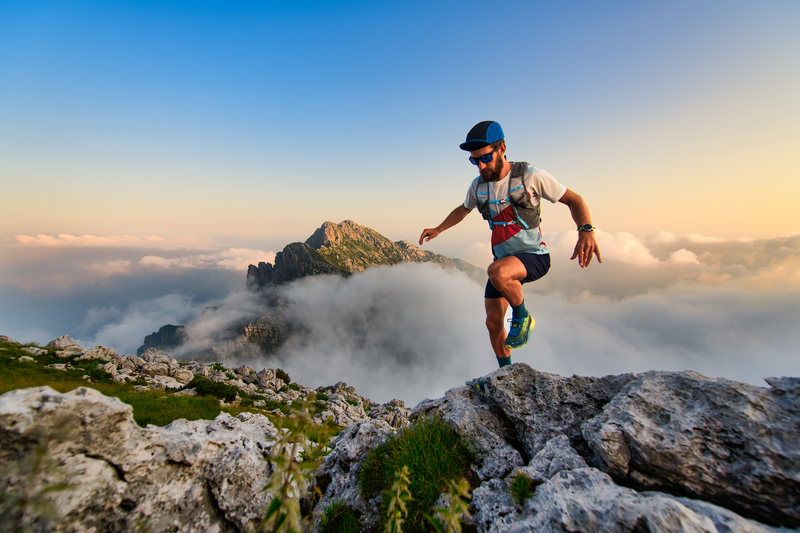
94% of researchers rate our articles as excellent or good
Learn more about the work of our research integrity team to safeguard the quality of each article we publish.
Find out more
ORIGINAL RESEARCH article
Front. Psychol. , 16 July 2019
Sec. Quantitative Psychology and Measurement
Volume 10 - 2019 | https://doi.org/10.3389/fpsyg.2019.01646
This article is part of the Research Topic Scale Development and Score Validation View all 23 articles
The popularity of information and communication technology (ICT) has had a significant influence on the reading proficiency of early adolescents. Achieving excellent reading proficiency, which is related not only to a student’s inherent talent but also to various impact factors, can greatly enhance the effectiveness of reading education. The Program for International Student Assessment (PISA) 2015 provides an international view on the reading proficiency of 15-year-olds in a computer-based testing environment. In this study, a multiple linear regression model was constructed using the computing language R to investigate the association between student-level ICT impact factors (the availability of ICT, the use of ICT and attitudes toward ICT) and reading proficiency among early adolescents. The sample included 37,155 15-year-olds from five representative countries with extremely high reading proficiency. The results showed that the students’ ICT-related attitudinal factors concerning their interest in ICT and perceived autonomy in using ICT, rather than ICT availability and ICT use, were closely associated with high reading proficiency. In addition, ICT devices should be integrated not only as instructional media but also as a cognitive tool for teaching reading with timely and appropriate scrutiny.
The concept of computer-based assessment of reading proficiency is of fundamental significance in the age of information and communication technology (ICT) (Naumann, 2015). The proliferation of ICT has a profound influence on the concept of reading proficiency (e.g., Liu, 2005; Coiro and Dobler, 2007) because it has largely reshaped students’ learning processes and reading activities (e.g., Gan et al., 2015; Mantoro et al., 2017) by engaging students in effective reading activities (e.g., Chen and Hu, 2018) and improving their reading comprehension ability (e.g., Whyte et al., 2014). As the benchmark of international large-scale assessment, the Program for International Student Assessment (PISA) has evaluated reading, science, and mathematics achievement among 15-year-olds from participating countries/economies of the Organization of Economic and Cultural Development (OECD) every 3 years since 2000. Reading proficiency in this influential assessment is recognized as “students’ ability to understand, use, reflect on and engage with written texts in order to achieve one’s goals, develop one’s knowledge and potential, and participate in society” (OECD, 2015, p. 30). This large-scale assessment facilitates the infrastructural and epistemological construction of global education work (Sellar and Lingard, 2013). For the first time, the PISA 2015 delivered the assessments of all three subjects via computer. Among the 72 participating economies, only 15 economies took the paper-based test due to technical problems. These changes have launched a new area of research, that is, the role played by myriad ICT impact factors in students’ reading proficiency because different types of reading activities and related impact factors have emerged (OECD, 2011).
The PISA reading proficiency test has been studied for nearly 20 years. From the long-term perspective, from the PISA 2000 to the PISA 2015, there has been no significant change in the framework of reading assessment among the six consecutive cycles of PISA 2000, PISA 2003, PISA 2006, PISA 2009, PISA 2012, and PISA 2015 (OECD, 2012, 2017). Thus, the whole reading framework and a large number of derived variables in the PISA 2015 were also taken from the previous PISA cycles without change as part of the trend content. In this sixth cycle of PISA assessment, a set of tasks including 103 questions was used in the PISA 2015 reading assessment (OECD, 2016, p. 146). Students’ reading proficiency scores were analyzed based on item response theory and officially released in the PISA 2015 Results. The proficiency levels described from the lowest to the highest are Level 1b, Level 1a, Level 2, Level 3, Level 4, Level 5, and Level 6. These seven proficiency levels used in the PISA 2015 reading assessment are the same as those established for the PISA 2009 assessment. The required reading skills at each proficiency level are described according to the three processes by which students answer the questions. These three processes are defined in the framework as “access and retrieve” (skills associated with finding, selecting and collecting information); “integrate and interpret” (processing what is read to make sense of a text); and “reflect and evaluate” (drawing on knowledge, ideas or values external to the text) (OECD, 2016, p. 162).
Starting with the PISA 2009, the OECD, for the first time, designed a computer-based reading assessment as an additional option for its reading proficiency test. Regarding the assessment contents of the paper-based and computer-based PISA 2015 reading proficiency assessment, the latter differs from the former only in format, i.e., the way of presenting long texts by screen and the basic knowledge of hardware usage. However, compared with other cycles of the ICT familiarity questionnaire in the PISA computer-based assessment of reading, four derived variables were newly developed in the PISA 2015, including students’ ICT interest (INTICT), perceived competence in ICT usage (COMPICT), perceived autonomy related to ICT usage (AUTICT) and the degree to which ICT is part of their daily social life (SOIAICT). In particular, the index for ICT use outside of school for academic purposes has changed over time: in the PISA 2006 ICT familiarity questionnaire, this index includes five questions that mainly address students’ degree of using a computer to write papers, create spreadsheets, draw or use graphics programs, use educational software and write computer programs (OECD, 2009). In the PISA 2012, this index is examined using seven measurements of browsing the Internet for schoolwork, using email for communication with other students about schoolwork, using email for communication with teachers and the submission of homework, downloading, uploading or browsing material from the school’s website, checking the school’s website for announcements, doing homework on the computer, and sharing school-related materials with other students (OECD, 2015). Finally, in the PISA 2015, the index is derived from 12 measurements, including all seven measurements that were examined in the PISA 2012. In addition, students’ degrees of browsing the Internet to follow up lessons, using social networks for communication with other students and teachers about schoolwork, doing homework on a mobile device, and downloading learning apps on a mobile device are also included (OECD, 2017).
Substantial effort has been made to investigate the impacts of certain factors on students’ reading proficiency based on the PISA assessment framework. The previous studies can be divided into three categories. The first category is that of sociodemographic factors. Gender, family background and immigration background are confirmed to be significant sociodemographic factors of computer-based assessment measuring reading proficiency. Specifically, 15-year-old girls tend to score higher in computer-based reading assessments on multiple layers of reading skills than boys of the same age (e.g., Stoet and Geary, 2015; Puteh et al., 2016; Torppa et al., 2018). In addition, parental education (e.g., Rajchert et al., 2014), early parental engagement in educational activities (e.g., Hemmerechts et al., 2016), and parental involvement in social and cultural exchange (e.g., Gotoh et al., 2013) are found to be positive factors of reading performance. For immigrant background factors, immigrant students perform consistently worse than native students (e.g., Liberto, 2014), which can be explained by insufficient family support and the control of immigrants (Santos et al., 2016). In the meantime, it has also been found that the sense of school belonging exerts a moderating effect in the mathematical achievement gap between immigrants and natives (Schachner et al., 2017); however, for reading performance, this moderating effect turns out to be insignificant (Mok et al., 2016). The second category is related to cognitive factors. Cognitive skills (rapid naming, phonological awareness, and letter knowledge) and cognitive learning strategies (elaboration and memorization) positively influence reading proficiency (e.g., Li and Chun, 2012; Eklund et al., 2018). The third category concerns instructional factors. Categorical instructions or curricula targeting students of different reading levels improve their reading results (e.g., Shin et al., 2013). In addition, teachers’ guidance of students when they encounter difficulties in reading, teachers’ stimulation of students’ reading processes and the classroom reading environment are all meaningful factors influencing students’ reading proficiency (Meng et al., 2017).
Previous studies have constructed statistical models using the theoretically-based rationale that ICT impact factors are related to reading proficiency. For instance, to examine the mediation effect from individual differences in the inner and outer states of ICT to the PISA reading proficiency, a partial mediation model was constructed (Lee and Wu, 2012). An ordered logit model was employed to estimate relationships between an ordinal dependent variable (i.e., PISA test score) and a set of independent variables (i.e., the student’s background, school characteristics, the home/family environment and the student’s access to ICT facilities) (Erdogdu and Erdogdu, 2015). In these studies, special attention was given to student-level ICT impact factors. Student-level ICT impact factors were obtained from the ICT familiarity questionnaire, which has been gradually developed since the PISA 2000. In the PISA 2015 questionnaire, these factors can be generalized into three main categories: the availability of ICT, the use of ICT and attitudes toward ICT. With regard to the impact of ICT availability, the mere availability of ICT at home is negatively related to reading proficiency, whereas ICT availability at school is not significantly correlated with reading performance (e.g., Lee and Wu, 2012; Hu et al., 2018).
Two relevant contextual factors have been identified in the previous literature with a focus on the impact of ICT use on reading proficiency. The first involves where the ICT is used, i.e., at school or outside of school. The findings regarding ICT use at school are complex; the association between ICT use at school and students’ reading achievement is recognized as having an inverted U-shape, which indicates that overuse of ICT at school may reverse the positive correlation between ICT use at school and students’ reading proficiency (Woessmann and Fuchs, 2005); however, ICT use at school is also found to be negatively correlated with students’ reading proficiency (Petko et al., 2017). Furthermore, this relationship varies among students in different grades. In particular, ICT use at school is found to be positively associated with the reading performance of fourth-grade students whereas it is negatively correlated with that of eighth-grade students (Skryabin et al., 2015). With regard to the second contextual factor, ICT is used outside of school for social entertainment or for web navigation. Specifically, the dimension of social entertainment involves the accessing of email, collaborative gaming, and the use of social media. The dimension of information seeking on the Internet includes reading online news, using e-dictionaries, consulting online encyclopedias and browsing websites for practical information. Some researchers have found that online navigation activities outside of school improve students’ reading proficiency whereas leisure activities decrease it (e.g., Woessmann and Fuchs, 2005; Lee and Wu, 2013). In contrast, some scholars discover that ICT use for entertainment at home is positively correlated with students’ reading performance (Skryabin et al., 2015). Additionally, ICT use for leisure is found to narrow the gender gap in students’ reading scores (e.g., Cheung et al., 2013; Rasmusson and Åberg-Bengtsson, 2015).
Attitude is a significant psychological construct that inheres in or characterizes a person (Richard, 2016). With regard to the ICT attitudinal variables included in the ICT familiarity questionnaire of the PISA 2015, students’ attitudes were found to positively influence students’ reading performance (Lee and Wu, 2012; Petko et al., 2017). In contrast, attitudes toward ICT for social interaction are negatively associated with reading proficiency (Hu et al., 2018). Researchers have used different indexes of ICT attitudes based on the PISA ICT familiarity questionnaire that they selected. For instance, Lee and Wu (2012) obtained one attitudinal index derived from four indicators based on the PISA 2009 ICT familiarity questionnaire. Petko et al. (2017) applied positive attitude toward ICT as a learning tool (ICTATTPOS) derived from six indicators based on the questionnaire in the PISA 2012. Considering that the constructs of ICT attitudes applied in the previous studies are not yet fully developed, a more comprehensive ICT familiarity questionnaire of the PISA 2015 is utilized in the current study to analyze the impacts of students’ ICT-related attitudes; this questionnaire includes four explicit indexes: interest in ICT, perceived ICT competence, perceived autonomy in using ICT, and enjoyment of social communication using ICT (OECD, 2017).
Achieving excellence in education can greatly enhance the effectiveness of education (OECD, 2009); excellence involves more than a student’s inherent talent as it is also related to various interactive factors (Hu and Wei, 2018). Most of the abovementioned studies investigated the ICT impact factors of students’ reading proficiency in one or more countries; however, the literature on the representativeness of countries with excellent reading proficiency remains insufficient. The top-performing countries should receive particular attention since relevant findings would certainly offer innovative insights leading to educational excellence for educators and policymakers around the world (Jerrim, 2015). Certain previous studies have investigated the relationship between impact factors and excellent subject performance by students. For instance, pedagogical impact factors of 4th-grade students with excellent reading proficiency were identified based on Progress in International Reading Literacy Study (PIRLS) (Xiao and Hu, 2019). Regarding the PISA-based analysis, a set of impact factors influencing top students’ science performance was explored (e.g., Chen et al., 2019). However, few of these studies have targeted ICT impact factors and 15-year-olds’ reading proficiency in high-performing countries. Therefore, this study aimed to identify the correlation between ICT impact factors and early adolescents’ reading proficiency in high-performing countries based on the large-scale educational assessment of the PISA 2015. Although the examination of the high-performing countries versus the low-performing ones can maximize the research scope, such comparisons may lead to invalid conclusions and weak representations of educational success because of the polar socioeconomic situations in different countries (OECD, 2016). Therefore, the study’s research objective is to survey the impact of ICT factors on secondary school students’ reading performance in five representative countries with extremely high reading proficiency.
The sample was drawn from the PISA 2015 dataset1, which is the latest PISA dataset, released in December of 2017. Different from the previous cycles, the assessments of all three domains of science, reading and mathematics were mainly conducted on computers in the PISA 2015. Of the 72 countries/economies that participated in this international assessment, 57 countries/economies (including all 35 OECD members) completed the computer-based assessment (CBA) whereas the remaining 15 participants who lacked computer-test access used the paper-based alternatives. Questionnaires were administered to students, principals, teachers, and parents to obtain relevant contextual information. Only the CBA countries/economies could choose whether to take the ICT familiarity questionnaire (OECD, 2017).
In the case of PISA, students are categorized into seven proficiency levels for each domain based on their test scores: Level 1b is the lowest described level, then Level 1a, Level 2, Level 3 and so on up to Level 6 as the highest proficiency level. Students reaching Level 5 or 6 on the reading proficiency scale are referred to as top performers. Level 6 tasks are more challenging and rigorous than Level 5 tasks. Students reaching Level 6 are typically able to integrate information from multiple texts, understand connotations on a sophisticated level, and expertly handle unfamiliar ideas.
According to the statistical results of the PISA 2015, only countries with at least 2% of performers at Level 6 may be regarded as representative countries with excellent reading proficiency (OECD, 2016) because high-performing educational systems can present better teaching resources, stronger school leadership, higher academic standards, broader educational outcomes, more innovative educational reforms and more international vision than others (Deng and Gopinathan, 2016). Among the seven representative countries with excellent reading proficiency, Canada and Norway did not take the ICT familiarity questionnaire. Thus, in the current study, Singapore (3.600% of Level 6 performers), New Zealand (2.600% of Level 6 performers), Australia (2.000% of Level 6 performers), Finland (2.000% of Level 6 performers) and France (2.000% of Level 6 performers) were selected as the five sample countries across Asia, Europe, and Oceania. Considering the representativeness of these five countries, all students were taken into consideration without distinguishing high- from low-achieving performers. The data of 37,155 sample students were retrieved by Perl computing language version 5.28.2. Boys account for 49.433% of the sample, and girls account for 50.567% of the sample. The age range of the participants was between 15 years and 3 (complete) months and 16 years and 2 (complete) months, as strictly required by the PISA (OECD, 2016, p. 210). In addition, the percentage of individuals with ICT availability at home (ICTHOME) or at school (ICTSCH) is at least 98.640% in five countries, respectively. Students with access to ICT both at home and at school are shown as 99.890% in total. The demographic information is presented in Table 1.
As it is impossible for each student to complete all test items, the PISA 2015 computed 10 plausible values (PVs) of reading scores to measure students’ performance (see Table 2). The present study followed the recommendations for addressing PVs in international large-scale assessments (OECD, 2009; Rutkowski et al., 2010), considering all 10 PVs simultaneously as the dependent variables for the purpose of obtaining unbiased and stable estimates.
Table 2. Descriptive statistics of plausible values of reading proficiency in the PISA 2015 computer-based reading assessment.
This study included three categories of student-level ICT factors as regressors (see Table 3), i.e., the availability of ICT (at school and outside of school), the use of ICT (at school or outside of school for academic and leisure purposes), and attitudes toward ICT (students’ interest in ICT, perceived autonomy related to ICT, perceived ICT competence, and ICT use for social interaction). In addition, the binary variable Gender and the derived variable of students’ gender and economic, social and cultural status (ESCS) were also considered. Based on the theoretical rationale in this study, all variables related to ICT availability, ICT use and attitudes toward ICT were included in the following analyses.
Table 3. Descriptive statistics of ICT availability, ICT use, ICT attitudes and student background based on the PISA 2015 computer-based reading assessment.
A regression model that contains more than one regressor variable is called a multiple regression model (Montgomery and Runger, 2007). An MLR model is “typically employed to measure the effects of the explanatory variables on performance” (Fariña et al., 2015, p. 179). It can accurately reflect the correlations among factors, indicate the degree of fit, and improve the effect of the regression equation (Holmes and Rinaman, 2015). Linear relationships among the various factors can be analyzed intuitively and promptly by using multiple sets of data.
In this study, considering that students’ reading proficiency is associated with multiple factors, it is effective and realistic to estimate the dependent variable by using the optimal combination of multiple independent variables, which can be accurately realized by an MLR model, in line with recommendations for PISA data analysis (Rutkowski et al., 2010). The equation for MLR is
where
yi refers to the dependent variables,
β0 refers to the intercept, and
βp refers to the partial regression coefficient, which gauges the unit change in the dependent variable per unit increase in the factors on the condition that the rest of the factors remain unchanged.
𝜀 refers to the error term.
In the current study, MLR modeling was performed using R computing language version 3.5.02. The data analysis procedure was as follows:
First, the data preprocessing procedure was conducted. Large-scale assessments (e.g., the PISA), conducted in the context of item response theory (Cui et al., 2019), generally contain missing values. In this context, the aggr() function from the R Language package ‘VIM’ was used to visualize the number and proportion of missing values. Deleting the missing values is one solution when the missing rate is lower than 5% for each variable; however, this solution could not be used in this study due to the high missing rate of over 10%. Therefore, to ensure the maximum number of observations, the imputation of missing values was conducted in this study. Many researchers have advocated the use of missForest, a non-parametric method based on the randomForest model, in working with samples that involve different data types (Stekhoven and Bühlmann, 2012; Jin et al., 2015; Finch et al., 2016). Thus, because the sample included in this study contains both continuous and dichotomous variables, the missForest() function was used to impute the missing values.
Second, the correlation coefficients among the nine independent variables and ten PVs of reading performance were computed, and they were within the acceptable limits. Further, the T-value and the F-value needed to be emphasized to determine the correlation between nine independent variables and reading proficiency.
Third, the lm() function from the core package ‘stats’ was used to compute the MLR model. For each plausible value of reading performance, the model was built by the regressors and covariates.
The summary statistics of variables are presented in Table 3. As there were ten PVs, ten MLR models were eventually produced. The residuals (𝜀), estimates (β), intercept (β0), standard error (SE), multiple R-squared (R2) and p-values of the T-statistic and F-statistic are shown in the results for further discussion.
Fourth, assumptions of homoscedasticity and endogeneity were checked. Widely used to verify whether a regression model contains heteroskedastic error (Jeong and Lee, 2008), White’s test (White, 1980) was applied in this study by the computing heteroscedasticity-robust standard error in test statistics (Wooldridge, 2003). Moreover, the problem of endogeneity might exist when the ICT use is an endogenous variable (Fariña et al., 2015). Therefore, the assumption of endogeneity was checked with all three covariates of ICT use, i.e., USESCH, HOMESCH and ENTUSE. The differences between two regression models of with and without any of these covariates for the rest of the variables were calculated and provided in Supplementary Tables S1–S6, respectively. The comparisons of result difference of each ICT use variable were presented in Table 4. No significant differences were found with and without these variables, respectively, in this process.
Table 4. Comparison of the results of the regression models with and without each ICT use factor of USESCH, HOMESCH, and ENTUSE.
This article aimed to examine the influence of ICT impactors on students’ reading proficiency in high-achieving countries; therefore, the five representative countries were assessed as a cohort with high-achieving reading proficiency.
Regarding the PISA reading proficiency, the fundamental demographic factors involved the ESCS and gender (Petko et al., 2017; Hu et al., 2018). Thus, these two factors were included as the two demographic covariates in this study. Both ESCS (β = 47.930, SE = 0.663, p < 0.001) and gender (β = -28.506, SE = 1.039, p < 0.010) were significantly correlated with reading proficiency in Table 5. Specifically, ESCS was positively associated with the students’ reading performance. For a one-point increment in ESCS, the students’ reading scores increased by 39.398 points (β∗SD), which demonstrated that the students in countries with higher ESCS tended to achieve better reading results.
Table 5 presents the results for all required coefficients for the statistically significantly related factors included in the optimal MLR model. As shown, the explained variance for the model varied from R2 = 0.209 to R2 = 0.214. In the fields of humanities and social sciences, these R2 values were within an acceptable range because it was not expected that all relevant variables would be included to indicate the subjects’ behavior. In the existing studies of regression analysis using the PISA dataset (e.g., Chiacchio et al., 2016; Naumann and Sälzer, 2017; Tay et al., 2017), the maximum R2 reached 0.310, 0.239, and 0.230, respectively. Even if the R2 was low in this study, the factors were significantly correlated, which means that important conclusions could still be drawn from the model (Neter et al., 2012). The detailed information of all statistical analyses conducted in this study are available upon request.
As shown in Table 5, ICT availability at home (β = -4.331, SE = 0.396, p < 0.001) and at school (β = -3.265, SE = 0.295, p < 0.001) was negatively associated with students’ reading proficiency: with a one-point improvement in the availability of ICT at home and at school, students’ reading scores decreased by -7.094 and -6.308 points (β∗SD), respectively. Regarding use, ICT use at school in general (β = -7.536, SE = 0.779, p < 0.001) was negatively related to reading performance; reading scores were decreased by 6.225 points (β∗SD), with one-point growth in the use of ICT at school. The use of ICT outside of school for entertainment (β = -8.148, SE = 0.746, p < 0.001) indicated a negative correlation with reading proficiency; the use of ICT outside of school for entertainment was increased by one point, and reading scores dropped by 7.236 points (β∗SD). No significant association was found between the use of ICT outside of school for schoolwork and reading proficiency. With regard to students’ attitudes toward ICT, all attitudinal factors examined were significantly related to reading performance: interest in ICT (β = 9.955, SE = 0.661, p < 0.001) and perceived autonomy related to ICT use (β = 23.529, SE = 0.775, p < 0.001) were positively related to reading scores, whereas perceived ICT competence (β = -2.931, SE = 0.796, p < 0.001) and enjoyment of social interactions through ICT (β = -16.001, SE = 0.709, p < 0.001) were negatively associated with reading performance. Specifically, reading scores increased by 9.308 and 21.076 (β∗SD) points with every one-point increase in students’ interest in ICT and perceived ICT autonomy, respectively. Conversely, with a one-point improvement in perceived ICT competence, students’ reading score decreased by 2.597 points (β∗SD). One point of growth in their enjoyment of ICT use for social interaction was found to reduce reading scores by 14.065 points (β∗SD).
Moreover, the relationship between ICT impact factors and students’ reading proficiency in each of the five performing countries was also investigated through the same procedure, respectively, in Table 6. As shown, the ICT availability at home (ICTHOME) and the gender (Gender) remained negatively associated with students’ reading proficiency in each of the five counties, and the interest in ICT (INTICT) and the ESCS remained positively correlated with students’ reading proficiency in each of the five counties. For the remaining factors, they were differently associated with reading proficiency among different countries. These results indicated that: four factors (i.e., ICTHOME, INTICT, ESCS, and Gender) were simultaneously identified for all five countries as closely relevant to the students’ reading proficiency, whereas the other factors were differently associated with students’ reading proficiency among countries. For example, the ICT availability at school (ICTSCH) was negatively associated with reading proficiency in Australia (p = 0.021, β = -1.296, SE = 0.562), France (p < 0.001, β = -9.138, SE = 0.725) and New Zealand (p < 0.001, β = -3.478, SE = 0.978). The correlation was insignificant in Finland (p = 0.098) and Singapore (p = 0.068).
The availability of ICT includes ICT availability at home (ICTHOME) and ICT availability at school (ICTSCH) (OECD, 2016). On one hand, ICTHOME is found to be inversely related to students’ reading achievement in high-achieving countries, which is consistent with the previous research (Lee and Wu, 2013). This finding might be explained by the low quality of students’ ICT use at home without proper guidance and timely supervision from their parents. Students with access to ICT devices at home (e.g., computers, cell phones, e-books, printers, portable music players) do possess more computer skills (Kuhlemeier and Hemker, 2007) and tend to perform better on reading when assessed by computer (Rasmusson and Åberg-Bengtsson, 2015). However, the overuse or abuse of ICT tends to form detrimental habits such as addiction to computer games, which in turn lowers reading proficiency (Rasmusson and Åberg-Bengtsson, 2015). Hence, parents are suggested to carefully monitor their children’s access to ICT facilities at home and to appropriately direct them to utilize online resources in a reasonable way (Lee and Wu, 2012). On the other hand, ICTSCH is negatively correlated with students’ reading performance in this study, which is consistent with Lai’s (2016) study. This result is closely related to ICT use at school, which is discussed in detail in the next section.
The use of ICT contains ICT use at school in general (USESCH), ICT use at home for schoolwork (HOMESCH), and ICT use at home for leisure (ENTUSE) (OECD, 2016). ICT use at school is negatively related to students’ reading scores, which is consistent with the findings of previous studies (Petko et al., 2017; Tay et al., 2017; Hu et al., 2018). During the process of using ICT in everyday education, teachers may encounter a number of barriers. Ertmer (1999) classified these barriers into two categories: extrinsic and intrinsic barriers. Extrinsic barriers include lack of access, time, support, resources and training, and intrinsic barriers include attitudes, beliefs, practices and resistance. In terms of intrinsic barriers with regard to teachers’ preparedness and perception, although teachers believe ICT use in education is beneficial and may be able to adeptly use the Internet, e-mail, Microsoft Word and PowerPoint for reading teaching, they might possess only limited knowledge in using ICT for more advanced functions, e.g., spreadsheets, concept mapping, programing languages, multimedia authoring and modeling software to compose adapted teaching materials or tailored approaches for students with different reading levels. This indicates a situation where the use of ICT in class is restricted to basic pedagogical practices rather than being effectively integrated into the school curriculum (Aydin, 2013). Therefore, schools are supposed to organize training programs to equip teachers with important ICT knowledge and sufficient ICT skills as well as provide in-time technical support once teachers encounter any difficulty in using ICT in class and so forth (Hadi and Zeinab, 2012). In this case, teachers would be able to use ICT as cognitive tools in class, contributing to an ideal technology-assisted learning environment (e.g., Kommers et al., 2001; Nissen and Tea, 2012; Wei and Hu, 2018; Wei et al., 2018).
The results regarding the influence of the use of ICT for academic purposes outside of school on reading proficiency have varied across the previous studies. In this study, no significant connection is found between ICT use at home for schoolwork and reading proficiency. In the existing studies, Petko et al. (2017) discovered that ICT use for schoolwork outside of school is positively associated with students’ reading performance, which aligns with the research finding of Skryabin et al. (2015). In contrast, Gumus and Atalmis (2011) discovered the negative relationship of ICT academic use at home. These conflicting results might be explained by the fact that the PISA ICT questionnaire have changed over time, as explained in the introduction. In detail, Skryabin et al. (2015) and Petko et al. (2017) applied the ICT questionnaire in the PISA 2012, at which time the index for ICT use outside of school for academic purposes was determined by seven measurements. In Gumus and Atalmis (2011) study, this index was based on five questions in the PISA 2006 ICT questionnaire (OECD, 2006). However, in the current study, the final index of ICT use outside of school for schoolwork is derived from twelve indexes in the PISA 2015 ICT questionnaire, including all seven indexes that were examined in the PISA 2012 (OECD, 2017).
In this study, ICT use outside of school for entertainment is found to be inversely correlated with reading proficiency, which contradicts the findings of some of the past studies. For instance, Gumus and Atalmis (2011) proposed that using ICT devices for leisure, such as playing computer games, may alleviate Turkish students’ stress, increase their momentum, and inspire them to learn more efficiently. However, the pattern of a negative correlation between ICT use outside of school for entertainment and reading performance is found in high-achieving countries (Woessmann and Fuchs, 2005; OECD, 2006, 2015; Petko et al., 2017). Another possible explanation might be the opportunity cost of spending most of the time online outside school for entertainment rather than spending that time reading (Petko et al., 2017). Educators should devote more effort to monitoring and evaluating students’ reading strategies to achieve meaningful e-teaching outcomes. However, ICT use at school in Australia is positively correlated with students’ reading proficiency, which might be caused by the education policies in Australia. These policies contribute a lot to the effectively use of ICT in schools (Radhika and Wu, 2015).
Regarding attitudes toward ICT, two attitudinal factors, i.e., students’ interest and perceived autonomy in using ICT, are closely associated with the reading proficiency in high-performing countries in this study. This finding is novel, as few studies have confirmed the predominant significant role of ICT-related motivation and self-efficacy in reading scores beyond students’ capabilities. The previous studies have found that the impact of these two attitudinal factors on students’ achievement scores is complex (e.g., Papanastasiou et al., 2004; Lee and Wu, 2012). Lee and Wu (2012) observed that students’ perceptions of educational technology were positively correlated with their academic performance based on the PISA 2009 dataset whereas Papanastasiou et al. (2004) suggested a negative correlation. The reason for the fundamental influence of interest might be the digital learning potential reflected by the items measuring students’ interest in ICT in the PISA 2015 ICT familiarity questionnaire. This potential is measured by two main items: (1) The Internet is a great resource for obtaining information in which I am interested, and (2) I am really excited about discovering new digital devices or applications (OECD, 2017). In effect, these two questions reflect students’ acceptance of ICT related technology. ICT has brought tremendous change by offering readers the opportunity to engage in more flexible reading activities via computers. Nonetheless, many adolescents born in the 1990s have uninterested, skeptical or even fearful attitudes toward e-learning because of the complicated and misleading navigation, non-intuitive design, and user-unfriendly operations, which might hinder their access to informative resources (Hyman et al., 2014). This attitude of rejection decreases students’ autonomy in utilizing ICT facilities for learning. Students without an interest in applying ICT to help them with their work are unlikely to delve into the manuals of electronic devices, choose helpful applications or install updated learning software independently. Hence, students’ indifference to ICT shows little possibility for automatic e-learning in further study, which may hinder their reading performance. This interpretation seems plausible in light of the previous studies on the gender gap in online reading, which observe that the advantage in female students over their male counterparts in paper-based reading decreases when they read online. Based on Bandura’s self-efficacy theory (1993), it is possible that boys’ greater interest and girls’ higher anxiety in the electronic reading environment contributed to the smaller gender gap in digital reading (Nele and Franziska, 2019). Therefore, new effective and technological tools used in the classroom should be geared to students’ interest; in particular, attractive educational applications could trigger students’ positive attitudes or behavior in class (Mera et al., 2019).
With regard to students’ perceived ICT competence in using digital devices, this study finds a slightly negative association. In the meantime, students’ ICT use for social interaction is negatively correlated with reading proficiency in the sample countries. In the PISA 2015 ICT familiarity questionnaire, the questions on this index can be generalized into two categories. One category is ICT as a theme of social communication, and the other is ICT use for social interaction. Although students might receive assistance in using digital devices from social media, using ICT for social communication exerts a greater negative correlation with reading proficiency. This result is consistent with those of previous studies (e.g., Fox et al., 2009; Jacobsen and Forste, 2011) that confirmed that concurrent ICT use for social communication and for reading were negatively associated with their efficiency. Jacobsen and Forste (2011) further proposed that the metacognitive mechanism behind this negative correlation is the distraction of attention and the impairment of short-term memory when performing multiple tasks. Additionally, this finding further explains the negative impact of ICT use at school as mentioned above. Areepattamannil and Khine (2017) revealed the close connection between the frequency of ICT use for social interaction and ICT use at school. In this case, the fact that ICT’s use at school is negatively associated with reading scores is attributed not only to teachers’ behavior but also to students’ reading activities at school. To solve this problem, appropriate direction and timely scrutiny are necessary to prevent students from becoming obsessed with online entertainment such as playing computer games and engaging in social networking activities. The significant negative impact of social interaction activities on students’ reading proficiency in high-achieving countries reflects the fact that social media addiction poses a great threat to reading proficiency with the popularity of ICT.
This study used multiple linear regression models to analyze the relationship between ICT impact factors and early adolescents’ reading proficiency in five countries with extremely high reading proficiency. It was found that students’ attitudes toward ICT including interest levels and perceived autonomy contributed most to students’ high reading proficiency, rather than ICT availability or ICT use. The current study makes the following three primary contributions to the field: (a) This study delves into the association between the proposed ICT-related factors and students’ reading proficiency in the context of representative countries with excellent reading proficiency based on the latest PISA dataset, and it makes reasonable inferences for illustration; (b) The study reflects upon the application of educational technology in an ICT-assisted learning environment and gives constructive advice with regard to the findings; and (c) Based on the previous literature, this study offers a comprehensive overview of how ICT influences reading performance.
Future research should address a few suggestions. First, considering the exploratory nature of the research, if possible, in the future, longitudinal research can expand the scale of the research. Second, since most of the questions in the PISA questionnaire were the self-reported answers of students, the endogeneity of variables might be a problem. In a pioneering PISA study (Fariña et al., 2015), the Hausman test (Hausman, 1978) was used to diagnose the appropriateness of the endogeneity assumption. Furthermore, propensity score matching approach can be applied to avoid the self-selection problem and obtain an unbiased sample (e.g., Crespo-Cebada et al., 2014). Although this problem does not exist in this study, it still deserves special attention in future research. Additionally, the application of more advanced statistical model, for instance, a linear mixed-effects model (e.g., Hesselmann, 2018), is also essential for future PISA-based studies.
The data that support the findings of this study are available at http://www.oecd.org/pisa/data/. This is public data released by the OECD.
This study was approved by the Research Ethics Board of Zhejiang University and granting agency, and was performed in accordance with the relevant guidelines and regulations.
YX designed the study, analyzed and interpreted the data, and wrote and revised the manuscript. YL revised the manuscript. JH supervised the study, designed the study, interpreted the data, and wrote and revised the manuscript.
This study was partially funded by the National Education Science Youth Project for the 13th Five-Year Plan (Grant No. CIA170274).
The authors declare that the research was conducted in the absence of any commercial or financial relationships that could be construed as a potential conflict of interest.
The authors would like to thank the reviewers for their constructive comments on this manuscript. The authors also express sincere gratitude to the OECD for its generous publication of the data and the valuable suggestions on the methods of addressing the data.
The Supplementary Material for this article can be found online at: https://www.frontiersin.org/articles/10.3389/fpsyg.2019.01646/full#supplementary-material
Areepattamannil, S., and Khine, M. S. (2017). Early adolescents’ use of information and communication technologies (ICTs) for social communication in 20 countries: examining the roles of ICT-related behavioral and motivational characteristics. Comput. Hum. Behav. 73, 263–272. doi: 10.1016/j.chb.2017.03.058
Aydin, S. (2013). Teachers’ perceptions about the use of computers in EFL teaching and learning: the case of Turkey. Comput. Assist. Lang. Learn. 26, 214–233. doi: 10.1080/09588221.2012.654495
Chen, J., and Hu, J. (2018). Enhancing L2 learners’ critical thinking skills through a connectivism-based intelligent learning system. Int. J. Engl. Linguist. 8, 12–21. doi: 10.5539/ijel.v8n6p12
Chen, J., Zhang, Y., Wei, Y., and Hu, J. (2019). Discrimination of the contextual features of top performers in scientific literacy using a machine learning approach. Res. Sci. Educ. [Preprint]. doi: 10.1007/s11165-019-9835-y
Cheung, K. C., Mak, S. K., and Sit, P. S. (2013). Online reading activities and ICT use as mediating variables in explaining the gender difference in digital reading literacy: comparing hong kong and Korea. Asia-Pac. Educ. Res. 22, 709–720. doi: 10.1007/s40299-013-0077-x
Chiacchio, C. D., Stasio, S. D., and Fiorilli, C. (2016). Examining how motivation toward science contributes to omitting behaviors in the Italian PISA 2006 sample. Learn. Individ. Differ. 50, 56–63. doi: 10.1016/j.lindif.2016.06.025
Coiro, J., and Dobler, E. (2007). Exploring the online reading comprehension strategies used by sixth-grade skilled readers to search for and locate information on the internet. Read. Res. Q. 42, 214–257. doi: 10.1598/RRQ.42.2.2
Crespo-Cebada, E., Pedraja-Chaparro, F., and Santin, D. (2014). Does school ownership matter? An unbiased efficiency comparison for regions of Spain. J. Prod. Anal. 41, 153–172. doi: 10.1007/s11123-013-0338-y
Cui, X. J., Yang, Q. X., Li, B., Tang, J., Zhang, X. Y., Li, S., et al. (2019). Assessing the effectiveness of direct data merging strategy in long-term and large-scale pharmacometabonomics. Front. Pharmacol. 10:127. doi: 10.3389/fphar.2019.00127
Deng, Z., and Gopinathan, S. (2016). PISA and high-performing education systems: explaining Singapore’s education success. Comput. Educ. 52, 449–472. doi: 10.1080/03050068.2016.1219535
Eklund, K., Torppa, M., Sulkunen, S., Niemi, P., and Ahonen, T. (2018). Early cognitive predictors of PISA reading in children with and without family risk for dyslexia. Learn. Individ. Differ. 64, 94–103. doi: 10.1016/j.lindif.2018.04.012
Erdogdu, F., and Erdogdu, E. (2015). The impact of access to ICT, student background and school/home environment on academic success of students in Turkey: an international comparative analysis. Comput. Educ. 82, 26–49. doi: 10.1016/j.compedu.2014.10.023
Ertmer, P. (1999). Addressing first- and second -order barriers to change: strategies for technology intergration. Educ. Technol. Res. Dev. 47, 47–61. doi: 10.1007/BF02299597
Fariña, P., San Martín, E., Preiss, D. D., Claro, M., and Jara, I. (2015). Measuring the relation between computer use and reading literacy in the presence of endogeneity. Comput. Educ. 80, 176–186. doi: 10.1016/j.compedu.2014.08.010
Finch, W. H., Finch, M. E. H., and Singh, M. (2016). Data imputation algorithms for mixed variable types in large scale educational assessment: a comparison of random forest, multivariate imputation using chained equations, and MICE with recursive partitioning. Int. J. Quant. Res. Educ. 3, 129–153. doi: 10.1504/IJQRE.2016.077803
Fox, A. B., Rosen, J., and Crawford, M. (2009). Distractions: does instant messaging affect college students’ performance on a concurrent reading comprehension task? Cyberpsychol. Behav. 12, 51–53. doi: 10.1089/cpb.2008.0107
Gan, B., Menkhoff, T., and Smith, R. (2015). Enhancing students’ learning process through interactive digital media: new opportunities for collaborative learning. Comput. Hum. Behav. 51, 652–663. doi: 10.1016/j.chb.2014.12.048
Gotoh, H., Murota, M., and Kamiyama, A. (2013). A cross-national analysis of parental involvement and student literacy. Int. J. Comp. Sociol. 4, 246–266. doi: 10.1177/0020715213501183
Gumus, S., and Atalmis, E. H. (2011). Exploring the relationship between purpose of computer usage and reading skills of Turkish students: evidence from PISA 2006. Turk. Online J. Educ. 10, 129–140. doi: 10.1080/1475939X.2011.588414
Hadi, S., and Zeinab, S. (2012). Challenges for using ICT in education: teachers’ insights. Int. J. e-Educ. e-Busi. e-Mana. e-Learn. 2, 40–43.
Hausman, J. (1978). Specification test in econometrics. Econometrica 46, 1251–1271. doi: 10.2307/1913827
Hemmerechts, K., Agirdag, O., and Kavadias, D. (2016). The relationship between parental literacy involvement, socio-economic status and reading literacy. Educ. Rev. 69, 85–101. doi: 10.1080/00131911.2016.1164667
Hesselmann, G. (2018). Applying linear mixed effects models (LMMs) in within-participant designs with subjective trial-based assessments of awareness-a caveat. Front. Psychol. 9:788. doi: 10.3389/fpsyg.2018.00788
Holmes, W., and Rinaman, W. (2015). Multiple Linear Regression. Statistical Literacy for Clinical Practitioners. New York, NY: Springer International Publishing, 367–396.
Hu, J., and Wei, Y. (2018). Review of creativity and english language teaching: from inspiration to implementation. Engl. Today 35, 60–62. doi: 10.1017/S0266078418000299
Hu, X., Gong, Y., Lai, C., and Leung, F. K. S. (2018). The relationship between ICT and student literacy in mathematics, reading, and science across 44 countries: a multilevel analysis. Comput. Educ. 125, 1–13. doi: 10.1016/j.compedu.2018.05.021
Hyman, J. A., Moser, M. T., and Segala, L. N. (2014). Electronic reading and digital library technologies: understanding learner expectation and usage intent for mobile learning. Educ. Technol. Res. Dev. 62, 35–52. doi: 10.1007/s11423-013-9330-5
Jacobsen, W. C., and Forste, R. (2011). The wired generation: academic and social outcomes of electronic media use among university students. Cyberpsychol. Beh. Soc. N. 14, 275–280. doi: 10.1089/cyber.2010.0135
Jeong, J., and Lee, K. (2008). Bootstrapped White’s test for heteroskedasticity in regression models. Econ. Lett. 63, 261–267. doi: 10.1016/S0165-1765(99)00036-1
Jerrim, J. (2015). Why do East Asian children perform so well in PISA? An investigation of Western-born children of East Asian descent. Oxford Rev. Educ. 41, 310–333. doi: 10.1080/03054985.2015.1028525
Jin, Y., Li, B., Chen, N., Li, X., and Hu, J. (2015). The discrimination of learning styles by bayes-based statistics: an extended study on ILS system. Control Intel. Syst. 43, 68–75. doi: 10.2316/Journal.201.2015.2.201-2666
Kommers, P. A. M., Jonassen, D. H., and Mayes, J. T. (2001). Cognitive Tools for Learning. New York, NY: Springer-Verlag.
Kuhlemeier, H., and Hemker, B. (2007). The impact of computer use at home on students’ internet skills. Comput. Educ. 49, 460–480. doi: 10.1016/j.compedu.2005.10.004
Lai, Y. H. (2016). Investigation on the relationship between information communication technology and reading literacy for northeast asian students. MATEC Web Conf. 71:03007. doi: 10.1051/matecconf/20167103007
Lee, Y. H., and Wu, J. Y. (2012). The effect of individual differences in the inner and outer states of ICT on engagement in online reading activities and PISA 2009 reading literacy: exploring the relationship between the old and new reading literacy. Learn. Individ. Differ. 22, 336–342. doi: 10.1016/j.lindif.2012.01.007
Lee, Y. H., and Wu, J. Y. (2013). The indirect effects of online social entertainment and information seeking activities on reading literacy. Comput. Educ. 67, 168–177. doi: 10.1016/j.compedu.2013.03.001
Li, J., and Chun, C. K. (2012). Effects of learning strategies on student reading literacy performance. Read. Matrix 12, 30–38.
Liberto, A. D. (2014). Length of stay in the host country and educational achievement of immigrant students. Int. J. Manpow. 36, 585–618. doi: 10.1108/IJM-11-2013-0261
Liu, Z. M. (2005). Reading behavior in the digital environment: Changes in reading behavior over the past ten years. J. Doc. 61, 700–712. doi: 10.1108/00220410510632040
Mantoro, T., Fitri, E. M., Rusdah, R., Ayu, M. A., and Usino, W. (2017). The impact of information and communication technology (ICT) toward learning process and students’ attitudes. Adv. Sci. Lett. 23, 844–847. doi: 10.1166/asl.2017.7554
Meng, L., Muñoz, M., Hess, K. K., and Liu, S. (2017). Effective teaching factors and student reading strategies as predictors of student achievement in PISA 2009: the case of China and the United States. Educ. Rev. 69, 1–17. doi: 10.1080/00131911.2016.1155537
Mera, C., Ruiz, G., Aguilar, M., Aragón, E., Delgado, C., Menacho, I., et al. (2019). Coming together: R&D and children’s entertainment company in designing apps for learning early Math. Front. Psychol. 9:2751. doi: 10.3389/fpsyg.2018.02751
Mok, S. Y., Martiny, S. E., Gleibs, I. H., Keller, M. M., and Froehlich, L. (2016). The relationship between ethnic classroom composition and Turkish-origin and German students’ reading performance and sense of belonging. Front. Psychol. 7:1071. doi: 10.3389/fpsyg.2016.01071
Montgomery, D. C., and Runger, G. C. (2007). Applied Statistics and Probability for Engineers. New York, NY: John Wiley and Sons, Inc.
Naumann, J. (2015). A model of online reading engagement: linking engagement, navigation, and performance in digital reading. Comput. Hum. Behav. 53, 263–277. doi: 10.1016/j.chb.2015.06.051
Naumann, J., and Sälzer, C. (2017). Digital reading proficiency in German 15-year olds: evidence from PISA 2012. Z. Erziehwiss. 20, 585–603. doi: 10.1007/s11618-017-0758-y
Nele, M., and Franziska, S. (2019). Gender gap in reading digitally? Examining the role of motivation and self-concept. J. Educ. Res. Online 11, 145–165. doi: 10.1016/j.chb.2015.06.051
Neter, J., Kutner, M. H., Nachtsheim, C. J., and Wasserman, W. (2012). Applied Linear Statistical Model. Washington, DC: American Statistical Association.
Nissen, E., and Tea, E. (2012). Going blended: new challenges for second generation L2 tutors. Comput. Assist. Lang. Learn. 25, 145–163. doi: 10.1080/09588221.2011.636052
OECD (2006). Are Students Ready for a Technology-Rich World?: What PISA Studies Tell Us. Paris: OECD Publishing.
OECD (2011). PISA 2009 Results: Students Online: Digital Technologies and Performance, Vol. VI. Paris: OECD Publications.
Papanastasiou, E. C., Zembylas, M., and Vrasidas, C. (2004). “Reexamining patterns of negative computer-use and achievement relationships. Where and why do they exist?,” in Proceedings of the IRC-2014, TIMSS, ed. C. Papanastasiou (Nicosia, CY: IEA-ETS Research Institute), 127–138.
Petko, D., Cantieni, A., and Prasse, D. (2017). Perceived quality of educational technology matters: a secondary analysis of students’ ICT use, ICT-related attitudes, and PISA 2012 test scores. J. Educ. Comput. Res. 54, 1070–1091. doi: 10.1177/0735633116649373
Puteh, M., Zin, Z. M., and Ismail, I. (2016). Reading performance of Malaysian students across gender in PISA 2012. 3L-Lang. Linguist. Lit. 22, 109–121. doi: 10.17576/3L-2016-2202-08
Radhika, G., and Wu, M. (2015). Leaning too far? PISA, policy and Australia’s top “five” ambitions. Discourse Abingdon 36, 647–664. doi: 10.1080/01596306.2014.930020
Rajchert, J. M., Żułtak, T., and Smulczyk, M. (2014). Predicting reading literacy and its improvement in the polish national extension of the PISA study: the role of intelligence, trait- and state-anxiety, socio-economic status and school-type. Learn. Individ. Differ. 33, 1–11. doi: 10.1016/j.lindif.2014.04.003
Rasmusson, M., and Åberg-Bengtsson, L. (2015). Does performance in digital reading relate to computer game playing? A study of factor structure and gender patterns in 15-year-olds’ reading literacy performance. Scand. J. Educ. Res. 59, 691–709. doi: 10.1080/00313831.2014.965795
Richard, M. P. (2016). The Dynamics of Persuasion: Communication and Attitudes in the Twenty-First Century. New York, NY: Routledge.
Rutkowski, L., Gonzalez, E., Joncas, M., and Davier, M. V. (2010). International large scale assessment data issues in secondary analysis and reporting. Educ. Res. 39, 142–151. doi: 10.3102/0013189X10363170
Santos, M. A., Godas, A., Ferraces, M. J., and Lorenzo, M. (2016). Academic performance of native and immigrant students: a study focused on the perception of family support and control, school satisfaction, and learning environment. Front. Psychol. 7:1560. doi: 10.3389/fpsyg.2016.01560
Schachner, M. K., He, J., Heizmann, B., and Van de Vijver, F. J. R. (2017). Acculturation and school adjustment of immigrant youth in six european countries: findings from the programme for international student assessment (PISA). Front. Psychol. 8:649. doi: 10.3389/fpsyg.2017.00649
Sellar, S., and Lingard, B. (2013). The OECD and the expansion of PISA: new global modes of governance in education. Brit. Educ. Res. J. 40, 917–936. doi: 10.1002/berj.3120
Shin, S. H., Slater, C. L., and Backhoff, E. (2013). Principal perceptions and student achievement in reading in Korea, Mexico, and the united states: educational leadership, school autonomy, and use of test results. Educ. Adm. Q. 49, 489–527. doi: 10.1177/0013161X12458796
Skryabin, M., Zhang, J. J., Liu, L., and Zhang, D. (2015). How the ICT development level and usage influence student achievement in reading, mathematics, and science. Comput. Educ. 85, 49–58. doi: 10.1016/j.compedu.2015.02.004
Stekhoven, D. J., and Bühlmann, P. (2012). Missforest – non-parametric missing value imputation for mixed-type data. Bioinformatics 28, 112–118. doi: 10.1093/bioinformatics/btr597
Stoet, G., and Geary, D. C. (2015). Sex differences in academic achievement are not related to political, economic, or social equality. Intelligence 48, 137–151. doi: 10.1016/j.intell.2014.11.006
Tay, L. Y., Nair, S. S., and Lim, C. P. (2017). A regression analysis of elementary students’ ICT usage vis-à-vis access to technology in Singapore. Educ. Media Int. 54, 1–14. doi: 10.1080/09523987.2017.1324362
Torppa, M., Eklund, K., Sulkunen, S., Niemi, P., and Ahonen, T. (2018). Why do boys and girls perform differently on PISA reading in Finland? The effects of reading fluency, achievement behaviour, leisure reading and homework activity. J. Res. Read. 41, 122–139. doi: 10.1111/1467-9817.12103
Wei, Y., and Hu, J. (2018). A cross-sectional evaluation of EFL students’ critical thinking dispositions in digital learning. Adv. Soc. Sci. Educ. Hum. Res. 195, 27–30. doi: 10.2991/iserss-18.2018.8
Wei, Y., Yang, Q., Chen, J., and Hu, J. (2018). The exploration of a machine learning approach for the assessment of learning styles changes. Mechatron. Syst. Contr. 46, 121–126. doi: 10.2316/Journal.201.2018.3.201-2979
White, H. (1980). A heteroskedasticity-consistent covariance matrix estimator and a direct test for heteroscedasticity. Econometrica 48, 817–838. doi: 10.2307/1912934
Whyte, S., Schmid, E. C., Thompson, S. V. H., and Oberhofer, M. (2014). Open educational resources for call teacher education: the iTILT interactive whiteboard project. Comput. Assist. Lang. Learn. 27, 122–148. doi: 10.1080/09588221.2013.818558
Woessmann, L., and Fuchs, T. (2005). Computers and student learning: bivariate and multivariate evidence on the availability and use of computers at home and at school. CESifo Working Pap. S. 47, 339–347. doi: 10.1080/15391523.2005.10782441
Wooldridge, J. (2003). Introductory Econometrics: A Modern Approach, 5th Edn. Ohio: Thomson South-Western.
Keywords: ICT impact factors, reading proficiency, multiple linear regression, early adolescent, PISA 2015
Citation: Xiao Y, Liu Y and Hu J (2019) Regression Analysis of ICT Impact Factors on Early Adolescents’ Reading Proficiency in Five High-Performing Countries. Front. Psychol. 10:1646. doi: 10.3389/fpsyg.2019.01646
Received: 01 March 2019; Accepted: 28 June 2019;
Published: 16 July 2019.
Edited by:
Elisa Pedroli, Italian Institute for Auxology (IRCCS), ItalyReviewed by:
Paula Fariña, Diego Portales University, ChileCopyright © 2019 Xiao, Liu and Hu. This is an open-access article distributed under the terms of the Creative Commons Attribution License (CC BY). The use, distribution or reproduction in other forums is permitted, provided the original author(s) and the copyright owner(s) are credited and that the original publication in this journal is cited, in accordance with accepted academic practice. No use, distribution or reproduction is permitted which does not comply with these terms.
*Correspondence: Jie Hu, aHVqQHpqdS5lZHUuY24=
orcid.org/0000-0003-2219-2587
Disclaimer: All claims expressed in this article are solely those of the authors and do not necessarily represent those of their affiliated organizations, or those of the publisher, the editors and the reviewers. Any product that may be evaluated in this article or claim that may be made by its manufacturer is not guaranteed or endorsed by the publisher.
Research integrity at Frontiers
Learn more about the work of our research integrity team to safeguard the quality of each article we publish.