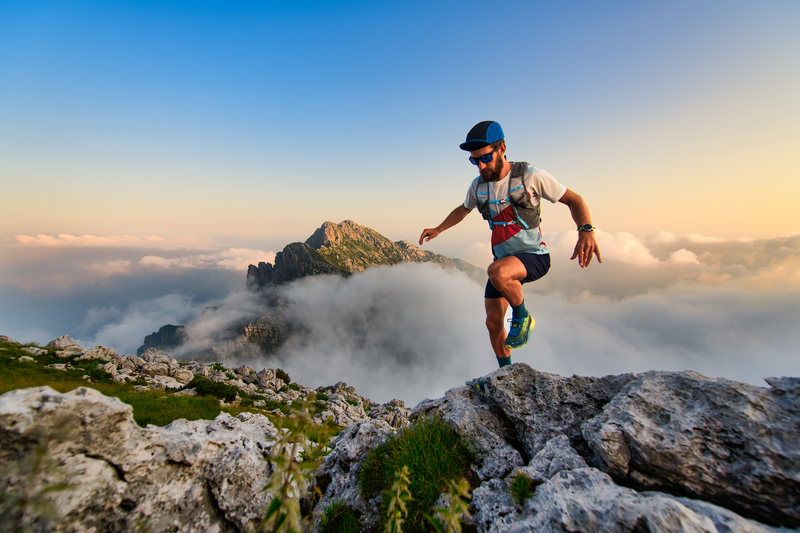
95% of researchers rate our articles as excellent or good
Learn more about the work of our research integrity team to safeguard the quality of each article we publish.
Find out more
ORIGINAL RESEARCH article
Front. Psychol. , 17 June 2021
Sec. Educational Psychology
Volume 12 - 2021 | https://doi.org/10.3389/fpsyg.2021.663202
This article is part of the Research Topic Cognitive, Affective, Behavioral and Multidimensional Domain Research in STEM Education: Active Approaches and Methods towards Sustainable Development Goals (SDGs) View all 16 articles
Schools promote all-round education for each of their students. This requires teachers to work on all of the possibilities offered by a subject, including mathematical ability. This process of adjustment and individualization is essential for students who have excellent performance or aptitudes. This study uses an ex post facto, descriptive and quantitative research methodology to examine the results of giving the online version of the Evaluation Battery for Mathematical Ability (BECOMA On) to 3795 5th-year primary school students. The sample was selected from 147 Spanish schools from 16 autonomous regions and 2 autonomous municipalities. Three levels of performance were identified, 3 being the highest, and different statistical indices were calculated for each of them. The results were also analyzed according to sex, with statistically significant differences in the highest performance level. In addition, the study highlighted a diagnostic gap in the identification of higher capacity students, a pending challenge for education systems for the educational inclusion of all students.
Educational processes nowadays are characterized by homogeneity and multidimensionality, which makes it difficult to deal with the diverse potentials, needs, and interests in the classroom. Occasionally, there may also be a lack of diagnostics that would allow for the modified, individualized educational responses which are common for students who are highly capable and have high aptitudes (García-Perales and Almeida, 2019). Discovering and working on talent should be a basic objective in an advanced society, and generalizing the detection process and targeting it at the entire school population would be an interesting way of achieving that aim. This study presents an example of that in the field of mathematics. The process allows various situations to be addressed flexibly based on specific student characteristics in order to encourage each student’s cognitive abilities to the highest level.
Mathematics is important because it is applicable in daily life and in solving various types of problems (Cázares et al., 2020), as well as having interdisciplinary connections to other parts of the curriculum (Gilat and Amit, 2013). This generalization to routine everyday contexts is a fundamental aspect of being included as a key skill in education (Méndez et al., 2015). In the case of mathematics, it is included in maths competency and basic competencies in science and technology. Maths competency is defined as “students’ ability to formulate, apply, and interpret mathematics in various contexts. It includes mathematical reasoning and using mathematical concepts, procedures, facts, and tools to describe, explain, and predict various kinds of phenomena” (Ministerio de Educación y Formación Profesional, 2019, p. 17). This definition provides a key aspect of maths evaluation, the measurement of mathematical ability in a broad range of contexts, with a view to highlighting the importance of generalizing what has been learned to a wide variety of situations, familiar or otherwise. The search for constructive, committed, reflective citizenship is a fundamental premise of educational processes, aspects which maths teaching has a strong influence over (Organization for Economic Cooperation, and Development, 2019b). Maths competence has been evaluated in all six editions of the Program for International Student Assessment (PISA) every three years from 2000 to 2018.
The PISA assessments are a fundamental reference for evaluation. The fact that there is a large, worldwide sample for the PISA tests means that the conclusions are extremely important in the development of education policy. Its conceptual framework has been used in many studies (García-Perales, 2014; Ferreira et al., 2017; Rodríguez-Mantilla et al., 2018; Fuentes and Renobell, 2019; Sason et al., 2020). The distinctive characteristics of PISA include (Ministerio de Educación y Formación Profesional, 2019): seeking to guide educational policies, integration of the concept of competence in assessment, the important role of autonomous and lifelong learning, regular deployment, and sensitive international coverage. When interpreting results for each item, PISA uses Item Response Theory (IRT). In this regard, children’s answers are considered according to the child’s level of ability in mathematical competence, in other words, estimates of student performance focus on the type of mathematical tasks that they can solve correctly (Ministerio de Educación y Formación Profesional, 2019). This means performance levels can be identified that allow each child to be placed on a continuous scale of competence for the measured construct (Roderer and Roebers, 2013), showing the percentage of subjects in each level together with their distinctive characteristics, in this case for mathematical competence. This methodology was used with the BECOMA On, the instrument in the present study, in which three performance levels were set based on the scores.
Among the many conclusions from PISA relating to mathematics, reports have stressed that students’ interest in and enjoyment of this area is low, and even noted the presence of personal issues such as anxiety and lack of confidence, especially in girls (Organization for Economic Cooperation, and Development, 2013; Mizala et al., 2015; Organization for Economic Cooperation and Development, 2019d). Throughout the PISA assessments, boys have always had better results than girls in mathematical competence (Ministerio de Educación y Formación Profesional, 2019), with sex being a predictor variable of mathematical performance (Farfán and Simón, 2017; Fuentes and Renobell, 2019; Palomares-Ruiz and García-Perales, 2020). Biological and social factors may act in an interrelated way (Chamorro-Premuzic et al., 2009; Muelas, 2014), including intellectual capacity (Schillinger et al., 2018), complex mathematical reasoning (Desco et al., 2011) and other factors of an individual nature with an impact on the mathematical learning process (Song et al., 2010; Marsh and Martin, 2011; Rodríguez and Guzmán, 2018), school (Carey et al., 2016; Dowker et al., 2016; Schillinger et al., 2018), and family (Pelegrina et al., 2002; Ferreira et al., 2017; Rodríguez-Mantilla et al., 2018). Analyzing students’ mathematical performance according to sex is one objective of the present study.
The results for mathematics performance in the 2012 PISA tests—the most recent that evaluated mathematics preferentially—and the 2018 tests—the most recent evaluation—are summarized briefly below. In PISA, student results are ranked in seven performance levels: below level 1, 1, 2, 3, 4, 5, and 6. In PISA 2012, Spanish boys scored an average of 492 points and Spanish girls averaged 476 points (Ministerio de Educación, Cultura y Deporte, 2014a). In PISA 2018, Spanish boys averaged 485, while the girls averaged 478. In both cases, the differences between sexes were statistically significant (Ministerio de Educación y Formación Profesional, 2019). Examining these differences more closely, the results for the highest levels 5 and 6 stand out; in PISA 2018, 8% of boys and 5.50% of girls were in one of these two levels. Other studies have also indicated these differences between the sexes in performance and higher ability (Llor et al., 2012; García-Perales and Almeida, 2019; Ministerio de Educación y Formación Profesional, 2020; Palomares-Ruiz and García-Perales, 2020). In contrast, 24.60% of boys and 24.80% of girls were in the lowest levels—1 and below 1—with no statistical significance between the sexes (Ministerio de Educación y Formación Profesional, 2019). As Figure 1 shows, at the higher performance levels the differences between the sexes begin to be more significant, with more boys than girls in those higher levels of mathematics performance (something which is also seen in the OECD average). This is an issue that raises concerns about the potential consequences for future academic and professional choices.
Figure 1. Performance levels and gender in PISA 2018 for Spain and the OECD. Source: Ministerio de Educación y Formación Profesional (2019), p. 89.
Continuing to look at children with excellent performance in mathematics, in PISA 2012, 8% of Spanish students exhibited excellent performance (6.70% and 1.30% in the two top performing groups), similar figures to previous editions of PISA for mathematics skills, whereas the OECD average was 9.30% and 3.30% in the top two groups (Instituto Nacional de Evaluación Educativa, 2013). In PISA 2018, 6% and 1% of Spanish students were in the top two groups, whereas the OECD mean was 9% and 2% respectively (Ministerio de Educación y Formación Profesional, 2019; Organization for Economic Cooperation, and Development, 2019a,b,c). This raises a fundamental question. In Spain do we not have high performing students? or is our own system not capable of identifying and cultivating them?
What makes a student highly capable at mathematics? PISA 2018, the most recent version, set out the following characteristics for achievement level 6 or higher for maths skills (Ministerio de Educación y Formación Profesional, 2019, p. 64):
“They know how to formulate concepts, generalize and use information based on their research and model complex problems, and they can use their knowledge in relatively atypical contexts. They can simultaneously relate different sources of information and representations, and switch between them flexibly. Students at this level have a high level of mathematical thinking and reasoning. These students can apply this comprehension, as well as their mastery of mathematical operations and symbolic, formal relationships to develop new approaches and strategies to address new situations. Students at this level can consider their actions, and precisely formulate and communicate their actions and thinking about their discoveries, interpretations, arguments, and adaptation to novel situations.”
Other research also influences the conceptualization of the most mathematically capable children (Geary and Brown, 1991; Greenes, 1997; Sriraman, 2003; Rotigel and Fello, 2004; Almeida et al., 2008; Desco et al., 2011; Jaime and Gutiérrez, 2017; Kurnaz, 2018; Ramírez and Cañadas, 2018). Within the field of higher abilities, it is worth paying particular attention to the female population. For example, in Spain, the percentages diagnosed as highly able in school year 2018/19 varied considerably by sex, 65.06% of those identified were boys and 34.94% were girls (Ministerio de Educación y Formación Profesional, 2020). Girls are a higher risk group among the highly able, the identification processes are more detrimental to them (Kerr, 2000; Landau, 2003; Jiménez, 2014) and stereotypes abound (Bian et al., 2017). In addition, even nowadays there are still inequalities in the socialization processes between the sexes (Hadjar et al., 2014; Ministerio de Educación y Formación Profesional, 2019), and girls’ potentials are occasionally undervalued (Pomar et al., 2009). UNESCO (2019, p.72) stated that “the disadvantaging of girls is not based on cognitive ability, but rather on the processes of socialization and learning they grow up with, which shape their identities, beliefs, behaviors, and life choices.”
There is research into maths competency indicating that boys tend to get better results (Preckel et al., 2008; Llor et al., 2012; Instituto Nacional de Evaluación Educativa, 2013; Ministerio de Educación y Formación Profesional, 2019) despite both sexes receiving similar mathematics teaching from the beginning of schooling. Perceptions of and attitudes toward mathematics are particularly important (González-Pienda et al., 2012; Mato et al., 2014; Ministerio de Educación, Cultura y Deporte, 2014b; Preckel et al., 2008; Ministerio de Educación y Formación Profesional, 2019; Cueli et al., 2020; Palomares-Ruiz and García-Perales, 2020), girls can exhibit anxiety and lack confidence in this area (Instituto Nacional de Evaluación Educativa, 2013; Rodríguez-Mantilla et al., 2018). It is essential to consider girls’ levels of attention or execution rates in approaching mathematical tasks (Boaler, 2016; Farfán and Simón, 2017; Hattie et al., 2017; Rodríguez and Guzmán, 2018; Cueli et al., 2020), as well as other motivational and emotional factors (Else-Quest et al., 2010; Rodríguez-Mantilla et al., 2018). Teacher training and practice must consider these discrepancies between aptitude and attitude toward mathematics (Nortes and Nortes, 2013; Rico et al., 2014; Ursini and Ramírez-Mercado, 2017). This variable is a key determiner of educational success in any academic discipline. The more interested students are and the more they believe learning mathematics to be a useful source of knowledge, the better their performance will be (Figueiredo and Guimarães, 2019). This becomes even more important when changing educational stages in the face of deteriorating attitudes toward learning (Mato et al., 2014). Self-efficacy also influences educational development and is a key variable to consider in students’ individual adjustment in the area of mathematics (Ruiz, 2005; Zalazar et al., 2011; Rosário et al., 2012). Better and deeper understanding of these attitudinal and motivational aspects is an essential challenge for mathematics teaching.
Understanding the dimensions that can have an impact on men’s and women’s educational paths is key and affects future academic and professional choices (Hadjar et al., 2014; UNESCO, 2019; García-Perales et al., 2021). It is essential to try to extrapolate from research to answer the question; why is there a difference in the choice of scientific and technical careers between men and women? This study focuses on mathematics, although the same challenge applies to other disciplines such as Science, Technology and Engineering. The goal is to achieve an equal, equitable educational system that allows all students to meet the changing demands of the globalized 21st century society (Ryu et al., 2021), regardless of gender, because there is currently a gender gap in these disciplines (Kijima and Sun, 2020).
The objective of this study was to analyze the results in the BECOMA On from students in the three levels of mathematics achievement. In order to understand and conceptualize these levels of performance, the results were examined in relation to the participants’ sex.
The study used an ex post facto, descriptive, quantitative research methodology with the aim of describing the relationships that exist between groups of quantitative data from a series of modulating variables.
The study sample was made up of 3795 5th year primary school students, aged around 10-11 years old, from 16 regions in Spain. Each regional education authority selected the schools to participate voluntarily in the study, depending on the schools’ availability to participate and them having suitable technological tools for performing the study. Instruments were applied to class groups in their usual classrooms using online devices. The distribution of the sample by sex was 2002 boys (52.75%) and 1793 girls (47.25%).
The sample was grouped by levels of performance. Based on the results, 3 similarly sized hierarchical levels were set, with 1 being the lowest and 3 being the highest performance. The levels for the BECOMA On are shown in Table 1.
The mean level was 1.97 (SD = 0.82), with asymmetry of.05, the distribution of the levels followed a symmetric curve, with kurtosis of -1.50, platykurtic distribution with negative excess kurtosis.
Mathematics competence was the main variable in this study. It was measured using the BECOMA On. As mentioned above, mathematics has a key role in educational processes, particularly because of its generalization to subjects’ daily lives, a fundamental aspect for effective, autonomous development in society. The other variable used was the participants’ sex, male (M) or female (F).
The BECOMA On is a battery that evaluates mathematical skills in 5th year primary schoolchildren online. It is made up of 30 items spread over 7 evaluation tests: Mathematical interpretation (Items 1-5; Statistics and Probability Dimension), Mental arithmetic (Items 6-11; Arithmetic Dimension), Geometrical properties (Items 12 and 13; Geometry Dimension), Logical numerical series (Items 14-19; Arithmetic Dimension), Discovering algorithms (Items 20 and 21; Arithmetic Dimension), Conventional units (Items 22-27; Magnitudes and Proportionality Dimension), and Logical series of figures (Items 28-30; Geometry Dimension). In establishing the content and evaluation indicators for the items in each dimension, Royal Decree 126/2014, of February 28, which establishes the basic curriculum for Primary Education, was used as a reference (Ministerio de Educación, Cultura y Deporte, 2014c). The instrument is structured as in Table 2.
Each item has a possible score of 0 (wrong), 1 (partially correct), or 2 (correct), giving a possible overall minimum score of 0 and a possible overall maximum score of 60. It takes 41 minutes to do the test. In terms of statistical validity (Palomares-Ruiz and García-Perales, 2020), the instrument had a reliability index of 0.83 using Cronbach’s Alpha, and validity indices between.78 and.86 (content and construct). The Difficulty Index (DI) for each item was as follows:
As Table 3 shows, the battery had a moderate difficulty index (DI = 0.45) and appeared reactive to various levels of difficulty. Item 28 was the most difficult (DI = 0.09) while item 13 was the easiest (DI = 0.75). Item selection was judged by a group of 51 professionals in mathematics from various educational stages, giving an overall validity index for the instrument of 0.81 and a Kappa statistic of 0.82.
A month before the data collection period, staff at each of the participating schools were given a training course covering the differential characteristics of the battery, and what they had to consider when applying it, with instructions and monitoring times. Data was collected throughout February 2019 through the online application of the instrument.
Consent was obtained from each participating student’s parents or guardians for them to take part in the study, requested on the researchers’ behalf by the director in each school. Subsequently, a list of children with family authorization was kept by the educational administration in each Spanish region.
Before presenting the results according to the study objectives, the descriptive statistics are presented for each item in the instrument: mean, standard deviation, frequencies and percentages.
As Table 4 indicates, the level of difficulty can be analyzed according to the average results from each item. The easiest items were Items 4 (M = 1.49, SD = 0.75), 5 (M = 1.54, SD = 0.71), 7 (M = 1.58, SD = 0.67), 13 (M = 1.61, SD = 0.72), and 14 (M = 1.63, SD = 0.65), and the most difficult items were 11 (M = 0.74, SD = 0.84), 16 (M = 0.81, SD = 0.77), 17 (M = 0.80, SD = 0.76), 21 (M = 0.82, SD = 0.89), and 28 (M = 0.56, SD = 0.65). The mean for the battery set was 34.83 (SD = 9.69). Figure 2 shows the item with the lowest difficulty level—number 14—and Figure 3 shows the item with the highest difficulty—number 28.
Figure 3. Item 28, the most difficult item in the instrument in this study. Authors’ own work (2020).
In terms of asymmetry, negative scores predominated—21 of the 30 items—, in other words more values appeared to the left of the mean. In terms of kurtosis, almost all the values—27 of the 30 items and the total score—were negative, a platykurtic distribution with a lower concentration of results around the mean, an interesting aspect when analyzing different levels of performance according to the results.
The results are presented based on the study objectives, first the results in the BECOMA On for the three performance levels and then the descriptive statistics. Following that, each level is examined in relation to sex.
Table 5 shows the results in the BECOMA On for the three performance levels. The frequency and percentages for each performance level are given. There were 1319 students (34.76%) in level 1, 1263 (33.28%) in level 2 and 1213 (31.96%) in level 3.
Table 5 gives the totals for each level and response option. In the mean results for all items, 15.45% of the students were in level 1 and scored 0, 10.25% were in level 1 and scored 1, and 9.06% were in level 1 and scored 2; 9.09% of students were in level 2 and scored 0, 9.63% were in level 2 and scored 1, and 14.56% were in level 2 and scored 2; 3.99% of the students were in level 3 and scored 0, 6.97% were in level 3 and scored 1, and 21.01% were in level 3 and scored 2. Level 1 and 2 student responses were more erratic and reflected a clear difference between levels. To determine statistically significant differences, Table 6 shows the means, standard deviations and the results of the ANOVA test.
The students in level 1 had a mean score of 24.49 (SD = 4.67), in level 2 the mean score was 34.93 (SD = 2.58), and in level 3 it was 45.97 (SD = 4.76). The students in level 3 had higher mean scores in all items. Furthermore, the differences between levels were statistically significant in all items, p < 0.001, with the level 3 students scoring higher. To complete the characterization of these three groups of students, another variable, students’ sex, was used for comparison between levels.
The sex distribution of the original sample of 3795 students was 2002 boys (52.75%) and 1793 girls (47.25%). The mean score in the instrument for boys was 35.18 (SD = 10.08) and for girls it was 34.44 (SD = 9.22), with a p-Value < 0.05. To more closely examine the significance of the differences between the sexes, the results were analyzed according to each level of performance. The frequencies and percentages for each sex in each of the three levels are given below.
At level 1 performance, there was little difference in the proportions for each score (Table 7): 23.12% of the responses were boys scoring 0, 15.68% were boys scoring 1, and 13.21% were boys scoring 2; 21.33% of the responses were girls scoring 0, 13.80% were girls scoring 1, and 12.86% were girls scoring 2. Looking at the frequencies of scores of 2 for both sexes, there were differences. There were more boys scoring 2 in items 15 (boys 5.69% and girls 3.26%), 20 (boys 17.44% and girls 14.94%), 22 (boys 25.78% and girls 22.52%), and 27 (boys 17.66% and girls 13.34%). More girls scored 2 in items 1 (boys 20.55% and girls 22.21%), 7 (boys 20.70% and girls 23.28%), 8 (boys 12.66% and girls 14.25%), and 12 (boys 23.43% and girls 25.02%).
For the level 2 students, the frequencies and percentages for each response option were as follows (Table 8):
At level 2 the results were similar to level 1 in terms of sex, with small differences between boys and girls. 13.53% of responses were boys scoring 0, 14.98% were boys scoring 1, and 21.84% were boys scoring 2; 13.78% were girls scoring 0, 13.95% were girls scoring 1, and 21.91% were girls scoring 3. Looking at the frequencies of scores of 2 for each item, there were also differences between the sexes. More boys scored 2 in items 15 (boys 16.94% and girls 9.74%), 17 (boys 8.71% and girls 5.38%), 18 (boys 16.31% and girls 12.67%), and 19 (boys 15.04% and girls 11.80%). More girls scored 2 in items 7 (boys 35.79% and girls 39.75%), 8 (boys 26.68% and girls 30.64%), 23 (boys 21.06% and girls 25.65%), and 30 (boys 22.33% and girls 27.40%).
For the level 3 students, the frequencies and percentages for each response option were as follows (Table 9):
At level 3 there were greater differences between the sexes, with boys scoring higher than girls. 6.78% of responses were boys scoring 0, 11.89% were boys scoring 1, and 37.38% were boys scoring 2; 5.70% of responses were girls scoring 0, 9.91% were girls scoring 1, and 28.33% were girls scoring 2. Looking at the scores of 2 for each item, there were large differences between the sexes. This was notable in items 15 (boys 39.57% and girls 20.86%), 19 (boys 34.05% and girls 20.61%), 22 (boys 53.01% and girls 39.32%) and 27 (boys 44.44% and girls 31.90%). At this level, the differences were smaller in items 3 (boys 34.46% and girls 29.51%), 26 (boys 23.99% and girls 18.14%), 28 (boys 7.67% and girls 6.92%), 29 (boys 25.47% and girls 23.50%) and 30 (boys 39.24% and girls 35.37%). There were no items in which more girls scored 2 than boys.
Once the frequencies were established for each level by sex, a t-test was performed to determine whether there were statistically significant differences according to sex. The results are given in Table 10.
As Table 10 shows, there were statistically significant differences at p < 0.05. This significance was due to an unequal frequency between the sexes at performance level 3, where there were 680 boys (17.92%) and 533 girls (14.04%). In the other two levels, 1 and 2, the results were more similar, level 1 included 686 (18.08%) boys and 633 (16.68%) girls, while level 2 included 636 boys (16.76%) and 627 girls (16.52%). According to statistics from the Ministry for Education (Ministerio de Educación y Formación Profesional, 2020) for non-university education in school year 2018/19 (the most recent available data), of the 35494 students identified as highly capable, 23092 were boys (65.06%), and 12402 were girls (34.94%). This reflects a continuing disparity between the sexes in the identification of highly capable students, with the diagnostic process being detrimental to girls. This indicates an inequality in education and the need to examine causal factors more deeply.
Society’s scientific and technological progress requires highly qualified professionals (Frey and Osborne, 2017) as there are constant innovations and shifting requirements (Macià and Garreta, 2018). Schools are fundamental in developing students’ talents (Mandelman et al., 2010). The most recent Spanish education laws address educational needs, and addressing and adapting to students’ needs from the very beginnings of schooling is fundamental. Equality and innovation promote quality and social development (Organization for Economic Cooperation, and Development, 2019a), and evaluation and research help monitor them in order to establish educational policies (Schleicher, 2018; Harju-Luukkainen et al., 2020).
This study focused on the analysis of mathematics skills in the three groups of students identified by performance following the application of the BECOMA On, an instrument with high indices of reliability and validity. Understanding the potential of the students in these three levels is of significant social and educational interest and understanding the complexity of the mathematical approaches and strategies they use in problem solving is fundamental (Jaime and Gutiérrez, 2017). Initially, the results were as expected, students in the highest level—3—demonstrated better results and assessments than students in levels 1 and 2. What is interesting is the presence of various statistically significant differences.
Just over a third, 1319 students (34.76%), were identified as belonging to performance level 1, 1263 (33.28%) to level 2 and 1213 (31.96%) to level 3. Comparing the results of these three groups, statistically significant differences were found, p < 0.001; level 3 students had higher scores in all of the items in the instrument. Level 3 students were the most capable in mathematics. It is important to consider the processes for identifying these highly capable students. From time to time, unfortunately, their potentials, needs, and interests seem to be neglected in the learning and teaching process, and occasionally there are various serious adaptation problems (Pomar et al., 2009; García-Perales and Almeida, 2019).
To complete the characterization of these three groups of students, the levels were compared in relation to students’ sex. In the study, 52.75% of the participating sample were boys and 47.25% girls. In performance levels 1 and 2, there were few discrepancies in performance between the sexes, with varying differences in favor of one sex or the other. However, at level 3, there were greater differences, and it was the boys who had the highest scores in all items. Boys in level 3 had 6.78% of responses scoring 0, 11.89% scoring 1, and 37.38% scoring 2. For the girls in level 3, the percentages were 5.70%, 9.91% and 28.33% for scores of 0, 1, and 2 respectively. This resulted in statistically significant differences, p < 0.05, since at performance level 3 or higher, there were 680 boys (17.92%) compared to 533 girls (14.04%). This reflects a continuing disparity between the sexes in the higher achievement levels for Mathematics, also seen in other research (Baye and Monseur, 2016; Hyde, 2016; Ministerio de Educación y Formación Profesional, 2019), demonstrating an inequality in education and the need to examine causal factors in depth (Calvo, 2018).
In short, the instrument used is functional and original because it establishes a relationship between assessment and mathematical and digital skills. Its close connection with the Spanish school curriculum for the 5th grade of primary education gives it a valuable practical component for use in developing educational practices. The detection of learning needs and potentials, in this case for mathematics using online evaluation, is key because of mathematics’ instrumental and interdisciplinary nature, and it opens up an interesting path for the generalization and application of such instruments.
Schools must develop educational practices that allow inclusive, quality education for all (Franco et al., 2017; Arnaiz-Sánchez et al., 2018, 2020). Educational administrations must ensure all students achieve functional and meaningful learning, making it a priority to support the existence of equitable, democratic schooling adjusted to each student’s needs and characteristics. Educational policies must be directed toward achieving this end. In this regard, it is essential to consider all the variables that influence the teaching and learning processes, including student sex (Hadjar et al., 2014; Farfán and Simón, 2017; Palomares-Ruiz and García-Perales, 2020), with a view to rethinking actions to foster improvement in academic performance and to promote innovation in education.
As noted in the introduction, biological factors, such as intelligence or certain personality traits, and contextual factors, such as stereotypes and the family itself, may explain differences between the sexes in mathematical performance, especially at higher performance levels. In this regard, analyzing the contexts in which boys and girls socialize is fundamental for studying these differences between the sexes (Hadjar et al., 2014; Mizala et al., 2015; Palomares-Ruiz and García-Perales, 2020), an issue that should be approached from various perspectives (Del Río et al., 2016). In addition, the differences between the sexes highlight the need to rethink educational practices from the perspective of equality and innovation, trying to prevent mathematical learning from leading to academic and professional segregation (Cantoral et al., 2014). In this regard, working on STEAM skills (Science, Technology, Engineering, Arts and Mathematics) may be a useful approach for promoting coeducation and gender equality in education (UNESCO, 2019; Ryu et al., 2021), including non-formal education (Juvera and Hernández-López, 2021), and may be generalizable to highly capable mathematics students (García-Perales and Almeida, 2019). Teacher training in teaching mathematics is especially important (Monroy and Marroquín, 2020) and is a key aspect for teaching and learning in the other STEAM fields (Román-Graván et al., 2020; Hernández-Barco et al., 2021; Ortiz-Revilla and Greca, 2021), in which women are underrepresented (Lehman et al., 2017; Botella et al., 2019; McCullough, 2020).
The large sample participating in this study underlines the importance of using ability tests for diagnostic processes, in this case for Mathematics. The generalization of specific activities for any schoolchild, whatever their abilities, means starting a process of educational adaptation and individualization (Díez and Jiménez, 2018; Torres, 2018). Currently, educational processes are characterized by their complexity and multidimensionality, with multiple factors that can have an impact on teaching and learning as part of mathematics teaching (Palomares-Ruiz and García-Perales, 2020). For this reason, it would be advisable to expand the variables of analysis in future studies with BECOMA On, and include variables such as academic performance, teachers’ and students’ perceptions of students’ interest in and motivation for mathematics, and whether highly capable students are detected. In addition, future studies will seek to generalize the application of this instrument to other educational levels, the sex of the students will be a fundamental variable. Generalizing studies for this variable to other educational levels would add weight to the results from this study. In addition, attempts will be made to perform repeated-measure replication study designs, similar to those used in other studies, using the written version of this instrument (García-Perales et al., 2020, 2021). Identifying any student’s potential for mathematics helps to offer an individualized educational response, which is a priority of inclusive, high-quality education.
The original contributions presented in the study are included in the article/supplementary material, further inquiries can be directed to the corresponding author/s.
Ethical review and approval was not required for the study on human participants in accordance with the local legislation and institutional requirements. Before the study began, written informed consent to participate in this study was provided by the regional administration of each school. These educational institutions did require written informed consent from the parents. We ensured the anonymity of the responses and the confidentiality of all data collected, with published results not containing any school identifying information.
RG designed the study, collected and analyzed the data, and wrote the manuscript. AP contributed to the interpretation of the data and wrote, revised, and refined the manuscript. RG and AP have participated in sending the article to the journal. Both authors contributed to the article and approved the submitted version.
The study was supported economically by the Castilla-La Mancha Regional Government (JCCM) and the European Regional Development Fund (FEDER) [Project Reference/Code SBPLY/19/180501/000149]. It has also had the support of the National Institute of Educational Technologies and Teacher Training (INTEF), the National University of Distance Education (UNED), and the University of Castilla-La Mancha (UCLM).
The authors declare that the research was conducted in the absence of any commercial or financial relationships that could be construed as a potential conflict of interest.
Almeida, L. S., Guisande, M. A., Primi, R., and Lemos, G. C. (2008). Contribuciones del factor general y de los factores específicos en la relación entre inteligencia y rendimiento escolar. Eur. J. Educ. Psychol. 1, 5–16. doi: 10.30552/ejep.v1i3.13
Arnaiz-Sánchez, P., De Haro, R., Alcaraz, S., and Mirete-Ruiz, A. B. (2020). Schools that promote the improvement of academic performance and the success of all students. Front. Psychol. 10:2920. doi: 10.3389/fpsyg.2019.02920
Arnaiz-Sánchez, P., De Haro, R., and Mirete, A. B. (2018). “Procesos de mejora e inclusión educativa en centros educativos de la Región de Murcia [Educational improvement and inclusion processes in educational centers in the Region of Murcia],” in Inclusión y Mejora Educativa [Inclusion and Educational Improvement], eds J. C. Torrego, L. Rayón, Y. Muñoz, and P. Gómez (Alcalá de Henares: Servicio Publicaciones Universidad), 271–281.
Baye, A., and Monseur, C. (2016). Gender differences in variability and extreme scores in an international context. Large Scale Assess. Educ. 4, 1–16. doi: 10.1186/s40536-015-0015-x
Bian, L., Leslie, S. J., and Cimpian, A. (2017). Gender stereotypes about intellectual ability emerge early and influence children’s interests. Science 355, 389–391. doi: 10.1126/science.aah6524
Boaler, J. (2016). Mathematical Mindsets: Unleashing Students’ Potential Through Creative Math, Inspiring Messages and Innovative Teaching. San Francisco, CA: Jossey-Bass.
Botella, C., Rueda, S., López-Iñesta, E., and Marzal, P. (2019). Gender diversity in STEM disciplines: a multiple factor problem. Entropy 21, 1–17. doi: 10.3390/e21010030
Calvo, G. (2018). Las identidades de género según las y los adolescentes. Percepciones, desigualdades y necesidades educativas. Contextos Educ. Rev. Educ. 21, 169–184. doi: 10.18172/con.3311
Cantoral, R., Reyes-Gasperini, D., and Montiel, G. (2014). Socioepistemología, Matemáticas y Realidad. Rev. Latinoamericana Etnomatemática 7, 91–116.
Carey, E., Hill, F., Devine, A., and Szücs, D. (2016). The chicken or the egg? The direction of the relationship between mathematics anxiety and mathematics performance. Front. Psychol. 6:1987. doi: 10.3389/fpsyg.2015.01987
Cázares, M. J., Páez, D. A., and Pérez, M. G. (2020). Discusión teórica sobre las prácticas docentes como mediadoras para potencializar estrategias metacognitivas en la solución de tareas matemáticas. Educ. Matemática 32, 221–240. doi: 10.24844/EM3201.10
Chamorro-Premuzic, T., Quiroga, M. A., and Colom, R. (2009). Intellectual competence and academic performance: a spanish study. Learn. Individ. Diff. 19, 486–491. doi: 10.1016/j.lindif.2009.05.002
Cueli, M., Areces, D., García, T., Alves, R. A., and González-Castro, P. (2020). Attention, inhibitory control and early mathematical skills in preschool students. Psicothema 32, 237–244. doi: 10.7334/psicothema2019.225
Del Río, M. F., Strasser, K., and Susperreguy, M. I. (2016). ¿Son las habilidades matemáticas un asunto de Género? Los estereotipos de género acerca de las matemáticas en niños y niñas de Kínder, sus familias y educadoras. Calidad Educación 45, 20–53. doi: 10.4067/S0718-45652016000200002
Desco, M., Navas-Sánchez, F. J., Sánchez-González, J., Reig, S., Robles, O., Franco, C., et al. (2011). Mathematically gifted adolescents use more extensive and more bilateral areas of the fronto-parietal network than controls during executive functioning and fluid reasoning tasks. NeuroImage 57, 281–292. doi: 10.1016/j.neuroimage.2011.03.063
Díez, L. P., and Jiménez, C. J. (2018). Influencia de la organización escolar en la educación de los alumnos de altas capacidades. Enseñanza Teach. 36, 151–178. doi: 10.14201/et2018361151178
Dowker, A., Sarkar, A., and Yen Looi, C. (2016). Mathematics anxiety: ¿what have we learned in 60 years?”. Front. Psychol. 7:508. doi: 10.3389/fpsyg.2016.00508
Else-Quest, N. M., Hyde, J. S., and Linn, M. C. (2010). Cross-national patterns of gender differences in mathematics: a meta-analysis. Psychol. Bull. 136, 103–127. doi: 10.1037/a0018053
Farfán, R. M., and Simón, G. (2017). Género y matemáticas: una investigación con niñas y niños talento. Acta Sci. 19, 427–446.
Ferreira, A. S., Flores, I., and Casas-Novas, T. (2017). Introdução ao Estudo, Porque Melhoraram os Resultados PISA em Portugal?: Estudo Longitudinal e Comparado (2000-2015). Lisboa: Fundação Francisco Manuel dos Santos.
Figueiredo, M., and Guimarães, H. M. (2019). A relevância dos fatores motivacionais nos estilos de aprendizagem da Matemaìtica no iniìcio do ensino secundaìrio. Quadrante 28, 79–99.
Franco, V., Melo, M., Santos, G., Apolónio, A., and Amaral, L. (2017). A national early intervention system as a strategy to promote inclusion and academic achievement in Portugal. Front. Psychol. 8:1137. doi: 10.3389/fpsyg.2017.01137
Frey, C., and Osborne, M. (2017). The future of employment: how susceptible are jobs to computerization? Technol. Forecast. Soc. Change 114, 254–280. doi: 10.1016/j.techfore.2016.08.019
Fuentes, S., and Renobell, V. (2019). La influencia del género en el aprendizaje matemático en España. Evidencias desde PISA. Rev. Sociol. Educ. RASE 13, 63–80. doi: 10.7203/RASE.13.1.16042
García-Perales, R. (2014). Diseño y Validación de Un Instrumento de Evaluación de la Competencia Matemática. Rendimiento Matemático de los Alumnos Más Capaces. dissertation/master’s thesis. Madrid: Universidad Nacional de Educación a Distancia (UNED).
García-Perales, R., and Almeida, L. S. (2019). Programa de enriquecimiento para alumnado con alta capacidad: efectos positivos para el currículum. Comunicar 60, 39–48. doi: 10.3916/C60-2019-04
García-Perales, R., Jiménez-Fernández, C., and Palomares-Ruiz, A. (2020). Seguimiento de un grupo de alumnos y alumnas con alta capacidad matemática. Rev. Investig. Educ. 38, 415–434. doi: 10.6018/rie.366541
García-Perales, R., Jiménez-Fernández, C., and Palomares-Ruiz, A. (2021). Elecciones académicas e interés vocacional en alumnado con alta capacidad matemática. Ensaio Avaliação e Políticas Públicas em Educação 29, 160–182. doi: 10.1590/S0104-40362020002802539
Geary, D. C., and Brown, S. C. (1991). Cognitive addition: strategy choice and speed-of-processing differences in gifted, normal, and mathematically disabled children. Dev. Psychol. 27, 398–406. doi: 10.1037/0012-1649.27.3.398
Gilat, T., and Amit, M. (2013). Exploring young students creativity: the effect of model eliciting activities. PNA 8, 51–59.
González-Pienda, J. A., Fernández, M. S., Suárez, N., Fernández, M. E., Tuero, E., García, T., et al. (2012). Diferencias de género en actitudes hacia las matemáticas en la enseñanza obligatoria. Rev. Iberoamericana Psicol. Salud 3, 55–73.
Hadjar, A., Krolak-Schwerdt, S., Priem, K., and Glock, S. (2014). Gender and educational achievement. Educ. Res. 56, 117–125. doi: 10.1080/00131881.2014.898908
Harju-Luukkainen, H., McElvany, N., and Stang, J. (eds) (2020). Monitoring Student Achievement in the 21st Century. European Policy Perspectives and Assessment Strategies. Berlin: Springer International Publishing.
Hattie, J., Fisher, D., and Frey, N. (2017). Visible Learning for Mathematics: What Works Best to Optimize Student Learning. Thousand Oaks, CA: Corwin.
Hernández-Barco, M., Cañada-Cañada, F., Corbacho-Cuello, I., and Sánchez-Martín, J. (2021). An exploratory study interrelating emotion, self-efficacy and multiple intelligence of prospective science teachers. Front. Educ. 6:604791. doi: 10.3389/feduc.2021.604791
Hyde, J. S. (2016). Sex and cognition: gender and cognitive functions. Curr. Opin. Neurobiol. 38, 53–56. doi: 10.1016/j.conb.2016.02.007
Instituto Nacional de Evaluación Educativa (2013). PISA 2012: Informe Español. Volumen I: Resultados y contexto. Madrid: Ministerio de Educación, Cultura y Deporte.
Jaime, A., and Gutiérrez, A. (2017). “Investigacioìn sobre estudiantes con alta capacidad matemaìtica,” in Investigacioìn en Educacioìn Matemaìtica XXI, eds J. M. Munoz-Escolano, A. Arnal-Bailera, P. Beltraìn-Pellicer, M. L. Callejo, and J. Carrillo (Zaragoza: SEIEM), 71–89.
Jiménez, C. (2014). El Desarrollo Del Talento: Educación y Alta Capacidad. Lección Inaugural del Curso Académico 2014-2015 de la UNED. Madrid: UNED.
Juvera, J., and Hernández-López, S. (2021). Steam in childhood and the gender gap: a proposal for non-formal education. EDU REVIEW. Int. Educ. Learn. Rev. 9, 9–25. doi: 10.37467/gka-revedu.v9.2712
Kerr, B. (2000). “Guiding gifted girls and young women,” in International Handbook of Giftedness and Talent, eds K. M. Heller, F. J. Mönks, R. J. Sternberg, and R. F. Subotnik (Oxford, UK: Pergamon Press), 649–657.
Kijima, R., and Sun, K. L. (2020). ‘Females don’t need to be reluctant’: employing design thinking to harness creative confidence and interest in STEAM. Int. J. Art Design Educ. 40, 66–81. doi: 10.1111/jade.12307
Kurnaz, A. (2018). Examining effects of mathematical problem-solving, mathematical reasoning and spatial abilities on gifted students’ mathematics achievement. World Sci. Res. 5, 37–43. doi: 10.20448/journal.510.2018.51.37.43
Lehman, K. J., Sax, L. J., and Zimmerman, H. B. (2017). Women planning to major in computer science: who are they and what makes them unique? J. Comput. Sci. Educ. 26, 277–298. doi: 10.1080/08993408.2016.1271536
Llor, L., Ferrando, M., Ferrandiz, C., Hernández, D., Sainz, M., Prieto, M. D., et al. (2012). Inteligencias Múltiples y Alta Habilidad. Aula Abierta 40, 27–38.
Macià, M., and Garreta, J. (2018). Accesibilidad y alfabetización digital: barreras para la integración de las TIC en la comunicación familia/escuela. Rev. Investig. Educ. 36, 239–257. doi: 10.6018/rie.36.1.290111
Mandelman, S. D., Tan, M., Aljughaiman, A. M., and Grigorenko, E. L. (2010). Intelectual gifteness: economic, political, cultural and psychological considerations. Learn. Individ. Diff. 20, 286–297.
Marsh, H. W., and Martin, A. J. (2011). Academic self-concept and academic achievement: relations and causal ordering. Br. J. Educ. Psychol. 81, 59–77. doi: 10.1348/000709910X503501
Mato, M. D., Espiñeira, E., and Chao, R. (2014). Dimensión afectiva hacia la matemática: resultados de un análisis en educación primaria. Rev. Investig. Educ. 32, 57–72. doi: 10.6018/rie.32.1.164921
McCullough, L. (2020). Proportions of Women in STEM Leadership in the Academy in the USA. Educ. Sci. 10, 1–13. doi: 10.3390/educsci10010001
Méndez, D., Méndez, A., and Fernández-Río, F. (2015). Análisis y valoración del proceso de incorporación de las Competencias Básicas en Educación Primaria. Rev. Investig. Educ. 33, 233–246. doi: 10.6018/rie.33.1.183841
Ministerio de Educación, Cultura y Deporte (2014a). Marco General de la evaluación de 3er curso de Educación Primaria. Madrid: Ministerio de Educación, Cultura y Deporte.
Ministerio de Educación, Cultura y Deporte (2014b). PISA 2012 Informe español. Resultados y contexto. Madrid: Ministerio de Educación, Cultura y Deporte.
Ministerio de Educación, Cultura y Deporte (2014c). Real Decreto 126/2014, de 28 de febrero, por el que se establece el currículo básico de la Educación Primaria. Boletín Oficial del Estado 52, 1–58.
Ministerio de Educación y Formación Profesional (2019). PISA 2018. Programa para la Evaluación Internacional de los Estudiantes. Informe Español. Available online at: https://cutt.ly/zr3uTgk (accessed September 25, 2020).
Ministerio de Educación y Formación Profesional (2020). Datos Estadísticos Para Enseñanzas No Universitarias. Available online at: https://cutt.ly/GgeQCdi (Accessed October 6, 2020).
Mizala, A., Martínez, F., and Martínez, S. (2015). Pre-service elementary school teachers’ expectations about student performance: how their beliefs are affected by their mathematics anxiety and Student’s gender. Teach. Teach. Educ. 50, 70–78. doi: 10.1016/j.tate.2015.04.006
Monroy, D., and Marroquín, B. (2020). Didáctica de la Matemática y su importancia en los profesores en formación. Rev. Guatemalteca Educ. Superior 3, 47–59. doi: 10.46954/revistages.v1i1.4
Muelas, A. (2014). Influencia de la variable de personalidad en el rendimiento académico de los estudiantes cuando finalizan la Educación Secundaria Obligatoria (ESO) y comienzan Bachillerato. Hist. Comun. Soc. 18, 115–126. doi: 10.5209/rev_HICS.2013.v18.44230
Nortes, A., and Nortes, R. (2013). Formación inicial de maestros: un estudio en el dominio de las Matemáticas. Prof. Rev. Curríc. Form. Prof. 17, 185–200.
Organization for Economic Cooperation, and Development (2013). PISA 2012 Assessment and Analytical Framework: Mathematics, Reading, Science, Problem Solving and Financial Literacy. Paris: OECD Publishing.
Organization for Economic Cooperation, and Development (2019a). Balancing School Choice and Equity: An International Perspective Based on PISA. Paris: OECD Publishing.
Organization for Economic Cooperation, and Development (2019b). PISA 2018 Assessment and Analytical Framework. Paris: OECD Publishing.
Organization for Economic Cooperation, and Development (2019c). PISA 2018 Results (Volume I): What Students Know and Can Do. Paris: OECD Publishing.
Organization for Economic Cooperation and Development (2019d). PISA 2018 Results (Volume II): Where All Students Can Succeed. Paris: OECD Publishing.
Ortiz-Revilla, J., and Greca, I. M. (2021). “Intentions Towards Following Science and Engineering Studies Among Primary Education Pupils Participating in Integrated STEAM Activities,” in The 11th International Conference on European Transnational Educational (ICEUTE 2020). Advances in Intelligent Systems and Computing, eds A. Herrero, C. Cambra, D. Urda, J. Sedano, H. Quintián, and E. Corchado (Cham: Springer).
Palomares-Ruiz, A., and García-Perales, R. (2020). Math performance and sex: the predictive capacity of self-efficacy, interest and motivation for learning mathematics. Front. Psychol. 11:1879. doi: 10.3389/fpsyg.2020.01879
Pelegrina, S., García, M. C., and Casanova, P. F. (2002). Los estilos educativos de los padres y la competencia académica de los adolescentes. Infancia Aprendizaje 25, 147–168. doi: 10.1174/021037002317417796
Pomar, C., Díaz, O., Sánchez, T., and Fernández, M. (2009). Habilidades matemáticas y verbales: diferencias de género en una muestra de 6ş de Primaria y 1ş de ESO. Rev. Int. Faisca de Altas Capacidades 14, 14–26.
Preckel, F., Goetz, T., Pekrun, R., and Kleine, M. (2008). Gender differences in gifted and average-ability students: comparing girls’ and boys’ achievement, self-concept, interest, and motivation in mathematic. Gift. Child Q. 52, 146–159. doi: 10.1177/0016986208315834
Ramírez, R., and Cañadas, M. C. (2018). Nominación y atención del talento matemático por parte del docente. UNO Rev. Didáctica de las Matemáticas 79, 23–30.
Rico, L., Gómez, P., and Cañadas, M. (2014). Formación inicial en educación matemática de los maestros de Primaria en España, 1991-2010. Rev. Educ. 363, 35–59. doi: 10.4438/1988-592X-RE-2012-363-169
Roderer, T., and Roebers, C. M. (2013). Children’s performance estimation in mathematics and science tests over a school year: a pilot study. Electron. J. Res. Educ. Psychol. 11, 5–24. doi: 10.25115/ejrep.v11i29.1555
Rodríguez, D., and Guzmán, R. (2018). Relación entre perfil motivacional y rendimiento académico en educación secundaria obligatoria. Estudios Sobre Educación 34, 199–217. doi: 10.15581/004.34.199-217
Rodríguez-Mantilla, J. M., Fernández-Díaz, M. J., and Jover, G. (2018). PISA 2015: predictores del rendimiento en Ciencias en España. Rev. Educ. 38, 75–102. doi: 10.4438/1988-592X-RE-2017-380-373
Román-Graván, P., Hervás-Gómez, C., Martín-Padilla, A. H., and Fernández-Márquez, E. (2020). Perceptions about the use of educational robotics in the initial training of future teachers: a study on STEAM sustainability among female teachers. Sustainability 12:4154. doi: 10.3390/su12104154
Rosário, P., Lourenço, A., Paiva, O., Rodrigues, A., Valle, A., and Tuero-Herrero, E. (2012). Predicción del rendimiento en matemáticas: efecto de variables personales, socioeducativas y del contexto escolar. Psicothema 24, 289–295.
Rotigel, J. V., and Fello, S. (2004). Mathematically gifted students: how can we meet their needs. Gift. Child Today 27, 46–51. doi: 10.4219/gct-2004-150
Ruiz, F. (2005). Influencia de la autoeficacia en el ámbito académico. Docencia Univ. 1, 1–16. doi: 10.19083/ridu.1.33
Ryu, J., Lee, Y., Kim, Y., Goundar, P., Lee, J., and Jung, J. Y. (2021). “STEAM in Gifted Education in Korea,” in Handbook of Giftedness and Talent Development in the Asia-Pacific, ed. S. R. Smith (Singapore: Springer).
Sason, H., Michalsky, T., and Mevarech, Z. (2020). Promoting middle school students’ science text comprehension via two self-generated “linking” questioning methods. Front. Psychol. 11:595745. doi: 10.3389/fpsyg.2020.595745
Schillinger, F. L., Vogel, S. E., Diedrich, J., and Grabner, R. H. (2018). Math anxiety, intelligence, and performance in mathematics: insights from the german adaptation of the Abbreviated Math Anxiety Scale (AMAS-G). Learn. Individ. Diff. 61, 109–119. doi: 10.1016/j.lindif.2017.11.014
Schleicher, A. (2018). World Class: How to Build a 21st-Century School System, Strong Performers and Successful Reformers in Education. Paris: OECD Publishing.
Song, L. J., Huang, G., Peng, Z., Law, K., Wong, C., and Chen, Z. (2010). The differential effects of general mental ability and emotional intelligence on academic performance and social interactions. Intelligence 38, 137–143. doi: 10.1016/j.intell.2009.09.003
Sriraman, B. (2003). Mathematical giftedness, problem solving, and the ability to formulate generalizations: the problem-solving experiences of four gifted students. J. Second. Gift. Educ. 14, 151–165. doi: 10.4219/jsge-2003-425
Torres, L. L. (2018). Culturas de escola e excelência: entre a integração de todos e a distinção dos melhores. Rev. Sociol. Educ. 11, 167–185. doi: 10.7203/RASE.10.1.9135
UNESCO (2019). Descifrar el Código: La Educación De Las Niñas en Ciencias, Tecnología, Ingeniería Y Matemáticas (STEM). Francia: UNESCO.
Ursini, S., and Ramírez-Mercado, M. P. (2017). Equidad, género y matemáticas en la escuela mexicana. Rev. Colomb. Educ. 73, 213–234. doi: 10.17227/01203916.73rce211.232
Keywords: math performance, assessment instrument, primary education, educational inclusion, sex
Citation: García Perales R and Palomares Ruiz A (2021) Comparison Between Performance Levels for Mathematical Competence: Results for the Sex Variable. Front. Psychol. 12:663202. doi: 10.3389/fpsyg.2021.663202
Received: 02 February 2021; Accepted: 19 May 2021;
Published: 17 June 2021.
Edited by:
Jin Su Jeong, University of Extremadura, SpainReviewed by:
João Leitão, University of Beira Interior, PortugalCopyright © 2021 García Perales and Palomares Ruiz. This is an open-access article distributed under the terms of the Creative Commons Attribution License (CC BY). The use, distribution or reproduction in other forums is permitted, provided the original author(s) and the copyright owner(s) are credited and that the original publication in this journal is cited, in accordance with accepted academic practice. No use, distribution or reproduction is permitted which does not comply with these terms.
*Correspondence: Ramón García Perales, UmFtb24uR2FyY2lhUGVyYWxlc0B1Y2xtLmVz
Disclaimer: All claims expressed in this article are solely those of the authors and do not necessarily represent those of their affiliated organizations, or those of the publisher, the editors and the reviewers. Any product that may be evaluated in this article or claim that may be made by its manufacturer is not guaranteed or endorsed by the publisher.
Research integrity at Frontiers
Learn more about the work of our research integrity team to safeguard the quality of each article we publish.