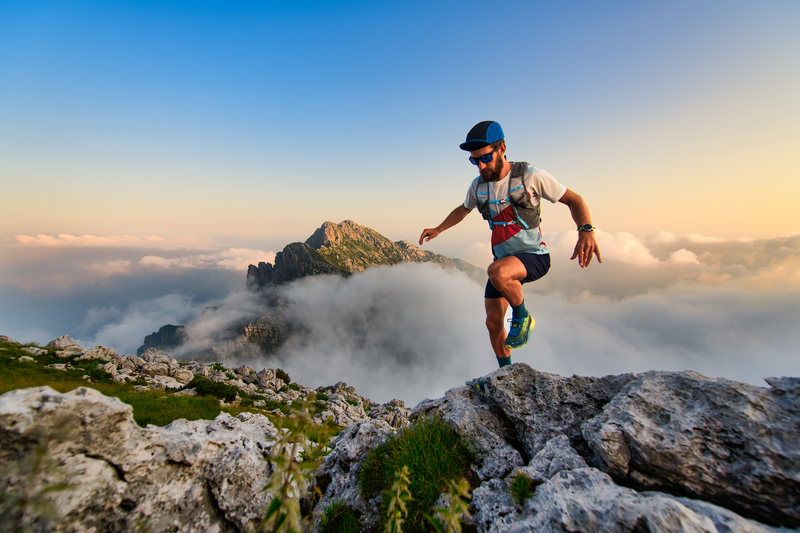
94% of researchers rate our articles as excellent or good
Learn more about the work of our research integrity team to safeguard the quality of each article we publish.
Find out more
CONCEPTUAL ANALYSIS article
Front. Psychol. , 24 September 2020
Sec. Eating Behavior
Volume 11 - 2020 | https://doi.org/10.3389/fpsyg.2020.554127
A correction has been applied to this article in:
Corrigendum: Core Neuropsychological Measures for Obesity and Diabetes Trials: Initial Report
Obesity and diabetes are known to be related to cognitive abilities. The Core Neuropsychological Measures for Obesity and Diabetes Trials Project aimed to identify the key cognitive and perceptual domains in which performance can influence treatment outcomes, including predicting, mediating, and moderating treatment outcome and to generate neuropsychological batteries comprised of well-validated, easy-to-administer tests that best measure these key domains. The ultimate goal is to facilitate inclusion of neuropsychological measures in clinical studies and trials so that we can gather more information on potential mediators of obesity and diabetes treatment outcomes. We will present the rationale for the project and three options for the neuropsychological batteries to satisfy varying time and other administration constraints. Future directions are discussed. Preprint version of the document is available at https://osf.io/preprints/nutrixiv/7jygx/.
Obesity and diabetes are associated with an array of cognitive and perceptual differences, some of which can be classified as mild impairments. Many of these differences are likely pre-existing and contribute to initial risk (e.g., Davis, 2009; Silveira et al., 2012), while others worsen or emerge as a consequence of the pathophysiological processes associated with obesity and diabetes. Determining the mechanisms and disentangling cause from consequence is a focus of ongoing research into the cognitive differences associated with obesity and diabetes. Typical treatment outcomes for obesity include change in body mass index (BMI), weight, and fat percentage; and change in HbA1C for (type 2) diabetes. For instance, lower cognitive ability in childhood is associated with weight gain in adulthood via diet and education levels (Chandola et al., 2006). Cognitive dysfunction, including executive dysfunction (e.g., impulsivity), poor memory, and decreased interoceptive awareness - among others - could hamper weight loss efforts (e.g., Koritzky et al., 2015; Schäfer et al., 2019).
This project aimed to identify the key cognitive and perceptual domains in which performance can influence treatment outcomes. Possible influences include predicting, mediating, and moderating treatment outcomes. The project serves as a basis for generating neuropsychological batteries composed of well-validated, easy-to-administer tests that best measure these key domains. The work builds upon several systematic reviews and meta analyses that link many different cognitive domains cross-sectionally to obesity (see Vainik et al., 2013; Wu et al., 2016; Emery and Levine, 2017; Yang et al., 2018). Typical effect sizes vary from r = 0.10 to 0.20 (Emery and Levine, 2017; Yang et al., 2018). However, not all cognitive tests from those analyses should be included, as cognitive tests also correlate with each other (Sharma et al., 2014; Eisenberg et al., 2019). Also, the meta-analyses may not include all tests that should be linked with obesity and diabetes. We therefore sought to have experts synthesize the previous findings outlined in the above-mentioned summaries, as well as highlight less-studied domains that should be included in future studies on obesity and diabetes.
Notably, there are more research domains than neuropsychology related to obesity and diabetes. One resource for additional measures and guidance is the ADOPT initiative (MacLean et al., 2018). We have also listed several approaches in Appendix B, such as personality tests and polygenic scores. Other data commonly used for clinical studies that include neuropsychological measures such as information on age, sex, education, and socioeconomic status, often used to develop normative data for performance evaluation, should be considered.
Here, the Core Neuropsychological Measures for Obesity and Diabetes Trials Project (CoreNP) expert group developed three options for the neuropsychological batteries to satisfy varying time and other administration constraints. The ultimate goal is to facilitate inclusion of cognitive measures in clinical studies and trials so that we can gather more information on potential mediators of obesity and diabetes treatment outcomes. To achieve this goal, the recommended batteries could be used in ongoing and future obesity and diabetes trials so that the relationship between cognition (broadly) and obesity and diabetes can be better understood, in turn identifying the most promising cognitive domains to include as predictors, mediators, and moderators in trials and as targets for intervention. Standardizing neuropsychological batteries would also facilitate data sharing and analyzing aggregate data across multiple studies.
For example, diet and physical activity are known to impact cognitive function and are both strongly associated with diabetes and obesity (Davidson et al., 2013; Freeman et al., 2014; Morris et al., 2015; Espeland et al., 2018). While we did not consider interactions between diet/physical activity and cognition in test selection, such interactions likely play an important modulatory role in treatment outcome. However, while most obesity and diabetes trials include measures of diet and physical activity, few also measure cognition. The addition of cognitive measures should therefore facilitate new insights into whether cognitive changes contribute to the efficacy of interventions that focus on diet and physical activity. For instance, a recent longitudinal study suggests that decline in cognitive ability precedes later decline in physical activity (Cheval et al., 2020).
The specific charge of the CoreNP expert group was to recommend one or two stand-alone neuropsychological tasks that spanned major cognitive domains (Table 1) and met many or most of the highest-priority criteria, which are listed in Table 2 and Appendix A. The psychometric properties of a task, especially task test–retest reliability, were of particular importance (further detail on test–retest reliability in Appendices B,E). The Executive Committee (Appendix C) prioritized the criteria, which were intended to serve as guidance for groups with expertise in six major cognitive domains: (1) cognitive control/executive function, (2) reward/motivation, (3) attention/working memory, (4) learning/memory, (5) sensation/perception, and (6) decision-making. Because the state of knowledge varies considerably across domains, the relative weight or importance of each criterion or set of criteria varied across domain groups. The groups were asked to indicate (a) the criteria considered in making task recommendations and the relative weight of each criterion that led to the final recommendations, and (b) the evidence base used to justify the recommendations.
The CoreNP expert group recommends three options to incorporate neuropsychological assessments in ongoing and future adult obesity and diabetes trials: (1) a 10-min General Cognitive Index (GCI); (2) a Standard Battery that includes the GCI; or (3) an Extended Neuropsychological Test Battery. The GCI is recommended as a “must” include, while the Standard and Extended batteries are recommended as “may” include because they require additional empirical support for their utility in predicting, mediating, or moderating outcomes in adult obesity and diabetes trials.
We note that the Standard Battery does not include tasks recommended for the reward/motivation or attention/working memory domains. Tasks from these domains were not included as there were insufficient data to support the psychometric properties of the task (reward/motivation) and/or the task administration time would extend the Standard Battery beyond 30 min (both reward/motivation and attention/working memory). Tests for these domains are included in the Extended Battery and are highly recommended. Some measures like those from the NIH Toolbox1, TestMyBrain.org, NIH Examiner2, and WAIS-IV3 may include a fee for some uses, but fees may also allow access to additional measures that may be of interest for obesity and diabetes trials. However, where possible, we selected measures without fees.
The Standard Battery includes the GCI and tests that meet the highest priority criteria. The Standard Battery can be administered in approximately 30 min. The Extended Battery includes the Standard Battery measures as well as high priority measures identified using the high priority criteria for task selection that were deemed promising but were not included in the Standard Battery either because the data supporting their relationship to treatment outcomes were insufficient or because the tests required too much time for preparation and administration. The Extended Battery will provide a more comprehensive assessment but will require approximately 80 min for administration.
Within this report, we list the currently available measures that are recommended for the GCI, Standard, and Extended Batteries. We also list tasks that were not included because they require further optimization, testing, and validation (Appendix D). To facilitate direct comparisons between tasks during selection, the expert group members rated each proposed task or measurement in the Task Recommendation Matrix using a scale of 1–5 (1 = no evidence, 3 = some evidence, 5 = strong evidence). Table 2 lists the criteria used for task selection. Additional criteria are included in Appendix A.
The goal of the General Cognitive Index (GCI) is to provide a brief (∼10 min) measure of global cognitive function for use in adult obesity and diabetes trials. The GCI provides a neuropsychological assessment that is sensitive to individual differences in overall cognition when a more comprehensive assessment is not feasible. The GCI also provides standard measures that can be used to interpret performance on other measures in the Standard and Extended Batteries, relative to overall cognitive ability. The GCI uses tests that are not highly dependent on English language skills, making it more appropriate for use across languages and cultures than language-dependent alternatives.
The GCI uses two measures that capture individual differences in non-verbal reasoning and processing speed:
(1) Visual pattern reasoning (matrix reasoning format), 7 min.
(2) Digit symbol matching speed (digit symbol substitution format), 2 min.
Baseline performance on the matrix reasoning task can be used as a stable marker of cognitive ability that may predict outcome; while changes in performance on digit symbol matching may indicate that improved (or impaired) cognition may be contributing to the efficacy of an intervention. Along with GCI, we recommend measuring educational attainment, for instance by counting years of education or by using the International Standard Classification of Education Standard. These two measures are interchangeable (e.g., Rietveld et al., 2014, Supplementary Table S1). Education is closely related to general cognitive ability (Lee et al., 2018), and education may explain the effect of cognition on weight change (Chandola et al., 2006). Recommendations for additional data to collect can be found in the “Next Steps” section.
The matrix reasoning task assesses non-verbal or visual reasoning, general cognitive ability, and/or fluid intelligence (Lezak et al., 2012). When compared to other standard neuropsychological measures, matrix reasoning tests tend to load most highly on the so-called ‘g’ factor of general cognitive ability (Carroll, 1993). Measures of matrix reasoning are a reliable method of assessing general cognitive functioning and can be easily administered on digital devices such as computers, tablets, and smartphones. Poorer scores on matrix reasoning tests have been linked with poorer outcomes in obesity and diabetes (Dore et al., 2008; Feinkohl et al., 2014). As matrix reasoning scores tend to be relatively stable over time and less sensitive to state-related changes, matrix reasoning is often considered a premorbid IQ or “hold” test (Schoenberg et al., 2006; Tranel et al., 2008) and performance is less affected by changes in health or brain damage than other cognitive tests (Ryan et al., 2005). Matrix reasoning scores also provide a useful potential covariate for understanding specific cognitive mechanisms, where the researcher wants to control for variations due to general cognitive ability. The stability of matrix reasoning performance over time makes this measure a useful predictor (or moderator) of outcomes in studies. This test is not recommended as an outcome measure in obesity and diabetes intervention studies because performance is relatively insensitive to changes in health status and any given form of matrix reasoning can only be given once. Thus, changes in scores over time on the same test are difficult to interpret and not necessarily meaningful.
The digit symbol matching test can be administered using digital devices. This 2-min test uses a standard format digit symbol substitution procedure, but with a keyboard or touchscreen rather than written responses. The test measures processing speed and a range of cognitive functions including visual scanning, sustained attention, and short-term memory (Lezak et al., 2012). Scores on this measure are sensitive and reliable despite very brief administration times. Performance on this test is less related to education and general cognitive ability as measured by more stable trait-like measures (e.g., matrix reasoning). Instead, the digit symbol matching test is a very sensitive measure of many state variables, including changes in health and all or nearly all forms of brain damage such as dementia or vascular disease (Glosser et al., 1977; Cukierman et al., 2005; Rapp and Reischies, 2005; Rosano et al., 2008). Performance on various digit symbol matching tests has been consistently linked with obesity and diabetes (Brands et al., 2005; Cukierman et al., 2005; Cournot et al., 2006; Palta et al., 2014). Due to its repeatability and sensitivity to state variables, this test can be administered many times in high frequency measurement designs or administered at single time points, and it could serve as an outcome of interventions.
Individually or in combination, the two GCI tests provide a means of understanding trait and state-related neuropsychological functioning. Taken together, these measures can be thought of as analogous to body mass index (BMI), which uses height (stable) and weight (variable) to capture an important aspect of health that varies across individuals. Insofar as the matrix reasoning scores can reflect “premorbid” or stable aspects of cognitive performance, the digit symbol matching scores can be interpreted with respect to matrix reasoning scores to identify discrepancies (e.g., abnormally slow processing speed given high non-verbal reasoning capability) that could be indicators of poorer health (Schoenberg et al., 2003; Ball et al., 2007; Lezak et al., 2012). However, the degree to which matrix reasoning scores are themselves sensitive to brain health can differ across conditions (Ryan et al., 2005).
The GCI can be administered using most digital devices like laptops, desktops, tablets, and smartphones and have existing large datasets that can be used for normative comparisons. These tests are available open source and adapted from standard format matrix reasoning items and also include item-level statistics that can be used for interpreting test scores and building shorter test versions. Please email dGVzdG15YnJhaW5AZ21haWwuY29t for access to a free study page with digital test versions for research use.
TestMyBrain.org Matrix Reasoning Test (DEMO LINK)
https://www.testmybrain.org/tests/matrix_discontinuerule/index_numbered.html.
TestMyBrain.org Digit Symbol Matching (DEMO LINK)
http://www.testmybrain.org/tests/DigSymbCoding/DSC.html.
The primary outcome variable for this test is accuracy across 35 test trials, which reflects general cognitive or non-verbal reasoning ability. Reaction times can also be used as a secondary measure but are most useful for identifying invalid data, such as trials on which the participant was not paying close attention to their responses. For the test link provided, accuracy is automatically calculated.
The primary outcome variable for this test is throughput, or number of correctly matched symbols, within the administration time (90 s), which reflects processing speed. While the accuracy is not used as an outcome measure, it provides important validity information. For example, poor accuracy, defined as <75% correct or less than 50% correct depending on whether the target population is healthy or impaired, can indicate the participant was not paying close attention and therefore the number of correct responses cannot be interpreted. For the test link provided, number of correctly matched symbols (throughput) is automatically calculated.
The Standard Battery (see Table 3) is constructed to be short (∼30 min total, including the GCI) and easy to use while also enabling measurement of the domains likely to be the best predictors, mediators, and/or moderators of outcomes in adult obesity and diabetes trials. It includes the GCI and four other tasks that assess executive function and cognitive control, learning and memory, and decision-making. The Extended Battery includes tasks that cover the remaining cognitive domains.
The tasks selected for the Standard Battery measure two constructs of executive function and cognitive control that are relevant to obesity and/or diabetes: response inhibition and task switching. We used the factor structure of executive function developed by Miyake et al. (2000), which categorizes executive function into 3 sub-domains: inhibition, working memory, and switching. Each of those three domains have been consistently related to obesity in the past (Vainik et al., 2013; Emery and Levine, 2017; Yang et al., 2018). However, working memory is strongly related to general intelligence (Eisenberg et al., 2019) measured by the GCI, which is why we decided to focus on inhibition and switching here. The selected tasks have high test–retest reliability, predictive power, and are available in open source formats. Additionally, the tasks measuring response inhibition and higher-order planning are face valid.
The CoreNP expert group selected the go/no-go task as a measure of response inhibition. This task involves presentation of a single stimulus on each trial and the participant is instructed to respond if the stimulus falls into a particular class (the “go” stimulus) but not to respond if it falls into another class (the “no go” stimulus). For example, a participant might respond to “X” but not to “O.” When the no-go condition occurs relatively infrequently (e.g., less than 1/3 of trials), success on the task is thought to require inhibition of the prepotent “go” response. The task should include sufficiently more go than no-go trials, use rare no-go trials, and short trial durations (Wessel, 2018). In the literature, d’ is the most common outcome measure reported; however, the commission error rate (i.e., the number of responses on no-go trials) may be a more direct measure of response inhibition.
Task switching is a major component in cognitive flexibility, and relationships between switching and obesity have been established with several tasks, such as Trail-Making Test B and the Wisconsin Card Sort Task (Vainik et al., 2013; Emery and Levine, 2017; Yang et al., 2018). We selected the Dimensional Change Card Sort (DCCS) task (Zelazo, 2006). This task involves presentation of a stimulus that varies along two dimensions, color and shape, and the participant is instructed to respond to only one of the dimensions on each trial. The rules governing responses change occasionally, and the primary measure is the number of correct responses. We proposed the use of DCCS, as it is available through the NIH toolbox and has also been linked to obesity (e.g., Vainik et al., 2018).
While there is not strong evidence for an association between memory and obesity in otherwise healthy young individuals, such an association appears to emerge with age. A higher BMI in middle age is associated with an accelerated age-related decline in memory ability (Cournot et al., 2006) and later dementia (Whitmer et al., 2005). Being overweight has been associated with a reduced hippocampal volume in otherwise healthy participants aged 60–64 years (Cherbuin et al., 2015). This effect is even more apparent in older adults who are obese, and this relationship is present even when cardiovascular and blood glucose factors are taken into account (Raji et al., 2010). Obesity might therefore accentuate other factors that compromise memory performance such as normal aging or age-related neurodegenerative disease. Tasks that are highly sensitive to hippocampal function and that require episodic or relational memory or that involve arbitrary associations are likely to be the most sensitive. Learning and memory appear to be more useful as outcome measures, reflecting the sensitivity of the hippocampal system to metabolic factors (Cheke et al., 2016; Hargrave et al., 2016; Higgs and Spetter, 2018).
The Picture-Sequence Memory task from the NIH Toolbox was selected given its psychometric properties, availability, and short duration. In this task, participants study sequences of pictures of objects and actions, and then must recall the order in which the items appeared. Hayden et al. (2018) used this task to assess cognition after an intensive lifestyle weight loss intervention in participants aged 45–76 years with type II diabetes. The intervention had no effect on the Picture-Sequence Memory task across participants, but a subset of participants with the highest BMI had decreased performance after the intervention, suggesting that significant weight loss might not have beneficial effects on memory in older participants (Hayden et al., 2018). Additional research is needed to determine the suitability of the Picture-Sequence memory task. There is one study suggesting that a verbal learning task could be sensitive to a western diet manipulation (Stevenson et al., 2020), but this evidence is not conclusive and demonstrates a need for further research in this area.
The Kirby Delay Discounting task was selected because temporal (delay) discounting has been widely studied in relation to obesity and overweight status and other impulsivity-related clinical conditions (Vainik et al., 2013, 2018; Volkow and Baler, 2015; Barlow et al., 2016; Yang et al., 2018). The discount factor k, or the rate at which people discount future rewards, can measured accurately with very brief tasks (Koffarnus et al., 2015). The primary outcome of a delay discounting task is the discount rate, with larger values of k indicating increased impatience and preference for rewards that are available immediately. Cross-sectional evidence suggests that delay discounting is steeper in individuals with overweight or obesity compared to healthy-weight groups, with small-to-moderate effect sizes. Though delay discounting variables are considered potential predictors or moderators of behavioral or health outcomes; to our knowledge, there are only limited data on delay discounting as an outcome in adult obesity and diabetes trials (Stein et al., 2017).
The extended battery includes the GCI, Standard Battery, and additional tasks below. The tasks are also summarized in Table 4.
The tasks chosen measure constructs of reward-seeking and motivational processes that are relevant to obesity and diabetes including the willingness to exert effort to acquire rewards and risk-taking (a shared construct with the decision-making domain). The CoreNP expert group defined reward seeking as the experiences that accompany receiving rewarding outcomes like money, food, positive feedback, etc. or failing to receive rewarding outcomes. This definition also includes Pavlovian and operant learning about cues that predict rewarding outcomes from experiences of receiving or not receiving such outcomes, determining how much effort is required to pursue rewards, and making choices that balance the risks of winning versus losing rewarding outcomes.
The performance on the effort expenditure for reward (EFfRT) task was chosen because it is a valid measure of effort allocation toward reward, has high test–retest reliability, is open-source, and tolerable (Reddy et al., 2015). This task has been linked to adherence to weight loss interventions (Mata et al., 2017). The EFfRT asks participants to choose between an easy task (21 button presses with dominant index finger) for $1 and a hard task (100 button presses with non-dominant pinky finger) for varying amounts of money (>$1). The amount of money a participant can earn for the hard task varies across trials ($1.60–$4.00+), as does the likelihood that they will actually be awarded the money should they successfully complete the tasks. The likelihood typically ranges between 50 and 88%, though some versions of the task also use a 12% condition.
The “Game of Dice” task was chosen as a measure of risk-taking because it is associated with decision-making processes involved in long-term weight loss (Brockmeyer et al., 2017). This task is a valid measure of risk behavior (Brand et al., 2005), has high test–retest reliability (Starcke et al., 2017), and is tolerable to participants. Participants are told they should try to obtain as much money as possible by predicting the outcome of a die roll. They are shown a screen and asked to predict which of two choices they think will contain the number that will appear on the die. The choices are between one specific single number or a series of 2–4 numbers. Choices with fewer number options are “riskier.” For example, choosing a single number is associated with a 1/6 win likelihood, with the outcome being ±$1,000. In contrast, choosing a string of 4 numbers is associated with a 4/6 win likelihood, but with an outcome of ±$100.
The tasks chosen are measures of sustained attention and working memory chosen are sensitive indicators of cognitive impairment, can be given in a relatively short amount of time, and can be flexible given the needs of the study. Both attention and working memory are involved in setting goals and decision making, which in the context of obesity and diabetes can include decisions on what to eat, when and how much to exercise, when and how much to sleep, and self-monitoring diet, physical activity and insulin. Digit Span is one of the most well used measures of attention and working memory. While Digit Span was once referred to as a test of attention, it has more recently been considered a measure of working memory or auditory working memory based on factor analytic studies (Holdnack et al., 2011). Digit Span has been associated with BMI (Elias et al., 2003; Di Blasio et al., 2010), in particular Digit Span forward has been negatively associated with BMI (Gunstad et al., 2007, 2010). Digit Span has shown differentiation between individuals with overweight or obesity and those who are a healthy weight (Elias et al., 2003; Coppin et al., 2014) and with binge eating disorder (Duchesne et al., 2010). Digit Span scores seem to improve with weight loss (Halyburton et al., 2007; Brinkworth et al., 2009). As in all research, there are a number of studies that show a lack of association between digit span and overweight and obesity (Strachan et al., 1997; Awad et al., 2004). However, it is important to consider additional factors, such as insulin sensitivity (Gonzales et al., 2010) and physical activity (Brown et al., 2012; Basso et al., 2015; Huang et al., 2018) which could be potential moderators among BMI, attention and working memory. The Dot Pattern Expectancy (DPX) and visual search tasks were chosen to measure the constructs of attention and working memory with relevance to obesity and/or diabetes. While there is a paucity of evidence supporting their role in obesity and/or diabetes, specifically, the other strengths of these tasks and the relationships to other important health behavior outcomes led the group to recommend these tasks. Both attention and working memory are involved in setting goals and decision-making, which in the context of obesity and diabetes can include decisions about what to eat, when and how much to exercise, when and how much to sleep, and the self-monitoring of diet, physical activity, and prescribed treatment. Further, the DPX task can also capture tendencies toward proactive or reactive control modes (Braver, 2012). Therefore, the task may also be useful to understand executive function.
The DPX task, which measures sustained attention and working memory, was selected by the NIMH Research Domains Criteria (RDoC) initiative expert group and the NIMH Cognitive Neuroscience Treatment Research to Improve Cognition in Schizophrenia (CNTRICS) group for assessment of working memory in psychotic disorders following analysis of its psychometric properties, feasibility, and validity data in schizophrenia and other psychotic disorders (see Barch et al., 2008; MacDonald, 2008; Lopez-Garcia et al., 2016). It was carried forward and further developed by the Cognitive Neuroscience Test Reliability and Clinical Applications for Schizophrenia (CNTRACs) Consortium. The task was coded for implementation using the E-Prime software package and is freely available on the CNTRACS website (see Table 4).
The visual search task measures selective attention while minimizing working memory and executive function requirements (Gold et al., 2007). We recommend a version of conjunction visual search implemented by Gold et al. (2007, 2018) that requires the shifting of covert attention between items to find the target. Participants are presented with a search array comprising a variable number of items (set size). The items are horizontal or vertical ellipses, which can be red or blue. Participants search for a target item defined by a conjunction of orientation and color (e.g., red + vertical), and report whether or not the target ellipse has a gap. The primary measure of visual search is reaction time (RT); however, the more important measure is the slope of the RT x set size function, which serves as an index of search efficiency. Accuracy is a secondary dependent measure. Search efficiency has not been studied as an outcome measure in obesity/diabetes.
In addition to the Picture-Sequence Memory task in the Standard Battery, the CoreNP expert group recommends the Spatial Reconstruction Test, which is relatively brief and has also been shown to be sensitive to hippocampal involvement (Watson et al., 2013). In this task, participants study an array of shapes on the screen in each trial. The shapes disappear, and after a short delay they appear at the top of the screen and the participant must drag each shape to the location in which it had appeared. In a recent study, performance on this task in overweight individuals was related to serum lutein (Cannavale et al., 2019). Because lutein is important for brain function and is reduced in individuals with obesity, these results suggest that this consequence of obesity may result in impaired hippocampal-dependent memory.
Sensation refers to the process of realizing internal and external environments through touch, taste, sight, sound, smell, and interoception. Perception refers to the ways in which these signals are interpreted. The CoreNP expert group evaluated the evidence for cross-sectional and predictive associations among BMI and eight domains of sensation and perception that included pain, taste, smell, body schema, interoception, and visuospatial perception. The mechanisms linking these domains to outcomes of obesity and diabetes trials were also considered, as were practical considerations such as cost and burden to the participant and investigators.
For the Extended Battery, we decided to measure interoceptive accuracy with the Schandry task (Schandry, 1981). Levels of interoceptive cardiac accuracy are low in type 2 diabetes (Grammes et al., 2018). This task is the most widely used measure of cardiac interoception due to its simplicity, assessment speed (6 min), and predictive validity for key variables of interest. It uses pulse-oximetry, a common and widely used medical tool. The Schandry task provides a measure of cardiac interoceptive accuracy by comparing how many heartbeats participants count to how many are measured by the pulse oximeter. This task was selected because performance is impaired in obese individuals, and a greater BMI is associated with impaired performance accuracy (Herbert et al., 2013; Herbert and Pollatos, 2014; Koch and Pollatos, 2014). Performance on the Schandry task is also related to gastric interoception (Van Dyck et al., 2016) and thus it offers a fast and efficient way to detect general visceral sensitivity.
In addition to delay discounting, the CoreNP expert group recommends the assessment of risk attitude with the Holt–Laury Risk Taking for Monetary Outcomes. While less-studied in obesity and diabetes, risk attitude (or risk preference), is conceptually and behaviorally related to temporal discounting. The term “risk” has many meanings that have been operationalized in different ways; we refer here to a formal economic notion of variability in the outcomes of monetary prospects. Like delay discounting, risk can be measured accurately with very brief tasks (Koffarnus et al., 2015). Risk variables are considered potential predictors or moderators of behavioral or health outcomes; to our knowledge, they have not been studied as outcomes.
The Holt–Laury task involves a series of decisions between 10 pairs of hypothetical lotteries, with more risky outcomes (e.g., 10% chance of winning $190, 90% chance of $5) vs. safer outcomes (e.g., 10% chance of winning $100, 90% chance of winning $80). The number of pairs for which the participant chooses the risky option (n/10 choices) gives a measure of risk attitude (with larger n indicating risk seeking and smaller n indicating risk averse preferences).
There were two overarching purposes of the initiative: (1) to identify the highest priority cognitive domains for obesity and diabetes research and clinical trials based on the current evidence and (2) to provide researchers with suggested measures within each domain, identifying specific measures that are valid and available in the public domain wherever possible. This publication represents the culmination of this first step for the NIH Core Neuropsychological Measures for Obesity and Diabetes Project. We will continue to evaluate specific measures, and additional recommendations or modifications will be updated in this paper as appropriate.
While the expert group has suggested strategies for implementing and disseminating these measures and batteries into studies, the workshop did not focus on implementation and dissemination. Challenges in using these measures and batteries were not considered or discussed in depth. For example, it is likely that burden to the researcher and participant in access and use of these measures could limit broad adoption of these batteries into adult obesity and diabetes studies. Future efforts should address these and other challenges to promote use of the best neuropsychological measures to advance obesity and diabetes research.
Additional data collection considerations and analysis tools are provided in Appendices B,E. Another exhaustive resource for important covariates is the Accumulating Data to Optimally Predict obesity Treatment (ADOPT) Core Measures Project. The project outlines factors from behavioral, biological, environmental, and psychosocial domains (MacLean et al., 2018).
To begin, we recommend communicating widely the importance of generating a neuropsychological profile of obesity and diabetes while simultaneously encouraging researchers engaged in upcoming and ongoing clinical trials to incorporate the General Cognitive Index (GCI) into their experimental designs. The two tests included in the GCI can be thought of as analogous to the height and weight measures researchers currently acquire to calculate the BMI. While matrix reasoning scores are expected to remain stable (like height), and provide an estimate of premorbid functioning, digit symbol matching scores are expected to be more sensitive to changes in brain health. The GCI can be easily acquired at no cost through TestMyBrain.org (email: dGVzdG15YnJhaW5AZ21haWwuY29t). We anticipate Phase I would last approximately 6–18 months.
We next recommend researchers engaged in upcoming and ongoing clinical trials on obesity and/or diabetes include the Standard or Extended Battery in their experimental designs. Finalized versions of the Standard Battery and Extended Battery might require a meeting of domain experts to agree on exact test parameters and recommended versions. It is important that there are inexpensive and easily accessible test options and at least one test option that can support a large-scale study requiring formal data management and software infrastructure. A small psychometric study, similar to MATRICS, could be helpful in identifying comparability between test versions and any weaknesses in particular test formats. It would also be helpful to identify, support, and/or develop infrastructure for aggregating data. We anticipate Phase II would last approximately 6–24 months.
We intend for the Standard and Extended Batteries to be evaluated and refined as needed. To this end, we recommend either a formal survey of grantees and investigators using the recommended batteries or the GCI, or that the batteries be incorporated into ongoing studies to rapidly evaluate the predictive validity and psychometrics of the recommended tests. Any potential issues with sociocultural biases and accessibility across groups should be evaluated and addressed. We also recommend support for trainees to write reviews or papers aggregating the results from the recommended tests across studies. Formal recommendations should be created for data sharing of all the neuropsychological battery data elements and common data models and for best practices around the return of research results. After evaluation, we recommend that tests showing no early evidence of utility be eliminated or replaced. We anticipate Phase III would last approximately 18–36 months.
We recommend the development of infrastructure to support the final batteries. Such infrastructure might rely partly or entirely on existing infrastructure, like NIH Toolbox. We also recommend the development of publicly available test norms, documentation, scoring procedures, and training protocols to support the use of the batteries as experimental tools in clinical trials.
When finalized, we recommend that the Core Neuropsychological Measures for Obesity and Diabetes Trials Project batteries use standardized text for grants, method sections in publications, Institutional Review Board proposals, online training, tutorials; and digital identifiers, where possible.
Our understanding of the best neuropsychological measures to predict responses in obesity and diabetes trials is still early, and the data are somewhat limited. Additional research is needed to validate the proposed measures and determine which are the most valuable predictors, mediators, and moderators of clinical outcome. Thus, as described in Phase III above, the proposed measures will undoubtedly be refined as they are incorporated into ongoing and new studies. Though the recommended batteries may be modified based on new results, they cannot be evaluated until they are incorporated into clinical obesity and diabetes trials. Based on existing results from neuropsychological studies of obesity and diabetes, we believe that the proposed cognitive tests are the best place to start.
There were a number of important research gaps identified. First, much of the existing research has focused on BMI and/or obesity with more limited research in insulin resistance and diabetes or in the contributions of diet, physical activity, sedentary behavior, diet, and/or caloric intake to cognitive functioning. Also, the task-BMI evidence was sometimes based on one-off studies that need replicating. While there is some evidence that performance on proposed tasks relates to treatment adherence or long term outcomes, we know little about whether variation in these constructs or performance on these tasks predict, e.g., BMI, insulin sensitivity, and/or glycemic variability across the lifespan, or the development of obesity and diabetes. Though the initial work on test–retest reliability is promising, some tasks in the extended battery had less than optimal reliability (r < 0.7), so future psychometric work may be needed to optimize those tasks or replace them with more stable tasks. Alternatively, the number of measurements per participant could be increased to improve the signal-to-noise ratio (Sliwinski et al., 2018). More work is also needed to ensure that tasks are well suited as predictors, mediators, and/or moderators of outcomes in observational and treatment trials. There is a particular need to test these measures as predictors of outcomes as there has been very limited, rigorous research in this area. In addition, change in cognitive performance could also be an outcome of obesity. For instance, bariatric surgery is known to improve performance in multiple cognitive domains (Thiara et al., 2017). Building an evidence base to identify which cognitive domains are predictors, moderators, and/or outcomes for obesity and diabetes trials is a clear need.
Some of the recommended tasks use monetary outcomes. While performance on these tasks has been shown to be related to obesity and BMI and to a lesser extent, diabetes, it is important to directly compare versions that use primary rewards (food, drink, etc.) versus secondary rewards (i.e., money) to determine if there is greater predictive utility for one type of reward versus another.
At this time, food-specific tasks have not been sufficiently validated or standardized to be recommended. However, such tasks can be briefly and validly measured and have some evidence of relevance in obesity, diabetes, and other aspects of health-related behaviors. There is also some evidence that such domain-specific approaches might have larger effect sizes than tasks using monetary outcomes (Wu et al., 2016). Existing tasks that use food cues or require decision-making in the obesity and diabetes context have high ecological validity, but are not standardized and would require careful consideration of socio-cultural influences and tailoring to meet assessment objectives.
Like many areas of physical and mental health, the roots of behaviors and developmental trajectories associated with obesity and diabetes emerge early in the course of development. As such, it has become increasingly clear that early detection and identification are critical. To effectively conduct work on early detection, identification, and prevention, we need to facilitate the use of paradigms and measures that can either be used in young populations or facilitate the translation of such measures for use in children, including very young children. This will be a challenge, as the majority of the measures described above have been validated in adults but have rarely been used in younger populations. Therefore, creative modifications of the same paradigms will be needed for use in younger children, along with appropriate validation studies. In addition, we will need to consider whether there are alternative ways to obtain measures of the same constructs in very young children, such as parent report or observational measures.
Finally, obesity and diabetes are diseases that are overrepresented in certain racial and ethnic groups. Unfortunately, many neuropsychological measures have inadequate or unknown performance properties in these groups (Manly, 2008; Henrich et al., 2010). We have tried to select tasks that are known to perform well (or should) in culturally and linguistically diverse individuals; however, there are still known limitations, even with these tasks. Therefore, more work is necessary to develop, adapt, and test the performance of tasks that do an adequate job of assessing the neuropsychological function of the culturally and linguistically diverse individuals that will be participants in our clinical studies and the recipients of our therapies (Barrett, 2020). A statement should be included as to whether task administration is appropriate for a given racial/ethnic and/or immigrant group.
KD’A, CS, DS, UV, and LS led the writing group and contributed substantially to coordination and preparation, writing and editing, and revision of the manuscript. All authors contributed to the article and approved the submitted version.
UV has been funded by Estonian Research Council grant MOBTP94.
The authors declare that the research was conducted in the absence of any commercial or financial relationships that could be construed as a potential conflict of interest.
We would like to thank the executive committee members for assistance with organization and planning the activities that led to this report and the executive committee and workshop participants for contributing content expertise, writing, and edits to the report (see Supplementary Appendix C for listed names and affiliations). We would also like to thank Drs. Patrick Bissett, Desiree Byrd, Xavier Cagigas, Laura Holsen, and Kristin Javaras for their review and insightful feedback on the preprint (i.e., NutriXiv) version 1 of the report. We also thank Richard Gershon and Molly Wagster who gave excellent input on development of the NIH Toolbox, which was helpful in crafting some of the thinking and language that ended up in the report. We would further like to acknowledge the writing contributions for the content of each section came from members of the project executive committee and workshop participants. The content is solely the responsibility of the authors and does not represent the official views of the NIH or federal government.
The Supplementary Material for this article can be found online at: https://www.frontiersin.org/articles/10.3389/fpsyg.2020.554127/full#supplementary-material
Awad, N., Gagnon, M., and Messier, C. (2004). The relationship between impaired glucose tolerance, type 2 diabetes, and cognitive function. J. Clin. Exp. Neuropsychol. 26, 1044–1080. doi: 10.1080/13803390490514875
Ball, J. D., Hart, R. P., Stutts, M. L., Turf, E., and Barth, J. T. (2007). Comparative utility of Barona Formulae, Wtar demographic algorithms, and WRAT-3 reading for estimating premorbid ability in a diverse research sample. Clin. Neuropsychol. 21, 422–433. doi: 10.1080/13854040600582577
Barch, D. M., Berman, M. G., Engle, R., Jones, J. H., Jonides, J., and MacDonald, A. III, et al. (2008). CNTRICS final task selection: working memory. Schizophrenia Bull. 35, 136–152. doi: 10.1093/schbul/sbn153
Barlow, P., Reeves, A., McKee, M., Galea, G., and Stuckler, D. (2016). Unhealthy diets, obesity and time discounting: a systematic literature review and network analysis. Obes. Rev. 17, 810–819. doi: 10.1111/obr.12431
Barrett, H. C. (2020). Towards a cognitive science of the human: cross-cultural approaches and their urgency. Trends Cogn. Sci. 24, 620–638. doi: 10.1016/j.tics.2020.05.007
Basso, J. C., Shang, A., Elman, M., Karmouta, R., and Suzuki, W. A. (2015). Acute exercise improves prefrontal cortex but not hippocampal function in healthy adults. J. Int. Neuropsychol. Soc. 21, 791–801. doi: 10.1017/s135561771500106x
Brand, M., Fujiwara, E., Borsutzky, S., Kalbe, E., Kessler, J., and Markowitsch, H. J. (2005). Decision-making deficits of Korsakoff patients in a new gambling task with explicit rules: associations with executive functions. Neuropsychology 19:267. doi: 10.1037/0894-4105.19.3.267
Brands, A. M., Biessels, G. J., De Haan, E. H., Kappelle, L. J., and Kessels, R. P. (2005). The effects of type 1 diabetes on cognitive performance: a meta-analysis. Diabetes Care 28, 726–735. doi: 10.2337/diacare.28.3.726
Braver, T. S. (2012). The variable nature of cognitive control: a dual mechanisms framework. Trends Cogn. Sci. 16, 106–113. doi: 10.1016/j.tics.2011.12.010
Brinkworth, G. D., Buckley, J. D., Noakes, M., Clifton, P. M., and Wilson, C. J. (2009). Long-term effects of a very low-carbohydrate diet and a low-fat diet on mood and cognitive function. Arch. Intern. Med. 169, 1873–1880. doi: 10.1001/archinternmed.2009.329
Brockmeyer, T., Simon, J. J., Becker, A., and Friederich, H. C. (2017). Reward-related decision making and long-term weight loss maintenance. Physiol. Behav. 181, 69–74. doi: 10.1016/j.physbeh.2017.09.008
Brown, B. M., Peiffer, J. J., Sohrabi, H. R., Mondal, A., Gupta, V. B., Rainey-Smith, S. R., et al. (2012). Intense physical activity is associated with cognitive performance in the elderly. Transl. Psychiatry 2:e191. doi: 10.1038/tp.2012.118
Cannavale, C. N., Hassevoort, K. M., Edwards, C. G., Thompson, S. V., Burd, N. A., Holscher, H. D., et al. (2019). Serum lutein is related to relational memory performance. Nutrients 11:768. doi: 10.3390/nu11040768
Carroll, J. B. (1993). Human Cognitive Abilities: A Survey of Factor-Analytic Studies. Cambridge: Cambridge University Press.
Chandola, T., Deary, I. J., Blane, D., and Batty, G. D. (2006). Childhood IQ in relation to obesity and weight gain in adult life: the national child development (1958) study. Int. J. Obes. 30, 1422–1432. doi: 10.1038/sj.ijo.0803279
Cheke, L. G., Simons, J. S., and Clayton, N. S. (2016). Higher body mass index is associated with episodic memory deficits in young adults. Q. J. Exp. Psychol. 69, 2305–2316. doi: 10.1080/17470218.2015.1099163
Cherbuin, N., Sargent-Cox, K., Fraser, M., Sachdev, P., and Anstey, K. J. (2015). Being overweight is associated with hippocampal atrophy: the PATH through life study. Int. J. Obes. 39:1509. doi: 10.1038/ijo.2015.106
Cheval, B., Orsholits, D., Sieber, S., Courvoisier, D., Cullati, S., and Boisgontier, M. P. (2020). Relationship between decline in cognitive resources and physical activity. Health Psychol. 39, 519–528. doi: 10.1037/hea0000857
Coppin, G., Nolan-Poupart, S., Jones-Gotman, M., and Small, D. M. (2014). Working memory and reward association learning impairments in obesity. Neuropsychologia 65, 146–155. doi: 10.1016/j.neuropsychologia.2014.10.004
Corbetta, M., Kincade, J. M., and Shulman, G. L. (2002). Neural systems for visual orienting and their relationships to spatial working memory. J. Cogn. Neurosci. 14, 508–523. doi: 10.1162/089892902317362029
Cournot, M. C. M. J., Marquie, J. C., Ansiau, D., Martinaud, C., Fonds, H., Ferrieres, J., et al. (2006). Relation between body mass index and cognitive function in healthy middle-aged men and women. Neurology 67, 1208–1214. doi: 10.1212/01.wnl.0000238082.13860.50
Cukierman, T., Gerstein, H. C., and Williamson, J. D. (2005). Cognitive decline and dementia in diabetes—systematic overview of prospective observational studies. Diabetologia 48, 2460–2469. doi: 10.1007/s00125-005-0023-4
Davidson, T. L., Hargrave, S. L., Swithers, S. E., Sample, C. H., Fu, X., Kinzig, K. P., et al. (2013). Inter-relationships among diet, obesity and hippocampal-dependent cognitive function. Neuroscience 253, 110–122. doi: 10.1016/j.neuroscience.2013.08.044
Davis, C. (2009). Psychobiological traits in the risk profile for overeating and weight gain. Int. J. Obes. (Lond.) 33(Suppl 2), S49–S53.
Di Blasio, A., Di Donato, F., D’Angelo, E., Berchicci, M., Gallina, S., Ripari, P., et al. (2010). Determinants of short-term memory: is there a difference between young adults and postmenopausal women? Minerva Med. 101, 295–303.
Dore, G. A., Elias, M. F., Robbins, M. A., Budge, M. M., and Elias, P. K. (2008). Relation between central adiposity and cognitive function in the Maine–Syracuse study: attenuation by physical activity. Ann. Behav. Med. 35, 341–350. doi: 10.1007/s12160-008-9038-7
Duchesne, M., Mattos, P., Appolinário, J. C., Freitas, S. R., Coutinho, G., Santos, C., et al. (2010). Assessment of executive functions in obese individuals with binge eating disorder. Revist. Bras. Psiquiatr. 32, 381–388. doi: 10.1590/s1516-44462010000400011
Eisenberg, I. W., Bissett, P. G., Zeynep Enkavi, A., Li, J., MacKinnon, D. P., Marsch, L. A., et al. (2019). Uncovering the structure of self-regulation through data-driven ontology discovery. Nat. Commun. 10:2319. doi: 10.1038/s41467-019-10301-1
Elias, M. F., Elias, P. K., Sullivan, L. M., Wolf, P. A., and D’agostino, R. B. (2003). Lower cognitive function in the presence of obesity and hypertension: the Framingham heart study. Int. J. Obes. 27:260. doi: 10.1038/sj.ijo.802225
Elliot, A. J. (2006). The hierarchical model of approach-avoidance motivation. Motiv. Emot. 30, 111–116. doi: 10.1007/s11031-006-9028-7
Emery, R. L., and Levine, M. D. (2017). Questionnaire and behavioral task measures of impulsivity are differentially associated with body mass index: a comprehensive meta-analysis. Psychol. Bull. 143, 868–902. doi: 10.1037/bul0000105
Espeland, M. A., Small, D. M., and Stoeckel, L. E. (2018). “Diet, obesity, and physical inactivity: linking diabetes and dementia,” in Type 2 Diabetes and Dementia, eds V. Srikanth and Z. Arvanitakis (Amsterdam: Elsevier), 117–141. doi: 10.1016/b978-0-12-809454-9.00007-x
Feinkohl, I., Aung, P. P., Keller, M., Robertson, C. M., Morling, J. R., McLachlan, S., et al. (2014). Severe hypoglycemia and cognitive decline in older people with type 2 diabetes: the Edinburgh type 2 diabetes study. Diabetes Care 37, 507–515. doi: 10.2337/dc13-1384
Freeman, L. R., Haley-Zitlin, V., Rosenberger, D. S., and Granholm, A.-C. (2014). Damaging effects of a high-fat diet to the brain and cognition: a review of proposed mechanisms. Nutr. Neurosci. 17, 241–251. doi: 10.1179/1476830513y.0000000092
Glosser, G., Butters, N., and Kaplan, E. (1977). Visuoperceptual processes in brain damaged patients on the digit symbol substitution test. Int. J. Neurosci. 7, 59–66. doi: 10.3109/00207457709147202
Gold, J. M., Fuller, R. L., Robinson, B. M., Braun, E. L., and Luck, S. J. (2007). Impaired top–down control of visual search in schizophrenia. Schizophrenia Res. 94, 148–155. doi: 10.1016/j.schres.2007.04.023
Gold, J. M., Robinson, B., Leonard, C. J., Hahn, B., Chen, S., McMahon, R. P., et al. (2018). Selective attention, working memory, and executive function as potential independent sources of cognitive dysfunction in schizophrenia. Schizophrenia Bull. 44, 1227–1234. doi: 10.1093/schbul/sbx155
Goldstein, E. B. (2014). Cognitive Psychology: Connecting Mind, Research and Everyday Experience. Scarborough, ON: Nelson Education.
Gonzales, M. M., Tarumi, T., Miles, S. C., Tanaka, H., Shah, F., and Haley, A. P. (2010). Insulin sensitivity as a mediator of the relationship between BMI and working memory−related brain activation. Obesity 18, 2131–2137. doi: 10.1038/oby.2010.183
Grammes, J., Schäfer, M., Benecke, A., Löw, U., Klostermann, A. L., Kubiak, T., et al. (2018). Fear of hypoglycemia in patients with type 2 diabetes: the role of interoceptive accuracy and prior episodes of hypoglycemia. J. Psychosom. Res. 105, 58–63. doi: 10.1016/j.jpsychores.2017.12.010
Gunstad, J., Lhotsky, A., Wendell, C. R., Ferrucci, L., and Zonderman, A. B. (2010). Longitudinal examination of obesity and cognitive function: results from the Baltimore longitudinal study of aging. Neuroepidemiology 34, 222–229. doi: 10.1159/000297742
Gunstad, J., Paul, R. H., Cohen, R. A., Tate, D. F., Spitznagel, M. B., and Gordon, E. (2007). Elevated body mass index is associated with executive dysfunction in otherwise healthy adults. Comp. Psychiatry 48, 57–61. doi: 10.1016/j.comppsych.2006.05.001
Halyburton, A. K., Brinkworth, G. D., Wilson, C. J., Noakes, M., Buckley, J. D., Keogh, J. B., et al. (2007). Low-and high-carbohydrate weight-loss diets have similar effects on mood but not cognitive performance. Am. J. Clin. Nutr. 86, 580–587. doi: 10.1093/ajcn/86.3.580
Hargrave, S. L., Jones, S., and Davidson, T. L. (2016). The outward spiral: a vicious cycle model of obesity and cognitive dysfunction. Curr. Opin. Behav. Sci. 9, 40–46. doi: 10.1016/j.cobeha.2015.12.001
Hayden, K. M., Baker, L. D., Bray, G., Carvajal, R., Demos-McDermott, K., Hergenroeder, A. L., et al. (2018). Long-term impact of intensive lifestyle intervention on cognitive function assessed with the National Institutes of Health Toolbox: the look AHEAD study. Alzheimers Dement. 10, 41–48. doi: 10.1016/j.dadm.2017.09.002
Henrich, J., Heine, S. J., and Norenzayan, A. (2010). The weirdest people in the world? Behav. Brain Sci. 33, 61–83. doi: 10.1017/s0140525x0999152x
Herbert, B. M., Blechert, J., Hautzinger, M., Matthias, E., and Herbert, C. (2013). Intuitive eating is associated with interoceptive sensitivity. Effects on body mass index. Appetite 70, 22–30. doi: 10.1016/j.appet.2013.06.082
Herbert, B. M., and Pollatos, O. (2014). Attenuated interoceptive sensitivity in overweight and obese individuals. Eat. Behav. 15, 445–448. doi: 10.1016/j.eatbeh.2014.06.002
Higgs, S., and Spetter, M. S. (2018). Cognitive control of eating: the role of memory in Appetite and weight gain. Curr. Obes. Rep. 7, 50–59. doi: 10.1007/s13679-018-0296-9
Holdnack, J. A., Zhou, X., Larrabee, G. J., Millis, S. R., and Salthouse, T. A. (2011). Confirmatory factor analysis of the WAIS-IV/WMS-IV. Assessment 18, 178–191. doi: 10.1177/1073191110393106
Huang, Z. Z., Zhang, Y. C., Zheng, Y., Guo, Y. F., Ruan, Y., Sun, S. Y., et al. (2018). Associations of obesity and physical activity with cognition in people aged 50 and above in Shanghai. Zhonghua Liu Xing Bing Xue Za Zhi 39, 273–279.
Kahneman, D., and Tversky, A. (2000). Choices, Values, and Frames. Cambridge: Russell Sage Foundation.
Kazdin, A. E. (2000). Encyclopedia of Psychology, Vol. 2. Washington, DC: American Psychological Association.
Koch, A., and Pollatos, O. (2014). Interoceptive sensitivity, body weight and eating behavior in children: a prospective study. Front. Psychol. 5:1003. doi: 10.3389/fpsyg.2014.01003
Koffarnus, M. N., Franck, C. T., Stein, J. S., and Bickel, W. K. (2015). A modified exponential behavioral economic demand model to better describe consumption data. Exp. Clin. Psychopharmacol. 23:504. doi: 10.1037/pha0000045
Koritzky, G., Rice, C., Dieterle, C., and Bechara, A. (2015). The biggest loser thinks long-term: recency as a predictor of success in weight management. Front. Psychol. 6:1864. doi: 10.3389/fpsyg.2015.01864
Lee, J. J., Wedow, R., Okbay, A., Kong, E., Maghzian, O., Zacher, M., et al. (2018). Gene discovery and polygenic prediction from a genome-wide association study of educational attainment in 1.1 million individuals. Nat. Genet. 50, 1112–1121.
Lezak, M. D., Howieson, D. B., Bigler, E. D., and Tranel, D. (2012). Neuropsychological Assessment. Oxford: Oxford University Press.
Lopez-Garcia, P., Cristobal-Huerta, A., Espinoza, L. Y., Molero, P., Sanchez-Pedreño, F. O., and Hernández-Tamames, J. A. (2016). The influence of the COMT genotype in the underlying functional brain activity of context processing in schizophrenia and in relatives. Prog. Neuro Psychopharmacol. Biol. Psychiatry 71, 176–182. doi: 10.1016/j.pnpbp.2016.07.005
MacDonald, A. W. III (2008). Building a clinically relevant cognitive task: case study of the AX paradigm. Schizophrenia Bull. 34, 619–628. doi: 10.1093/schbul/sbn038
MacLean, P. S., Rothman, A. J., Nicastro, H. L., Czajkowski, S. M., Agurs-Collins, T., Rice, E. L., et al. (2018). The accumulating data to optimally predict obesity treatment (ADOPT) core measures project: rationale and approach. Obesity 26(Suppl 2), S6–S15.
Manly, J. J. (2008). Critical issues in cultural neuropsychology: profit from diversity. Neuropsychol. Rev. 18:179. doi: 10.1007/s11065-008-9068-8
Mata, F., Treadway, M., Kwok, A., Truby, H., Yücel, M., Stout, J. C., et al. (2017). Reduced willingness to expend effort for reward in obesity: link to adherence to a 3−month weight loss intervention. Obesity 25, 1676–1681. doi: 10.1002/oby.21948
Miller, E. K., and Wallis, J. D. (2009). Executive function and higher-order cognition: definition and neural substrates. Encycl. Neurosci. 4, 99–104. doi: 10.1016/b978-008045046-9.00418-6
Miyake, A., Friedman, N. P., Emerson, M. J., Witzki, A. H., Howerter, A., and Wager, T. D. (2000). The unity and diversity of executive functions and their contributions to complex. Cogn. Psychol. 41, 49–100. doi: 10.1006/cogp.1999.0734
Morris, M. J., Beilharz, J. E., Maniam, J., Reichelt, A. C., and Westbrook, R. F. (2015). Why is obesity such a problem in the 21st century? The intersection of palatable food, cues and reward pathways, stress, and cognition. Neurosci. Biobehav. Rev. 58, 36–45. doi: 10.1016/j.neubiorev.2014.12.002
Palta, P., Schneider, A. L., Biessels, G. J., Touradji, P., and Hill-Briggs, F. (2014). Magnitude of cognitive dysfunction in adults with type 2 diabetes: a meta-analysis of six cognitive domains and the most frequently reported neuropsychological tests within domains. J. Int. Neuropsychol. Soc. 20, 278–291. doi: 10.1017/s1355617713001483
Raji, C. A., Ho, A. J., Parikshak, N. N., Becker, J. T., Lopez, O. L., Kuller, L. H., et al. (2010). Brain structure and obesity. Hum. Brain Mapp. 31, 353–364. doi: 10.1002/hbm.20870
Rapp, M. A., and Reischies, F. M. (2005). Attention and executive control predict Alzheimer disease in late life: results from the Berlin Aging Study (BASE). Am. J. Geriatr. Psychiatry 13, 134–141. doi: 10.1097/00019442-200502000-00007
Reddy, L. F., Horan, W. P., Barch, D. M., Buchanan, R. W., Dunayevich, E., Gold, J. M., et al. (2015). Effort-based decision-making paradigms for clinical trials in schizophrenia: part 1—psychometric characteristics of 5 paradigms. Schizophrenia Bull. 41, 1045–1054. doi: 10.1093/schbul/sbv089
Rietveld, C. A., Conley, D., Eriksson, N., Esko, T., Medland, S. E., Vinkhuyzen, A. A. E., et al. (2014). Replicability and robustness of genome-wide-association studies for behavioral traits. Psychol. Sci. 25, 1975–1986.
Rosano, C., Newman, A. B., Katz, R., Hirsch, C. H., and Kuller, L. H. (2008). Association between lower digit symbol substitution test score and slower gait and greater risk of mortality and of developing incident disability in well−functioning older adults. J. Am. Geriatr. Soc. 56, 1618–1625. doi: 10.1111/j.1532-5415.2008.01856.x
Ryan, J. J., Carruthers, C. A., Miller, L. J., Souheaver, G. T., Carruthers, C. A., Gontkovsky, S. T., et al. (2005). The WASI matrix reasoning subtest: performance in traumatic brain injury, stroke, and dementia. Int. J. Neurosci. 115, 129–136. doi: 10.1080/00207450490512704
Schäfer, L., Hübner, C., Carus, T., Herbig, B., Seyfried, F., Kaiser, S., et al. (2019). Pre-and postbariatric subtypes and their predictive value for health-related outcomes measured 3 years after surgery. Obes. Surg. 29, 230–238. doi: 10.1007/s11695-018-3524-1
Schandry, R. (1981). Heart beat perception and emotional experience. Psychophysiology 18, 483–488. doi: 10.1111/j.1469-8986.1981.tb02486.x
Schoenberg, M. R., Duff, K., Scott, J. G., and Adams, R. L. (2003). An evaluation of the clinical utility of the OPIE-3 as an estimate of premorbid WAIS-III FSIQ. Clin. Neuropsychol. 17, 308–321. doi: 10.1076/clin.17.3.308.18088
Schoenberg, M. R., Lange, R. T., Iverson, G. L., Chelune, G. J., Scott, J. G., and Adams, R. L. (2006). Clinical validation of the general ability index—estimate (GAI-E): estimating premorbid GAI. Clin. Neuropsychol. 20, 365–381. doi: 10.1080/138540491005866
Sharma, L., Markon, K. E., and Clark, L. A. (2014). Toward a theory of distinct types of “impulsive” behaviors: a meta-analysis of self-report and behavioral measures. Psychol. Bull. 140, 374–408. doi: 10.1037/a0034418
Silveira, P. P., Agranonik, M., Faras, H., Portella, A. K., Meaney, M. J., and Levitan, R. D. (2012). Preliminary evidence for an impulsivity-based thrifty eating phenotype. Pediatr. Res. 71:293. doi: 10.1038/pr.2011.39
Sliwinski, M. J., Mogle, J. A., Hyun, J., Munoz, E., Smyth, J. M., and Lipton, R. B. (2018). Reliability and validity of ambulatory cognitive assessments. Assessment 25, 14–30. doi: 10.1177/1073191116643164
Starcke, K., Agorku, J. D., and Brand, M. (2017). Exposure to unsolvable anagrams impairs performance on the Iowa Gambling Task. Front. Behav. Neurosci. 11:114. doi: 10.3389/fnbeh.2017.00114
Stein, J. S., Sze, Y. Y., Athamneh, L., Koffarnus, M. N., Epstein, L. H., and Bickel, W. K. (2017). Think fast: rapid assessment of the effects of episodic future thinking on delay discounting in overweight/obese participants. J. Behav. Med. 40, 832–838. doi: 10.1007/s10865-017-9857-8
Stevenson, R. J., Francis, H. M., Attuquayefio, T., Gupta, D., Yeomans, M. R., Oaten, M. J., et al. (2020). Hippocampal-dependent appetitive control is impaired by experimental exposure to a Western-style diet. R. Soc. Open Sci. 7:191338. doi: 10.1098/rsos.191338
Strachan, M. W., Deary, I. J., Ewing, F. M., and Frier, B. M. (1997). Is type II diabetes associated with an increased risk of cognitive dysfunction: a critical review of published studies. Diabetes Care 20, 438–445. doi: 10.2337/diacare.20.3.438
Thiara, G., Cigliobianco, M., Muravsky, A., Paoli, R. A., Mansur, R., Hawa, R., et al. (2017). Evidence for neurocognitive improvement after bariatric surgery: a systematic review. Psychosomatics 58, 217–227. doi: 10.1016/j.psym.2017.02.004
Tranel, D., Manzel, K., and Anderson, S. W. (2008). Is the prefrontal cortex important for fluid intelligence? A neuropsychological study using matrix reasoning. Clin. Neuropsychol. 22, 242–261. doi: 10.1080/13854040701218410
Treadway, M. T., Bossaller, N. A., Shelton, R. C., and Zald, D. H. (2012). Effort-based decision-making in major depressive disorder: a translational model of motivational anhedonia. J. Abnorm. Psychol. 121:553.
Vainik, U., Baker, T. E., Dadar, M., Zeighami, Y., Michaud, A., Zhang, Y., et al. (2018). Neurobehavioral correlates of obesity are largely heritable. Proc. Natl. Acad. Sci. U.S.A. 115, 9312–9317.
Vainik, U., Dagher, A., Dubé, L., and Fellows, L. K. (2013). Neurobehavioural correlates of body mass index and eating behaviours in adults: a systematic review. Neurosci. Biobehav. Rev. 37, 279–299.
Van Dyck, Z., Vögele, C., Blechert, J., Lutz, A. P., Schulz, A., and Herbert, B. M. (2016). The water load test as a measure of gastric interoception: development of a two-stage protocol and application to a healthy female population. PLoS One 11:e0163574. doi: 10.1371/journal.pone.0163574
Volkow, N. D., and Baler, R. D. (2015). NOW vs LATER brain circuits: implications for obesity and addiction. Trends Neurosci. 38, 345–352.
Watson, P. D., Voss, J. L., Warren, D. E., Tranel, D., and Cohen, N. J. (2013). Spatial reconstruction by patients with hippocampal damage is dominated by relational memory errors. Hippocampus 23, 570–580. doi: 10.1002/hipo.22115
Wessel, J. R. (2018). Prepotent motor activity and inhibitory control demands in different variants of the go/no−go paradigm. Psychophysiology 55:e12871.
White, N. M. (1989). Reward or reinforcement: what’s the difference? Neurosci. Biobehav. Rev. 13, 181–186.
Whitmer, R. A., Gunderson, E. P., Barrett-Connor, E., Quesenberry, C. P. Jr., and Yaffe, K. (2005). Obesity in middle age and future risk of dementia: a 27 year longitudinal population based study. BMJ 330:1360. doi: 10.1136/bmj.38446.466238.E0
Wu, M., Brockmeyer, T., Hartmann, M., Skunde, M., Herzog, W., and Friederich, H. C. (2016). Reward-related decision making in eating and weight disorders: a systematic review and meta-analysis of the evidence from neuropsychological studies. Neurosci. Biobehav. Rev. 61, 177–196.
Yang, Y., Shields, G. S., Guo, C., and Liu, Y. (2018). Executive function performance in obesity and overweight individuals: a meta-analysis and review. Neurosci. Biobehav. Rev. 84, 225–244.
Keywords: executive function, cognitive control, reward, motivation, decision-making, memory, sensation, perception
Citation: D’Ardenne K, Savage CR, Small D, Vainik U and Stoeckel LE (2020) Core Neuropsychological Measures for Obesity and Diabetes Trials: Initial Report. Front. Psychol. 11:554127. doi: 10.3389/fpsyg.2020.554127
Received: 21 April 2020; Accepted: 27 August 2020;
Published: 24 September 2020.
Edited by:
Boris C. Rodríguez-Martín, Fundación Recal, SpainReviewed by:
Naiman A. Khan, University of Illinois at Urbana–Champaign, United StatesCopyright © 2020 D’Ardenne, Savage, Small, Vainik and Stoeckel. This is an open-access article distributed under the terms of the Creative Commons Attribution License (CC BY). The use, distribution or reproduction in other forums is permitted, provided the original author(s) and the copyright owner(s) are credited and that the original publication in this journal is cited, in accordance with accepted academic practice. No use, distribution or reproduction is permitted which does not comply with these terms.
*Correspondence: Cary R. Savage, Y3NhdmFnZUB1bmwuZWR1; Luke E. Stoeckel, bHVrZS5zdG9lY2tlbEBuaWguZ292
Disclaimer: All claims expressed in this article are solely those of the authors and do not necessarily represent those of their affiliated organizations, or those of the publisher, the editors and the reviewers. Any product that may be evaluated in this article or claim that may be made by its manufacturer is not guaranteed or endorsed by the publisher.
Research integrity at Frontiers
Learn more about the work of our research integrity team to safeguard the quality of each article we publish.