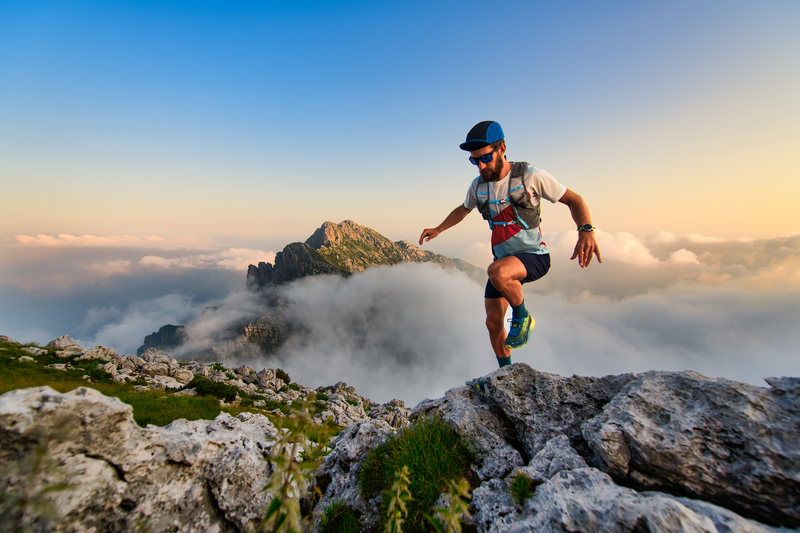
95% of researchers rate our articles as excellent or good
Learn more about the work of our research integrity team to safeguard the quality of each article we publish.
Find out more
ORIGINAL RESEARCH article
Front. Psychol. , 22 July 2021
Sec. Educational Psychology
Volume 12 - 2021 | https://doi.org/10.3389/fpsyg.2021.679197
The COVID-19 pandemic has brought a tremendous impact on the pedagogy and learning experience of students in sub-degree education sector of Hong Kong. Online learning has become the “sole” solution to deal with student learning challenges during this chaotic period. In this study, we explore online learning for sub-degree students by using a community of inquiry (CoI). As such, confirmatory factor analysis (CFA) was conducted on survey data gathered from 287 sub-degree students from the business and engineering disciplines. Results indicated that the network speed for online education determines the perceived cognitive presence, social presence, and teaching presence of students, whereas gender and academic disciplines of students are not moderating factors that create a significant difference in perceived cognitive presence, social presence, and teaching presence of students. Our study findings for creating and sustaining a purposeful online learning community are highlighted.
In general face-to-face (also named as a traditional classroom), online, and blended (also named as a hybrid, inverted, or flipped) are the common teaching pedagogy methods in higher education institutions. The adoption of technology into teaching pedagogy has arisen in different research agendas and directions in recent years (Rasheed et al., 2020). The current advancement of digital technologies, open-source software communities, and applications provides remarkable opportunities to deliver online education. However, authors like Shea and Bidjerano (2009) consider that although the emergence and establishment of online education are well-documented, the online learning context is in need of change. This is especially true under the social conditions derived from the COVID-19 pandemic.
According to Sangrà et al. (2012, p. 152), online education is “an approach to teaching and learning, representing all or part of the educational model applied, that is based on the use of electronic media and devices as tools for improving access to training, communication and interaction and that facilitates the adoption of new ways of understanding and developing learning.” Online education is emerging as the main modality of higher education (Singh and Holt, 2013). Singh and Holt (2013, p. 98) reinforced “The Sloan Consortium found that 66% of post-secondary institutions reported an increased demand for new distance education course offerings as well as an increased demand for their existing distance education coursework.” As such, online learning is now recognized as an adaptable and portable approach for students to “possess new skills, new knowledge and new ways of learning” in a digital era (Wood and Shirazi, 2020, p. 1). Students having the opportunity to access or use an online learning system can engage in instructional materials without time and place restrictions (Lee, 2008). Also, students are able to gain a learning process from the peer assessment and question creation via various media styles simultaneously (Yu and Wu, 2011). In addition, students can participate in managing the content and progress of their learning (Lee, 2008). Wood and Shirazi (2020) addressed that the control of the learning environment is gradually changed from the teacher to the learner. However, the teacher is expected to provide well-structured learning materials and subject design to improve student participation and ensure relevance.
Recently, teaching supports using technologies are important for the younger generation to enhance their learning interests. Mo and Tang (2017) adopted the problem-based learning approach to facilitate students learning 3D printing technologies. Tang and Yu (2018) make use of mobile devices to deliver teaching materials to the students anytime and anywhere. Although these technologies are good to encourage learning motivation of students, the communication and interaction between peers and teachers, as well as the ability of students to confirm and reflect what they have learned, are also essential. Sub-degree students nowadays are described as “GEN Z,” “millennials,” “digital natives,” and “net generations.” As such, social networking, multimedia, and interactivity are part of the daily experiences of students. It is somehow paradoxical that the traditional way of learning and teaching in higher education comprises a lecture-style arrangement, including a massive number of students sitting in lecture theaters with minimal interaction with their teachers and peers. Such non-participating and transmission-based experiences seem to be failing to fulfill the expectations of students (Wood and Shirazi, 2020), particularly in Hong Kong, where the conditions seem to be ideal for more interactive, technology-based approaches. Hong Kong is known for having a superb infrastructure, which meets the needs of its population and contributes to the efficiency and growth of the economy. For example, according to data from the Measurement Lab (M-Lab)1 in 2020, it performed the second-best internet speed out of 192 countries (we will come back to this point later in this section).
Although the policies of both developing and developed countries strive toward investing in online learning systems, the behavioral intention of students and academics to use online learning systems is relatively low (Alhabeeb and Rowley, 2018). Despite this political will and the fact that the material conditions are set for online learning systems in many countries, some educators have identified key challenges from the perspectives of different stakeholders. From the perspectives of students, students may face unfolded challenges like self-regulation, technological competency and literacy, isolation, and technological complexity. From the perspective of teachers, teachers may encounter various challenges, including online learning tools, beliefs, technological operation, and technological competency and literacy. From the perspective of an institution, technology infrastructure, teacher training, and technological provision are the main challenges (Alhabeeb and Rowley, 2018; Rasheed et al., 2020). Apart from the past research studies, this study was inspired by problems related to online learning mentioned in constructive feedback given by students during formal Student Staff Consultative Group Meetings in Hong Kong higher education institutions. The researchers gained an insight into the technical problems affecting the online learning progress of students. To better understand how these technological problems affect online learning of students, the researchers considered representative academic studies, The community of inquiry (CoI) framework was identified by Garrison et al. (2000).
The CoI framework was developed on Dewey's (1959) collaborative–constructivist learning philosophy and was later applied to online learning environments. In general, the CoI framework includes three main kinds of presence, namely, cognitive presence, social presence, and teaching presence. These three types of presence are supposed to interconnect with the CoI theoretical framework. Figure 1 illustrates the interrelated relationship of the three main kinds of presence considered in the CoI framework.
Figure 1. The interrelated relationship of key elements in the CoI framework. Source: Garrison et al. (2000).
Teaching presence is described as any activity that involves teaching, such as organization and curriculum design, discourse simplification, and direct coaching to students. Social presence is defined as the activities of communicating and developing social relationships among the members of a community, such as classmates. Cognitive presence is relevant to the intellectual tasks of knowledge-building. Based on Garrison et al. (2001), cognitive presence is “the extent to which the learners are able to construct and confirm meaning through sustained reflection and discourse.” All these three presences are interrelated—the teaching presence is “the design, facilitation, and direction of cognitive and social processes to realize personally meaningful and educationally worthwhile learning outcomes” (Anderson et al., 2001).
Based on feedback collected during student–staff consultative group meetings and the CoI framework, the researchers had addressed three key research questions: (1) Is the network speed for online education associated with the perception of students of their CoI (i.e., cognitive presence, social presence, and teaching presence)? (2) Is gender of students associated with their perception of their CoI (i.e., cognitive presence, social presence, and teaching presence)? (3) Is academic discipline of students associated with their perception of their CoI (i.e., cognitive presence, social presence, and teaching presence)? The last two research questions are addressed as previous studies have found gender and academic discipline differences in technology usage behavior. For example, Venkatesh et al. (2003) theorized that gender has a moderating effect on technology usage behavior, while Park et al. (2012) found that the majority of relevance (that is, academic discipline) determines technology usage behavior. In this sense, gender and academic discipline of the students are associated with their actual usage of online learning technology. However, neither Venkatesh et al. (2003) nor Park et al. (2012) used CoI as a methodological approach. In this study, we aim at unveiling a more nuanced account of how these two factors are related to technology usage behavior through the use of a CoI approach.
To a certain extent, large-scale online learning has been forced to happen during the COVID-19 pandemic. It is a new norm that has not been explored extensively. It is argued here that what is required is a model that gives the educators a framework to understand as well as tools to overcome the challenges posed by synchronous online teaching in a particular virtual place. In this study case, the participants were sub-degree students. Specifically, these students enrolled in sub-degree programs (i.e., Associate Degree and Higher Diploma) because they attained poor examination results in their Hong Kong Diploma of Secondary Education (HKDSE). The sub-degree qualification is a significant independent exit qualification for further studies and employment in management and administrative positions at the entry level (Lau et al., 2018). Most of them were within the same age group (average 19 years of age) and had a similar experience of using online education technology. Indeed, we highlight that all the face-to-face classes were suspended in the case of higher education institutions at the outbreak of COVID-19 (also named Corona Virus) from January to May 2020. The rapid spread of COVID-19 forced various colleges and universities to close temporarily. Indeed, a normal teaching time is still questionable until we explore an effective vaccine in the world. In order to minimize the negative impacts on the learning opportunities of students, the use of online education technology was not voluntary during that time (Dhawan, 2020). For this reason, the key elements of age, the voluntariness of use, and experience were not taken into account in our study. The researchers only investigated gender and academic discipline as moderating factors. Also, the network speed issue is associated with effort expectancy—the higher the network speed for online education, the less effort an online education learner has to make. Although Hong Kong is classified as Asia World City, there is a wide wealth gap. Some sub-degree students are now living in cage-homes or bed-space apartment homes. Also, a part of sub-degree students may need to share common rooms or learning facilities in order to access to an Internet connection. Furthermore, feedback was given by students during formal Student Staff Consultative Group Meetings (see above), suggesting that the Internet speed is a common problem for sub-degree students who struggle with within Hong Kong.
The rest of the paper is divided into six main sections. The introduction is given in section Introduction; a literature review is given in section Literature Review; research methodology and results are given in sections Research Methodology and Results, respectively. Finally, the discussion of the key research findings and the main conclusion of the investigation are found in sections Discussion and Conclusion, respectively.
In this section, we conduct a comprehensive literature review by exploring the terms that are relevant to our research questions. We identify theoretical descriptors (e.g., “Use of Technology,” “Community of Inquiry/CoI”); independent variable descriptors (e.g., “Gender,” “Academic Discipline,” “Male,” “Female,” “Network/Internet Speed/Efficiency,” “Students' Satisfaction Level of Network/Internet Speed/Efficiency,” “Online Education Network/Internet Speed/Efficiency”); and outcome descriptors (e.g., “Community of Inquiry/CoI Elements,” “Teaching Presence/TP,” “Social Presence/SP,” “Cognitive Presence/CP”).
In the context of online education, few research studies were addressing specific disciplines like business, education, and engineering in the CoI model (Carlon et al., 2012; Jiang and Koo, 2020). Shea and Bidjerano (2009) consider that in such particular disciplines, it is relatively complicated to incorporate technologies (e.g., wikis, blogs, streaming video) into online learning contexts in approaches that will improve student learning. Garrison and Arbaugh (2007) proposed a model for research studies to examine the generalizability of the CoI model across diverse disciplines. Later, Garrison et al. (2010a) revisited the evolution of the model, recognizing that the model still requires extra validation across disciplines and student demographics (e.g., gender and age). In response, Carlon et al. (2012) and Choy and Choon (2016) reinforced the finding that key demographics (e.g., gender and academic disciplines) affect perceptions of students of their CoI in the online learning context. Moreover, Garrison et al. (2010b, p. 32) concluded that “program of study varies according to discipline, each with unique teaching paradigms, styles of discourse and epistemologies. Given the interactive and inquiry-based focus of online communities of inquiry, different disciplines may result in unique patterns of relationships among presences. This may also be true of gender. Such differences may result in a difference in social presence, an element central to learning in an online community of inquiry.”
Our review of the literature in the field revealed that research studies investigating gender differences across disciplines in the CoI model are scarce, notably in business and engineering disciplines. Because of the known disciplinary effects in the business and engineering disciplines, these kinds of studies are urgently demanded. Also, there was a significant research gap in exploring the effect of satisfaction level of students regarding network speed for online learning, particularly framed by the CoI elements; no previous work was found that evaluated whether gender and academic discipline moderated the association between the satisfaction level of students regarding network/Internet speed for online education/learning considering the CoI elements. Furthermore, most CoI research studies (e.g., Arbaugh et al., 2010; Garrison et al., 2010a,b; Carlon et al., 2012; Arbaugh, 2013; Dempsey and Zhang, 2019; Heilporn and Lakhal, 2020) used data from undergraduate and postgraduate samples. The context of Hong Kong sub-degree students has been seriously overlooked even though it represents a large student population and is considered a unique education sector in the academic world.
Many previous studies explored the relationships (e.g., correlations and causal relationships) among the elements in the original CoI framework without involving our variables of interest (i.e., satisfaction level of students regarding network speed for online learning, along with the moderating impacts on academic discipline and gender in the proposed study). The following are some examples of these previous studies. Akyol et al. (2009) adopted mixed methods to conduct a CoI investigation, examine the transcript of online discussions, and carry out interviews to explore the main difference in the understanding of students of the CoI elements between the blended courses and online courses. Akyol et al. (2011) used mixed methods to conduct a CoI survey and analyze the transcript of online discussions to identify the difference in understanding of students of the CoI elements in long-term (13-week) and short-term (6-week) blended courses. Garrison et al. (2010b) found “teaching and social presence have a significant perceived influence on the cognitive presence and that teaching presence is perceived to influence social presence” (p. 31). Gutiérrez-Santiuste et al. (2015) used multiple regression analysis and also found that social presence and teaching presence brought an effect of the cognitive presence, while social presence has a higher effect and there is no significant collinearity between social presence and teaching presence. Rolim et al. (2019) uncovered the association between cognitive and social presence in the CoI by adopting epistemic network analysis. Some studies investigated the practice of the CoI framework, such as the studies using the Quality Matters rubric and CoI framework to guide the iterative redesign of courses to facilitate students attain learning outcomes (Swan et al., 2012, 2014), the study of combining seven fundamentals of good practice (Sorensen and Baylen, 2009) and the CoI framework to formulate online pedagogic activities for practitioners and instructors (Fiock, 2020), and the study of using computer-mediated discourse analysis on the online discussion postings to explore how the CoI elements manifested (Zhu et al., 2019).
Many other studies revised the CoI framework by integrating some new elements into the CoI framework and by examining the relationships between these new elements and the original three CoI elements. For example, Lin et al. (2015) found that self-efficacy is a complete mediator between cognitive presence and social presence. In this case, self-efficacy is a new element integrated into the CoI framework. There are other examples: Shea and Bidjerano (2010, 2012) and Shea et al. (2014) advocated the inclusion of learner presence in the CoI framework. Building on these works, Ma et al. (2017) examined the revised CoI framework and used the path analysis to find that a similar element, called learning presence, has a significant partial mediating effect in the association between the cognitive presence and the teaching presence, as well as in the association between the cognitive presence and the social presence. Furthermore, Shea and Bidjerano (2009, 2013) explored that gender, age, and academic level could generate the mediating effects of student online experience in the CoI framework.
Similar to this proposed study, some previous studies explored the relationships among the CoI elements and some new elements without revising the original CoI framework. For example, Akyol and Garrison (2008) analyzed the transcripts of online discussion postings and identified significant relationships among the CoI elements and two new elements, namely, learning motivation and expectation of students within the online program. Law et al. (2019) investigated the mediating effects of the CoI elements in the association between student recruitment and learning achievement, and between the rationale behind learning and learning achievement in a blended learning context. They found that such two new variables (i.e., student enrollment and learning motivation) influence the elements of the CoI framework, while the CoI elements influence learning performance (another new external element). In the proposed study, the new external elements are the satisfaction level of students regarding network speed for online learning and the gender and academic disciplines of students. The researchers attempted to explore the direct effect of the satisfaction level of students regarding network speed for online learning on the CoI elements and also explore the moderating effect between the satisfaction level of students regarding network speed for online learning and the CoI elements.
The studies involving human participants were reviewed and approved by the Hong Kong Polytechnic University. In the ethical approval process, we need to submit an ethics review checklist of human subjects (e.g., financial inducements, repetitive testing, psychological stress or anxiety, blood or tissue samples, DNA or RNA, children, unconscious patients, mentally handicapped people). Thus, our study excluded human subjects. Also, written informed consent to participate in this study was provided by the participants. The consent form addressed the information obtained from this research that may be used in future research and published.
The sub-degree students participated in this study in Hong Kong during the COVID-19 outbreak in February 2020. During the study period, all face-to-face classes were completely suspended. Different learning platforms, including Zoom, Moodle, and edX, were used to facilitate online learning of students, while an online survey tool was used to facilitate data collection. The survey was open for 3 weeks after the commencement of online teaching on February 10, 2020. Six targeted academic courses in the business and engineering areas were randomly selected for the survey, and the students taking these subjects were invited to participate in the survey. The research procedure was fully explained to the participating students, and the consent of the participating students was collected when the participating students completed the online questionnaire for the survey. The students who participated in the survey study voluntarily had the right to question any part of the procedure and could withdraw from the survey at any time.
The survey questions were discussed with educators and researchers to identify appropriate content and question design. To this end, it can foster the validity of the content and make sure the correctness of the survey instruments. In particular, ambiguous wordings and double-barreled questions have been fully taken out. This process is the so-called face validity (Ngai et al., 2008). After that, we proposed our target survey respondent to conduct a pilot test of the survey. Then, we employed principal components analysis (PCA) to identify the fundamental factors that are being examined by our survey questions. Cronbach's alpha reliability values attained 0.6 as a reference point to calculate the internal consistency in our study (Nunnally, 1967). According to our analysis, the values of factor loadings were above 0.6. In other words, we identified that the reliability had obtained a satisfactory level. In the end, we arranged the ordering of the questions to create an appropriate layout of the questionnaire (Iacobucci and Churchill, 2010).
To measure the CoI presence, the online questionnaire was divided into two core parts. In the first part, student demographic information such as gender and study program was collected. The second part contained the core questions; a typical 5-point Likert scale was used to measure the level of agreement of responders with the measured statements, from strongly disagree (1) to strongly agree (5). The core part of the questionnaire consisted of 18 questions, of which five questions were used to measure the teaching presence of students, while seven and six questions were used to measure the cognitive presence and social presence of students, respectively. Table A illustrates the survey instrument used in this study. Based on the related literature (i.e., Stenbom, 2018; Gene et al., 2019; Tang et al., 2021), we construct our methodology, survey questionnaire design, and measurement scale. Through the questionnaire, we investigated whether the gender, academic discipline, and network speed of students are correlated with the perception of students about their CoI. To this end, we applied the CoI concept as the theoretical foundation to respond to our study objectives and design the questionnaire.
In this study, we distributed an online questionnaire with 400 students. About 287 respondents completed the online questionnaire with valid responses. Accordingly, the response rate was 71.75%. A total of 135 (47.04%) were male and 152 (52.96%) were female. Most were from engineering disciplines (54.01%), while 45.99% were from business disciplines. The students were participating in online learning using Microsoft Teams and Moodle. Table 1 summarizes the descriptive analysis of this study.
We first used confirmatory factor analysis (CFA) to create latent variables for the theoretical constructs postulated by the CoI model. Once we ensured the applicability of the theoretical model to our empirical data, we used a t-test to investigate the differences in the CoI elements by gender and academic disciplines, and ANOVA to investigate the differences in network speed.
The statistical analysis packages SPSS and AMOS were used to perform CFA in order to evaluate the hypothetical model at the beginning of this study (Lau et al., 2021). We adopted several commonly used models to determine the fitness acceptance levels of the CoI theoretical framework, including chi-square to degrees of freedom (χ2/df), comparative fit index (CFI), and standardized root mean square residual (SRMR). There is no consensus regarding an acceptable ratio for these statistics; recommendations for the level of acceptance for the χ2/df value of < 5 were adopted in this study (Wheaton, 1987; Schumacker and Lomax, 2004). On the other hand, we adopted the models proposed by Hair et al. (2010) and Hooper et al. (2008) for investigating the fitness of the proposed model. Recently, the SRMR enhanced the conventional RMR and provided a more meaningful interpretation of the results, while CFI was revised to form the normed fit index (NFI), which takes sample size into account and is capable of performing well even for a small sample size. The values for the CFI and SRMR range from 0 to 1.0. The CFI with values closer to 1.0 indicated a good fit, while a smaller value for the SRMR was preferred. A cutoff criterion of CFI ≥ 0.90 and SRMR < 0.05 is suggested in order to represent the close fit of the hypothetical model in the study (Bentler, 1995; Rigdon, 1996).
Then the mean scores and standard deviations of the 18 measured items corresponding to three CoI items were computed. The statistical difference based on gender and academic discipline differences of students was also compared. On the other hand, the effects of network speed for online learning on CoI of students in social presence, cognitive presence, and teaching presence were also investigated. Independent-samples t-test was used to perform the statistical test for determining the gender and academic discipline differences toward CoI, while the effect of network speed satisfaction toward CoI dimensions was compared by running ANOVA. CoI is considered an important process of inquiry and formulating scientific knowledge. It usually refers to an individual or group involved in the inquiry process in a problematic situation. Therefore, it is interesting to investigate further the difference between students studying different sub-degree programs via online learning. In this study, independent-samples t-test was also used to compare the difference between engineering and business students toward CoI in online learning. In all tests, p < 0.05 were adopted to identify statistical significance.
Confirmatory factor analysis was used to validate the hypothetical model and investigate the level of fitness of the proposed framework. The model fit tests were performed using AMOS statistical software. The fit index values of the proposed model, including χ2/df, CFI, and SRMR, are summarized in Table 2. In summary, it was determined that χ2/df = 3.856 < 5, CFI = 0.917 ≥ 0.9, and SRMR = 0.0434 < 0.05. All the models fit measurement statistics, indicating a good fit to fit the index of the target model. Factor loadings for each item were used. The values were over the value of 0.5 as proposed by Hair et al. (2010). For the cognitive presence variables, seven items were used and the factor loadings ranged between 0.78 and 0.88. The teaching presence included five items, and the factor loadings ranged between 0.82 and 0.85. There were six items included in the social presence and the factor loadings between 0.68 and 0.83. Factor loadings of all the measured items are summarized in Figure 2.
Statistical analyses were also performed to determine the reliability and validity of this study. The Cronbach's reliability was used, and the overall reliability is 0.936. The Cronbach's alpha for social presence, cognitive presence, and teaching presence deleted are 0.933, 0.891, and 0.894, respectively, which means the reliability was high and the items should not be removed. The correlation analysis was performed and shown that all items were significantly correlated. The correlation was between 0.804 and 0.877. The composite reliability (CR) and the average variance extracted (AVE) were used to determine the convergent validity of the analysis. The results are summarized in Table 3. The results have shown that the value of AVE for social presence was 0.4. However, according to Tang et al. (2021), as the measured CR was relatively high (>0.6), the convergent validity of the construct was still adequate.
The discriminant validity was calculated by using the heterotrait–monotrait (HTMT) ratio of the correlations (Henseler et al., 2005). The HTMT values are illustrated in Table 4. It was found that the cognitive and teaching presences pair indicated only the HTMT inference discriminant validity, while another two pairs of constructs were smaller than the HTMT0.9 criterion (Mat Yusoff et al., 2020).
In order to analyze the CoI elements of students, the mean scores were computed. It was found that the mean scores for social presence, cognitive presence, and teaching presence were 3.24, 3.43, and 3.40, respectively. On the other hand, the differences in CoI means of students were compared based on the gender of students. An independent-samples t-test was used for comparison. The results show that the p-values for the gender differences in social presence, cognitive presence, and teaching presence were 0.580, 0.513, and 0.426, respectively. Table 5 summarizes the difference between males and females in the mean scores of each CoI element. The values were higher than the level of significance (i.e., p > 0.05), so it is concluded that there was no significant difference in the CoI elements between males and females.
Community of inquiry refers to an individual involved in the inquiry process in a problematic situation related to online learning. There exist many different sub-degree programs available for prospective students, and it is interesting to investigate whether CoI values are different for different programs. These results can provide important insights for the development of scientific literacy in online learning. The statistical analysis was conducted using an independent-sample t-test. The students were categorized based on their study programs (i.e., engineering and business).
The mean scores for different measurement dimensions in CoI are summarized in Table 6. The results have revealed that in online learning, there were no significant differences in social presence for the engineering and business students (p = 0.716 > 0.05), cognitive presence (p = 0.258 > 0.05), or teaching presence (p = 0.091 > 0.05). The mean difference between engineering and business students was −0.032, 0.097, and 0.154 for social presence, cognitive presence, and teaching presence, respectively. A positive value means engineering students having a higher mean score than business students.
Table 6. The statistical analysis of each CoI measurement dimension between engineering and business students.
Because network speed is one of the core elements that enhance experience of students about online learning, further analysis was therefore performed to determine the CoI elements of students for online learning based on their satisfaction levels regarding their network speed. ANOVA was used for statistical analysis. The results are summarized in Table 7. It was found that most of the students, numbering 169 (58.9%), were satisfied with network speed, 87 (30.3%) were neutral, and 31 (10.80%) were not satisfied. On the other hand, the results revealed that the mean scores for all core CoI elements were directly proportional to the network satisfaction levels. The mean score for students satisfied with network speed was significantly higher than that for students who were neutral or not satisfied with network speed at a level of p = 0.000 < 0.01.
The CoI framework has been widely adopted in online learning research for 20 years. Through the CoI framework, we aimed to investigate whether the gender, academic discipline, and network speed of gender are associated with the students; perception of their CoI, for example, as to incorporate new knowledge with their current knowledge (Hilliard and Stewart, 2019). We found a non-statistically significant difference in the CoI elements between males and females, as well as between students enrolled in business and engineering programs. In addition, we found that the network speed is crucial to strengthen the potential of students to create an in-depth and purposeful learning experience (collaborative–constructivist) (Garrison et al., 2000). In other words, it brings a considerable effect on the perceived cognitive presence, social presence, and teaching presence of students.
Gender has been recognized as a key interpreter in forecasting the learning interests and expectations of students (Moses et al., 2016). In previous studies, gender is a determining factor in affecting the academic performance of students. Traditionally, the Internet and computer culture have consorted with men (Cuadrado-García et al., 2010). In this sense, male students incline toward exhibiting superior to female students in associated computer subjects (González-Gómez et al., 2012). Authors like Ong and Lai (2006) and Lu and Chiou (2010) reinforced these findings by stating that female students felt more pessimistic about e-learning than their male counterparts. Furthermore, these authors also identified that e-learning satisfaction and value are lower among female students than among male students. Specifically, the outbreak of COVID-19 has fundamentally transformed from a traditional face-to-face classroom learning method to a new, innovative online learning method. Such an unexpected scenario has completely changed the learning environment of students, making it extremely difficult to predict how the intrinsic individual personality of students could influence their learning outcomes. It is valuable to further investigate the gender differences to inform the design of reactive strategies to support inclusive online learning during this chaotic situation.
Surprisingly, our findings indicated that gender differences are not statistically significant in the CoI elements. In other words, both male and female students had performed at comparable levels in all readiness measurements including behaviors and attitudes toward learner control, independent learning, online communication self-efficacy, the rationale behind learning, and Internet/computer self-efficacy (Hung et al., 2010). The result is similar to previous research (e.g., Kay and Knaack, 2008; Chu, 2010; Cuadrado-García et al., 2010; Hung et al., 2010). To our best knowledge, we found that our survey respondents belong to the generation known as Generation Z or Zoomers. Such generations were birthed start from 1995 (Priporas et al., 2017). Most students of Generation Z have adopted digital technology from a young age. In this sense, they are confident users of social media, innovative technological tools, and the Internet (The Washington Post, 2020). Indeed, Spender (1995) and Garrison et al. (2010a) addressed that abundant computer resources can narrow down the gender gap in online learning. Both males and females have the opportunities to master HTML and Internet protocols at the same level, or use technology with similar approaches (McSporran and Young, 2001). In addition, our female students reflected that they have not encountered the online learning environment problem described by Müller (2008) as “multiple responsibilities, insufficient interaction with faculty, technology, and coursework ranked highest as barriers to women's persistence in online environments” (Müller, 2008, p. 1). As suggested by Cuadrado-García et al. (2010), the higher education institutions design a number of well-structured exercises, provide constant teaching staff support, and integrate the team and individual activities to give fair chances for all students. Such a favorable situation could help explain why our female students score at the same levels as their male peers in the CoI instrument.
Based on Arbaugh et al. (2010) and Arbaugh (2013) studies, business and engineering disciplines are considered as “Hard” and “Applied.” Our research found that students in these two academic disciplines are no different in terms of their CoI scores. This finding suggests that the CoI framework may be appropriate for applied disciplines. According to our research findings, both business and engineering students indicated that cognitive presence, social presence, and teaching presence are important to online learning. Students are assumed to be linear philosophers who are under applied, hard disciplines; our findings propose that teaching activities will be informative and concentrated. Therefore, instructors may require to focus on fostering direct instructions. Business and engineering disciplines come to be harder in orientation; our study suggests that teachers may require performing their position as content experts for students to enrich their learning participation (Arbaugh et al., 2010). In response, Wammes et al. (2016) pointed out that mind-wandering is closely related to levels of task-based motivation. In the context of COVID-19, students could only take video classes. Students indicated that video classes make them in a low motivation for learning and increase in mind-wandering over time. Accordingly, students would take fewer notes, pay low on-task attention, and reduce memory of lecture material. Interestingly, students have a greater degree of mind-wander, and their retention of the information in the lecture is reduced. As a result, students performed poorly on an assessment (e.g., tests, examination). Teachers adopt that a naturalistic teaching approach or contextual learning is notably important (Wammes and Smilet, 2017).
Furthermore, authors like Hayes (2007), Laird et al. (2008), and Arbaugh (2013) explained that students exhibited a proactive player in generating social presence in the hard and applied fields. Based on our study, we propose that teachers may need to create chances of group cohesion and collaboration within the class. To this end, the teacher may produce learning communities of students on LinkedIn, Facebook, Twitter, and WhatsApp to enable students to maintain friendships and obtain valuable learning experiences. To a certain extent, the digital world improved collaboration and supported learning. Moreover, business and engineering disciplines are concentrated on applying latterly obtained knowledge. So, it is sensible that students expect the teacher can help them to build up new knowledge via online learning. Discussion forums and stimulation games online are examples of intellectual activities to generate this kind of knowledge.
The growth of network technology performs global reachability and information dissemination and permits physically segregated various students to study and communicate with each other (Kardan et al., 2013). In doing so, a larger capacity and higher-speed telecommunications systems are crucial to give the means to students to improve common communications of space over distances with different students and teachers (Ichiko et al., 2001) and facilitate pedagogical processes (Johannesen et al., 2012). In various educational research studies, network speed reflects system reliability, Internet quality, and sound technology infrastructure (Sun et al., 2008; Alhabeeb and Rowley, 2018). The higher network speed can motivate students to higher usage of online resources. Accordingly, the high-speed network environment is a “must” of an online learning system (Lee, 2008). However, Rasheed et al. (2020) provided a critical review of literature from 384 research papers. They concluded that the technological sufficiency challenges cannot be overlooked and most of the students were concerned about the possibility that low network speed could affect their online activities. Educators identified that students who have less access to a laptop or Internet access due to home location are the possibility to attain low academic achievement and unable to keep up with their classmates (Pruet et al., 2016). Osorio-Saea et al. (2021) further investigated the effect of COVID-19 on parental engagement across 23 countries. Their home location determines the engagement, acceptance, and confidence of students toward the online learning environment and culture. To our best knowledge, students live in a rural area where there is a poor technological infrastructure or share a single device with siblings. Such an unfavorable situation generates a barrier for students to full engagement in remote learning and connectivity during the pandemic.
Clearly, the growing speed of data transfer and connectivity generates higher opportunities for interfacing within the learning and teaching spaces. Extensive connectivity via Bluetooth, infrared, and WiFi fosters the adoption of mobile devices like laptops, mobile phones, and tablets (Wood and Shirazi, 2020). If adequate network speeds and advanced mobile devices are available, teachers can respond and support discussions that explicate ideas and encourage learning. In this way, network speed fostering teaching presence produces student-centric and energetic learning environments where teachers and students are an equal technological playing field in the learning experience. To a certain extent, network speed encourages teachers to set up learning tasks, timetables, and module content, to monitor and manage intentional reflection and teamwork, and to make sure that students attain the intended learning outcomes by giving real-time information and clear orientation and determining needs (Garrison et al., 2010a).
Currently, higher education institutions are inclined toward establishing student-centric learning environments. Such student-centric learning environments are created by social presence. This generates “a climate that supports and encourages probing questions, skepticism, and the contribution of explanatory ideas” (Garrison, 2017, p. 37). Optimal network speed is expected to optimize learning impact on peer assessment and equip teachers to give constructive and prompt responses about the work of each student. Indeed, high network speed supports important pedagogical characteristics (e.g., online submission, automatic notification, asynchronous and synchronous interaction, online discussion forum, and various kinds of instant feedback, to name but a few; Yu and Wu, 2011). Wood and Shirazi (2020) stated that the majority of students expressed that network speed and different communication formats foster discussion with their classmates. Nevertheless, network speed facilitates social presence to perform group cohesion, open communication, and effective communication. Students who perform a high level of social presence can increase their involvement and participation in the learning environment and collaborate with their classmates to strive toward a common goal (Hilliard and Stewart, 2019).
Under CoI, cognitive presence indicates the inquiry and learning process, including recognizing a task/problem, combining ideas, and analyzing possible solutions. In principle, cognitive presence is anticipated and formed by teaching and social presences (Garrison et al., 2010b). In the research study, we explored that the network speed for online education associated with the perception of students for all key CoI elements. In this sense, the network speed enables students to participate in knowledge creation and critical thinking through sustained communication and reflection. Network speed supports a higher level of learning. In turn, a higher level of learning is a comprehensive multi-based exercise that is connected with an inspiring incident, examination, amalgamation, and intention (Kanuka and Garrison, 2004). Eventually, it can foster the transformation of “metacognitive awareness essential to worthwhile and continued learning” (Garrison, 2017, p. 52). In the online learning process, metacognitive awareness fosters modification backed by the response. It is students have access to instant communication with teachers, facilitated by the network speed (Hilliard and Stewart, 2019). In addition, the network speed can help students minimize interruptions when setting learning goals and enable their learning progress to go smoothly. As a result, students tended to achieve considerable improvement in their learning outcomes when they had metacognitive awareness (Azevedo and Cromley, 2004).
With the effect of COVID-19, the traditional face-to-face teaching approach has been completely replaced by an innovative online teaching pedagogy. Nevertheless, different stakeholders (e.g., teachers, students, and higher education institutions) are under a transition period in a response to the online learning environment. In this context, our study focuses on Hong Kong sub-degree students. We consider that it is important to focus on this modality of education because, as evidenced by the literature, sub-degree students have been seriously overlooked in the past two decades, and because the sub-degree student sector represents a large proportion of the higher education sector in Hong Kong. In our research study, we adopt the CoI framework to explore the association between its three interrelated elements (i.e., cognitive presence, social presence, and teaching presence) and program for students about the study, gender, and satisfaction level of network speed.
Our results show that gender has no significant association with none of the CoI elements: cognitive presence, social presence, and teaching presence. We claim that one of the reasons for this somewhat surprising finding is that members of Generation Z have more positive attitudes toward innovative technological tools, the Internet, and social media. They are, therefore, more comfortable and better prepared suddenly imposed online learning environment (regardless of their gender). Clearly, it is easier for teachers to design and implement courses and to attain learning outcomes when students are more engaged in communication and collaboration during the learning process. In other words, it is easier when students are more capable of knowledge construction and critical thinking (Hilliard and Stewart, 2019). Our findings also suggest that there exists no statistically remarkable distinction neither in cognitive presence, in social presence, nor in teaching presence between students in business and engineering disciplines. The rationale behind this, we claim, is that both disciplines are classified as hard and applied. Previous research has found that as disciplines moved closer to hard and applied approaches, online learning generates a stronger association with the CoI framework (Arbaugh, 2013).
The students who participated in our study declared to be concerned with their online learning in connection with the network speed. As expected, the satisfaction level of students about the network speed for online education has a positive effect on the cognitive presence, social presence, and teaching presence. In practice, the network speed is determined by different factors, for instance, technological tools, online learning software capabilities, study locations, to name but a few. Indeed, the slow network speed induces an interruption of the teaching process, poor online learning experience, and student isolation.
Some limitations for consideration in future research have been explored in our research. Nevertheless, this research study generates a foundation work for future research. First, this research study only focused on the “Hard & Applied” discipline. Further research could include projects that compare students from different disciplines like “Hard & Applied” and “Soft & Pure.” This could contribute to generalize our research findings and deepen our awareness of the associations between the cognitive presence, social presence, and teaching presence of students and their disciplines. Second, our research study only considered online learning pedagogy. Accordingly, a future study could examine different learning pedagogies, for example, conventional face-to-face teaching, blended learning, and innovative online learning modes within the same group of students. This type of project can provide useful guidance and constructive advice to teachers and higher education institutions to design and implement appropriate learning pedagogies for different types of students accordingly, particularly in chaotic times. Third, self-reported data were employed that may be depending on the report of survey respondents accurately and willingness to answer. Students may be not willing to report actual behavior because of insufficient knowledge and possibly personal repercussions. Finally, the data were gathered mainly from students. In the future, we may collect data from numerous stakeholders like government bodies, policymakers, educators, and service providers (i.e., higher education institutions) through focus group discussions to obtain wider perspectives and create thorough data for analysis. As such, mixed research approaches, including quantitative and qualitative, could counteract the pitfalls of a purely quantitative or qualitative research approach.
The raw data supporting the conclusions of this article will be made available by the authors, without undue reservation.
The studies involving human participants were reviewed and approved by the Hong Kong Polytechnic University. Written informed consent to participate in this study was provided by the participants.
Y-yL contributed to the first draft of the article, conception, and design of the study. YT contributed to the first draft of the article, performed the statistical analysis, and organized the database. KC secured the research funding and data collection. LV and AS-H proof read the article and data collection. SW performed data collection. All authors contributed to manuscript revision, read, and approved the submitted version.
The authors declare that the research was conducted in the absence of any commercial or financial relationships that could be construed as a potential conflict of interest.
The authors would like to acknowledge the support of the Macau Higher Education Fund (Ref.: TET-CITYU-2020-04) for this research.
1. ^M-Lab, a nonprofit consortium of researchers and corporations, tasked with documenting the quality of the Internet. More information can be found at: https://www.measurementlab.net/.
Akyol, Z., and Garrison, D. R. (2008). The development of a community of inquiry over time in an online course: understanding the progression and integration of social, cognitive and teaching presence. J. Asynchronous Learn. Netw. 12, 3–22. doi: 10.24059/olj.v12i3.66
Akyol, Z., Garrison, D. R., and Ozden, M. Y. (2009). Online and blended communities of inquiry: Exploring the developmental and perceptional differences. Int. Rev. Res. Open Distance Learn. 10, 65–83. doi: 10.19173/irrodl.v10i6.765
Akyol, Z., Vaughan, N., and Garrison, D. R. (2011). The impact of course duration on the development of a community of inquiry. Interact. Learn. Environ. 19, 231–246. doi: 10.1080/10494820902809147
Alhabeeb, A., and Rowley, J. (2018). E-learning critical success factors: Comparing perspectives from academic staff and students. Comput. Educ. 127, 1–12. doi: 10.1016/j.compedu.2018.08.007
Anderson, T., Rourke, L., Garrison, D. R., and Archer, W. (2001). Accessing teaching presence in a computer conferencing context. J. Asynchron. Learn. Netw. 5:1875. doi: 10.24059/olj.v5i2.1875
Arbaugh, J. B. (2013). Does academic discipline moderate CoI-course outcomes relationships in online MBA courses? Internet Higher Educ. 17, 16–28. doi: 10.1016/j.iheduc.2012.10.002
Arbaugh, J. B., Bangert, A., and Cleveland-Innes, M. (2010). Subject matter effects and the Community of Inquiry (CoI) framework: an exploratory study. Internet Higher Educ. 13, 37–44. doi: 10.1016/j.iheduc.2009.10.006
Azevedo, R., and Cromley, J. G. (2004). Does training on self-regulated learning facilitate students' learning with hypermedia? J. Educ. Psychol. 96, 523–535. doi: 10.1037/0022-0663.96.3.523
Carlon, S., Bennett-Woods, D., Berg, B., Claywell, L., LeDuc, K., Marcisz, N., et al. (2012). The community of inquiry instrument: validation and results in online health care disciplines. Comput. Educ. 59, 215–221. doi: 10.1016/j.compedu.2012.01.004
Choy, J. L. F., and Choon, L. Q. (2016). Modelling relationships between students' academic achievement and community of inquiry in an online learning environment for a blended course. Austr. J. Educ. Technol. 32, 106–124. doi: 10.14742/ajet.2500
Chu, R. J. (2010). How family support and Internet self-efficacy influence the effects of e-learning among higher aged adults - analyses of gender and age differences. Comput. Educ. 55, 255–264. doi: 10.1016/j.compedu.2010.01.011
Cuadrado-García, M., Ruiz-Molina, M. E., and Montoro-Pons, J. D. (2010). Are there gender differences in e-learning use and assessment? Evidence from an interuniversity online project in Europe. Proc. Soc. Behav. Sci. 2, 367–371. doi: 10.1016/j.sbspro.2010.03.027
Dempsey, P. R., and Zhang, J. (2019). Re-examining the construct validity and casual relationships of teaching, cognitive, and social presence in community of inquiry framework. Online Learn. 23, 62–79. doi: 10.24059/olj.v23i1.1419
Dewey, J. (1959). “My pedagogic creed,” in Dewey on Education, ed J. Dewey (New York, NY: Teachers College, Columbia University).
Dhawan, S. (2020). Online learning: A panacea in the time of COVID-19 crisis. J. Educ. Technol. Systems 49, 5–22. doi: 10.1177/0047239520934018
Fiock, H. S. (2020). Designing a community of inquiry in online courses. Int. Rev. Res. Open Distrib. Learn. 21, 135–152. doi: 10.19173/irrodl.v20i5.3985
Garrison, D. R. (2017). E-Learning in the 21st Century: A Community of Inquiry Framework for Research and Practice, 3rd Edition. New York, NY: Routledge.
Garrison, D. R., Anderson, T., and Archer, W. (2000). Critical inquiry in a text-based environment: Computer conferencing in higher education. Internet Higher Educ. 2, 87–105. doi: 10.1016/S1096-7516(00)00016-6
Garrison, D. R., Anderson, T., and Archer, W. (2001). Critical thinking, cognitive presence and computer conferencing in distance education. Am. J. Distance Educ. 15, 7–23. doi: 10.1080/08923640109527071
Garrison, D. R., Anderson, T., and Archer, W. (2010a). The first decade of the community of inquiry model: a retrospective. Internet Higher Educ. 13, 5–9. doi: 10.1016/j.iheduc.2009.10.003
Garrison, D. R., and Arbaugh, J. B. (2007). Researching the community of inquiry model: review, issues, and future directions. Internet Higher Educ. 10, 157–172. doi: 10.1016/j.iheduc.2007.04.001
Garrison, D. R., Cleveland-Innes, M., and Fung, T. S. (2010b). Exploring causal relationships among teaching, cognitive and social presence: Student perceptions of the community of inquiry framework. Internet Higher Educ. 13, 31–36. doi: 10.1016/j.iheduc.2009.10.002
Gene, S., Law, K. M. Y., and Niu, B. (2019). Investigating self-directed learning and technology readiness in blending learning environment. Int. J. Educ. Technol. High Educ. 16:17. doi: 10.1186/s41239-019-0147-0
González-Gómez, F., Guardiola, J., Rodríguez, O. M., and Alonso, M. A. M. (2012). Gender differences in e-learning satisfaction. Comput. Educ. 58, 283–290. doi: 10.1016/j.compedu.2011.08.017
Gutiérrez-Santiuste, E., Rodriquez-Sabiote, C., and Gallego-Arrufat, M. J. (2015). Cognitive presence through social and teaching presence in communities of inquiry: A correlational-predictive study. Austr. J. Educ. Technol. 31, 349–362. doi: 10.14742/ajet.1666
Hair, J. F., Black, W. C., Babin, B. J., and Anderson, R. E. (2010). Multivariate Data Analysis, 7th Edition. Upper Saddle River, NJ: Prentice Hall.
Hayes, S. K. (2007). Principles of finance: Design and implementation of an online course. J. Online Teach. Learn. 3, 460–465.
Heilporn, G., and Lakhal, S. (2020). Investigating the reliability and validity of the community of inquiry framework: An analysis of categories within each presence. Comput. Educ. 145:103712. doi: 10.1016/j.compedu.2019.103712
Henseler, J., Ringle, C. M., and Sarstedt, M. (2005). A new criterion for assessing discriminant validity in variance-based structural equation modeling. J. Acad. Mark. Sci. 43, 115–135. doi: 10.1007/s11747-014-0403-8
Hilliard, L. P., and Stewart, M. K. (2019). Time well spent: creating a community of inquiry in blended first-year writing courses. Internet Higher Educ. 41, 11–24. doi: 10.1016/j.iheduc.2018.11.002
Hooper, D., Coughlan, J., and Mullen, M. (2008). Structural equation modelling: Guidelines for determining model fit. Electr. J. Business Res. Methods 6, 53–60.
Hung, M. L., Chou, C., Chen, C. H., and Own, Z. Y. (2010). Learner readiness for online learning: Scale development and student perceptions. Comput. Educ. 55, 1080–1090. doi: 10.1016/j.compedu.2010.05.004
Iacobucci, D., and Churchill, G. A. (2010). Marketing Research: Methodological Foundations, 10th ed. London: Thomson South Western.
Ichiko, T., Yamamoto, M., Kawamura, Y., and Hanano, M. (2001). Advanced multimedia telecommunications using a high speed broadband backbone network beyond all aspects of the current internet (revised). Comput. Educ. 37, 211–224. doi: 10.1016/S0360-1315(01)00047-1
Jiang, M., and Koo, K. (2020). Emotional presence in building an online learning community among non-traditional graduate students. Online Learn. 24, 93–111. doi: 10.24059/olj.v24i4.2307
Johannesen, M., Erstad, O., and Habib, L. (2012). Virtual learning environments as sociomaterial agents in the network of teaching practice. Comput. Educ. 59, 785–792. doi: 10.1016/j.compedu.2012.03.023
Kanuka, H., and Garrison, D. R. (2004). Cognitive presence in online learning. J. Comput. Higher Educ. 15, 30–49. doi: 10.1007/BF02940928
Kardan, A. A., Sadeghi, H., Ghidary, S. S., and Sani, M. R. F. (2013). Prediction of student course selection in online higher education institutes using neural network. Comput. Educ. 65, 1–11. doi: 10.1016/j.compedu.2013.01.015
Kay, R. H., and Knaack, L. (2008). A formative analysis of individual differences in the effectiveness of learning objects in secondary school. Comput. Educ. 51, 1304–1320. doi: 10.1016/j.compedu.2008.01.001
Laird, T. F. N., Shoup, R., Kuh, G. D., and Schwartz, M. J. (2008). The effects of discipline on deep approaches to student learning and college outcomes. Res. High. Educ. 49, 469–494. doi: 10.1007/s11162-008-9088-5
Lau, Y. Y., Lu, C. S., and Weng, H. K. (2021). The effects of safety delivery and safety awareness on passenger behaviour in the ferry context. Maritime Policy Manage. 48, 46–60. doi: 10.1080/03088839.2020.1750720
Lau, Y. Y., Ng, A. K. Y., Tam, K. C., and Chan, E. K. K. (2018). An investigation on the professionalization of education in maritime logistics and supply chains. Maritime Business Rev. 3, 394–413. doi: 10.1108/MABR-08-2018-0029
Law, K. M. Y., Geng, S., and Li, T. (2019). Student enrollment, motivation and learning performance in a blended learning environment: The mediating effects of social, teaching, and cognitive presence. Comp. Educ. 136, 1–12. doi: 10.1016/j.compedu.2019.02.021
Lee, Y. C. (2008). The role of perceived resources in online learning adoption. Comput. Educ. 50, 1423–1438. doi: 10.1016/j.compedu.2007.01.001
Lin, S., Hung, T. C., and Lee, C. T. (2015). Revalidate forms of presence in training effectiveness: Mediating effect of self-efficacy. J. Educ. Comp. Res. 53, 32–54. doi: 10.1177/0735633115588772
Lu, H., and Chiou, M. (2010). The impact of individual differences on e-learning system satisfaction: A contingency approach. Br. J. Educ. Technol. 41, 307–323. doi: 10.1111/j.1467-8535.2009.00937.x
Ma, Z., Wang, J., Wang, Q., Kong, L., Wu, Y., and Yang, H. (2017). Verifying causal relationships among the presences of the community of inquiry framework in the Chinese context. Int. Rev. Res. Open Distrib. Learn. 18, 213–230. doi: 10.19173/irrodl.v18i6.3197
Mat Yusoff, A., Fan, S. P., Razak, F., and Mustafa, W. (2020). Discriminant validity assessment of religious teacher acceptance: the use of HTMT criterion. J. Phys. Confer. Series 1529:042045. doi: 10.1088/1742-6596/1529/4/042045
McSporran, M., and Young, S. (2001). Does gender matter in online learning? Res. Learn. Technol. 9, 3–15. doi: 10.1080/0968776010090202
Mo, J. P. T., and Tang, Y. M. (2017). Project-based learning of systems engineering V model with the support of 3D printing. Austr. J. Eng. Educ. 22, 3–13. doi: 10.1080/22054952.2017.1338229
Moses, H., Admiraal, W. F., and Berry, A. K. (2016). Gender and gender role differences in student-teachers' commitment to teaching. Soc. Psychol. Educ. 19, 475–492. doi: 10.1007/s11218-016-9340-3
Müller, T. (2008). Persistence of women in online degree-completion programs. Int. Rev. Res. Open Distance Learn. 9, 1–18. doi: 10.19173/irrodl.v9i2.455
Ngai, E. W. T., Lai, K. H., and Cheng, T. C. E. (2008). Logistics information systems: The Hong Kong experience. Int. J. Product. Econ. 113, 223–234. doi: 10.1016/j.ijpe.2007.05.018
Ong, C. S., and Lai, J. Y. (2006). Gender differences in perceptions and relationships among dominants of e-learning acceptance. Comput. Human Behav. 22, 816–829. doi: 10.1016/j.chb.2004.03.006
Osorio-Saea, E. M., Eryilmaz, N., Sandoval-Hernandez, A., Lau, Y. Y., Barahona, E., and Bhatti, A. A. (2021). Survey data on the impact of COVID-19 on parental engagement across 23 countries. Data Brief 35:106813. doi: 10.1016/j.dib.2021.106813
Park, S. Y., Nam, M. W., and Cha, S. B. (2012). University students' behavioral intention to use mobile learning: Evaluating the technology acceptance model. Br. J. Educ. Technol. 43, 592–605. doi: 10.1111/j.1467-8535.2011.01229.x
Priporas, C. V., Stylos, N., and Fotiadis, A. K. (2017). Generation Z consumers' expectations of interactions in smart retailing: A future agenda. Comput. Human Behav. 77, 374–381. doi: 10.1016/j.chb.2017.01.058
Pruet, P., Ang, C. S., and Farzin, D. (2016). Understanding tablet computer usage among primary school students in underdeveloped areas: Students' technology experience, learning styles and attitudes. Comput. Human Behav. 55, 1131–1144. doi: 10.1016/j.chb.2014.09.063
Rasheed, R. A., Kamsin, A., and Abdullah, N. A. (2020). Challenges in the online component of blended learning: A systematic review. Comput. Educ. 144:103701. doi: 10.1016/j.compedu.2019.103701
Rigdon, E. (1996). CFI versus RMSEA: A comparison of two fit indexes for structural equation modeling. Struct. Eqn. Model. 3, 369–379. doi: 10.1080/10705519609540052
Rolim, V., Ferreira, R., Lins, R. D., and Găsević, D. (2019). A network-based analytic approach to uncovering the relationship between social and cognitive presences in communities of inquiry. Internet Higher Educ. 42, 53–65. doi: 10.1016/j.iheduc.2019.05.001
Sangrà, A., Vlachopoulos, D., and Cabrera, N. (2012). Building an inclusive definition of e-learning: An approach to the conceptual framework. Int. Rev. Res. Open Distance Learn. 13, 145–159. doi: 10.19173/irrodl.v13i2.1161
Schumacker, R. E., and Lomax, R. G. (2004). A Beginner's Guide to Structural Equation Modeling (2nd ed.). Mahwah, NJ: Lawrence Erlbaum Associates. doi: 10.4324/9781410610904
Shea, P., and Bidjerano, T. (2009). Community of inquiry as a theoretical framework to foster “epistemic engagement” and “cognitive presence” in online education. Comp. Educ. 52, 543–553. doi: 10.1016/j.compedu.2008.10.007
Shea, P., and Bidjerano, T. (2010). Learning presence: towards a theory of self-efficacy, self-regulation, and the development of a communities of inquiry in online and blended learning environments. Comp. Educ. 55, 1721–1731. doi: 10.1016/j.compedu.2010.07.017
Shea, P., and Bidjerano, T. (2012). Learning presence as a moderator in the community of inquiry model. Comp. Educ. 59, 316–326. doi: 10.1016/j.compedu.2012.01.011
Shea, P., and Bidjerano, T. (2013). Understanding distinctions in learning in hybrid, and online environments: An empirical investigation of the community of inquiry framework. Interact. Learn. Environ. 21, 355–370. doi: 10.1080/10494820.2011.584320
Shea, P., Hayes, S., Uzuner-Smith, S., Gozza-Cohen, M., Vickers, J., and Bidjerno, T. (2014). Reconceptualizing the community of inquiry framework: An exploratory analysis. Internet Higher Educ. 23, 9–17. doi: 10.1016/j.iheduc.2014.05.002
Singh, V., and Holt, L. (2013). Learning and best practices for learning in open-source software communities. Comput. Educ. 63, 98–108. doi: 10.1016/j.compedu.2012.12.002
Sorensen, C. K., and Baylen, D. M. (2009). “Learning online: adapting the seven principles of good practice to a web-based instructional environment,” in The Perfect Online Course: Best Practices for Designing and Teaching, eds A. Orellana, T. L. Hudgins, and M. Samonson (Charlotte, NY: Information Age Publishing).
Spender, D. (1995). Nattering on the Net: Women Power and Cyberspace. Melbourne: Spinifex Press. doi: 10.3138/9781442602762
Stenbom, S. (2018). A systematic review of the community of inquiry survey. Internet Higher Educ. 39, 22–32. doi: 10.1016/j.iheduc.2018.06.001
Sun, P. C., Tsai, R. J., Finger, G., Chen, Y. Y., and Yeh, D. (2008). What drives a successful e-Learning? An empirical investigation of the critical factors influencing learner satisfaction. Comp. Educ. 50, 1183–1202. doi: 10.1016/j.compedu.2006.11.007
Swan, K., Day, S. L., Bogle, L. R., and Matthews, D. B. (2014). A collaborative, design-based approach to improving an online program. Internet Higher Educ. 21, 74–81. doi: 10.1016/j.iheduc.2013.10.006
Swan, K., Matthews, D. B., Bogle, L. R., Boles, E., and Day, S. L. (2012). Linking online course design and implementation to learning outcomes: A design experiment. Internet Higher Educ. 15, 81–88. doi: 10.1016/j.iheduc.2011.07.002
Tang, Y. M., Chen, P. C., Law, K. M., Wu, C. H., Lau, Y.-,y., Guan, J., et al. (2021). Comparative analysis of student's live online learning readiness during the coronavirus (COVID-19) pandemic in the higher education sector. Comput. Educ. 168, 1–17. doi: 10.1016/j.compedu.2021.104211
Tang, Y. M., and Yu, K. M. (2018). Development and evaluation of a mobile platform for teaching mathematics of CAD subjects. Comput. Aided Des. Appl. 2, 164–169. doi: 10.1080/16864360.2017.1375665
The Washington Post (2020). Available online at: https://www.washingtonpost.com/business/2020/05/27/millennial-recession-covid/ (accessed June 15, 2020).
Venkatesh, V., Morris, M. G., Davis, G. B., and Davis, F. D. (2003). User acceptance of information technology: toward a unified view. MIS Q. 27, 425–478. doi: 10.2307/30036540
Wammes, J. D., Seli, P., Cheyne, J. A., and Boucher, P. (2016). Mind wandering during lecture II: Relation to academic performance. Scholarship Teach. Learn. Psychol. 2, 33–48. doi: 10.1037/stl0000055
Wammes, J. D., and Smilet, D. (2017). Examining the influence of lecture format on degree of mind wandering. J. Appl. Res. Memory Cognit. 6, 174–184. doi: 10.1016/j.jarmac.2017.01.015
Wheaton, B. (1987). Assessment of fit in overidentified models with latent variables. Sociol. Methods Res. 16, 118–154. doi: 10.1177/0049124187016001005
Wood, R., and Shirazi, S. (2020). A systematic review of audience response systems for teaching and learning in higher education: the student experience. Comp. Educ. 153:103896. doi: 10.1016/j.compedu.2020.103896
Yu, F. Y., and Wu, C. P. (2011). Different identity revelation modes in an online peer-assessment learning environment: effects on perceptions toward assessors, classroom climate and learning activities. Comp. Educ. 57, 2167–2177. doi: 10.1016/j.compedu.2011.05.012
Zhu, M., Herring, S. C., and Bonk, C. J. (2019). Exploring presence in online learning through three forms of computer-mediated discourse analysis. Distance Educ. 40, 205–225. doi: 10.1080/01587919.2019.1600365
Keywords: online learning, community of inquiry, sub-degree students, network speed, gender, academic discipline, COVID-19
Citation: Lau Y-y, Tang YM, Chau KY, Vyas L, Sandoval-Hernandez A and Wong S (2021) COVID-19 Crisis: Exploring Community of Inquiry in Online Learning for Sub-Degree Students. Front. Psychol. 12:679197. doi: 10.3389/fpsyg.2021.679197
Received: 26 March 2021; Accepted: 18 June 2021;
Published: 22 July 2021.
Edited by:
David Gonzalez-Gomez, University of Extremadura, SpainReviewed by:
Suzanne Fergus, University of Hertfordshire, United KingdomCopyright © 2021 Lau, Tang, Chau, Vyas, Sandoval-Hernandez and Wong. This is an open-access article distributed under the terms of the Creative Commons Attribution License (CC BY). The use, distribution or reproduction in other forums is permitted, provided the original author(s) and the copyright owner(s) are credited and that the original publication in this journal is cited, in accordance with accepted academic practice. No use, distribution or reproduction is permitted which does not comply with these terms.
*Correspondence: Ka Yin Chau, Z2F2aW5jaGF1QGNpdHl1Lm1v
Disclaimer: All claims expressed in this article are solely those of the authors and do not necessarily represent those of their affiliated organizations, or those of the publisher, the editors and the reviewers. Any product that may be evaluated in this article or claim that may be made by its manufacturer is not guaranteed or endorsed by the publisher.
Research integrity at Frontiers
Learn more about the work of our research integrity team to safeguard the quality of each article we publish.