- 1Department of Psychosocial Science, University of Bergen, Bergen, Norway
- 2Norwegian Competence Center for Sleep Disorders, Haukeland University Hospital, Bergen, Norway
- 3Optentia, The Vaal Triangle Campus of the North-West University, Vanderbijlpark, South Africa
- 4Department of Global Public Health and Primary Care, University of Bergen, Bergen, Norway
Objectives: No systematic review or meta-analysis concerning the prevalence of shift work disorder (SWD) has been conducted so far. The aim was thus to review prevalence studies of SWD, to calculate an overall prevalence by a random effects meta-analysis approach and investigate correlates of SWD prevalence using a random-effects meta-regression.
Methods: Systematic searches were conducted in ISI Web of Science, PsycNET, PubMed, and Google Scholar using the search terms “shift work disorder” and “shift work sleep disorder.” No restrictions in terms of time frame were used. Included studies had to present original data on the prevalence of SWD in an occupational sample published in English. A total of 349 unique hits were made. In all, 29 studies were finally included from which two authors independently extracted data using predefined data fields. The meta-regression included four predictors (diagnostic criteria, study country, type of workers, and sample size).
Results: The overall prevalence of SWD was 26.5% (95% confidence interval = 21.0–32.8). Cochran Q was 1,845.4 (df = 28, p < 0.001), and the I2 was 98.5%, indicating very high heterogeneity across the observed prevalence estimates. Diagnostic criteria (International Classification of Sleep Disorders-2 = 0, International Classification of Sleep Disorders-3 = 1) and sample size were inversely related to SWD prevalence.
Conclusions: The prevalence of SWD was high across the included studies. The between-study disparity was large and was partly explained by diagnostic criteria and sample size. In order to facilitate comparative research on SWD, there is a need for validation and standardization of assessment methodology as well as agreement in terms of sample restrictions.
Introduction
Shift work implies working in different shifts, e.g., morning, evening, and night, and can vary along several dimension, such as intensity and speed of rotating (European Parliament of the Council, 2003). Night work, which is a special type of shift work, can be defined as work that covers at least 3 h of work between 11 p.m and 6 a.m (Garde et al., 2019). Still, it should be noted that different studies use definitions that may deviate somewhat from the aforementioned ones. Shift work and night work are common work schedules, and 2017 data from the European Union show that these include 21 and 19% of the workforce, respectively (Eurofound, 2017).
A vast amount of studies show that shift and night works negatively impact health. Such working arrangements, for example, have been associated with cardiovascular disease (Torquati et al., 2018), cancers (Wang et al., 2015; Gan et al., 2018; Pahwa et al., 2018), metabolic disturbances (Watanabe et al., 2018; Gao et al., 2020), sleep disturbances (Pallesen et al., 2010), gastrointestinal disorders (Knutsson and Bøggild, 2010), and impaired reproductive health (Stocker et al., 2014), as well as impaired mental health (Torquati et al., 2019). Furthermore, shift work and night work have also been linked to negative organizational outcomes such as accidents (Fischer et al., 2017), impaired cognitive efficiency (Di Muzio et al., 2020), sick leave (Merkus et al., 2012), low job satisfaction (Jamal, 1981), and turnover and turnover intention (Pisarski et al., 2006; Flinkman et al., 2008). The underlying mechanisms for the negative health consequences are not fully understood but involve most likely circadian disruption leading to neuroendocrine and cardiometabolic stress, curtailed and disturbed sleep causing altered immune functioning and cellular stress, and risk behaviors and psychosocial stress with cognitive impairment and poor emotion regulation as consequences (Kecklund and Axelsson, 2016).
When a shift or night worker experiences sleep disturbance that is associated with clinically significant distress or impairment of social, occupational, or other areas of functioning, he/she may be suffering from shift work disorder (SWD; Wright et al., 2013). SWD was termed “shift work sleep disorder” in the first edition of the International Classification of Sleep Disorders (American Sleep Disorders Association, 1990). According to the second edition of the International Classification of Sleep Disorders (ICSD-2), SWD is diagnosed on four essential points: (1) a complaint of insomnia or excessive sleepiness that is temporally associated with a recurring work schedule that overlaps the usual time for sleep; (2) the symptoms are associated with the shift work schedule over the course of 1 month; (3) sleep log or actigraphy monitoring for at least 7 days demonstrates disturbed circadian and sleep-time misalignment; and (4) the sleep disturbance is not better explained by another current sleep disorder, medical or neurological disorder, mental disorder, medication use, or substance use disorder (American Academy of Sleep Medicine, 2005). When the third edition of the diagnostic system (ICSD-3) was released in 2014, three notable amendments of the diagnostic criteria for SWD were made: (1) the insomnia/sleepiness complaint must be accompanied by a reduction of total sleep time; (2) the duration of the symptoms must be at least 3 months; and (3) sleep log or actigraphy monitoring has to be conducted for at least 14 days and needs to include both work and free days (American Academy of Sleep Medicine, 2014).
As insomnia is the most prevalent sleep disorder, a plausible means of evaluating whether SWD is highly prevalent in the shift-working population is by comparing SWD prevalence to the prevalence of insomnia among daytime workers. In this regard, insomnia prevalence among daytime workers varies, but has been estimated at 27.6% in home nursing caregivers in Japan (Takahashi et al., 2008), 8.5% (Yong et al., 2017) and 18.0% (Drake et al., 2004) in two large US cross-occupational samples, 9.9% in a Norwegian cross-occupational sample (Ursin et al., 2009), and 12.4% in textile factory workers in Iran (Yazdi et al., 2014).
Although sleep diaries or actigraphic recordings are requirements for a formal diagnosis, most large-scale epidemiological studies have estimated SWD prevalence based on different types of self-report questionnaires. Across these, no consensus in terms of how to estimate SWD prevalence seems to have been established. Some emphasize the differential prevalence of the core symptoms (insomnia or sleepiness) between day workers and shift/night workers to make proper estimates (Drake et al., 2004). Others assess SWD with a specific questionnaire based on a discrimination function analysis (Barger et al., 2012), whereas other scholars anchor their estimates in a minimum of questions adhering as closely as possible to formal diagnostic criteria (Waage et al., 2009). Another unresolved matter concerns who can be diagnosed. Some reserve this for night workers only (Rajaratnam et al., 2011), whereas others argue that SWD may even affect day workers (Flo et al., 2012), as it is conceivable that night owls, for example, having day work with an early start, will work at a time overlapping with their usual sleep time (Facer-Childs et al., 2019). These factors may impact the estimated prevalences of SWD. Another factor that should be taken into consideration is that of work hours. Developing countries typically exhibit longer work hours on average, frequently >40 h per week, compared to developed countries where work hours are concentrated in the range of 30–40 h per week (Messenger and Ray, 2013). As long work hours may interfere with sleep (Virtanen et al., 2009) and cause excessive sleepiness (Wilsmore et al., 2013), study country may moderate prevalence estimates of SWD. Moreover, although sample size (e.g., small study effect) probably affects estimates less often in prevalence studies than in trial studies, sample size can nevertheless not be discounted as a moderator (Richter et al., 2019).
So far, neither a systematic review nor meta-analysis regarding the prevalence of SWD has been conducted. Against this backdrop, we aimed at: (1) presenting an overview of the current published literature on the prevalence of SWD, (2) synthesizing the prevalences using a random effects meta-analysis, and (3) exploring the correlates of potential significant between-study heterogeneity of SWD prevalence.
Materials and Methods
Search Strategy and Inclusion Criteria
We conducted a systematic and comprehensive literature search in Google Scholar, ISI Web of Science, PsycNET, and PubMed. The following keywords were used: “shift work disorder” OR “shift work sleep disorder.” A total of 645 hits (including the first 200 of 5,860 hits in Google Scholar) were identified from the database search. The Google Scholar search was conducted in order to identify gray literature. One record was identified through ad hoc searches. After removing duplicates, 349 records were available for screening. Of this pool, 41 records were removed after screening their titles. Next, the abstracts of the remaining 308 records were inspected. A total of 53 records were available after going through the abstracts. After screening the 53 full-text records for eligibility, 29 were included in the analysis.
The key inclusion criteria were that the study or record presented original data on the prevalence of SWD in an occupational sample and published in English. The literature search was conducted from February 12, 2020, to March 13, 2020. We conducted the literature search and selection in line with the Preferred Reporting Items for Systematic Reviews and Meta-Analyses (PRISMA) procedure (Moher et al., 2009), and the guidelines of the Meta-analysis of Observational Studies in Epidemiology (Stroup et al., 2000) group. Figure 1 presents the literature search and selection process. See Appendix for a completed PRISMA-guideline checklist.
Data Extraction
The first and last authors independently conducted literature searches and selection of articles based on the aforementioned criteria. Using a standardized data extraction form, the following data were extracted from the identified studies and coded: first author name and publication year, data collection period, country, sample of shift workers, shift type, SWD assessment/measure, sample size (total, female, and male), age of the participants (range, mean ± SD), SWD prevalence, and response rate (Table 1). Discrepant extractions were resolved through discussion and further review until consensus was reached.
Statistical Analysis
We used a random-effects model in the prevalence meta-analysis, using the DerSimonian and Laird approach for estimating the between-study variance (DerSimonian and Laird, 1986). Prevalence estimates and their corresponding 95% confidence intervals (95% CIs) were calculated. The preference for a random-effects model is based on its propensity for higher external validity or generalizability of findings and recommendation when included studies are assumed to represent different populations of studies (Borenstein et al., 2009). For between-study heterogeneity, we conducted a random-effects meta-regression analysis to examine whether the following predictors explained the heterogeneity in SWD prevalence: (a) diagnostic criteria (ICSD-2 vs. ICSD-3), (b) country of study; developing (China, Egypt, Ethiopia, India, Nigeria, Thailand) vs. developed (Australia, Canada, Finland, Greece, Japan, the Netherlands, Norway, Romania, South Korea, United States) countries, (c) type of work (includes night work: no/yes), and (d) sample size. As a scatter plot suggested a curvilinear relationship between prevalence (logit) and sample size, the latter variable was transformed by its natural logarithm before entering it to the regression model.
Heterogeneity was assessed using Cochran Q. The I2 statistic was calculated and reflects the proportion of variation in observed effects that is due to variation in true effects (Borenstein et al., 2017). An I2 of 0% suggests no heterogeneity, 25% indicates low heterogeneity, 50% indicates moderate heterogeneity, and 75% indicates high heterogeneity, respectively (Higgins et al., 2003). We also calculated the 95% prediction interval, which represents the interval within which the effect size of a future study would fall given that the study was randomly selected from the same population as the studies included in the present meta-analysis (IntHout et al., 2016). Publication bias was investigated using Egger test, denoting a regression model where the standardized effect size comprises the dependent variable and the inverse of the standard error is the independent variable. An intercept significantly different from zero suggests bias (Egger et al., 1997). Also, the trim-and-fill procedure by Duval and Tweedie (2000) was used for investigation of publication bias. This procedure is based on the funnel plot, where effect sizes are depicted along the x axis and where the inverse of the variance (sample size) is represented on the y axis. This creates a funnel plot with the largest and most precise studies situated at the top of the funnel. In the absence of publication bias, the funnel plot is symmetrical. Publication bias often entails lack of small studies with small effects. The trim-and-fill procedure trims off asymmetric outlying studies and replaces them with studies around the center, whereupon an adjusted effect size and 95% CI are calculated.
Additionally, we assessed study quality or risk of presenting biased prevalence estimates using a quality assessment checklist for prevalence studies (Hoy et al., 2012). The checklist comprises items reflecting 10 characteristics of the included studies, each scored 0 (low risk of bias) or 1 (high risk of bias). High risk was indicated by each of the following items: (1) study target population is not representative of the national working population, (2) sampling frame is not a representation of the target population, (3) random selection is not used, (4) response rate is <75%, (5) data are collected from a proxy, (6) an acceptable case definition is not used, (7) the study instrument is not shown to have reliability or validity, (8) same mode of data collection is not used for all subjects, (9) the shortest prevalence period for the parameter is not appropriate, and (10) one or more of the numerator(s) or denominator(s) is inappropriate. Hence, the total score ranged from 0 to 10 and was categorized as follows: high quality/low risk (0 to 3), moderate quality/risk (4 to 6), and low quality/high risk (7–10) (Table 2). The meta-analysis and metaregression analysis were conducted using the Comprehensive Meta-Analysis 3.0 software (Biostat Inc., 2014). When calculating the prevalences, the software logit transforms the prevalences in order to carry out all of the statistical analyses, before they are back-transformed to the metric of the prevalences. The transformation is based on the formula Logit = Ln(p/(1–p)), where p is the prevalence rate, and Ln, the natural logarithm. The formula for transforming the sampling variance (V) is V(Logit) = 1/np + 1/n(1–p). The back-transformation is based on the following formula: p = eLogit/(eLogit + 1), with e being the base of the natural logarithm.
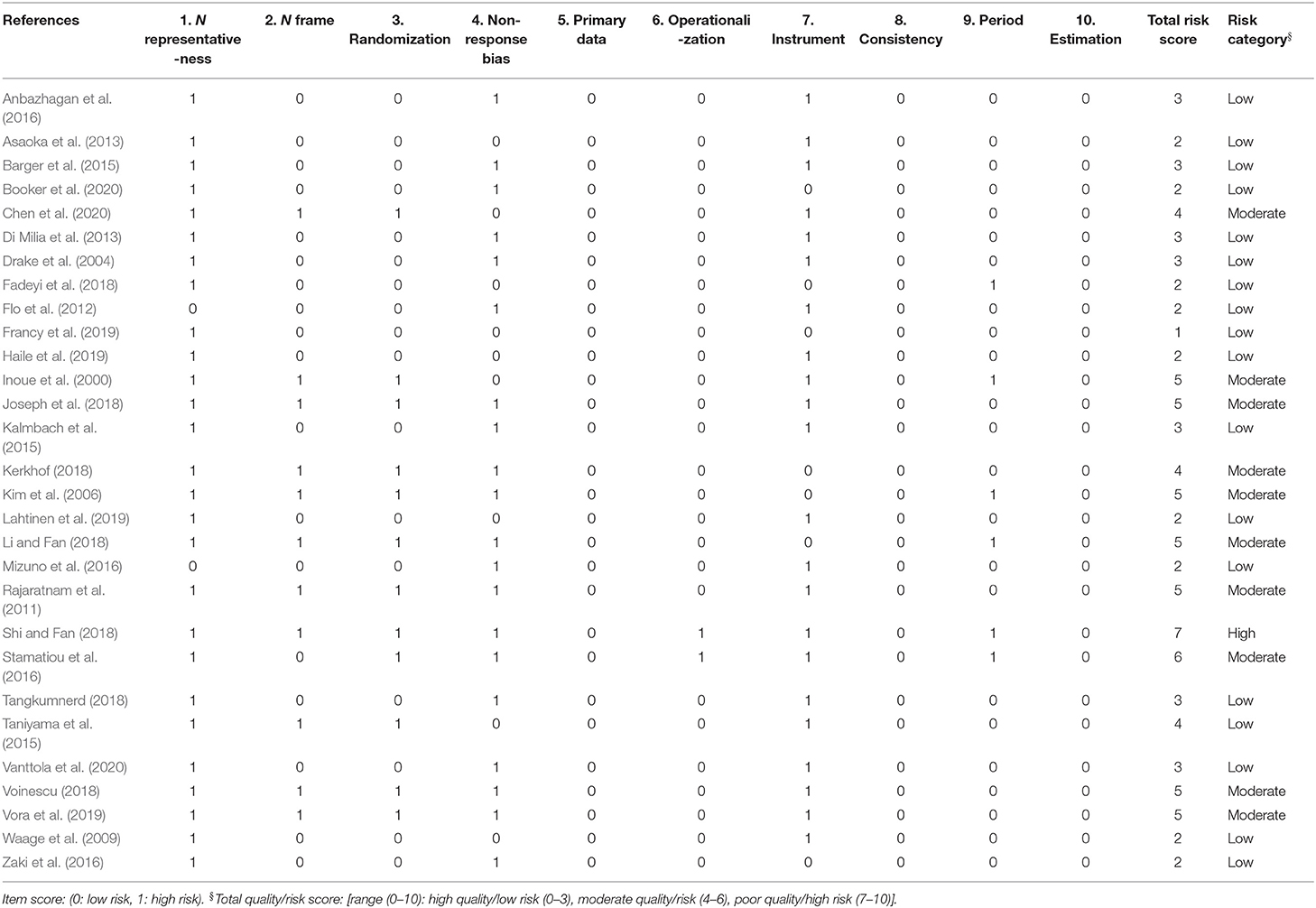
Table 2. Risk of bias/methodological quality (Hoy et al., 2012) of included studies.
Results
Description of Studies
Of the 29 included studies, publication years ranged from 2000 (Inoue et al., 2000) to 2020 (Booker et al., 2020; Chen et al., 2020; Vanttola et al., 2020). Studies were conducted in India (k = 4: Anbazhagan et al., 2016; Joseph et al., 2018; Francy et al., 2019; Vora et al., 2019), Japan (k = 4: Inoue et al., 2000; Asaoka et al., 2013; Taniyama et al., 2015; Mizuno et al., 2016), China (k = 3: Li and Fan, 2018; Shi and Fan, 2018; Chen et al., 2020), United States (k = 3: Drake et al., 2004; Barger et al., 2015; Kalmbach et al., 2015), Australia (k = 2: Di Milia et al., 2013; Booker et al., 2020), Finland (k = 2: Lahtinen et al., 2019; Vanttola et al., 2020), Norway (k = 2: Waage et al., 2009; Flo et al., 2012), and one study each from the following countries: Canada/United States (Rajaratnam et al., 2011), Egypt (Zaki et al., 2016), Ethiopia (Haile et al., 2019), Greece (Stamatiou et al., 2016), the Netherlands (Kerkhof, 2018), Nigeria (Fadeyi et al., 2018), Romania (Voinescu, 2018), South Korea (Kim et al., 2006), and Thailand (Tangkumnerd, 2018).
Samples were predominantly nurses (k = 13: Kim et al., 2006; Flo et al., 2012; Asaoka et al., 2013; Anbazhagan et al., 2016; Vedaa et al., 2016; Zaki et al., 2016; Fadeyi et al., 2018; Joseph et al., 2018; Shi and Fan, 2018; Tangkumnerd, 2018; Francy et al., 2019; Haile et al., 2019; Booker et al., 2020; Chen et al., 2020), other health care workers (k = 3: Stamatiou et al., 2016; Vora et al., 2019; Vanttola et al., 2020), various types of workers (k = 5: Drake et al., 2004; Di Milia et al., 2013; Kalmbach et al., 2015; Kerkhof, 2018; Voinescu, 2018), industrial workers (k = 3: Inoue et al., 2000; Taniyama et al., 2015; Li and Fan, 2018), airline workers (Lahtinen et al., 2019), flight controllers (Mizuno et al., 2016), fire fighters (Barger et al., 2015), oil rig workers (Waage et al., 2009), and police officers (Rajaratnam et al., 2011).
The majority of studies (k = 20) assessed SWD using the ICSD-2 or Waage et al. (2009) measure (Drake et al., 2004; Kim et al., 2006; Waage et al., 2009; Rajaratnam et al., 2011; Flo et al., 2012; Asaoka et al., 2013; Di Milia et al., 2013; Barger et al., 2015; Taniyama et al., 2015; Anbazhagan et al., 2016; Mizuno et al., 2016; Stamatiou et al., 2016; Zaki et al., 2016; Fadeyi et al., 2018; Li and Fan, 2018; Shi and Fan, 2018; Tangkumnerd, 2018; Francy et al., 2019; Haile et al., 2019; Booker et al., 2020). In addition, seven studies used the ICSD-3 or similar criteria (Kalmbach et al., 2015; Joseph et al., 2018; Kerkhof, 2018; Voinescu, 2018; Lahtinen et al., 2019; Vora et al., 2019; Chen et al., 2020), one study used the ICSD-1 (Inoue et al., 2000), and one study (Vanttola et al., 2020) used both the ICSD-2 and ICSD-3 criteria.
The studies included a total of 22,014 participants, ranging from 26 (Lahtinen et al., 2019) to 5,771 (Barger et al., 2015) with a mean of 759.1 (SD = 1,356.6) participants. Of the total, 9,876 were females, whereas 881 were males (rest not accounted for in terms of sex). Table 1 presents further characteristics of the included studies.
Prevalence Estimates and Heterogeneity
The results of the meta-analysis are presented in Figure 2. The overall prevalence across all 29 studies was 26.5% (95% CI = 21.0–32.8). Cochran Q was significant (Q = 1,845.36, df = 28, p < 0.001), suggesting heterogeneity across the prevalence estimates, and the I2 statistic was 98.5%, indicating very high heterogeneity. The 95% prediction interval was 0.06–0.67.
Correlates of SWD Prevalence
Because of the significant heterogeneity, a metaregression analysis based on a random-effects model was conducted including diagnostic criteria (ICSD-2 = 0, ICSD-3 = 1), country of study (developed = 0, developing =1), and type of workers (not only night workers = 0, night workers only = 1) as predictors. The results are presented in Table 3. Overall, the regression model was significant (Q = 13.54, df = 4, p = 0.009, R2 = 33%). Diagnostic criteria (b = −0.914, p = 0.027) and the natural logarithm of sample size (b = −0.344, p = 0.006) were negatively related to SDW prevalence. Still, there was unexplained variance (Q = 979.51, df = 24, p < 0.001).

Table 3. Results of meta regression of diagnostic criteria, study country, night work and sample size on SWD prevalence.
Publication Bias
The results of Egger test (b = 3.80, 95% CI = −1.50–9.10, t = 1.47, p = 0.15) did not suggest publication bias. The trim-and-fill procedure trimmed 0 studies and consequently did not change the overall prevalence estimate.
Discussion
A total of 29 studies fulfilled the inclusion criteria and were consequently included in the meta-analysis, amounting to an overall SWD prevalence of 26.5%. The dispersion of effect sizes was significant, ranging from 2.3% (Voinescu, 2018) to 84.0% (Zaki et al., 2016).
The prevalence of SWD was relatively high across the included studies, suggesting that approximately one in four is affected. This indicates that shift work in general takes a heavy toll on worker's health and is as such in line with several meta-analyses underlining the health detrimental effects of shift work (Stocker et al., 2014; Wang et al., 2015; Gan et al., 2018; Liu et al., 2018; Pahwa et al., 2018; Torquati et al., 2018, 2019; Garbarino et al., 2019). The finding suggests that focus on prevention and treatment of SWD should be prioritized (Gupta et al., 2019). Additionally, the overall SWD prevalence was high compared to typical prevalences estimated for insomnia in daytime workers (Drake et al., 2004; Takahashi et al., 2008; Ursin et al., 2009; Yazdi et al., 2014; Yong et al., 2017).
The high disparity of prevalences suggests that the included studies differ on several dimensions. In order to elucidate this further, a meta-regression with four independent variables was conducted. The independent variables comprised diagnostic system (ICSD-2 vs. ICSD-3), country (developed vs. developing), night work (all types of workers vs. night workers only), and sample size. The prevalences were higher when studies used the ICSD-2 diagnostic criteria (American Academy of Sleep Medicine, 2005), compared to the ICSD-3 criteria (American Academy of Sleep Medicine, 2014). This is understandable as the ICSD-3 criteria are more stringent than the ICSD-2 criteria. Sample size was also inversely related to prevalences, which might suggest the presence of a small study effect (Richter et al., 2019), although the Egger test (Egger et al., 1997) and the trim-and-fill procedure (Duval and Tweedie, 2000) suggested otherwise. Other predictors that may explain additional variance in SWD prevalence are profession (Barger et al., 2009), shift work experience (Saksvik-Lehouillier et al., 2013), codetermination of work schedule (Albertsen et al., 2008), sample characteristics such as age and sex (Saksvik et al., 2011), general working conditions (Costa, 2003), speed and direction of rotation (Knauth, 1995), shift start times (Sallinen and Hublin, 2015), and other sample characteristics such as work–family spillover (Kunst et al., 2014). Future studies should thus more stringently investigate predictors of SWD.
There was a large variation between studies in terms of study quality, and this was also strongly related to study dimension. Only two studies (Flo et al., 2012; Mizuno et al., 2016) had national representative samples of specific professions, and no study included national representative studies of workers in general. Hence, more studies on the prevalence of SWD should be based on national representative samples. Additionally, fewer than half of the studies had a sampling frame reflecting the study population or described a proper random selection of participants. Moreover, few studies used an instrument with known validity and/or reliability when assessing SWD. Hence, future studies should improve especially on these study dimensions.
In terms of assessment, both the ICSD-2 and the ICSD-3 require sleep diaries or actigraphy for fulfillment of the SWD diagnosis (American Academy of Sleep Medicine, 2005, 2014). With a few exceptions (Kim et al., 2006; Mizuno et al., 2016; Lahtinen et al., 2019), such measures were not included in the prevalence studies reviewed. This seems reasonable in the context of epidemiological research. Some validated questionnaires reflecting SWD have been developed (Barger et al., 2012), but may still be too extensive for large-scale survey studies. Hence, the need for development of a short scale validated (e.g., in terms of sensitivity and specificity) against proper diagnostic procedures would advance the field. This could also facilitate consensus in terms of operationalization of the disorder and easing comparisons across study comparisons. Another issue of which the field would benefit from reaching consensus concerns which work schedules might be relevant for the SWD diagnosis. Some scholars seem, for example, to restrict the SWD diagnosis to night workers (Barger et al., 2015; Kalmbach et al., 2015), whereas others include all types of workers, including day workers only (Flo et al., 2012; Voinescu, 2018).
Most of the included studies were cross-sectional. A few exceptions to this were noted as some studies assessed potential predictors among study participants before they started working (e.g., at nursing school) and then assessed the prevalence of SWD some time following introduction to work life (e.g., 3 and 6 months) (Chen et al., 2020). Such studies may yield other prevalences than studies conducted among well-established shift workers, due to the healthy shift worker effect (e.g., those not coping with shift work quit) (Knutsson, 2004) associated with the latter type of studies. This should also be taken into consideration when interpreting the prevalence of SWD.
Strengths and Limitations
The present meta-analysis targeted the inclusion of gray literature, as recommended for the calculation of non-biased estimates in meta-analyses (Borenstein et al., 2009). All prevalence data and quality assessment of the included studies were coded independently by two of the authors, ensuring reliability. Searches were conducted across several databases, and no restrictions in terms of time frame were applied. The meta-analysis was conducted in line with the PRISMA guidelines (Moher et al., 2009).
Some articles presented limited study information, which made the table of study characteristics of the included studies somewhat incomplete. Still, it should be noted that the authors of the present meta-analysis contacted authors to obtain missing information. In the few cases of disagreement about study coding between the raters, agreement was reached by consulting the article in question and through discussions. However, records of initial disagreement between raters were not kept, preventing calculation of interrater reliability.
Conclusion
The prevalence of SWD was overall high (26.5%) across the included studies, although the single estimates varied strongly. This suggests that focus on prevention and treatment of SWD should be prioritized. Diagnostic criteria (ICSD-2 = 0 vs. ICSD-3 = 1) and sample size were inversely related to SWD prevalence emphasizing the need for consensus in the field in terms of SWD assessment and sample restrictions.
Data Availability Statement
The raw data supporting the conclusions of this article will be made available by the authors, without undue reservation.
Author Contributions
Study conceptualization, literature search, data analysis and coding of studies were conducted by SP and DS. All authors contributed to interpretation of data, writing and revising the work critically for important intellectual content, read and approved the final version of the work to be published, and agreed to be accountable for all aspects of the work in ensuring that questions to the accuracy of any part of the work are appropriately investigated and resolved.
Funding
This study was funded from Nordforsk, Nordic Program on Health and Welfare (74809).
Conflict of Interest
The authors declare that the research was conducted in the absence of any commercial or financial relationships that could be construed as a potential conflict of interest.
Supplementary Material
The Supplementary Material for this article can be found online at: https://www.frontiersin.org/articles/10.3389/fpsyg.2021.638252/full#supplementary-material
References
Albertsen, K., Rafnsdottir, G. L., Grimsmo, A., Tomasson, K., and Kauppinen, K. (2008). Workhours and worklife balance. Scand. J. Work. Environ. Health Suppl. 5, 14–21.
American Academy of Sleep Medicine (2005). The International Classification of Sleep Disorders. Westchester, IL: American Academy of Sleep Medicine.
American Academy of Sleep Medicine (2014). International Classification of Sleep Disorders. Darien, IL: American Academy of Sleep Medicine.
American Sleep Disorders Association (1990). International Classification of Sleep Disorders. Diagnostic and coding manual. Lawrence, KS: Allen Press Inc.
Anbazhagan, S., Ramesh, N., Nisha, C., and Joseph, B. (2016). Shift work disorder and related health problems among nurses working in a tertiary care hospital, Bangalore, South India. Indian J. Occup. Environ. Med. 20, 35–38. doi: 10.4103/0019-5278.183842
Asaoka, S., Aritake, S., Komada, Y., Ozaki, A., Odagiri, Y., Inoue, S., et al. (2013). Factors associated with shift work disorder in nurses working with rapid-rotation schedules in Japan: the nurses' sleep health project. Chronobiol. Int. 30, 628–636. doi: 10.3109/07420528.2012.762010
Barger, L. K., Lockley, S. W., Rajaratnam, S. M. W., and Landrigan, C. P. (2009). Neurobehavioral, health, and safety consequences associated with shift work in safety-sensitive professions. Curr. Neurol. Neurosci. Rep. 9, 155–164. doi: 10.1007/s11910-009-0024-7
Barger, L. K., Ogeil, R. P., Drake, C. L., O'Brien, C. S., Ng, K. T., and Rajaratnam, S. M. W. (2012). Validation of a questionnaire to screen for shift work disorder. Sleep 35, 1693–1703. doi: 10.5665/sleep.2246
Barger, L. K., Rajaratnam, S. M. W., Wang, W., O'Brien, C. S., Sullivan, J. P., Qadri, S., et al. (2015). Common sleep disorders increase risk of motor vehicle crashes and adverse health outcomes in firefighters. J. Clin. Sleep Med. 11, 233–249. doi: 10.5664/jcsm.4534
Booker, L. A., Sletten, T. L., Alvaro, P. K., Barnes, M., Collins, A., Chai-Coetzer, C. L., et al. (2020). Exploring the associations between shift work disorder, depression, anxiety and sick leave taken amongst nurses. J. Sleep Res. 29:e12872. doi: 10.1111/jsr.12872
Borenstein, M., Hedges, L. V., Higgins, J. P. T., and Rothstein, H. R. (2009). Introduction to Meta-Analysis. Chichester: Wiley. doi: 10.1002/9780470743386
Borenstein, M., Higgins, J. P. T., Hedges, L. V., and Rothstein, H. R. (2017). Basics of meta-analysis: I2 is not an absolute measure of heterogeneity. Res. Synth. Methods 8, 5–18. doi: 10.1002/jrsm.1230
Chen, D. X., Jiang, M., Shi, X. L., Geng, F. L., Qi, H. Y., Zhang, Y. C., et al. (2020). Predictors of the initiation of shift work disorder among Chinese intern nurses: a prospective study. Sleep Med. 68, 199–206. doi: 10.1016/j.sleep.2019.11.1263
Costa, G. (2003). Factors influencing health of workers and tolerance to shift work. Theor. Issues Ergon. Sci. 4, 263–288. doi: 10.1080/14639220210158880
DerSimonian, R., and Laird, N. (1986). Meta-analysis in clinical trials. Control. Clin. Trials 7, 177–188. doi: 10.1016/0197-2456(86)90046-2
Di Milia, L., Waage, S., Pallesen, S., and Bjorvatn, B. (2013). Shift work disorder in a random population sample - prevalence and comorbidities. PLoS ONE 8:e55306. doi: 10.1371/journal.pone.0055306
Di Muzio, M., Diella, G., Di Simone, E., Novelli, L., Alfonsi, V., Scarpelli, S., et al. (2020). Nurses and night shifts: poor sleep quality exacerbates psychomotor performance. Front. Neurosci. 14:579938. doi: 10.3389/fnins.2020.579938
Drake, C. L., Roehrs, T., Richardson, G., Walsh, J. K., and Roth, T. (2004). Shift work sleep disorder: prevalence and consequences beyond that of symptomatic day workers. Sleep 27, 1453–1462. doi: 10.1093/sleep/27.8.1453
Duval, S., and Tweedie, R. (2000). Trim and fill: a simple funnel-plot-based method of testing and adjusting for publication bias in meta-analysis. Biometrics 56, 455–463. doi: 10.1111/j.0006-341X.2000.00455.x
Egger, M., Smith, G. D., Schneider, M., and Minder, C. (1997). Bias in meta-analysis detected by a simple, graphical test. Br. Med. J. 315, 629–634. doi: 10.1136/bmj.315.7109.629
Eurofound (2017). 6th European Working Conditions Survey. 2017 Update. Luxembourg: Publications Office of the European Union.
European Parliament and of the Council (2003). Directive 2003/88/EC Concerning Certain Aspects of the Organisation of Working Time. Brussels: European Parliament.
Facer-Childs, E. R., Middleton, B., Skene, D. J., and Bagshaw, A. P. (2019). Resetting the late timing of 'night owls' has a positive impact on mental health and performance. Sleep Med. 60, 236–247. doi: 10.1016/j.sleep.2019.05.001
Fadeyi, B. A., Ayoka, A. O., Fawale, M. B., Alabi, Q. K., Oluwadaisi, A. M., and Omole, J. G. (2018). Prevalence, predictors and effects of shift work sleep disorder among nurses in a Nigerian teaching hospital. Sleep. Sci. Pract. 2:6. doi: 10.1186/s41606-018-0027-x
Fischer, D., Lombardi, D. A., Folkard, S., Willetts, J., and Christiani, D. C. (2017). Updating the “risk index”: a systematic review and meta-analysis of occupational injuries and work schedule characteristics. Chronobiol. Int. 34, 1423–1438. doi: 10.1080/07420528.2017.1367305
Flinkman, M., Laine, M., Leino-Kilpi, H., Hasselhorn, H. M., and Salantera, S. (2008). Explaining young registered finnish nurses' intention to leave the profession: a questionnaire survey. Int. J. Nurs. Stud. 45, 727–739. doi: 10.1016/j.ijnurstu.2006.12.006
Flo, E., Pallesen, S., Magerøy, N., Moen, B. E., Grønli, J., Nordhus, I. H., et al. (2012). Shift work disorder in nurses. Assessment, prevalence and related health problems. PLoS ONE 7:e33981. doi: 10.1371/journal.pone.0033981
Francy, A., Nisha, C., and Abraham, J. (2019). Pevalence of shift work disorder among nurses working in a tertiary care hospital in Thrissur District, Kerala, India. Int. J. Med. Sci. Curr. Res. 2, 320–325.
Gan, Y., Li, L. Q., Zhang, L. W., Yan, S. J., Gao, C., Hu, S., et al. (2018). Association between shift work and risk of prostate cancer: a systematic review and meta-analysis of observational studies. Carcinogenesis 39, 87–97. doi: 10.1093/carcin/bgx129
Gao, Y. Y., Gan, T., Jiang, L. L., Yu, L., Tang, D. M., Wanga, Y. H., et al. (2020). Association between shift work and risk of type 2 diabetes mellitus: a systematic review and dose-response meta-analysis of observational studies. Chronobiol. Int. 37, 29–46. doi: 10.1080/07420528.2019.1683570
Garbarino, S., Guglielmi, O., Puntoni, M., Bragazzi, N. L., and Magnavita, N. (2019). Sleep quality among police officers: Implications and insights from a systematic review and meta-analysis of the literature. Int. J. Environ. Res. Public Health 16:885. doi: 10.3390/ijerph16050885
Garde, A. H., Harris, A., Vedaa, Ø., Bjorvatn, B., Hansen, J., Hansen, A. M., et al. (2019). Working hour characteristics and schedules among nurses in three Nordic countries - a comparative study using payroll data. BMC Nurs. 18:12. doi: 10.1186/s12912-019-0332-4
Gupta, A., Roth, T., Roehrs, T., and Drake, C. L. (2019). Shift work: a perspective on shift work disorder. Is prevention the answer? J. Clin. Sleep Med. 15, 1863–1865. doi: 10.5664/jcsm.8104
Haile, K. K., Asnakew, S., Waja, T., and Kerbih, H. B. (2019). Shift work sleep disorders and associated factors among nurses at federal government hospitals in Ethiopia: a cross-sectional study. BMJ Open 9:e029802. doi: 10.1136/bmjopen-2019-029802
Higgins, J., Thompson, S. G., Deeks, J. J., and Altman, D. G. (2003). Measuring inconsistency in meta-analyses BMJ 327 557–560. doi: 10.1136/bmj.327.7414.557
Hoy, D., Brooks, P., Woolf, A., Blyth, F., March, L., Bain, C., et al. (2012). Assessing risk of bias in prevalence studies: modification of an existing tool and evidence of interrater agreement. J. Clin. Epidemiol. 65, 934–939. doi: 10.1016/j.jclinepi.2011.11.014
Inoue, Y., Hiroe, Y., Nishida, M., and Shirakawa, S. (2000). Sleep problems in Japanese industrial workers. Psychiatry Clin. Neurosci. 54, 294–295. doi: 10.1046/j.1440-1819.2000.00684.x
IntHout, J., Ioannidis, J. P. A., Rovers, M. M., and Goeman, J. J. (2016). Plea for routinely presenting prediction intervals in meta-analysis. BMJ Open 6:e010247. doi: 10.1136/bmjopen-2015-010247
Jamal, M. (1981). Shift work related to job-attitudes, social participation and withdrawal behavior - a study of nurses and industrial workers. Pers. Psychol. 34, 535–547. doi: 10.1111/j.1744-6570.1981.tb00494.x
Joseph, A., Brooke, M., Jelby, N. N., Pushpalatha, N. N., Sharon, N. N., and Sunitha, N. N. (2018). The prevalence of shift work sleep disorder and the quality of sleep among nurses working in selected hospitals, Hyderabad. Indian J. Psychiatr. Nurs. 15, 10–12. doi: 10.4103/2231-1505.262498
Kalmbach, D. A., Pillai, V., Cheng, P., Arnedt, J. T., and Drake, C. L. (2015). Shift work disorder, depression, and anxiety in the transition to rotating shifts: the role of sleep reactivity. Sleep Med. 16, 1532–1538. doi: 10.1016/j.sleep.2015.09.007
Kecklund, G., and Axelsson, J. (2016). Health consequences of shift work and insufficient sleep. BMJ 355:i5210. doi: 10.1136/bmj.i5210
Kerkhof, G. A. (2018). Shift work and sleep disorder comorbidity tend to go hand in hand. Chronobiol. Int. 35, 219–228. doi: 10.1080/07420528.2017.1392552
Kim, J. Y., Kim, J. H., Kim, S. H., Lee, H., and Choi, K. G. (2006). Sleep and health related problems and shift work sleep disorder in nurses with rapid rotating shift work schedules. Neurology 66:A79.
Knauth, P. (1995). Speed and direction of shift rotation. J. Sleep Res. 4, 41–46. doi: 10.1111/j.1365-2869.1995.tb00225.x
Knutsson, A. (2004). Methodological aspects of shift-work research. Chronobiol. Int. 21, 1037–1047. doi: 10.1081/CBI-200038525
Knutsson, A., and Bøggild, H. (2010). Gastrointestinal disorders among shift workers. Scand. J. Work Environ. Health 36, 85–95. doi: 10.5271/sjweh.2897
Kunst, J. R., Løset, G. K., Hosøy, D., Bjorvatn, B., Moen, B. E., Magerøy, N., et al. (2014). The relationship between shift work schedules and spillover in a sample of nurses. Int. J. Occup. Saf. Ergon 20, 3–11. doi: 10.1080/10803548.2014.11077030
Lahtinen, A., Puttonen, S., Vanttola, P., Viitasalo, K., Sulkava, S., Pervjakova, N., et al. (2019). A distinctive DNA methylation pattern in insufficient sleep. Sci. Rep. 9:1193. doi: 10.1038/s41598-018-38009-0
Li, Y., and Fan, F. (2018). Shift work sleep disorder in industrial workers: a survey. Sleep 41:A65. doi: 10.1093/sleep/zsy061.167
Liu, Q. Y., Shi, J., Duan, P., Liu, B., Li, T. F., Wang, C., et al. (2018). Is shift work associated with a higher risk of overweight or obesity? A systematic review of observational studies with meta-analysis. Int. J. Epidemiol. 47, 1956–1971. doi: 10.1093/ije/dyy079
Merkus, S. L., van Drongelen, A., Holte, K. A., Labriola, M., Lund, T., van Mechelen, W., et al. (2012). The association between shift work and sick leave: a systematic review. Occup. Environ. Med. 69, 701–712. doi: 10.1136/oemed-2011-100488
Messenger, J. C., and Ray, N. (2013). The Distribution of Hours of Work in Developed and Developing Countries: What are the Main Differences and Why? Geneva: International Labour Office.
Mizuno, K., Matsumoto, A., Aiba, T., Abe, T., Ohshima, H., Takahashi, M., et al. (2016). Sleep patterns among shift-working flight controllers of the international space station: an observational study on the JAXA Flight Control Team. J. Physiol. Anthropol. 35:19. doi: 10.1186/s40101-016-0108-4
Moher, D., Liberati, A., Tetzlaff, J., Altman, D. G., and Grp, P. (2009). Preferred reporting items for systematic reviews and meta-analyses: the PRISMA Statement. PLoS Med. 6:e1000097. doi: 10.1371/journal.pmed.1000097
Pahwa, M., Labreche, F., and Demers, P. A. (2018). Night shift work and breast cancer risk: what do the meta-analyses tell us? Scand. J. Work Environ. Health 44, 432–435. doi: 10.5271/sjweh.3738
Pallesen, S., Bjorvatn, B., Magerøy, N., Saksvik, I. B., Waage, S., and Moen, B. E. (2010). Measures to counteract the negative effects of night work. Scand. J. Work Environ. Health 36, 109–120. doi: 10.5271/sjweh.2886
Pisarski, A., Brook, C., Bohle, P., Gallois, C., Watson, B., and Winch, S. (2006). Extending a model of shift-work tolerance. Chronobiol. Int. 23, 1363–1377. doi: 10.1080/07420520601055316
Rajaratnam, S. M. W., Barger, L. K., Lockley, S. W., Shea, S. A., Wang, W., Landrigan, C. P., et al. (2011). Sleep disorders, health, and safety in police officers. JAMA 306, 2567–2578. doi: 10.1001/jama.2011.1851
Richter, D., Wall, A., Bruen, A., and Whittington, R. (2019). Is the global prevalence rate of adult mental illness increasing? Systematic review and meta-analysis. Acta Psychiat. Scand. 140, 393–407. doi: 10.1111/acps.13083
Saksvik, I. B., Bjorvatn, B., Hetland, H., Sandal, G. M., and Pallesen, S. (2011). Individual differences in tolerance to shift work - a systematic review. Sleep Med. Rev. 15, 221–235. doi: 10.1016/j.smrv.2010.07.002
Saksvik-Lehouillier, I., Bjorvatn, B., Hetland, H., Sandal, G. M., Moen, B. E., Magerøy, N., et al. (2013). Individual, situational, and lifestyle factors related to shift work tolerance among nurses who are new to - and experienced in night work. J. Adv. Nurs. 69, 1136–1146. doi: 10.1111/j.1365-2648.2012.06105.x
Sallinen, M., and Hublin, C. (2015). Fatigue-inducing factors in transportation operators. Rev Hum Factors Ergon 10, 138–173. doi: 10.1177/1557234X15574828
Shi, X., and Fan, F. (2018). Shift work sleep disorder and associated psychological factors among Chinese practice nurses: a prospective study. Sleep 41, A240–A241. doi: 10.1093/sleep/zsy061.647
Stamatiou, K., Margariti, M., Nousi, E., Mistrioti, D., Lacroix, R., and Saridi, M. (2016). Female sexual dysfunction (FSD) in women health care workers. Mater. Sociomed. 28, 178–182. doi: 10.5455/msm.2016.28.178-182
Stocker, L. J., Macklon, N. S., Cheong, Y. C., and Bewley, S. J. (2014). Influence of shift work on early reproductive outcomes a systematic review and meta-analysis. Obstet. Gynecol. 124, 99–110. doi: 10.1097/AOG.0000000000000321
Stroup, D. F., Berlin, J. A., Morton, S. C., Olkin, I., Williamson, G. D., Rennie, D., et al. (2000). Meta-analysis of observational studies in epidemiology - a proposal for reporting. JAMA 283, 2008–2012. doi: 10.1001/jama.283.15.2008
Takahashi, M., Iwakiri, K., Sotoyama, M., Higuchi, S., Kiguchi, M., Hirata, M., et al. (2008). Work schedule differences in sleep problems of nursing home caregivers. Appl. Ergon. 39, 597–604. doi: 10.1016/j.apergo.2008.01.003
Tangkumnerd, M. (2018). Factors Associated With Shift Work Disorder Among Nurses in a Private Hospital Bangkok Thailand. (Master), Bangkok: Chulalongkorn University.
Taniyama, Y., Yamauchi, T., Takeuchi, S., and Kuroda, Y. (2015). PER1 polymorphism associated with shift work disorder. Sleep Biol. Rhythms 13, 342–347. doi: 10.1111/sbr.12123
Torquati, L., Mielke, G. I., Brown, W. J., Burton, N. W., and Kolbe-Alexander, T. L. (2019). Shift work and poor mental health: a meta-analysis of longitudinal studies. Am. J. Public Health 109, E13–E20. doi: 10.2105/AJPH.2019.305278
Torquati, L., Mielke, G. I., Brown, W. J., and Kolbe-Alexander, T. (2018). Shift work and the risk of cardiovascular disease. A systematic review and meta-analysis including dose-response relationship. Scand. J. Work. Environ. Health 44, 229–238. doi: 10.5271/sjweh.3700
Ursin, R., Baste, V., and Moen, B. E. (2009). Sleep duration and sleep-related problems in different occupations in the Hordaland health study. Scand. J. Work Environ. Health 35, 193–202. doi: 10.5271/sjweh.1325
Vanttola, P., Puttonen, S., Karhula, K., Oksanen, T., and Harma, M. (2020). Prevalence of shift work disorder among hospital personnel: a cross-sectional study using objective working hour data. J. Sleep Res. 29:e12906. doi: 10.1111/jsr.12906
Vedaa, Ø., Krossbakken, E., Grimsrud, I. D., Bjorvatn, B., Sivertsen, B., Magerøy, N., et al. (2016). Prospective study of predictors and consequences of insomnia: personality, lifestyle, mental health, and work-related stressors. Sleep Med. 20, 51–58. doi: 10.1016/j.sleep.2015.12.002
Virtanen, M., Ferrie, J. E., Gimeno, D., Vahtera, J., Elovainio, M., Singh-Manoux, A., et al. (2009). Long working hours and sleep disturbances: the whitehall II prospective cohort study. Sleep 32, 737–745. doi: 10.1093/sleep/32.6.737
Voinescu, B. I. (2018). Common sleep, psychiatric, and somatic problems according to work schedule: an internet survey in an Eastern European country. Int. J. Behav. Med. 25, 456–464. doi: 10.1007/s12529-018-9719-y
Vora, D., Mehta, S., and Patil, S. (2019). Shift work disorder - prevalence & burden of illness in healthcare providers of India. Med. Clin. Res. 4, 1–9. doi: 10.33140/MCR.04.06.06
Waage, S., Moen, B. E., Pallesen, S., Eriksen, H. R., Ursin, H., Åkerstedt, T., et al. (2009). Shift work disorder among oil rig workers in the North Sea. Sleep 32, 558–565. doi: 10.1093/sleep/32.4.558
Wang, X., Ji, A., Zhu, Y., Liang, Z., Wu, J., Li, S., et al. (2015). A meta-analysis including dose-response relationship between night shift work and the risk of colorectal cancer. Oncotarget 6, 25046–25060. doi: 10.18632/oncotarget.4502
Watanabe, K., Sakuraya, A., Kawakami, N., Imamura, K., Ando, E., Asai, Y., et al. (2018). Work-related psychosocial factors and metabolic syndrome onset among workers: a systematic review and meta-analysis. Obes. Rev. 19, 1557–1568. doi: 10.1111/obr.12725
Wilsmore, B. R., Grunstein, R. R., Fransen, M., Woodward, M., Norton, R., and Ameratunga, S. (2013). Sleep habits, insomnia, and daytime sleepiness in a large and healthy community-based sample of New Zealanders. J. Clin. Sleep Med. 9, 559–566. doi: 10.5664/jcsm.2750
Wright, K. P., Bogan, R. K., and Wyatt, J. K. (2013). Shift work and the assessment and management of shift work disorder (SWD). Sleep Med. Rev. 17, 41–54. doi: 10.1016/j.smrv.2012.02.002
Yazdi, Z., Sadeghniiat-Haghighi, K., Loukzadeh, Z., Elmizadeh, K., and Abbasi, M. (2014). Prevalence of sleep disorders and their impacts on occupational performance: A comparison between shift workers and nonshift workers. Sleep Disord. 2014:870320. doi: 10.1155/2014/870320
Yong, L. C., Li, J., and Calvert, G. M. (2017). Sleep-related problems in the US working population: prevalence and association with shiftwork status. Occup. Environ. Med. 74, 93–104. doi: 10.1136/oemed-2016-103638
Keywords: review, meta-regression, meta-analysis, prevalence, shift work disorder, shift work sleep disorder
Citation: Pallesen S, Bjorvatn B, Waage S, Harris A and Sagoe D (2021) Prevalence of Shift Work Disorder: A Systematic Review and Meta-Analysis. Front. Psychol. 12:638252. doi: 10.3389/fpsyg.2021.638252
Received: 05 December 2020; Accepted: 15 February 2021;
Published: 23 March 2021.
Edited by:
Gabriela Topa, National University of Distance Education (UNED), SpainReviewed by:
Luigi De Gennaro, Sapienza University of Rome, ItalyJulio Sánchez-Meca, University of Murcia, Spain
Copyright © 2021 Pallesen, Bjorvatn, Waage, Harris and Sagoe. This is an open-access article distributed under the terms of the Creative Commons Attribution License (CC BY). The use, distribution or reproduction in other forums is permitted, provided the original author(s) and the copyright owner(s) are credited and that the original publication in this journal is cited, in accordance with accepted academic practice. No use, distribution or reproduction is permitted which does not comply with these terms.
*Correspondence: Ståle Pallesen, c3RhYWxlLnBhbGxlc2VuJiN4MDAwNDA7dWliLm5v