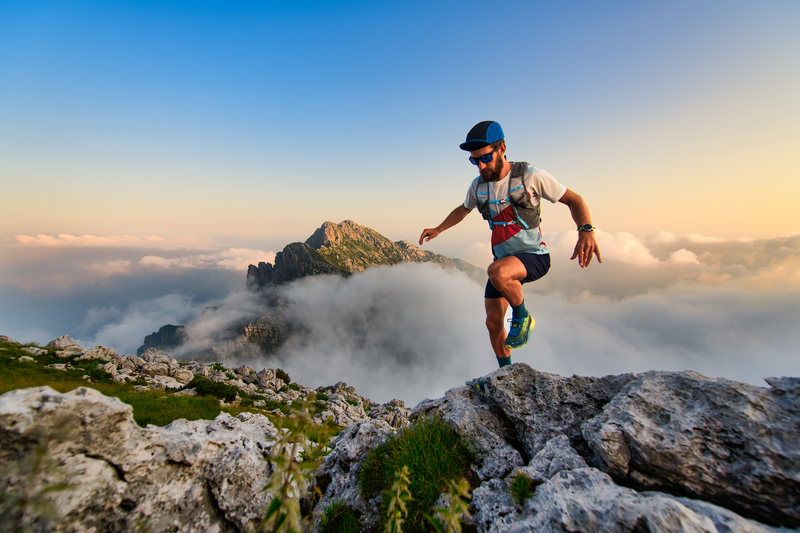
95% of researchers rate our articles as excellent or good
Learn more about the work of our research integrity team to safeguard the quality of each article we publish.
Find out more
ORIGINAL RESEARCH article
Front. Psychol. , 30 November 2020
Sec. Personality and Social Psychology
Volume 11 - 2020 | https://doi.org/10.3389/fpsyg.2020.577743
This article is part of the Research Topic Coronavirus Disease (COVID-19): Psychological Reactions to the Pandemic View all 66 articles
The COVID-19 pandemic poses a major challenge to policy makers on how to encourage compliance to social distancing and personal protection rules. This paper compares the effectiveness of two policies that aim to increase the frequency of responsible health behavior using smartphone-tracking applications. The first involves enhanced alert capabilities, which remove social externalities and protect the users from others’ reckless behavior. The second adds a rule enforcement mechanism that reduces the users’ benefit from reckless behavior. Both strategies should be effective if agents are expected-value maximizers, risk averse, and behave in accordance with cumulative prospect theory (Tversky and Kahneman, 1992) or in accordance with the Cognitive Hierarchy model (Camerer et al., 2004). A multi-player trust-game experiment was designed to compare the effectiveness of the two policies. The results reveal a substantial advantage to the enforcement application, even one with occasional misses. The enhanced-alert strategy was completely ineffective. The findings align with the small samples hypothesis, suggesting that decision makers tend to select the options that lead to the best payoff in a small sample of similar past experiences. In the current context, the tendency to rely on a small sample appears to be more consequential than other deviations from rational choice.
China’s success in fighting the spread of COVID-19 is attributed, at least in part, to an aggressive use of smartphone tracking applications (apps). These apps allowed authorities to identify and isolate those who might be spreading the virus (Huang et al., 2020), and punish those who violated social distancing and personal protection rules. For example, the apps issued color codes—green, yellow, or red—that indicated whether the holder poses an infection–transmission risk. A green light granted people an unrestricted pass (e.g., to the subway, work office, and other public places) and was essential for daily life. Yellow and especially red codes were extremely confining; both indicated that their holder should be quarantined and could not travel from one place to another. Identifying a person traveling with a red code was a sufficient reason to call the police. Thus, to enforce public health regulations, authorities may have severely penalized yellow or red code holders who broke quarantine.
When COVID-19 spread to western countries, their policy makers tried to emulate the success of China’s tracking apps. Yet, possibly due to privacy and civil rights concerns, authorities in many western democracies held back the development of aggressive and intrusive enforcement features. Instead, most tracking apps were designed to only alert their users, under the assumption that a reliable alert would suffice to discourage reckless behaviors. However, it is unclear whether virus-tracking apps, which only alert users and forgo regulation enforcement, are sufficiently effective in discouraging reckless behaviors.
The current research compares the effectiveness of two strategies that could guide the design of less aggressive, but potentially effective, tracking apps. One provides enhanced alerts and the other gently enforces the rules. Our comparative analysis rests on four observations. The first is that in their day-to-day life during of a pandemic, people regularly and frequently make small decisions between behaving responsibly and behaving recklessly (e.g., adhering to physical distancing guidelines or not). The second observation is that the probability that each particular decision will result in an infection is small. The third observation is that responsible behavior further decreases the chances of getting infected but often entails a small cost relative to reckless behavior, as it is more cumbersome and less convenient. The last observation is that the individual’s infection risk in a pandemic depends not only on one’s own behavior, but also on the behavior of others. Life during a pandemic presents risk even to those who maintain social distancing and other health protection guidelines. In that respect, health related behavior during pandemics is similar to driving; sharing the road with other drivers presents risk even to cautious drivers. To combat the virus, it is therefore essential to understand not only the individuals’ risk-taking behaviors but also the social dynamics that may arise in such situations. For example, it is possible that a minority of people who engage in reckless behaviors (behaviors that potentially increase the risk of infection) would make other people’s effort to behave responsibly futile, and in so doing drive otherwise responsible people to behave recklessly (Erev et al., 2020b)1.
Following these observations, we chose to abstract the decision environment of people in a pandemic in the context of a multi-person repeated game in which the (rare) risk imposed on each agent depends on the agent’s own decisions and the decisions of others. Specifically, we first analyzed the 4-person “Reckless or Responsible” game described in the upper panel of Table 1. This game models an environment in which reckless behavior is beneficial most of the time, but if none of the agents are reckless, behaving responsibly is the best choice on average2.
Table 1. Variations of the reckless or responsible game, predictions, and the observed responsible-rate.
The basic game has two Nash equilibria (choice profiles in which no agent wants to change choice unilaterally): An efficient equilibrium in which all agents choose “Responsible” and earn 0 with certainty, and an inefficient equilibrium whereby all agents choose “Reckless” and suffer an expected loss of 0.22. While agents should prefer the efficient equilibrium, at least two factors could impair coordination and drive them to the inefficient reckless equilibrium. The first is fear (or expectation) of reckless behavior on other agents’ side. Agents who worry that others will choose Reckless are expected to choose Reckless as well. Fear of this type is predicted, for example, by the popular “levels of reasoning” models of behavior in games (Nagel, 1995; Stahl and Wilson, 1995; Costa-Gomes et al., 2001; Camerer et al., 2004). Under such models, agents have some “level of reasoning” and play best-response to lower levels. Specifically, some agents (who are “level-0”) choose randomly and other agents (e.g., “level-1”) choose the best response to those playing level-0. Here, best-response implies acting Recklessly. As a result, higher-level agents (who chose best response to agents that are one level below them) will also choose Reckless. Furthermore, such beliefs were shown to lead to inefficient equilibria in variants of the “weakest link” game (Harrison and Hirshleifer, 1989; Van Huyck et al., 1990; Knez and Camerer, 1994), where the payoff of the individuals is affected by the lowest contributor (but see Riedl et al., 2016, for an extensive review of how to overcome such inefficiency).
A second relevant factor is that decision makers tend to select the options that lead to the best payoff in a small sample of similar past experiences (Nevo and Erev, 2012; Plonsky et al., 2015; Roth et al., 2016)3. In the basic game, this tendency implies a high rate of Reckless behavior because small samples are not likely to include the rare loss. For example, the probability that a 2% event will be included in a random sample of five events is only 0.096.
If agents act in line with the “level of reasoning” or “small samples” hypotheses, then most of the agents in the basic “Reckless or Responsible” game will choose to act recklessly. In search of a strategy to encourage responsible behavior we examine two variations of the basic game. The first involves elimination of the negative social externalities that lead agents to expect a higher utility from reckless behavior. This solution implies the design of an alert app that protects the agent from the reckless behavior of other agents. This would include, for example, sending alerts when approaching people who tend to exhibit reckless behavior. The second panel in Table 1 presents a variant of the basic game with a “perfectly protecting” Alert app4. Under this solution, the reckless behavior of others does not affect those who choose Responsible because they adhered to the alert and avoided the risk of infection. Therefore, choosing Responsible maximizes the expected value. Under the “levels of reasoning” hypothesis, the Alert app ensures that level-1 and more sophisticated agents will behave responsibly. In addition, Responsible choice minimizes risk and should be selected if losses loom larger than gains (Kahneman and Tversky, 1979) and if the agents are risk-averse (Holt and Laury, 2002) or ambiguity-averse (Fox and Tversky, 1995). Yet, under the reliance on small samples hypothesis, people choose to behave recklessly because it is better most of the time for them (regardless of the choices others make). Thus, reliance on small samples hypothesis predicts that the Alert app would have very little influence on behavior.
The second solution involves gentle rule enforcement (Erev et al., 2010; Schurr et al., 2014), i.e., a high probability that a reckless behavior will be gently penalized (without eliminating the social externalities). One way to implement gentle enforcement in a pandemic is to use tracking applications that continuously monitor a person’s behavior, and recommend the avoidance of detected reckless activities. For example, if the agent approaches a crowded place, the app will start to make an annoying sound every few seconds (similar to the seat belt beeping, see related idea in Okeke et al., 2018). The third panel in Table 1 presents a variant of the basic game that demonstrates this solution with a gentle but certain punishment (loss of 1.2 points) for each Reckless choice. Under such a regime, reckless behavior is never the best choice, and agents are expected to choose Responsible action even if they rely on small samples.
The central columns in Table 1 present the predicted “Responsible” choice rate in the current games under the “levels of reasoning” model (Cognitive Hierarchy; Camerer et al., 2004) and two abstractions of the reliance on small samples hypothesis (see Supplementary Appendix 1). The predictions of the Cognitive Hierarchy model were derived with the parameter proposed by Camerer et al. (2004). According to the basic naïve sampler model, agents would choose Responsible in the first trial, and then select the option that led to the best outcome in a random sample of five previous experiences (Erev and Roth, 2014). SAW (sampling and weighting) is a generalization of the naïve sampler model that adds noise and sensitivity to the average payoffs. The current predictions of SAW were derived with the parameters estimated in Erev et al. (2020a). Table 1 shows that the Cognitive Hierarchy model predicts that the Alert app will be as effective as the Always Enforce app, but that reliance on small samples models predicts that only the Gently Enforce app will be effective. The experiment described below was designed to test these predictions.
One hundred fifty-eight MTurk workers from the USA and Canada participated in the experiment in exchange for monetary compensation. Each session included one of the three conditions: Basic (n = 48, 12 groups, 30 males; Mage = 41), Alert (n = 52, 13 groups, 34 males5; Mage = 34), or Enforcement (n = 52, 13 groups, 31 males, Mage = 37). Each participant could participate in only one of the sessions. The monetary payoff included a show-up fee of $1 and an additional guaranteed $2 if the participant made more than 66% of the choices (i.e., more than 40 out of the 60 choices) on time6 as well as a chance to earn $1 bonus, based on the number of points accumulated during the experiment7 (mean final pay = $3.35, see Supplementary Appendix 2 for the precise instructions).
The experiment was run in groups of four participants. Participants could proceed to the next round only after all four players made their choices. To ensure that the experiment ran smoothly, we told participants that they had 12 seconds (20 seconds the first three trials) to make their choice in each round, after which the program would automatically submit a choice for them, and they would receive a penalty of 2 points. Unbeknownst to participants, when the program auto-submitted a choice on their behalf, it made the same choice that the participant made in the previous trial (in the first trial the program auto-submitted the Responsible choice)8.
The experiment, programmed with OTree (Chen et al., 2016), employed a variant of the clicking paradigm (Barron and Erev, 2003). In each of the 60 trials, the participants deliberated between two keys, “A” and “B.” Unbeknownst to the participants, “A” always represented the Responsible choice, while “B” represented the Reckless choice. Participants saw a complete description of the incentives structure and after each trial received feedback regarding their obtained and forgone payoff (see Figure 1).
Figure 1. Screens presented to participants, in the “Reckless or Responsible game, “Alert” app condition. The upper image (“Please make your choice”) presents the screen at the beginning of each trial. The lower image (“Results”) presents the screen at the end of each trial.
The right-hand column in Table 1 presents the mean choice rate of the responsible option (Responsible-rate) in the first three conditions. The rates are 9% (SD = 7.7%), 9% (SD = 9.8%), and 85% (SD = 10.3%) in the basic, alert, and always enforce conditions, respectively. This suggests that the alert app was ineffective, while the enforcement app was highly effective in increasing the Responsible-rate. The difference between the Responsible choice rate in the basic and the alert conditions is insignificant, Welch t(22.5) = 0.21. The difference between the Responsible rates in the basic and the enforcement condition is significant, t(22.1) = −20.78, 95% CI [68.0, 83.0], and so is the difference in Responsible rates between the enforcement and alert conditions, t(24) = 19.3, 95% CI [68.0, 84.3]. Responsible rates are not driven by outlier groups but represent a general pattern. The responsible rates are lower than 30% in all basic and alert groups and higher than 66% in all enforcement groups. Figure 2 presents the effect of the experience on each participant in the first 10 groups, and over all groups. The recurring pattern is of relatively flat curves, with a tendency to converge toward an equilibrium.
Figure 2. Individual and Group level “Responsible choice rates in the “Basic” (up), “Alert” (second), “Always Enforce” (third), and “Mostly Enforce” (down) conditions. Only the first 10 groups in each condition, and the mean over all groups in that condition, are shown. Each line represents a Responsible choice rate in five blocks (of 12 trials) by a participant in the respective group and condition. The bold line shows the mean Responsible choice rate of the group. The rightmost plot (of each condition) presents the overall mean Responsible rate of the respective condition.
The similar Responsible rates in the basic and the alert conditions suggest that in the current setting participants neglected the social externalities (i.e., the impact that their behavior had on others; Coase, 1960) associated with their actions. These results are consistent with previous research (Falk and Szech, 2013; Bartling et al., 2020), but more extreme (complete ignorance to the impact on others). Yet, analysis of the Responsible-rate in the very first trial reveals that the initial (pre-experience) tendency is inconsistent with complete neglect of social externalities. The initial Responsible choice rates are 28% (SD = 20.0%) and 15% (SD = 27.4%) in the Basic and Alert conditions, respectively. While the difference between these conditions is not statistically significant, t(22.67) = 1.67, this may just be due to lack of power. Hence, initially, participants were more likely to make responsible choices when their actions involved social externalities (Basic) than when they did not (Alert). Still, the effect appears to be small. One possible ad hoc explanation is shared guilt (Inderst et al., 2019), according to which people assume that even if they choose to act responsibly, others would choose to behave recklessly; therefore, the individuals’ choice to act recklessly and the guilt associated with it are attributed to others. This explanation is consistent with the fact that the effect of social externalities dissipates over time, when participants see that being responsible is pointless.
Notice that the instructions in the Alert condition imply an individual choice task. Thus, the low initial Responsible rate (only 15%) questions the generality of the tendency to overvalue rare events decisions from description; the results reveal undervaluing of rare events from description and from experience. This pattern supports the assertion that the tendency to overvalue rare events in decisions from description is not a general phenomenon; it appears to be sensitive to the framing of the choice task (see Harbaugh et al., 2010; Marchiori et al., 2015).
Under the reliance on small samples hypothesis, the effectiveness of the enforcement application in Study 1a is triggered by the fact that it ensures that the payoff from responsible behavior is higher than the payoff from reckless behavior in most small samples. Study 1b was designed to compare this explanation to two alternative explanations to the effectiveness of the enforcement in Study 1a. The first is that the effect is triggered by the large decrease in the expected return from reckless behavior implied by the enforcement app. In Study 1a, enforcement decreased the expected return from reckless behavior by 1.2 points and implied a decrease of 120% from the maximal payoff. The second alternative explanation is that the effect of the enforcement in Study 1 results from the dominance of the Responsible choice; it ensured that Responsible always led to better payoff than Reckless.
In order to compare the three explanations, we designed a new condition, simulating a tracking app that does not decrease the expected return of the Reckless choice and does not make Responsible the dominant choice. Specifically, this “Mostly Enforce” app leads to a loss of 1.2 points 95% of the time and to a gain of 24 points 5% of the time. Thus, it increases the expected payoff from Reckless behavior (the expected change is −1.2(0.95) + 24∗(0.05) = + 0.06), and leads to better outcomes than Responsible 5% of the time. Yet, the reliance on small samples hypothesis predicts that it will enhance responsible choices relative to the basic setting. The lower panel in Table 1 presents the implied payoff distribution, and the predictions of the two quantifications of the reliance on small samples hypothesis is considered here.
The Mostly Effective app abstracts natural settings in which the effort to enforce a specific behavior increases the expected benefit from selecting it. For example, consider a service provider (e.g., a plumber or a hairdresser) who is recklessly attempting to serve as many clients as possible. In the rare cases that this attempt goes unpunished, the service provider gets increased utility since competition is scarce.
Forty-eight9 MTurk workers participated in the Mostly Enforcing app game (n = 48, 12 groups, 29 males, Mage = 38) in exchange for monetary compensation. This post hoc study used the same procedure as the main study, but with a different payoff structure for reckless behavior (see lower panel of Table 1).
The mean Responsible rate was 59.8% (SD = 11.8%). This rate is significantly different from the basic [t(19.0) = 12.37, 95% CI [41.8, 58.9]], alert [t(21.6) = 11.7, 95% CI [42.0, 60.1]], and always enforcing [t(21.9) = 5.65, 95% CI [15.9, 34.3]] conditions. Thus, although the mostly enforce app was inferior to the always enforce app, it was still effective in increasing the Responsible choice rate compared to the basic app, and much more effective than the alert app. The latter result is rather illuminating in light of the expected value of Reckless choice (−0.22 in the alert app vs. −0.16 in the mostly enforce app conditions). In other words, on average, reckless behavior is less harmful in the mostly enforce app, but it is chosen more than twice more often in the alert app (40 vs. 92%). Furthermore, if the participants believe that at least one other participant will choose Reckless, this is the EV maximizing alternative (which is not the case in the Alert app condition).
Our analysis distinguishes between two contributors to reckless behaviors that can spread infection during a pandemic. The first involves the belief that the effort to behave responsibly is pointless; it cannot reduce the probability of infection because other members of the decision makers’ social network are likely to behave recklessly. Beliefs of this type are predicted, for example, based on the hierarchical levels of reasoning model of social behavior. The second contributor involves the tendency to undervalue rare events. This tendency is predicted under the reliance on small samples hypothesis. Understanding the relative importance of the two contributors can help predict the impact of different policies designed to facilitate responsible behavior. Assuming that the main contributor is the belief that other members will behave recklessly, responsible behavior can be enhanced by effective alert systems. However, if the main contributor is reliance on small samples, alert systems are not likely to be effective, and enforcement is necessary.
The current experiments compare the relative importance of the two contributors in an abstract 4-person game. The results support the prediction of the reliance on small samples hypothesis. Simulated alert applications, expected to facilitate responsible behavior under the hierarchical levels of reasoning model, had no effect. In contrast, simulated enforcement systems were found to be highly effective. In addition, our results demonstrate that enforcement can be effective even if it does not use harsh punishments and does not reduce the expected return from reckless choices. When responsible behavior implies an efficient Nash equilibrium (the environment examined here), it is enough to ensure that the enforcement increases the probability that responsible behavior leads to the best possible payoffs toward 1 (to 0.95 in the current study, see similar observation in Erev et al., 2019).
Our results should be viewed in light of the fact that the experimental studies focused on a simplified abstract setting that differs from natural pandemics-related dilemmas in many ways. For example, to avoid framing and impression management effects, our participants did not know that we aimed to study behavior in a pandemic. It is possible that some people are more (or less) prosocial in making these less abstract decision choices (Campos-Mercade et al., 2020). Furthermore, in our setting, people were fully informed about the potential consequences of their actions and their probabilities. In real life, this is unlikely, and misinformation may also be a highly relevant factor (Bursztyn et al., 2020). Also, we chose to focus on a static setting in which the outcomes and their corresponding probabilities do not change over time or as a function of the participants’ decisions, or the policies that are set forth. This is clearly a simplification of the highly dynamic nature of a pandemic. Finally, the current study compares potential policy solutions that implicitly assume universal and mandatory adoption of the suggested apps. For example, in the enforcement conditions of our experiments, one could not simply “uninstall the application” and avoid the gentle punishments associated with reckless behaviors. In most western democracies, mandatory tracking is probably unlikely. In a follow-up study (Plonsky et al., 2020), we investigated the potential of a voluntary gentle enforcement app and showed that with smart design, it can get significant traction. Despite these limitations, we believe our results can be of significant practical value as they highlight some of the basic choice tendencies people have in decision-making settings that have the same general structure we study (games with rare negative events and social externalities), like pandemics.
The raw data supporting the conclusions of this article will be made available by the authors, without undue reservation.
The studies involving human participants were reviewed and approved by the University of Haifa Faculty of Social Welfare Ethical Review board. The participants provided their written informed consent to participate in this study. The patients/participants provided their written informed consent to participate in this study.
YR, OP, and IE conceptualized the ideas. YR prepared, ran and analyzed the results of the experiments. IE prepared and ran the simulation models. All authors wrote and agreed upon the final version of the manuscript.
This research was supported by the Israel Science Foundation (grant no. 535/17).
The authors declare that the research was conducted in the absence of any commercial or financial relationships that could be construed as a potential conflict of interest.
The Supplementary Material for this article can be found online at: https://www.frontiersin.org/articles/10.3389/fpsyg.2020.577743/full#supplementary-material
Barron, G., and Erev, I. (2003). Small feedback-based decisions and their limited correspondence to description-based decisions. J. Behav. Decis. Mak. 16, 215–233. doi: 10.1002/bdm.443
Bartling, B., Fehr, E., and Özdemir, Y. (2020). Does Market Interaction Erode Moral Values? Available at: SSRN: https://ssrn.com/abstract=3683775 (accessed at August 25, 2020).
Bursztyn, L., Rao, A., Roth, C., and Yanagizawa-Drott, D. (2020). Misinformation During a Pandemic, Working Paper, (2020–2044), Chicago: University of Chicago.
Camerer, C. F., Ho, T. H., and Chong, J. K. (2004). A cognitive hierarchy model of games. Q. J. Econ. 119, 861–898. doi: 10.1162/0033553041502225
Campos-Mercade, P., Armando, M., Schneider, F., and Wengström, E. (2020). Prosociality Predicts Health Behaviors During the COVID-19 Pandemic, Working Paper, No. 346, Zurich: University of Zurich. doi: 10.5167/uzh-187672.
Chen, D. L., Schonger, M., and Wickens, C. (2016). oTree—An open-source platform for laboratory, online, and field experiments. J. Behav. Exp. Finance 9, 88–97. doi: 10.1016/j.jbef.2015.12.001
Coase, R. H. (1960). “The problem of social cost,” in Classic Papers in Natural Resource Economics, ed. A. Gopalakrishnan, (London: Palgrave Macmillan), 87–137. doi: 10.1057/9780230523210_6
Costa-Gomes, M., Crawford, V. P., and Broseta, B. (2001). Cognition and behavior in normal-form games: an experimental study. Econometrica 69, 1193–1235. doi: 10.1111/1468-0262.00239
Erev, I., and Roth, A. E. (2014). Maximization, learning, and economic behavior. Proc. Natl. Acad. Sci. U.S.A. 111(Suppl. 3), 10818–10825. doi: 10.1073/pnas.1402846111
Erev, I., Ert, E., Plonsky, O., and Roth, Y. (2020a). Six Contradicting Deviations from Rational Choice, and the Impact of Experience, Working Paper.
Erev, I., Ert, E., Plonsky, O., Cohen, D., and Cohen, O. (2017). From anomalies to forecasts: toward a descriptive model of decisions under risk, under ambiguity, and from experience. Psychol. Rev. 124, 369–409. doi: 10.1037/rev0000062
Erev, I., Gilboa Freedman, G., and Roth, Y. (2019). The impact of rewarding medium effort and the role of sample size. J. Behav. Decis. Mak. 32, 507–520. doi: 10.1002/bdm.2125
Erev, I., Ingram, P., Raz, O., and Shany, D. (2010). Continuous punishment and the potential of gentle rule enforcement. Behav. Processes 84, 366–371. doi: 10.1016/j.beproc.2010.01.008
Erev, I., Plonsky, O., and Roth, Y. (2020b). Complacency, panic, and the value of gentle rule enforcement in addressing pandemics. Nat. Hum. Behav. 4, 1095–1097. doi: 10.1038/s41562-020-00939-z
Fox, C. R., and Tversky, A. (1995). Ambiguity aversion and comparative ignorance. Q. J. Econ. 110, 585–603. doi: 10.2307/2946693
Harbaugh, W. T., Krause, K., and Vesterlund, L. (2010). The fourfold pattern of risk attitudes in choice and pricing tasks. Econ. J. 120, 595–611. doi: 10.1111/j.1468-0297.2009.02312.x
Harrison, G. W., and Hirshleifer, J. (1989). An experimental evaluation of weakest link/best shot models of public goods. J. Polit. Econ. 97, 201–225. doi: 10.1086/261598
Holt, C. A., and Laury, S. K. (2002). Risk aversion and incentive effects. Am. Econ. Rev. 92, 1644–1655. doi: 10.1257/000282802762024700
Huang, Y., Sun, M., and Sui, Y. (2020). How Digital Contact Tracing Slowed Covid-19 in East Asia. Harvard Business Review. Available at https://hbr.org/2020/04/how-digital-contact-tracing-slowed-covid-19-in-east-asia (accessed at April 15, 2020).
Inderst, R., Khalmetski, K., and Ockenfels, A. (2019). Sharing guilt: How better access to information may backfire. Manag. Sci. 65, 3322–3336. doi: 10.1287/mnsc.2018.3101
Kahneman, D., and Tversky, A. (1979). Prospect theory: an analysis of decision under risk. Econometrica 47, 263–292. doi: 10.2307/1914185
Knez, M., and Camerer, C. (1994). Creating expectational assets in the laboratory: coordination in ‘weakest-link’games. Strateg. Manag. J. 15, 101–119. doi: 10.1002/smj.4250150908
Marchiori, D., Di Guida, S., and Erev, I. (2015). Noisy retrieval models of over-and undersensitivity to rare events. Decision 2:82. doi: 10.1037/dec0000023
Nagel, R. (1995). Unraveling in guessing games: an experimental study. Am. Econ. Rev. 85, 1313–1326.
Nevo, I., and Erev, I. (2012). On surprise, change, and the effect of recent outcomes. Front. Psychol. 3:24.
Okeke, F., Sobolev, M., Dell, N., and Estrin, D. (2018). “Good vibrations: can a digital nudge reduce digital overload?,” in Proceedings of the 20th International Conference on Human-Computer Interaction with Mobile Devices and Services, (Spain: Barcelona), 1–12.
Plonsky, O., Apel, R., Ert, E., Tennenholtz, M., Bourgin, D., Peterson, J. C., et al. (2019). Predicting human decisions with behavioral theories and machine learning. arXiv [preprint]. Available at: https://arxiv.org/abs/1904.06866 (accessed November 18, 2020).
Plonsky, O., Roth, Y., and Erev, I. (2020). Underweighting of Rare Events in Social Interactions and its Implications to the Design of Voluntary Health Applications. arXiv [preprint]. Available online at: https://psyarxiv.com/9q3db (accessed November 18, 2020).
Plonsky, O., Teodorescu, K., and Erev, I. (2015). Reliance on small samples, the wavy recency effect, and similarity-based learning. Psychol. Rev. 122, 621–647. doi: 10.1037/a0039413
Riedl, A., Rohde, I. M. T., and Strobel, M. (2016). Efficient coordination in weakest-link games. Rev. Econ. Stud. 83, 737–767. doi: 10.1093/restud/rdv040
Roth, Y., Wänke, M., and Erev, I. (2016). Click or skip: the role of experience in easy-click checking decisions. J. Consum. Res. 43, 583–597. doi: 10.1093/jcr/ucw053
Schurr, A., Rodensky, D., and Erev, I. (2014). The effect of unpleasant experiences on evaluation and behavior. J. Econ. Behav. Organ. 106, 1–9. doi: 10.1016/j.jebo.2014.05.012
Stahl, D. O., and Wilson, P. W. (1995). On players’ models of other players: theory and experimental evidence. Games Econ. Behav. 10, 218–254. doi: 10.1006/game.1995.1031
Tversky, A., and Kahneman, D. (1992). Advances in prospect theory: cumulative representation of uncertainty. J. Risk Uncertain. 5, 297–323. doi: 10.1007/bf00122574
Keywords: decisions from experience, rare-events, social networks, levels or reasoning, trust game
Citation: Roth Y, Plonsky O, Shalev E and Erev I (2020) On the Value of Alert Systems and Gentle Rule Enforcement in Addressing Pandemics. Front. Psychol. 11:577743. doi: 10.3389/fpsyg.2020.577743
Received: 24 July 2020; Accepted: 29 October 2020;
Published: 30 November 2020.
Edited by:
Eduard Brandstätter, Johannes Kepler University of Linz, AustriaReviewed by:
Ulrich Hoffrage, University of Lausanne, SwitzerlandCopyright © 2020 Roth, Plonsky, Shalev and Erev. This is an open-access article distributed under the terms of the Creative Commons Attribution License (CC BY). The use, distribution or reproduction in other forums is permitted, provided the original author(s) and the copyright owner(s) are credited and that the original publication in this journal is cited, in accordance with accepted academic practice. No use, distribution or reproduction is permitted which does not comply with these terms.
*Correspondence: Yefim Roth, cm90aGVmaW1AZ21haWwuY29t
Disclaimer: All claims expressed in this article are solely those of the authors and do not necessarily represent those of their affiliated organizations, or those of the publisher, the editors and the reviewers. Any product that may be evaluated in this article or claim that may be made by its manufacturer is not guaranteed or endorsed by the publisher.
Research integrity at Frontiers
Learn more about the work of our research integrity team to safeguard the quality of each article we publish.