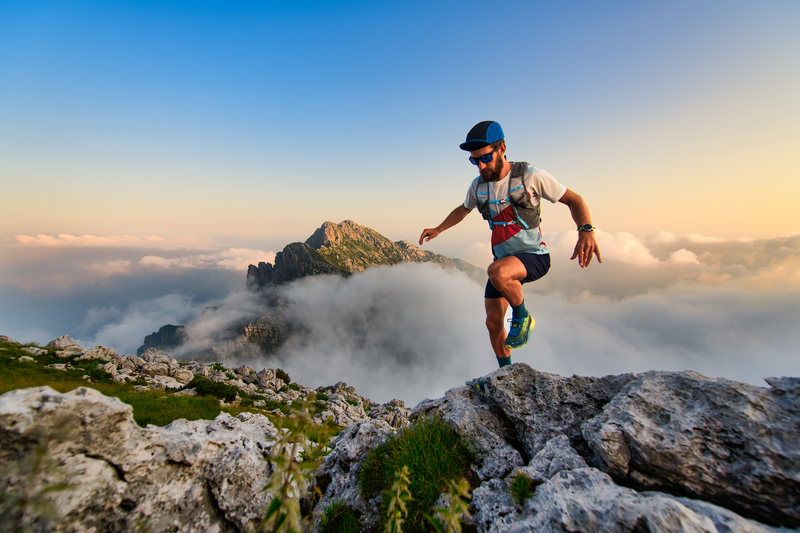
95% of researchers rate our articles as excellent or good
Learn more about the work of our research integrity team to safeguard the quality of each article we publish.
Find out more
ORIGINAL RESEARCH article
Front. Psychol. , 31 August 2016
Sec. Emotion Science
Volume 7 - 2016 | https://doi.org/10.3389/fpsyg.2016.01346
This article is part of the Research Topic Recognizing Microexpression: An Interdisciplinary Perspective View all 11 articles
A commentary has been posted on this article:
Commentary: Electrophysiological Evidence Reveals Differences between the Recognition of Microexpressions and Macroexpressions
Microexpressions are fleeting facial expressions that are important for judging people’s true emotions. Little is known about the neural mechanisms underlying the recognition of microexpressions (with duration of less than 200 ms) and macroexpressions (with duration of greater than 200 ms). We used an affective priming paradigm in which a picture of a facial expression is the prime and an emotional word is the target, and electroencephalogram (EEG) and event-related potentials (ERPs) to examine neural activities associated with recognizing microexpressions and macroexpressions. The results showed that there were significant main effects of duration and valence for N170/vertex positive potential. The main effect of congruence for N400 is also significant. Further, sLORETA showed that the brain regions responsible for these significant differences included the inferior temporal gyrus and widespread regions of the frontal lobe. Furthermore, the results suggested that the left hemisphere was more involved than the right hemisphere in processing a microexpression. The main effect of duration for the event-related spectral perturbation (ERSP) was significant, and the theta oscillations (4 to 8 Hz) increased in recognizing expressions with a duration of 40 ms compared with 300 ms. Thus, there are different EEG/ERPs neural mechanisms for recognizing microexpressions compared to recognizing macroexpressions.
Facial expressions serve important social functions, and the recognition of emotional facial expressions is vital for everyday life (Niedenthal and Brauer, 2012). However, emotion is not necessarily displayed on the face at all times. In a number of interpersonal situations, people hide, disguise, or inhibit their true feelings (Ekman, 1971), leading to partial or very rapid production of expressions of emotion, which are called microexpressions (Ekman and Friesen, 1969; Bhushan, 2015).
A microexpression is a facial expression that lasts between 1/25 and 1/5 of a second, revealing an emotion that a person is trying to conceal (Ekman and Friesen, 1969; Ekman, 1992, 2003; Porter and ten Brinke, 2008). A microexpression resembles one of the universal emotions: disgust, anger, fear, sadness, happiness, or surprise. Microexpressions usually occur in high-stakes situations in which people have something valuable to gain or lose (Ekman et al., 1992). According to Ekman (2009), microexpressions are believed to reflect a person’s true intent, especially one of a hostile nature. Therefore, microexpressions can provide an essential behavioral clue for lie detection and can be employed to detect a dangerous demeanor (Metzinger, 2006; Schubert, 2006; Weinberger, 2010).
Little is known regarding the characteristics that differentiate microexpressions and macroexpressions. The most important difference between microexpressions and macroexpressions is their duration (Svetieva, 2014). However, there are different estimates of the duration of microexpressions (Shen et al., 2012). According to Shen et al. (2012), there are at least six estimates of the duration of microexpressions, and 200 ms duration can be used as a boundary for differentiating microexpressions and macroexpressions. However, it is unclear whether there are different neural indicators for recognizing expressions with durations of less than 200 ms and those with durations of longer than 200 ms. If microexpressions and macroexpressions are qualitatively different (from the viewpoint of the perceiver, they should be recognized as different objects), then it can be expected that there are different brain mechanisms for processing facial expressions with a duration shorter than or longer than the duration boundary (200 ms).
Thus, we aimed to investigate the neural mechanisms for recognizing expressions with different durations, which can aid in the evidence-based separation of microexpressions from macroexpressions (i.e., to determine the boundary between microexpressions and macroexpressions). In other words, if differences in the neural characteristics that recognize one group of expressions with one kinds of duration and another group of expressions with other kinds of duration exist, we can say that the two groups of expressions are different. If we can find the discrepancy in the electroencephalogram (EEG)/event-related potentials (ERPs) between expressions with different durations, we can divide expressions with different EEG/ERPs characteristics into two groups. One group can be called microexpressions (with a short duration), and the other can be called macroexpressions (with a longer duration). Given the behavioral difference in recognition of microexpressions and macroexpressions and the disagreement regarding the conceptual definition for the duration of a microexpression, we seek to find electrophysiological evidence of the boundary (200 ms) that separates microexpressions from macroexpressions.
The EEG can indicate the characteristic temporal, spatial, and spectral signatures of specific cognitive processes. We explored the EEG activities during recognizing expressions with different duration (40, 120, 200, and 300 ms) to examine whether there is a turning point near 200 ms as indicated by the EEG measurements. Two different objects or ideas should only be thought of as separate entities when they have a number of differing characteristics. If neural differences are present before and after the turning point (e.g., 200 ms), then we can safely say that the duration of the conceptual definition of a microexpression is less than the turning point (the upper limit of microexpression duration). As there are different behavioral characteristics in the recognition of expressions with a duration of less than 200 ms and expressions with a duration longer than 200 ms (Shen et al., 2012), we hypothesized that recognizing expressions with a duration of less than 200 ms and expressions with a duration of longer than 200 ms will show different EEG characteristics (i.e., amplitude, oscillatory dynamics, and source location). Consequently, there should be different brain mechanisms for recognizing microexpressions and macroexpressions. Hence, the present study aimed to provide evidence for separating microexpressions and macroexpressions by investigating EEG/ERPs and synchronized oscillatory activity.
We used an affective priming paradigm, in which a picture of a facial expression is the prime and an emotional word is the target. Meanwhile, we mainly focused on the ERPs components of N400 and N170. The N400 can be produced not only in instances of semantic mismatch but also in other incongruous meaningful stimuli, such as words and faces. The effects of the N400 can also be observed in response to line drawings, pictures, and faces when primed by single items or sentence contexts, but not in the absence of priming (Kutas and Federmeier, 2011). In a pilot experiment, we found that the N400 could be elicited in the expression – emotional word priming paradigm. This N400 amplitude is more negative for incongruent than for congruent emotional content of face-word pairs. To produce a greater N400 effect, an incongruent condition in the experiment that elicits a greater negative-going wave than does the congruent condition should also be present. Therefore, we employed pictures of facial expressions (happy, fearful, and neutral) as priming stimuli and emotional words (positive and negative) as targets. Consequently, there were three conditions (congruent, incongruent, and control) with respect to the congruence of emotional valence.
Expressions can have different durations. There are expressions with short duration (e.g., less than 200 ms). If we recognize them as the same because of the limited time to process them, then during conditions of short duration for recognizing expressions, there is no congruence or incongruence due to expressions with different short durations being observed as the same by the participants. On the contrary, expressions with different long durations (e.g., greater than 200 ms) will appear to be different to the participants due to the extensive time for processing, which can result in congruence and incongruence. Thus, there will be no effect of congruence at the short duration; however, the effect of congruence at the long duration will be significant. To put it another way, when the presentation of an expression is transient, there is no top-down influences on the recognition of the expression. Consequently, there should be no difference between the congruent and incongruent conditions. Only when the duration of the expression is sufficiently long do the participants engage in top-down processing and recognize the expressions differently, which results in the congruent and incongruent conditions. Therefore, we could expect that there will be a significant relationship between duration and congruence while measuring N400 effects which reflect the top-down influences (Newman and Connolly, 2009). If we could extensively process expressions with short duration (less than 200 ms), meaning there was no difference between expressions with short and long duration, then there would be no relationship between duration and congruence.
For facial processing, one of the most prominent components in the ERPs is the N170 (Rossion and Jacques, 2011), and the face-sensitive N170 is modified by facial expressions of emotion (Batty and Taylor, 2003; Righart and De Gelder, 2008). As noted by Joyce and Rossion (2005), Eimer (2011), the N170 may be a vertex positive potential (VPP), resulting from changing the reference electrodes from the mastoid to the common average reference. The N170/VPP may be a valuable tool for studying the cognitive and neurobiological mechanisms underlying expression recognition. If there is a turning point in accuracy for recognizing expressions with different durations (i.e., there is a duration boundary for microexpressions and macroexpressions; see Shen et al., 2012), then we can expect that the main effect of duration will be significant. Specifically, there should be a significant difference between an expression with duration of less than 200 ms (microexpression) and an expression with duration of longer than 200 ms (macroexpression) while measuring N170/VPP. That is, there should be two groups of N170/VPP, one for microexpressions and one for macroexpressions (which can lead to a conclusion that expressions with short and long durations fall into two different categories).
It is worth noting that the priming paradigm provides an avenue for studying expression perception and recognition, which is appropriate for our aims. First, we wanted to investigate the effect of duration on the ERPs of expression recognition; when the duration of the expression is longer (macroexpression), the valence of the expression will be processed and the later processing of the emotional word will be facilitated or inhibited. Consequently, the N400 will reflect the facilitated or inhibited effect, i.e., there should be a smaller N400 when the valence of the expression and the emotional word are congruent. However, when the duration of expression is shorter (microexpression) the valence of the expression may not be fully processed, and there may be no facilitated or inhibited effect. Therefore, the N400 for the processing of microexpressions should not be affected, regardless of the congruence of the emotional valence. Second, this paradigm offers insights into the time course of the perception and the recognition of microexpressions and macroexpressions while measuring N170/ VPP.
The information regarding oscillatory dynamics from the EEG signal is largely lost by the time-locked averaging of single trials in the traditional ERPs approach. Researching functional correlates of brain oscillations is an important current trend in neuroscience. The traditional spectral analysis cannot fully address the issue of rapidly changing neural oscillations. Time–frequency analysis of an EEG allows researchers to study the changes of the signal spectrum over time, taking into account the power (or amplitude) of the EEG signal at a given frequency as well as changes in the phase or latency (Buzsáki, 2006; Roach and Mathalon, 2008; Güntekin and Baȿsar, 2014). Some recent studies investigated the mechanisms of perception and categorization of emotional stimuli through brain oscillatory activity extracted from EEG signals (Keil, 2013). Oscillatory dynamics of theta, alpha, beta, and gamma bands, and the interplay of these frequencies, relates to the processing of emotional stimuli (Güntekin and Baȿsar, 2014). Furthermore, some EEG studies show that the theta band activities, which are associated with subcortical brain regions and are considered to be the fingerprint of all limbic structures, are involved in affective processes (Knyazev and Slobodskaya, 2003). Meanwhile, theta band activity was observed during emotional stimulus presentation and it was associated with emotion comprehension (Balconi and Pozzoli, 2007). Therefore, this study mainly explores the dynamic oscillatory patterns of theta bands activities in the EEG signal while recognizing microexpressions and macroexpressions.
Previous studies (Esslen et al., 2004; Costa et al., 2014) had found that different emotional conditions had different activation patterns in different brain regions by using the low resolution brain electromagnetic tomography. In the current study, we also employed Standardized Low Resolution Brain Electromagnetic Tomography (sLORETA; Fuchs et al., 2002; Pascual-Marqui, 2002; Jurcak et al., 2007) to identify brain regions involved in recognizing expressions with long and short durations.
All experimental protocols were approved by the Institutional Review Board of the Institute of Psychology, Chinese Academy of Sciences. The methods were carried out in accordance with the approved guidelines.
Sixteen paid volunteers (8 female, ages 20 to 25 years, mean age = 22.3; 8 male, ages 22 to 24, mean age = 22.6) with no history of neurological injury or disorder were recruited from local college campuses. They gave written informed consent before participating. All participants had normal or corrected-to-normal vision and were predominantly right-handed (self-reported). Data from four participants containing too many artifacts were excluded from the analysis (including one participant with higher score of SDS, see the Results section), and the final analyses were conducted on twelve participants (7 female, mean age ± SD: 22.4 ± 1.4 years).
The pictures of faces consisted of 10 different individuals displaying fear (negative), happiness (positive) or a neutral expression; a total of 30 pictures of facial expressions were selected from 10 models taken from the Pictures of Facial Affect (POFA1). The emotional words consisted of 50 positive and 50 negative Chinese words selected from Wang and Fu (2011). The picture stimuli were 200 pixels × 300 pixels, and the word stimuli were 100 pixels × 150 pixels.
The stimuli were presented at a viewing distance of approximately 80 cm and displayed at a moderate contrast (black letters on a silver-gray background) in the center of a 17-inch computer screen with a refresh rate of 60 Hz. The experimental design was as follows: 4 durations (40, 120, 200, and 300 ms) × 3 congruencies (Congruence, Incongruence, and Control).
The participants were seated in a comfortable armchair in a dimly lit, sound damped booth. Emotional faces and words were presented using a priming paradigm. Subjects were asked to remember all of the content displayed on the screen to focus their attention on the task and to ensure the depth of processing of the words and pictures. No other tasks were imposed on the subjects during the ERPs recordings to avoid confounding the EEG for emotion processing with electrophysiological activity associated with motion for response selection and response execution. The experiment was divided into four blocks according to duration, with each lasting approximately 15 min. At the end of each block, the participants were given a test of recognition. After each block, the subjects were allowed to rest for 2 min. After the EEG recordings, each subject was asked to rate their mood using the Chinese version of the Zung Self Rating Anxiety and Depression Scales (SAS), SDS, selected from Wang et al. (1993).
Stimuli appeared one at a time in trials consisting of pictures of faces and emotional words. Four blocks were divided by the duration of exposure to the pictures of faces, which were 40, 120, 200, and 300 ms. Each trial consisted of a succession of stimuli: a fixation (with a duation randomly selected from 300 to 500 ms), a facial expression picture expressing one of the three emotions (with duration of 40, 120, 200, or 300 ms), a blank screen (the range in duration from 100 to 400 ms), one of the positive or negative emotional words (with duration of 1000 ms), and an interval (the range in duration from 1200 to 1500 ms). There were 300 trials per block. The order of presentation of the four blocks was randomized between subjects. The trial order within each block was randomized. At the end of each block, there was a recognition task (the participants had to judge whether some items including pictures and words were presented before), and the accuracy was measured to monitor the degree of cooperation of the participants. A break of approximately 2 min controlled by the participants separated each successive block.
Data were acquired from a 32-channel NuAmps Quickcap, 40-channel NuAmps DC amplifier and Scan 4.5 Acquisition Software (Compumedics Neuroscan, Inc., Charlotte, NC, USA). The EEG data were recorded from 30 scalp sites (Fp1, Fp2, F7, F8, F3, F4, FT7, FT8, T3, T4, FC3, FC4, C3, C4, CP3, CP4, TP7, TP8, T5, T6, P3, P4, O1, O2, Fz, FCz, Cz, CPz, Pz, and Oz). The NuAmps (Model 7181) amplifier had a fixed range of ±130 mV sampled with a 22-bit A/D converter, where the least significant bit was 0.063 μV. The impedance of the recording electrodes was monitored for each subject prior to data collection, and the threshold was always kept below 5 KΩ. The amplifier was set at a gain of 19, with a sampling rate of 1000 Hz and with a signal band limited to 70 Hz. In addition, no notch filter was applied. The electro-oculograms (EOG) were measured to exclude them from the EEG recordings. Vertical EOG (VEOG) was recorded by electrodes 2 cm above and below the left eye and in line with the pupil. The horizontal EOG (HEOG) was recorded by electrodes placed 2 cm from the outer canthi of both eyes. The ground electrode was positioned 10 mm anterior to Fz. The right mastoid electrode (M2) was used as the reference for all recordings and all data were offline re-referenced to a common average reference.
The EEG was later reconstructed into discrete, single-trial epochs. For analyzing the N170/VPP of facial expressions with different durations, an EEG epoch length of 400 ms was used, with a 100 ms pre-stimulus baseline and a 300 ms period, following the onset of the emotional faces. EEG epochs that exceeded ±100 μV were excluded, all trials were visually scanned for further artifacts generated by non-cerebral sources, and corrections were made for eye blinks. Participants had no fewer than 90 accepted epochs in any condition. The accepted epochs were recomputed to the average reference offline and were baseline corrected. The ERPs were averaged separately for each experimental condition. For the averaged N170/VPP wave, a mean amplitude measure within a 140–200 ms time window from onset of the facial stimuli of each participant was provided. The mean amplitude of the N170/VPP then was analyzed by a repeated-measures analyses of variance (ANOVA), in which the factors Valence (positive, negative, and neutral) × Duration (40, 120, 200, and 300 ms) to the mean amplitude were compared.
Facial stimuli under the incongruent condition elicited greater centroparietal ERPs negativity than those under the congruent condition. We termed this negative-going waveform as N400. For this ERPs wave, the epoch length of 1000 ms was used, with a 200 ms pre-stimulus baseline and an 800 ms period, following the onset of the emotional words. A mean amplitude measure within a 350–500 ms time window from the emotional word stimulus onset was provided. The time window of the N400 was selected by visually inspecting, and it more closely resembled a conventional time window of N400. An ANOVA was performed on the N400 mean amplitude.
The N400 was typically maximal over the centro-parietal electrode sites. Therefore, electrodes Cz and CPz were selected for further N400 statistical analysis (one-way analysis of variance, ANOVA), which was carried out on the mean N400 amplitude measurements at the midline central (Cz) and parietal (CPz) electrode locations separately, in which the factors Condition (congruent, incongruent, and control) × Duration (40, 120, 200, and 300 ms) were compared to the N400 mean amplitude. A Greenhouse–Geisser correction to p-values was used when appropriate to decrease the risk of falsely significant results.
Time–frequency analysis can be used to reveal event-related oscillations properties, which cannot be depicted by ERPs (Roach and Mathalon, 2008). Time–frequency analysis can represent the energy content of the EEG signal time-locked to an event in the joint time–frequency domain, in which a complex number is estimated for each time point in the time-domain signal, yielding both time and frequency domain information. According to the time–frequency decomposition, the Event-Related Spectral Perturbation [ERSP, the mean change in spectral power (in dB) compared to baseline] analysis was performed (see Makeig et al., 2004; Roach and Mathalon, 2008), particularly the ERSP of theta band activities were analyzed based on the analysis in the introduction. The eeglab 13 (Delorme and Makeig, 2004) was employed for the time–frequency analysis.
To compare cortical source differences between EEG activities of expressions with a long duration (>200 ms, macroexpressions) and expressions with a short duration (<200 ms, microexpressions), the standardized low resolution brain electromagnetic tomography (sLORETA) software (publicly available free academic software2) was used to estimate the underlying source activity by an equivalent distributed linear inverse solution (Pascual-Marqui et al., 1994, 1999, 2002). sLORETA is an improvement over the previously developed tomography LORETA (Pascual-Marqui et al., 1994). LORETA solves the “inverse problem” by finding the smoothest of all solutions with no a priori assumptions about the number, location, or orientation of the generators. It is important to emphasize that sLORETA has no localization bias even in the presence of measurement and biological noise (Pascual-Marqui et al., 2002).
In the current implementation of sLORETA, computations were performed in a realistic head model (Fuchs et al., 2002) using the MNI152 template (Mazziotta et al., 2001), with the three-dimensional solution space restricted to cortical gray matter as determined by the probabilistic Talairach atlas (Lancaster et al., 2000). The standard electrode positions on the MNI152 scalp were taken from Jurcak et al. (2007) and Oostenveld and Praamstra (2001). The intracerebral volume was partitioned in 6239 voxels at a 5 mm spatial resolution. To find the underlying neural generator activity that was most likely responsible for the differences in the recorded scalp potentials, sLORETA calculated the current density (A/m2) at each voxel allocated by a dipolar source.
To find the brain regions that are most likely involved in processing expressions with different durations, we calculated difference waves by subtracting the N170/VPP for 300 ms trials from the N170/VPP for 40 ms trials during a time window of 140–200 ms. Similarly, we calculated difference waves by subtracting the N400 of incongruent trials from the N400 of congruent trials during a time window of 350–500 ms.
In the survey of the Chinese version of the Zung Self Rating Anxiety and Depression Scales [SAS, SDS, cf., Lui et al. (2009), all scores of our participants were below the critical value of 50 for SAS (mean score = 35.9, SD = 5.7), and the scores of all but one participant (who scored 58 and was excluded from further analysis) were under the critical value of 53 for the SDS (mean score = 41.5, SD = 7.4). The results of the SAS and SDS clearly demonstrated the participants’ normal mood state. All the participants reached accuracy of greater than 80% during all the recognition tasks.
The face-sensitive potential of VPP was maximal at the central electrode sites. Therefore, electrodes Cz and CPz were selected for statistical analysis. A 2 Channel (Cz and CPz) × 4 durations (40, 120, 200, and 300 ms) × 3 valence (happiness, fear, and neutral) repeated measures analysis of variance (ANOVA) was conducted. The main effect of Channel is significant, F(1,11) = 5.567, p = 0.038, ηp2 = 0.336; the main effects of duration and valence are both significant, F(3,33) = 4.176, p = 0.037, ηp2 = 0.275; F(2,22) = 10.412, p = 0.001, ηp2 = 0.486. In order to better evaluate the effect of duration and valence on the N170/VPP effect, another ANOVA was conducted for Cz and CPz electrodes separately.
For electrode Cz, there was a main effect of duration, F(3,33) = 5.027, p = 0.006, ηp2 = 0.314. There was also a main effect of valence, F(2,22) = 10.824, p = 0.001, ηp2 = 0.496, and a significant interaction was present, F(6,66) = 2.766, p = 0.018, ηp2 = 0.201. Follow-up t-tests indicated that there is no difference between the N170/VPP mean amplitudes of happiness and fear at duration of 40 and 300 ms [t(11) = -0.166; p < 0.871; t(11) = -1.006; p < 0.336]. However, the N170/VPP mean amplitudes of happiness was bigger than that of fear at duration of 120 and 200 ms [t(11) = -3.612; p = 0.004; t(11) = -4.127; p = 0.002]. Planned comparisons of durations showed that the N170/VPP amplitude was larger for 40 ms than for 200 ms (p = 0.022, see Figure 1A). Pairwise comparisons of valence revealed that the N170/VPP amplitude was larger for fearful than for happy faces (p = 0.004). There was no difference between other pairings. For the electrode CPz, there was a main effect of duration, F(3,33) = 2.965, p = 0.046, ηp2 = 0.212. There was also a main effect of valence, F(2,22) = 6.628, p = 0.006, ηp2 = 0.376; however, there were no significant interactions, F(6,66) = 1.002, p = 0.432, ηp2 = 0.083. Figure 1 illustrates the grand average waveforms of N170/VPP at the electrodes Cz and CPz (Panel A). The scalp potential 3D maps of mean amplitude at 140–200 ms for the four corresponding levels of duration are depicted in Panel B).
FIGURE 1. The electroencephalogram (EEG)/event-related potentials (ERPs) results at the Cz and CPz electrode sites. (A) The grand-averaged ERPs waveforms (N170/VPP) elicited by a fleeting facial expression with a duration of 40 (green solid), 120 (red solid), 200 (blue dashed), and 300 ms (purple dashed) at the Cz and CPz electrode sites. (B) Scalp potential 3D maps reveal the topography of the N170/VPP for the time window (140–200 ms). (C) Event-Related Spectral Perturbation (ERSP) plot showing the mean increases or decreases in spectral power following stimulation. Non-green areas in the time/frequency plane show significant (p < 0.01) post-stimulus increases or decreases (see color scale) in log spectral power at the CPz electrode site relative to the mean power in the averaged 1-s pre-stimulus baseline (the interval for the ERSP analysis was –1000–1500 ms).
As shown in Panel C of Figure 1, the results of the ERSP showed that the mean post-stimulus spectral power for fleeting facial expressions with durations of 40 and 120 ms were similar (see the solid red box), and facial expressions with durations of 200 and 300 ms had a similar ERSP pattern (see the dashed purple box).
As shown in Figure 1C, the amplitude of theta response (4 to 8 Hz, as traditionally employed based on Berger’s studies; see Buzsáki, 2006) was higher for expressions with short duration (<200 ms) than for expressions with longer duration (>200 ms). Therefore, data of theta band activities from 100 to 260 ms of CPz were exported for performing a one-way ANOVA with repeated measures. The results showed that there was a main effect of duration, F(3,33) = 3.238, p = 0.035, ηp2 = 0.227. A post hoc pairwise comparison of the theta response of expressions with four levels of duration showed that theta band activity of recognition for expressions with a duration of 40 ms was significantly higher than that of 200 and 300 ms (p = 0.006; p = 0.039). The comparisons found no significant difference in the theta response for pairs of expressions with durations of 40 and 120 ms or pairs of expressions with durations of 200 and 300 ms (p = 0.308; p = 0.920).
Based on the scalp-recorded electric potential distribution, sLORETA was used to compute the cortical three-dimensional distribution of the current density of facial expressions with different durations. First, we explored standardized current density maxima for facial expressions with durations of 40, 120, 200, and 300 ms. All durations showed the same activation areas (fusiform gyrus, BA 20). To identify possible differences in the N170/VPP neural activation between the groups with durations of 40 and 300 ms, non-parametric statistical analyses of functional sLORETA images (Statistical non-Parametric Mapping; SnPM, c.f. Nichols and Holmes, 2002) were performed for the paired group while employing a t statistic (on log-transformed data). The results corresponded to maps of t statistics for each voxel, for a corrected p < 0.05. Figure 2 shows sLORETA statistical non-parametric maps comparing the electric neuronal activity of recognizing expressions with durations of 40 and 300 ms at the N170/VPP latency of 140 to 200 ms. The Figure 2 shows that the most active area of the cortex localized in the left hemisphere, in the Superior Frontal Gyrus (Brodmann area 8).
FIGURE 2. The estimated sources of N170/VPP during a time window of 140–200 ms. sLORETA-based statistical non-parametric maps (SnPM) comparing the standardized current density values between facial expressions with durations of 40 and 300 ms (n = 12) at the N170/VPP latency (140–200 ms). Significantly increased activation (p < 0.05) at the 40 ms duration compared to the 300 ms duration is shown in red. Each map consists of axial, sagittal, and coronal planes. The maxima are color coded as yellow. L, left; R, right; A, anterior; P, posterior.
An ANOVA on the factors of duration (40, 120, 200, and 300 ms) and congruence (congruent, incongruent, and control) was performed on the mean amplitude (350–500 ms) of the N400 to determine whether the N400 effects were influenced by the different durations.
For the electrode Cz, there was no significant main effect of duration [F(3,33) = 2.319, p = 0.093, ηp2 = 0.174], and the main effect of congruence was significant [F(2,22) = 4.503, p = 0.023, ηp2 = 0.290]. The duration showed no significant interaction with congruence [F(6,66) = 1.986, p = 0.080, ηp2 = 0.153]. For the electrode CPz, there was no significant main effect for duration [F(3,33) = 2.250, p = 0.101, ηp2 = 0.170]. The main effect of congruence was significant [F(2,22) = 3.731, p = 0.040, ηp2 = 0.253]. The effect of interaction of duration and congruence was not significant [F(6,66) = 1.142, p = 0.348, ηp2 = 0.094]. Because there appears to be no effect of duration, Figure 3 shows the N400 collapsed across all duration levels.
FIGURE 3. The grand-averaged ERPs of the N400. The grand-averaged ERPs waveforms of the N400 under the conditions of incongruence (blue solid), congruence (green dashed), and control (red) at the Cz and CPz electrode sites.
Statistical analysis demonstrated significant differences (p < 0.05) between the levels of duration of 40 and 300 ms in the beta 2 (19–21 Hz) and beta 3 (22–30 Hz) frequency bands. In the beta 2 band, 371 voxels showed significant current-source density differences. In the beta 3 band, 964 voxels showed significant differences. A comparison of current density images between the 40 and 300 ms durations for beta 2 and beta 3 is shown in Figure 4. Yellow areas correspond to significantly higher activity in the 40 ms condition (p < 0.05, t threshold = 1.314).
FIGURE 4. sLORETA differences in two frequency bands (beta 2 and beta 3) between the 40 and 300 ms duration conditions (collapsed across all three congruence conditions). In the beta 2 (upper panel) and beta 3 frequency bands (lower panel), activity was significantly higher for 40 ms than for 300 ms in widespread areas, including the medial frontal gyrus and superior frontal gyrus. Images depicting statistical parametric maps observed from different perspectives are based on voxel by-voxel log of F-ratio values of differences between the two groups for the beta 2 and beta 3 bands. Structural anatomy is shown in gray scale (A, anterior; P, posterior; S, superior; I, inferior; LH, left hemisphere; RH, right hemisphere; BH, both hemispheres; LV, left view; RV, right view; BV, bottom view). Yellow indicates increases for 40 ms compared to 300 ms (t0.05 = 1.314, t0.01 = 1.510, one tail), which are mainly in the medial frontal gyrus and the superior frontal gyrus.
The aim of the current study was to determine if there are different neural mechanisms underlying the recognition of microexpressions and macroexpressions. The results indicate that there are different ERPs and ERSP characteristics for recognizing microexpressions and macroexpressions. The brain regions responsible for the differences might be the inferior temporal gyrus and widespread areas in the frontal lobe. Furthermore, the left hemisphere was more involved in processing microexpressions. These results suggest that different neural mechanisms are responsible for the recognition of microexpressions and macroexpressions.
For expressions, there is a critical factor for recognition that is less well understood: the duration. A microexpression is presented for a short duration, which may result in the recipient barely perceiving it. The most commonly cited description of the duration of a microexpression: microexpressions (1/25–1/5 of a second). Thus, the duration is the core difference between microexpressions and macroexpressions. Moreover, as ten Brinke and Porter (2013, p. 227) noted, a microexpression is “a brief but complete facial expression.” Therefore, the key characteristic differentiating microexpressions from macroexpressions is not the completeness of the expression (which may be related to intensity of emotion) but the duration of the expression. Considering that duration is the critical feature of a microexpression, in the current study, we manipulated the durations of expressions and expected that there would be different brain mechanisms for recognizing microexpressions and macroexpressions. The duration boundary may be around 200 ms, which can be used to differentiate a microexpression from a macroexpression (see Shen et al., 2012). The findings of this study show that recognizing expressions with durations of less than 200 ms and expressions with durations of greater than 200 ms are associated with different EEG/ERPs characteristics. Thus, we further confirmed that the boundary of the duration of expressions for differentiating microexpressions and macroexpressions is around 200 ms.
The present study manipulated the duration of facial expressions and examined the influence of duration on expression recognition by exploring the N170/VPP, the N400 effect and related EEG indicators. For the N170/VPP, there is a main effect of duration that clearly indicates the effects of duration on processing facial expressions with different durations. As shown in Figure 1A, there are two groups of ERPs, one for expressions with durations of greater than 200 ms and one for expressions with durations of less than 200 ms, suggesting that a duration boundary of 200 ms can differentiate microexpressions and macroexpressions. As for the interaction of duration and valence at electrode Cz, we should be cautious to draw any inference because there is no interaction at electrode CPz. The interaction of duration and valence should be elucidated further in the future.
As shown in Figure 1A, there is an enhanced N170/VPP in response to expressions with a short duration (<200 ms) compared to expressions with a long duration (>200 ms). On the one hand, the results may be due in part to attention (as a mediator variable). Attention to faces and facial expressions can modulate the N170 amplitude (Eimer, 2000, 2011; Eimer and Holmes, 2007). In the current study, recognizing expressions with short durations (e.g., 40 ms) may need significantly more attention resources (because short-duration expressions are somewhat difficult to perceive) than do expressions with long durations (e.g., 300 ms), which may result in a higher amplitude of N170/VPP for recognizing expressions with short durations. On the other hand, we automatically mimic the exposed facial expressions while recognizing them (Dimberg et al., 2000; Tamietto et al., 2009), if the exposing duration is short (say less than 200 ms, it is the case in microexpression recognition), then there is not much time to mimic the transient expression with short duration. Therefore, the mimicry of microexpression has to consult the memory to reach recognition, which may result in a stronger processing in the brain than the recognition of macroexpression, because recognizing macroexpression (with duration of greater than 200 ms) can only rely on the perceptual features of expressions.
As shown in Figure 1, sharp contrasts in scalp potential maps (Figure 1B) and ERSP (Figure 1C) are present between microexpressions (durations of less than 200 ms) and macroexpressions (durations of greater than 200 ms). The microexpressions elicited stronger power changes in theta band activities than did macroexpressions (see the comparison of the box of a solid line and the box of a dashed line in Figure 1C), which might also be interpreted as relating to the larger attention demands that are imposed on recognizing fleeting microexpressions.
In the current study, the ERSP results of N170/VPP showed that the amplitude of theta response was higher for microexpressions (with durations of less than 200 ms) than for macroexpressions (with durations of greater than 200 ms), which suggests that the theta response is also modulated by the duration of emotional expressions. Meanwhile, cognitive load may be related to the theta oscillatory activity (Bates et al., 2009). The reduced theta oscillatory activity for expressions with longer durations may be partly explained by cognitive load. For expressions with longer durations, there should be a lower cognitive load and there should be higher cognitive load for expressions with short durations. Meanwhile, the brain oscillations in the theta band are involved in active maintenance of memory representations (Jensen and Tesche, 2002). For expressions with shorter duration, one should make much more efforts to maintain the representations for further processing. For expressions with longer durations, one can check it anytime; therefore, the load for holding the representation is low. As shown in Figure 2, the neural generators that respond to the difference between recognizing expressions with durations of 40 and of 300 ms are located in the frontal lobe while measuring the N170/VPP, which is consistent some previous work that showed the frontal theta power increased with the cognitive load (Scheeringa et al., 2009). There are distinct EEG mu responses while viewing positively and negatively valenced emotional faces (see Moore et al., 2012), therefore, besides the beta rhythm, we should use mu response to further investigate the recognition of microexpression and macroexpression in ther future.
The statistical results of the N400 effects show no effects of duration and only a marginal significant interaction between duration and congruence at Cz, which does not support the prediction regarding the N400 effects (there should be a significant interaction). The effect of congruence is significant in the N400, which can be observed in Figure 3. The results suggest that even under the condition of short duration of expression, the participants could engage in top-down processing and the meaning of the expression was processed regardless of the duration (long or short in the current study), which implies that the fleeting emotional expressions (even with a duration of 40 ms) can be rapidly identified at a conceptual level (Potter, 2012). The results are consistent with some previous studies (Murphy and Zajonc, 1993; Milders et al., 2008).
As shown in Figure 4, there are significant differences in the profiles of the beta 2 and beta 3 powers between the 40 ms duration condition and the 300 ms duration condition, which suggests a strong involvement of beta-band synchronization in the processing of duration of an expression. Beta rhythm has been observed experimentally under the conditions of extensive recruitment of excitatory neurons (Whittington et al., 2000), suggesting there are more excitatory neurons for processing a facial expression with a duration of 40 ms than there are for an expression with a duration of 300 ms. Meanwhile, from the results of the sLORETA in Figure 4, we can observe that there is an increase in the power of beta activities. The locations are mainly in the frontal lobe and temporal lobe and involve more left than right hemispheric voxels (a similar neural activities pattern that involve more left than right hemispheric voxels can be seen in Figure 2). The results suggest there is left-hemisphere dominance for recognizing microexpressions. The lateralization of emotion has long been studied (Indersmitten and Gur, 2003) and many studies show evidence supporting right-hemispheric dominance for emotion processing (Schwartz et al., 1975; Hauser, 1993). There is, however, some debate regarding right-hemispheric dominance (De Winter et al., 2015). The current results show that the left hemisphere might respond during the processing of fleeting (<200 ms) expressions, regardless of valence. The effect of duration on the hemispheric dominance for emotional expressions processing should be further investigated and some objective indexes such as weighted lateralization index (see De Winter et al., 2015) should be provided.
It should be noted that the differences between the recognition of microexpressions and macroexpressions is not the same as the differences in recognizing supraliminal and subliminal facial expression (Balconi and Lucchiari, 2005). According to Shen et al. (2012), the accuracy of recognition for expressions with durations of 40 ms is above 40%, which is higher than the chance level (1/6), which means that the recognizing microexpression is conscious. Even the expressions cannot be perceived consciously, we still can unconsciously “resonate” the facial expressions we have seen during emotion communication (Dimberg et al., 2000; Tamietto et al., 2009), which may facilitate the recognition of microexpression and macroexpression.
In summary, we wanted to determine the exact differences in neural substrates for recognizing microexpression and macroexpression in the current study. The EEG/ERPs results revealed a distinct amplitude of the N170/VPP and oscillatory neuronal dynamics in response to microexpressions (with durations of less than 200 ms) and macroexpressions (with durations of greater than 200 ms). These results suggest that the EEG/ERPs characteristics are different between the recognition of microexpressions and macroexpressions.
Our understanding of how we perceive and recognize microexpressions and macroexpressions will be further advanced by studying the EEG/ERPs, their oscillatory neuronal dynamics, and their association with the processes of recognition. Based on this understanding of microexpression recognition, we can further explore the association between microexpressions and deception. Although controversial, microexpressions are closely related to deception and are used as a vital behavioral clue for lie detection (Frank and Svetieva, 2015). According to Weinberger (2010), few published peer-review studies address microexpressions for political reasons. Linking microexpressions to deception is “a leap of gargantuan dimensions” (for a review, see Weinberger, 2010). Many more studies are needed to understand the mechanisms underlying recognition of microexpression and its association with deception.
In the future, dynamic facial expressions with greater ecological validity should be employed. The brain mechanisms involved in recognizing a number of fleeting social emotions, including shame, guilt, and remorse (ten Brinke et al., 2012), and the fundamental properties of microexpressions recognition (Svetieva, 2014) should be explored. The influence of some factors, for instance, contextual cues (Van den Stock and de Gelder, 2014) which co-occur with facial microexpresion and macroexpression, age (Zhao et al., 2016), empathy (Svetieva and Frank, 2016), on how we recognize microexpresion and macroexpression and the underlying brain mechanisms should also be investigated.
XF and XS conceived the experiments. QW and KZ conducted the experiments. XS analyzed the results and wrote the main manuscript text and prepared all figures and tables. All authors reviewed the manuscript.
This project was partially supported by the National Natural Science Foundation of China (No. 61375009, 31460251) and the MOE (Ministry of Education in China) Youth Fund Project of Humanities and Social Sciences (Project No. 13YJC190018).
The authors declare that the research was conducted in the absence of any commercial or financial relationships that could be construed as a potential conflict of interest.
The authors would like to thank Bo Wang for his comments on the initial manuscript.
Balconi, M., and Lucchiari, C. (2005). In the face of emotions: event-related potentials in supraliminal and subliminal facial expression recognition. Genet. Soc. Gen. Psychol. Monogr. 131, 41–69. doi: 10.3200/MONO.131.1.41-69
Balconi, M., and Pozzoli, U. (2007). Event-related oscillations (EROs) and event-related potentials (ERPs) comparison in facial expression recognition. J. Neuropsychol. 1, 283–294. doi: 10.1348/174866407X184789
Bates, A. T., Kiehl, K. A., Laurens, K. R., and Liddle, P. F. (2009). Low-frequency EEG oscillations associated with information processing in schizophrenia. Schizophr. Res. 115, 222–230. doi: 10.1016/j.schres.2009.09.036
Batty, M., and Taylor, M. J. (2003). Early processing of the six basic facial emotional expressions. Cogn. Brain Res. 17, 613–620. doi: 10.1016/S0926-6410(03)00174-5
Bhushan, B. (2015). “Study of facial micro-expressions in psychology,” in Understanding Facial Expressions in Communication, eds M. K. Mandal and A. Awasthi (New Delhi: Springer), 265–286.
Costa, T., Cauda, F., Crini, M., Tatu, M.-K., Celeghin, A., de Gelder, B., et al. (2014). Temporal and spatial neural dynamics in the perception of basic emotions from complex scenes. Soc. Cogn. Affect. Neur. 9, 1690–1703. doi: 10.1093/scan/nst164
De Winter, F.-L., Zhu, Q., Van den Stock, J., Nelissen, K., Peeters, R., de Gelder, B., et al. (2015). Lateralization for dynamic facial expressions in human superior temporal sulcus. Neuroimage 106, 340–352. doi: 10.1016/j.neuroimage.2014.11.020
Delorme, A., and Makeig, S. (2004). EEGLAB: an open source toolbox for analysis of single-trial EEG dynamics including independent component analysis. J. Neurosci. Methods 134, 9–21. doi: 10.1016/j.jneumeth.2003.10.009
Dimberg, U., Thunberg, M., and Elmehed, K. (2000). Unconscious facial reactions to emotional facial expressions. Psychol. Sci. 11, 86–89. doi: 10.1111/1467-9280.00221
Eimer, M. (2000). Attentional modulations of event-related brain potentials sensitive to faces. Cogn. Neuropsychol. 17, 103–116. doi: 10.1080/026432900380517
Eimer, M. (2011). “The face-sensitive N170 component of the event-related brain potential,” in Oxford handbook of Face Perception, eds G. Rhodes, A. Calder, M. Johnson, and J. V. Haxby (New York, NY: Oxford University Press), 329–344.
Eimer, M., and Holmes, A. (2007). Event-related brain potential correlates of emotional face processing. Neuropsychologia 45, 15–31. doi: 10.1016/j.neuropsychologia.2006.04.022
Ekman, P. (1971). “Universals and cultural differences in facial expressions of emotion,” in Nebraska Symposium on Motivation, ed. J. Cole (Lincoln: University of Nebraska Press), 207–283.
Ekman, P. (1992). Telling Lies: Clues to Deceit in the Marketplace, Politics, and Marriage. New York, NY: Norton.
Ekman, P. (2003). Emotions revealed: Recognizing Faces and Feelings to Improve Communication and Emotional Life. New York, NY: Times Books.
Ekman, P. (2009). “Lie Catching and Microexpressions,” in The Philosophy of Deception, ed. C. Martin (New York, NY: Oxford University Press), 118–133.
Ekman, P., and Friesen, W. V. (1969). Nonverbal Leakage and Clues to Deception. Psychiatry 32, 88–106.
Ekman, P., Rolls, E., Perrett, D., and Ellis, H. (1992). Facial expressions of emotion: an old controversy and new findings [and discussion]. Philos. Trans. R. Soc. Lond. B Biol. Sci. 335, 63–69. doi: 10.1098/rstb.1992.0008
Esslen, M., Pascual-Marqui, R., Hell, D., Kochi, K., and Lehmann, D. (2004). Brain areas and time course of emotional processing. Neuroimage 21, 1189–1203. doi: 10.1016/j.neuroimage.2003.10.001
Frank, M. G., and Svetieva, E. (2015). “Microexpressions and deception,” in Understanding Facial Expressions in Communication, eds M. K. Mandal and A. Awasthi (New Delhi: Springer), 227–242.
Fuchs, M., Kastner, J., Wagner, M., Hawes, S., and Ebersole, J. S. (2002). A standardized boundary element method volume conductor model. Clin. Neurophysiol. 113, 702–712. doi: 10.1016/S1388-2457(02)00030-5
Güntekin, B., and Baȿsar, E. (2014). A review of brain oscillations in perception of faces and emotional pictures. Neuropsychologia 58, 33–51. doi: 10.1016/j.neuropsychologia.2014.03.014
Hauser, M. D. (1993). Right hemisphere dominance for the production of facial expression in monkeys. Science 261, 475–477. doi: 10.1126/science.8332914
Indersmitten, T., and Gur, R. C. (2003). Emotion processing in chimeric faces: hemispheric asymmetries in expression and recognition of emotions. J. Neurosci. 23, 3820–3825.
Jensen, O., and Tesche, C. D. (2002). Frontal theta activity in humans increases with memory load in a working memory task. Eur. J. Neurosci. 15, 1395–1399. doi: 10.1046/j.1460-9568.2002.01975.x
Joyce, C., and Rossion, B. (2005). The face-sensitive N170 and VPP components manifest the same brain processes: the effect of reference electrode site. Clin. Neurophysiol. 116, 2613–2631. doi: 10.1016/j.clinph.2005.07.005
Jurcak, V., Tsuzuki, D., and Dan, I. (2007). 10/20, 10/10, and 10/5 systems revisited: their validity as relative head-surface-based positioning systems. Neuroimage 34, 1600–1611. doi: 10.1016/j.neuroimage.2006.09.024
Keil, A. (2013). “Electro-and magnetoencephalography in the study of emotion,” in The Cambridge Handbook of Human Affective Neuroscience, eds J. Armony and P. Vuilleumier (New York, NY: Cambridge University Press), 107–132.
Knyazev, G. G., and Slobodskaya, H. R. (2003). Personality trait of behavioral inhibition is associated with oscillatory systems reciprocal relationships. Int. J. Psychophysiol. 48, 247–261. doi: 10.1016/S0167-8760(03)00072-2
Kutas, M., and Federmeier, K. D. (2011). Thirty years and counting: finding meaning in the N400 component of the event-related brain potential (ERP). Annu. Rev. Psychol. 62, 621–647. doi: 10.1146/annurev.psych.093008.131123
Lancaster, J. L., Woldorff, M. G., Parsons, L. M., Liotti, M., Freitas, C. S., Rainey, L., et al. (2000). Automated Talairach atlas labels for functional brain mapping. Hum. Brain Mapp. 10, 120–131. doi: 10.1002/1097-0193(200007)10:3<120::AID-HBM30>3.0.CO;2-8
Lui, S., Huang, X., Chen, L., Tang, H., Zhang, T., Li, X., et al. (2009). High-field MRI reveals an acute impact on brain function in survivors of the magnitude 8.0 earthquake in China. Proc. Natl. Acad. Sci. U.S.A. 106, 15412–15417. doi: 10.1073/pnas.0812751106
Makeig, S., Debener, S., Onton, J., and Delorme, A. (2004). Mining event-related brain dynamics. Trends Cogn. Sci. 8, 204–210. doi: 10.1016/j.tics.2004.03.008
Mazziotta, J., Toga, A., Evans, A., Fox, P., Lancaster, J., Zilles, K., et al. (2001). A probabilistic atlas and reference system for the human brain: international consortium for brain mapping (ICBM). Philos. Trans. R. Soc. Lond. B Biol. Sci. 356, 1293–1322. doi: 10.1098/rstb.2001.0915
Metzinger, T. (2006). Exposing Lies. Sci. Am. Mind 17, 32–37. doi: 10.1038/scientificamericanmind1006-32
Milders, M., Sahraie, A., and Logan, S. (2008). Minimum presentation time for masked facial expression discrimination. Cogn. Emot. 22, 63–82. doi: 10.1080/02699930701273849
Moore, A., Gorodnitsky, I., and Pineda, J. (2012). EEG mu component responses to viewing emotional faces. Behav. Brain Res. 226, 309–316. doi: 10.1016/j.bbr.2011.07.048
Murphy, S. T., and Zajonc, R. B. (1993). Affect, cognition, and awareness: affective priming with optimal and suboptimal stimulus exposures. J. Pers. Soc. Psychol. 64, 723–739. doi: 10.1037/0022-3514.64.5.723
Newman, R., and Connolly, J. (2009). Electrophysiological markers of pre-lexical speech processing: evidence for bottom–up and top–down effects on spoken word processing. Biol. Psychol. 80, 114–121. doi: 10.1016/j.biopsycho.2008.04.008
Nichols, T. E., and Holmes, A. P. (2002). Nonparametric permutation tests for functional neuroimaging: a primer with examples. Hum. Brain Mapp. 15, 1–25. doi: 10.1002/hbm.1058
Niedenthal, P. M., and Brauer, M. (2012). Social functionality of human emotion. Annu. Rev. Psychol. 63, 259–285. doi: 10.1146/annurev.psych.121208.131605
Oostenveld, R., and Praamstra, P. (2001). The five percent electrode system for high-resolution EEG and ERP measurements. Clin. Neurophysiol. 112, 713–719. doi: 10.1016/S1388-2457(00)00527-7
Pascual-Marqui, R. D. (2002). Standardized low-resolution brain electromagnetic tomography (sLORETA): technical details. Methods Find. Exp. Clin. Pharmacol. 24(Suppl. D), 5–12.
Pascual-Marqui, R. D., Esslen, M., Kochi, K., and Lehmann, D. (2002). Functional imaging with low resolution brain electromagnetic tomography (LORETA): review, new comparisons, and new validation. Jpn. J. Clin. Neurophysiol. 30, 81–94.
Pascual-Marqui, R. D., Lehmann, D., Koenig, T., Kochi, K., Merlo, M. C. G., Hell, D., et al. (1999). Low resolution brain electromagnetic tomography (LORETA) functional imaging in acute, neuroleptic-naive, first-episode, productive schizophrenia. Psychiatry Res. 90, 169–179. doi: 10.1016/S0925-4927(99)00013-X
Pascual-Marqui, R. D., Michel, C. M., and Lehmann, D. (1994). Low resolution electromagnetic tomography: a new method for localizing electrical activity in the brain. Int. J. Psychophysiol. 18, 49–65. doi: 10.1016/0167-8760(84)90014-X
Porter, S., and ten Brinke, L. (2008). Reading between the lies: identifying concealed and falsified emotions in universal facial expressions. Psychol. Sci. 19, 508–514. doi: 10.1111/j.1467-9280.2008.02116.x
Potter, M. C. (2012). Conceptual short term memory in perception and thought. Front. Psychol. 3:113. doi: 10.3389/fpsyg.2012.00113
Righart, R., and De Gelder, B. (2008). Rapid influence of emotional scenes on encoding of facial expressions: an ERP study. Soc. Cogn. Affect. Neur. 3, 270–278. doi: 10.1093/scan/nsn021
Roach, B. J., and Mathalon, D. H. (2008). Event-related EEG time-frequency analysis: an overview of measures and an analysis of early gamma band phase locking in schizophrenia. Schizophr. Bull. 34, 907–926. doi: 10.1093/schbul/sbn093
Rossion, B., and Jacques, C. (2011). “The N170: understanding the time-course of face perception in the human brain,” in The Oxford Handbook of ERP Components, eds S. Luck and E. Kapenhman (New York, NY: Oxford University Press), 115–142.
Scheeringa, R., Petersson, K. M., Oostenveld, R., Norris, D. G., Hagoort, P., and Bastiaansen, M. C. (2009). Trial-by-trial coupling between EEG and BOLD identifies networks related to alpha and theta EEG power increases during working memory maintenance. Neuroimage 44, 1224–1238. doi: 10.1016/j.neuroimage.2008.08.041
Schubert, S. (2006). A look tells all. Sci. Am. Mind 17, 26–31. doi: 10.1038/scientificamericanmind1006-26
Schwartz, G. E., Davidson, R. J., and Maer, F. (1975). Right hemisphere lateralization for emotion in the human brain: interactions with cognition. Science 190, 286–288. doi: 10.1126/science.1179210
Shen, X., Wu, Q., and Fu, X. (2012). Effects of the duration of expressions on the recognition of microexpressions. J. Zhejiang Univ. Sci. B 13, 221–230. doi: 10.1631/jzus.B1100063
Svetieva, E. (2014). Seeing the Unseen: Explicit and Implicit Communication Effects of Naturally Occuring Emotion Microexpressions. Ph.D. dissertation, State University of New York at Buffalo, New York, NY.
Svetieva, E., and Frank, M. G. (2016). Empathy, emotion dysregulation, and enhanced microexpression recognition ability. Motiv. Emot. 40, 309–320. doi: 10.1007/s11031-015-9528-4
Tamietto, M., Castelli, L., Vighetti, S., Perozzo, P., Geminiani, G., Weiskrantz, L., et al. (2009). Unseen facial and bodily expressions trigger fast emotional reactions. Proc. Natl. Acad. Sci. U.S.A. 106, 17661–17666. doi: 10.1073/pnas.0908994106
ten Brinke, L., MacDonald, S., Porter, S., and O’Connor, B. (2012). Crocodile tears: facial, verbal and body language behaviours associated with genuine and fabricated remorse. Law Hum. Behav. 36, 51–59. doi: 10.1037/h0093950
ten Brinke, L., and Porter, S. (2013). “Discovering deceit: applying laboratory and field research in the search for truthful and deceptive behavior,” in Applied Issues in Investigative Interviewing, Eyewitness Memory, and Credibility Assessment, eds B. S. Cooper, D. Griesel, and M. Ternes (New York, NY: Springer), 221–237.
Van den Stock, J., and de Gelder, B. (2014). Face identity matching is influenced by emotions conveyed by face and body. Front. Hum. Neurosci. 8:53. doi: 10.3389/fnhum.2014.00053
Wang, B., and Fu, X. (2011). Time course of effects of emotion on item memory and source memory for Chinese words. Neurobiol. Learn. 95, 415–424. doi: 10.1016/j.nlm.2011.02.001
Wang, X., Wang, X., and Ma, H. (eds) (1993). Rating Scales for Mental Health. Beijing: Publisher of Chinese Mental Health Journal.
Weinberger, S. (2010). Airport security: intent to deceive? Nature 465, 412–415. doi: 10.1038/465412a
Whittington, M., Faulkner, H., Doheny, H., and Traub, R. (2000). Neuronal fast oscillations as a target site for psychoactive drugs. Pharmacol. Ther. 86, 171–190. doi: 10.1016/S0163-7258(00)00038-3
Keywords: microexpression, macroexpression, recognition, EEG/ERPs, ERSP, sLORETA
Citation: Shen X, Wu Q, Zhao K and Fu X (2016) Electrophysiological Evidence Reveals Differences between the Recognition of Microexpressions and Macroexpressions. Front. Psychol. 7:1346. doi: 10.3389/fpsyg.2016.01346
Received: 30 April 2016; Accepted: 23 August 2016;
Published: 31 August 2016.
Edited by:
Marco Tamietto, Tilburg University, NetherlandsReviewed by:
Jan Van Den Stock, Katholieke Universiteit Leuven, BelgiumCopyright © 2016 Shen, Wu, Zhao and Fu. This is an open-access article distributed under the terms of the Creative Commons Attribution License (CC BY). The use, distribution or reproduction in other forums is permitted, provided the original author(s) or licensor are credited and that the original publication in this journal is cited, in accordance with accepted academic practice. No use, distribution or reproduction is permitted which does not comply with these terms.
*Correspondence: Xiaolan Fu, ZnV4bEBwc3ljaC5hYy5jbg==
Disclaimer: All claims expressed in this article are solely those of the authors and do not necessarily represent those of their affiliated organizations, or those of the publisher, the editors and the reviewers. Any product that may be evaluated in this article or claim that may be made by its manufacturer is not guaranteed or endorsed by the publisher.
Research integrity at Frontiers
Learn more about the work of our research integrity team to safeguard the quality of each article we publish.