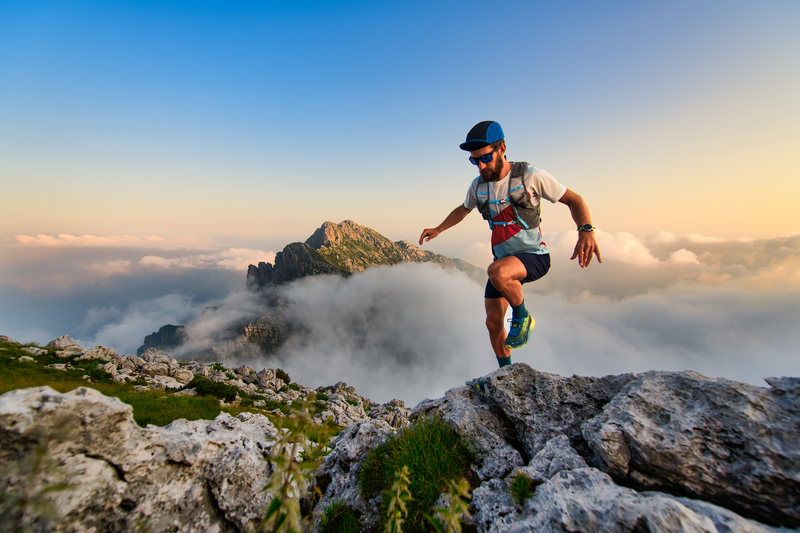
95% of researchers rate our articles as excellent or good
Learn more about the work of our research integrity team to safeguard the quality of each article we publish.
Find out more
ORIGINAL RESEARCH article
Front. Psychol. , 11 October 2017
Sec. Psychology for Clinical Settings
Volume 8 - 2017 | https://doi.org/10.3389/fpsyg.2017.01769
This article is part of the Research Topic Behaviour change: digital health and wellbeing View all 7 articles
Background: Patients prescribed with medication that treats mental health conditions benefit the most compared to those prescribed with other types of medication. However, they are also the most difficult to adhere. The development of mobile health (mHealth) applications (“apps”) to help patients monitor their adherence is fast growing but with limited evidence on their efficacy. There is no evidence on the content of these apps for patients taking psychotropic medication. The aim of this study is to identify and evaluate the aims and functioning of available apps that are aiming to help and educate patients to adhere to medication that treats mental health conditions.
Method: Three platform descriptions (Apple, Google, and Microsoft) were searched between October 2015 and February 2016. Included apps need to focus on adherence to medication that treats mental health conditions and use at least a reinforcement strategy. Descriptive information was extracted and apps evaluated on a number of assessment criteria using content analysis.
Results: Sixteen apps were identified. All apps included self-monitoring properties like reminders and psycho-educational properties like mood logs. It was unclear how the latter were used or how adherence was measured. Major barriers to medication adherence like patients' illness and medication beliefs and attitudes were not considered nor where information to patients about mediation side effects. Very few apps were tailored and none was developed based on established theories explaining the processes for successful medication adherence like cognitions and beliefs. Reported information on app development and validation was poor.
Discussion: A variety of apps with different properties that tackle both intentional and unintentional non-adherence from a different perspective are identified. An evidence-based approach and co-creation with patients is needed. This will ensure that the apps increase the possibility to impact on non-adherence. Theories like social cognition models can be useful in ensuring that patients' education, motivation, skills, beliefs, and type of adherence are taken into consideration when developing the apps. Findings from this study can help clinicians and patients make informed choices and pursue policy-makers to integrate evidence when developing future apps. Quality-assurance tools are needed to ensure the apps are systematically evaluated.
Medication adherence is usually defined as the extent that the medication taken reflects prescribed intention (Sanchez et al., 2005). Patients taking medication that treats mental health conditions have the greatest benefits when following the medication regimen compared to those prescribed with other types of medication (Zygmunt et al., 2002; Nose et al., 2003). At the same time, they are also usually difficult to adhere. For example, patients diagnosed with psychosis or depression adhere less to their medication compared to patients with physical illness (Cramer and Rosenheck, 1998).
The problem of non-adherence to medication that treats mental health conditions varies in prevalence, but is serious. Primary or continuous non-adherence ranges from 35 to 45% for patients with bipolar disorder (Colom et al., 2000; Rosa et al., 2007; Rakofsky et al., 2011), 50–60% for patients with schizophrenia (Lacro et al., 2002; Perkins, 2002), and 45% for patients with Post-Traumatic Stress Disorder (PTSD) (Rakofsky et al., 2011). The consequences of non-adherence to medication that treats mental health conditions can also be severe. For example, patients may experience increased risk of episode relapse, hospital readmission, and suicide (Angst et al., 2005; Berk et al., 2010; Murru et al., 2012). Non-adherence may also affect termination of treatment (Kemp et al., 2009) and recovery (Velligan et al., 2009). Patients may discontinue their medication and evidence are needed related to effective strategies aiming to educate and support patients with self-monitoring (Salomon and Hamilton, 2013).
The World Health Organization (WHO) published a guide for clinicians and policy makers, advising them to develop strategies in order to improve medication adherence (Sabaté, 2003). Currently in the US, the national hospital system allows clinicians to encourage their patients to use mobile health (mHealth) apps (Dayer et al., 2013). In the UK, the National Health System (NHS) introduced the Health App Library in 2013, to peer-review and list clinically safe and tailored apps for patients living in the UK. The UK national guidelines suggest tailoring support related to adherence to address the motivational and capability barriers of individuals. This approach aims to involve patients in decision making (National Institute for Health Clinical, Excellence, 2009). However, most successful interventions are usually expensive and complex (Burnier, 2000; Haynes et al., 2002; Murri et al., 2004) and mHealth apps can overcome this problem.
It is now established that patients with mental health conditions are equally likely to use mobile devices with the general population (Varshney and Vetter, 2012). The mHealth market is large with 158,000 available health-related apps (Research2guidance, 2016). These apps can be cost-effective and individually tailored to patients (Free et al., 2013). For example, combining new technologies to help patients with chronic conditions to adhere to medication has multiple benefits (Weingarten et al., 2002; Ofman et al., 2004). Despite the availability of mHealth apps, empirical analyses of their usability and benefits are lacking (Dayer et al., 2013). A recent study identified 229 available general medication adherence-related apps (Stawarz et al., 2014) but no similar evaluation is available for medication that treats mental health conditions. Also, even though using theoretical approaches can impact non-adherence (Horne and Weinman, 1999), it is not clear how these are operationalized in available apps.
Medication non-adherence can be unintentional (forgetting) with interventions focusing on shared goals as well as intentional (not wanting) with interventions focusing on relational strategies related to patients' goals, needs, and barriers. Interventions may have modest but significant impact and reinforcement strategies like reminders are recommended (Conn et al., 2015). The most effective, practical, and promising approaches targeting non-adherence to medication that treats mental health conditions come from combining psycho-educational interventions together with patient reminders (Mundt et al., 2001; Haynes et al., 2002; Pekkala and Merinder, 2002; Ran et al., 2003; Murri et al., 2004). Reminders usually do not involve providing the patient with information on their adherence (Dayer et al., 2013).
Using mHealth apps as reminders may also create a synergy between the patient and their clinician on decision-making. Nevertheless, very little evidence is available on how these apps support adherence using frameworks for evaluating the efficacy and effectiveness of complex interventions (Campbell et al., 2000; Craig et al., 2008) as well as evidence from non-mHealth complex adherence interventions (Nieuwlaat et al., 2014; Farmer et al., 2016).
Adhering to medication is a health behavior which relies on decisions based on cognitive processing (Lehane and McCarthy, 2007). Social cognition models like the Common Sense Model (Leventhal et al., 1992), Social Cognitive Theory (Bandura, 1986), the Theory of Planned Behavior (Ajzen, 1991), and the Health Belief Model (Rosenstock et al., 1988) can be used to explain cognitions related to medication non-adherence. These models pertain the assumption that beliefs about illness and medication influence how patients process information and experiences (Weinman and Petrie, 1997).
Higher intentional adherence is related to greater belief on the benefits of the treatment and adequate understanding of the illness and medication (Okuno et al., 2001; Lacro et al., 2002; Perkins, 2002; Kao and Liu, 2010; Levin et al., 2015). Improving these perceptions can also improve adherence through better doctor-patient communication (Okuno et al., 2001; Lacro et al., 2002). Leventhal's Common Sense Model suggests that patients will adhere if prescriptions make sense in light of their beliefs about medication and their past experiences (Leventhal et al., 1992). The Capability, Opportunity, and Motivation (COM-B) model suggests that health behaviors are influenced by how motivated and capable is an individual (Michie et al., 2011). Moreover, the Necessity-Concern model refers to how patients compromise their concerns against their perceived necessity to take their medication and has clinical benefit on explaining non-adherence (Horne and Weinman, 1999). One study suggests that this model may explain non-adherence more than any other risk factor (Kalichman et al., 2015). These models constitute useful frameworks when evaluating the effectiveness of interventions aiming at changing intentional non-adherence.
Smartphone apps usually target barriers to medication adherence, which are complex and interactive. Most of the barriers are patient-controlled and therefore, indicative of the patient's important role on following the clinicians' prescriptions (Osterberg and Blaschke, 2005). Patients' motivations and experiences are particularly relevant for medication that treats mental health conditions (Swarbrick and Roe, 2011). As a result, an individual's perceptions about medication and their illness can influence their intentional non-adherence. The use of mHealth apps for medication adherence comes with benefits for the patient (ease of access, mobility, opportunity for tailoring features) and potentially for the health system (cost-effectiveness by less use of the system's facilities).
Evidence of theoretical or empirical frameworks informing the medication adherence apps' properties is needed to allow for comparison between the apps. At the same time rigorous evaluation will determine their efficacy. Previous research is focused on general adherence apps (Dayer et al., 2013) whilst this study evaluates the apps' aims and functioning. The aim of this study is to review all mHealth apps available in 2016 targeting intentional non-adherence to medication that treats mental health conditions.
Smartphone apps focused on medication that treats mental health conditions for use by the general public were included in the search. The apps had to include multiple reinforcement strategies that target intentional non-adherence (i.e., reminder systems which target unintentional non-adherence only, with no other strategy included were not selected). Reinforcement strategies include properties like logs, reminders, and psycho-education. Finally, the search was restricted in apps that included a description in English in the web platforms irrespective of the apps' language functioning.
Three mobile platforms were screened, Apple App Store for iPhone, Google Play for Android and Microsoft Store for Microsoft smartphones. These platforms were searched since Apple and Google include the 90.6% of available medication adherence apps internationally (Dayer et al., 2013) whilst there is no previous evidence for available apps for Microsoft smartphones. Moreover, Apple and Google are the two most popular platforms that account for 90% of smartphone users (Malhotra, 2014). The following search keywords were used: “medication adherence,” “medication monitoring,” “medication compliance,” “dose,” “drug,” “med(s),” “medication(s),” “reminder,” “intake,” and “treatment.” All data were collected in October 2015 and an update followed in February 2016. The chosen period of time was pragmatic and reflects the availability in that specific period but the learning outcomes help with developing suggestions for future apps' development. It also follows from a previous review of generic adherence apps (Dayer et al., 2013). Three authors searched the platforms and screened the app descriptions independently and all apps that met the inclusion criteria were included. A fourth author crosschecked the apps to confirm eligibility. The most common reasons for excluding apps was: no focus on mental health and/or medication that treats mental health conditions (general medication apps), apps focusing on information provision only, no English description available in the platforms and apps for entertainment.
The descriptions that were available in the platforms through the searches were assessed and data extracted using two types of information: descriptive information and assessment criteria. Based on both types of information, an assessment form was developed for data extraction. The descriptive information assessed were: developer, developer's background (company, individual, or charity/non-governmental organization), country of origin, cost (free or not), platforms the app is available, targeted mental health condition(s), the latest update reported and descriptive information available in the online platforms.
The evidence from the app descriptions in the platforms were synthesized and evaluated based on a list of pre-defined criteria. The assessment criteria considered (Table 1) were derived from a scoping review of the literature related to using mHealth apps for medication adherence (Sackett and Haynes, 1976; Haynes and Sackett, 1979; Horne, 1998; Horne and Weinman, 1998; Osterberg and Blaschke, 2005), the NHS NICE guidelines for medication adherence (National Institute for Health and Clinical Excellence, 2009) and adherence-related theoretical frameworks like the COM-B, the Common Sense and the Necessity-Concern models. The assessment criteria were also evaluated in terms of their relevance and usefulness by one author (RH) with significant experience in medication adherence research. They were grouped into six groups: patient education/content, psycho-education/strategy, patient self-monitoring, personalization, interactivity, and “other.” The criteria are defined and operationalized in Table 1. Then, the online descriptions were searched for any reference to any peer-reviewed papers in order to determine the evidence-base for the apps' development, design and evaluation. Additionally, the apps' names were used as keywords in two popular health-related search engines, PubMed and PsycInfo.
Table 1. The assessment criteria for properties of smartphone applications for adherence to medication that treats mental health conditions.
Three authors performed all data extraction. Any discrepancies were resolved by consensus within group discussions with a fourth author. To increase reliability of assessment the 20% of assessment forms for each author was double-checked by a second author performing an independent assessment. Content analysis and critical appraisal were then performed on information available on the online descriptions of the identified apps. All emerging findings were discussed between the authors whose diverse experience and expertise facilitated the discussion of findings.
Using combinations of the keywords, eighty apps were identified as potentially eligible after removing duplicates. These were the apps that were identified using the keywords before screening based on the inclusion criteria. Sixteen unique smartphone apps were identified that met the eligibility criteria. The app characteristics are summarized in Table 2. Six apps were developed in the USA, two in Spain whereas one each was developed in Australia, Brazil, Canada, China, Egypt, India, Ireland, and the UK. The majority of apps were commercial and developed by companies (11, 68.75%), four were developed by charities or non-governmental organizations (25%) and one developed by an un-specified group of individuals (6.25%). Universities or other academic or research institutions developed none of the available apps. Half of the included apps were available for both Apple iPhone and Google Android devices (8, 50%), a third available for Google Android devices only (5, 31.25%), two available for Apple iOS, Google Android, and Windows devices (12.5%) and one for Apple iOS devices only (6.25%). Overall, the majority of apps were available to download for free (13, 81, 25%), with the remaining requiring a fee.
Among the apps, the range of update dates was between 2013 and 2016. Overall, one was updated in 2013, 5 in 2014, 5 in 2015 and 6 in 2016. Two apps had their iOS and Android versions update in different years (Medica reminders and ADDA Health Storylines). However, updates can be anything from scientific update to a technical (bug) update.
All of the available apps included more than one type of properties for medication adherence (Table 3). For example, Life Reboot—Fight Depression included only patient self-monitoring properties (i.e., medication reminders), while ADDA Health Storylines included all properties from the pre-specified assessment criteria except patient education/content. The patient self-monitoring group of criteria, which includes properties related with the ability of the patients to monitor their daily-prescribed medication use, was met in all 16 apps. Of the patient self-monitoring criteria, all apps used medication reminders (criterion G), and 14 included medication logs (criterion E, 87.5%). On the other hand, tailoring-related properties were found only in four applications (25%), representing the less applicable criterion. A third of the apps were developed in order to address all mental health conditions (6, 37.5%) or ADHD and/or ADD (5, 31.25%). In addition, all apps except one (My Mind Western Trust) included also information about other log properties (criterion F, 93.75%). Mood log and agenda/diary were the most frequently used extra logs (Table 4). Finally, only 2 apps (12.5%) were available in other languages than English (ADHD Adults and ADHD Kids).
Table 3. Properties of smartphone applications for adherence to medication that treats mental health conditions.
Table 4. Information about other log properties except medication logs available in smartphone applications for adherence to medication that treats mental health conditions.
In general the available apps were focused on self-monitoring and much less on psycho-education/strategy (Figure 1). Among the psycho-educational properties, mood logs were used in six apps (37.5%) but it is not clear how mood was used except in one app. The TARA app uses mood, anxiety, sleep, and stressors tracking to demonstrate graphically to the patient the positive impact of adherence (when the patient “feels better”). The mood logs were mostly used as a wider self-management technique and to warn the patient when they report lower levels of mood than the “standard thresholds.” No information is available on these thresholds. In the rest of the apps, like Start-Medication Manager for Depression, the mood logging aims to enable the patient to self-evaluate whether the app is improving their condition or not. Surprisingly there were interactivity properties in a number of apps but it was the patient who could share information with their health care providers rather than using an interactive channel with providers to enable communication.
Figure 1. Characteristics of available apps for adherence to medication that treats mental health conditions based on number of properties under each assessment criteria. The Y axis consists of number of properties related to each group of assessment criteria (outlined in detail in Table 3).
None of the available apps acknowledge or reference any peer-review study or any empirical-based method of development. Moreover, the input of health professionals is unclear even though in some cases they were involved in the development of the apps. In addition, when the search engines were searched (PubMed, Medline, PsycInfo) none of the apps were found to be validated or being in progress of validation. Finally, only one app reported using a validated screening measure (Start-Medication Manager for Depression) but none reported using a validated method of assessing medication adherence.
This study provides evidence about 16 smartphone apps across three different platforms aiming to educate or help patients adhere to medication that treats mental health conditions. Similarly to other types of medication adherence interventions, the smartphone apps focus on reminders, reinforcing techniques, psycho-education, providing information and counseling (Haynes et al., 1996; Graves et al., 2010). It is promising that included apps are not used only as reminders but combine a range of techniques. On the other hand, illness and medication beliefs are not taken into consideration and apps' development is not theory-based. Measuring adherence is one of the challenges in the field since no gold standard method exists. However, none of the available apps report a validated method of measuring adherence. A range of self-report measures exist with satisfactory reliability and validity which constitute possible options (Garfield et al., 2011). For example, a version of the Medication Adherence Report Scale (Horne and Weinman, 1999, 2002) or a Visual Analogue Scale can be used to assess whether the patient adheres to medication. Using medication logs can be useful in terms of providing information about the user's daily intake, but evidence suggests that combining two methods (in this case validated questionnaires and medication logs) can lead to a more valid assessment of patients' adherence (Barnestein-Fonseca et al., 2011). It is important to highlight that medication adherence is a relational issue and mHealth apps if validated can contribute toward shared decision-making. In this context, patients' goals and targets are explored with the clinician and the patient is able to monitor outcomes in a continuous learning process.
The COM-B model (Michie et al., 2011) can be used as useful framework when apps target patients' perceptions about medication and illness, their motivations or their ability to adhere (i.e., reminders). Collecting information about the patients' beliefs about illness and medication can help tailoring the app, which is the less common criterion. Tailoring can potentially improve both the sustainability of using the apps but also non-adherence, if the apps help with changing perceptions (Williams et al., 2008; Gatwood et al., 2014). Poor tailoring was also recently reported in a recent evaluation of health behavior change apps (McMillan et al., 2016).
The level that patients' emotions are taken into consideration in the included apps is not clear. For example, none of the apps makes use of patients' concerns toward medication. A third of the apps use mood logs for self-management but it is not clear how mood is used to improve non-adherence except in one (TARA: Mental Health Nurse for Android and TARA: Mood Log and Medication Reminder for iOS). An app may be more acceptable by patients if it takes into account how they feel and when. It is also surprising that information about coping with side effects is included only in two apps. Side effects can be a serious barrier to medication adherence and patients can benefit if they have information about their medication's side effects. Assessment of emotions, depression and anxiety is insufficiently used. App developers and researchers should consider using the Experience Sampling Method (Csikszentmihalyi and Larson, 2014) to reduce bias of assessment. Apps like PsyMate can be used as a point of reference to overcome validity and reliability biases of assessment of moods (Boyce, 2011).
Furthermore, none of the apps takes into account patients' perceptions about the necessity to take their medication even though this is one of the strongest predictors of intentional non-adherence (Horne and Weinman, 2002; Horne et al., 2004; Clifford et al., 2008). The Necessity-Concern Framework (Horne and Weinman, 1999) can be used in order to assess and modify patients' concerns of prescribed medication and how necessary they perceive them to be. If patients' perceptions are assessed the intervention can be targeted if the patient has negative perceptions that may compromise adherence. Successful ways of influencing these perceptions can be the focus of future research.
Even though the focus of this review is intentional non-adherence, there are properties that target unintentional non-adherence like reminders. Medication reminders are the most popular properties in the included apps. On the other hand, none of the apps is a medication reminder only—they combine reminders with other reinforcement techniques. This holistic approach designates both process-based and relational approaches next to reminders that target unintentional non-adherence. Therefore, there are various ways medication reminders can work to tackle prospective and retrospective memory which both influence adherence (Kliegel et al., 2008). Future applications may consider tailoring reminders and using theory-driven concepts and algorithms to determine timing of messages which was recently proposed (Gatwood et al., 2014). However, non-adherence may not be a one-dimensional concept; it can be intentional (consciously deciding) or unintentional (forgetting). Recent evidence suggest using both when designing interventions in clinical trials (Wroe, 2002; Atkins and Fallowfield, 2006; Clifford et al., 2008; Iihara et al., 2014). Even if it is positive that a number of apps use a variety of properties that stem from both types of adherence, very few apps assess the patients' type of non-adherence. Therefore, it is difficult to tailor the properties to whether the patient intentionally or unintentionally does not adhere to medication. For example, pill reminders can be helpful when non-adherence in unintentional but not when it is intentional. The efficacy of the apps can be compromised if reminders are provided to patients who intentionally do not adhere to their medication. Reminders may tackle unintentional adherence whilst psycho-educational properties may tackle intentional adherence.
Interventions using tailored apps combining psycho-educational properties with patient self-monitoring and reminders have the greatest potential to improve adherence (Williams et al., 2008). Moreover, educating patients can be one of the most effective approaches in medication adherence (Haynes et al., 2008; Conn et al., 2009). Psycho-education/strategy is used in half of the available apps but it is not clear what the content and validity of the psycho-education/strategy properties are for each app.
Furthermore, interactivity with health professionals and the patients' family are used in ten of the identified apps but with limited information on how this is implemented. For example, patients can email adherence reports to health professionals or share the report during the consultation. For the ADDA Storylines app there is also the opportunity to provide information immediately to the family of the patient. Interactivity has the potential to keep the health professionals and family informed if the patient is non-adherent in a peer-support model of patient education. More research is needed on how to safely and accurately share patients' information or help with referrals when psychological interventions may be needed or shared decision-making.
Addressing mental health conditions can be implemented more successfully using evidence-based treatments and properties. There was a call recently for Universities to adopt a more active role in the research and development of mHealth apps (Kumar et al., 2015). However, no academic institute or professional organization other than commercial companies and NGOs/Charities were involved in the development of the identified apps. This study confirms recent evidence that empirical analyses of the efficacy of medication adherence apps is lacking (Sposaro and Tyson, 2009; Wohlers et al., 2009; Dayer et al., 2013). Based on this, there is a need for studies to test the validity, utility, and efficacy of the available apps. In relation to implementation of apps into healthcare, a recent report suggested an Evidentiary Standards Model to ensure patient safety there is a need for at least two efficacy and two effectiveness trials and evidence on dissemination and cost-effectiveness (Tomlinson et al., 2013). On the other hand, it is possible that evidence was taken into consideration during the development of the apps but this is not clearly stated in the app descriptions and no peer-reviewed paper is available on apps' development.
There is also a need to publish the development process of the apps so that evaluation and validation is possible. Research and evaluation should take place during the development process, with input from health professionals, patients and application developers in order to improve the apps' feasibility and usability. “Co-creation” with patients during the development stage is necessary so that apps reflect patients' needs and so that acceptability is improved. This was demonstrated in a recent study with patients with schizophrenia, which revealed that usability testing led to overcoming system vulnerabilities (Ben-Zeev et al., 2013). It was also highlighted in a review of apps for health behavior change where evidence on collaboration with health professionals or patients and apps' evaluation was also lacking (McMillan et al., 2016). Even though studies on the efficacy of available mHealth apps for medication adherence are limited, the evidence demonstrates the potential for benefits for the patients if well designed and validated. It is important to note that it is positive that apps exist with different and diverse properties. Not all apps should have the same functioning but differently designed apps may work in different aspects of medication non-adherence.
To our knowledge, this is the first study that evaluates the content of apps related with adherence medication that treats mental health conditions but limitations should be taken into consideration. The study captures the market space at a given time (late 2015, early 2016). As a result, it provides the state of knowledge and availability of properties on that given time period because the three most popular web platforms with a large user base were reviewed. However, we acknowledge that this is a fast growing area and different apps might emerge. On the other hand, learning points from this study can inform future apps' development. The study is also limited by the fact that even though some of the apps were downloaded for testing the analysis is based on the app descriptions. However, users should be made aware of the app development process and the evidence taken into consideration before they download an app so they can make an informed choice. Only three platforms were reviewed and other smaller platforms may have other apps but more likely these small platforms will use the same apps with the ones reviewed. The study is restricted to apps with English language used in the web platforms. However, since the majority is developed in the USA, it is unlikely that a lot of apps were missed. In this study PubMed was used to search for papers referring the apps but papers in other databases may have been missed or papers referring to the apps in different names. However, the focus of the evaluation was on whether there is available evidence to ensure patient safety similarly to the Evidentiary Standards Model (Tomlinson et al., 2013). Finally, we may have missed some generic apps because of the restrictions created by the inclusion criteria. However, even if some apps are missed we are confident that the current review captures the majority that aim to help patients to adhere to medication and provides insights into how these are developed.
The area of mHealth can contribute toward personalized medicine. A more holistic approach which takes into consideration both intentional and unintentional non-adherence is useful to design effective mHealth apps. Strategies that target intentional non-adherence can also be useful in terms of medical tapering which refers to gradual discontinuation or reduction of medication. Medication tapering can be benefited by approaches that lead to shared-decision making. There is a need for scientifically and empirically validated apps combining research, clinical experience, and marketing policy. Moreover, systemic evaluation of existing apps in real-life conditions is necessary to provide evidence on how apps can be used within the current health system. Developers are encouraged to use an evidence-based approach in designing and developing similar apps. This study may inform mental health professionals who are encouraging the use of apps as well as the patients that use them to make an informed choice. The current study highlights the need for developing quality assurance tools for medication adherence apps. It can inform policy-makers on the need of integrating evidence-based knowledge into app development and evaluation and pushing for regulating and monitoring the large and fast growing mHealth field. Finally, more time should be spent in co-creating the apps with patients. If apps are validated, this may potentially influence clinicians' confidence in recommending them and policy makers into integrating these interventions into the local health systems.
Future apps that target intentional non-adherence need to improve patients' understanding of mental health conditions and medication attitudes (Kao and Liu, 2010; Levin et al., 2015) to address the negative attitudes toward daily medication and barriers of coping with daily routines (Sajatovic et al., 2009) and help patients setting their goals. Finally, it would be useful if apps could identify if the patient is intentionally or unintentionally non-adherent. Co-creation of apps with patients and health professionals is highlighted to increase the possibility of more efficacious, acceptable, and useful interventions (Camerini et al., 2013).
AK and GG conceived the study initially with the contribution of EP and AD in study design. The initial searches were conducted by GG, EP, and AD and discussed between the three authors and AK. The assessment criteria were drafted by AK and GG with RH contributing to validation. AK drafted the first draft with all authors contributing with critical comments. All authors approved the final draft.
The authors declare that the research was conducted in the absence of any commercial or financial relationships that could be construed as a potential conflict of interest.
The handling Editor declared a shared affiliation, though no other collaboration with two of the authors, APK and RH.
Thank you to Giorgos Iniatis and Silouanos Nicolaou for their comments.
Ajzen, I. (1991). The theory of planned behavior. Organ. Behav. Hum. Decis. Process. 50, 179–211. doi: 10.1016/0749-5978(91)90020-T
Angst, J., Angst, F., Gerber-Werder, R., and Gamma, A. (2005). Suicide in 406 mood-disorder patients with and without long-term medication: a 40 to 44 years' follow-up. Arch. Suicide Res. 9, 279–300. doi: 10.1080/13811110590929488
Atkins, L., and Fallowfield, L. (2006). Intentional and non-intentional non-adherence to medication amongst breast cancer patients. Eur. J. Cancer 42, 2271–2276. doi: 10.1016/j.ejca.2006.03.004
Bandura, A. (1986). Social Foundations of Thought and Action: A Social Cognitive Theory. New Jersey: Prentice-Hall, Inc.
Barnestein-Fonseca, P., Leiva-Fernández, J., Vidal-Espa-a, F., García-Ruiz, A., Prados-Torres, D., and Leiva-Fernández, F. (2011). Is it possible to diagnose the therapeutic adherence of patients with COPD in clinical practice? A cohort study. BMC Pulm. Med. 11:6. doi: 10.1186/1471-2466-11-6
Ben-Zeev, D., Kaiser, S. M., Brenner, C. J., Begale, M., Duffecy, J., and Mohr, D. C. (2013). Development and usability testing of FOCUS: a smartphone system for self-management of schizophrenia. Psychiatr. Rehabil. J. 36:289. doi: 10.1037/prj0000019
Berk, L., Hallam, K. T., Colom, F., Vieta, E., Hasty, M., Macneil, C., et al. (2010). Enhancing medication adherence in patients with bipolar disorder. Hum. Psychopharmacol. Clin. Exp. 25, 1–16. doi: 10.1002/hup.1081
Boyce, N. (2011). The lancet technology: August, 2011. ancet 378, 475. doi: 10.1016/S0140-6736(11)61237-3
Burnier, M. (2000). Long-term compliance with antihypertensive therapy: another facet of chronotherapeutics in hypertension. Blood Press. Monit. 5, S31–S34. doi: 10.1097/00126097-200005001-00006
Camerini, L., Camerini, A.-L., and Schulz, P. J. (2013). Do participation and personalization matter? A model-driven evaluation of an Internet-based patient education intervention for fibromyalgia patients. Patient Educ. Couns. 92, 229–234. doi: 10.1016/j.pec.2013.04.007
Campbell, M., Fitzpatrick, R., Haines, A., Kinmonth, A. L., Sandercock, P., Spiegelhalter, D., et al. (2000). Framework for design and evaluation of complex interventions to improve health. BMJ 321, 694–696. doi: 10.1136/bmj.321.7262.694
Clifford, S., Barber, N., and Horne, R. (2008). Understanding different beliefs held by adherers, unintentional nonadherers, and intentional nonadherers: application of the necessity–concerns framework. J. Psychosom. Res. 64, 41–46. doi: 10.1016/j.jpsychores.2007.05.004
Colom, F., Vieta, E., Martínez-Arán, A., Reinares, M., Benabarre, A., and Gastó, C. (2000). Clinical factors associated with treatment noncompliance in euthymic bipolar patients. J. Clin. Psychiatry 61, 549–55. doi: 10.4088/JCP.v61n0802
Conn, V. S., Hafdahl, A. R., Cooper, P. S., Ruppar, T. M., Mehr, D. R., and Russell, C. L. (2009). Interventions to improve medication adherence among older adults: meta-analysis of adherence outcomes among randomized controlled trials. Gerontologist 49, 447–462. doi: 10.1093/geront/gnp037
Conn, V. S., Ruppar, T. M., Enriquez, M., and Cooper, P. (2015). Medication adherence interventions that target subjects with adherence problems: systematic review and meta-analysis. Res. Soc. Adm. Pharm. 12, 218–246. doi: 10.1016/j.sapharm.2015.06.001
Craig, P., Dieppe, P., Macintyre, S., Michie, S., Nazareth, I., Petticrew, M., et al. (2008). Developing and evaluating complex interventions: the new Medical Research Council guidance. BMJ 337:a1655. doi: 10.1136/bmj.a1655
Cramer, J. A., and Rosenheck, R. (1998). Compliance with medication regimens for mental and physical disorders. Psychiatr. Serv. 49, 196–201. doi: 10.1176/ps.49.2.196
Csikszentmihalyi, M., and Larson, R. (2014). “Validity and reliability of the experience-sampling method,” in Flow and the Foundations of Positive Psychology (Springer), 35–54. Available onlne at: http://link.springer.com/10.1007/978-94-017-9088-8_3 (Accessed November 16, 2016).
Dayer, L., Heldenbrand, S., Anderson, P., Gubbins, P. O., and Martin, B. C. (2013). Smartphone medication adherence apps: potential benefits to patients and providers. J. Am. Pharm. Assoc. 53, 172. doi: 10.1331/JAPhA.2013.12202
Farmer, A. J., McSharry, J., Rowbotham, S., McGowan, L., Ricci-Cabello, I., and French, D. P. (2016). Effects of interventions promoting monitoring of medication use and brief messaging on medication adherence for people with Type 2 diabetes: a systematic review of randomized trials. Diabet. Med. 33, 565–579. doi: 10.1111/dme.12987.
Free, C., Phillips, G., Galli, L., Watson, L., Felix, L., Edwards, P., et al. (2013). The effectiveness of mobile-health technology-based health behaviour change or disease management interventions for health care consumers: a systematic review. PLoS Med. 10:e1001362. doi: 10.1371/journal.pmed.1001362
Garfield, S., Clifford, S., Eliasson, L., Barber, N., and Willson, A. (2011). Suitability of measures of self-reported medication adherence for routine clinical use: a systematic review. BMC Med. Res. Methodol. 11:149. doi: 10.1186/1471-2288-11-149
Gatwood, J., Balkrishnan, R., Erickson, S. R., An, L. C., Piette, J. D., and Farris, K. B. (2014). Addressing medication nonadherence by mobile phone: development and delivery of tailored messages. Res. Soc. Adm. Pharm. 10, 809–823. doi: 10.1016/j.sapharm.2014.01.002
Graves, M. M., Roberts, M. C., Rapoff, M., and Boyer, A. (2010). The efficacy of adherence interventions for chronically ill children: a meta-analytic review. J. Pediatr. Psychol. 35, 368–382. doi: 10.1093/jpepsy/jsp072
Haynes, R. B., Ackloo, E., Sahota, N., McDonald, H. P., and Yao, X. (2008). Interventions for enhancing medication adherence. Cochrane Database Syst. Rev. 16:CD000011. doi: 10.1002/14651858.CD000011.pub3
Haynes, R. B., and Sackett, D. L. (1979). Compliance in Health Care. Baltimore: Johns Hopkins University Press.
Haynes, R. B., McDonald, H., Garg, A. X., and Montague, P. (2002). Interventions for helping patients to follow prescriptions for medications. Cochrane Database Syst. Rev. 2002:CD000011. doi: 10.1002/14651858.CD000011
Haynes, R. B., McKibbon, K. A., and Kanani, R. (1996). Systematic review of randomised trials of interventions to assist patients to follow prescriptions for medications. Lancet 348, 383–386. doi: 10.1016/S0140-6736(96)01073-2
Horne, R. (1998). Adherence to Medication: A Review of Existing Research. Available online at: http://psycnet.apa.org/psycinfo/1998-07760-011 (Accessed October 14, 2015).
Horne, R., and Weinman, J. (1998). “Predicting treatment adherence,” in Adherence Treat. Med. Cond, ed Lynn Myers Kenny Midence (London: Harwood Academic Publishers) 25–50.
Horne, R., and Weinman, J. (1999). Patients' beliefs about prescribed medicines and their role in adherence to treatment in chronic physical illness. J. Psychosom. Res. 47, 555–567. doi: 10.1016/S0022-3999(99)00057-4
Horne, R., and Weinman, J. (2002). Self-regulation and self-management in asthma: exploring the role of illness perceptions and treatment beliefs in explaining non-adherence to preventer medication. Psychol. Health 17, 17–32. doi: 10.1080/08870440290001502
Horne, R., Buick, D., Fisher, M., Leake, H., Cooper, V., and Weinman, J. (2004). Doubts about necessity and concerns about adverse effects: identifying the types of beliefs that are associated with non-adherence to HAART. Int. J. STD. AIDS 15, 38–44. doi: 10.1258/095646204322637245
Iihara, N., Nishio, T., Okura, M., Anzai, H., Kagawa, M., Houchi, H., et al. (2014). Comparing patient dissatisfaction and rational judgment in intentional medication non-adherence versus unintentional non-adherence. J. Clin. Pharm. Ther. 39, 45–52. doi: 10.1111/jcpt.12100
Kalichman, S. C., Pellowski, J., Kegler, C., Cherry, C., and Kalichman, M. O. (2015). Medication adherence in people dually treated for HIV infection and mental health conditions: test of the medications beliefs framework. J. Behav. Med. 38, 632–641. doi: 10.1007/s10865-015-9633-6
Kao, Y.-C., and Liu, Y.-P. (2010). Compliance and schizophrenia: the predictive potential of insight into illness, symptoms, and side effects. Compr. Psychiatry 51, 557–565. doi: 10.1016/j.comppsych.2010.03.007
Kemp, D. E., Canan, F., Goldstein, B. I., and McIntyre, R. S. (2009). Long-acting risperidone: a review of its role in the treatment of bipolar disorder. Adv. Ther. 26, 588–599. doi: 10.1007/s12325-009-0039-0
Kliegel, M., Jäger, T., Altgassen, M., and Shum, D. (2008). “Clinical neuropsychology of prospective memory,” in Prospective Memory: Cognitive, Neuroscience, Developmental, and Applied Perspectives, eds M. Kliegel, M. A. McDaniel, and G. O. Einstein (New York, NY: Taylor & Francis Group; Lawrence Erlbaum Associates), 283–308.
Kumar, N., Khunger, M., Gupta, A., and Garg, N. (2015). A content analysis of smartphone–based applications for hypertension management. J. Am. Soc. Hypertens. 9, 130–136. doi: 10.1016/j.jash.2014.12.001
Lacro, J. P., Dunn, L. B., Dolder, C. R., Leckband, S. G., and Jeste, D. V. (2002). Prevalence of and risk factors for medication nonadherence in patients with schizophrenia: a comprehensive review of recent literature. J. Clin. Psychiatry 63, 892–909. doi: 10.4088/JCP.v63n1007
Lehane, E., and McCarthy, G. (2007). Intentional and unintentional medication non-adherence: a comprehensive framework for clinical research and practice? A discussion paper. Int. J. Nurs. Stud. 44, 1468–1477. doi: 10.1016/j.ijnurstu.2006.07.010
Leventhal, H., Diefenbach, M., and Leventhal, E. A. (1992). Illness cognition: using common sense to understand treatment adherence and affect cognition interactions. Cogn. Ther. Res. 16, 143–163. doi: 10.1007/BF01173486
Levin, J. B., Tatsuoka, C., Cassidy, K. A., Aebi, M. E., and Sajatovic, M. (2015). Trajectories of medication attitudes and adherence behavior change in non-adherent bipolar patients. Compr. Psychiatry 58, 29–36. doi: 10.1016/j.comppsych.2014.11.023
Malhotra, R. (2014). What to Develop - Android Apps or An iPhone Application? Valuecoders. Available online at: http://www.valuecoders.com/blog/technology-and-apps/android-apps-vs-iphone-application/ (Accessed February 29, 2016).
McMillan, B., Hickey, E., Patel, M. G., and Mitchell, C. (2016). Quality assessment of a sample of mobile app-based health behavior change interventions using a tool based on the National Institute of Health and Care Excellence behavior change guidance. Patient Educ. Couns. 99, 429–435. doi: 10.1016/j.pec.2015.10.023
Michie, S., van Stralen, M. M., and West, R. (2011). The behaviour change wheel: a new method for characterising and designing behaviour change interventions. Implement. Sci. 6:42 doi: 10.1186/1748-5908-6-42
Mundt, J. C., Clarke, G. N., Burroughs, D., Brenneman, D. O., and Griest, J. H. (2001). Effectiveness of antidepressant pharmacotherapy: the impact of medication compliance and patient education. Depress. Anxiety 13, 1–10. doi: 10.1002/1520-6394(2001)13:1<1::AID-DA1>3.0.CO;2-S
Murri, R., Ammassari, A., Trotta, M. P., De Luca, A., Melzi, S., Minardi, C., et al. (2004). Patient-reported and physician-estimated adherence to HAART. J. Gen. Intern. Med. 19, 1104–1110. doi: 10.1111/j.1525-1497.2004.30248.x
Murru, A., Pacchiarotti, I., Nivoli, A. M., Bonnin, C. M., Patrizi, B., Amann, B., et al. (2012). Rates and clinical correlates of treatment non-adherence in schizoaffective bipolar patients. Acta Psychiatr. Scand. 125, 412–418. doi: 10.1111/j.1600-0447.2012.01837.x
National Institute for Health Clinical, Excellence. (2009). Medicines Adherence: Involving Patients in Decisions about Prescribed Medicines and Supporting Adherence. NICE guidelines [CG76]. Available online at: https://www.nice.org.uk/guidance/cg76 (Accessed March 22, 2016).
Nieuwlaat, R., Wilczynski, N., Navarro, T., Hobson, N., Jeffery, R., Keepanasseril, A., et al. (2014). “Interventions for enhancing medication adherence,” in Cochrane Database of Systematic Reviews, (John Wiley & Sons, Ltd.). Available online at: http://onlinelibrary.wiley.com/doi/10.1002/14651858.CD000011.pub4/abstract (Accessed July 30, 2016).
Nose, M., Barbui, C., Gray, R., and Tansella, M. (2003). Clinical interventions for treatment non-adherence in psychosis: meta-analysis. Br. J. Psychiatry 183, 197–206. doi: 10.1192/bjp.183.3.197
Ofman, J. J., Badamgarav, E., Henning, J. M., Knight, K., Gano, A. D., Levan, R. K., et al. (2004). Does disease management improve clinical and economic outcomes in patients with chronic diseases? A systematic review. Am. J. Med. 117, 182–192. doi: 10.1016/j.amjmed.2004.03.018
Okuno, J., Yanagi, H., and Tomura, S. (2001). Is cognitive impairment a risk factor for poor compliance among Japanese elderly in the community? Eur. J. Clin. Pharmacol. 57, 589–594. doi: 10.1007/s002280100347
Osterberg, L., and Blaschke, T. (2005). Adherence to medication. N. Engl. J. Med. 353, 487–497. doi: 10.1056/NEJMra050100
Pekkala, E. T., and Merinder, L. B. (2002). Psychoeducation for schizophrenia. Cochrane Libr. 2011:CD002831. doi: 10.1002/14651858.CD002831
Perkins, D. O. (2002). Predictors of noncompliance in patients with schizophrenia. J. Clin. Psychiatry 63, 1121–1128. doi: 10.4088/JCP.v63n1206
Rakofsky, J. J., Levy, S. T., and Dunlop, B. W. (2011). Conceptualizing treatment nonadherence in patients with bipolar disorder and PTSD. CNS Spectr. 16, 11–20. doi: 10.1017/S1092852912000119
Ran, M.-S., Xiang, M.-Z., Chan, C. L., Leff, J., Simpson, P., Huang, M.-S., et al. (2003). Effectiveness of psychoeducational intervention for rural Chinese families experiencing schizophrenia. Soc. Psychiatry Psychiatr. Epidemiol. 38, 69–75. doi: 10.1007/s00127-003-0601-z
Research2guidance (2016). mHealth App Developer Economics 2016. Research2guidance. Available online at: http://research2guidance.com/product/mhealth-app-developer-economics-2016/ (Accessed November 16, 2016).
Rosa, A. R., Marco, M., Fachel, J. M., Kapczinski, F., Stein, A. T., and Barros, H. M. (2007). Correlation between drug treatment adherence and lithium treatment attitudes and knowledge by bipolar patients. Prog. Neuropsychopharmacol. Biol. Psychiatry 31, 217–224. doi: 10.1016/j.pnpbp.2006.08.007
Rosenstock, I. M., Strecher, V. J., and Becker, M. H. (1988). Social learning theory and the health belief model. Health Educ. Behav. 15, 175–183. doi: 10.1177/109019818801500203
Sabaté, E. (2003). Adherence to Long-Term Therapies: Evidence for Action. World Health Organization. Available online at: https://books.google.com/books?hl=en&lr=&id=kcYUTH8rPiwC&oi=fnd&pg=PR5&dq=sabate+2003+WHO+adherence+medication&ots=tA1Lhs-juX&sig=ewaJ3wip7O26JXEoQHJ9_xkijL4 (Accessed February 7, 2015).
Sackett, D. L., and Haynes, R. B. (eds.). (1976). Compliance with Therapeutic Regimens. Baltimore, MD: Johns Hopkins University Press.
Sajatovic, M., Ignacio, R. V., West, J. A., Cassidy, K. A., Safavi, R., Kilbourne, A. M., et al. (2009). Predictors of nonadherence among individuals with bipolar disorder receiving treatment in a community mental health clinic. Compr. Psychiatry 50, 100–107. doi: 10.1016/j.comppsych.2008.06.008
Salomon, C., and Hamilton, B. (2013). “All roads lead to medication?” Qualitative responses from an Australian first-person survey of antipsychotic discontinuation. Psychiatr. Rehabil. J. 36, 160–165. doi: 10.1037/prj0000001
Sanchez, R. J., Crismon, M. L., Barner, J. C., Bettinger, T., and Wilson, J. P. (2005). Assessment of adherence measures with different stimulants among children and adolescents. Pharmacother. J. Hum. Pharmacol. Drug Ther. 25, 909–917. doi: 10.1592/phco.2005.25.7.909
Sposaro, F., and Tyson, G. (2009). “iFall: an Android application for fall monitoring and response,” in Annual International Conference of the IEEE Engineering in Medicine and Biology Society, 6119–6122. Available online at: http://ieeexplore.ieee.org/xpls/abs_all.jsp?arnumber=5334912 (Accessed February 29, 2016).
Stawarz, K., Cox, A. L., and Blandford, A. (2014). “Don't forget your pill!: designing effective medication reminder apps that support users' daily routines,' in Proceedings of the 32nd Annual ACM Conference on Human Factors in Computing Systems (ACM), 2269–2278. Available online at: http://dl.acm.org/citation.cfm?id=2557079 (Accessed January 31, 2015).
Swarbrick, M., and Roe, D. (2011). Experiences and motives relative to psychiatric medication choice. Psychiatr. Rehabil. J. 35, 45–50. doi: 10.2975/35.1.2011.45.50
Tomlinson, M., Rotheram-Borus, M. J., Swartz, L., and Tsai, A. C. (2013). Scaling up mhealth: where is the evidence? PLoS Med. 10:e1001382. doi: 10.1371/journal.pmed.1001382
Varshney, U., and Vetter, R. (2012). “Medication adherence for patients with mental illness,” in Annual International Conference of the IEEE Engineering in Medicine and Biology Society, 2182–2185. Available online at: http://ieeexplore.ieee.org/xpls/abs_all.jsp?arnumber=6346394 (Accessed February 8, 2015).
Velligan, D. I., Weiden, P. J., Sajatovic, M., Scott, J., Carpenter, D., Ross, R., et al. (2009). The expert consensus guideline series: adherence problems in patients with serious and persistent mental illness. J. Clin. Psychiatry 70, 1–46. doi: 10.4088/JCP.7090su1cj
Weingarten, S. R., Henning, J. M., Badamgarav, E., Knight, K., Hasselblad, V., Gano, A. Jr., et al. (2002). Interventions used in disease management programmes for patients with chronic illness which ones work? Meta-analysis of published reports. BMJ 325:925. doi: 10.1136/bmj.325.7370.925
Weinman, J. A., and Petrie, K. J. (1997). “Perceptions of health and illness,” in Perceptions of Health and Illness, eds K. J. Petrie and J. A. Weinman (Amsterdam: Harwood Academic Publishers), 1–17.
Williams, A., Manias, E., and Walker, R. (2008). Interventions to improve medication adherence in people with multiple chronic conditions: a systematic review. J. Adv. Nurs. 63, 132–143. doi: 10.1111/j.1365-2648.2008.04656.x
Wohlers, E. M., Sirard, J. R., Barden, C. M., and Moon, J. K. (2009). “Smart phones are useful for food intake and physical activity surveys,” in Annual International Conference of the IEEE Engineering in Medicine and Biology Society, 5183–5186. Available online at: http://ieeexplore.ieee.org/xpls/abs_all.jsp?arnumber=5333721 (Accessed February 29, 2016).
Wroe, A. L. (2002). Intentional and unintentional non adherence: a study of decision making. J. Behav. Med. 25, 355–372. doi: 10.1023/A:1015866415552
Keywords: adherence, psychotropic, mHealth, smartphone, compliance, health education, mental health, digital health
Citation: Kassianos AP, Georgiou G, Papaconstantinou EP, Detzortzi A and Horne R (2017) Smartphone Applications for Educating and Helping Non-motivating Patients Adhere to Medication That Treats Mental Health Conditions: Aims and Functioning. Front. Psychol. 8:1769. doi: 10.3389/fpsyg.2017.01769
Received: 09 August 2016; Accepted: 25 September 2017;
Published: 11 October 2017.
Edited by:
Henry W. W. Potts, University College London, United KingdomReviewed by:
Stefano Manzo, Anima Research Institute, ItalyCopyright © 2017 Kassianos, Georgiou, Papaconstantinou, Detzortzi and Horne. This is an open-access article distributed under the terms of the Creative Commons Attribution License (CC BY). The use, distribution or reproduction in other forums is permitted, provided the original author(s) or licensor are credited and that the original publication in this journal is cited, in accordance with accepted academic practice. No use, distribution or reproduction is permitted which does not comply with these terms.
*Correspondence: Angelos P. Kassianos, YW5nZWxvcy5rYXNzaWFub3NAdWNsLmFjLnVr
Disclaimer: All claims expressed in this article are solely those of the authors and do not necessarily represent those of their affiliated organizations, or those of the publisher, the editors and the reviewers. Any product that may be evaluated in this article or claim that may be made by its manufacturer is not guaranteed or endorsed by the publisher.
Research integrity at Frontiers
Learn more about the work of our research integrity team to safeguard the quality of each article we publish.