Model. 9, 233–255. doi: 10.1207/S15328007SEM0902_5
Coussement, K., Phan, M., De Caigny, A., Benoit, D. F., and Raes, A. (2020). Predicting student dropout in subscription-based online learning environments: The beneficial impact of the logit leaf model. Decis. Support Syst. 135, 113325. doi: 10.1016/j.dss.2020.113325
Csikszentmihalyi, M.. (1996). Creativity: Flow and the psychology of discovery and invention. New York, NY: HarperPerennial.
Csikszentmihalyi, M.. (2014a). The systems model of creativity. Dordrecht: Springer. doi: 10.1007/978-94-017-9085-7
Csikszentmihalyi, M.. (2014b). Flow and the foundations of positive psychology. Dordrecht: Springer. doi: 10.1007/978-94-017-9088-8
Csikszentmihalyi, M.. (2014c). Applications of flow in human development and education. Dordrecht: Springer. doi: 10.1007/978-94-017-9094-9
Csikszentmihalyi, M., and Csikszentmihalyi, I. S. (1992). Optimal Experience: Psychological Studies of Flow in Consciousness. Cambridge: Cambridge University Press.
Csikszentmihalyi, M.. (1990). Flow: The psychology of optimal experience. New York, NY: Harper and Row.
Deci, E. L., and Ryan, R. M. (2008). Facilitating optimal motivation and psychological well-being across life's domains. Can. Psychol. 49, 14. doi: 10.1037/0708-5591.49.1.14
Diener, E., Emmons, R. A., Larsen, R. J., and Griffin, S. (1985). The satisfaction with life scale. J. Pers. Assess. 49, 71–76. doi: 10.1207/s15327752jpa4901_13
Dillenbourg, P.. (2021). Classroom analytics: Zooming out from a pupil to a classroom. In OECD (Ed.), Digital education outlook 2021: Pushing the frontiers with AI, blockchain, and robots. OECD Publishing p. 105–118. doi: 10.1787/336f4ebf-en
Engeser, S., and Rheinberg, F. (2008). Flow, moderators of challenge-skill-balance and performance. Motiv. Emot. 32, 158–172. doi: 10.1007/s11031-008-9102-4
Engeser, S., Schiepe-Tiska, A., and Peifer, C. (2021). Historical lines and an overview of current research on flow. Adv. Flow Res. 1–29. doi: 10.1007/978-3-030-53468-4_1
Fenouillet, F., Heutte, J., and Vallerand, R.-J. (2015). Validation of the adult education motivation scale. Orlando, FL: 4th World Congress on Positive Psychology (IPPA).
Fenouillet, F., Martin-Krumm, C., Heutte, J., and Besançon, M. (2014). An urgent call for change: Flow, motivation and well-being in French School students. Amsterdam, the Netherlands: 7th European Conference on Positive Psychology (ECPP).
Fong, C. J., Zaleski, D. J., and Leach, J. K. (2015). The challenge–skill balance and antecedents of flow: A meta-analytic investigation. J. Posit. Psychol. 10, 425–446. doi: 10.1080/17439760.2014.967799
Fu, F. L., Su, R. C., and Yu, S. C. (2009). EGameFlow: A scale to measure learners' enjoyment of e-learning games. Comput. Educ. 52, 101–112. doi: 10.1016/j.compedu.2008.07.004
Fulmer, S. M., and Tulis, M. (2016). Adding nuance to the challenge-skill relationship: The interaction of perceived and actual skill. Int. J. Educ. Res. 77, 143–154 doi: 10.1016/j.ijer.2016.04.002
Ghani, J. A., and Deshpande, S. P. (1994). Task characteristics and the experience of optimal flow in human—computer interaction. J. Psychol. 128, 381–391. doi: 10.1080/00223980.1994.9712742
Gute, G. Csikszentmihalyi (2018). Experience sampling comparison of engagement, mood, and purpose: High school students in a traditional school environment vs. students in an initiative-based, learner-centered environment. Presentation at the 9th European Conference on Positive Psychology, Education Division “Engaging Education,” Budapest, Hungary.
Heutte, J.. (2011). La part du collectif dans la motivation et son impact sur le bien-être comme médiateur de la réussite des étudiants: complémentarités et contributions entre l'autodétermination, l'auto-efficacité et l'autotélisme (Unpublished doctoral thesis). Université Paris Ouest-Nanterre-La Défense, Nanterre. Récupéré de https://tel.archives-ouvertes.fr/tel-00933690/
Heutte, J.. (2017). Motivations, volition et expérience du flow: quelques références théoriques pour l'étude des communautés d'apprenance. In O. Las Vergnas (dir.), Le e-learning informel? Des apprentissages diffus, noyés dans la participation en ligne. Paris, France: Éditions des archives contemporaines. p. 199–214.
Heutte, J.. (2019). Les fondements de l'éducation positive: Perspective psychosociale et systémique de l'apprentissage. Dunod.
Heutte, J.. (2020). Psychologie positive et formation des adultes: le flow ou le plaisir de comprendre tout au long de la vie. Savoirs. 3, 17–61. doi: 10.3917/savo.054.0017
Heutte, J.. (2021). L'expérience autotélique dans les EIAH: genèse socio-historique, épistémologique et critique de l'émergence des technologies positives pour l'apprentissage. Sciences et Technologies de l'Information et de la Communication pour l'Éducation et la Formation (STICEF). 27, 14–82.
Heutte, J., Fenouillet, F., Boniwell, I., Martin-Krumm, C., and Csikszentmihalyi, M. (2014a). Optimal learning experience in digital environments: theoretical concepts, measure and modelisation. Proceedings of Symposium Digital Learning in 21st Century Universities. Atlanta, GA: Georgia Institute of Technology.
Heutte, J., Fenouillet, F., Kaplan, J., Martin-Krumm, C., and Bachelet, R. (2016a). The EduFlow model - A contribution toward the study of optimal learning environments. In, L. Harmat, F. Ø. Andersen, F. Ullén, J. Wright & G. Sadlo (dir.). Flow Experience: Empirical Research and Applications. Cham, Suisse: Springer p. 124–143. doi: 10.1007/978-3-319-28634-1_9
Heutte, J., Fenouillet, F., Martin-Krumm, C., Boniwell, I., and Csikszentmihalyi, M. (2016b). Proposal for a conceptual evolution of the flow in education (EduFlow) model. Proceedings of 8th European Conference on Positive Psychology (ECPP 2016), Angers, France.
Heutte, J., Galaup, M., Lelardeux, C., Lagarrigue, P., and Fenouillet, F. (2014b). Etude des déterminants psychologiques de la persistance dans l'usage d'un jeux sérieux: évaluation de l'environnement optimal d'apprentissage avec Mecagenius. STICEF (Sciences et Technologies de l'Information et de la Communication pour l'Éducation et la Formation). 21, 519–552. doi: 10.3406/stice.2014.1110
Heutte, J., and Ghouch, F. (2018), Evaluation des formations MEEF par les étudiants de l'ESPE Lille Nord de France, Rapport de l'Evaluation de la Qualité des Formations, des Enseignements et du Fonctionnement des processus et des dispositifs (EQFEF) de l'ESPE-LNF.
Hooper, D., Coughlan, J., and Mullen, M. (2008). Structural equation modelling: Guidelines for determining model fit. Electronic Journal of Business Research Methods 6, 53–60.
Hu, L., and Bentler, P. M. (1999). Cutoff criteria for fit indexes in covariance structure analysis: Conventional criteria versus new alternatives. Struct. Equ. Model. 6, 1–55. doi: 10.1080/10705519909540118
Jackson, S. A., and Eklund, R. C. (2002). Assessing flow in physical activity: The Flow State Scale-2 and Dispositional Flow State Scale-2. J Sport Exerc Psychol. 24, 133–115. doi: 10.1123/jsep.24.2.133
Jackson, S. A., and Marsh, H. W. (1996). Development and validation of a scale to measure optimal experience: The Flow State Scale. J Sport Exerc Psychol. 18, 17–35. doi: 10.1123/jsep.18.1.17
Kahn, J. H.. (2006). Factor analysis in counseling psychology research, training, and practice: principles, advances, and applications. Couns. Psychol. 34, 684–718. doi: 10.1177/0011000006286347
Kawabata, M., and Mallett, C. J. (2011). Flow experience in physical activity: Examination of the internal structure of flow from a process-related perspective. Motivat. Emot. 35, 393–402. doi: 10.1007/s11031-011-9221-1
Keller, J., Ringelhan, S., and Blomann, F. (2011). Does skills-demands compatibility result in intrinsic motivation? Experimental test of a basic notion proposed in the theory of flow-experiences. J. Posit. Psychol. 6, 408–417. doi: 10.1080/17439760.2011.604041
Lee, D., and LaRose, R. (2007). A socio-cognitive model of video game usage. J Broadcast Electron Media. 51, 632–650. doi: 10.1080/08838150701626511
Leroy, N., Bressoux, P., Sarrazin, P., and Trouilloud, D. (2013). Un modèle sociocognitif des apprentissages scolaires: style motivationnel de l'enseignant, soutien perçu des élèves et processus motivationnels. Revue française de pédagogie 182, 71–92. doi: 10.4000/rfp.4008
Marsh, H. W., Morin, A. J. S., Parker, P. D., and Kaur, G. (2014). Exploratory structural equation modeling: An integration of the best features of exploratory and confirmatory factor analysis. Annu. Rev. Clin. Psychol. 10, 85–110. doi: 10.1146/annurev-clinpsy-032813-153700
Marsh, H. W., Vallerand, R. J., Lafrenière, M.-A. K., Parker, P., Morin, A. J. S., et al. (2013). Passion: does one scale fit all? Construct validity of two-factor passion scale andpsychometric invariance over different activities and languages. Psychological Assessment. 25, 796–809. doi: 10.1037/a0032573
Martinez-Maldonado, R., Yan, L., Deppeler, J., Phillips, M., and Gašević, D. (2021). Classroom Analytics: Telling Stories about Learning Spaces using Sensor Data. In E. Gil, Y. Mor, Y. Dimitriadis & C. Köppe (Eds.), Hybrid Learning Spaces. Cham, Switzerland: Springer (to appear).
Mayer, P.. (1978). Flow in adolescence and its relation to school experience. Unpublished doctoral dissertation. University of Chicago.
Mesurado, B., Richaud, M. C., and Mateo, N. J. (2016). Engagement, Flow, Self-Efficacy, and Eustress of University Students: A Cross-National Comparison Between the Phillipines and Argentina. J. Psychol. 150, 281–299. doi: 10.1080/00223980.2015.1024595
Meyer, A., Klingenberg, K., and Wilde, M. (2016). The Benefits of Mouse Keeping – an empirical study on students' flow and intrinsic motivation in biology lessons. Res. Sci. Edu. 46, 79–90. doi: 10.1007/s11165-014-9455-5
Moneta, G. B.. (2021). On the conceptualization and measurement of flow. In Advances in flow research. Springer, Cham p. 31–69. doi: 10.1007/978-3-030-53468-4_2
Novak, T. P., Hoffman, D. L., and Yung, Y. F. (2000). Measuring the flow construct in online environments: A structural modeling approach. Market. Sci. 19, 22–42. doi: 10.1287/mksc.19.1.22.15184
Oertig, D., Schüler, J, Brandstätter, V., and Augustine, A. (2014). The influence of avoidance-based achievement goals on flow. J. Personal. 82, 171–181. doi: 10.1111/jopy.12043
Peifer, C., Wolters, G., Harmat, L., Heutte, J., Tan, J., et al. (2021). A Scoping Review of Flow Research. Front. Psychol. 815665.
Pekrun, R.. (2020). Self-report is indispensable to assess students' learning. Front. Learn. Res. 8, 185–193. doi: 10.14786/flr.v8i3.637
Pirsoul T. Parmentier and M. Nils F. (2019). The rocky road to emotion measurement in learning and career development: on the use of self-reports. 18th Biennial EARLI Aachen, Germany: Conference for Research on Learning and Instruction. Available online at: http://hdl.handle.net/2078.1/218801
Prieto, L. P., Sharma, K., Dillenbourg, P., and Jesús, M. (2016). Teaching analytics: towards automatic extraction of orchestration graphs using wearable sensors. In Proceedings of the Sixth International Conference on Learning Analytics & Knowledge (LAK '16). Association for Computing Machinery, New York, NY, USA. p. 148–157. doi: 10.1145/2883851.2883927
Procci, K., Singer, A. R., Levy, K. R., and Bowers, C. (2012). Measuring the flow experience of gamers: An evaluation of the DFS-2. Comput. Hum. Behav. 28, 2306–2312. doi: 10.1016/j.chb.2012.06.039
Raca, M., Tormey, R., and Dillenbourg, P. (2014). Sleepers' lag - study on motion and attention. Proceedings of the Fourth International Conference on Learning Analytics And Knowledge. p. 36–43. doi: 10.1145/2567574.2567581
Rheinberg, F., and Engeser, S. (2012). Motivational competence: The joint effect of implicit and explicit motives on self-regulation and flow experience. In D. Leontiev (Dir.) Motivation, consciousness, and self-regulation. New York, NY: Nova Science Publishers. p. 79–87.
Rheinberg, F., Vollmeyer, R., and Engeser, S. (2003). Die Erfassung des Flow-Erlebens [The assessment of flow]. Diagnostik von Motivation und Selbstkonzept p. 261–279.
Rodriguez-Sanchez, A. M., Salanova, M., Cifre, E., and Schaufeli, W. B. (2011). When good is good: A virtuous circle of self-efficacy and flow at work among teachers. Revista de Psicologia Social. 26, 427–441. doi: 10.1174/021347411797361257
Salanova, M., Bakker, A. B., and Llorens, S. (2006). Flow at work: evidence for an upward spiral of personal and organizational resources*. J. Happiness Stud. 7, 1–22. doi: 10.1007/s10902-005-8854-8
Salanova, M., Rodriguez-Sanchez, A. M., Schaufeli, W. B., and Cifre, E. (2014). Flowing together: a longitudinal study of collective efficacy and collective flow among workgroups. J. Psychol. 148, 435–455. doi: 10.1080/00223980.2013.806290
Schattke, K. P.. (2011). Flow Experience as Consequence and Self-Determination as Antecedence of Congruence Between Implicit and Explicit Motives. Available online at: https://mediatum.ub.tum.de/doc/1078244/1078244.pdf
Schüler, J., Sheldon, K. M., and Fröhlich, S. M. (2010). Implicit need for achievement moderates the relationship between competence need satisfaction and subsequent motivation. J. Res. Pers. 44, 1–12. doi: 10.1016/j.jrp.2009.09.002
Schwarzer, R., and Jerusalem, M. (1995). “Optimistic self-beliefs as a resource factor in coping with stress,” in Extreme Stress and Communities: Impact and Intervention (Dordrecht: Springer), 159–177.
Sheldon, K. M., Fredrickson, B., Rathunde, K., Haidt, J., and Csikszentmihalyi, M. (2000). “Positive psychology manifesto,” in Manifesto Presented at Akumal 1 Conference and Revised During the Akumal 2 Meeting. Available online at: https://ppc.sas.upenn.edu/sites/default/files/Positive%20Psychology%20Manifesto.docx
Shernoff, D. J., and Csikszentmihalyi, M. (2009). Cultivating engaged learners and optimal learning environments. Handb. Positive Psychol. Sch. 131:145
Shernoff, D. J., Csikszentmihalyi, M., Schneider, B., and Shernoff, E. S. (2003). Student engagement in high school classrooms from the perspective of flow theory. Sch. Psychol. Q. 18, 158–176. doi: 10.1521/scpq.18.2.158.21860
Steele, J. P., and Fullagar, C. J. (2009). Facilitators and outcomes of student engagement in a college setting. J. Psychol. 143, 5–27. doi: 10.3200/JRLP.143.1.5-27
Valenzuela, R., and Codina, N. (2014). Habitus and flow in primary school musical practice: relations between family musical cultural capital, optimal experience and music participation. Music Edu. Res. 16, 505–520. doi: 10.1080/14613808.2013.859660
Van Acker, B. B., Bombeke, K., Durnez, W., Parmentier, D. D., Mateus, J. C., Biondi, A., Saldien, J., and Vlerick, P. (2020). Mobile pupillometry in manual assembly: A pilot study exploring the wearability and external validity of a renowned mental workload lab measure. Int. J. Ind. Ergon. 75. doi: 10.1016/j.ergon.2019.102891
Van de Schoot, R., Lugtig, P., and Hox, J. (2012). A checklist for testing measurement invariance. Eur. J. Dev. Psychol. 9, 486–492. doi: 10.1080/17405629.2012.686740
Vanneste, P., Oramas, J., Verelst, T., Tuytelaars, T., Raes, A., and Depaepe, F. (2021). Computer vision and human behaviour, emotion and cognition detection: A use case on student engagement. Mathematics. 9, 287. doi: 10.3390/math9030287
Vanneste, P., Raes, A., Morton, J., Bombeke, K., Bram, B., Van, A., et al. (2020). Towards measuring cognitive load through multimodal physiological data. Cognition, Technology & Work. 23, 567–585. doi: 10.1007/s10111-020-00641-0
Williams, G. C., and Deci, E. L. (1996). Internalization of biopsychosocial values by medical students: a test of self-determination theory. J Pers. Soc. Psychol. 70, 767. doi: 10.1037/0022-3514.70.4.767
Keywords: flow, optimal experience, autotelic experience, loss of self-consciousness, adult learning, adult education, motivation, well-being
Citation: Heutte J, Fenouillet F, Martin-Krumm C, Gute G, Raes A, Gute D, Bachelet R and Csikszentmihalyi M (2021) Optimal Experience in Adult Learning: Conception and Validation of the Flow in Education Scale (EduFlow-2). Front. Psychol. 12:828027. doi: 10.3389/fpsyg.2021.828027
Received: 03 December 2021; Accepted: 17 December 2021;
Published: 30 December 2021.
Edited by:
Majid Elahi Shirvan, University of Bojnord, IranReviewed by:
Tahereh Taherian, Yazd University, IranCopyright © 2021 Heutte, Fenouillet, Martin-Krumm, Gute, Raes, Gute, Bachelet and Csikszentmihalyi. This is an open-access article distributed under the terms of the Creative Commons Attribution License (CC BY). The use, distribution or reproduction in other forums is permitted, provided the original author(s) and the copyright owner(s) are credited and that the original publication in this journal is cited, in accordance with accepted academic practice. No use, distribution or reproduction is permitted which does not comply with these terms.
*Correspondence: Jean Heutte, amVhbi5oZXV0dGVAdW5pdi1saWxsZS5mcg==
Disclaimer: All claims expressed in this article are solely those of the authors and do not necessarily represent those of their affiliated organizations, or those of the publisher, the editors and the reviewers. Any product that may be evaluated in this article or claim that may be made by its manufacturer is not guaranteed or endorsed by the publisher.
Research integrity at Frontiers
Learn more about the work of our research integrity team to safeguard the quality of each article we publish.