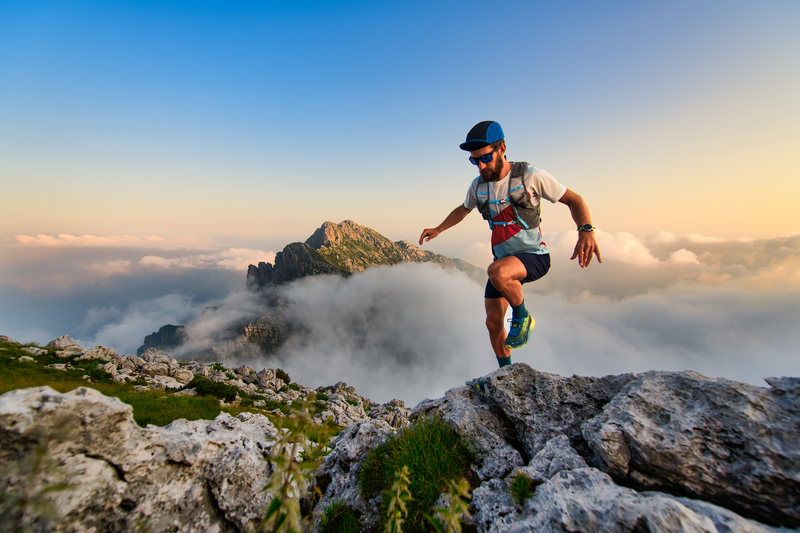
94% of researchers rate our articles as excellent or good
Learn more about the work of our research integrity team to safeguard the quality of each article we publish.
Find out more
ORIGINAL RESEARCH article
Front. Psychol. , 24 May 2021
Sec. Neuropsychology
Volume 12 - 2021 | https://doi.org/10.3389/fpsyg.2021.603974
This article is part of the Research Topic Physical and Cognitive Frailty in the Elderly: An Interdisciplinary Approach View all 13 articles
Background: Cognitive frailty (CF) includes reversible and potentially reversible subtypes; the former is known as concurrent physical frailty (PF) and pre-mild cognitive impairment subjective cognitive decline (pre-MCI SCD), whereas the latter is known as concurrent PF and MCI. The diagnoses of pre-MCI SCD and MCI are based on clinical criteria and various subjective cognitive decline questionnaires. Heterogeneous assessment of cognitive impairment (CI) results in significant variability of CI, CF, and their subtype prevalence in various population-based studies.
Objective: This study aimed to compare the classification differences in CI and CF subtypes from PF and normal cognition by applying clinical and objective cognitive criteria. Clinical criteria comprised Fried PF and clinical MCI criteria combined with the SCD questionnaire, whereas objective criteria comprised Fried PF and objective cognitive criteria based on the norm-adjusted six neuropsychological test scores.
Methods: Of the 335 volunteers (age ≥ 60 years) in this study, 191 were diagnosed with CI based on clinical cognitive diagnosis criteria, and 144 were identified as robust normal based on objective cognitive assessment from the community-dwelling older adult cohort. Individuals with clinical CI, including 94 with MCI and 97 with pre-MCI SCD, were reclassified into different z-score-derived MCI, pre-MCI SCD, and normal subgroups based on objective cognitive criteria. The classification diagnostic accuracy of normal cognition, PF, pre-MCI, MCI, CF, and CF subtypes based on clinical and objective criteria was compared before and after adjusting for age, sex, and education level.
Results: The reclassification of objective assessments indicated better performance than that of clinical assessments in terms of discerning CI severity among different subgroups before adjusting for demographic factors. After covariate adjustment, clinical assessments significantly improved the ability to cognitively discriminate normal individuals from those with pre-MCI SCD and MCI but not the z-score-derived pre-MCI SCD and MCI groups from the robust normal group. Furthermore, the adjustment did not improve the ability to discriminate among individuals with reversible CF from those with potentially reversible CF and pre-MCI only SCD from MCI only SCD.
Conclusions: Objective criteria showed better performance than clinical criteria in the diagnosis of individuals with CI or CF subtypes. Rapid clinical cognitive screening in combination with normative z-scores criteria is cost effective and sustainable in clinical practice.
Cognitive frailty (CF) is defined as a clinical disorder with concurrent physical frailty (PF) and cognitive impairment (CI) but without dementia (Kelaiditi et al., 2013). Numerous epidemiological studies have shown that PF increases the risk of future cognitive decline and all-type dementia (Robertson et al., 2013; Kojima et al., 2016; Ma et al., 2019; Wallace et al., 2019). The combination of PF/physical prefrailty (PPF) and CI could better assess the risk for adverse outcomes in older adults (Forti et al., 2014; Yu et al., 2018; Aliberti et al., 2019; Meiner et al., 2020). CF can further be classified into two subtypes: reversible CF (RCF) and potentially reversible CF (PRCF). RCF is an optimal target for preventing elderly dependence (Ruan et al., 2015). However, the incidences of RCF and PRCF reportedly vary—from 2.5 and 1% to 19.86 and 6.3%, respectively—according to various population-based studies (Panza et al., 2018; Sugimoto et al., 2018; Ruan et al., 2020a). The use of different CF models, such as PPF or premild CI (MCI) subjective cognitive decline (SCD) that includes early (or cognitive compensation stage) and two later stages of subtle cognitive decline (Jessen et al., 2014), is considered a cause of such diversity; moreover, the different tools available for the assessment of PF have contributed to a marked variability in results (Canevelli and Cesari, 2017). The PF phenotype that includes PPF and PF, developed in the US Cardiovascular Health Study, is widely used by researchers to screen PF. Typically, CF models only contain the PF phenotype that was assessed using these modified criteria (Fried et al., 2001). Most studies involved PRCF resulting from the combination of PF and MCI. Only two studies have involved RCF resulting from the combination of PF and pre-MCI SCD (Solfrizzi et al., 2017) and that of PF/PPF and pre-MCI SCD (Ruan et al., 2020a).
Another more important cause of the significant variability of CF prevalence in various population-based studies is the heterogeneous assessment of CI. For the assessment of MCI, major studies have adopted global cognition screening measures, such as the Mini-Mental State Evaluation (MMSE) and the Montreal Cognitive Assessment (MoCA), to diagnose CI according to predetermined cutoff ranges (Trzepacz et al., 2015; Bosco et al., 2017, 2020). Although these measures can assess cognitive performance in different cognitive domains, the MMSE was less likely to detect early MCI because of ceiling effects in healthy controls (Trzepacz et al., 2015). The MoCA is a more difficult test than the MMSE, has fewer floor and ceiling effects, and is more sensitive than the latter for the detection of early cognitive decline (Larner, 2012; Trzepacz et al., 2015). However, the MoCA may yield scores lower than the cutoff values owing to the effect of demographic factors (Rossetti et al., 2011). A Clinical Dementia Rating (CDR) score of 0.5 is unlikely to differentiate MCI severity and MCI subtypes (Chang et al., 2011). To date, only two studies have adopted pre-MCI SCD for RCF diagnosis (Solfrizzi et al., 2017; Ruan et al., 2020a). Although the pre-MCI SCD criteria frame proposed by the SCD-I Working Group (Solfrizzi et al., 2017) was adopted, it is not suitable for the identification of slightly abnormal cognitive performance or subtle cognitive decline. Furthermore, pre-MCI SCD diagnosis in major studies is based on various self-reported questionnaires related to the memory domain (Rami et al., 2014; Rabin et al., 2015). Therefore, establishing objective cognitive criteria is essential for accurately diagnosing CI, CF, and their subtypes.
In the past few years, normative z-scores on the neuropsychological test battery have been established based on data collected in a cognitively normal population (Weintraub et al., 2009; Weintraub et al., 2018; Ruan et al., 2020b). It was possible to objectively identify pre-MCI SCD, MCI, and MCI subtypes. The false-positive diagnostic errors caused by the clinical MCI criteria (Edmonds et al., 2015a,b) significantly decreased using normative z-scores of domain-specific tests. When process z-scores obtained from learning and memory tests were integrated into non-invasive objective criteria, early pre-MCI SCD could be objectively diagnosed (Thomas et al., 2018). Moreover, patients with objective pre-MCI SCD showed early entorhinal pathological changes and faster amyloid accumulation but less widespread medial temporal neurodegeneration than the observations in patients with MCI (Thomas et al., 2020). Therefore, if objective criteria for MCI and pre-MCI SCD are integrated into the CF criteria, the accuracy of the diagnosis of CF subtypes would significantly improve.
The differentiating factor between the clinical and objective criteria is the number of tasks used—only screening tests versus additional tests for each cognitive domain. Objective criteria are more effective when they are based on additional cognitive tests. The present study aimed to explore the diagnostic accuracy of CI, CF, and their subtypes by comparing the discordance between clinical [clinical MCI criteria combined with the Spanish SCD questionnaire (SCD-Q) MyCog scores] (Rami et al., 2014) and objective assessments of CI in different subgroups based on cognitive status and CF stratifications.
Overall, 367 volunteers (age ≥ 60 years) were recruited from communities across Shanghai via face-to-face communication in each setting. Clinical assessment and neuropsychological tests were conducted from September 2018 to June 2019. After excluding individuals with severe disability, complete loss of hearing and vision, and dementia, 335 eligible individuals (age ≥ 60 years) were included in the study. Among these participants, 94 met the clinical criteria for MCI (Petersen et al., 2001), and 97 met the criteria for pre-MCI SCD based on the SCD-Q MyCog scores (Rami et al., 2014) after excluding individuals with MCI. Furthermore, 144 robust normal individuals having at least 1 year of follow-up data and meeting the normal cognition criteria based on objective diagnosis (the z-scores of six neuropsychological tests) at the second annual study visit were used as controls in the study (Sliwinski et al., 1996; Ruan et al., 2020b). The 191 participants diagnosed with MCI or pre-MCI SCD using clinical cognitive criteria were further reclassified by objective cognitive diagnosis. This study was approved by the Ethics Committee of Huadong hospital, and written informed consent was obtained from each volunteer or authorized representative.
Participants were classified as MCI if they met the following criteria: (1) subjective memory complaint if their MyCog score was ≥7 (Rami et al., 2014); (2) CDR score of 0.5; (3) an MMSE score of 19–30 for education levels (cutoff scores: >19 for illiteracy, >22 for primary school, and >26 for middle school and above; Petersen et al., 2001); (4) no or minimal impairment in activities of daily living as determined by a clinical interview with the patient and informant (Lawton and Brody, 1969); and (5) not demented based on the Diagnostic and Statistical Manual of Mental Disorders, Fourth Edition (American Psychiatric Association, 1994). Participants were classified as pre-MCI SCD if their MyCog score was ≥7 (Rami et al., 2014) after excluding MCI. Depression symptomatology was excluded using the short form of the Geriatric Depression Scale (GDS) (Chau et al., 2006). The cognitive and non-cognitive subscales of the Alzheimer’s Disease Assessment Scale (ADAS-Cog and ADAS-Non-cog, respectively) were used to evaluate the severity of CI and behavior alteration (Rosen et al., 1984). The self-report severity scores based on a brief version of the Neuropsychiatric Inventory Questionnaire (NPI-Q) were used to evaluate the severity of neuropsychiatric symptoms (Kaufer et al., 2000).
According to the criteria reported in the literature with minor modification (Edmonds et al., 2015b; Thomas et al., 2018), MCI and pre-MCI SCD status were assessed using the normative z-scores of six neuropsychological tests and process z-scores of the Hopkins Verbal Learning Test-Revised (HVLT-R) (Ruan et al., 2020b). The tests are as follows: Trail Making Test A and B (TMT A and B) for executive or attention domain; Boston Naming Test and Animal List generation for language domain; HVLT-R for memory domain, including delayed free correct responses and HVLT-R recognition; and three process scores from the HVLT-R for identifying early pre-MCI SCD. Briefly, the HVLT-R is a 12-item (4 words from 3 semantic categories) word-list learning and memory test that includes three learning trials (List A, Trials 1–3); an interference trial with a different list (List B); a short-delay free recall trial (Trial 4) for List A; a long-delay free recall trial (Trial 5) for List A after 25 min; and delayed recognition of 24 words, including 12 List A words and 6 related and 6 unrelated non-List A words. The three process scores from the HVLT-R included the following: learning slope [(List A Trial 3–List A Trail 1)/3], retroactive interference (List A Trial 4/List A Trial 3), and intrusion errors (total number of extralist intrusion errors across all recall trials). Other neuropsychological test batteries, including digit span forward or backward and digit symbol (Ruan et al., 2020b), to assess attention/processing speed domain were also performed for verifying the correction of objective criteria based on six previous neuropsychological test batteries and three memory process scores.
All raw total or process scores were converted to age-, education-, and sex-adjusted z-scores based on regression coefficients derived from our robust normal samples (Ruan et al., 2020b). If a participant had z-scores of >1 standard deviation (SD) from the norm on TMT A, TMT B, and intrusion errors or z-scores of <1 SD from the norm on the other measures of six neuropsychological test batteries, the individual was considered to have an impaired total score (the normative z-scores of six neuropsychological tests) or process score (Supplementary Table 1). The 191 participants with CI as diagnosed by clinical criteria were further classified by z-scores as pre-MCI SCD [two impaired process scores or one impaired process score and one impaired total score or impaired total score on two measures across different cognitive domains or Functional Assessment Questionnaire (FAQ) score of 6–8] or MCI [impaired total score on two measures in the same domain or one impaired score in each of three cognitive domains (memory, executive function, and language domains) or FAQ score of ≥9]; the remainder were classified as cognitively normal after exclusion of CI.
The five-item Fried PF scale (fatigue, weakness, slowness, low physical activity, and weight loss) with Chinese reference values (Hao et al., 2017) was used to assess PF phenotypes in the sample. Scores on the Fried PF scale ranged from 0 to 5, with scores of 1–2 representing pre-frail and 3–5 representing frail.
Participants were classified as RCF if they had both PPF/PF and pre-MCI SCD and as PRCF if they had both PPF/PF and MCI (Ruan et al., 2015).
Two single-nucleotide polymorphisms (rs429358 and rs7412) were genotyped to identify the APOE genotypes containing the APOE ε2, ε3, and ε4 alleles using a SNaPshot minisequencing assay from peripheral whole blood samples (Kim et al., 2010). The individuals were divided into the following subgroups according to the frequency of ε4: 0, 1, and 2.
All continuous variables were assessed using one-sample Kolmogorov–Smirnov test and were deemed to be non-normally distributed. Descriptive statistics were reported as medians and interquartile ranges for continuous variables and as absolute numbers and percentages for categorical variables. The differences among the demographic, neuropsychological, and clinical characteristics of subgroups based on objective cognitive criteria were compared using the Kruskal–Wallis and chi-squared tests. Statistical significance was determined using a cutoff P-value of 0.050. These multiple comparisons of clinical and objective measures were further analyzed using nominal regression analyses after adjusting for age, sex, and education level. Data were analyzed using the SPSS 18.0 software.
The 191 participants with pre-MCI SCD or MCI diagnosed using clinical cognitive criteria were divided into z-score-derived pre-MCI SCD and MCI and z-score-derived normal subgroups based on objective cognitive criteria. The characteristics of the three subgroups and robust normal controls are presented in Table 1. Age in the z-score-derived MCI group was higher than that in the z-score-derived normal groups (P < 0.05). The education level of the z-score-derived MCI and pre-MCI SCD groups was significantly lower than that of the robust normal control group (P < 0.001); furthermore, the education level of the z-score-derived MCI group was lower than that of the z-score-derived normal groups (P < 0.01). The frequency of ApoE ε4 was significantly lower in the robust normal control group, whereas ApoE ε4/ε4 was only observed in the z-score-derived MCI and pre-MCI SCD groups. Among the 94 individuals with MCI diagnosed using clinical criteria, only 34 (36.2%) were accurately diagnosed, whereas the other 26 (27.7%) had normal cognitive function. The remaining 34 (36.2%) individuals were diagnosed with pre-MCI SCD using objective criteria (Table 1 and Figure 1A). Among the 97 individuals with pre-MCI SCD diagnosed using clinical criteria, only 27 (27.8%) were accurately diagnosed; 30 (30.9%) individuals had MCI, and the remaining 40 (41.24%) had normal cognitive function as determined by objective criteria (Table 1 and Figure 1A). The z-score-derived pre-MCI SCD group showed the highest prevalence of PPF, whereas the z-score-derived MCI group exhibited the highest prevalence of PF. The z-score-derived normal group had a significantly higher prevalence of PF and PPF than the robust normal control group. Only 68.8% of the individuals with z-score-derived MCI had PRCF and 72.1% of the individuals with z-score-derived pre-MCI SCD had RCF (Table 1).
Table 1. Demographic, neuropsychological, and clinical characteristics [medians and interquartile ranges (Q25–Q75) for continuous variables and absolute numbers or percentages for categorical variables] of experimental samples after reclassification by adopting objective cognitive assessment (neuropsychological test z-scores) criteria and for the robust normal control group.
Figure 1. Number of CI (A) and CF subtypes (B) based on clinical criteria was reclassified according to objective cognitive criteria.
All raw scores of objective measures, excluding the process scores of intrusion errors, revealed significant differences among the four subgroups (Table 1). However, clinical measures demonstrated less ability to discriminate CI severity compared with objective raw scores. Only ADAS-Cog scores were significantly different between the z-score-derived normal or robust normal groups and the z-score-derived MCI and pre-MCI SCD groups and could differentiate between the z-score-derived MCI and pre-MCI SCD groups as well as between the z-score-derived normal and robust normal groups (Table 1). The MMSE scores were significantly higher in the z-score-derived normal or robust normal groups than in the z-score-derived MCI and pre-MCI SCD groups. However, the MMSE scores did not significantly differ between the z-score-derived MCI and pre-MCI SCD groups. ADAS Non-Cog scores were significantly different between the z-score-derived normal and pre-MCI SCD groups and between the pre-MCI SCD and MCI groups. The CDR scores and SCD-Q MyCog scores were not significantly different among the z-score-derived normal, MCI, and pre-MCI SCD groups.
After adjusting for demographic factors, the scores of objective cognitive measures, including TMT A, TMT B, HVLT-R recognition, learning slope, retroactive interference, digit span forward, digit span backward, and digit symbol, were significantly different between the z-score-derived normal or robust normal and the z-score-derived MCI and pre-MCI SCD groups and not between the z-score-derived normal or robust normal groups (Table 2). Only the scores of intrusion errors showed no significant difference among the four groups. The discriminating ability of clinical measures for CI evidently improved after adjusting for age, sex, and education level. The MMSE, ADAS-Cog, and SCD-Q MyCog scores significantly differed between the z-score-derived normal or robust normal and the z-score-derived MCI and pre-MCI SCD groups as well as between the z-score-derived normal and robust normal groups (Table 2). Compared with the robust normal control, the scores of the non-cognitive measures ADAS Non-Cog, GDS, FAQ, and NPI-Q were significantly higher in the z-score-derived MCI and pre-MCI SCD groups. In addition, the GDS and NPI-Q scores were significantly or marginally high in the z-score-derived normal group.
Table 2. Results from nominal regression analyses that evaluated the difference of cognitive stratification by clinical or objective measures after adjusting by sex, age, and education level (the reference category is robust normal).
Characteristics of CF, PF/PPF only, CI only (z-scored derived pre-MCI SCD/MCI), and normal (no PF/PPF and CI) subgroups reclassified according to the Fried PF/PPF, objective cognitive, and CF criteria are presented in Table 3. There were significant differences in age and education level but not in sex. The frequency of ApoE ε4 was lower in the normal group (12.96%) than in other subgroups, and individuals with ApoE ε4/ε4 were only observed in the CF and CI groups.
Table 3. Demographic, neuropsychological, and clinical characteristics (medians and interquartile ranges [Q25–Q75] for continuous variables, and absolute numbers or percentages for categorical variables) of experimental samples in four stratifications after reclassification by adopting objective cognitive assessment (neuropsychological test z-scores) combined with Fried PF score criteria.
When the clinical cognitive criteria were integrated into the CF criteria, 63 of 94 individuals were diagnosed with PRCF and 68 of 97 were diagnosed with RCF (Table 3). However, when objective cognitive criteria replaced the clinical criteria in the CF criteria, only 22 of 63 (34.9%) individuals were diagnosed with PRCF. Conversely, 25 (39.7%) individuals were diagnosed with RCF, and 16 (25.4%) were cognitively normal. Similarly, only 20 of 68 (29.4%) individuals were diagnosed with RCF. Of the remaining individuals, 22 (32.4%) were diagnosed with PRCF, and 26 (38.2%) were cognitively normal (Figure 1B).
The raw scores of objective tests demonstrated better discriminative ability than clinical cognitive assessment scores. Apart from the scores of intrusion errors, the scores of the other nine objective tests could accurately discriminate the cognitive status of CF, PF/PPF only, pre-MCI SCD/MCI only, and normal groups; only TMT A and HVLT-R delayed recall scores could not accurately discriminate the cognitive status between PF/PPF only and normal groups. However, the clinical MMSE and SCD-Q MyCog scores could accurately discriminate the cognitive status among CI groups (CF and pre-MCI SCD/MCI only groups), PF/PPF only, and normal groups (Table 3). Furthermore, only ADAS-Cog scores could accurately discriminate the cognitive status of the four groups.
After covariate adjustment, the discriminating ability of objective and clinical measures for CI showed distinct improvement. Apart from the scores of the two processes (intrusion errors and retroactive interference) and the measure digit span backward, the other objective measures for CI diagnosis could accurately discriminate the cognitive status of CF, PF/PPF only, pre-MCI SCD/MCI only, and normal groups (Table 4). The MMSE, SCD-Q MyCog, and ADAS-Cog scores could accurately discriminate the cognitive status of the four abovementioned groups (Table 4). Compared with the CF group, the scores of non-cognitive measures were significantly lower in the other three groups.
Table 4. Results from nominal regression analyses that evaluated the difference of cognitive stratification by clinical or objective measures after adjusting by sex, age, and education level (the reference category is cognitive frailty).
The CF and pre-MCI SCD/MCI only groups in Table 3 were divided into two subtypes according to CI severity (Table 5). Significant differences were noted in RCF, PRCF, pre-MCI SCD only, MCI only, and normal groups with respect to age and education level but not sex. ApoE ε4/ε4 was only observed in the RCF and MCI only groups.
Table 5. Demographic, neuropsychological, and clinical characteristics (medians and interquartile ranges [Q25–Q75] for continuous variables, and absolute numbers or percentages for categorical variables) of experimental samples in four stratifications after reclassification by adopting objective cognitive assessment (neuropsychological test z-scores) combined with Fried PF score criteria.
Five of six total raw scores (excluding HVLT-R recognition) for objective cognitive assessment were significantly different between the RCF and PRCF groups, and two of six total raw scores were significantly different in the pre-MCI SCD only and MCI only groups. However, two scores (MMSE and SCD-Q MyCog) for clinical cognitive assessment were not significantly different between the RCF and PRCF groups and between the pre-MCI SCD only and MCI only groups.
After covariate adjustment, the six total scores for objective cognition assessment were significantly different between the RCF and PRCF groups (Table 6). Among the six total scores, the TMT A and TMT B scores significantly differed between the RCF and MCI only groups, whereas the HVLT-R delayed recall and HVLT-R recognition scores marginally differed between the RCF and only MCI groups. Among the three process scores, the learning slope scores significantly differed between the RCF and normal groups. Moreover, the digit span backward and digit symbol scores significantly differed between the RCF and PRCF groups but not between the RCF and only MCI groups. However, the clinical MMSE and SCD-Q MyCog scores did not significantly differ between the RCF and PRCF groups and between the RCF and MCI only groups. The ADAS-Cog scores significantly differed between the RCF and PRCF groups but not between the RCF and MCI only groups. The non-cognitive performance scores significantly differed between the normal and other groups. Compared with the RCF group, the ADAS Non-Cog and FAQ scores were significantly higher in the PRCF group, and the GDS and NPI-Q scores were significantly lower in the MCI only group.
Table 6. Results from nominal regression analyses that evaluated the difference of cognitive stratification by clinical or objective measures after adjusting by sex, age, and education level (the reference category is RCF).
In this comparative study, we established objective criteria for CI and CF subtypes based on the normative z-scores of six neuropsychological test batteries (two memory, two attention or executive, and two language domains) and three process scores of memory domain. According to objective criteria, 191 individuals with pre-MCI SCD or MCI were reclassified into z-score-derived pre-MCI SCD, MCI, and z-score-derived normal subgroups and compared with the robust normal group. The main findings are as follows:
(1) The normative z-scores could improve the differentiation performance for CI subtypes (Tables 1, 2), CF (Tables 3, 4), and CF subtypes (Tables 5, 6) among four different subgroups compared with the raw scores of six neuropsychological test batteries.
(2) The three other neuropsychological test batteries (digit span forward or backward and digit symbol) further confirmed the previous finding.
(3) Among the clinical measures, only ADAS-Cog scores could differentiate the z-score-derived normal subgroup from the z-score-derived pre-MCI SCD and MCI groups and RCF from the PRCF before and after adjusting for demographic factors.
(4) The raw MMSE scores could differentiate the z-score-derived normal from the z-score-derived MCI and pre-MCI SCD groups (Table 1) and the CF and pre-MCI SCD/MCI only groups from the PF/PPF only and normal groups (Table 3) but not the z-score-derived MCI group from the pre-MCI SCD group (Table 1) and the RCF group from the PRCF group (Table 5).
(5) SCD-Q MyCog raw scores were not significantly different among the z-score-derived normal, MCI, and pre-MCI SCD groups (Table 1). However, the scores varied between the CF and pre-MCI SCD/MCI only groups from PF/PPF only and normal groups (Table 3) but not between the RCF and PRCF groups and pre-MCI SCD only and MCI only groups (Table 5).
(6) After adjusting for demographic factors, the MMSE and SCD-Q MyCog scores could differentiate between the z-score-derived normal or robust normal and z-score-derived MCI and pre-MCI SCD groups, between the z-score-derived normal and robust normal groups (Table 2), and between the PF/PPF only and normal groups and the CF and pre-MCI SCD/MCI only groups (Table 4). However, the scores could not distinguish RCF from PRCF and pre-MCI SCD only from MCI (Table 6).
(7) The Non-Cog scores were higher in the CF group than in other subgroups, and the GDS and NPI-Q scores were significantly lower in the MCI only group than in the RCF group.
Although clinical criteria, including the MMSE and SCD-Q MyCog scores, could better differentiate CI or CF from those with normal cognitive function after covariate adjustment, these clinical tools failed to differentiate between the subtypes of CI (pre-MCI SCD and MCI) and CF (RCF and PRCF). The construct of MCI clinical criteria including SCD may be one of the critical factors owing to which covariate adjustment improves the differentiation in some groups but not in others. Therefore, as indicated by our findings, clinical cognitive criteria based on conventional MCI criteria combined with the SCD questionnaire may be important causes of prevalence variability in CI and CF subtypes in different population studies. The ADAS-Cog scores, typically used as an outcome measure in AD clinical trials (Wattmo and Wallin, 2017), is a better tool for differentiating pre-MCI SCD from MCI and RCF from PRCF. Furthermore, demographic factors had a minor influence on ADAS-Cog scores. A previous study demonstrated the adequacy of the ADAS-Cog scores for assessing psychometric properties in older low-literacy adults in sub-Saharan Africa (Paddick et al., 2017). However, a small-sample study showed that the ADAS-Cog scores were significantly affected by age and education (Ben Jemaa et al., 2017). The different effects of demographic factors on the ADAS-Cog scores, as demonstrated in our study, require further investigation in the future.
Objective criteria for pre-MCI SCD used in the present study have previously been verified to provide more sensitive criteria for individuals at risk for progression to MCI, indicate early pathological alterations in the brain (Thomas et al., 2020), and improve diagnostic precision, biomarker associations, and progression rates of MCI (Bondi et al., 2014; Edmonds et al., 2015a). While determining our z-scores criteria, we only replaced the Auditory Verbal Learning Test with a relatively brief measure—the HVLT-R (Ruan et al., 2020b). Moreover, the normative z-scores of the remaining three neuropsychological test batteries (digit span forward or backward and digit symbol) further verified the abovementioned objective cognitive criteria. Therefore, we conclude that objective cognitive criteria were better than clinical criteria for classifying MCI and pre-MCI SCD as well as RCF and PRCF. The diagnostic errors caused by clinical criteria for CI and normal cognition decreased after covariate adjustment.
Few studies have operationally defined pre-MCI SCD (Jessen et al., 2014; Solfrizzi et al., 2017; Margioti et al., 2020; Ruan et al., 2020a), and SCD is often substituted for pre-MCI SCD (Rami et al., 2014; Rabin et al., 2015), particularly for preclinical Alzheimer’s disease (Ávila-Villanueva and Fernández-Blázquez, 2017; Lin et al., 2019). The composite score can be disproportionally influenced by poor cognitive performance on only one test; our pre-MCI SCD criteria required two impaired scores, including process scores (1 SD below or above the normative mean) in two different cognitive domains, thereby significantly improving the sensitivity and specificity of the pre-MCI SCD diagnosis (Thomas et al., 2018). Meanwhile, the clinical MCI criteria also included SCD, which further increased the diagnostic errors of pre-MCI SCD and MCI. SCD occurs with different objective cognitive function trajectories, which could result from various causes (Ávila-Villanueva and Fernández-Blázquez, 2017; Jessen et al., 2020). Reversible SCD is related to depressive symptoms, personality features, side effects from medication, and intermittent sleep disturbances (Reid and Maclullich, 2006; Jessen et al., 2020). Furthermore, our results showed that the RCF group experienced significantly higher depression scores than the MCI group (Table 6). Thus, the SCD-Q MyCog score may be a primary source of diagnostic errors. Conventional MCI criteria may be another source of diagnostic errors. The global CDR is not sensitive to MCI severity and prognosis (Chang et al., 2011), is susceptible to recall bias, and is influenced by psychiatric factors (Saxton et al., 2009). Furthermore, the MMSE and SCD-Q MyCog scores significantly improved in categorizing individuals with CI from those without CI after covariate adjustment (Tables 2, 4). However, the adjustments did not indicate an improvement in discriminating the cognitive status among RCF, PRCF, pre-MCI only, and MCI only groups (Tables 5, 6). Instead, the ADAS-Cog scores indicated better consistency with the neuropsychological test scores and could discriminate z-score-derived MCI and pre-MCI SCD from cognitively normal and robust normal controls (Table 2), CF from only PF/PPF and normal groups (Table 4), and RCF from other subgroups (Table 6). These findings indicate that clinical criteria results in several diagnostic errors in CF subtypes. In addition, the scores of non-cognitive measures were significantly higher in individuals of the PRCF group than in those of the RCF group and in individuals of the CF group than in those of the PF/PPF, only MCI, only pre-MCI SCD, and normal groups.
A limitation of this study was the small sample size of normative z-scores from robust normal controls (Ruan et al., 2020b), resulting in a failure to discriminate pre-MCI SCD from cognitively normal individuals in z-scores of intrusion errors and retroactive interference. Increasing the sample size of normative z-scores from robust normal controls from community-dwelling individuals will improve diagnostic accuracy. Another limitation was the small sample size of the MCI only and pre-MCI SCD only groups. In addition, although the normative z-scores contain the memory, language, and attention/executive domains, the visuospatial domain was not evaluated in our sample, and this limits the possibility to detect the deficits of the visuospatial domain. The visuospatial domain should be evaluated in subsequent studies. Nevertheless, some tests, such as digit span forward, digit span backward, digit symbol, and TMT A for attention or processing speed, also indicated consistent changes.
In summary, the use of clinical criteria for distinguishing MCI from pre-MCI SCD and cognitively normal individuals resulted in numerous diagnostic errors. Covariate adjustment could improve the discriminating ability of clinical cognitive measures. The combination of clinical criteria with objective criteria is implementable and cost effective and will considerably reduce the number of diagnostic errors in CI and CF subtypes in clinical practice.
The original contributions generated for this study are included in the article/Supplementary Material, further inquiries can be directed to the corresponding authors.
The studies involving human participants were reviewed and approved by the study protocol was approved by Hudong Hospital Research Ethics Committee, Fudan University. The patients/participants provided their written informed consent to participate in this study.
QR and ZY: conceptualization. QR, ZY, and JC: data curation. QR, WZ, and JR: analysing and interpretation of data. QR, WZ, JR, JC, and ZY: investigation and methodology. QR: original writing. ZY and JC: reviewing and editing. All authors contributed to the article and approved the submitted version.
This work received financial support from the Medical Science and Technology Support Project of Shanghai Science, Technology Commission (Grant No. 18411962200), the Shanghai Clinical Key Discipline Construction (Grant No. 2017ZZ02010), the Shanghai Science and Technology Innovation Project (Grant No. 17411950702), and National Key Research and Innovation Project (2018YFC2002000). The funding sources played no role in the design or conduct of the study, collection, management, analysis, or interpretation of the data; or preparation, review, or approval of the manuscript.
The authors declare that the research was conducted in the absence of any commercial or financial relationships that could be construed as a potential conflict of interest.
The Supplementary Material for this article can be found online at: https://www.frontiersin.org/articles/10.3389/fpsyg.2021.603974/full#supplementary-material
Aliberti, M. J. R., Cenzer, I. S., Smith, A. K., Lee, S. J., Yaffe, K., and Covinsky, K. E. (2019). Assessing risk for adverse outcomes in older adults: the need to include both physical frailty and cognition. J. Am. Geriatr. Soc. 67, 477–483. doi: 10.1111/jgs.15683
American Psychiatric Association (1994). Diagnostic and Statistical Manual of Mental Disorders, 4th Edn. Washington DC: American Psychiatric Association.
Ávila-Villanueva, M., and Fernández-Blázquez, M. A. (2017). Subjective cognitive decline as a preclinical marker for Alzheimer’s disease: the challenge of stability over time. Front. Aging Neurosci. 9:377.
Ben Jemaa, S., Attia Romdhane, N., Bahri-Mrabet, A., Jendli, A., Le Gall, D., and Bellaj, T. (2017). An arabic version of the cognitive subscale of the Alzheimer’s disease assessment scale (ADAS-Cog): reliability, validity, and normative data. J. Alzheimers Dis. 60, 11–21. doi: 10.3233/jad-170222
Bondi, M. W., Edmonds, E. C., Jak, A. J., Clark, L. R., Delano-Wood, L., McDonald, C. R., et al. (2014). Neuropsychological criteria for mild cognitive impairment improves diagnostic precision, biomarker associations, and progression rates. J. Alzheimers Dis. 42, 275–289. doi: 10.3233/jad-140276
Bosco, A., Caffò, A. O., Spano, G., and Lopez, A. (2020). “Beyond the cutoffs: a Bayesian approach to the use of the montreal cognitive assessment as a screening tool for mild cognitive impairment and dementia,” in Diagnosis and Management in Dementia, eds C. R. Martin and V. R. Preedy (Cambridge: Academic Press).
Bosco, A., Spano, G., Caffò, A. O., Lopez, A., Grattagliano, I., Saracino, G., et al. (2017). Italians do it worse: montreal cognitive assessment (MoCA) optimal cut-off scores for people with probable Alzheimer’s disease and with probable cognitive impairment. Aging Clin. Exp. Res. 29, 1113–1120. doi: 10.1007/s40520-017-0727-6
Canevelli, M., and Cesari, M. (2017). Cognitive frailty: far from clinical and research adoption. J. Am. Med. Dir. Assoc. 18, 816–818. doi: 10.1016/j.jamda.2017.07.004
Chang, Y. L., Bondi, M. W., McEvoy, L. K., Fennema-Notestine, C., Salmon, D. P., and Galasko, D. (2011). Global clinical dementia rating of 0.5 in MCI masks variability related to level of function. Neurology 76, 652–659. doi: 10.1212/wnl.0b013e31820ce6a5
Chau, J., Martin, C. R., Thompson, D. R., Chang, A. M., and Woo, J. (2006). Factor structure of the Chinese version of the geriatric depression scale. Psychol. Health Med. 11, 48–59. doi: 10.1080/13548500500093688
Edmonds, E. C., Delano-Wood, L., Clark, L. R., Jak, A. J., Nation, D. A., and McDonald, C. R. (2015a). Susceptibility of the conventional criteria for mild cognitive impairment to false-positive diagnostic errors. Alzheimers Dement. 11, 415–424. doi: 10.1016/j.jalz.2014.03.005
Edmonds, E. C., Delano-Wood, L., Galasko, D. R., Salmon, D. P., and Bondi, M. W. (2015b). Subtle cognitive decline and biomarker staging in preclinical Alzheimer’s disease. J. Alzheimers Dis. 47, 231–242. doi: 10.3233/jad-150128
Forti, P., Maioli, F., Lega, M. V., Montanari, L., Coraini, F., and Zoli, M. (2014). Combination of the clock drawing test with the physical phenotype of frailty for the prediction of mortality and other adverse outcomes in older community dwellers without dementia. Gerontology 60, 204–211. doi: 10.1159/000356701
Fried, L. P., Tangen, C. M., Walston, J., Newman, A. B., Hirsch, C., Gottdiener, J., et al. (2001). Frailty in older adults: evidence for a phenotype. J. Gerontol. A Biol. Sci. Med. Sci. 56, M146–M156.
Hao, Q. K., Li, J., and Dong, B. R. (2017). Chinese experts consensus on assessment and intervention for elderly patients with frailty. Chin. J. Geriatr. 36, 251–256.
Jessen, F., Amariglio, R. E., Buckley, R. F., van der Flier, W. M., Han, Y., Molinuevo, J. L., et al. (2020). The characterisation of subjective cognitive decline. Lancet Neurol. 19, 271–278.
Jessen, F., Amariglio, R. E., van Boxtel, M., Breteler, M., Ceccaldi, M., Chételat, G., et al. (2014). Group a conceptual framework for research on subjective cognitive decline in preclinical Alzheimer’s disease. Alzheimers Dement. 10, 844–852.
Kaufer, D. I., Cummings, J. L., Ketchel, P., Smith, V., MacMillan, A., Shelley, T., et al. (2000). Validation of the NPI-Q, a brief clinical form of the neuropsychiatric inventory. J. Neuropsychiatr. Clin. Neurosci. 12, 233–239. doi: 10.1176/jnp.12.2.233
Kelaiditi, E., Cesari, M., Canevelli, M., van Kan, G. A., Ousset, P. J., and Gillette-Guyonnet, S. (2013). Cognitive frailty: rational and definition from an (I.A.N.A./I.A.G.G.) international consensus group. J. Nutr. Health Aging 17, 726–734. doi: 10.1007/s12603-013-0367-2
Kim, S. W., Heo, J. H., Kim, C. H., Yoo, D. C., Won, D. H., Lee, S. G., et al. (2010). Rapid and direct detection of apolipoprotein E genotypes using whole blood from humans. J. Toxicol. Environ. Health A 73, 1502–1510. doi: 10.1080/15287394.2010.511573
Kojima, G., Taniguchi, Y., Iliffe, S., and Walters, K. (2016). Frailty as a predictor of Alzheimer disease, vascular dementia, and all dementia among community-dwelling older people: a systematic review and meta-analysis. J. Am. Med. Dir. Assoc. 17, 881–888. doi: 10.1016/j.jamda.2016.05.013
Larner, A. J. (2012). Screening utility of the montreal cognitive assessment (MoCA): in place of—or as well as—the MMSE? Int. Psychogeriatr. 24, 391–396. doi: 10.1017/s1041610211001839
Lawton, M. P., and Brody, E. M. (1969). Assessment of older people: self-maintaining and instrumental activities of daily living. Gerontologist 9, 179–186. doi: 10.1093/geront/9.3_part_1.179
Lin, Y., Shan, P. Y., Jiang, W. J., Sheng, C., and Ma, L. (2019). Subjective cognitive decline: preclinical manifestation of Alzheimer’s disease. Neurol. Sci. 40, 41–49. doi: 10.1007/s10072-018-3620-y
Ma, L., Zhang, L., Sun, F., Li, Y., and Tang, Z. (2019). Cognitive function in prefrail and frail community-dwelling older adults in China. BMC Geriatr. 19:53.
Margioti, E., Kosmidis, M. H., Yannakoulia, M., Dardiotis, E., Hadjigeorgiou, G., Sakka, P., et al. (2020). Exploring the association between subjective cognitive decline and frailty: the Hellenic longitudinal investigation of aging and diet study (HELIAD). Aging Ment. Health 24, 137–147. doi: 10.1080/13607863.2018.1525604
Meiner, Z., Ayers, E., and Verghese, J. (2020). Motoric cognitive risk syndrome: a risk factor for cognitive impairment and dementia in different populations. Ann. Geriatr. Med. Res. 24, 3–14. doi: 10.4235/agmr.20.0001
Paddick, S. M., Kisoli, A., Mkenda, S., Mbowe, G., Gray, W. K., Dotchin, C., et al. (2017). Adaptation and validation of the Alzheimer’s disease assessment scale - cognitive (ADAS-Cog) in a low-literacy setting in sub-Saharan Africa. Acta Neuropsychiatr. 29, 244–251. doi: 10.1017/neu.2016.65
Panza, F., Lozupone, M., Solfrizzi, V., Sardone, R., Dibello, V., Di Lena, L., et al. (2018). Different cognitive frailty models and health and cognitive-related outcomes in older age: from epidemiology to prevention. J. Alzheimers Dis. 62, 993–1012. doi: 10.3233/jad-170963
Petersen, R. C., Stevens, J. C., Ganguli, M., Tangalos, E. G., Cummings, J. L., and DeKosky, S. T. (2001). Practice parameter: early detection of dementia: mild cognitive impairment (an evidence-based review). Report of the quality standards subcommittee of the american academy of neurology. Neurology 56, 1133–1142. doi: 10.1212/wnl.56.9.1133
Rabin, L. A., Smart, C. M., Crane, P. K., Amariglio, R. E., Berman, L. M., Boada, M., et al. (2015). Subjective cognitive decline in older adults: an overview of self-report measures used across 19 international research studies. J. Alzheimers Dis. 48, S63–S86.
Rami, L., Mollica, M. A., García-Sanchez, C., Saldaña, J., Sanchez, B., Sala, I., et al. (2014). The subjective cognitive decline questionnaire (SCD-Q): a validation study. J. Alzheimers Dis. 41, 453–466. doi: 10.3233/jad-132027
Reid, L. M., and Maclullich, A. M. (2006). Subjective memory complaints and cognitive impairment in older people. Dement. Geriatr. Cogn. Disord. 22, 471–485. doi: 10.1159/000096295
Robertson, D. A., Savva, G. M., and Kenny, R. A. (2013). Frailty and cognitive impairment—a review of the evidence and causal mechanisms. Ageing Res. Rev. 12, 840–851. doi: 10.1016/j.arr.2013.06.004
Rosen, W. G., Mohs, R. C., and Davis, K. L. (1984). A new rating scale for Alzheimer’s disease. Am. J. Psychiatry 141, 1356–1364.
Rossetti, H. C., Lacritz, L. H., Cullum, C. M., and Weiner, M. F. (2011). Normative data for the montreal cognitive assessment (MoCA) in a population-based sample. Neurology 77, 1272–1275. doi: 10.1212/wnl.0b013e318230208a
Ruan, Q., Xiao, F., Gong, K., Zhang, W., Zhang, M., Ruan, J., et al. (2020a). Prevalence of cognitive frailty phenotypes and associated factors in a community-dwelling elderly population. J. Nutr. Health Aging 24, 172–180. doi: 10.1007/s12603-019-1286-7
Ruan, Q., Xiao, F., Gong, K., Zhang, W., Zhang, M., Ruan, J., et al. (2020b). Demographically corrected normative Z-scores on the neuropsychological test battery in cognitively normal older Chinese adults. Dem. Geriatr. Cogn. Disord. 49, 375–383. doi: 10.1159/000505618
Ruan, Q., Yu, Z., Chen, M., Bao, Z., Li, J., and He, W. (2015). Cognitive frailty, a novel target for the prevention of elderly dependency. Ageing Res. Rev. 20, 1–10. doi: 10.1016/j.arr.2014.12.004
Saxton, J., Snitz, B. E., Lopez, O. L., Ives, D. G., Dunn, L. O., Fitzpatrick, A., et al. (2009). Functional and cognitive criteria produce different rates of mild cognitive impairment and conversion to dementia. J. Neurol. Neurosurg. Psychiatry 80, 737–743. doi: 10.1136/jnnp.2008.160705
Sliwinski, M., Lipton, R. B., Buschke, H., and Stewart, W. (1996). The effects of preclinical dementia on estimates of normal cognitive functioning in aging. J. Gerontol. B Psychol. Sci. Soc. Sci. 51, 217–225.
Solfrizzi, V., Scafato, E., Seripa, D., Lozupone, M., Imbimbo, B. P., D’Amato, A., et al. (2017). Reversible cognitive frailty, dementia, and all-cause mortality: the Italian longitudinal study on aging. J. Am. Med. Dir. Assoc. 18, 89.e1–89.e8.
Sugimoto, T., Sakurai, T., Ono, R., Kimura, A., Saji, N., Niida, S., et al. (2018). Epidemiological and clinical significance of cognitive frailty: a mini review. Ageing Res. Rev. 44, 1–7. doi: 10.1016/j.arr.2018.03.002
Thomas, K. R., Bangen, K. J., Weigand, A. J., Edmonds, E. C., Wong, C. G., Cooper, S., et al. (2020). Objective subtle cognitive difficulties predict future amyloid accumulation and neurodegeneration. Neurology 94, e397–e406.
Thomas, K. R., Edmonds, E. C., Eppig, J., Salmon, D. P., and Bondi, M. W. (2018). Using neuropsychological process scores to identify subtle cognitive decline and predict progression to mild cognitive impairment. J. Alzheimers Dis. 64, 195–204. doi: 10.3233/jad-180229
Trzepacz, P. T., Hochstetler, H., Wang, S., Walker, B., and Saykin, A. J. (2015). Relationship between the montreal cognitive assessment and mini-mental state examination for assessment of mild cognitive impairment in older adults. BMC Geriatr. 15:107.
Wallace, L. M. K., Theou, O., Godin, J., Andrew, M. K., Bennett, D. A., and Rockwood, K. (2019). Investigation of frailty as a moderator of the relationship between neuropathology and dementia in Alzheimer’s disease: a cross-sectional analysis of data from the Rush memory and aging project. Lancet Neurol. 18, 177–184. doi: 10.1016/s1474-4422(18)30371-5
Wattmo, C., and Wallin, ÅK. (2017). Early- versus late-onset Alzheimer’s disease in clinical practice: cognitive and global outcomes over 3 years. Alzheimers Res. Ther. 9:70.
Weintraub, S., Besser, L., Dodge, H. H., Teylan, M., Ferris, S., Goldstein, F. C., et al. (2018). Version 3 of the Alzheimer disease Centers’ Neuropsychological Test Battery in the Uniform Data Set (UDS). Alzheimer Dis. Assoc. Disord 32, 10–17. doi: 10.1097/wad.0000000000000223
Weintraub, S., Salmon, D., Mercaldo, N., Ferris, S., Graff-Radford, N. R., Chui, H., et al. (2009). The Alzheimer disease centers’ uniform data set (UDS): the neuropsychologic test battery. Alzheimer Dis. Assoc. Disord. 23, 91–101. doi: 10.1097/wad.0b013e318191c7dd
Keywords: neuropsychological test, mild cognitive impairment, pre-mild cognitive impairment subjective cognitive decline, physical pre-frailty, physical frailty, reversible cognitive frailty, potential reversible cognitive frailty
Citation: Ruan Q, Zhang W, Ruan J, Chen J and Yu Z (2021) Clinical and Objective Cognitive Measures for the Diagnosis of Cognitive Frailty Subtypes: A Comparative Study. Front. Psychol. 12:603974. doi: 10.3389/fpsyg.2021.603974
Received: 08 September 2020; Accepted: 08 April 2021;
Published: 24 May 2021.
Edited by:
Matteo De Marco, The University of Sheffield, United KingdomReviewed by:
Antonella Lopez, University of Bari Aldo Moro, ItalyCopyright © 2021 Ruan, Zhang, Ruan, Chen and Yu. This is an open-access article distributed under the terms of the Creative Commons Attribution License (CC BY). The use, distribution or reproduction in other forums is permitted, provided the original author(s) and the copyright owner(s) are credited and that the original publication in this journal is cited, in accordance with accepted academic practice. No use, distribution or reproduction is permitted which does not comply with these terms.
*Correspondence: Qingwei Ruan, MTM2NjE3MTczNDZAMTYzLmNvbQ==; Jie Chen, bGF1Z2hjaGVuQDEyNi5jb20=
Disclaimer: All claims expressed in this article are solely those of the authors and do not necessarily represent those of their affiliated organizations, or those of the publisher, the editors and the reviewers. Any product that may be evaluated in this article or claim that may be made by its manufacturer is not guaranteed or endorsed by the publisher.
Research integrity at Frontiers
Learn more about the work of our research integrity team to safeguard the quality of each article we publish.