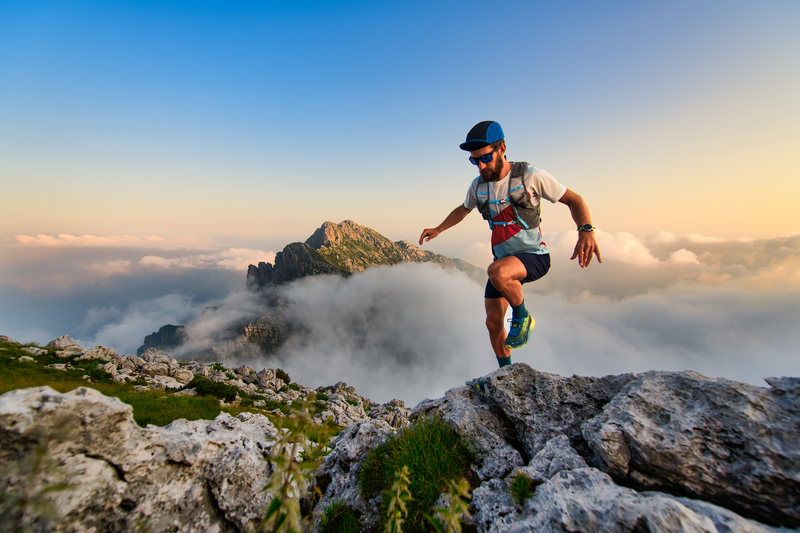
94% of researchers rate our articles as excellent or good
Learn more about the work of our research integrity team to safeguard the quality of each article we publish.
Find out more
ORIGINAL RESEARCH article
Front. Psychol. , 13 September 2021
Sec. Environmental Psychology
Volume 12 - 2021 | https://doi.org/10.3389/fpsyg.2021.743306
This article is part of the Research Topic Effects of Economic Shocks on Human Behavior, Mental Life and the Environment: Implications for the Post- COVID-19 Crisis Era View all 19 articles
In a DID model, this study examines the impact of the coronavirus disease 2019 (COVID-19) pandemic on the investor sentiment in the financial market of China using monthly panel data on newly listed Chinese companies between October 2019 and June 2020. The outbreak of the pandemic is shown to exert a significant negative impact on investor sentiment. A future industry heterogeneity analysis shows that the pandemic has driven up investor sentiment in the pharmaceutical sector while having a significantly negative impact on non-pharmaceutical sectors. The pandemic is shown to have a negative impact on the private sector and foreign-invested sector in China while a significantly positive impact on the state-owned sector. This study contributes to the existing literature on the investigation of how significant the impact of public health emergencies on investor sentiment is.
The 2020 outbreak of the coronavirus disease 2019 (COVID-19) pandemic, a global public health crisis, has hit the Chinese economy and the world economy hard. According to the WHO statistics, the pandemic has swept across more than 200 countries, causing a total of 83 million people worldwide with confirmed infection while killing over 1.8 million people.
The impact of the COVID-19 pandemic on the financial market of China is substantial. Shanghai Composite dipped by around 7.7%, a 5-year single-day low. At the same time, CSI Aggregate Bond rose by about 0.62%, a second decade-high. The forex market and currency market are also hit to various extents. Investor sentiment can reflect the general market trend and market vitality (Yu et al., 2020). Then, does the pandemic exert an impact on investor sentiment? If the answer is yes, then how should we measure the impact? Does investor sentiment vary across firm types and industries? How should China respond to the change? The answers to the above questions may help policymakers take measures to stabilize the financial market and formulate solutions to financial market turmoil. However, there is a minimal existing research literature on the impact of major public health events on investor sentiment. This study is intended to contribute to the understanding of this issue by looking at the impact of the COVID-19 pandemic on investor sentiment in the financial market of China.
This study uses a difference-in-difference (DID) model to study the impact of the COVID-19 pandemic on investor sentiment in the financial market of China. The improved turnover rate is used to measure the investor sentiment on individual stocks. This study focuses on the following variables: trading volume, free float equity, market value, financial indicators, etc., trying to study the impact of major economic shocks on investor sentiment in theA-share market of China. This study has important policy value for economic policies in responding to major public health incidents, stabilizing the A-share market, and safeguarding economic development in China.
The rest of the study is roughly organized as follows. The “Literature review” section reviews the previous studies. The “Model, variable selection, and data sources” section explains the model, variable, and data. The “Empirical analysis and findings” section provides the details of the empirical analyses. The “Conclusion” section concludes.
Behavioral finance theories imply a considerable impact of the psychological factors of the investor on the financial market. Existing approaches to measuring investor sentiment include direct proxy, indirect proxy, and text mining. In direct proxy, Brown and Cliff (2005) used a questionnaire survey to understand the outlooks of the investor on market trends. Kenneth and Meir (2000) categorized the investors into three subcategories, namely, large investors, medium-size investors, and small investors. Jiang et al. (2021) regarded the Baidu index as an indicator of investor sentiment and believed that investor sentiment is usually affected by the information provided by the Baidu search engine, which may cause stock prices to fluctuate. As single-indicator-based measurement may be subjected to bias, some scholars constructed an index system to measure investor sentiment. Baker and Wurgler (2006) and Yi and Mao (2009) cited six independent proxy indices to construct the investor sentiment composite index based on principal component analysis.
Individual investor sentiment is measured primarily based on big data analysis methods as data analysis and text mining. Baker and Stein (2004) pointed out that liquidity measured by turnover rate can be an indicator of investor sentiment. Das and Chen (2008) proposed a method of gauging the sentiment of small investors from a web-based message board by comparing the optimistic and pessimistic views. Balke et al. (2017) and Zhang (2019) provided methods to measure sentiment based on surveys and government policies. Yang et al. (2016) used big data mining to measure the investor sentiment index using big data mining from around 900,000 posts issued by listed companies on the website of Eastmoney Securities. Fang et al. (2020) used the data from Baidu, the leading search engine in China, to construct an indicator of investor sentiment for forecasting of returns of the Chinese stock market.
The existing literature also investigates how investor sentiment exerts an impact on the stock market and what factors drive investor sentiment (Delong et al., 1990; Fisher and Statman, 2000). In studying the correlation of asset pricing with investor sentiment, Brown and Cliff (2005) suggested the latter indicator has an impact on the former, i.e., investor sentiment change leads to stock fluctuation. Based on the statistics of the Chinese stock market, Xie and Tang (2021) concluded that positive investor sentiment can drive up the yield rate to some degree and this impact can last for around a year. Gozgor et al. (2019) pointed out that economic policy uncertainty affects gold returns, which in turn affects investor behavior and investor sentiment. During periods of high economic policy uncertainty, especially during the early 2020s and the COVID-19 pandemic, economic policy uncertainty exerts a considerable impact on the financial stock market and affects investment returns (Wu et al., 2021). Li et al. (2017) and Zhang et al. (2021) constructed investment sentiment indicators and analyzed the impact of external shocks on the sentiment of Chinese investors.
Since the twenty-first century, there have been five grave pandemics of infectious diseases defined by WHO as major public health events on a global scale or higher. Many scholars have assessed the impact of these events on the growth of the economy as a whole (Brainerd and Sieglar, 2003; Wu, 2003; Hanna and Huang, 2006). Barro et al. (2020), based on the death toll during the 1918–1929 influenza pandemic and the death toll in World War I, estimated the death toll of the COVID-19 pandemic and its economic impact, finding the severely impacted countries registered a 6% drop and an 8% drop in GDP and consumption, respectively. The outbreak of the COVID-19 pandemic interrupts the product supply chain, and the global economy is in face of a recession (Shang et al., 2021). Brem et al. (2021) discussed 10 technologies that play a major role in the COVID-19 crisis and found that technological innovation has a key role in response to the epidemic and subsequent economic recovery. Cai et al. (2021) analyzed the impact of the explosive pandemic on the labor market of China. The results showed that under the impact of the pandemic, overall employment assumed a “V”-shaped trend. Based on the monthly panel data of Chinese provinces and cities, Zhang and Zhu (2021) used DID model to analyze the impact of the pandemic on various industries and concluded that the pandemic has a significant negative impact on international trade and the road freight industry.
External shocks tend to exert a significant impact on the financial stock market (Baker et al., 2020; Zhao et al., 2021). Chen (2020) used the event analysis method to analyze the impact of the pandemic on the stock market of China, and the results showed that the return rate of the market at large had dropped significantly during the pandemic and impacted on different types of firms to varying degrees. Wang et al. (2020) used the panel VAR model and dynamic econometric model to evaluate the impact of the COVID-19 on stock price fluctuation and concluded that fear and anxiety from the pandemic may drive investors to sell stocks and to cause price fluctuation. Sun et al. (2021) studied the impact of investor sentiment during the pandemic on pharmaceutical stock trading in mainland China, Hong Kong, South Korea, Japan, and the United States, and the results showed that ERAs had a significant positive effect on pharmaceutical investment portfolios in these markets.
The related investigations focus on investor sentiment mainly from the perspective of investor attention metrics and the impact of investor sentiment on the financial stock markets; major public health events are studied based on the impact on the economy and the financial market. Few studies employ investor sentiment as an explained variable to study the impact of the pandemic as an external shock on investor sentiment. Our study is intended to make three contributions to the existing literature. First, we introduced the listed firms on the A-share stock market of China as the research object and analyzed the impact of the pandemic on investor sentiment at the firm level. Second, the authors introduced new research methods by considering the pandemic as a quasi-natural experiment and building a DID model intended to assess the impact of the pandemic on investor sentiment. Meanwhile, the propensity score matching-difference-in-difference (PSM-DID) method and the placebo-controlled test were used to conduct a series of robustness tests. Third, the heterogeneity analysis was applied to the model being built to illustrate the impact of the pandemic on investor sentiment based on the firm type and industry differences.
In January 2020, the pandemic first broke out in Wuhan, China and then quickly spread to other cities. However, the Chinese government decisively adopted strict lockdown measures, which resulted in effective control of the spread of the virus. To analyze the impact of the pandemic on domestic investor sentiment, we took the 2020 outbreak as the start time, regarded the pandemic as an external shock, and studied investor sentiment based on a DID model. This model is widely used to evaluate the effects of policies in different pilot regions. Similar to the implementation of policies in different pilot regions, the outbreak of the COVID-19 pandemic in different regions can also be regarded as a quasi-natural experiment.
We considered those Chinese provinces with a cumulative number of confirmed infection cases not <1,000 (Zhang and Zhu, 2021). Listed firms registered after December 2019 in Hubei, listed firms registered after February 2020 in Guangdong, Henan, and Zhejiang, and listed firms registered after March 2020 in Hunan are used as the treatment groups, while listed firms listed in other provinces served as the control groups. The time series of provinces with a cumulative number of confirmed cases over 1,000 are shown in Table 1.
Table 1. The time series of confirmed cases with a cumulative number of over 1,000 in the provinces.
The model is as follows:
The explained variable sent represents investor sentiment on the individual listed firm of i at a specific time (t). All the listed firms in the sample fall into four groups. If postit = 1 and treatit = 1, the firm falls into the post-pandemic treatment group; if postit = 0 and treatit = 1, the firm falls into the pre-pandemic treatment group; if postit = 1 and treatit = 0, the firm falls into the post-pandemic control group; and if postit = 0 and treatit = 0, the firm falls into the pre-pandemic control group. In this study, the core explanatory variable was postit × treatit, and β1 measures the impact of the pandemic on investor sentiment. If β1 <0, the pandemic is shown to reduce the turnover rate and make the investors pessimistic; if β1>0, the pandemic on its full-scale tends to increase the turnover rate. Besides, Xit is a series of control variables, νi is the individual fixed effects, μt is the time fixed effects, and εit is the random error term.
sentiment, used in this study as an explained variable, refers to theoretically inexplicable factors that drive investors make stock market forecasts based on macroeconomics and company data. It can be seen as a specific risk in connection with capital market development and reflects the market forecasts and confidence of investors in their own investment strategy. The turnover rate is an indicator normally used in measuring investor sentiment on an individual stock and is calculated generally as trading volume divided by total equity. However, a vast number of the stocks on the A-share stock market are traded off the exchange. In this study, we improved the approach to measuring investor sentiment on individual stocks. We used turnover divided by free float as the proxy variable for investor sentiment. We do not include (i) the free floats of shareholders who held more than 5% of the outstanding shares of the firm and (ii) the free floats of shareholders who held <5% of the outstanding shares of the firm and whose associated shareholders held 5% of the outstanding shares of the firm. According to the studies of Wang and Wang (2014), Larrain and Urzúa (2013), and Tian et al. (2020), we selected seven control variables, namely, firm size (size), independent director percentage (Indep), firm age (lnage), percentage of holdings of top shareholder (top1), market value and book value (mvba), enterprise accounting performance (epa), and financial leverage (lev). Table 2 shows the definitions of the control variables in detail.
We used monthly panel data of the firms listed on the A-share stock market of China dated from October 2019 to June 2020 as the initial samples. Since the New Year Holiday and the Chinese New Year fell on January 2020, this study eliminated the possible impact of such factors on the A-share stock market by excluding the data on January 2020. In addition, considering the importance of accuracy and robustness of the research findings, we processed the initial samples by excluding real property developers, financial institutions, ST stocks, *ST stocks, samples with data missing, and samples traded for <15 days per month. Finally, 16,983 observation values were returned. All the statistics are sourced from the GTA CSMAR database.
Table 3 shows the descriptive statistics of our selected variables.
First, we made a DID estimation of equation (1). The basic regression results are shown in Table 4. The first column showed the estimates of the time fixed effect and the individual fixed effect. The explanatory variable post × treat equaled to −0.0552 and was significant at the 1% level, indicating that the outbreak of the pandemic drove down the turnover rates on the A-share stock market and led to pessimistic investor sentiment. The second column, including control variables, shows significant results, which indicate that in those provinces hit harder by the pandemic, the listed firms are subject to a more negative shock on investor sentiment. This was due to waning confidence and an overall pessimistic outlook on the financial market.
An important assumption for the DID estimation is that the treatment groups and the control groups are assumed to have the same trend without an obvious difference. This study shows that the parallel trend test results are in a 95% CI. As shown in Figure 1, the treatment groups and the control groups had no obvious difference before the pandemic broke out. In early February 2020 when the pandemic broke out, the panic on the A-share stock market of China caused the investors to sell off the holdings in large quantities, and therefore, there was a decline in investor sentiment. After the outbreak, there was a significantly negative, aggravating impact on investor sentiment, which is an important cause for the obvious difference in investor sentiment between the treatment groups and the control groups. In April 2020, the impact on investor sentiment rebounded to some degree thanks to government policy support and effective control measures.
The impact of the pandemic on investor sentiment may vary with the characteristics of the listed firms; therefore, the impact of the pandemic is analyzed from the perspective of the sector and firm ownership.
The impact of the pandemic on investor sentiment also varies across industries, i.e., the pharmaceutical or non-pharmaceutical sector. This study divided the listed firms on the A-share stock market into the pharmaceutical sector and the non-pharmaceutical sector. As shown in Table 5, when it comes to the pharmaceutical sector, the outbreak of the pandemic had a significantly positive impact on investor sentiment, but when it comes to the non-pharmaceutical sector, there was a significantly negative impact. This result makes sense as the pandemic leads to an upsurge in demand for medical supplies. The government invested more in the pharmaceutical sector, and the investors also expect the sector to be promising.
This study classified the firms traded on the A-share stock market based on firm ownership. Firms involving a state-owned stake were categorized as a state-owned firm, firms involving a privately owned stake were categorized as a privately owned firm, and firms involving a foreign-owned stake were categorized as a foreign-owned firm. In Table 6, columns 1–3 show the regression results of the firms of different ownerships. For the privately owned and foreign-owned firms, investor sentiment has a negative coefficient. In contrast, for the state-owned firms, the investor sentiment coefficient was significantly positive at a 1% level. For the foreign-owned firm, the negative impact of the pandemic on investor sentiment makes sense mainly because the pandemic is an international public health event. The pandemic began to sweep across China in early 2020 and from March 2020, transmitted on a large scale to other countries. The pandemic worsened quickly and countries worldwide took emergency measures in response. Hence, the world economy faced a recession, international demand shrank by a large margin, and import and export markets of China suffered heavily. Therefore, the investors had a generally prudential, or pessimistic, attitude to invest in these firms. Privately owned firms were generally weak in risk resistance, so they were the most impacted. In quite a few provinces, the SMEs went broke under heavy impact. Compared with the privately owned firm and the foreign-owned firm, the state-owned firm was less hit by the pandemic because of government backing as an advantage. When the panic about the pandemic enshrouded the whole market, the market investors chose to buy in more stocks of the state-owned firm, hence its high turnover rate. The investors were, in themselves, optimistic about the state-owned firm. Therefore, the ability of the privately owned firm and the foreign-owned firm to adapt to external shocks should be improved. When a major crisis breaks out, the government should increase its support for such enterprises. The enterprises themselves should increase investment in Science and Technology, promote technological innovation, improve their own anti-risk capabilities, and increase investor confidence in them.
Table 6. Ownership regression results (state-owned firm vs. privately owned firm vs. foreign-owned firm).
To test if investor sentiment is driven by other unobservable factors, a certain number of groups were sampled on a random basis for the purpose of the robustness test. In this study, 500 random samplings were conducted and for each sampling, some of the provinces sampled served as the treatment groups and randomly sampled months between October 2019 and April 2020 served as the time of the outbreak. Our analysis gives the estimated coefficient of investor sentiment as a dependent variable. Based on the basic regression of statistics as shown in Table 4, the estimated coefficient with investor sentiment as the dependent variable was −0.0552, obviously different from the coefficient returned by the placebo-controlled test as shown in Figure 2. Therefore, the existence of other unobservable factors on investor sentiment was insignificant.
This study conducted a PSM analysis of investor sentiment to reduce the selection bias associated with sampling. The various matching variables, i.e., lnage, size, the debt-to-asset ratio, and mvba, were used as a basis for PSM to select appropriate control groups, and the matched samples underwent DID estimation. An important assumption for PSM is the fulfillment of overlapping one. If both treatment groups and control groups have high propensity scores, it points to a high degree of overlap and the effectiveness of the PSM model, and the reverse is true. Figure 3 shows the results of the overlapping assumption test. There was an overlap of the treatment groups with the control groups, i.e., the overlapping assumption was fulfilled. Also, addressing selection bias with PSM has to fulfill the balance assumption, i.e., except for investor sentiment, there should be no significant difference between the treatment groups and the control groups. It is generally supposed that a post-matching SD <20% points to a good matching effect. Table 7 shows the balancing test results, which shows that the post-matching SDs of the sample variables dropped below 20%, indicative of the fulfillment of the balancing assumption.
With the above overlapping assumption test and balancing test, a PSM-robustness test was conducted. 1:3 nearest neighbor matching, radius matching, and kernel matching were implemented. Table 8 shows the relative regression results, which shows that the coefficient of the variable post × treat remained significantly at a 1% level.
Table 8. Propensity score matching test (nearest neighbor matching vs. radius matching vs. kernel matching).
To evaluate how and to what extent the COVID-19 pandemic exerts an impact on investor sentiment, this study conducted research on the performance of the A-share stock market of China during the pandemic by doing a quasi-experiment based on the pandemic. Besides, the DID model was used to investigate the impact of the arrived at three conclusions. First, the pandemic drove down the turnover rate on the A-stock market and made the investors pessimistic, so those currently without holdings did not involve themselves in market trading. Second, the pandemic impacted negatively on investor sentiment and brought it down significantly when it comes to the privately owned firms and the foreign-owned firms; in contrast, the state-owned firms attracted many individual investors on the strength of their unique advantages. Third, the outbreak of the pandemic drew broad attention to the pharmaceutical industry from the government and people, so it boosted up investor sentiment in the pharmaceutical industry while having a significantly negative impact on the non-pharmaceutical industry.
In general, considering the pandemic as an external shock that exerts a substantial impact on investor sentiment, the Chinese government and the competent regulatory agencies should implement regulations and establish an early risk warning system. Education and protection should be provided for individual investors to maintain financial security and stability. Based on the aforesaid research findings, this study proposed the following three policy recommendations. First, an early risk warning mechanism and a set of emergency measures should be introduced to the A-share stock market, so in the event of the outbreak of major incidents, i.e., the COVID-19 pandemic, market risk associated with investor sentiment fluctuations can be precluded; besides, appropriate regulatory intervention should be made to limit irrational A-share stock market trading and make the stock market stabler. Second, the competent regulatory agency should educate the investors pertinently, improve their expectations, and guide them to respond reasonably to the external shock of the market. Investor sentiment is an important factor that causes financial stock market volatility under the pandemic. Therefore, psychological monitoring or emotional management intervention during a pandemic outbreak helps people not only to identify mental health problems but also to control their emotions and avoid unreasonable investment behaviors. Third, the impact of the pandemic on investor sentiment should be looked at reasonably. In general, although there was a negative impact on investor sentiment, the pandemic was inversely correlated with the pharmaceutical industry and the state-owned firms, i.e., investigation of investor sentiment at the firm level could be important. Stringent pandemic control measures should be combined with other measures in favor of effective optimistic investor sentiment.
The original contributions presented in the study are included in the article/supplementary material, further inquiries can be directed to the corresponding author/s.
YS: conceptualization, methodology, formal analysis, writing—original draft preparation, and funding acquisition. XH: data processing, formal analysis, and writing—original draft preparation. ZL: methodology, project management, and funding acquisition. All authors contributed to the article and approved the submitted version.
This study obtained the financial support of the National Social Science Foundation (the impact of heterogeneity of regional trade in service agreements on the reconstruction of the global value chain of the manufacturing industry in China; Project No: 20BJY091) and the innovation team project of Philosophy and Social Sciences in Colleges and Universities of Henan Province (coordinated development of urban and rural areas and rural revitalization; Project No: 2021-CXTD-04).
The authors declare that the research was conducted in the absence of any commercial or financial relationships that could be construed as a potential conflict of interest.
All claims expressed in this article are solely those of the authors and do not necessarily represent those of their affiliated organizations, or those of the publisher, the editors and the reviewers. Any product that may be evaluated in this article, or claim that may be made by its manufacturer, is not guaranteed or endorsed by the publisher.
Baker, M., and Stein, J. C. (2004). Market liquidity as a sentiment indicator. J. Financial Mark. 7, 271–299. doi: 10.1016/j.finmar.2003.11.005
Baker, M. P., and Wurgler, J. (2006). Investor sentiment and the cross-section of stock returns. J. Finance 61, 1645–1680. doi: 10.1111/j.1540-6261.2006.00885.x
Baker, S. R., Bloom, N., Davis, S. J., Kost, K. J., Sammon, M. C., and Viratyosin, T. (2020). The unprecedented stock market impact of COVID-19. Rev. Asset Pric. Stud. 10, 742–758. doi: 10.1093/rapstu/raaa008
Balke, N. S., Fulmer, M., and Zhang, R. (2017). Incorporating the beige journal into a quantitative index of economic activity. J. Forecast. 36, 497–514. doi: 10.1002/for.2450
Barro, R. J., Ursúa, J. F., and Weng, J. (2020). The coronavirus and the great influenza pandemic: lessons from the“Spanish Flu” for the Coronavirus's potential effects on mortality and economic activity. NBER Working Paper, No. 26866. doi: 10.3386/w26866
Brainerd, E., and Sieglar, M. V. (2003). The economic effects of the 1918 influenza epidemic CEPR Discussion Paper, No. 3791.
Brem, A., Viardot, E., and Nylund, P. A. (2021). Implications of the coronavirus (COVID-19) outbreak for innovation: which technologies will improve our lives? Technol. Forecast. Soc. Change 163:120451. doi: 10.1016/j.techfore.2020.120451
Brown, G. W., and Cliff, M. T. (2005). Investor sentiment and asset valuation. J. Bus. 78, 405–440. doi: 10.1086/427633
Cai, F., Zhang, D. D., and Liu, Y. X. (2021). The impact of COVID-19 on the chinese labor market—a comprehensive analysis based on the individual tracking survey. Econ. Res. J. 56, 4–21.
Chen, F. G. (2020). The heterogeneous impact of COVID-19 on Chinese enterprises—empirical research based on the perspective of stock price fluctuations. Ind. Technol. Econ. 39, 3–14. doi: 10.3969/j.issn.1004-910X.2020.10.001
Das, S. R., and Chen, M. Y. (2008). Yahoo! For Amazon: Sentiment extraction from small talk on the web. Oper. Res. 48, 601–602. doi: 10.1287/mnsc.1070.0704
Delong, J. B., Shleifer, A., Summers, L. H., and Robert, J. W. (1990). Noise trader risk in financial markets. J. Polit. Econ. 98, 703–738. doi: 10.1086/261703
Fang, G., Gozgor, G., Lau, C., and Lu, Z. (2020). The impact of Baidu index sentiment on the volatility of China's stock markets. Finance Res. Lett. 32:101099. doi: 10.1016/j.frl.2019.01.011
Fisher, K. L., and Statman, M. (2000). Investor sentiment and stock returns. Financial Anal. J. 56, 16–23. doi: 10.2469/faj.v56.n2.2340
Gozgor, G., Lau, C. K. M., Sheng, X., and Yarovaya, L. (2019). The role of uncertainty measures on the returns of gold. Econ. Lett. 185:108680. doi: 10.1016/j.econlet.2019.108680
Hanna, D., and Huang, Y. (2006). The impact of SARS on asian economies. Asian Econ. Pap. 3, 102–112. doi: 10.1162/1535351041747978
Jiang, B., Zhu, H., Zhang, J. H., Yan, C., and Shen, R. (2021). Investor sentiment and stock returns during the COVID-19 pandemic. Front. Psychol. 12:708537. doi: 10.3389/fpsyg.2021.708537
Larrain, B., and Urzúa, F. (2013). Controlling shareholders and market timing in share issuance. J. Financ. Econ. 109, 661–681. doi: 10.1016/j.jfineco.2013.03.013
Li, Z. W., Kang, C. Y., and Mao, X. X. (2017). The impact of external shocks on chinese investors' sentiment. J. Dalian Univ. Technol. 38, 71–76. doi: 10.19525/j.issn1008-407x.2017.02.012
Shang, Y., Li, H., and Zhang, R. (2021). Effects of pandemic outbreak on economies: evidence from business history context. Front. Public Health 9:632043. doi: 10.3389/fpubh.2021.632043
Sun, Y., Bao, Q., and Lu, Z. (2021). Coronavirus (Covid-19) outbreak, investor sentiment, and medical portfolio: evidence from China, Hong Kong, Korea, Japan, and U.S. Pacific. Basin Finance J. 65:101463. doi: 10.1016/j.pacfin.2020.101463
Tian, X., Kou, G., and Zhang, W. (2020). Geographic distance, venture capital and technological performance: evidence from Chinese enterprises. Technol. Forecast. Social Change 158, 120–155. doi: 10.1016/j.techfore.2020.120155
Wang, Q., Wang, Z. L., Li, S. X., and Xue, F. Z. (2020). The immediate impact of COVID-19 on China's stock price fluctuation. Rev. Econ. Manag. 6, 16–27. doi: 10.13962/j.cnki.37-1486/f.2020.06.002
Wang, Y. F., and Wang, Y. M. (2014). The role of investor sentiment in asset pricing[J]. Manage. Rev. 26, 42–55. doi: 10.14120/j.cnki.cn11-5057/f.2014.06.039
Wu, Q. F. (2003). Empirical study on impact of SARS epidemic on the equity funds in China. Manag. Rev. 15, 8–11.
Wu, W., Tiwari, A. K., Gozgor, G., and Leping, H. (2021). Does economic policy uncertainty affect cryptocurrency markets? Evidence from twitter-based uncertainty measures. Res. Int. Bus. Finance 58:101478. doi: 10.1016/j.ribaf.2021.101478
Xie, S. Q., and Tang, S. S. (2021). The impact of investor sentiment and macro economy fluctuations on stock market returns. Macroeconomics 2, 99–109. doi: 10.16304/j.cnki.11-3952/f.2021.02.00
Yang, X. L., Shen, H. B., and Zhu, Y. (2016). The effect of local bias in investor attention and investor sentiment on stock markets: evidence from online forum. J. Financ. Res. 438, 143–158.
Yi, Z. G., and Mao, N. (2009). Research on the measurement of investor sentiment in Chinese Stock market: the CICSI's construction. J. Financ. Res. 11, 174–184.
Yu, C., Zhang, R., An, L., and Yu, Z. X. (2020). Has China's belt and road initiative intensified bilateral trade links between China and the involved countries? Sustainability 17, 1–19. doi: 10.3390/su12176747
Zhang, B. O., Hu, W. X., and Yang, X. A. (2021). Research on the formatting mechanism of investor sentiment. Chin. J. Manag. Sci. 29, 185–195. doi: 10.16381/j.cnki.issn1003-207x.2021.01.018
Zhang, J. P., and Zhu, Y. X. (2021). The impact of COVID-19 epidemic on China's service economy in post epidemic era—a study based on a multi period double difference model. Ind. Technol. Econ. 40, 58–67. doi: 10.3969/j.issn.1004-910X.2021.04.007
Zhang, R. (2019). News shocks and the effects of monetary policy. Macroecon. Dyn. 10, 1–41. doi: 10.2139/ssrn.3348466
Keywords: investor sentiment, COVID-19 pandemic, difference-in-differences model, financial market, A-share listed companies
Citation: Song Y, Hao X and Lu Z (2021) The Impact of the Coronavirus Disease 2019 Pandemic on Investor Sentiment—Evidence From A-Share Listed Companies in China. Front. Psychol. 12:743306. doi: 10.3389/fpsyg.2021.743306
Received: 18 July 2021; Accepted: 16 August 2021;
Published: 13 September 2021.
Edited by:
Giray Gozgor, Istanbul Medeniyet University, TurkeyReviewed by:
Ren Zhang, Texas State University, United StatesCopyright © 2021 Song, Hao and Lu. This is an open-access article distributed under the terms of the Creative Commons Attribution License (CC BY). The use, distribution or reproduction in other forums is permitted, provided the original author(s) and the copyright owner(s) are credited and that the original publication in this journal is cited, in accordance with accepted academic practice. No use, distribution or reproduction is permitted which does not comply with these terms.
*Correspondence: Zhou Lu, bHV6aG91NTlAdGpjdS5lZHUuY24=
Disclaimer: All claims expressed in this article are solely those of the authors and do not necessarily represent those of their affiliated organizations, or those of the publisher, the editors and the reviewers. Any product that may be evaluated in this article or claim that may be made by its manufacturer is not guaranteed or endorsed by the publisher.
Research integrity at Frontiers
Learn more about the work of our research integrity team to safeguard the quality of each article we publish.