- 1Department of Medical Psychology, Air Force Medical University, Xi’an, China
- 2Department of Psychology, The Ohio State University, Columbus, OH, United States
- 3School of Nursing, Yueyang Vocational Technical College, Yueyang, China
- 4Department of Nursing, Air Force Medical University, Xi’an, China
- 5Department of Psychology, School of Education Science, Huaiyin Normal University, Huai’an, China
- 6School of Arts and Design, Zhengzhou University of Light Industry, Zhengzhou, China
- 7Department of Psychology, Hebei Normal University, Shijiazhuang, China
- 8Department of Systems Neuroscience, Universitätsklinikum Hamburg-Eppendorf, Hamburg, Germany
It is well known that extensive practice of a perceptual task can improve visual performance, termed perceptual learning. The goal of the present study was to evaluate the dependency of visual improvements on the features of training stimuli (i.e., spatial frequency). Twenty-eight observers were divided into training and control groups. Visual acuity (VA) and contrast sensitivity function (CSF) were measured and compared before and after training. All observers in the training group were trained in a monocular grating detection task near their individual cutoff spatial frequencies. The results showed that perceptual learning induced significant visual improvement, which was dependent on the cutoff spatial frequency, with a greater improvement magnitude and transfer of perceptual learning observed for those trained with higher spatial frequencies. However, VA significantly improved following training but was not related to the cutoff spatial frequency. The results may broaden the understanding of the nature of the learning rule and the neural plasticity of different cortical areas.
Introduction
During the last few decades, perceptual learning has been found to improve visual performance from simple visual feature discrimination to complex object recognition, such as contrast (Yu et al., 2004; Moret et al., 2018; Zhang et al., 2018), stimulus orientation (Wang et al., 2010; Jehee et al., 2012), motion (Ball and Sekuler, 1987; Larcombe et al., 2018), stereoacuity (Ding and Levi, 2011; Xi et al., 2014), vernier acuity (Fahle and Edelman, 1993; Hung and Seitz, 2014), shape (Gilbert and Sigman, 2000; Gilbert et al., 2009), and facial recognition (Gold et al., 1999). Previous studies have shown that the improvement in learning is highly specific to the characteristics of the trained task or stimulus (Fahle and Morgan, 1996; Li et al., 2004; Huang et al., 2007) or even to the trained eye or retinal location (Karni and Sagi, 1991). However, an increasing number of studies have identified the generalization of learning to other stimuli, tasks, and contexts (Jeter et al., 2010; McGovern et al., 2012). Task difficulty (Ahissar and Hochstein, 1997; Liu, 1999) or precision (Jeter et al., 2009) of the training and/or transfer tasks, training protocol (Xiao et al., 2008; Hung and Seitz, 2014; Huang et al., 2017), and the brain stimulation (Contemori et al., 2019) were found to influence the degree of transfer or specificity. This transference substantially broadens the value of the implications of perceptual learning.
Notably, perceptual learning has been found to have great potential to improve visual functions such as visual acuity (VA) and contrast sensitivity (CS) in individuals with amblyopia (Chung et al., 2006; Zhou et al., 2006; Campana et al., 2014; Barollo et al., 2017), myopia (Tan and Fong, 2008; Camilleri et al., 2014; Casco et al., 2014), presbyopia (Polat et al., 2012; Sterkin et al., 2018), central or peripheral vision loss (Casco et al., 2018; Zhang et al., 2009; Chung, 2011; Maniglia et al., 2016), dyslexia (Gori and Facoetti, 2014), and even normal vision (Deveau et al., 2014). For example, Huang et al. (2008) trained observers with amblyopia or normal vision in a grating detection task near each individual’s cutoff spatial frequency. For the amblyopic observers, training significantly improved VA in the amblyopic eye (2.7 dB). No significant VA improvement was observed in normal observers. Additionally, for amblyopic and normal observers, training at the trained spatial frequency induced significant improvements in CS by 10.7 and 5.6 dB, respectively. Therefore, perceptual learning has begun to be considered a non-invasive rehabilitation method for various clinical conditions (Lu et al., 2011).
A successful vision training protocol that is suitable for application must consider both effectiveness and cost (Lu et al., 2016). To obtain the greatest benefits from training, it is necessary to better classify the subtypes of the population using visual performance assessment batteries. An individual customized training procedure based on subgroups contributes to maximizing the training effect. Several previous studies using a grating detection task trained observers near each individual’s cutoff spatial frequency, which was defined as the spatial frequency at which the contrast threshold of the pretraining CS function (CSF) was a constant (Zhou et al., 2006, 2012; Huang et al., 2008; Yan et al., 2015; Zhang et al., 2018); that is, each observer was trained at individual spatial frequencies. Thus, an interesting question is who gains the most from perceptual learning. Do observers who were trained at a high cutoff spatial frequency gain greater visual improvement? Different spatial frequencies involve different cortical sites. Specifically, V1 neurons at the occipital pole (close to the skull) have higher preferred spatial frequencies than those located deeper within the calcarine sulcus (Horton, 2006; Henriksson et al., 2008; Yu et al., 2010). Therefore, the current study focused on the effect of trained cutoff spatial frequencies on visual improvement following perceptual learning, which also contributes to understanding the mechanisms underlying neural plasticity in different cortical areas.
The current study aimed to assess whether the gains following perceptual learning vary with the trained cutoff spatial frequencies. All observers were trained for eight daily sessions in a monocular grating detection task near their individual cutoff spatial frequencies. The same training protocol has been employed to train both normal and amblyopic populations and has been shown to be able to improve visual performance (Zhou et al., 2006, 2012; Huang et al., 2008). The cutoff spatial frequency was calculated as the spatial frequency at which the contrast threshold of pretraining CSF was 0.50. The current study provides empirical evidence regarding a customized training program for observers with different cutoff frequencies.
Materials and Methods
Observers
The participants consisted of 28 senior high school male students with cycloplegic spherical equivalence (SE) not exceeding ±0.5 diopter (D). Their VA on the Chinese Tumbling E Chart was better than 0.8 (20/25) (see Table 1 for details). None of the participants were aware of the purpose of the study. This study involving human participants was reviewed and approved in advance by the Research Ethics Committee at the Air Force Medical University and adhered to the principles of the Declaration of Helsinki. The participants and their legal guardians provided written informed consent for participation in this study.
Apparatus
The study was performed on a computer running MATLAB programs with PsychToolbox extensions (Brainard, 1997). Stimuli were displayed on a gamma-corrected monitor with a spatial resolution of 1600 × 900 pixels, a refresh rate of 75 Hz, and a mean luminance of 35 cd/m2. An 8-bit screen was used in this study. To improve the measurement accuracy of low contrast stimuli, the Bit-Stealing technique was used to increase the number of levels of gray (Tyler et al., 1992). Observers placed their head on a chin rest and viewed the display monocularly in a dimly lit room, with an opaque patch on the other eye. The display subtended 5.56° × 3.13° at a viewing distance of 4.64 m. The entire experiment was performed without optical correction.
Experimental Design
Visual acuity was measured with the Chinese Tumbling E Chart and converted to MAR acuity. All observers were randomly divided into training (S1–S18) and control groups (S19–S28). The eye with poorer VA served as the trained eye.
For the training group, the experiment contained three consecutive phases: (a) pretraining measurements of the monocular VA and CSF in the trained eye, (b) eight daily sessions of monocular training in the sine-wave grating detection task at each individual’s cutoff spatial frequency, and (c) post-training measurements that were the same as the pretraining measurements. To exclude the possibility that the visual improvements were only due to the repetition of the same VA and CSF tests at the pre- and post-training measurements, we collected a small sample of ten observers with the same criteria as the training group. All observers practiced approximately 50 trials before the pretraining measurements. Retention of the CSF and VA improvements was also assessed at least 5 months after training.
The CSF measure contained seven blocks of 100 trials and lasted approximately 45 min. CS was defined as the reciprocal of the contrast threshold for detecting a sine-wave grating with 79.3% accuracy at seven spatial frequencies of 1, 4, 8, 16, 24, 32, and 48 c/°. All spatial frequencies were interleaved among trials. The stimuli were vertical sinusoidal gratings of 2° in diameter with a half-Gaussian ramp (σ = 0.25).
The training phase contained eight daily sessions, each with seven blocks of 80 trials, and lasted approximately 35 min. Observers were trained at their individual cutoff spatial frequencies, defined as the spatial frequency at which the contrast threshold of the pretraining CSF was 0.50. Observers received 20¥ once the contrast threshold in the current session was lower than that in the last session; otherwise, there was no monetary reward.
Procedure
A two-interval, forced-choice, sine-wave grating detection task was used for the assessment of the CSF and training. A target grating was randomly presented in one of two 100 ms temporal intervals that were signaled by a brief tone at the beginning of each and separated by 500 ms. Observers indicated the signal interval using the computer keyboard by pressing the left button when the signal was present in the first interval and pressing the right button otherwise. During training, a brief tone followed each correct response. During the CSF measurements, a brief tone followed each response regardless of its accuracy. The response also initiated the next trial.
Thresholds were assessed using an adaptive staircase method, termed a three-down/one-up staircase procedure (Levitt, 1971). This method, expected to asymptote at 79.3% correct, decreased signal contrast by 10% (multiplying the previous value by 0.9) after every three consecutive correct responses and increased signal contrast by 10% after every incorrect response. One hundred trials were used to estimate the contrast threshold for detecting a sine-wave grating at a particular spatial frequency. A reversal results when the staircase changes its direction (changing from increasing to decreasing contrast or vice versa). The first five (if the number of total reversals was odd) or four (if even) reversals were excluded. We averaged the contrasts of the remaining reversals to assess the contrast threshold for detecting the grating of a certain spatial frequency. Based on the results of the pilot testing, the starting contrast for each staircase was set close to the expected threshold.
Data Analysis
Unless noted otherwise, the significance level was p < 0.05 throughout the paper. Pearson correlation with two-tailed probability values was used for all reported correlations.
The log CSF graphs log CS (1/threshold) as a function of spatial frequency. The area under the log CSF (AULCSF), which provides a broad measure of CS across all spatial frequencies, was calculated to evaluate the improvement (Koop et al., 1996).
For VA and CS, the magnitude of the improvements was calculated as follows:
In the section “Results,” the average magnitude of improvements for group level is reported: Igroup = ∑Iindividual/N (N is valid sample size). Group percent improvement can be calculated from the average dB improvement:
For VA and AULCSF, the retention of training effects was calculated by(VAretest−VApre)/(VApost−VApre)×100% and (AULCSFretest−AULCSFpre)/(AULCSFpost−AULCSFpre)×100%, respectively.
To obtain the learning curves, an exponential function was used (Zhang et al., 2019; Zhao et al., 2019):
where α is the asymptotic performance level, λ is the dynamic range of learning, γ is the time constant of the exponential function, and t is the training session number. Figure 1A shows the exponential learning curve with the three parameters.
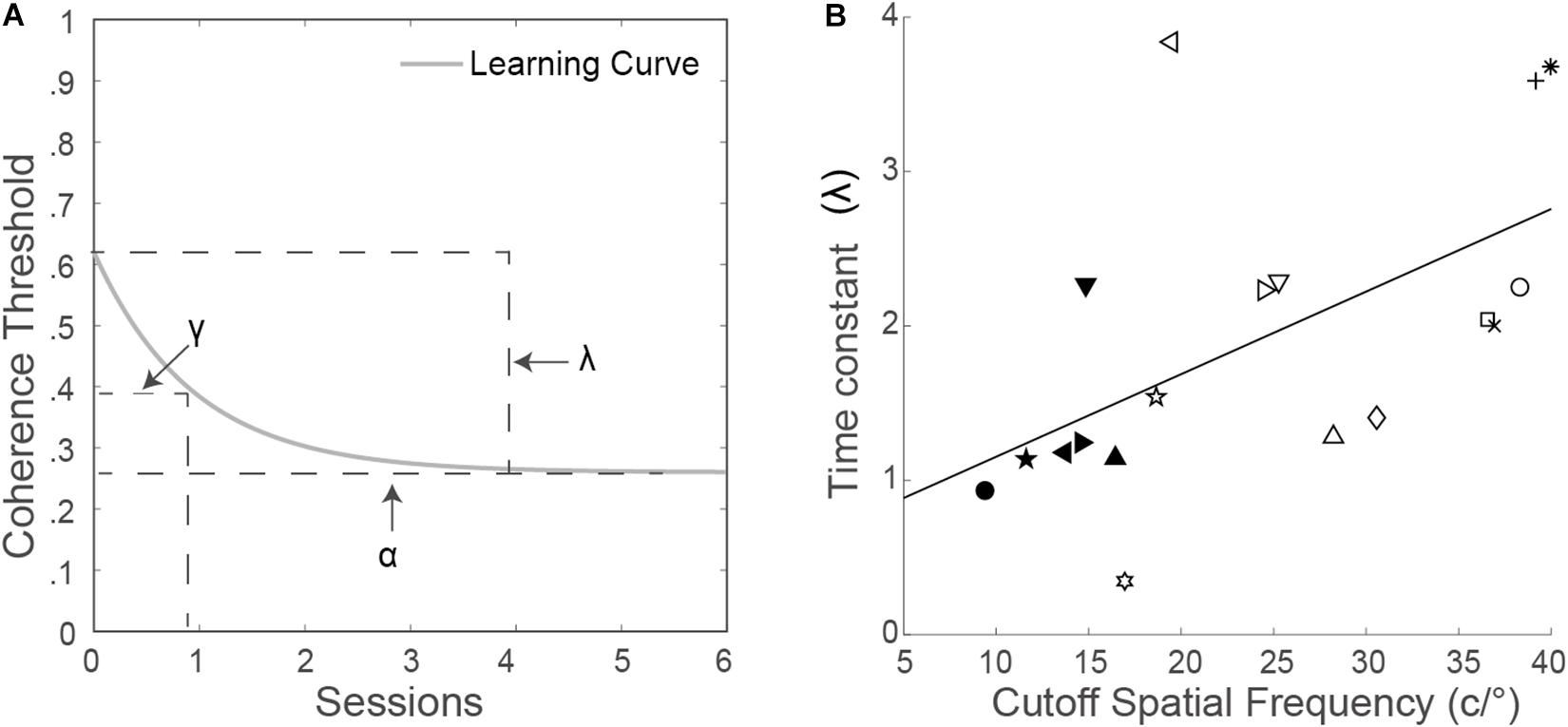
Figure 1. (A) Exponential function with the three parameters. (B) Time constant (γ) as a function of the cutoff spatial frequency. Each symbol represents an observer. The solid line is the best fitting regression line.
A non-linear least square method, implemented in MATLAB (MathWorks, Natick, MA, United States), was used to minimize the sum of squared differences between the model predictions and measured values. The goodness of fit was estimated by the following:
where ymeasured and ypredicted represent the measured and predicted values, respectively. The mean(ymeasured) is the mean of all the measured values.
Results
The Time Constant to Plateau
The time constant γ was the time to reach asymptote, calculated by fitting the exponential function with the non-linear least square method. Ten contrast thresholds at trained cutoff spatial frequencies can be used to fit the function including eight training sessions and two testing sessions (Supplementary Figure S2). The time constant γ was positively correlated with the trained cutoff spatial frequency (r = 0.57, p = 0.014; Figure 1B).
CSF Improvements
Based on the pre- and post-training CSF (Supplementary Figure S1), CS at the trained spatial frequency was calculated. Significant improvement in CS at the trained spatial frequency was found for the trained eye, t(17) = 11.61, p < 0.001, d = 2.74. Averaged across observers, the magnitude of improvement was 5.87 ± 0.61 dB (mean ± SE, range: 0.83–9.19 dB; or Pgroup = 96.56%). The improvement was significantly correlated with the cutoff spatial frequency (r = 0.63, p = 0.005), with higher gains observed for those with higher cutoff frequencies (Figure 2A).
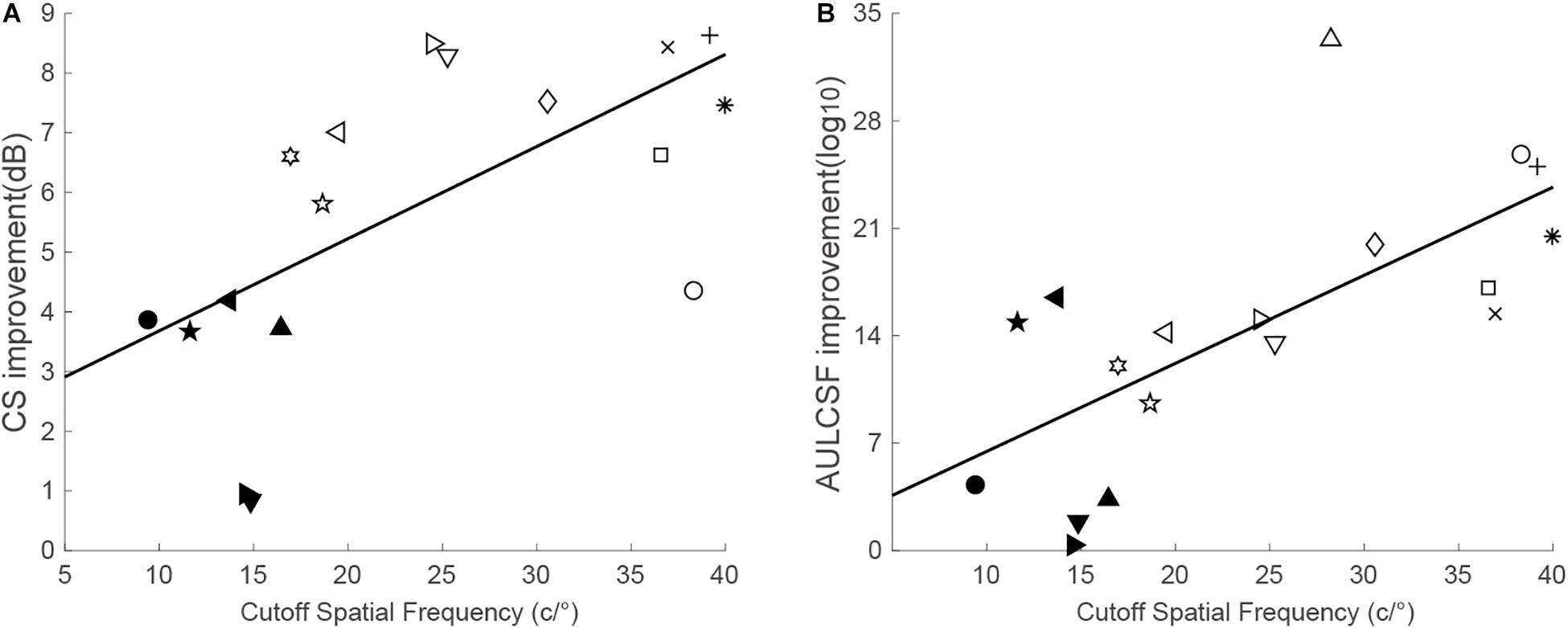
Figure 2. (A) CS improvement at the trained spatial frequency as a function of the cutoff spatial frequency. (B) AULCSF improvement as a function of the cutoff spatial frequency. The solid line is the best fitting regression line.
Training at the cutoff spatial frequency improved CS over a range of spatial frequencies (Huang et al., 2008). Thus, AULCSF was calculated to evaluate the improvements across all spatial frequencies. Similarly, the post-training AULCSF was significantly larger than the pretraining AULCSF, t(17) = 7.12, p < 0.001, d = 1.68. The absolute improvements ranged from 0.36 to 33.29 log10 units (mean ± SE: 14.60 ± 2.05 log10 units). In addition, the improvement in AULCSF was significantly related to the cutoff spatial frequency (r = 0.69, p = 0.001), indicating that training in higher cutoff spatial frequencies generated greater AULCSF improvement (Figure 2B).
Generalization of Perceptual Learning
To quantify the generalizability to other untrained spatial frequencies, we computed the bandwidth of perceptual learning, that is, the bandwidth of the difference between the post-training and pre-training CSFs in the trained eye (Huang et al., 2008). Only observers with a significant amount of performance improvement at the training frequency were included in the results reported here. The correlation analysis showed that the bandwidth of perceptual learning was positively connected with the cutoff spatial frequency (r = 0.54, p = 0.025; Figure 3). This suggests that observers with higher spatial frequencies have broader bandwidths of perceptual learning. On average, the bandwidth was 5.70 ± 0.65 octaves (mean ± SE, range: 1.41–9.46 octaves).
Visual Acuity
The paired t-test showed that training significantly improved VA, t(17) = 9.032, p < 0.001, d = 2.13. Furthermore, the best-fitting linear regression model was performed with the pre- and post-training VA of each observer. Almost all observers improved, as indicated by the clustering of data below the identity line. The model had a slope of 0.99, r2 = 0.89, p < 0.001. F-tests were conducted to compare the slope of the linear model and 1. If the linear model and the identity line were parallel, no effect of pretraining VA was found on the training improvement of VA. As shown in Figure 4A, no significant difference in the trained eye was found, F(1,32) = 0.007, p = 0.936.
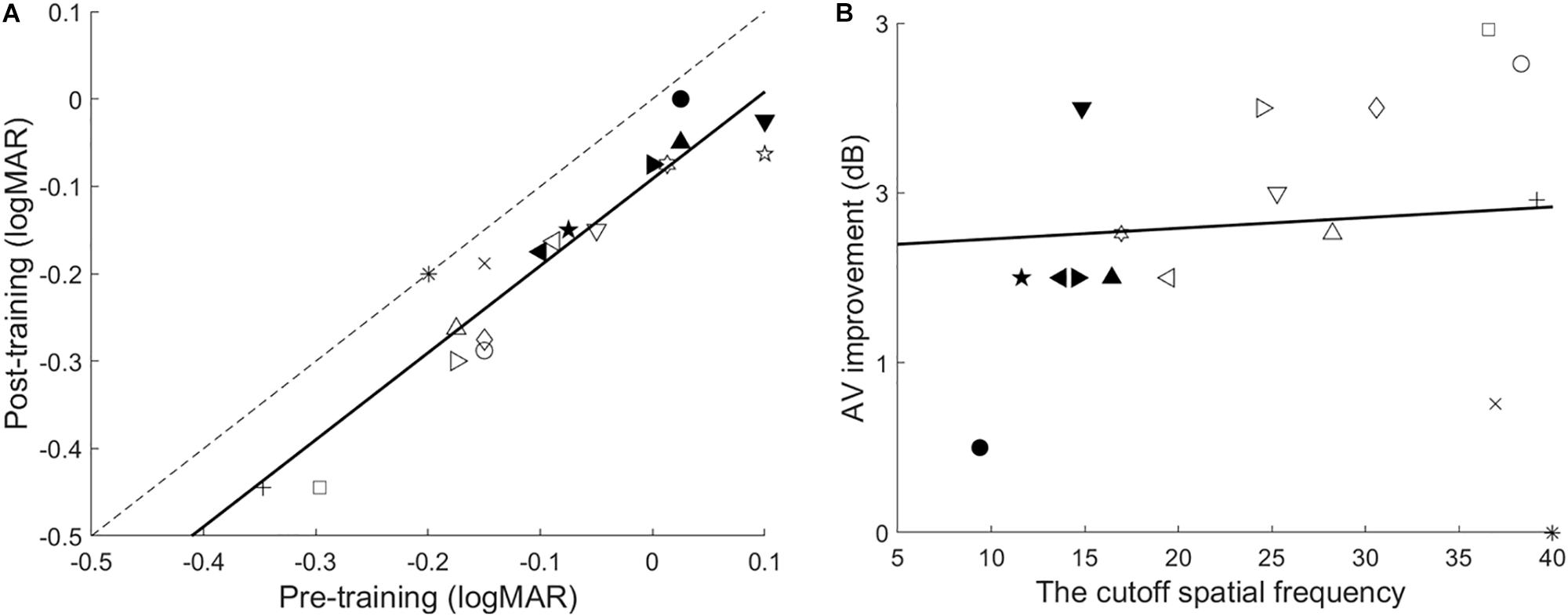
Figure 4. (A) The correlation of pre-training and post-training VA. The dashed line is the identity line with a slope of 1. The data points representing improved VA are located below this line. The solid line is the best-fitting linear model. (B) VA improvement as a function of the cutoff spatial frequency. The solid line is the best fitting regression line.
Additionally, the magnitude of the VA improvements was not correlated with the cutoff spatial frequency (r = 0.08, p = 0.760), indicating that the trained cutoff frequency does not influence the VA gains (Figure 4B). The magnitude of improvement was 1.82 ± 0.20 dB (mean ± SE, range: 0–3.26 dB; or Pgroup = 23.31%).
Retention
We remeasured the VA and CSF of the trained eye 5 months after training in 11 of the 18 observers. The observers retained 67.44% and 59.41% of their VA and AULCSF improvements, respectively. In addition, the trained cutoff spatial frequency was not correlated with the retention of training effects for VA (r = 0.41, p = 0.209) and AULCSF (r = 0.38, p = 0.256).
Control Group
For the control group, there was no significant change in AULCSF improvements, t(9) = 0.955, p = 0.364, d = 0.30. The same pattern was found for VA; that is, no significant change in VA after training was found, t(9) = 0.599, p = 0.564, d = 0.19.
Discussion
The current research aimed to investigate the effect of the participants’ individual cutoff spatial frequencies on the time constant for perceptual learning, magnitude, and transfer of perceptual learning after training in a monocular grating detection task. The results showed that perceptual learning induced significant visual improvements (i.e., CS at the cutoff spatial frequency, AULCSF, and VA), of which CS and AULCSF were closely related to the trained spatial frequency, with higher gains observed for those with higher spatial frequencies. In particular, after eight training sessions, the time constant to reach asymptote was positively related to the cutoff spatial frequency. A smaller time constant occurred at lower spatial frequencies. In contrast, the magnitude of CS improvement at higher spatial frequencies was larger than it was at lower spatial frequencies. Furthermore, the magnitude of AULCSF improvement at higher spatial frequencies was larger than that at lower spatial frequencies. However, the cutoff spatial frequency did not influence the VA improvements. Additionally, the bandwidth of perceptual learning was broader at higher cutoff spatial frequencies. Finally, the retention of VA and AULCSF improvements after 5 months was not related to the cutoff spatial frequency.
Overall, these results suggest that the magnitude and transfer of perceptual learning are tied to the trained cutoff spatial frequencies, with higher gains observed for higher cutoff spatial frequencies. However, the cutoff spatial frequency has no effect on improvements in VA and retention. These results may have important practical implications for the development of personalized training programs and theoretical importance for the understanding of neural plasticity in different cortical areas.
Contrast sensitivity function describes how visual sensitivity varies as a function of grating spatial frequency, providing a comprehensive measure of the visual system over a wide range of spatial frequencies in both normal and abnormal vision (Ginsburg, 2003). The CSF is closely correlated with daily visual functions, because our visual environment includes a variety of visual stimuli with a broad spatial frequency spectrum and a wide range of contrasts (Hou et al., 2015). Different spatial frequencies may involve various cortical areas. In a study using transcranial direct current stimulation (tDCS), the effects of tDCS were the largest for stimuli presented at higher spatial frequencies (Richard et al., 2015). The authors suggested that the effects of tDCS on CS were the greatest when higher spatial frequency gratings were used, as neurons with higher preferred spatial frequencies would be most influenced by tDCS. Since the magnitude of the electric field generated by tDCS is greater at the cortical surface, the superficial layers in the primary visual cortex near the apex of the calcarine sulcus contain neurons with higher preferred spatial frequencies than cells further from the apex (Horton, 2006; Henriksson et al., 2008; Yu et al., 2010). In the current study, observers with higher cutoff spatial frequencies generated larger magnitude and greater transfer of perceptual learning than those with lower cutoff spatial frequencies. That is, training at different spatial frequencies induced different levels of visual improvement, which is regarded as a manifestation of various degrees of cortical plasticity (Gilbert et al., 2001; Yotsumoto et al., 2008). Since various spatial frequencies recruit diverse cortical areas, it is plausible that the neural plasticity of diverse cortical areas is different. More specifically, V1 neurons at the occipital pole (close to the skull) are more plastic than those located deeper within the calcarine sulcus. Overall, the larger improvements and broader bandwidth, suggesting more plasticity in higher trained spatial frequencies, provides a strong empirical and theoretical basis for perceptual learning.
Previous evidence has also found that there was an asymmetric effect of training at high and low spatial frequencies (Huang et al., 2008). Specifically, training near the cutoff spreads broadly across lower spatial frequencies, whereas training at higher spatial frequencies spreads narrowly, which is opposite with our results. The possible reason is the differences in study samples. Huang et al. (2009) required the adults with normal or corrected-to-normal vision to train at higher spatial frequencies, and adults with unilateral anisometropic amblyopia to train at lower spatial frequencies. The results showed that the bandwidth of perceptual learning in the amblyopic visual system (lower trained spatial frequencies) is much broader than that in the normal visual system. However, all observers in the current study had normal or close to normal vision. Many previous studies found that neural plasticity is more robust in amblyopia (Huang et al., 2009; Astle et al., 2011). Thus, the neural plasticity of amblyopia may cover the plastic differences that result from various spatial frequencies.
Interestingly, our results showed that observers who gained more improvement had larger time constant. Li et al. (2008) found that those with mild amblyopia more quickly reach asymptote than those with severe amblyopia (5.55 and 18.75 h, respectively). Obviously, the amount of improvement is positively related to the time constants; that is, more time is needed to reach asymptote. In their study, individuals with deep amblyopia gained more improvement and thus required more time. If so, in current study, observers training at high spatial frequencies improved more, thus they need more time (larger exponential time constant) to reach plateau. The time constant to reach plateau was dependent on the amount of improvement, with a larger time constant observed for those with larger improvement.
Generally, the cutoff frequencies are positively related to the initial VA; that is, observers with high cutoff frequencies tend to have better pretraining VA. This pattern of results was also verified in current study (p < 0.001). Thus, our results may also suggest that the initial VA had a positive effect on visual improvement, which is opposite to previous studies. For example, Yan et al. (2015) found greater acuity improvement for observers with worse initial VA. Yehezkel et al. (2016) also found that subjects with more advanced presbyopia and thus poorer initial VA showed the greatest improvement in VA. Even so, there were at least two differences between these previous studies and this study. First, the initial vision and age differed. Yan et al. (2015) recruited myopic adult observers; Zhou et al. (2012) recruited presbyopic adults; and the present study recruited adolescent observers with normal or close to normal vision. Second, the incentive conditions were different. In contrast with the other two studies, the current study provided a high monetary reward once the participants’ performance was better than that in the last session. These factors may be the moderating variables that change the effect of initial performance on perceptual learning. The issue regarding the relationship between initial performance and improvement remains ambiguous. Specifically, patients with age-related macular degeneration learned at an equivalent rate to age-matched participants with normal vision (Astle et al., 2015). After equating the observers’ initial thresholds at the various eccentricities by spatially scaled stimuli, or introducing different levels of crowding, the original different amounts of improvement at each eccentricity disappeared (Astle et al., 2013). Thus, the effect of pretraining performance on perceptual learning must be deeply investigated by strictly controlling the experimental conditions in future research.
In contrast with the magnitude and transfer of perceptual learning, the trained cutoff frequency failed to relate to the VA improvement and retention of training effects. VA refers to the ability to discriminate two stimuli separated in space at high contrast relative to the background. It is different from CSF, which measures the CS in a wide range of spatial frequencies. Regarding retention, the results of this study suggest that the trained spatial frequency influences the training effect rather than the retention of the training effect.
Although we did not get the Bonferroni corrected/adjusted p-value for correlations in section “Result,” it is still meaningful to discuss this issue. Since the cutoff spatial frequency has been used in seven different correlations, the Bonferroni corrected p is 0.05/7 = 0.007. With this conservative criterion, the correlations between trained cutoff spatial frequency and time constant to plateau and the bandwidth become not significant. In other words, only the magnitude of perceptual learning is tied to the trained cutoff spatial frequencies. This may be due to the short training paradigm that a few subjects did not reach the plateau. On the one hand, the time constant to plateau and trained spatial frequencies was not correlated after Bonferroni correction. Based on the individuals’ learning curves, a few of observers did not reach their final plateau, especially those with higher cutoff spatial frequencies. But it does not influence our finding: observers who gained more improvement had larger time constant. If observers with higher cutoff frequencies achieved asymptotic performance with enough sessions, the time constant would be larger. On the other hand, the bandwidth of perceptual leaning did not relate to trained spatial frequencies. Based on the previous studies, the relationship between generalization and the amount of learning remains controversial (Lengyel and Fiser, 2019). Intuitively, generalization and learning should go hand in hand. However, excessive training could induce overfitting, which could weaken extra learning, but it also substantially decreases generalization. Indeed, several behavioral studies have confirmed this conjecture (Jeter et al., 2010; Hussain et al., 2012). Thus, the learning and generalization should be deeply investigated in future research.
The current study trained observers with a sine-wave grating detection task and found that the training effect was modulated by the trained spatial frequency. It is unclear whether the conclusions of the current study can be generalized to other tasks. For example, observers in a coherent motion detection task who trained at different coherence levels show a fixed percent correct. It has been demonstrated that various tasks recruit different brain areas. For example, the grating detection task used in the current study is related to the primary visual cortex (V1; Schoups et al., 2001; Ghose et al., 2002; Furmanski et al., 2004), while V4 is engaged in the orientation discrimination task (Yang and Maunsell, 2004). Thus, the generalizability of this study to other perceptual learning tasks regarding different brain areas should be further investigated.
From a practical standpoint, the present research indicates that it would be beneficial to translate perceptual learning from the laboratory to applications. Many studies have suggested that perceptual learning is a potential treatment for amblyopia, low myopia, presbyopia, and other issues. The ideal vision training would be able to obtain maximal gains with minimal costs. Redundant training wastes time and costs, and insufficient training does not produce the expected training effect. Thus, it is important to optimize vision training. The current study found that individual spatial frequencies have an effect on the magnitude of visual improvement, which provides empirical support for optimizing and customizing training programs for various observers. Additionally, the pretraining vision in this study was normal or close to normal. Regardless, the vision significantly improved after training, indicating perceptual learning can improve vision, which has important practical value for occupations that have special requirements for vision such as pilots. Additionally, this study has important theoretical implications for understanding the neural plasticity of different cortical areas. In the primary visual cortex, V1 neurons at the superficial layers near the apex of the calcarine sulcus have higher preferred spatial frequencies, inducing greater improvement after perceptual learning. Thus, this result provides insights into the neural mechanisms underlying the efficiency of perceptual learning.
In summary, we found that observers with higher cutoff spatial frequencies generated a higher magnitude and generalization of visual improvement than those with lower cutoff spatial frequencies after contrast detection training, even though all the observers had normal or close to normal vision. This finding suggests that the training stimulus is an important component in applications of perceptual learning. The development of a successful training protocol should consider individual differences in the training stimuli.
Data Availability Statement
All datasets generated for this study are included in the article/Supplementary Material.
Ethics Statement
The study involving human participants was reviewed and approved in advance by the Research Ethics Committee at the Air Force Medical University and adhered to the principles of the Declaration of Helsinki. The participants and their legal guardians provided written informed consent for participation in this study.
Author Contributions
DW, PZ, GC, and NL performed the research. DW and PZ analyzed the data. DW and WJ wrote the manuscript. DW, PZ, CL, WR, and YS revised the manuscript and work on the review. PZ programmed the code. WX supervised the whole work.
Funding
This research was supported by the Major Project of Medicine Science and Technology (Grant No. AWS13J003) to WX.
Conflict of Interest
The authors declare that the research was conducted in the absence of any commercial or financial relationships that could be construed as a potential conflict of interest.
Supplementary Material
The Supplementary Material for this article can be found online at: https://www.frontiersin.org/articles/10.3389/fpsyg.2020.00265/full#supplementary-material
References
Ahissar, M., and Hochstein, S. (1997). Task difficulty and the specificity of perceptual learning. Nature 387, 401–406. doi: 10.1038/387401a0
Astle, A. T., Blighe, A. J., Webb, B. S., and McGraw, P. V. (2015). The effect of normal aging and age-related macular degeneration on perceptual learning. J. Vis. 15, 1–16. doi: 10.1167/15.10.16
Astle, A. T., Li, R. W., Webb, B. S., Levi, D. M., and McGraw, P. V. (2013). A Weber-like law for perceptual learning. Sci. Rep. 3:1158. doi: 10.1038/srep01158
Astle, A. T., Webb, B. S., and McGraw, P. V. (2011). The pattern of learned visual improvements in adult amblyopia. Invest. Ophthalmol. Vis. Sci. 52, 7195–7204. doi: 10.1167/iovs.11-7584
Ball, K., and Sekuler, R. (1987). Direction-specific improvement in motion discrimination. Vision Res. 27, 953–965. doi: 10.1016/0042-6989(87)90011-3
Barollo, M., Contemori, G., Battaglini, L., Pavan, A., and Casco, C. (2017). Perceptual learning improves contrast sensitivity, visual acuity, and foveal crowding in amblyopia. Restor. Neurol. Neurosci. 35, 483–496. doi: 10.3233/RNN-170731
Camilleri, R., Pavan, A., Ghin, F., Battaglini, L., and Campana, G. (2014). Improvement of uncorrected visual acuity and contrast sensitivity with perceptual learning and transcranial random noise stimulation in individuals with mild myopia. Front. Psychol. 5:1234. doi: 10.3389/fpsyg.2014.01234
Campana, G., Camilleri, R., Pavan, A., Veronese, A., and Lo Giudice, G. (2014). Improving visual functions in adult amblyopia with combined perceptual training and transcranial random noise stimulation (tRNS): a pilot study. Front. Psychol. 5:1402. doi: 10.3389/fpsyg.2014.01402
Casco, C., Barollo, M., Contemori, G., and Battaglini, L. (2018). Neural restoration training improves visual functions and expands visual field of patients with homonymous visual field defects. Restor. Neurol. Neurosci. 36, 275–291. doi: 10.3233/rnn-170752
Casco, C., Guzzon, D., Moise, M., Vecchies, A., Testa, T., and Pavan, A. (2014). Specificity and generalization of perceptual learning in low myopia. Restor. Neurol. Neurosci. 3, 639–653. doi: 10.3233/RNN-140389
Chung, S. T. L. (2011). Improving reading speed for people with central vision loss through perceptual learning. Invest. Ophthalmol. Vis. Sci. 52, 1164–1170. doi: 10.1167/iovs.10-6034
Chung, S. T. L., Li, R. W., and Levi, D. M. (2006). Identification of contrast-defined letters benefits from perceptual learning in adults with amblyopia. Vision Res. 46, 3853–3861. doi: 10.1016/j.visres.2006.06.014
Contemori, G., Trotter, Y., Cottereau, B. R., and Maniglia, M. (2019). tRNS boosts perceptual learning in peripheral vision. Neuropsychologia 125, 129–136. doi: 10.1016/j.neuropsychologia.2019.02.001
Deveau, J., Ozer, D. J., and Seitz, A. R. (2014). Improved vision and on-field performance in baseball through perceptual learning. Curr. Biol. 24, R146–R147. doi: 10.1016/j.cub.2014.01.004
Ding, J., and Levi, D. M. (2011). Recovery of stereopsis through perceptual learning in human adults with abnormal binocular vision. Proc. Natl. Acad. Sci. U.S.A. 108, E733–E741. doi: 10.1073/pnas.1105183108
Fahle, M., and Edelman, S. (1993). Long-term learning in vernier acuity: Effects of stimulus orientation, range and of feedback. Vision Res. 33, 397–412. doi: 10.1016/0042-6989(93)90094-d
Fahle, M., and Morgan, M. (1996). No transfer of perceptual learning between similar stimuli in the same retinal position. Curr. Biol. 6, 292–297. doi: 10.1016/s0960-9822(02)00479-7
Furmanski, C. S., Schluppeck, D., and Engel, S. A. (2004). Learning strengthens the response of primary visual cortex to simple patterns. Curr. Biol. 14, 573–578. doi: 10.1016/j.cub.2004.03.032
Ghose, G. M., Yang, T., and Maunsell, J. H. R. (2002). Physiological correlates of perceptual learning in monkey V1 and V2. J. Neurophysiol. 87, 1867–1888. doi: 10.1152/jn.00690.2001
Gilbert, C. D., Li, W., and Piech, V. (2009). Perceptual learning and adult cortical plasticity. J. Physiol. 587, 2743–2751. doi: 10.1113/jphysiol.2009.171488
Gilbert, C. D., and Sigman, M. (2000). Learning to find a shape. Nat. Neurosci. 3, 264–269. doi: 10.1038/72979
Gilbert, C. D., Sigman, M., and Crist, R. E. (2001). The neural basis of perceptual learning. Neuron 31, 681–697. doi: 10.1016/S0896-6273(01)00424-X
Ginsburg, A. P. (2003). Contrast sensitivity and functional vision. Int. Ophthalmol. Clin. 43, 5–15. doi: 10.1097/00004397-200343020-00004
Gold, J., Bennett, P. J., and Sekuler, A. B. (1999). Signal but not noise changes with perceptual learning. Nature 402, 176–178. doi: 10.1038/46027
Gori, S., and Facoetti, A. (2014). Perceptual learning as a possible new approach for remediation and prevention of developmental dyslexia. Vision Res. 99, 78–87. doi: 10.1016/j.visres.2013.11.011
Henriksson, L., Nurminen, L., Hyvarinen, A., and Vanni, S. (2008). Spatial frequency tuning in human retinotopic visual areas. J. Vis. 8, 1–13. doi: 10.1167/8.10.5
Horton, J. C. (2006). Ocular integration in the human visual cortex. Can. J. Ophthalmol. 41, 584–593. doi: 10.1016/s0008-4182(06)80027-x
Hou, F., Lesmes, L., Bex, P., Dorr, M., and Lu, Z. (2015). Using 10AFC to further improve the efficiency of the quick CSF method. J. Vis. 15, 1–18. doi: 10.1167/15.9.2
Huang, C., Lu, Z., and Zhou, Y. (2009). Mechanisms underlying perceptual learning of contrast detection in adults with anisometropic amblyopia. J. Vis. 9, 1–24. doi: 10.1167/9.11.24
Huang, C., Tao, L., Zhou, Y., and Lu, Z. (2007). Treated amblyopes remain deficient in spatial vision: a contrast sensitivity and external noise study. Vision Res. 47, 22–34. doi: 10.1016/j.visres.2006.09.015
Huang, C., Zhou, Y., and Lu, Z. (2008). Broad bandwidth of perceptual learning in the visual system of adults with anisometropic amblyopia. Proc. Natl. Acad. Sci. U.S.A. 105, 4068–4073. doi: 10.1073/pnas.0800824105
Huang, J., Liang, J., Zhou, Y., and Liu, Z. (2017). Transfer in motion discrimination learning was no greater in double training than in single training. J. Vis. 17, 1–10. doi: 10.1167/17.6.7
Hung, S. C., and Seitz, A. R. (2014). Prolonged training at threshold promotes robust retinotopic specificity in perceptual learning. J. Neurosci. 34, 8423–8431. doi: 10.1523/JNEUROSCI.0745-14.2014
Hussain, Z., Bennett, P. J., and Sekuler, A. B. (2012). Versatile perceptual learning of textures after variable exposures. Vision Res. 61, 89–94. doi: 10.1016/j.visres.2012.01.005
Jehee, J. F. M., Ling, S., Swisher, J. D., van Bergen, R. S., and Tong, F. (2012). Perceptual learning selectively refines orientation representations in early visual cortex. J. Neurosci. 32, 16747–16753. doi: 10.1523/JNEUROSCI.6112-11.2012
Jeter, P. E., Dosher, B. A., Liu, S., and Lu, Z. (2010). Specificity of perceptual learning increases with increased training. Vision Res. 50, 1928–1940. doi: 10.1113/jphysiol.2009.171488
Jeter, P. E., Dosher, B. A., Petrov, A., and Lu, Z. L. (2009). Task precision at transfer determines specificity of perceptual learning. J. Vis. 9, 1–13. doi: 10.1167/9.3.1
Karni, A., and Sagi, D. (1991). Where practice makes perfect in texture discrimination: evidence for primary visual cortex plasticity. Proc. Natl. Acad. Sci. U.S.A. 88, 4966–4970. doi: 10.1073/pnas.88.11.4966
Koop, M. R., Applegate, R. A., and Howland, H. C. (1996). Changes in the area under the log contrast sensitivity function (AULCSF) with myopic refractive error. Invest. Ophthalmol. Vis. Sci. 37:S322.
Larcombe, S. J., Kennard, C., and Bridge, H. (2018). Increase in MST activity correlates with visual motion learning: a functional MRI study of perceptual learning. Hum. Brain. Mapp. 39, 145–156. doi: 10.1002/hbm.23832
Lengyel, G., and Fiser, J. (2019). The relationship between initial threshold, learning, and generalization in perceptual learning. J. Vis. 19:28. doi: 10.1167/19.4.28
Levitt, H. (1971). Transformed up-down methods in psychoacoustics. J. Acoust. Soc. Am. 49, 467–477. doi: 10.1121/1.1912375
Li, R. W., Klein, S. A., and Levi, D. M. (2008). Prolonged perceptual learning of positional acuity in adult amblyopia: perceptual template retuning dynamics. J Neurosci. 28, 14223–14229. doi: 10.1523/JNEUROSCI.4271-08.2008
Li, W., Piëch, V., and Gilbert, C. D. (2004). Perceptual learning and top-down influences in primary visual cortex. Nat. Neurosci. 7, 651–657. doi: 10.1038/nn1255
Liu, Z. (1999). Perceptual learning in motion discrimination that generalizes across motion directions. Proc. Natl. Acad. Sci. U.S.A. 96, 14085–14087. doi: 10.1073/pnas.96.24.14085
Lu, Z., Hua, T., Huang, C., Zhou, Y., and Dosher, B. A. (2011). Visual perceptual learning. Neurobiol. Learn. Mem. 95, 145–151. doi: 10.1016/j.nlm.2010.09.010
Lu, Z., Lin, Z., and Dosher, B. A. (2016). Translating perceptual learning from the laboratory to applications. Trends Cogn. Sci. 20, 561–563. doi: 10.1016/j.tics.2016.05.007
Maniglia, M., Pavan, A., Sato, G., Contemori, G., Montemurro, S., Battaglini, L., et al. (2016). Perceptual learning leads to long lasting visual improvement in patients with central vision loss. Restor. Neurol. Neuros. 34, 697–720. doi: 10.3233/RNN-150575
McGovern, D. P., Webb, B. S., and Peirce, J. W. (2012). Transfer of perceptual learning between different visual tasks. J. Vis. 12, 1–11. doi: 10.1167/12.11.4
Moret, B., Camilleri, R., Pavan, A., Lo Giudice, G., Veronese, A., Rizzo, R., et al. (2018). Differential effects of high-frequency transcranial random noise stimulation (hf-tRNS) on contrast sensitivity and visual acuity when combined with a short perceptual training in adults with amblyopia. Neuropsychologia 114, 125–133. doi: 10.1016/j.neuropsychologia.2018.04.017
Polat, U., Schor, C., Tong, J., Zomet, A., Lev, M., Yehezkel, O., et al. (2012). Training the brain to overcome the effect of aging on the human eye. Sci. Rep. 2:278. doi: 10.1038/srep00278
Richard, B., Johnson, A. P., Thompson, B., and Hansen, B. C. (2015). The Effects of tDCS across the spatial frequencies and orientations that comprise the contrast sensitivity function. Front. Psychol. 6:1784. doi: 10.3389/fpsyg.2015.01784
Schoups, A., Vogels, R., Qian, N., and Orban, G. (2001). Practising orientation identification improves orientation coding in V1 neurons. Nature 412, 549–553. doi: 10.1038/35087601
Sterkin, A., Levy, Y., Pokroy, R., Lev, M., Levian, L., Doron, R., et al. (2018). Vision improvement in pilots with presbyopia following perceptual learning. Vision Res. 152, 61–73. doi: 10.1016/j.visres.2017.09.003
Tan, D. T. H., and Fong, A. (2008). Efficacy of neural vision therapy to enhance contrast sensitivity function and visual acuity in low myopia. J. Cataract. Refract. Surg. 34, 570–577. doi: 10.1016/j.jcrs.2007.11.052
Tyler, C. W., Chan, H., Lei, L., Mcbride, B., and Kontsevich, L. L. (1992). Bit stealing: how to get 1786 or more gray levels from an 8-bit color monitor. Proc. SPIE 1666, 351–364. doi: 10.1117/12.135981
Wang, Y., Song, Y., Qu, Z., and Ding, Y. (2010). Task difficulty modulates electrophysiological correlates of perceptual learning. Int. J. Psychophysiol. 75, 234–240. doi: 10.1016/j.ijpsycho.2009.11.006
Xi, J., Jia, W., Feng, L., Lu, Z., and Huang, C. (2014). Perceptual learning improves stereoacuity in amblyopia. Invest. Ophthalmol. Vis. Sci. 55, 2384–2391. doi: 10.1167/iovs.13-12627
Xiao, L., Zhang, J., Wang, R., Klein, S. A., Levi, D. M., and Yu, C. (2008). Complete transfer of perceptual learning across retinal locations enabled by double training. Curr. Biol. 18, 1922–1926. doi: 10.1016/j.cub.2008.10.030
Yan, F., Zhou, J., Zhao, W., Li, M., Xi, J., Lu, Z., et al. (2015). Perceptual learning improves neural processing in myopic vision. J. Vis. 15:12. doi: 10.1167/15.10.12
Yang, T., and Maunsell, J. H. R. (2004). The effect of perceptual learning on neuronal responses in monkey Visual area V4. J. Neurosci. 24, 1617–1626. doi: 10.1523/JNEUROSCI.4442-03.2004
Yehezkel, O., Sterkin, A., Lev, M., Levi, D. M., and Polat, U. (2016). Gains following perceptual learning are closely linked to the initial visual acuity. Sci. Rep. 6:25188. doi: 10.1038/srep25188
Yotsumoto, Y., Watanabe, T., and Sasaki, Y. (2008). Different dynamics of performance and brain activation in the time course of perceptual learning. Neuron 57, 827–833. doi: 10.1016/j.neuron.2008.02.034
Yu, C., Klein, S. A., and Levi, D. M. (2004). Perceptual learning in contrast discrimination and the (minimal) role of context. J. Vis. 4, 169–182. doi: 10.1167/4.3.4
Yu, H. H., Verma, R., Yang, Y., Tibballs, H. A., Lui, L. L., Reser, D. H., et al. (2010). Spatial and temporal frequency tuning in striate cortex: functional uniformity and specializations related to receptive field eccentricity. Eur. J. Neurosci. 31, 1043–1062. doi: 10.1111/j.1460-9568.2010.07118.x
Zhang, J., Zhang, T., Xue, F., Liu, L., and Yu, C. (2009). Legibility of Chinese characters in peripheral vision and the top-down influences on crowding. Vision Res. 49, 44–53. doi: 10.1016/j.visres.2008.09.021
Zhang, P., Hou, F., Yan, F., Xi, J., Lin, B., Zhao, J., et al. (2018). High reward enhances perceptual learning. J. Vis. 18, 1–21. doi: 10.1167/18.8.11
Zhang, P., Zhao, Y., Dosher, B. A., and Lu, Z. (2019). Assessing the detailed time course of perceptual sensitivity change in perceptual learning. J. Vis. 19, 1–19. doi: 10.1167/19.5.9
Zhao, Y., Lesmes, L., and Lu, Z. (2019). Efficient assessment of the time course of perceptual sensitivity change. Vision Res. 154, 21–43. doi: 10.1016/j.visres.2018.10.009
Zhou, J., Zhang, Y., Dai, Y., Zhao, H., Liu, R., Hou, F., et al. (2012). The eye limits the brain’s learning potential. Sci. Rep. 2:364. doi: 10.1038/srep00364
Keywords: perceptual learning, cutoff spatial frequency, visual acuity, contrast sensitivity function, visual improvement
Citation: Wu D, Zhang P, Li C, Liu N, Jia W, Chen G, Ren W, Sun Y and Xiao W (2020) Perceptual Learning at Higher Trained Cutoff Spatial Frequencies Induces Larger Visual Improvements. Front. Psychol. 11:265. doi: 10.3389/fpsyg.2020.00265
Received: 04 November 2019; Accepted: 04 February 2020;
Published: 21 February 2020.
Edited by:
Gianluca Campana, University of Padova, ItalyReviewed by:
Luca Battaglini, University of Padova, ItalyClaudia Lunghi, UMR8248 Laboratoire des Systèmes Perceptifs, France
Copyright © 2020 Wu, Zhang, Li, Liu, Jia, Chen, Ren, Sun and Xiao. This is an open-access article distributed under the terms of the Creative Commons Attribution License (CC BY). The use, distribution or reproduction in other forums is permitted, provided the original author(s) and the copyright owner(s) are credited and that the original publication in this journal is cited, in accordance with accepted academic practice. No use, distribution or reproduction is permitted which does not comply with these terms.
*Correspondence: Wei Xiao, NDI5NTg3MzJAcXEuY29t
†These authors have contributed equally to this work and share first authorship