- 1Department of Clinical Psychology, Faculty of Medicine and Health Sciences, University of East Anglia, Norwich, United Kingdom
- 2Medical Research Council (MRC) Cognition and Brain Sciences Unit, University of Cambridge, Cambridge, United Kingdom
Children and adolescents with developmental problems are at increased risk of experiencing mental health problems. The Strengths and Difficulties Questionnaire (SDQ) is widely used as a screener for detecting mental health difficulties in these populations, but its use thus far has been restricted to groups of children with diagnosed disorders (e.g., ADHD). Transdiagnostic approaches, which focus on symptoms and soften or remove the boundaries between traditional categorical disorders, are increasingly adopted in research and practice. The aim of this study was to assess the potential of the SDQ to detect concurrent mental health problems in a transdiagnostic sample of children. The sample were referred by health and educational professionals for difficulties related to learning (N = 389). Some had one diagnosis, others had multiple, but many had no diagnoses. Parent-rated SDQ scores were significantly positively correlated with parent ratings of mental health difficulties on the Revised Child Anxiety and Depression Scale (RCADS). Ratings on the SDQ Emotion subscale significantly predicted the likelihood of having concurrent clinical anxiety and depression scores. Ratings on the Hyperactivity subscale predicted concurrent anxiety levels. These findings suggest the SDQ could be a valuable screening tool for identifying existing mental health difficulties in children recognized as struggling, as it can be in typically developing children and those with specific diagnoses.
Introduction
Mental health problems affect up to 15% of the school-age population and are more common in children with learning-related problems than typically developing children (Emerson and Hatton, 2007; Francis et al., 2019; Public Health England, 2019). Yet less than a third of children with learning problems receive mental health support (Dekker and Koot, 2003; Strømme and Diseth, 2007). This leads to poor long-term outcomes for individuals and increased economic and societal costs due to elevated mental health care needs, greater risks of school-exclusion and unemployment, and increased levels of antisocial behavior (Green et al., 2005; Joint Commissioning Panel for Mental Health, 2013; Ford et al., 2018; Anderson et al., 2019). The Strengths and Difficulties Questionnaire (SDQ; Goodman, 1997), a brief behavioral questionnaire that can be completed by teachers, parents, or children, has been used widely in community samples to predict psychopathology (Goodman et al., 2003; Goodman and Goodman, 2009). The aim of this study is to investigate whether parent ratings on the SDQ can be used to predict concurrent mental health symptoms in children with learning-related problems who are likely to be at increased risk of anxiety and depression. The sample are a large heterogeneous cohort of struggling learners recruited to the Centre for Attention, Learning and Memory (CALM) study. It includes children with learning-related difficulties such as dyslexia and ADHD, and others with impairments in attention, learning or memory that did not meet diagnostic thresholds but nonetheless were recognized by a health or educational professional as compromising the child’s school progress.
Children with developmental difficulties are at increased risk of mental health problems. Internalizing affective disorders, like anxiety and depression, are more common in children with diagnosed developmental disorders such as attention deficit hyperactivity disorder (ADHD; Biederman et al., 1996; Ford et al., 2003; Larson et al., 2011) and autism spectrum disorder (ASD; de Bruin et al., 2007; Simonoff et al., 2008; White et al., 2009; Strang et al., 2012; Rodgers and Ofield, 2018). Learning-related difficulties are also associated with heightened levels of affective symptoms. Children with specific language impairment (SLI, now termed developmental language disorder, DLD) are at increased risk of emotional problems and depressive disorders (Goh et al., 2013), and a diagnosis of DLD in early childhood significantly increases the risk of anxiety disorders in adolescence and young adulthood (Beitchman et al., 2001). Elevated symptoms of anxiety (Murray, 1978) and depression (Boetsch et al., 1996) are also common in children with dyslexia and literacy difficulties (Carroll et al., 2005). Children with comorbid developmental or learning problems and mental ill-health typically have lower self-esteem (Stevenson and Romney, 1984), poorer relationships with family and peers, and elevated levels of aggression (Kim et al., 2000) compared to children with only one condition. Providing these children with timely support is therefore paramount and underscores the need to evaluate tools for identifying symptoms of mental health disorders in at-risk groups.
Traditional models of identifying mental ill-health involve referrals and assessments by clinicians. Demands on health services often outstrip resources (Belfer, 2008; Hunt and Eisenberg, 2010), meaning alternative approaches to identifying children at-risk of poor mental health have been evaluated. These include school-wide screening programmes, curriculum-based pupil education, staff training, and teacher, parent or child questionnaires (see Anderson et al., 2019 for a review). These methods have been linked with increased support and better long-term mental health outcomes relative to children who have been identified through healthcare settings (Ford et al., 2008; Nemeroff et al., 2008; Sayal et al., 2010; Husky et al., 2011; Lyon et al., 2016). Brief questionnaires measuring psychopathological symptoms are increasingly used to identify children who need to access mental health services. Typical measures include the brief Rutter questionnaires (Elander and Rutter, 1996), the more comprehensive Child Behavior Checklist (CBCL; Achenbach and Edelbrock, 1991), and the SDQ (Goodman, 1997). The SDQ asks about positive and negative attributes across 5 scales with a total of 25 items: Emotional symptoms, Conduct problems, Hyperactivity/Inattention, Peer Relationship Problems and Prosocial Behavior. One advantage of the SDQ is that each scale is comprised of only five items. It is also widely and freely available for use by teachers and parents. Other tools such as the CBCL or direct assessments of children’s mental health symptoms (e.g., the Revised Child and Anxiety and Depression Scale (RCADS; Chorpita et al., 2000) are more commonly used in clinical settings. Further, the SDQ correlates strongly with the longer Rutter and CBCL scales and has been used successfully to predict the likelihood of psychiatric illness in the Mental Health of Children and Young People in England Survey (MHCYP) in 1999 (Meltzer et al., 2003), 2004 (Green et al., 2005) and 2017 (Sadler et al., 2018). These data show SDQ ratings are linearly related to the prevalence of a psychopathological disorders in community samples (Goodman and Goodman, 2009).
In terms of at-risk groups, SDQ scores provide a good indication of comorbid affective problems in children with ADHD, with stronger relationships for externalizing than internalizing problems (Bekker et al., 2016). In adolescents with ASD, SDQ scores at age 12 predict severe mood problems at age 16 (Simonoff et al., 2012), and in children with dyslexia, higher SDQ scores are associated with lower self-esteem (Terras et al., 2009). The SDQ has been used with groups of children with intellectual disabilities, but its utility with severely impaired learners is limited (Emerson et al., 2010). To date, there have been no investigations into the utility of the SDQ for identifying symptoms of mental health problems in the common struggling learner.
In the present study, we assessed the potential utility of the SDQ for mental health screening in a large sample of children experiencing learning-related problems. Children with developmental problems are typically categorized into discrete and highly selective diagnostic groups such as ADHD, ASD, or specific learning and communication disorders according to symptom criteria outlined in international classification systems such as the Diagnostic and Statistical Manual-5 (DSM-5; American Psychiatric Association, 2013). These criteria constitute a framework for defining children’s difficulties that is used both to guide practical support in schools and clinics, to study the causes and characteristics of individuals who are struggling, and to frame our understanding of the links between developmental disorders and mental health problems. The problem with this traditional diagnostic nosology is that it fails to capture the variability, high levels of co-occurrence, and symptom overlap of common cognitive, behavioral and learning problems (Kotov et al., 2017). Contemporary thinking within developmental psychology is that we should adopt a transdiagnostic approach that softens or removes the boundaries between discrete categories of disorder (e.g., Coghill and Sonuga-Barke, 2012; Holmes et al., 2019).
In line with a transdiagnostic approach, we recruited a highly heterogeneous population of poor learners through the larger Centre for Attention, Learning and Memory (CALM) project (Holmes et al., 2019). The sample is representative of the majority of children who are struggling in the classroom. All children were referred by a health or educational professional for difficulties in attention, learning and/or memory. In this way, they were functionally defined as struggling. They did not fit traditional discrete categories of developmental and learning disorders; some had a single diagnosis, others had multiple diagnoses, but the majority were undiagnosed despite coming to the attention of a health or educational professional for experiencing difficulties that were affecting their school progress. Using this unique sample, the aim of this study is to provide a critical test of the utility of the parent-rated SDQ for identifying concurrent mental health problems in a transdiagnostic sample of struggling learners who are common in the classroom. This study was pre-registered on AsPredicted (#13396).
Materials and Methods
Procedure
Data were collected through the Centre for Attention, Learning and Memory (CALM) a developmental research clinic based at the MRC Cognition and Brain Sciences Unit, University of Cambridge, United Kingdom. Children aged 5–18 years were referred by health and education professionals for problems in attention, learning and/or memory. Families accepted into the study attended the clinic. Children completed a 3.5–4 h cognitive assessment, and parents/carers completed questionnaires measuring the child’s behavior, family history and mental health. See Holmes et al. (2019) for the study protocol. The study was approved by the local NHS Research Ethics Committee (13/EE/0157). Children assented to participation and parents/carers provided written informed consent on behalf of the child. For the majority of children, questionnaires were completed by parents, but the reporting agent in a small number of cases was a legal guardian/carer.
Participants
The sample was drawn from the wider CALM project that includes 805 children who attended the CALM clinic between February 2014 and January 2019. The data analyzed include the SDQ (Goodman, 1997) and the RCADS-P (Chorpita et al., 2000). Children with complete data on these measures were included for analysis (N = 389). The RCADS-P was not introduced into the CALM testing protocol until December 2016, and it was only administered to children aged 8+. It was therefore only completed by 400 of the 805 children. This explains why the number of children included here does not correspond to the 805 in the full CALM cohort. Participant demographics, diagnoses, and referral routes are presented in Table 1.
Materials
The SDQ queries positive and negative attributes displayed by the child in the past 6 months across five subscales: Emotional Symptoms (e.g., often unhappy, downhearted), Conduct Problems (e.g., fights with other children), Hyperactivity/Inattention (e.g., constantly fidgeting or squirming), Peer Relationship Problems (e.g., tends to play alone) and Prosocial Behavior (e.g., considerate of other people’s feelings). Each subscale includes five items and the parent/carer rates each item as either: Never = 0, Somewhat True = 1 or Certainly True = 2. SDQ total scores of 17 and above are considered to be abnormal. Raw scores are used in the analyses reported here. Clinical cut-off raw scores for the subscales (out of a possible 10) are: Emotion ≥ 5, Conduct ≥ 4, Hyperactivity ≥ 7, Peer Problems ≥ 4, Prosocial Behavior ≤ 4.
The RCADS-P is designed to assess children’s symptoms in relation to DSM anxiety and major depressive disorders (Chorpita et al., 2000). Parents rate the frequency of their child’s feelings and behaviors on 47 items (Never = 0, Sometimes = 1, Often = 2 or Always = 3). RCADS-P has five subscales corresponding to anxiety disorders: Separation Anxiety Disorder (e.g., fears being alone at home), Social Phobia (e.g., worries they might look foolish), Generalized Anxiety Disorder (e.g., worries bad things will happen to them), Panic Disorder (e.g., suddenly feels really scared for no reason) and Obsessive Compulsive Disorder (e.g., has to do things over and over again). The sixth subscale is Depression (e.g., feels nothing is much fun anymore). Three total scores are derived: Total Anxiety (the five anxiety subscales), Depression (one scale), and Total Anxiety and Depression (all six subscales). Raw scores are used for the analyses, but T-scores are used for interpretation in the binomial regressions to categorize whether children’s scores were in the clinical range. For reference, T-scores of 65–69 are within the borderline clinical range. T-scores of 70 + are above the clinical cut-off. Both point to a need for further clinical investigation (Chorpita et al., 2000). RCADS-P is standardized for children aged 8 years and older. Norms were derived using the most recent version (3.3) of the scoring tool.
Statistical Analyses
A statistical analysis plan was pre-registered with AsPredicted (#13396). The pre-registered analysis plan states that linear, stepwise and binomial logistic regression models would be used. However, stepwise regressions were replaced with simultaneous regression methods because stepwise methods are considered exploratory and are best avoided outside of exploratory model building (Field, 2009). Our original plan was to explore the relationship between different subgroups within the sample (e.g., those with and without ADHD or ASD, and boys and girls). On re-consideration the decision was made prior to the commencement of analysis to remove these subgroup comparisons for three reasons. First, it would be paradoxical to compare children with and without different diagnoses such as ADHD in a study that explicitly seeks to put aside diagnostic labels to explore use of the SDQ in a transdiagnostic sample. Second, previous studies have explored the use of the SDQ in children with specific diagnoses (e.g., Simonoff et al., 2012; Bekker et al., 2016). And finally, the sample consisted of substantially more boys than girls, meaning our power to explore patterns of relationships in the girls only would have been limited. In addition to the analyses outlined in the pre-registration, confirmatory factor analyses (CFA) were run on the SDQ and RCADS to check they fit the data collected here.
The analyses conducted were as follows. Regression analyses were conducted using simple and simultaneous multiple regressions, and binomial logistic regressions. Regression analyses were followed-up with Receiver Operator Characteristics (ROC) to determine optimal SDQ cut-off scores for distinguishing children with clinical levels of Total Anxiety, Depression, and Total Anxiety and Depression. Simple and simultaneous linear regressions were used to test whether SDQ scores (total and subscales) predicted ratings of Total Anxiety, Depression, and Total Anxiety and Depression as reported in the RCADS-P. For significant predictors, a series of regression models were compared to examine the relative contribution of each significant SDQ subscale in predicting RCADS-P scores. The contributions of the different predictors were compared using change in R2 and F. The models were compared in pairs (model 1 vs. model 2, and model 2 vs. model 3 etc.) to test whether there was a significant change with the addition of each predictor. Binomial logistic regressions were then used to test whether SDQ scores (total and subscales) predicted the likelihood of a child scoring within the clinical range on the RCADS-P. Where SDQ total and subscales significantly predicted the likelihood of a clinical RCADS-P score, ROC curves were used to identify optimum SDQ cut-off scores.
The RCADS-P manual provides age-standardized T-scores, but the SDQ does not. For this reason, raw scores were entered into the analyses for both questionnaires. To check whether controlling for age affected the outcomes, the analyses were also conducted accounting for age: regression analyses were conducted using age-controlled residual scores for each subscale and total scores of the RCADS-P and the SDQ. These were calculated by predicting raw scores from age and saving the residuals for inclusion in the analyses (see Supplementary Materials). The overall conclusions did not differ when age was taken into account. For this reason, the analyses reported here are conducted on raw scores. All outcomes controlling for age are reported in the Supplementary Materials.
Results
Descriptive statistics for all measures are presented in Table 2. Approximately half of the sample were rated within the abnormal range on the SDQ Emotion (49%), Conduct (52%), Peer Problems (47%), and Prosocial Behavior (46%) subscales. Larger proportions were in the abnormal range for hyperactivity (64%) and overall difficulties (Total 67%). Close to a third of the sample were rated as having scores within the clinical range on the RCADS for Total Anxiety (29%) and Total Anxiety and Depression (34%), and almost half were within the clinical range for Depression (43%).
A CFA was used to test the fit of the standard five-factor structure of the SDQ in which the 25 items load on to five factors measuring Conduct Problems, Emotion, Hyperactivity, Peer Problems and Prosocial Behaviors (see Supplementary Figure 1). Fit statistics indicated that the five-factor model was an acceptable fit to the data, χ2(265) = 1006.44, p < 0.0001; RMSEA = 0.061; CFI = 0.977; SRMR = 0.074 (Schermelleh-Engel et al., 2003). Similar outcomes have been reported for participants with ADHD (Becker et al., 2006; Hall et al., 2019) and typically developing children in a similar age range to those included here (Goodman, 2001; Woerner et al., 2004; Van Roy et al., 2008; Niclasen et al., 2012).
A second CFA was conducted to test the fit of the standard 6 factor structure of the RCADS, in which the 47 items load on to measures of Separation Anxiety, Social Phobia, Generalized Anxiety Disorder, Panic Disorder, Obsessive Compulsive Disorder, and Major Depressive Disorder (see Supplementary Figure 2). Fit statistics indicated that the six-factor model was an acceptable fit to the data, χ2(1,019) = 2618.36, p < 0.0001; RMSEA = 0.071; CFI = 0.976; SRMR = 0.088. This underscores the validity of the RCADS with the current sample, and the use of the sum scales for anxiety and depression in subsequent analyses.
Significant associations were found between all subscales of the SDQ and RCADS-P (see Supplementary Figure 3). SDQ Total scores were strongly related to ratings of Total Anxiety (r = 0.57), Depression (r = 0.68), and Total Anxiety and Depression (r = 0.62). SDQ Emotion scores also strongly correlated with ratings of Total Anxiety (r = 0.75), Depression (r = 0.64), and Total Anxiety and Depression (r = 0.77), all p < 0.01.
Do SDQ Total and Subscale Scores Predict RCADS Ratings of Anxiety and Depression?
Simple and multiple linear regressions were conducted to test whether SDQ scores predicted ratings of mental health. Regressions with the addition of each significant predictor were then compared to determine how much unique variance each predictor contributed to the model. Significant changes in F and R2 were recorded. Results for the simple linear and multiple regressions are summarized in Table 3, and model comparison showing significant changes in F and R2 are summarized in Table 4.
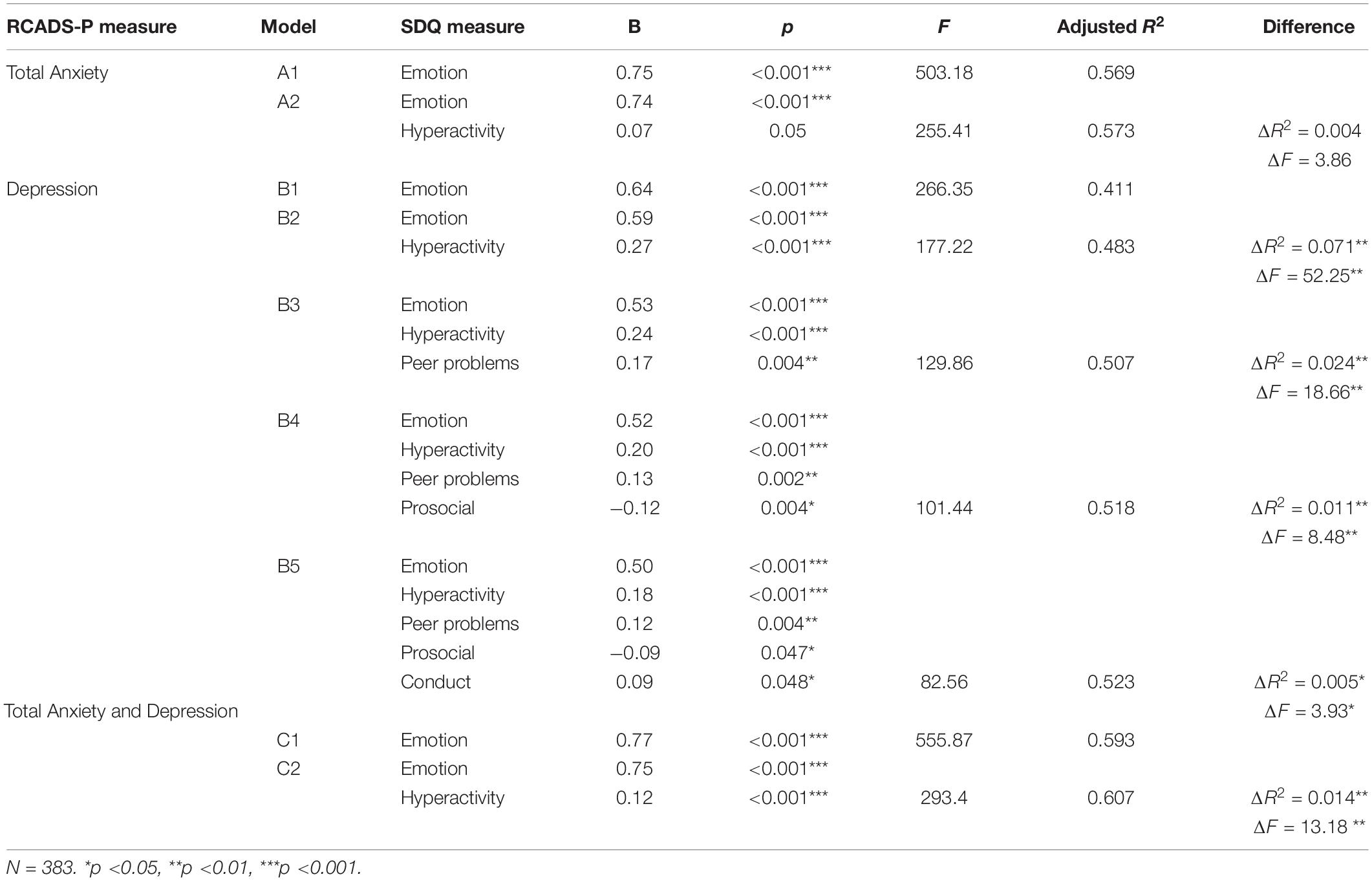
Table 4. Model comparisons for SDQ subscales identified as significant predictors of RCADS-P scores in simultaneous linear regressions.
Simple linear regression showed that Total SDQ ratings significantly predicted Total Anxiety scores, F(1, 381) = 179.2, p <0.001, and accounted for approximately 30% of the variance. A multiple linear regression model with all subscales was significant, F(5, 377) = 103.2, p <0.0001. However, only the Emotion and Hyperactivity subscales significantly predicted anxiety ratings, and accounted for ∼57% variance (see Table 3). The model including both the Emotion and Hyperactivity (model A2) subscales accounted for marginally more variance than the Emotion subscale alone, ΔF(1, 380) = 3.86, p = 0.05, ΔR2 = 0.004 (model A1) (see Table 4). This reveals that the Hyperactivity subscale makes an important contribution to Total Anxiety scores, but it should be noted that this contribution is less than 1% after variance accounted for by the Emotion subscale is taken into account.
Total SDQ scores accounted for 46% of variance in depression ratings, F(1, 381) = 330.3, p <0.001). All five SDQ subscales, Emotion, Conduct, Hyperactivity, Peer Problems and Prosocial Behavior predicted 52% of Depression scores, F(5, 377) = 82.56, p <0.0001 (see Table 3). Each scale contributed a significant unique amount of variance and improved the model fit, all ΔF, p <0.05 (Table 4). The model comparisons revealed the best model was one including all predictors (model B5). The Emotion subscale accounted for 41% variance in depression ratings, while Hyperactivity, ΔR2 = 0.071, Peer Problems, ΔR2 = 0.024, Prosocial Behavior, ΔR2 = 0.011, and Conduct, ΔR2 = 0.005, collectively contributed a further 11% of variance.
Total SDQ scores predicted 39% of variance in combined Total Anxiety and Depression scores, F(1, 381) = 244.1, p <0.001. Multiple linear regressions revealed that both the Emotion and Hyperactivity subscales significantly predicted Total Anxiety and Depression scores, accounting for 61% of variance, F(5, 377) = 118.3, p <0.001 (see Table 3). A model including both predictors (C2) was significantly better than a model with only Emotion (C1) entered; ΔF(1, 380) = 13.18, p <0.001, ΔR2 = 0.014 (see Table 4). The Emotion subscale accounted for 59% variance in ratings, while Hyperactivity contributed a further 2% of variance.
Do SDQ Total and Subscale Scores Predict Clinical Levels of Anxiety and Depression?
Binomial logistic regressions were used to test whether SDQ scores predicted the likelihood of a child scoring within the clinical range on the RCADS-P measures (see Table 5). Higher SDQ Total scores were significantly associated with an increased likelihood of having a score in the clinical range on all three RCADS-P scales, Total Anxiety, χ2(1) = 102.57, p <0.0001, Depression, χ2(1) = 144.23, p <0.0001, Total Anxiety and Depression, χ2(1) = 108.62, p <0.0001.
Logistic regressions including all five SDQ subscales revealed that together the subscales were significantly associated with an increased likelihood of scoring within the clinical range on Total Anxiety, χ2(5) = 165.81, p <0.0001, Depression, χ2(5) = 171.11, p <0.0001, and Total Anxiety and Depression, χ2(5) = 179.62, p <0.0001. The Emotion and Hyperactivity subscales were the only significant predictors in each case. An increase in 1 point on the Emotion subscale increased the odds of clinical range Total Anxiety scores by 1.94, Depression by 1.59, and Total Anxiety and Depression by 1.94. Similarly, an increase in 1 point on the Hyperactivity subscale increased the odds of clinical range Total Anxiety scores by 1.27, Depression by 1.25, and Total Anxiety and Depression by 1.20.
What SDQ Cut-off Scores Predict Anxiety and Depression?
ROC analyses were used to identify optimum cut-off scores for the SDQ Emotion and Hyperactivity subscales that would indicate a child has elevated symptoms of anxiety and depression. Plotting SDQ Emotion against RCADS-P Total Anxiety and Total Anxiety and Depression showed excellent levels of discrimination (Hosmer et al., 2013): Total Anxiety, 0.900, 95% CI = 0.860–0.940; Total Anxiety and Depression, 0.901, 95% CI = 0.864–0.938). Visual inspection of the coordinates of the curve suggest an optimal cut-off score of 5 on the SDQ Emotion subscale predicts clinical levels of Total Anxiety and Total Anxiety and Depression. The SDQ Emotion and RCADS-P Depression scales showed an adequate level of discrimination (0.850, 95% CI = 0.809–0.890), and visual inspection of the curve also suggested a cut-off score of 5 on the Emotion scale predicted clinical depression scores.
Plotting SDQ Hyperactivity against Total Anxiety, Depression, and Total Anxiety and Depression showed acceptable levels of discrimination: Anxiety, 0.744, 95% CI = 0.686–0.802; Depression, 0.763, 95% CI = 0.713–0.812, Total Anxiety and Depression, 0.710, 95% CI = 0.653–0.767). Visual inspection of the coordinates of the curve suggest an optimal cut-off score of 10 on the SDQ Hyperactivity subscale predicts clinical levels of Total Anxiety, Depression and Total Anxiety and Depression.
Discussion
The primary aim of this study was to test whether a short parent rating scale, the Strengths and Difficulties Questionnaire (SDQ; Goodman, 1997), was associated with high scores on the RCADS (Chorpita et al., 2000) measures of anxiety and/or depression in a large mixed sample of struggling learners. The SDQ has been used widely with groups of children with developmental problems categorized according to traditional diagnostic nosology (e.g., those with ADHD or ASD). This study is the first test of its utility with a heterogeneous, transdiagnostic sample of poor learners. It is both timely and important. The frequency of depression and anxiety in children and adolescents is growing (Sadler et al., 2018), and mental health difficulties are far more common in children with developmental difficulties than typically developing children (Boetsch et al., 1996; Beitchman et al., 2001; Dekker et al., 2002; Carroll et al., 2005; Morgan et al., 2008; Goh et al., 2013; McIntosh et al., 2013). Developmental problems are increasingly being studied using transdiagnostic approaches that aim to understand the processes and causes of symptoms that occur across individuals irrespective of diagnostic status (Casey et al., 2014; Sonuga-Barke and Coghill, 2014; Sonuga-Barke et al., 2016; Kotov et al., 2017). It is therefore vital to evaluate tools to identify mental health symptoms in children who are struggling at school, irrespective of diagnoses, so assessments remain useful as the field progresses.
Total SDQ scores were strong predictors of concurrent mental health problems in the current sample, as they are both for community samples (Goodman and Goodman, 2009) and children with traditionally categorized developmental disorders such as ADHD and dyslexia (Terras et al., 2009; Simonoff et al., 2012; Bekker et al., 2016). This demonstrates the validity of the SDQ as a tool with which to detect existing mental health problems in mixed groups of struggling learners, an important finding given both the move toward transdiagnostic approaches and increasing pressure on schools to identify children struggling with mental health problems (Department of Health and Education, 2018). The SDQ is freely available and simple to administer. It contains only 25 items, can be completed by parents, teachers or children themselves, and requires no clinical interpretation. The current data were collected from parents, but the items are identical for teachers and ratings between teachers and parents are often consistent (Stone et al., 2010). This suggests the tool could be used in schools with children who are falling behind cognitively and academically to determine whether they have elevated levels of anxiety and depression that would warrant further investigation.
The Emotion subscale, which includes items related to children’s mood and feelings, predicted more variance in RCADS Total Anxiety, Depression and Total Anxiety and Depression scores than Total SDQ scores. This is not surprising due to the overlap in items between the Emotion subscale of the SDQ and the RCADS. What is important, however, is that the Emotion subscale of the SDQ is shorter (only five items) and easier for parents and teachers to access and administer than RCADS and other scales that directly assess symptoms of anxiety and depression. ROC analyses identified that a cut-off score of 5 or above on the Emotion scale identified elevated levels of depression, anxiety and combined anxiety and depression on the RCADS. This score aligns with that set by Goodman (1997) in the original UK community study, and suggests the Emotion subscale cut-off is appropriate for both typically developing children and struggling learners in the United Kingdom. This is important because standard SDQ cut-off values are not valid across all samples and countries. A 2018 scoping review of studies in African nations determined that the UK-based SDQ cut-off scores were not appropriate (Hoosen et al., 2018), and in other countries, including China (Du et al., 2008) and India (Bhola et al., 2016), revised cut-off scores have been created. Revised values have also been identified for groups of children with specific neurodevelopmental differences. For example, Bekker and colleagues identified a higher Emotion subscale cut-off of 6 for determining externalizing comorbidities for a sample of children with ADHD (Bekker et al., 2016). The current data therefore reinforce the use of the Emotion subscale as a tool with which to identify concurrent symptoms of mental health problems in children who are struggling at school.
Anxiety was uniquely linked with hyperactivity in our sample of struggling learners. Although the effects were small, models containing both the Emotion and Hyperactivity subscales of the SDQ explained significantly more variance in both RCADS scales measuring anxiety (Total Anxiety and Total Anxiety and Depression) than models containing Emotion alone. Children with higher scores on Hyperactivity were also more likely to have clinical range Total Anxiety scores. Why might hyperactivity and anxious traits co-occur in this sample of poor learners? The cohort is characterized by poor executive function skills (see Holmes et al., 2020), like many other more specific groups of children with academic and learning-related problems (e.g., Carretti et al., 2009; Szucs et al., 2013; Yeniad et al., 2013). Executive function impairments are also implicated in both hyperactivity (Tripp and Alsop, 2001; Willcutt et al., 2005; Castellanos et al., 2006) and anxiety (Russell, 2003; Gotlib and Joorman, 2010; Crocker et al., 2013; Sharp et al., 2015). Executive function deficits might therefore contribute to all three characteristics of our sample: hyperactivity, anxiety and poor school achievement. A related possibility is that anxiety and hyperactivity have a shared neurocognitive basis (Schatz and Rostain, 2006). For example, executive dysfunction (Barkley, 1997; Brown, 2000; Holmes et al., 2014), dysregulated arousal (Aston-Jones et al., 2000), and abnormal patterns of brain activity and connectivity originating from the prefrontal cortex (PFC) are shared in both anxiety and hyperactivity (Levy, 2004). PFC activity is related to executive functions and gates emotional activity through projections to limbic regions such as the amygdala and nucleus accumbens. Inefficient PFC responsivity might therefore lead to both dysregulated cognitive control and emotional over-reactivity, explaining comorbid symptoms of anxiety and hyperactivity (Beesdo et al., 2009).
In contrast to anxiety, elevated symptoms of depression were linked more broadly to externalizing symptoms measured by the SDQ. Ratings on the Emotion subscale were the strongest predictor of Total Depression scores, but the other SDQ subscales also predicted a small yet significant amount of variance in depressive symptoms. The association between conduct problems and depression might reflect the presence of oppositional behaviors that can appear in childhood alongside bipolar disorder (Axelson, 2013) and form part of Disruptive Mood Dysregulation Disorder (DSM-5, 2013). For hyperactivity, depressive traits could be misdiagnosed signs of demoralization that hyperactive children experience in the face of social and school failure (Biederman et al., 1991). However, it may be that hyperactivity and depression have independent courses that can co-occur, and that the impacts of hyperactivity and interpersonal difficulties on the persistence of depression are separable but potentially additive (Biederman et al., 1998). Longitudinal data are needed to test the causal pathways between these externalizing problems and depression.
Low levels of prosocial behavior and elevated problems with peers also predicted elevated levels of depression in the struggling learners. Again, these effects were small so should not be over-interpreted, but they are consistent with a broader literature showing that children who fall behind academically are at increased risk of being bullied (Mishna, 2003; Twyman et al., 2010). Children who are bullied experience high levels of depression (Seals and Young, 2003; Brunstein Klomek et al., 2007; Perren et al., 2010) and longitudinal studies show that the relationship between bullying and depression is mediated by the quality of friendships (van Harmelen et al., 2016), prosociality (Martin and Huebner, 2007; Andrade and Tannock, 2014) and peer support (Griese and Buhs, 2014). Together with our current findings, these data suggest that interventions focussing on social experiences and skills may help build resilience against low mood in children who are struggling at school.
Conclusion
In conclusion, the current study demonstrates that the parent version of the SDQ, and in particular the Emotion subscale, can be used to predict concurrent symptoms of anxiety and depression in a mixed sample of children who are struggling at school. At a practical level, this provides evidence for the use of the Emotion scale of the SDQ by teachers and parents to index children who may currently have elevated symptoms of anxiety and depression, removing the need to use tools such as the RCADS that are restricted to clinical use. A specific link was found between hyperactivity and anxiety, while a broader set of behaviors including conduct problems and the quality of social interactions were associated with depression alongside hyperactivity. These associations were significant, but the coefficients and effect sizes small, so caution should be exercised when interpreting them: the Hyperactivity subscale of the SDQ should not be used to index possible current symptoms of anxiety based on the current data, nor should the Hyperactivity, Prosocial or Peer Problems scales be used to detect current levels of low mood. The weak associations between hyperactivity and anxiety and depression likely reflect the well-documented comorbidity between disorders of impulsivity/hyperactivity and anxious-depressive traits (Mennin et al., 2000; Schatz and Rostain, 2006): an estimated 20–30% of children and adolescents with hyperactive disorders have a comorbid internalizing disorder (Biederman et al., 1996, 2005; Ford et al., 2003; Chronis-Tuscano et al., 2010; Rucklidge, 2010). The links between social problems and low mood point towards promoting good friendship groups and positive behaviour to affect low mood in children who struggle at school, but future longitudinal studies are needed to test the robustness of the associations reported here.
Data Availability Statement
The data analyzed in this study is subject to the following licenses/restrictions. The data from the larger study from which these data were drawn will be made available via managed open access when the study is complete. Requests to access these datasets should be directed to calm@mrc-cbu.cam.ac.uk.
Ethics Statement
The studies involving human participants were reviewed and approved by local NHS Research Ethics Committee (13/EE/0157). Written informed consent to participate in this study was provided by the participants’ legal guardian/next of kin.
Author Contributions
AB and JH designed the study. The CALM Team collected the data. AB and JG completed the data analyses. AB, JG, and JH wrote the manuscript. All authors contributed to the article and approved the submitted version.
Funding
This research was funded by intramural funding awarded to the MRC Cognition and Brain Sciences Unit, University of Cambridge, by the Medical Research Council of Great Britain.
Conflict of Interest
The authors declare that the research was conducted in the absence of any commercial or financial relationships that could be construed as a potential conflict of interest.
Acknowledgments
The CALM Team includes lead investigators Duncan Astle, Kate Baker, Susan Gathercole, Joni Holmes, Rogier Kievit and Tom Manly. Data collection is assisted by a team of researchers and PhD students that includes Joe Bathelt, Marc Bennett, Giacomo Bignardi, Sarah Bishop, Erica Bottacin, Lara Bridge, Diandra Brkic, Annie Bryant, Sally Butterfield, Elizabeth Byrne, Gemma Crickmore, Edwin Dalmaijer, Fánchea Daly, Tina Emery, Laura Forde, Grace Franckel, Delia Furhmann, Andrew Gadie, Sara Gharooni, Jacalyn Guy, Erin Hawkins, Agnieszka Jaroslawska, Sara Joeghan, Amy Johnson, Jonathan Jones, Silvana Mareva, Elise Ng-Cordell, Sinead O’Brien, Cliodhna O’Leary, Joseph Rennie, Ivan Simpson-Kent, Roma Siugzdaite, Tess Smith, Stephani Uh, Francesca Woolgar, Mengya Zhang. We wish to thank the many professionals working in children’s services in the South-East and East of England for their support, and to the children and their families for giving up their time to visit the clinic.
Supplementary Material
The Supplementary Material for this article can be found online at: https://www.frontiersin.org/articles/10.3389/fpsyg.2020.587821/full#supplementary-material
References
Achenbach, T. M., and Edelbrock, C. (1991). Child behavior checklist. Burlington, VT: University of Vermont. Department of psychiatry.
Anderson, J. K., Ford, T., Soneson, E., Coon, J. T., Humphrey, A., Rogers, M., et al. (2019). A systematic review of effectiveness and cost-effectiveness of school-based identification of children and young people at risk of, or currently experiencing mental health difficulties. Psychol. Med. 49, 9–19. doi: 10.1017/S0033291718002490
Andrade, B. F., and Tannock, R. (2014). Sustained impact of inattention and hyperactivity-impulsivity on peer problems: mediating roles of prosocial skills and conduct problems in a community sample of children. Child Psychiatry Human Dev. 45, 318–328. doi: 10.1007/s10578-013-0402-x
American Psychiatric Association. (2013). Diagnostic and statistical manual of mental disorders (DSM-5®). Washington, DC: American Psychiatric Publishing.
Aston-Jones, G., Rajkowski, J., and Cohen, J. (2000). Locus Coeruleus and regulation of behavioral flexibility and attention. Prog. Brain Res. 126, 165–182. doi: 10.1016/s0079-6123(00)26013-5
Axelson, D. (2013). Taking disruptive mood dysregulation disorder out for a test drive. Am. J. Psychiatry 170, 136–139. doi: 10.1176/appi.ajp.2012.12111434
Barkley, R. A. (1997). Behavioral inhibition, sustained attention, and executive functions: constructing a unifying theory of ADHD. Psychol. Bull. 121, 65–94. doi: 10.1037/0033-2909.121.1.65
Becker, A., Steinhausen, H., Baldursson, G., Dalsgaard, S., Lorenzo, J. M., Ralston, J. S., et al. (2006). Psychopathological screening of children with ADHD: strengths and difficulties questionnaire in a pan-European study. Eur. Child Adolesc. Psychiatry 15, 56–62. doi: 10.1007/s00787-006-1008-7
Beesdo, K., Lau, J. Y. F., Guyer, A. E., McClure-Tone, E. B., Monk, C. S., Nelson, E. E., et al. (2009). Common and distinct amygdala-function perturbations in depressed vs anxious adolescents. Arch. Gen. Psychiatry 66, 275–285. doi: 10.1001/archgenpsychiatry.2008.545
Beitchman, J., Wilson, B., Johnson, C., Atkinson, L., Young, A., Adlaf, E., et al. (2001). Fourteen-Year follow-up of speech/language-impaired and control children: psychiatric outcome. J. Am. Acad. Child Adolesc. Psychiatry 40, 75–82. doi: 10.1097/00004583-200101000-00019
Bekker, J., Bruck, D., and Sciberras, E. (2016). Congruent validity of the strengths and difficulties questionnaire to screen for comorbidities in children with ADHD. J. Atten. Disord. 20, 879–888. doi: 10.1177/1087054713496462
Belfer, M. L. (2008). Child and adolescent mental disorders: the magnitude of the problem across the globe. J. Child Psychol. Psychiatry 49, 226–236. doi: 10.1111/j.1469-7610.2007.01855.x
Bhola, P., Sathyanarayanan, V., Rekha, D. P., Daniel, S., and Thomas, T. (2016). Assessment of self-reported emotional and behavioral difficulties among pre-university college students in Bangalore, India. Indian J. Community Med. 41, 146–150. doi: 10.4103/0970-0218.177536
Biederman, J., Faraone, S., Milberger, S., Guite, J., Mick, E., Chen, L., et al. (1996). A prospective 4-Year follow-up study of Attention-Deficit Hyperactivity and related Disorders. Arch. Gen. Psychiatry 53, 437–446. doi: 10.1001/archpsyc.1996.01830050073012
Biederman, J., Faraone, S. V., Keenan, K., and Tsuang, M. T. (1991). Evidence of familial association between attention deficit disorder and major affective disorders. Arch. Gen. Psychiatry 48, 633–642. doi: 10.1001/archpsyc.1991.01810310051009
Biederman, J., Kwon, A., Aleardi, M., Chouinard, V.-A., Marino, T., Cole, H., et al. (2005). Absence of gender effects on attention deficit hyperactivity disorder: findings in nonreferred subjects. Am. J. Psychiatry 162, 1083–1089. doi: 10.1176/appi.ajp.162.6.1083
Biederman, J., Mick, E., and Faraone, S. V. (1998). Depression in attention deficit hyperactivity disorder (ADHD) children: ‘true’ depression or demoralization? J. Affect. Disord. 47, 113–122. doi: 10.1016/s0165-0327(97)00127-4
Boetsch, E. A., Green, P. A., and Pennington, B. F. (1996). Psychosocial correlates of dyslexia across the life span. Dev. Psychopathol. 8, 539–562. doi: 10.1017/S0954579400007264
Brown, T. (2000). Attention-deficit disorders and comorbidities in children, adolescents, and adults. Arlington, TX: American Psychiatric Publishing.
Brunstein Klomek, A., Marrocco, F., Kleinman, M., Schonfeld, I. S., and Gould, M. S. (2007). Bullying, depression, and Suicidality in adolescents. J. Am. Acad. Child Adolesce. Psychiatry 46, 40–49. doi: 10.1097/01.CHI.0000242237.84925.18
Carretti, B., Borella, E., Cornoldi, C., and De Beni, R. (2009). Role of working memory in explaining the performance of individuals with specific reading comprehension difficulties: a meta-analysis. Learn. Individ. Differ. 19, 246–251. doi: 10.1016/j.lindif.2008.10.002
Carroll, J. M., Maughan, B., Goodman, R., and Meltzer, H. (2005). Literacy difficulties and psychiatric disorders: evidence for comorbidity. J. Child Psychol. Psychiatry 46, 524–532. doi: 10.1111/j.1469-7610.2004.00366.x
Casey, B. J., Oliveri, M. E., and Insel, T. (2014). A neurodevelopmental perspective on the research domain criteria (RDoC) framework. Biol. Psychiatry 76, 350–353. doi: 10.1016/j.biopsych.2014.01.006
Castellanos, F. X., Sonuga-Barke, E. J. S., Milham, M. P., and Tannock, R. (2006). Characterizing cognition in ADHD: beyond executive dysfunction. Trends Cogn. Sci. 10, 117–123. doi: 10.1016/j.tics.2006.01.011
Chorpita, B. F., Yim, L., Moffitt, C., Umemoto, L. A., and Francis, S. E. (2000). Assessment of symptoms of DSM-IV anxiety and depression in children: a revised child anxiety and depression scale. Behav. Res. Ther. 38, 835–855. doi: 10.1016/S0005-7967(99)00130-8
Chronis-Tuscano, A., Molina, B. S. G., Pelham, W. E., Applegate, B., Dahlke, A., Overmyer, M., et al. (2010). Very early predictors of adolescent depression and suicide attempts in children with Attention-Deficit/Hyperactivity Disorder. Arch. Gen. Psychiatry 67, 1044–1051. doi: 10.1001/archgenpsychiatry.2010.127
Coghill, D., and Sonuga-Barke, E. J. S. (2012). Annual research review: categories versus dimensions in the classification and conceptualisation of child and adolescent mental disorders–implications of recent empirical study. J. Child Psychol. Psychiatry 53, 469–489. doi: 10.1111/j.1469-7610.2011.02511.x
Crocker, L. D., Heller, W., Warren, S. L., O’Hare, A. J., Infantolino, Z. P., and Miller, G. A. (2013). Relationships among cognition, emotion, and motivation: implications for intervention and neuroplasticity in psychopathology. Front. Human Neurosci. 7:261. doi: 10.3389/fnhum.2013.00261
de Bruin, E. I., Ferdinand, R. F., Meester, S., de Nijs, P. F. A., and Verheij, F. (2007). High rates of psychiatric Co-Morbidity in PDD-NOS. J. Autism Dev. Disord. 37, 877–886. doi: 10.1007/s10803-006-0215-x
Dekker, M., and Koot, H. M. (2003). DSM-IV disorders in children with borderline to moderate intellectual disability. I: prevalence and impact. J. Am. Acad. Child Adolesc. Psychiatry 42, 915–922. doi: 10.1097/01.CHI.0000046892.27264.1A
Dekker, M. C., Koot, H. M., van der Ende, J., and Verhulst, F. C. (2002). Emotional and behavioral problems in children and adolescents with and without intellectual disability. J. Child Psychol. Psychiatry 43, 1087–1098. doi: 10.1111/1469-7610.00235
Department of Health and Education (2018). Government response to the consultation on transforming children and young people’s mental health provision: A green paper and next steps. Available online at: https://assets. publishing.service.gov.uk/government/uploads/system/uploads/attachment_ data/file/728892/government-response-to-consultation-on-transforming-children-and-young-peoples-mental-health.pdf (accessed July, 2018).
Du, Y., Kou, J., and Coghill, D. (2008). The validity, reliability and normative scores of the parent, teacher and self report versions of the Strengths and Difficulties Questionnaire in China. Child Adolesc. Psychiatry Ment. Health 2:8. doi: 10.1186/1753-2000-2-8
Elander, J., and Rutter, M. (1996). Use and development of the Rutter parents’ and teachers’ scales. Int. J. Methods Psychiatr. Res. 6, 63–78. doi: 10.1002/(SICI)1234-988X(199607)6:2<63:AID-MPR151<3.3.CO;2-M
Emerson, E., Einfeld, S., and Stancliffe, R. J. (2010). The mental health of young children with intellectual disabilities or borderline intellectual functioning. Soc. Psychiatry Psychiatr. Epidemiol. 45, 579–587. doi: 10.1007/s00127-009-0100-y
Emerson, E., and Hatton, C. (2007). Mental health of children and adolescents with intellectual disabilities in Britain. Br. J. Psychiatry 191, 493–499. doi: 10.1192/bjp.bp.107.038729
Field, A. (2009). Discovering statistics using SPSS:(and sex and drugs and rock’n’roll). Thousand Oaks, CA: Sage.
Ford, T., Goodman, R., and Meltzer, H. (2003). The british child and adolescent mental health survey 1999: the prevalence of DSM-IV Disorders. J. Am. Acad. Child Adolesc. Psychiatry 42, 1203–1211. doi: 10.1097/00004583-200310000-00011
Ford, T., Hamilton, H., Meltzer, H., and Goodman, R. (2008). Predictors of service use for mental health problems among british schoolchildren. Child Adolesc. Ment. Health 13, 32–40. doi: 10.1111/j.1475-3588.2007.00449.x
Ford, T., Parker, C., Salim, J., Goodman, R., Logan, S., and Henley, W. (2018). The relationship between exclusion from school and mental health: a secondary analysis of the British Child and Adolescent Mental Health Surveys 2004 and 2007. Psychol. Med. 48, 629–641. doi: 10.1017/s003329171700215x
Francis, D. A., Caruana, N., Hudson, J. L., and McArthur, G. M. (2019). The association between poor reading and internalising problems: a systematic review and meta-analysis. Clin. Psychol. Rev. 67, 45–60. doi: 10.1016/J.CPR.2018.09.002
Goh, S., Yew, K., and O’Kearney, R. (2013). Emotional and behavioural outcomes later in childhood and adolescence for children with specific language impairments: meta-analyses of controlled prospective studies. J. Child Psychol. Psychiatry 54, 516–524. doi: 10.1111/jcpp.12009
Goodman, A., and Goodman, R. (2009). Strengths and difficulties questionnaire as a dimensional measure of child mental health. J. Am. Acad. Child Adolesc. Psychiatry 48, 400–403. doi: 10.1097/CHI.0B013E3181985068
Goodman, R. (1997). The strengths and difficulties questionnaire: a research note. J. Child Psychol. Psychiatry 38, 581–586. doi: 10.1111/j.1469-7610.1997.tb01545.x
Goodman, R. (2001). Psychometric properties of the strengths and difficulties questionnaire. J. Am. Acad. Child Adolesc. Psychiatry 40, 1337–1345.
Goodman, R., Ford, T., Simmons, H., Gatward, R., and Meltzer, H. (2003). Using the Strengths and Difficulties Questionnaire (SDQ) to screen for child psychiatric disorders in a community sample. Int. Rev. Psychiatry 15, 166–172. doi: 10.1080/0954026021000046128
Gotlib, I. H., and Joorman, J. (2010). Cognition and depression: current status and future directions. Ann. Rev. Clin. Psychol. 6, 285–312. doi: 10.1146/annurev.clinpsy.121208.131305
Green, H., Mcginnity, Á, Meltzer, H., Ford, T., and Goodman, R. (2005). Mental health of children and young people in Great Britain, 2004. Available online at: https://files.digital.nhs.uk/publicationimport/pub06xxx/pub06116/ment-heal-chil-youn-peop-gb-2004-rep1.pdf (accessed August 9,2018).
Griese, E. R., and Buhs, E. S. (2014). Prosocial behavior as a protective factor for children’s peer Victimization. J. Youth Adolesc. 43, 1052–1065. doi: 10.1007/s10964-013-0046-y
Hall, C. L., Guo, B., Valentine, A. Z., Groom, M. J., Daley, D., Sayal, K., et al. (2019). The validity of the Strengths and Difficulties Questionnaire (SDQ) for children with ADHD symptoms. PLoS One 14:e0218518. doi: 10.1371/journal.pone.0218518
Holmes, J., Bryant, A., Gathercole, S. E., and Team, C. (2019). Protocol for a transdiagnostic study of children with problems of attention, learning and memory (CALM). BMC Pediatr. 19:10. doi: 10.1186/s12887-018-1385-3
Holmes, J., Guy, J., Kievit, R., Bryant, A., Mareva, S., and Calm Team (2020). Cognitive dimensions of learning in children with problems in attention, learning and memory. J. Educ. Psychol. (in press).
Holmes, J., Hilton, K. A., Place, M., Alloway, T. P., Elliott, J. G., and Gathercole, S. E. (2014). Children with low working memory and children with ADHD: same or different? Front. Human Neurosci. 8:976. doi: 10.3389/fnhum.2014.00976
Hoosen, N., Davids, E. L., de Vries, P. J., and Shung-King, M. (2018). The Strengths and Difficulties Questionnaire (SDQ) in Africa: a scoping review of its application and validation. Child Adolesc. Psychiatry Ment. Health 12:6.
Hosmer, D. W., Lemeshow, S., and Sturdivant, R. X. (2013). Applied logistic regression, Vol. 398. Hoboken, NJ: John Wiley & Sons Inc.
Hunt, J., and Eisenberg, D. (2010). Mental health problems and help-seeking behavior among college students. J. Adolesc. Health 46, 3–10. doi: 10.1016/J.JADOHEALTH.2009.08.008
Husky, M. M., Kaplan, A., McGuire, L., Flynn, L., Chrostowski, C., and Olfson, M. (2011). Identifying adolescents at risk through voluntary school-based mental health screening. J. Adolesc. 34, 505–511. doi: 10.1016/J.ADOLESCENCE.2010.05.018
Joint Commissioning Panel for Mental Health (2013). Guidance for Commissioners: Child and Adolescent Mental Health Services. Available online at: www.jcpmh.info (accessed February 2, 2019)
Kim, J. A., Szatmari, P., Bryson, S. E., Streiner, D. L., and Wilson, F. J. (2000). The prevalence of anxiety and mood problems among children with autism and Asperger syndrome. Autism 4, 117–132. doi: 10.1177/1362361300004002002
Kotov, R., Krueger, R. F., Watson, D., Achenbach, T. M., Althoff, R. R., Bagby, R. M., et al. (2017). The Hierarchical Taxonomy of Psychopathology (HiTOP): a dimensional alternative to traditional nosologies. J. Abnorm. Psychol. 126, 454–477. doi: 10.1037/abn0000258
Larson, K., Russ, S. A., Kahn, R. S., and Halfon, N. (2011). Patterns of comorbidity, functioning, and service use for US children with ADHD. Pediatrics 127, 462–470. doi: 10.1542/peds.2010-0165
Levy, F. (2004). Synaptic gating and ADHD: a biological theory of comorbidity of ADHD and anxiety. Neuropsychopharmacology 29, 1589–1596. doi: 10.1038/sj.npp.1300469
Lyon, A. R., Ludwig, K., Wasse, J. K., Bergstrom, A., Hendrix, E., and McCauley, E. (2016). Determinants and functions of standardized assessment use among school mental health clinicians: a mixed methods evaluation. Adm. Policy Ment. Health 43, 122–134. doi: 10.1007/s10488-015-0626-0
Martin, K. M., and Huebner, E. S. (2007). Peer victimization and prosocial experiences and emotional well-being of middle school students. Psychol. Sch. 44, 199–208. doi: 10.1002/pits.20216
McIntosh, K., Ty, S. V., and Miller, L. D. (2013). Effects of School-Wide positive behavioral interventions and supports on internalizing problems: current evidence and future directions. J. Posit. Behav. Interv. 16, 209–218. doi: 10.1177/1098300713491980
Meltzer, H., Gatward, R., Goodman, R., and Ford, T. (2003). Mental health of children and adolescents in Great Britain. Int. Rev. Psychiatry 15, 185–187. doi: 10.1080/0954026021000046155
Mennin, D., Biederman, J., Mick, E., and Faraone, S. V. (2000). Towards defining a meaningful anxiety phenotype for research in ADHD children. J. Atten. Disord. 3, 192–199. doi: 10.1177/108705470000300402
Mishna, F. (2003). Learning disabilities and bullying. J. Learn. Disabil. 36, 336–347. doi: 10.1177/00222194030360040501
Morgan, P. L., Farkas, G., Tufis, P. A., and Sperling, R. A. (2008). Are reading and behavior problems risk factors for each other? J. Learn. Disabil. 41, 417–436. doi: 10.1177/0022219408321123
Murray, M. E. (1978). The relationship between personality adjustment and success in remedial programs in dyslexic children. Contemp. Educ. Psychol. 3, 330–339. doi: 10.1016/0361-476X(78)90035-8
Nemeroff, R., Levitt, J. M., Faul, L., Wonpat-Borja, A., Bufferd, S., Setterberg, S., et al. (2008). Establishing ongoing, early identification programs for mental health problems in our schools: a feasibility study. J. Am. Acad. Child Adolesc. Psychiatry 47, 328–338. doi: 10.1097/CHI.0b013e318160c5b1
Niclasen, J., Teasdale, T. W., Andersen, A.-M. N., Skovgaard, A. M., Elberling, H., and Obel, C. (2012). Psychometric properties of the danish strength and difficulties questionnaire: the sdq assessed for more than 70,000 raters in four different cohorts. PLoS One 7:e32025. doi: 10.1371/journal.pone.0032025
Perren, S., Dooley, J., Shaw, T., and Cross, D. (2010). Bullying in school and cyberspace: associations with depressive symptoms in Swiss and Australian adolescents. Child Adolesc. Psychiatry Ment. Health 4:28. doi: 10.1186/1753-2000-4-28
Public Health England (2019). Mental health and wellbeing: JSNA toolkit. 5: Children and young people. Wellington House: Public Health England.
Rodgers, J., and Ofield, A. (2018). Understanding, recognising and treating Co-occurring anxiety in autism. Curr. Dev. Disord. Rep. 5, 58–64. doi: 10.1007/s40474-018-0132-7
Rucklidge, J. J. (2010). Gender differences in attention-deficit/hyperactivity disorder. Psychiatr. Clin. North America 33, 357–373. doi: 10.1016/j.psc.2010.01.006
Russell, J. A. (2003). Core affect and the psychological construction of emotion. Psychol. Rev. 110, 145–172. doi: 10.1037/0033-295x.110.1.145
Sadler, K., Vizard, T., and Ford, T. (2018). Mental health of children and young people in England, 2017: Summary of key findings. Available online at: https://dera.ioe.ac.uk/32622/1/MHCYP 2017 Summary.pdf (accessed April 28, 2020).
Sayal, K., Owen, V., White, K., Merrell, C., Tymms, P., and Taylor, E. (2010). Impact of early school-based screening and intervention programs for ADHD on children’s outcomes and access to services. Arch. Pediatr. Adolesc. Med. 164, 462–469. doi: 10.1001/archpediatrics.2010.40
Schatz, D. B., and Rostain, A. L. (2006). ADHD with comorbid anxiety: a review of the current literature. J. Atten. Disord. 10, 141–149. doi: 10.1177/1087054706286698
Schermelleh-Engel, K., Moosbrugger, H., and Müller, H. (2003). Evaluating the fit of structural equation models: tests of significance and descriptive goodness-of-fit measures. Methods Psychol. Res. Online 8, 23–74.
Seals, D., and Young, J. (2003). Bullying and victimization: prevalence and relationship to gender, grade level, ethnicity, self esteem, and depression. Adolescence 152, 735–748.
Sharp, P. B., Miller, G. A., and Heller, W. (2015). Transdiagnostic dimensions of anxiety: neural mechanisms, executive functions, and new directions. Int. J. Psychophysiol. 98, 365–377. doi: 10.1016/j.ijpsycho.2015.07.001
Simonoff, E., Jones, C. R. G., Pickles, A., Happé, F., Baird, G., and Charman, T. (2012). Severe mood problems in adolescents with autism spectrum disorder. J. Child Psychol. Psychiatry 53, 1157–1166. doi: 10.1111/j.1469-7610.2012.02600.x
Simonoff, E., Pickles, A., Charman, T., Chandler, S., Loucas, T., and Baird, G. (2008). Psychiatric disorders in children with autism spectrum disorders: prevalence, comorbidity, and associated factors in a Population-Derived Sample. J. Am. Acad. Child Adolesc. Psychiatry 47, 921–929. doi: 10.1097/CHI.0B013E318179964F
Sonuga-Barke, E. J. S., and Coghill, D. (2014). Editorial Perspective: laying the foundations for next generation models of ADHD neuropsychology. J. Child Psychol. Psychiatry 55, 1215–1217. doi: 10.1111/jcpp.12341
Sonuga-Barke, E. J. S., Cortese, S., Fairchild, G., and Stringaris, A. (2016). Annual Research Review: transdiagnostic neuroscience of child and adolescent mental disorders–differentiating decision making in attention-deficit/hyperactivity disorder, conduct disorder, depression, and anxiety. J. Child Psychol. Psychiatry 57, 321–349. doi: 10.1111/jcpp.12496
Stevenson, D. T., and Romney, D. M. (1984). Depression in learning disabled children. J. Learn. Disabil. 17, 579–582. doi: 10.1177/002221948401701002
Stone, L. L., Otten, R., Engels, R. C. M. E., Vermulst, A. A., and Janssens, J. M. A. M. (2010). Psychometric properties of the parent and teacher versions of the strengths and difficulties questionnaire for 4- to 12-year-olds: a review. Clin. Child Family Psychol. Rev. 13, 254–274. doi: 10.1007/s10567-010-0071-2
Strang, J. F., Kenworthy, L., Daniolos, P., Case, L., Wills, M. C., Martin, A., et al. (2012). Depression and anxiety symptoms in children and adolescents with autism spectrum disorders without intellectual disability. Res. Autism Spectr. Disord. 6, 406–412. doi: 10.1016/j.rasd.2011.06.015
Strømme, P., and Diseth, T. H. (2007). Prevalence of psychiatric diagnoses in children with mental retardation: data from a population-based study. Dev. Med. Child Neurol. 42, 266–270. doi: 10.1111/j.1469-8749.2000.tb00083.x
Szucs, D., Devine, A., Soltesz, F., Nobes, A., and Gabriel, F. (2013). Developmental dyscalculia is related to visuo-spatial memory and inhibition impairment. Cortex 49, 2674–2688. doi: 10.1016/j.cortex.2013.06.007
Terras, M. M., Thompson, L. C., and Minnis, H. (2009). Dyslexia and psycho-social functioning: an exploratory study of the role of self-esteem and understanding. Dyslexia (Chichester, England) 15, 304–327. doi: 10.1002/dys.386
Tripp, G., and Alsop, B. (2001). Sensitivity to reward delay in children with attention deficit hyperactivity disorder (ADHD). J. Child Psychol. Psychiatry 42, 691–698. doi: 10.1111/1469-7610.00764
Twyman, K. A., Saylor, C. F., Saia, D., Macias, M. M., Taylor, L. A., and Spratt, E. (2010). Bullying and ostracism experiences in children with special health care needs. J. Dev. Behav. Pediatr. 31, 1–8. doi: 10.1097/DBP.0b013e3181c828c8
van Harmelen, A.-L., Gibson, J. L., St Clair, M. C., Owens, M., Brodbeck, J., Dunn, V., et al. (2016). Friendships and family support reduce subsequent depressive symptoms in At-Risk Adolescents. PLoS One 11:e0153715. doi: 10.1371/journal.pone.0153715
Van Roy, B., Veenstra, M., and Clench-Aas, J. (2008). Construct validity of the five-factor Strengths and Difficulties Questionnaire (SDQ) in pre-, early, and late adolescence. J. Child Psychol. Psychiatry 49, 1304–1312. doi: 10.1111/j.1469-7610.2008.01942.x
White, S. W., Oswald, D., Ollendick, T., and Scahill, L. (2009). Anxiety in children and adolescents with autism spectrum disorders. Clin. Psychol. Rev. 29, 216–229.
Willcutt, E. G., Doyle, A. E., Nigg, J. T., Faraone, S. V., and Pennington, B. F. (2005). Validity of the executive function theory of attention-deficit/hyperactivity disorder: a meta-analytic review. Biol. Psychiatry 57, 1336–1346. doi: 10.1016/j.biopsych.2005.02.006
Woerner, W., Becker, A., and Rothenberger, A. (2004). Normative data and scale properties of the German parent SDQ. Eur. Child Adolesc. Psychiatry 13, (Suppl. 2), II3–II10.
Keywords: Strength and Difficulties Questionnaire, mental health, learning difficulties, screening, transdiagnostic
Citation: Bryant A, Guy J, The CALM Team and Holmes J (2020) The Strengths and Difficulties Questionnaire Predicts Concurrent Mental Health Difficulties in a Transdiagnostic Sample of Struggling Learners. Front. Psychol. 11:587821. doi: 10.3389/fpsyg.2020.587821
Received: 27 July 2020; Accepted: 20 October 2020;
Published: 12 November 2020.
Edited by:
Ann Dowker, University of Oxford, United KingdomReviewed by:
Timo Lüke, Technical University Dortmund, GermanySabine Heim, Rutgers, The State University of New Jersey, United States
Copyright © 2020 Bryant, Guy and Holmes. This is an open-access article distributed under the terms of the Creative Commons Attribution License (CC BY). The use, distribution or reproduction in other forums is permitted, provided the original author(s) and the copyright owner(s) are credited and that the original publication in this journal is cited, in accordance with accepted academic practice. No use, distribution or reproduction is permitted which does not comply with these terms.
*Correspondence: Joni Holmes, joni.holmes@mrc-cbu.cam.ac.uk
†These authors share first authorship