- 1Institute of Psychology, University of Innsbruck, Innsbruck, Austria
- 2Department of Medical Psychology, Medical University Innsbruck, Innsbruck, Austria
Reports of medical students experiencing burnout-related symptoms (e.g., cynicism) have increased in recent years. Little is known about the developmental process of this phenomenon and its relations with signature character strengths and well-being. The aim of this longitudinal analysis was to explore changes in the level of cynicism of medical students while in preclinical education. We further examined how the applicability of signature character strengths and well-being are related to this developmental process. Medical students (N = 99) participated in three online surveys over 3 years during medical school. Latent growth modeling, latent class growth modeling, general mixed modeling was conducted, and post hoc mixed ANOVA, Friedman test and Welch test analyses were examined. The results showed an increase in cynicism among medical students from first to last measurement. Two groups with distinct developmental trajectory patterns of cynicism were identified. Students with high levels of cynicism (high-level group) and students with changing levels of cynicism (increasing group) perceived higher applicability of signature character strengths in private life compared to the study context. Moreover, the high-level group experienced significantly lower psychological well-being (in particular mastery, optimism, and relationship) in their first year of medical education. This explorative study offers a comprehensive understanding of cynicism development in medical students during medical school and its relations to the applicability of signature character strengths and well-being. Prospective replication studies are needed to replicate the results obtained in this study.
Introduction
Medical universities are responsible for theoretical and practical scientific education, aiming to train future physicians to become professional, skillful and occupationally competent graduates. Therefore, universities provide a curriculum offering diverse methods such as lectures, supervised practice mentoring, and communication training in order to reach these goals. For a considerable number of medical students, this longstanding training process takes its toll regarding their physical health (e.g., infections due to exposure to bacteria or viruses in hospitals), mental health (e.g., Brazeau et al., 2014), and well-being (e.g., Dunn et al., 2008).
One of the continuous major concerns of researchers is the development of burnout during the time in medical school (e.g., Dahlin and Runeson, 2007; Dyrbye and Shanafelt, 2016), which is associated with several consequences affecting health, such as alcohol abuse (Jackson et al., 2016) and suicidal ideation (Dyrbye et al., 2008; Kosik et al., 2017). According to Dyrbye et al. (2014), 56% of medical students experienced symptoms of burnout and over 11% reported suicidal thoughts (Rotenstein et al., 2016). Furthermore, a meta-analysis by Frajerman et al. (2018) exposed that burnout is prevalent among 44.2% of medical students. In line with these results, Prinz et al. (2012) described similar findings for a German sample. Burnout among students is considered a three-dimensional psychological syndrome of emotional exhaustion, cynicism, and reduced personal accomplishment, which results in the deterioration of academic engagement (Schaufeli et al., 2002b). Furthermore, it plays a potentially important role in predicting future burnout after becoming a graduated professional (Robins et al., 2017). Emotional exhaustion and cynicism are often perceived as the main dimensions of burnout (Mäkikangas and Kinnunen, 2016), whereas reduced personal accomplishment was not as strongly related to these two burnout components, and therefore challenged for its questionable validity (Bresó et al., 2007; Schaufeli and Salanova, 2007).
Maslach and Leiter (2016) criticized the approach of solely reducing burnout to the dimension of emotional exhaustion, as it tends to be done increasingly nowadays. According to Leiter and Maslach (2016), cynicism does play a crucial role in work environments, as it reflects “the poor quality of social relationships at work and the lack of critical resources” (p. 98). Furthermore, the substance of cynicism has been emphasized, as it seems to have a more important role in the burnout experience than emotional exhaustion alone, which is why the authors suggested that further analyses should pay more attention to the dimension of cynicism (Leiter and Maslach, 2016). “The cynicism dimension was originally called depersonalization (given the nature of human services occupations), but was also described as negative or inappropriate attitudes toward clients, irritability, loss of idealism, and withdrawal” (Maslach and Leiter, 2016, p. 103). Regarding the medical education process, cynicism is more of a distant or indifferent attitude toward one’s own studies and their potential usefulness.
Many researchers have described the increase of cynicism beginning in medical school (e.g., Wolf et al., 1989; Dyrbye et al., 2005) and progressing during residency (Collier et al., 2002). One might suppose that most medical students start their training with the idea of helping people (Pagnin et al., 2013) and doing the “right thing,” which is reflected in some sense of idealism. Although this attitude seems to be noble, it will not last for a long time (Morley et al., 2013). Fading idealism is associated with increased weariness concerning the medical profession, reduced interest in helping underserved people and reduced feelings of responsibility for the health of society in general (Crandall et al., 1993; Woloschuk et al., 2004; Smith and Weaver, 2006). The combined decline of idealism and increase of cynicism was initially described in the mid-1950s (Eron, 1955). Although decreases in empathy and growth of cynicism during medical school have been well narrated, there is still some debate on whether both processes occur as a result of training progress and its formal, informal and hidden curriculum (e.g., Feudtner et al., 1994; Hojat et al., 2009; Neumann et al., 2011). In general, cynicism was found to be related to declines in physical health, indicated by higher BMI scores and C-reactive protein levels, a decrease in heart rate variability and self-rated physical health (Viljoen and Claassen, 2017). Apart from the links with physical health, there are also drawbacks concerning the interpersonal and mental level. For example, a cynical attitude in the environment of medical training contributes to the erosion of professionalism (Dyrbye et al., 2005). Moreover, cynicism is crucial to the burnout experience, as it was found to predict turnover in the health care sector (Leiter and Maslach, 2009), and study-related cynicism forecasted work-related cynicism as noticed by a longitudinal analysis (Robins et al., 2017). Furthermore, a qualitative study by Peng et al. (2018) revealed that cynicism was associated with negative effects on physicians’ career satisfaction, quality of patient care and quality of mentorship for following generations. The development of cynicism in medical school is possibly associated with a depersonalized attitude as physician, which results in poorer communication skills (Brown et al., 2009), lower patient satisfaction or longer post-discharge recovery time (Halbesleben and Rathert, 2008). In contrast to these findings, cynicism may be seen to act as coping mechanism to reduce stress (McManus et al., 2002), handle new situations (Wolf et al., 1989) or create distance between oneself and the patients’ hardship (Kopelman, 1983; Peng et al., 2018).
The Job Demands-Resources Model by Bakker and Demerouti (2007) assumes that burnout occurs when people encounter an imbalance between job demands and resources. Therefore, high demands (e.g., work overload or time pressure) in work environments are risk factors for the development of health-related problems (e.g., symptoms of burnout). This can be reduced by job resources (e.g., supportive colleagues, autonomy) capable of dealing with such demands (Schaufeli and Bakker, 2004). This model has also shown to be applicable in the context of health professional studies (Gusy et al., 2016). Some researchers blame the unfavorable circumstances in the learning environment of medical education for the development of burnout (e.g., Dyrbye and Shanafelt, 2016). This environment is characterized by highly demanding study conditions, while condition-related resources often appear to lack (Dahlin et al., 2005). Although it might seem clear that structures and conditions should be changed in order to create an environment contributing to health, this process is not an easy one. Therefore, it is necessary to consider personal resources.
A study by Heinen et al. (2017), conducted with a sample of medical students, found that personal resources (e.g., optimism, self-efficacy, and resilient coping) can act as a buffer in the perception of study-related stressors and emotional distress. “Personal resources are aspects of the self that are generally linked to resiliency” (p. 632) and these resources are related to the individuals’ sense of their ability to master or influence their environment successfully (Hobfoll et al., 2003). According to Bandura (2000), environmental factors play a crucial role in nurturing the individual level of personal resources, and in turn, these resources are connected to people’s capability to perceive and adapt to their environment.
Character strengths as a concept of (Seligman and Csikszentmihalyi, 2000) positive psychology can be seen as such personal resources. They are components of the good character, morally valued, trait-like and can be facilitated by rituals or institutions (Peterson and Seligman, 2004). Furthermore, they are considered essential, as they relate to stress coping behavior in health care professionals (Harzer and Ruch, 2015). Character strengths have positive associations with overall perceived health (Proyer et al., 2014) and a unique value in predicting physical health (Hanks et al., 2014). Furthermore, physical and mental health were positively related to character strengths (Leontopoulou and Triliva, 2012). A study by Hausler et al. (2017b) found character strengths to be linked with well-being and mental health but not with physical health in a sample of medical students and resident physicians. Moreover, character strengths are related to physicians’ engagement and patient care (Seoane et al., 2016). Peterson and Seligman (2004) proposed that most people have 3–5 core or “signature” character strengths, which are typical of the respective individual. These signature character strengths are the ones a person “celebrates, and frequently exercises” and it is believed that their “exercise […] is fulfilling” (Peterson and Seligman, 2004, p. 18). Signature character strengths fulfill several criteria (Peterson and Seligman, 2004), e.g., “feeling of invigoration when using a signature strength” or “desire to act in accordance with the signature strength.” It might be assumed that the use of signature character strengths leads to better relations, positive emotions and meaning (Seligman, 2011). Several studies have shown that they can also contribute to physical and mental health, well-being, academic work and life satisfaction (Peterson and Seligman, 2004; Seligman et al., 2005; Peterson and Park, 2011; Allan and Duffy, 2014).
Besides the sole possession of character strengths, it is important to account for their applicability. According to Harzer and Ruch (2012, 2013) two conditions must be met to apply character strengths: Firstly, one’s possession of the character strength is crucial to show strength-related behavior. Secondly, circumstantial conditions (e.g., private life or workplace/studies) allow or call for the display of character strengths. Hence, the “Applicability of Character Strengths Rating Scale” ACS-RS (Harzer and Ruch, 2013) was developed to measure the individually perceived influence of four conditions: two external ones: (I) normative demands and (II) appropriateness of strength-related behavior in given situations; two internal ones: (III) perceived presence of factors that may facilitate or hamper strength-related behavior and (IV) intrinsic motivation to show certain behavior (Harzer and Ruch, 2012, 2013). Recently conducted cross-sectional studies with samples of medical students and physicians emphasized the important role of one’s applicable signature character strengths (applicability of signature character strengths in the context of study and work = ASCS-S/-W; and private life = ASCS-P) to foster mental health, well-being, burnout and work-engagement (Hausler et al., 2017a; Huber et al., 2019; Strecker et al., 2019). Furthermore, Höge et al. (2019) could demonstrate with a sample of hospital physicians that the ASCS-W at the first measurement (T1) had an impact on the perception of the socio-moral climate of the medical department at the second measurement (T2) and reversed effects of the socio-moral climate at T1 on the ASCS at work at T2. Moreover, cross-sectional results showed an indirect effect of the socio-moral climate on work engagement and psychological well-being via the ASCS at work.
Altogether, studies in the field of positive psychology have rarely focused on medical students. In particular, due to a lack of longitudinal data in this area, it merits investigating potential variables and their interrelations contributing to the health of medical students. Thus, this study takes a closer look at the following research gaps:
Firstly, there is no information regarding the homogeneity of the developmental process of cynicism in Austrian medical students. The authors assume that an increase in cynicism is prevalent among preclinical medical students during their time in medical school, which has also been shown in other studies with different samples.
Secondly, to the best of the authors’ knowledge there have been no studies so far exploring the developmental process of cynicism and possible differences among individuals. Therefore, this study investigated whether there are different trajectory patterns and how groups of students may differ from each other.
Thirdly, the applicability of signature character strengths could be a personal resource when it comes to predicting burnout-symptoms (e.g., Leiter et al., 2012). Although studies highlighted the relevance of the congruence between persons characteristics and university-related environmental fit on health outcomes (e.g., Jiang and Jiang, 2015), there is a research gap regarding the potential role of the applicability of signature character strengths on the development of cynicism during medical school. Thus, associations between the applicability of signature character strengths and developmental patterns of cynicism were analyzed. Furthermore, we examined the relationship between subjective well-being, psychological well-being (autonomy, engagement, mastery, meaning, optimism, and relationship) and trajectory patterns of cynicism.
Materials and Methods
Participants and Procedure
The longitudinal data collection was conducted between January 2015 and March 2017 at a medical university in Austria. The institutional review board gave approval and participants completed online questionnaires after being invited via email at three time points. Offered incentives were medical education credits, as well as a raffle of medical books relevant to their current semester. Of all 504 participating medical students, 85.5% participated at the first (N1 = 431), 53% at the second (N2 = 267), and N3 = 101 (20%) at all three measurements. We only used complete data sets and no data imputation methods were applied. After matching those students who participated in all three annual measurement waves and excluding two outliers with overall extreme response patterns regarding relevant variables (critical z-values ± 3.29; Osborne and Overbay, 2004; Tabachnick and Fidell, 2007), the final sample consisted of 99 medical students. At baseline measurement, the medical students’ mean age was 20.3 (SD = 2.1; range = 18–28) being in their first year of medical education. Nearly two thirds of the participants were female (63.6%), and 52.5% of the participating students were Austrians followed by 25% Germans. At baseline, 74.7% of medical students were not in a relationship and of all participants, 45.5% lived in a shared apartment. 34.5% of students invested at least 40 h per week into their studies. Having a part-time job, on which they spent between 2 and 16 h per week, was reported by 16%.
Measures
The cynicism of medical students was measured with the cynicism-subscale of the German version of the “Maslach-Burnout-Inventory Student Survey” (MBI-SS-GV; Gumz et al., 2013; original: Schaufeli et al., 2002a). The cynicism-subscale consists of 4 items rated on a seven-point scale from 0 = never to 6 = daily, with higher values indicating more cynicism symptoms. An item example is: “I have become more cynical about the potential usefulness of my studies.” Cronbach’s alpha for the cynicism scale in this study was at T1 α = 0.76, at T2 α = 0.79, and at T3 α = 0.85.
Character strengths were measured with the “Values in Action Inventory of Strengths” (VIA-IS; Peterson et al., 2005). The original version of the VIA-IS has 240 Items, but for economic reasons the shorter validated 120-item German questionnaire (Höfer et al., 2019) was used to measure the 24 individual character strengths consisting of five items each, rated on a five-point scale from 1 = strongly disagree to 5 = strongly agree. Item examples are: “I am never too busy to help a friend” (kindness) or “I always keep my promises” (honesty). The German short-form has comparable psychometric properties to the 240-item version with Cronbach’s alpha ranging between α = 0.63 (honesty) and α = 0.90 (spirituality) (Höfer et al., 2019). For our sample the internal consistency was between α = 0.65 (teamwork) and α = 0.89 (spirituality). The five highest character strengths of each individual (so-called signature character strengths; Peterson and Seligman, 2004) were identified. Furthermore, following recommendations by Harzer and Ruch (2012), we considered candidates’ possessing signature character strengths, when the VIA-IS score was 3.5 or higher.
The “Applicability of Character Strengths Rating Scale” (ACS-RS; Harzer and Ruch, 2013) was used to assess the applicability of signature character strengths regarding private life (ASCS-P) and studies (ASCS-S) related conditions, independent of the possession of character strengths. The items ask if the character strength is “demanded,” “helpful,” “used,” and “important for me” in private life and studies. In the present dataset, the applicability of the five highest signature character strengths was measured after identifying them with the VIA-120. To assess the applicability, eight items (four referring to private life and the other four referring to studies) for each signature character strength were rated on a five-point scale ranging from 1 = never to 5 = (almost) always. Internal consistencies (median) were for ASCS-P at T1 α = 0.81, T2 α = 0.84, and T3 α = 0.87, for ASCS-S at T1 α = 0.76, T2 α = 0.77, and T3 α = 0.78.
Well-being was examined by using the “Comprehensive Inventory of Thriving” (CIT; Su et al., 2014). It measures seven components of well-being with 18 subscales three assigned to subjective well-being (SWB): life satisfaction, positive and negative feelings; 15 to psychological well-being (PWB): autonomy, engagement, mastery, meaning, optimism and relationship, composed of 54 items rated on a five-point scale ranging from 1 = strongly disagree to 5 = strongly agree. In addition to the calculation of a composite score of SWB and PWB, a general well-being (thriving) mean score can be computed. Item examples are: “I feel good most of the time” (SWB; positive feelings) or “I expect more good things in my life than bad” (PWB; optimism). The German version has been validated by Hausler et al. (2017), who demonstrated its reliability and validity. Internal consistencies for our sample ranged between α = 0.93 (T1), α = 0.92 (T2), and α = 0.95 (T3).
Data Analysis
Development of Cynicism
In order to examine medical students’ trajectories of cynicism, latent growth models were applied (LGM) in Mplus Version 8.2 (Muthén and Muthén, 1998–2018). LGM is a modeling technique that is capable of capturing inter-individual (between-person) variability in intra-individual (within-person) patterns of change over time (Curran et al., 2010) via estimating growth parameters. For example, intercept is the average mean beginning value, and slope is the average rate of change (e.g., linear, quadratic). According to Hamilton et al. (2003) our sample size for this analysis is adequate. Due to robustness against non-independence and non-normality of the variables, the robust maximum likelihood estimation (MLR) was selected. The following fit indices were decisive for picking the best-fitting model: (a) non-significant chi-square statistic, the (b) root mean square error of approximation (RMSEA) with values ≤ 0.08, and the (c) comparative fit index (CFI) with values > 0.90, indicating satisfying model fit (Hoyle, 2012). The LGM with a linear slope term (i.e., assuming there is a linear growth in cynicism over time) was compared to the intercept-only model (i.e., assuming there is a stable overall level of cynicism over time) by using the Satorra–Bentler scaled chi-square difference test (Satorra and Bentler, 2001).
Capturing Heterogeneity in the Development of Cynicism
Since a conventional LGM is only capable of estimating one set of growth parameters for an entire sample, it is not possible to capture “subgroups” of people who may deviate from that average-level trajectory. Therefore, growth mixture modeling (GMM; Muthén, 2004) and latent class growth analyses (LCGA; Nagin, 2005), as exploratory data driven processes, were conducted. GMM assumes that populations can be divided into subpopulations or “classes,” each following their own distribution (Muthén, 2004). Therefore, each class has its own set of growth parameters which may be allowed to vary within and between classes. Loosely speaking, a one-class GMM with two growth parameters (i.e., intercept, linear) is in essence a conventional LGM. LCGA – as a special type of GMM – on the other hand allows no within class variability of the growth parameters, which means it is assumed that individuals within these classes are taxonomically similar regarding their outcome variables (Nagin and Odgers, 2010; Frankfurt et al., 2016).
Both methods (LCGA and GMM) assume that the number of trajectory patterns is unknown but finite. Due to this assumption, it is necessary to choose the number of classes beforehand and use a combination of several criteria to validate the given solution. LCGA can be used as first exploratory step, prior to a GMM analysis, to identify the number of classes for further analyses (Jung and Wickrama, 2008). Furthermore, LCGA is usually more “robust” to convergence problems often appearing in GMM, since it estimates fewer parameters. To face estimation problems in LCGA and GMM (e.g., finding local maxima), it is recommended to set the number of random sets of starting values for analyzed mixture models between 400 and 1000 (MPlus version 8.2; default = 20) and the number of optimizations in the final stage between 100 and 250 (MPlus version 8.2; default = 4). For the bootstrapped generated data, the number of random sets of starting values and the optimizations in the final stage for the k-class model are set to 40 respectively 8 by default in MPlus version 8.2. However, setting the value of these numbers for the analyzed and bootstrapped model mainly depends on whether replicated solutions are obtained successfully. For our analyses we chose 1000 random sets of starting values and 250 optimizations in the final stage for the analyzed model and 500 random sets of starting values and 200 optimizations in the final stage for the generated bootstrapped k-class model. Moreover, to ensure sufficient precision of the estimated p-value of the bootstrapped likelihood ratio test (BLRT) we selected 250 bootstrap-samples.
Although there is no standard procedure in identifying the “most suitable” model with an explicit number of classes, we applied eight criteria to determine the best solution: (a) the Bayesian information criterion (BIC; Schwarz, 1978), as commonly used model fit indicator, should be smaller if model fit improves; (b) sample-size-adjusted BIC (aBIC) is a more precise fit index for small sample sizes (Henson et al., 2007); (c) the Vuong–Lo–Mendell–Rubin likelihood ratio test (VLMR-LRT; Vuong, 1989; Lo et al., 2001) compares the k-1 versus the k-class solution (e.g., 2- vs. 3-classes) and indicates via significance an improved fit of the k-class solution; (d) the adjusted Lo–Mendell–Rubin likelihood ratio test (adj. LMR-LRT; Muthén, 2004) tends to produce fewer type I errors (Henson et al., 2007); (e) the bootstrapped likelihood ratio test (BLRT; McLachlan and Peel, 2000) is used to double-check the LRT solution, since it has proven to be significantly better (Nylund et al., 2007); (f) the entropy value ranges from 0 to 1 and is available for models with at least two classes. Entropy does not serve as a fit index as it is a measure for individual classification accuracy. Values of 0.75 or higher are acceptable (Reinecke, 2006); (g) each class should at least comprise 5% of the sample (Nelemans et al., 2014), although we tightened this prerequisite, as each group should include at least ten students (10.1%); (h) and finally deciding on the model with parsimonious properties (i.e., fewer number of parameters).
The chosen best-fitting model was further examined with mixed ANOVA testing and post hoc analyses regarding class differences in cynicism, applicability of signature character strengths and well-being components. For violated sphericity assumptions Huynh–Feldt correction was used, if eta (ε) was > 0.75. Post hoc analyses were corrected by Bonferroni-method in order to account for alpha error inflation. For this purpose, IBM SPSS Statistics 24 (IBM Corporation, 2016) was used.
Results
Descriptive Statistics
Table 1 presents the (inter-) correlations, means, standard deviations and internal consistencies of all variables over the period of the three annual measurement waves. Pearson correlations of cynicism (r = 0.31 to r = 0.65) and SWB (r = 0.38 to r = 0.63) vary strongly between successive waves, indicating large individual differences in the stability of cynicism and SWB over time. It can also be noted that the mean value of cynicism increases over time. There was a higher stability for ASCS-S (r = 0.42 to r = 0.60), ASCS-P (r = 0.46 to r = 0.61) and PWB (r = 0.66 to r = 0.77). Significant correlations can be found between cynicism and the applicability of signature character strengths in private life at the first (r = −0.29), second (r = −0.21) and between the first and second measurement (r = −0.32). Furthermore, ASCS-S is significantly associated with cynicism over the whole measurement period, ranging between r = −0.23 and r = −0.38. Negative correlations are shown between cynicism and SWB (r = −0.21 to r = −0.35), and even more strongly between cynicism and PWB (r = −0.26 to r = −0.49).
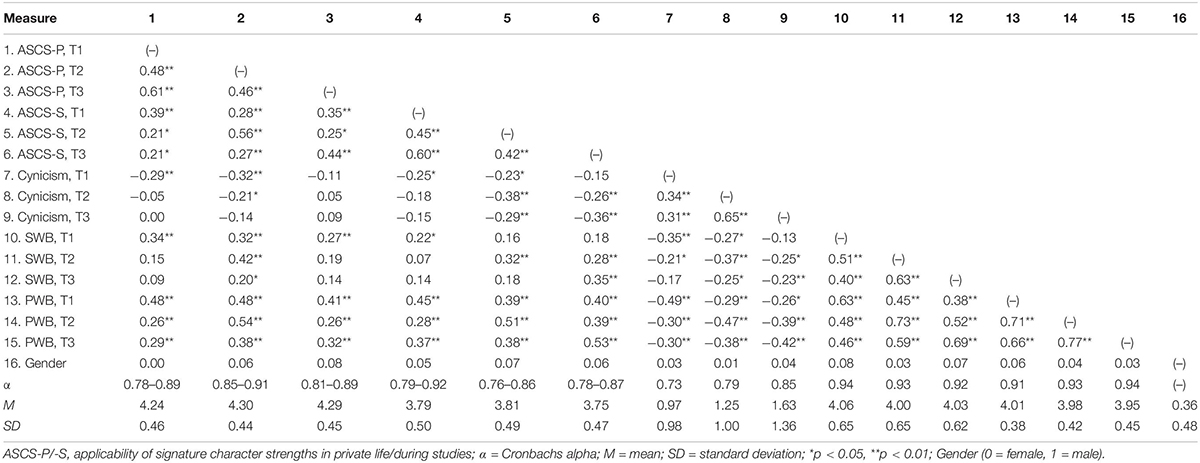
Table 1. (Inter-) correlations, means, standard deviations and internal consistencies among the variables of the study population (N = 99).
Growth Analysis of Cynicism
The next step of our analysis was to determine if the model that assumes no linear growth (intercept-only model) fits better than the model supposing linear growth (linear model) in cynicism over three measurement points. Comparing the intercept-only model with the linear LGM of cynicism [Δχ2SB (3) = 60.67, p < 0.01] revealed a better fit for the linear model (χ2 (1) = 0.54, p = 0.46, CFI = 1.00, RMSEA ≤ 0.001, 90% CI = [0.00, 0.24]). Medical students reported an initial intercept mean of 0.95 (p < 0.01) and an increasing linear slope mean of 0.33 (p < 0.01). Variances of intercept and slope were both significant (p < 0.01), indicating individual differences in starting values and rate of change in cynicism.
Latent Class Growth Analysis and Growth Mixture Modeling Comparison (LCGA vs. GMM)
All tested models and their properties are depicted in Tables 2, 3. As an initial exploratory step, LCGAs were applied to the data and up to five classes were tested until converge issues occurred. Since likelihood ratio test statistics and entropy values are not available for one-class solutions, the empty cells were filled with dashes. From all tested LCGA-models, the one with the three-class solution provided the best fit according to BIC, aBIC, LMR and entropy. Next, we conducted GMM analyses with the variances of the intercept to be estimated (GMMI). Although the BIC and aBIC suggested a slightly better fit of the three-class model, taking also the LRTs into account, a better fit of the two-class solution was evident. We then further examined GMM solutions that allowed freely varying linear slope growth parameters (GMML). Here, the best fitting model had two classes. Lastly, we compared a final set of different GMMs, with estimated intercept and linear slope growth parameters (GMMIL). According to the fit statistics, the two-class model provided the best fit. After an overall comparison between all tested models (LCGA, GMMI, GMML and GMMIL) regarding the eight mentioned criteria above (a–h), and due to the significant variances of intercept and slope of the initially tested linear LGM, the GMMIL with two classes was chosen. It was most appropriate for identifying group differences in trajectories of cynicism.
Figure 1 shows the classification solution of the GMMIL with two trajectories of cynicism. Figure 2 shows a general depiction of a GMM. The first group, labeled as high-level group, consisted of 34% of the students and was described by an initially relatively high level of cynicism, with a significant 22.5% decrease after 1 year in medical training returning to the initial level at 3 years. The second group comprised 66% of the sample and was labeled increasing group, due to the relatively strong increase in cynicism over 3 years of medical training.
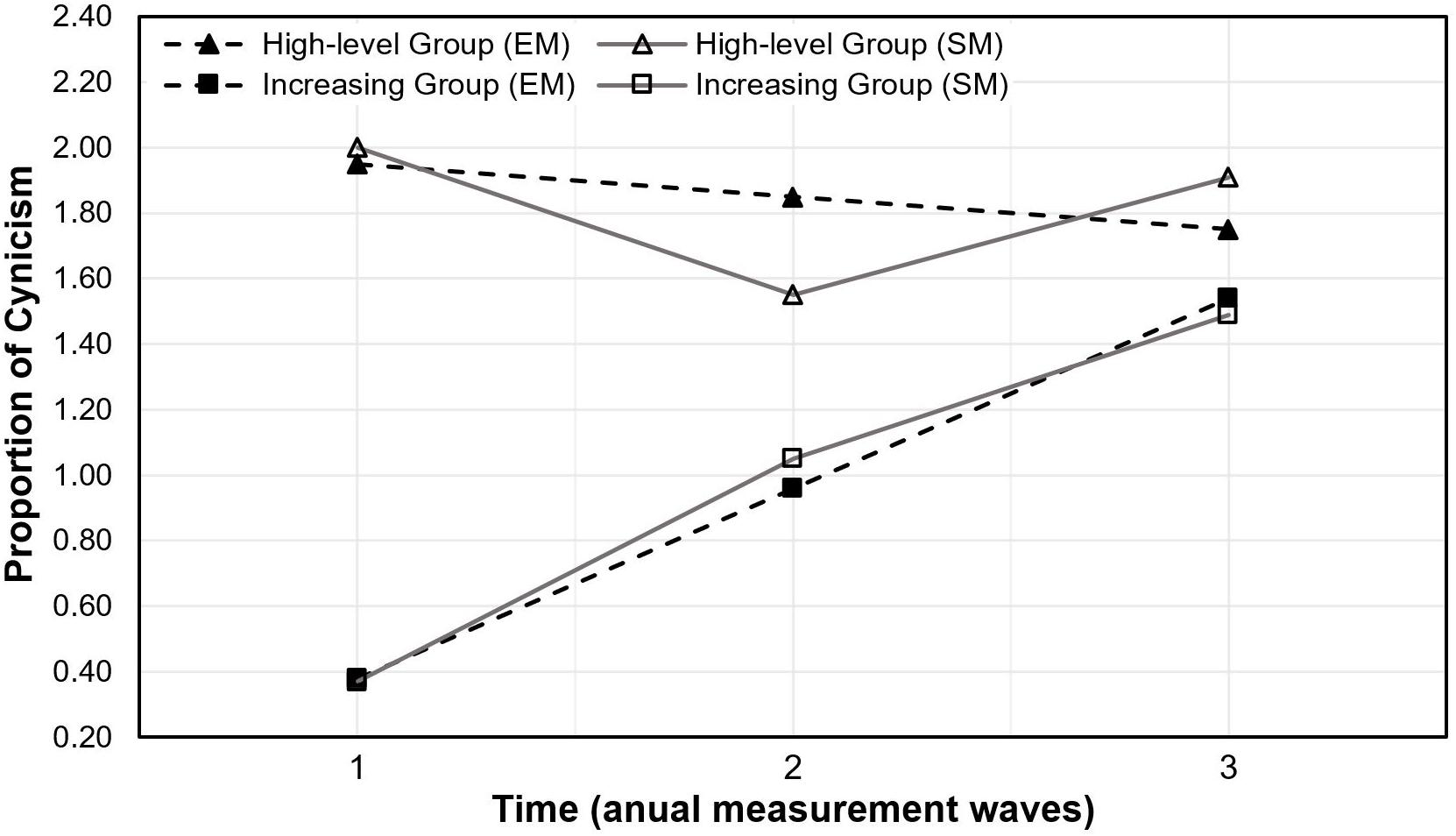
Figure 1. Two classes for trajectories of cynicism from the first to the third measurement. The high-level group is composed of 34% of the sample; the increasing group is composed of 66% of the sample. EM = estimated means; SM = sample means.
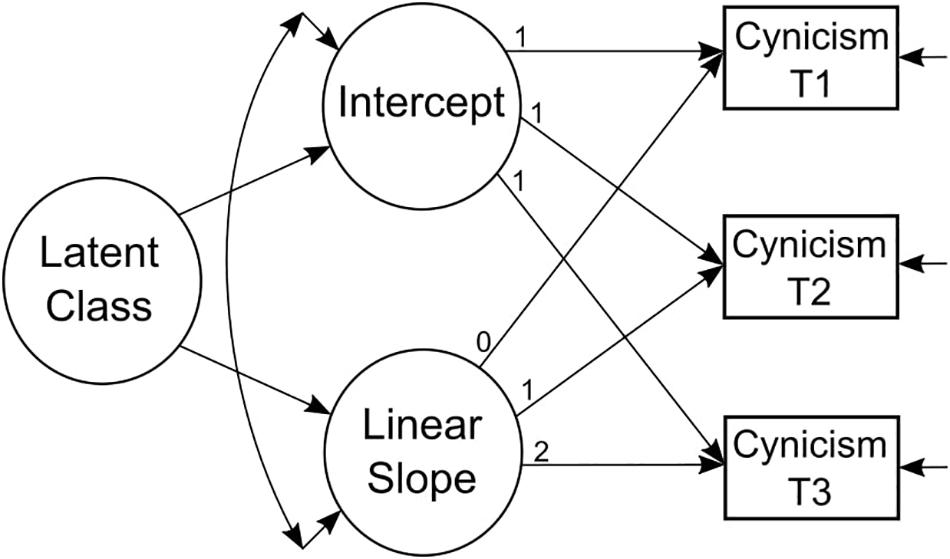
Figure 2. Growth mixture model (GMM) examines the development of cynicism in medical students from first to third year in medical training.
Post hoc Analyses of the Classification Result
Table 4 shows the mixed ANOVA results with interaction and main effects. Table 5 shows means, standard deviations, repeated measures ANOVA and Friedman test results. In each class, 36.4% were male and 63.6% female. Both the high-level and the increasing group comprised 24.2% medical students in a relationship (T1). At initial measurement, 36.4% of the students in the high-level group and 50% in the increasing group lived in a shared apartment. Students of the increasing group were more diligent regarding their academic work, as 39.4% of them invested at least 40 h per week into their studies (T1) compared to the high-level group (24.2%). On the other hand, compared to the increasing group, the high-level group had an additional 5% of students, who had a side job. Furthermore, the high-level group experienced a higher workload in their part-time employment, since 45.4% had to work 10 h or more, in contrast to the increasing group (33.1%).
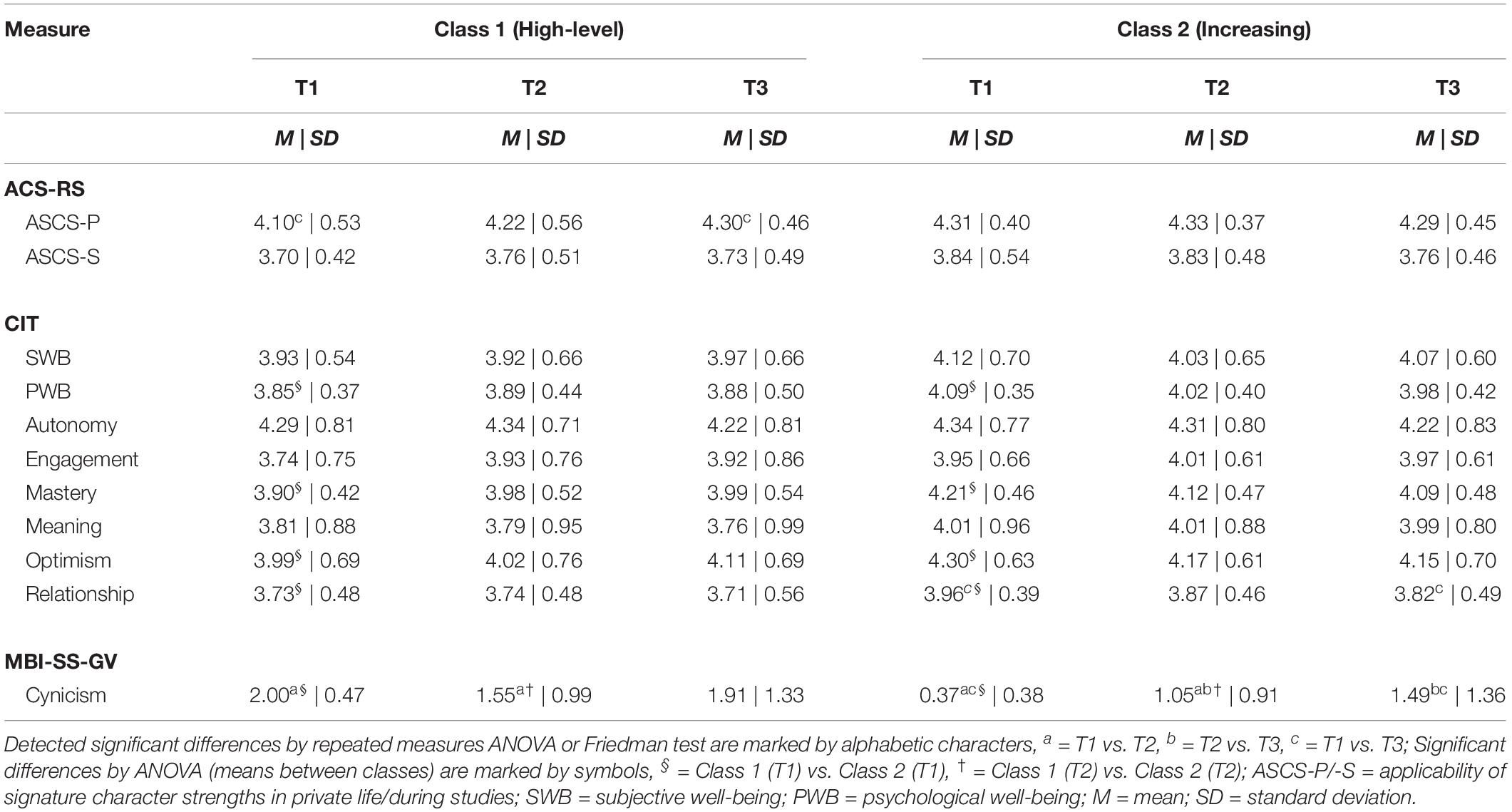
Table 5. Means, standard deviations (repeated measures) ANOVA and Friedman test results of the two classified cynicism groups.
We took a closer look at interaction and main effects between and within the high-level and the increasing group regarding cynicism. There was homogeneity of the error variances (Levene’s test; p > 0.05) and co-variances as assessed by Box’s test (p > 0.05). The Huynh–Feldt adjustment was used to correct for violations of sphericity (Mauchly’s test; p < 0.01). We found a statistically significant interaction between time and group [F(1.69, 164.11) = 18.98 p < 0.001, = 0.164), which can be seen in Figure 1. Differences between groups were significant at T1 [F(1, 97) = 339.92, p < 0.001, ω2 = 0.773] and T2 [F(1, 97) = 6.29, p = 0.014, ω2 = 0.051] with lower cynicism values in the increasing group. The cynicism score changes in the increasing group [Huynh–Feldt F(1.60, 103.78) = 42.24 p < 0.001, = 0.394] occurred at a significant level between all three measurements (Bonferroni corrected; p < 0.001) with increasing cynicism scores from the initial to the last measurement. The Bonferroni corrected post hoc analyses in the high-level group [F(2, 64) = 3.08, p = 0.049, = 0.089] were significant between T1 and T2 (p = 0.029) with decreasing scores in cynicism.
Regarding mixed ANOVA analyses for the applicability of signature character strengths outcome in private life (ASCS-P), we faced homogeneity of the error variances except for ASCS-P at T2 (Levene’s test; mean based p = 0.008). Conducting Box–Cox transformation did not yield a reasonable result (p = 0.035). Homogeneity of the co-variances (Box’s test; p = 0.004) was not present. Sphericity assumptions were not violated (Mauchly’s test; p > 0.05). Next, we analyzed differences within and between the two cynicism classes using a Friedman test and Welch tests. A Friedman test of differences among repeated measures was conducted and revealed a significant difference in ASCS-P depending on time point measured [χ2(2) = 8.99, p = 0.011] in the high-level group. Post hoc analysis was conducted and Bonferroni correction applied, resulting in significant differences between T1 and T3 (p = 0.011). The ASCS-P over the three annual measurements was stable in the increasing group. Welch tests did not report significant differences between both groups at any time point.
Concerning the testing for main effects and interaction regarding the applicability of signature character strengths during medical studies (ASCS-S), homogeneity of the error variances (Levene’s test; p > 0.05) and homogeneity of co-variances (Box’s test; p > 0.05) were assumed. Furthermore, there was no violation of sphericity (Mauchly’s test; p > 0.05). No statistically significant interaction between time and class [F(2, 194) = 0.56 p = 0.575, = 0.006] was perceived. Moreover, we found a main effect neither for time, nor for class. Although there was initially a slightly higher score in the increasing group, which decreased over the span of the three annual measurements, no statistically significant differences within and between both groups could be discovered. In general, the ASCS-P was distinctively higher than during studies in both groups and at all measurements.
Assumptions of sphericity for the mixed ANOVA of SWB had been violated (Mauchly’s test; p = 0.027). SWB showed homogeneity for the error variances (Levene’s test; p > 0.05) and homogeneity of co-variances (Box’s test; p > 0.05). Huynh–Feldt corrected results [F(1.92, 186.15) = 0.30 p = 0.731, = 0.003] did not show a significant interaction or main effect for time or class. Notwithstanding the fact that SWB in general tended to be lower in the high-level group compared with the increasing group, according to ANOVA test results, there was no significant difference within and between groups.
Mixed ANOVA testing with PWB demonstrated homogeneity of the error variances (Levene’s test; p > 0.05) and homogeneity of co-variances (Box’s test; p > 0.05). Sphericity assumptions had not been violated (Mauchly’s test; p > 0.05). Results neither revealed an interaction [F(2, 96) = 2.18 p = 0.118, = 0.022] nor main effects of class or time. Although PWB in general tended to be lower in the high-level compared with the increasing group, significant differences can only be found at T1 [F(1, 97) = 8.74, p = 0.004, ω2 = 0.073] with higher PWB values in the increasing group. Score changes of cynicism in the increasing group appeared to be significant [Huynh–Feldt F(1.83, 118.88) = 3.68 p = 0.032, = 0.054], but Bonferroni corrected post hoc analyses revealed no significant differences between time points (p > 0.05).
We further took a closer look at PWB components and conducted mixed ANOVA analyses. Autonomy, engagement, mastery, meaning, optimism and relationship showed homogeneity of the error variances (Levene’s test; p > 0.05) and assumptions of sphericity had not been violated (Mauchly’s test; p > 0.05). Heterogeneity of co-variances was present in engagement (Box’s test; p = 0.002), meaning (Box’s test; p = 0.023) and optimism (Box’s test; p = 0.034). Analyses did not show significant interactions for autonomy [F(2, 194) = 0.075 p = 0.93, = 0.001], engagement [F(2, 194) = 0.18 p = 0.398, = 0.009], mastery [F(2, 194) = 6.98, p = 0.07, = 0.027], meaning [F(2, 194) = 0.03, p = 0.975, < 0.001], optimism [F(2, 194) = 2.10 p = 0.125, = 0.02], and relationship [F(2, 194) = 1.13, p = 0.33, = 0.012]. For all components there were no main effects, except for mastery with a main effect for group [F(1, 97) = 4.55 p = 0.035, = 0.045]. Differences between high-level and increasing group were significant at T1 [F(1, 97) = 10.54, p = 0.002, ω2 = 0.088] with higher scores of mastery in the increasing group. Moreover, higher scores at T1 in the increasing group compared with the high-level group were also found for optimism [F(1, 97) = 5.06, p = 0.027, ω2 = 0.039], and relationship [F(1, 97) = 6.20, p = 0.014, ω2 = 0.05]. Autonomy, engagement, mastery, meaning and optimism were not significantly different between measurement waves within high-level and increasing group. Score differences between measurements of relationship appeared in the increasing group [F(2, 130) = 4.68, p = 0.011, = 0.067], post hoc analysis proved decreasing values between T1 and T3 (Bonferroni corrected; p = 0.02).
Discussion
This study was set out to assess how cynicism, as a component of burnout, develops in medical students from their first to their third year in medical school. Firstly, we applied latent growth modeling and saw an increase in cynicism among medical students as the training progresses. Secondly, we were interested in possible different developmental patterns of cynicism, thus applied latent class growth modeling and growth mixture modeling. Our sample was classified into a high-level group (34%) and an increasing group (66%). The high-level group experienced relatively stable heightened cynicism, whereas in the increasing group cynicism gradually developed over the three annual measurements. Thirdly, we examined the relationships between obtained classified groups with different trajectory patterns of cynicism and the applicability of signature character strengths, SWB, and PWB (autonomy, engagement, mastery, meaning, optimism, and relationship). Medical students overall reported higher scores on applicability of signature character strengths in private life than during studies over the whole measurement period. Furthermore, students in the increasing group experienced significantly higher scores of PWB, mastery, optimism, and relationship at the first measurement compared to the high-level group.
Regarding our first hypothesis, concerning the gradual development of cynicism as found in our study’s sample, one explanation could be provided by the training process and learning environment (formal, informal, and hidden curriculum) itself. It may contribute to the deterioration of mental health, quality of life (Klier, 2010; Brazeau et al., 2014) and empathy decline (Neumann et al., 2011) in medical students. According to several studies, there is evidence that these problems, continuously pervading this profession, have their origin in medical education (West et al., 2006; West and Shanafelt, 2007; Dyrbye et al., 2010). Regrettably, during medical school interpersonal skills linked to being an active member of an institution are underrepresented in curricula, compared to the heavily emphasized training of technical skills linked with being a physician (Montgomery et al., 2013). The professional identity of medical students and their perception of what includes “bad and good doctoring” (e.g., professionalism) are shaped during the time in medical school (Hafferty, 1998; Elliott et al., 2009). They experience a learning environment characterized by formal, informal, and hidden aspects, contributing significantly to this identity-shaping process (Hafler et al., 2011). While professionalism may be valued in the formal curriculum, the hidden curriculum values competitive or performance-oriented behavior over collaboration. The hidden curriculum is a huge part of the learning process, comprising e.g., customs, rituals or aspects that are taken for granted. A hidden curriculum is defined as a conglomeration of cultural influences, which are conveyed through structural or institutional levels (Hafferty, 1998). Feudtner et al. (1994) stated, there is an increase in cynicism and development of ethical erosion due to the contribution of the hidden curriculum. In this regard, Arthur et al. (2015) highlighted the importance of implementing character strengths and virtue training in the formal curricula at universities and informal training by senior staff to help resolve ethical dilemmas which physicians are faced with. Thus, the moral character of good doctors can be formed, in order to contribute to the health of patients and doctors.
Concerning the development of cynicism, one could further argue that not all freshmen at the medical university are used to growing pressure, limited support or hierarchical structures. Thus, having limited resources to deal with new and challenging circumstances may result in a growing cynical attitude toward your own studies, resulting in emotional exhaustion or spill over to other domains of life, negatively affecting them (Bergmann et al., 2019). Therefore, students are encouraged to find healthy coping strategies (e.g., personally reflection, peer support) or strong mentors to protect themselves against this negative trend (Cohen et al., 2009). Moreover, not only students are asked to maintain their own well-being, also universities should be held responsible. Since guidelines for medical schools are limited, Kemp et al. (2019) have taken up this issue and provided comprehensive recommendations for an educational program in order to promote well-being and learning in medical students. They do not only recommend addressing changes in the curricula, but further expanding on students’ selection process, learning environment and staff selection. Although they provide a wide range of supporting documents, a sound evidence base developed by longitudinal data is still needed.
According to our analysis, there are two different medical student groups regarding cynicism development. How can it be explained that one part of the students is more cynical at the beginning of their studies (high-level group) and others become cynical over time (increasing group)? As Moir et al. (2018) emphasized, traits of medical students can play an important role in deteriorating mental health. Therefore, it might be conceivable that students from the high-level group possess more of a cynicism trait-like characteristic, compared to students in the increasing group. Examining our two groups, this would mean that some students are quite stable in their cynicism values (as seen in the high-level group), whereas other students’ cynicism scores are more adaptable over time and situations. At the beginning of the second year, medical students start to gather first experiences with patients which may be leading to a divergence between expected and perceived experiences in the health care setting. The ones having preliminary lower cynical attitudes toward their studies might also have higher expectations regarding its potential usefulness in the beginning. Therefore, some students might perhaps become disillusioned as they progress through medical training. In this regard, it might be beneficial while being in the phase of occupational orientation (e.g., in schools, counseling facilities, during open days, etc.) to present contents and difficulties to be expected during medical studies, so that interested young adults get a more realistic picture of what they can expect during medical school and from first patient interactions.
Despite being a rather robust statistical method, whenever assumptions of the mixed ANOVAs were not met, they were subsequently corrected. Facing violations of sphericity assumptions and heteroscedasticity between the high-level and the increasing group were present in several variables (e.g., applicability of signature character strengths in private life). The violation of these requirements can stem from unequal group sizes or within group variation of the respective variable. If not corrected, this could lead to false positive result interpretation (Type I error). Although, corrected for violated assumptions of mixed ANOVA, it cannot be ruled out that the data may contain other information that was not discovered by our analyses.
Our findings revealed that both groups generally rated the applicability of signature character strengths in private life higher than during studies. Huber et al. (2019) reported similar results for a sample of physicians. These findings could be explained by the fact that character strengths and their application are not mentioned or trained in the medical curriculum per se. Consequently, medical students often do not know their character strengths and how they can be applied in the context of study whereas in private context they might apply them intuitively. One further explanation for this could be the standardized curriculum, which mainly consists of lectures in the preclinical years. Therefore, students may have had fewer opportunities to apply their character strengths in the specific academic context. In particular, the applicability of signature character strengths in private life in the high-level group increased from initial to the last measurement, which might be explained by the possibility that these students seek more activities in their private lives that build upon their character strengths. In addition, it is conceivable that the benefits of applying one’s signature character strengths in the private context can spill over to the study-related context, thus potentially affecting the cynical attitude, as can be seen by significantly decreasing cynicism between T1 and T2 in the high-level group. Since character strengths are related to stress coping behavior (Harzer and Ruch, 2015) and health (Proyer et al., 2014), we argue that the applicability of signature character strengths may play a role in influencing the development of cynicism, as it reflects the opportunity to deploy personal resources, thus being beneficial for the academic performance of medical students (Trucchia et al., 2013) and potentially buffering harsh and adverse influences occurring during medical training.
Apart from the applicability of signature character strengths, components of well-being may also play a vital role in the developmental process of cynicism. Our results showed optimism to be significantly higher at the initial measurement in the increasing group compared to the high-level group. Optimism may play a role in developing cynicism at the time of entering medical school, which would be in line with Chang et al. (2000), who found optimism to be negatively related to cynicism. Concerning the PWB components mastery and relationship, one could argue that students with higher values are less cynical. This would be comparable to results by Bentea (2017), who found teachers with lower levels of mastery and relationship having higher levels of cynicism. Overall, well-being proved to be significantly different between both groups at initial measurement. Training well-being of medical students is important as it is directly related to better academic performance (Trucchia et al., 2013), reduced stress levels (Rosenzweig et al., 2003), empathy (Thomas et al., 2007), resilience and patient care (Dunn et al., 2008). Our results underscore the importance of early interventions that may contribute to students’ well-being to prevent a continuing decline or failing increase.
Limitations and Future Research
Finally, a number of important limitations need to be considered:
Since our longitudinal study was explorative, it cannot provide causal relations between observed variables. Growth mixture modeling has become of increasing interest to researchers in psychology in recent years. GMM is in several regards a useful exploratory procedure (e.g., for identifying unobserved subpopulations), but it has its constraints. In general, mixture models account for non-normality in observed data. Non-normality may occur due to poor measurement, mixed groups of individuals or true non-normally distributed data. Therefore, finding false unobserved groups by misinterpreting GMM results is a real danger (Ram and Grimm, 2009). Although usually large samples are recommended for SEM and GMM, there is no general rule regarding the necessary minimum sample size (Ram and Grimm, 2009). The required sample size depends on many factors (e.g., number of latent classes, model size, distribution and reliability of the variables), but using small sample sizes in growth models may result in the reduction of power (Muthén and Muthén, 2002). Furthermore, the relatively small sample size can lead to attrition biased data interpretation, which is a common problem in longitudinal research (e.g., Goodman, 1996). This selection process can often result in reduced variance (Pearson, 1903) and therefore, may lead to a biased (e.g., homogenous) sample or might limit the generalizability of this data set. For example, participants who continued with all three annual measurements may have other characteristics (e.g., persistence) compared to people who dropped out earlier (e.g., questionnaire was too long for them, reward not satisfying enough, etc.). Moreover, the participants in our sample were chosen from one medical university only. These problems (e.g., misinterpretation, and loss of power) can be addressed by replicating results with new data. Thus, future studies should investigate larger samples of medical students at other universities in order to validate the results obtained in our study. Future research might also take universities from different countries and cultural backgrounds into account with large, multi-centered studies including students from other subjects in order to obtain generalizable results. Since we did not measure the influence of the formal, informal or hidden curriculum on the development of cynicism, studies are necessary that allow their examination. Moreover, studies should identify other personal and training-related features influencing cynicism.
We further measured the applicability of character strengths, based on each individual’s top five character strengths. The top five character strengths differed from student to student and are spread amongst the six virtues. It is conceivable that this approach has influenced our results. In a similar medical sample, Hausler et al. (2017b) found stronger relations between happiness-related strengths (hope, zest, gratitude, curiosity, and love) and components of well-being than with other character strengths. Thus, medical students in our sample who shared this happiness-related strengths profile may generally experience higher levels of well-being and lower levels of cynicism. It might be possible that participants with signature character strengths originating from different virtues be distinct from those who have a strengths profile from one single virtue. Further, it would be interesting to see if students fitting the character strengths profile required in the context of medical studies have different health-related outcomes compared with students who do not match this profile. Therefore, future studies should take up this idea to facilitate a better understanding of the underlying mechanisms. Merritt et al. (2018) found that the daily variation of the application of signature character strengths is linked to daily strain and daily job satisfaction, whereas global strength use was associated with global strain and global job satisfaction. This may also relate to medical students. In this context, it would be useful to assess intervention programs regarding their effectivity to help students to identify and apply their signature character strengths. Furthermore, implementing well-being curricula for medical students may be reasonable, since improved well-being of physicians has positive effects on reflective practice, empathy and communication skills (Neumann et al., 2011). Focusing on strengths interventions, Niemiec (2014) provides an extensive strategy in implementing mindfulness-based strength practice in the training of physicians.
Conclusion
In conclusion, our study has the following implications:
This explorative study has shown that medical students in Austria experience an increase of cynicism during their 3-year preclinical education in medical school. Two different patterns of cynicism development were identified: high-level vs. increasing. Data showed that students from both groups perceived lower applicability of signature character strengths, in particular during studies. Moreover, students allocated to the group with continuously higher levels of cynicism reported lower PWB (mastery, optimism, and relationship). Broad strategies for identifying character strengths and implementing well-being as well as character trainings into curricula and workplace culture may be a beneficial approach, in order to avoid early onset of burnout-related symptoms. These strategies should consider characteristics of the targeted individuals/workplaces and need to be empirically tested. This research project expands on the knowledge of the developmental process of cynicism as a burnout burnout-related symptom in medical students and its relations to the applicability of signature character strengths and well-being.
Data Availability Statement
The datasets generated for this study are available on request to the corresponding author.
Ethics Statement
This study was carried out in accordance with the recommendations of the Board for Ethical Questions in Science of the University of Innsbruck with written informed consent from all subjects. All subjects gave written informed consent in accordance with the Declaration of Helsinki. The protocol was approved by the Board for Ethical Questions in Science of the University of Innsbruck.
Author Contributions
AH, CS, TH, and SH were substantially involved in the planning and conducting of the study. TK carried out the data analysis and the drafting of the manuscript. All authors revised the manuscript critically for important intellectual content, and read and approved the accepted version.
Funding
This research was funded by the Austrian Science Fund (FWF) under project number P27228-G22 (Principal Investigator SH and Co-Principal Investigator TH). TK received a Ph.D.-research grant (2018/Psy/Sport-10) awarded by the University of Innsbruck.
Conflict of Interest
The authors declare that the research was conducted in the absence of any commercial or financial relationships that could be construed as a potential conflict of interest.
Acknowledgments
We express our gratitude to Mirjam Brenner, MSc, for her comprehensive support concerning data administration and processing.
References
Allan, B. A., and Duffy, R. D. (2014). Examining moderators of signature strengths use and well-being: calling and Signature Strengths Level. J. Happiness Stud. 15, 323–337. doi: 10.1007/s10902-013-9424-0
Arthur, J., Kristjánsson, K., Thomas, H., Kotzee, B., Ignatowicz, A., and Qiu, T. (2015). Virtuous Medical Practice: Research Report. Birmingham: University of Birmingham.
Bakker, A. B., and Demerouti, E. (2007). The job demands-resources model: state of the art. J. Manage. Psychol. 22, 309–328. doi: 10.1108/02683940710733115
Bandura, A. (2000). “Cultivate self-efficacy for personal and organizational effectiveness,” in Handbook of Principles of Organization Behavior, ed. E. A. Locke (Oxford: Blackwell), 120–136.
Bentea, C. (2017). Teacher self-efficacy, teacher burnout and psychological well-being. Paper Presented at the 7th International Conference of the Educational World, Pitesti.
Bergmann, C., Muth, T., and Loerbroks, A. (2019). Medical students’ perceptions of stress due to academic studies and its interrelationships with other domains of life: a qualitative study. Med. Educ. Online 24:1603526. doi: 10.1080/10872981.2019.1603526
Brazeau, C. M. L. R., Shanafelt, T. D., Durning, S. J., Massie, F. S., Eacker, A., Moutier, C., et al. (2014). Distress among matriculating medical students relative to the general population. Acad. Med. 89, 1520–1525. doi: 10.1097/ACM.0000000000000482
Bresó, E., Salanova, M., and Schaufeli, W. B. (2007). In search of the “Third Dimension” of burnout: efficacy or inefficacy? Appl. Psychol. 56, 460–478. doi: 10.1111/j.1464-0597.2007.00290.x
Brown, R., Dunn, S., Byrnes, K., Morris, R., Heinrich, P., and Shaw, J. (2009). Doctors’ stress responses and poor communication performance in simulated bad-news consultations. Acad. Med. 84, 1595–1602. doi: 10.1097/ACM.0b013e3181baf537
Chang, E. C., Rand, K. L., and Strunk, D. R. (2000). Optimism and risk for job burnout among working college students: stress as a mediator. Pers. Individ. Dif. 29, 255–263. doi: 10.1016/s0191-8869(99)00191-9
Cohen, M. J. M., Kay, A., Youakim, J. M., Balaicuis, J. M., and Balacius, J. M. (2009). Identity transformation in medical students. Am. J. Psychoanal. 69, 43–52. doi: 10.1057/ajp.2008.38
Collier, V. U., McCue, J. D., Markus, A., and Smith, L. (2002). Stress in medical residency: status quo after a decade of reform? Ann. Intern. Med. 136, 384–390. doi: 10.7326/0003-4819-136-5-200203050-00011
Crandall, S. J., Volk, R. J., and Loemker, V. (1993). Medical students’ attitudes toward providing care for the underserved. Are we training socially responsible physicians? JAMA 269, 2519–2523. doi: 10.1001/jama.1993.03500190063036
Curran, P. J., Obeidat, K., and Losardo, D. (2010). Twelve frequently asked questions about growth curve modeling. J. Cogn. Dev. 11, 121–136. doi: 10.1080/15248371003699969
Dahlin, M. E., Joneborg, N., and Runeson, B. (2005). Stress and depression among medical students: a cross-sectional study. Med. Educ. 29, 594–604. doi: 10.1111/j.1365-2929.2005.02176.x
Dahlin, M. E., and Runeson, B. (2007). Burnout and psychiatric morbidity among medical students entering clinical training: a three year prospective questionnaire and interview-based study. BMC Med. Educ. 7:6. doi: 10.1186/1472-6920-7-6
Dunn, L. B., Iglewicz, A., and Moutier, C. (2008). A conceptual model of medical student well-being: promoting resilience and preventing burnout. Acad. Psychiatry 32, 44–53. doi: 10.1176/appi.ap.32.1.44
Dyrbye, L. N., Massie, F. S.Jr., Eacker, A., Harper, W., Power, D., Durning, S. J., et al. (2010). Relationship between burnout and professional conduct and attitudes among US medical students. JAMA 304, 1173–1180. doi: 10.1001/jama.2010.1318
Dyrbye, L., and Shanafelt, T. (2016). A narrative review on burnout experienced by medical students and residents. Med. Educ. 50, 132–149. doi: 10.1111/medu.12927
Dyrbye, L. N., Thomas, M. R., Massie, F. S., Power, D. V., Eacker, A., Harper, W., et al. (2008). Burnout and suicidal ideation among U.S. medical students. Ann. Intern. Med. 149, 334–341. doi: 10.7326/0003-4819-149-5-200809020-00008
Dyrbye, L. N., Thomas, M. R., and Shanafelt, T. D. (2005). Medical student distress: causes, consequences, and proposed solutions. Mayo Clin. Proc. 80, 1613–1622. doi: 10.4065/80.12.1613
Dyrbye, L. N., West, C. P., Satele, D., Boone, S. L., Tan, L., Sloan, J., et al. (2014). Burnout among U.S. medical students, residents, and early career physicians relative to the general U.S. population. Acad. Med. 89, 443–451. doi: 10.1097/ACM.0000000000000134
Elliott, D. D., May, W., Schaff, P. B., Nyquist, J. G., Trial, J., Reilly, J. M., et al. (2009). Shaping professionalism in pre-clinical medical students: professionalism and the practice of medicine. Med. Teach. 31, e295–e302. doi: 10.1080/01421590902803088
Feudtner, C., Christakis, D. A., and Christakis, N. A. (1994). Do clinical clerks suffer ethical erosion? Students’ perceptions of their ethical environment and personal development. Acad. Med. 69, 670–679. doi: 10.1097/00001888-199408000-00017
Frajerman, A., Morvan, Y., Krebs, M.-O., Gorwood, P., and Chaumette, B. (2018). Burnout in medical students before residency: a systematic review and meta-analysis. Eur. Psychiatry 55, 36–42. doi: 10.1016/j.eurpsy.2018.08.006
Frankfurt, S., Frazier, P., Syed, M., and Jung, K. R. (2016). Using group-based trajectory and growth mixture modeling to identify classes of change trajectories. Couns. Psychol. 44, 622–660. doi: 10.1177/0011000016658097
Goodman, J. (1996). Assessing the non-random sampling effects of subject attrition in longitudinal research. J. Manage. 22, 627–652. doi: 10.1016/s0149-2063(96)90027-6
Gumz, A., Erices, R., Brähler, E., and Zenger, M. (2013). Faktorstruktur und Gütekriterien der deutschen Übersetzung des Maslach-Burnout-Inventars für Studierende von Schaufeli et al. (MBI-SS) [Factorial structure and psychometric criteria of the German translation of the Maslach Burnout Inventory–Student Version by Schaufeli et al. (MBI-SS)]. Psychother. Psychosom. Med. Psychol. 63, 77–84. doi: 10.1055/s-0032-1323695
Gusy, B., Wörfel, F., and Lohnmann, K. (2016). Erschöpfung und Engagement im Studium: eine Anwendung des Job Demands-Resources Modells. Z. Gesundheitspsychol. 24, 41–53. doi: 10.1026/0943-8149/a000153
Hafferty, F. W. (1998). Beyond curriculum reform: confronting medicine’s hidden curriculum. Acad. Med. 73, 403–407. doi: 10.1097/00001888-199804000-00013
Hafler, J. P., Ownby, A. R., Thompson, B. M., Fasser, C. E., Grigsby, K., Haidet, P., et al. (2011). Decoding the learning environment of medical education: a hidden curriculum perspective for faculty development. Acad. Med. 86, 440–444. doi: 10.1097/ACM.0b013e31820df8e2
Halbesleben, J. R. B., and Rathert, C. (2008). Linking physician burnout and patient outcomes: exploring the dyadic relationship between physicians and patients. Health Care Manage. Rev. 33, 29–39. doi: 10.1097/01.HMR.0000304493.87898.72
Hamilton, J., Gagne, P. E., and Hancock, G. R. (2003). The effect of sample size on latent growth models. Paper Presented at the Annual Meeting of the American Educational Research Association, Chicago, IL.
Hanks, R. A., Rapport, L. J., Waldron-Perrine, B., and Millis, S. R. (2014). Role of character strengths in outcome after mild complicated to severe traumatic brain injury: a positive psychology study. Arch. Phys. Med. Rehabil. 95, 2096–2102. doi: 10.1016/j.apmr.2014.06.017
Harzer, C., and Ruch, W. (2012). When the job is a calling: the role of applying one’s signature strengths at work. J. Posit. Psychol. 7, 362–371. doi: 10.1080/17439760.2012.702784
Harzer, C., and Ruch, W. (2013). The application of signature character strengths and positive experiences at work. J. Happiness Stud. 14, 965–983. doi: 10.1007/s10902-012-9364-0
Harzer, C., and Ruch, W. (2015). The relationships of character strengths with coping, work-related stress, and job satisfaction. Front. Psychol. 6:165. doi: 10.3389/fpsyg.2015.00165
Hausler, M., Huber, A., Strecker, C., Brenner, M., Höge, T., and Höfer, S. (2017). Validierung eines Fragebogens zur umfassenden Operationalisierung von Wohlbefinden - Die deutsche Version des Comprehensive Inventory of Thriving (CIT) und die Kurzversion Brief Inventory of Thriving (BIT). Diagnostica 63, 219–228. doi: 10.1026/0012-1924/a000174
Hausler, M., Strecker, C., Huber, A., Brenner, M., Höge, T., and Höfer, S. (2017a). Associations between the application of signature character strengths, health and well-being of health professionals. Front. Psychol. 8:1307. doi: 10.3389/fpsyg.2017.01307
Hausler, M., Strecker, C., Huber, A., Brenner, M., Höge, T., and Höfer, S. (2017b). Distinguishing relational aspects of character strengths with subjective and psychological well-being. Front. Psychol. 8:1159. doi: 10.3389/fpsyg.2017.01159
Heinen, I., Bullinger, M., and Kocalevent, R.-D. (2017). Perceived stress in first year medical students - associations with personal resources and emotional distress. BMC Med. Educ. 17:4. doi: 10.1186/s12909-016-0841-8
Henson, J. M., Reise, S. P., and Kim, K. H. (2007). Detecting mixtures from structural model differences using latent variable mixture modeling: a comparison of relative model fit statistics. Struct. Equ. Model. 14, 202–226. doi: 10.1080/10705510709336744
Hobfoll, S. E., Johnson, R. J., Ennis, N., and Jackson, A. P. (2003). Resource loss, resource gain, and emotional outcomes among inner city women. J. Pers. Soc. Psychol. 84, 632–643. doi: 10.1037/0022-3514.84.3.632
Höfer, S., Hausler, M., Huber, A., Strecker, C., Renn, D., and Höge, T. (2019). Psychometric characteristics of the german values in action inventory of strengths 120-item short form. Appl. Res. Qual. Life 29:254. doi: 10.1007/s11482-018-9696-y
Höge, T., Strecker, C., Hausler, M., Huber, A., and Höfer, S. (2019). Perceived socio-moral climate and the applicability of signature character strengths at work: a study among hospital physicians. Appl. Res. Qual. Life. doi: 10.1007/s11482-018-9697-x
Hojat, M., Vergare, M. J., Maxwell, K., Brainard, G., Herrine, S. K., Isenberg, G. A., et al. (2009). The devil is in the third year: a longitudinal study of erosion of empathy in medical school. Acad. Med. 84, 1182–1191. doi: 10.1097/ACM.0b013e3181b17e55
Huber, A., Strecker, C., Hausler, M., Kachel, T., Höge, T., and Höfer, S. (2019). Possession and applicability of signature character strengths: what is essential for well-being, work engagement, and burnout? Appl. Res. Qual. Life 15:323. doi: 10.1007/s11482-018-9699-8
IBM Corporation, (2016). Released, IBM SPSS Statistics for Windows, Version 24.0. Armonk, NY: IBM Corp.
Jackson, E. R., Shanafelt, T. D., Hasan, O., Satele, D. V., and Dyrbye, L. N. (2016). Burnout and alcohol abuse/dependence among U.S, medical students. Acad. Med. 91, 1251–1256. doi: 10.1097/ACM.0000000000001138
Jiang, Z., and Jiang, X. (2015). Core self-evaluation and life satisfaction: the person-environment fit perspective. Pers. Individ. Dif. 75, 68–73. doi: 10.1016/j.paid.2014.11.013
Jung, T., and Wickrama, K. A. S. (2008). An introduction to latent class growth analysis and growth mixture modeling. Soc. Pers. Psychol. Compass 2, 302–317. doi: 10.1111/j.1751-9004.2007.00054.x
Kemp, S., Hu, W., Bishop, J., Forrest, K., Hudson, J. N., Wilson, I., et al. (2019). Medical student wellbeing - a consensus statement from Australia and New Zealand. BMC Med. Educ. 19:69. doi: 10.1186/s12909-019-1505-2
Klier, S. (2010). Lebensqualität von Medizinstudenten: Eine Studie zur Arbeitsbelastung, Gesundheit und der Bedeutung partnerschaftlicher Beziehungen im Medizinstudium (Dissertation). Gieβen: Justust-Liebig-University. Available online at: https://d-nb.info/1002871603/34
Kopelman, L. (1983). Cynicism among medical students. JAMA 250, 2006–2010. doi: 10.1001/jama.1983.03340150048025
Kosik, R. O., Nguyen, T., Ko, I., and Fan, A. P. (2017). Suicidal ideation in medical students. Neuropsychiatry 7, 9–11. doi: 10.4172/Neuropsychiatry.1000172
Leiter, M. P., Hakanen, J. J., Ahola, K., Toppinen-Tanner, S., Koskinen, A., and Väänänen, A. (2012). Organizational predictors and health consequences of changes in burnout: a 12-year cohort study. J. Org. Behav. 34, 959–973. doi: 10.1002/job.1830
Leiter, M. P., and Maslach, C. (2009). Nurse turnover: the mediating role of burnout. J. Nurs. Manage. 17, 331–339. doi: 10.1111/j.1365-2834.2009.01004.x
Leiter, M. P., and Maslach, C. (2016). Latent burnout profiles: a new approach to understanding the burnout experience. Burn. Res. 3, 89–100. doi: 10.1016/j.burn.2016.09.001
Leontopoulou, S., and Triliva, S. (2012). Explorations of subjective wellbeing and character strengths among a Greek University student sample. Int. J. Wellbeing 2, 251–270. doi: 10.5502/ijw.v2.i3.6
Lo, Y., Mendell, N. R., and Rubin, D. B. (2001). Testing the number of components in a normal mixture. Biometrika 88, 767–778. doi: 10.1093/biomet/88.3.767
Mäkikangas, A., and Kinnunen, U. (2016). The person-oriented approach to burnout: a systematic review. Burn. Res. 3, 11–23. doi: 10.1016/j.burn.2015.12.002
Maslach, C., and Leiter, M. P. (2016). Understanding the burnout experience: recent research and its implications for psychiatry. World Psychiatry 15, 103–111. doi: 10.1002/wps.20311
McManus, I. C., Winder, B. C., and Gordon, D. (2002). The causal links between stress and burnout in a longitudinal study of UK doctors. Lancet 359, 2089–2090. doi: 10.1016/S0140-6736(02)08915-8
Merritt, S., Huber, K., and Bartkoski, T. (2018). Application of signature strengths at work: a dual-level analysis. J. Posit. Psychol. 14, 113–124. doi: 10.1080/17439760.2018.1519589
Moir, F., Yielder, J., Sanson, J., and Chen, Y. (2018). Depression in medical students: current insights. Adv. Med. Educ. Pract. 9, 323–333. doi: 10.2147/AMEP.S137384
Montgomery, A., Todorova, I., Baban, A., and Panagopoulou, E. (2013). Improving quality and safety in the hospital: the link between organizational culture, burnout, and quality of care. Br. J. Health Psychol. 18, 656–662. doi: 10.1111/bjhp.12045
Morley, C. P., Roseamelia, C., Smith, J. A., and Villarreal, A. L. (2013). Decline of medical student idealism in the first and second year of medical school: a survey of pre-clinical medical students at one institution. Med. Educ. Online 18:21194. doi: 10.3402/meo.v18i0.21194
Muthén, B. (2004). “Latent variable analysis: growth mixture modeling and related techniques for longitudinal data,” in The SAGE Handbook of Quantitative Methodology for the Social Sciences, ed. D. Kaplan (Thousand Oaks, CA: SAGE Publications), 346–369. doi: 10.4135/9781412986311.n19
Muthén, L. K., and Muthén, B. O. (2002). How to use a Monte Carlo study to decide on sample size and determine power. Struct. Equ. Model. 9, 599–620. doi: 10.1207/S15328007SEM0904_8
Muthén, L. K., and Muthén, B. O. (1998–2018). Mplus User’s Guide, 8th Edn. Los Angeles, CA: Muthén & Muthén.
Nagin, D. S., and Odgers, C. L. (2010). Group-based trajectory modeling in clinical research. Annu. Rev. Clin. Psychol. 6, 109–138. doi: 10.1146/annurev.clinpsy.121208.131413
Nelemans, S. A., Hale, W. W., Branje, S. J. T., van Lier, P. A. C., Jansen, L. M. C., Platje, E., et al. (2014). Persistent heightened cortisol awakening response and adolescent internalizing symptoms: a 3-year longitudinal community study. J. Abnorm. Child Psychol. 42, 767–777. doi: 10.1007/s10802-013-9820-2
Neumann, M., Edelhäuser, F., Tauschel, D., Fischer, M. R., Wirtz, M., Woopen, C., et al. (2011). Empathy decline and its reasons: a systematic review of studies with medical students and residents. Acad. Med. 86, 996–1009. doi: 10.1097/ACM.0b013e318221e615
Niemiec, R. M. (2014). “Mindfulness-based strengths practice (MBSP) for physicians: integrating core areas to promote positive health,” in Positive Health: Flourishing Lives, Well-Being in Doctors, ed. M. W. Snyder (Bloomington, IN: Balboa Press), 247–263.
Nylund, K. L., Asparouhov, T., and Muthén, B. O. (2007). Deciding on the number of classes in latent class analysis and growth mixture modeling: a Monte Carlo simulation study. Struct. Equ. Model. 14, 535–569. doi: 10.1080/10705510701575396
Osborne, J. W., and Overbay, A. (2004). The power of outliers (and researchers should ALWAYS check for them). Pract. Assess. Res. Eval. 9, 1–12. doi: 10.1016/j.compbiomed.2014.05.010
Pagnin, D., de Queiroz, V., de Oliveira Filho, M. A., Gonzalez, N. V. A., Salgado, A. E. T., Cordeiro e Oliveira, B., et al. (2013). Burnout and career choice motivation in medical students. Med. Teach. 35, 388–394. doi: 10.3109/0142159X.2013.769673
Pearson, K. (1903). Mathematical contributions to the theory of evolution: II, On the influence of natural selection on the variability and correlation of organs. R. Soc. Philos. Trans. 200, 1–66.
Peng, J., Clarkin, C., and Doja, A. (2018). Uncovering cynicism in medical training: a qualitative analysis of medical online discussion forums. BMJ Open 8:e022883. doi: 10.1136/bmjopen-2018-022883
Peterson, C., and Park, N. (2011). “Character strengths and virtues: Their role in well-being,” in Applied Positive Psychology: Improving Everyday Life, Health, Schools, Work, and Society, eds S. I. Donaldson, M. Csikszentmihalyi, and J. Nakamura (New York, NY: Psychology Press), 49–62.
Peterson, C., Park, N., and Seligman, M. E. P. (2005). “Assessment of character strengths,” in Psychologists’ Desk Reference, 2nd Edn, eds G. P. Koocher, J. C. Norcross, and S. S. Hill (Oxford: Oxford University Press), 93–98.
Peterson, C., and Seligman, M. E. P. (2004). Character Strengths and Virtues: A Handbook and Classification. Washington, DC: American Psychological Association.
Prinz, P., Hertrich, K., Hirschfelder, U., and de Zwaan, M. (2012). Burnout, depression and depersonalisation - Psychological factors and coping strategies in dental and medical students. GMS J. Med. Educ. 29:Doc10. doi: 10.3205/zma000780
Proyer, R. T., Wellenzohn, S., Gander, F., and Ruch, W. (2014). Toward a better understanding of what makes positive psychology interventions work: predicting happiness and depression from the person × intervention fit in a follow-up after 3.5 years. Appl. Psychol. Health and Well Being 7, 108–128. doi: 10.1111/aphw.12039
Ram, N., and Grimm, K. J. (2009). Growth mixture modeling: a method for identifying differences in longitudinal change among unobserved groups. Int. J. Behav. Dev. 33, 565–576. doi: 10.1177/0165025409343765
Reinecke, J. (2006). Longitudinal analysis of adolescents’, Deviant and Delinquent Behavior. Methodology 2, 100–112. doi: 10.1027/1614-2241.2.3.100
Robins, T. G., Roberts, R. M., and Sarris, A. (2017). The role of student burnout in predicting future burnout: exploring the transition from university to the workplace. J. High. Educ. Res. Dev. 37, 115–130. doi: 10.1080/07294360.2017.1344827
Rosenzweig, S., Reibel, D. K., Greeson, J. M., Brainard, G. C., and Hojat, M. (2003). Mindfulness-based stress reduction lowers psychological distress in medical students. Teach. Learn. Med. 15, 88–92. doi: 10.1207/S15328015TLM1502_03
Rotenstein, L. S., Ramos, M. A., Torre, M., Segal, J. B., Peluso, M. J., Guille, C., et al. (2016). Prevalence of depression, depressive symptoms, and suicidal ideation among medical students: a systematic review and meta-analysis. JAMA 316, 2214–2236. doi: 10.1001/jama.2016.17324
Satorra, A., and Bentler, P. M. (2001). A scaled difference chi-square test statistic for moment structure analysis. Psychometrika 66, 507–514. doi: 10.1007/BF02296192
Schaufeli, W. B., and Bakker, A. B. (2004). Job demands, job resources, and their relationship with burnout and engagement: a multi-sample study. J. Org. Behav. 25, 293–315. doi: 10.1002/job.248
Schaufeli, W. B., Martínez, I. M., Pinto, A. M., Salanova, M., and Bakker, A. B. (2002a). Burnout and engagement in university students: a cross-national study. J. Cross Cult. Psychol. 33, 464–481. doi: 10.1177/0022022102033005003
Schaufeli, W. B., and Salanova, M. (2007). Efficacy or inefficacy, that’s the question: burnout and work engagement, and their relationships with efficacy beliefs. Anxiety Stress Coping 20, 177–196. doi: 10.1080/10615800701217878
Schaufeli, W. B., Salanova, M., González-Romá, V., and Bakker, A. B. (2002b). The measurement of engagement and burnout: a two sample confimatory factor analytic approach. J. Happiness Stud. 3, 71–92. doi: 10.1023/A:1015630930326
Schwarz, G. (1978). Estimating the dimension of a model. Ann. Stat. 6, 416–464. doi: 10.1214/aos/1176344136
Seligman, M. E. P. (2011). Flourish: A Visionary New Understanding of Happiness and Well-Being. New York, NY: Free Press.
Seligman, M. E. P., and Csikszentmihalyi, M. (2000). Positive psychology: an introduction. Am. Psychol. 55, 5–14. doi: 10.1037//0003-066X.55.1.5
Seligman, M. E. P., Steen, T. A., Park, N., and Peterson, C. (2005). Positive psychology progress: empirical validation of interventions. Am. Psychol. 60, 410–421. doi: 10.1037/0003-066X.60.5.410
Seoane, L., Tompkins, L. M., de Conciliis, A., and Boysen, P. G. (2016). Virtues education in medical school: the foundation for professional formation. Ochsner J. 16, 50–55.
Smith, J. K., and Weaver, D. B. (2006). Capturing medical students’ idealism. Ann. Fam. Med. 4, 32–60. doi: 10.1370/afm.543
Strecker, C., Huber, A., Höge, T., Hausler, M., and Höfer, S. (2019). Identifying thriving workplaces in hospitals: Work characteristics and the applicability of character strengths at work. Appl. Res. Qual. Life
Su, R., Tay, L., and Diener, E. (2014). The Development and Validation of the Comprehensive Inventory of Thriving (CIT) and the Brief Inventory of Thriving (BIT). Appl. Psychol. Health Well Being 6, 251–279. doi: 10.1111/aphw.12027
Tabachnick, B. G., and Fidell, L. S. (2007). Using Multivariate Statistics, 5th Edn. Boston, MA: Allyn and Bacon.
Thomas, M. R., Dyrbye, L. N., Huntington, J. L., Lawson, K. L., Novotny, P. J., Sloan, J. A., et al. (2007). How do distress and well-being relate to medical student empathy? A multicenter study. J. Gen. Intern. Med. 22, 177–183. doi: 10.1007/s11606-006-0039-6
Trucchia, S. M., Lucchese, M. S., Enders, J. E., and Fernández, A. R. (2013). Relationship between academic performance, psychological well-being, and coping strategies in medical students. Rev. Fac. Cien. Med. 70, 144–152.
Viljoen, M., and Claassen, N. (2017). Cynicism as subscale of burnout. Work 56, 499–503. doi: 10.3233/WOR-172518
Vuong, Q. (1989). Likelihood ratio tests for model selection and non-nested hypotheses. Econometrica 57, 307–333. doi: 10.2307/1912557
West, C. P., Huschka, M. M., Novotny, P. J., Sloan, J. A., Kolars, J. C., Habermann, T. M., et al. (2006). Association of perceived medical errors with resident distress and empathy: a prospective longitudinal study. JAMA 296, 1071–1078. doi: 10.1001/jama.296.9.1071
West, C. P., and Shanafelt, T. D. (2007). The influence of personal and environmental factors on professionalism in medical education. BMC Med. Educ. 7:29. doi: 10.1186/1472-6920-7-29
Wolf, T. M., Balson, P. M., Faucett, J. M., and Randall, H. M. (1989). A retrospective study of attitude change during medical education. Med. Educ. 23, 19–23. doi: 10.1111/j.1365-2923.1989.tb00807.x
Keywords: signature character strengths, burnout, cynicism, medical students, well-being, latent growth modeling (LGM), latent class growth analysis (LCGA), growth mixture modeling (GMM)
Citation: Kachel T, Huber A, Strecker C, Höge T and Höfer S (2020) Development of Cynicism in Medical Students: Exploring the Role of Signature Character Strengths and Well-Being. Front. Psychol. 11:328. doi: 10.3389/fpsyg.2020.00328
Received: 28 August 2019; Accepted: 11 February 2020;
Published: 27 February 2020.
Edited by:
Katharina Naswall, University of Canterbury, New ZealandReviewed by:
Jennifer Hoi Ki Wong, University of Canterbury, New ZealandLi Lin, The University of Oklahoma, United States
Copyright © 2020 Kachel, Huber, Strecker, Höge and Höfer. This is an open-access article distributed under the terms of the Creative Commons Attribution License (CC BY). The use, distribution or reproduction in other forums is permitted, provided the original author(s) and the copyright owner(s) are credited and that the original publication in this journal is cited, in accordance with accepted academic practice. No use, distribution or reproduction is permitted which does not comply with these terms.
*Correspondence: Stefan Höfer, stefan.hoefer@i-med.ac.at