- 1Laboratory of Neurophysiology and Movement Biomechanics, Université Libre de Bruxelles Neuroscience Institut, Université Libre de Bruxelles, Brussels, Belgium
- 2Laboratory of Electrophysiology, Université de Mons-Hainaut, Mons, Belgium
- 3Haute Ecole Condorcet, Charleroi, Belgium
- 4Inkendaal Rehabilitation Hospital, Vlezembeek, Belgium
Brain dynamics is at the basis of top performance accomplishment in sports. The search for neural biomarkers of performance remains a challenge in movement science and sport psychology. The non-invasive nature of high-density electroencephalography (EEG) recording has made it a most promising avenue for providing quantitative feedback to practitioners and coaches. Here, we review the current relevance of the main types of EEG oscillations in order to trace a perspective for future practical applications of EEG and event-related potentials (ERP) in sport. In this context, the hypotheses of unified brain rhythms and continuity between wake and sleep states should provide a functional template for EEG biomarkers in sport. The oscillations in the thalamo-cortical and hippocampal circuitry including the physiology of the place cells and the grid cells provide a frame of reference for the analysis of delta, theta, beta, alpha (incl.mu), and gamma oscillations recorded in the space field of human performance. Based on recent neuronal models facilitating the distinction between the different dynamic regimes (selective gating and binding) in these different oscillations we suggest an integrated approach articulating together the classical biomechanical factors (3D movements and EMG) and the high-density EEG and ERP signals to allow finer mathematical analysis to optimize sport performance, such as microstates, coherency/directionality analysis and neural generators.
Introduction
For top performance accomplishment in sports, the brain dynamics needs to be taken into account because it determines both motor control and crucial psychological factors, such as intrinsic motivation (Pedersen, 2002), selective attention (Arjona et al., 2014; Abdollahipour et al., 2015), goal setting (West and Thorn, 2001), working memory (Dipoppa and Gutkin, 2013), decision making (Renfree et al., 2014), positive self-concept (Badami et al., 2012), and self-control (Ali et al., 2012; Chiviacowsky et al., 2012).
In practical terms, neuronal oscillations can be readily recorded in a non-invasive way in human allowing the possibility to follow the dynamics of brain working during activity. The optimized state of performance reached by sport elites offers a privileged domain for studying the different neuronal oscillations linked to sensorimotor and cognitive control getting to final success or failure. For example, Figure 1 illustrates the recent integrated approach performed by our team in elite hockey players during the accomplishment of the sleep push movement. The sleep push involves a player crouching low down, picking the ball up on the shaft of the stick, then moving in a forceful slinging action of the ball around the running up body. This allows accelerating the ball in the direction of the goal. Such approach allows establishing functional links between the multiple neurophysiological signals in order to identify the different neural generators in the brain (Figure 1).
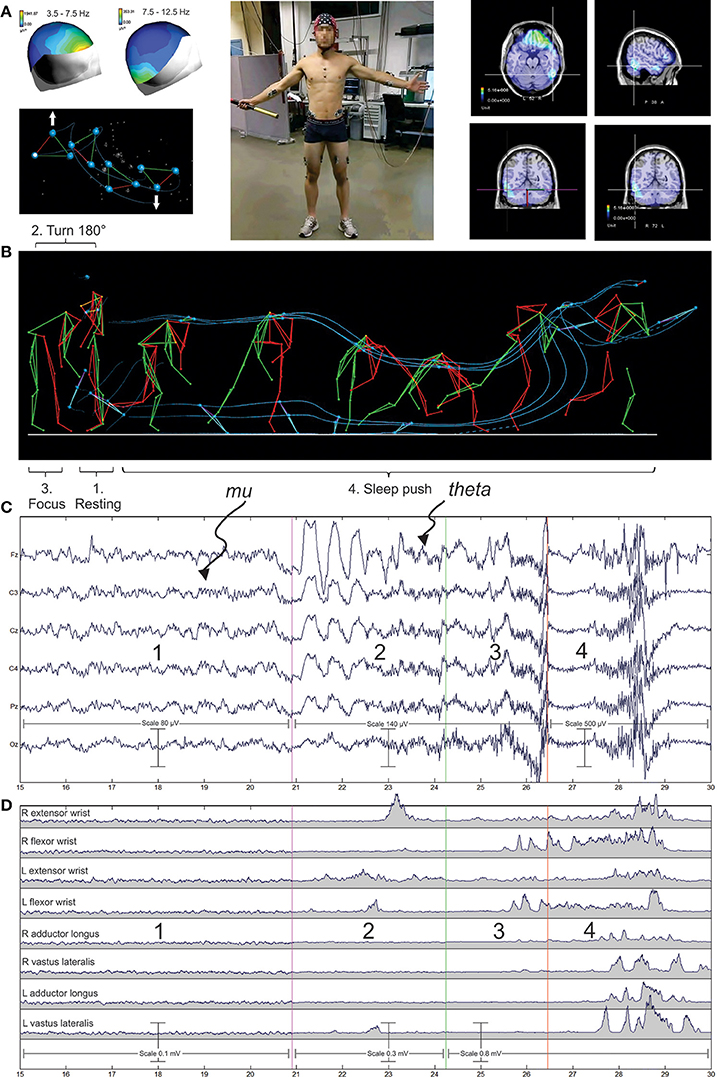
Figure 1. Illustration of an integrated neurophysiological approach in sport. The sleep push or drag flicking in field hockey sport is here illustrated as an example of complex movement involving the whole body. It is an attacking movement mainly performed within the penalty corner but also realized outside the penalty corner situation. Here in our experimental setup, the player was placed in front of the laboratory wall with the field of action in its back. He was asked to relax in this position before turning in front of the action field. Then he performed the sleep push movement at its self-paced mode in order to put the ball inside of the goal. (A) The player was equipped with a 128 electrodes cap (ANT), the cables of the cap were connected to the amplifier which were placed in a traveling box piloted by the experimenter in order to assume the follow-up of the player's running. (B) Surfaces electrodes () were placed on muscles of interest and allowed the electromyographic (EMG) recording (see, G). (C) Retro-reflective passive markers were fixed on the skin overlying the following bony landmarks on both sides of the body: LM, lateral malleolus; MT, fifth metatarsal head; LE, lateral condyle of the knee; GT, greater trochanter; and IL, tubercule of the anterosuperior iliac crest then a stick diagram of the whole body representation was constructed. (D) Kinematics of the sleep push movement, successive pictures of the main phase are presented and 3 markers placed on the head and 3 other placed on the distal part of the stick are joined by continuous lines (blue) giving a global representation of the movement signature.
The aim of such studies is the characterization of an array of electroencephalographic (EEG) dynamics biomarkers of sport performance analogous to those proposed in mental health (Başar et al., 2013; Olbrich and Arns, 2013; Loo et al., 2015). However, our current understanding of the neuronal oscillations that occur before, during and after a dedicated sport movement remains problematic because of the absence of consensus about physiological significance of the different oscillatory signals recorded from the scalp, the absence of standardized methodologies for sport domain, and the presence of different artifacts due to sport movement itself (Thompson et al., 2008; Reinecke et al., 2011; Castermans et al., 2014; Kline et al., 2015). In addition, useful biomarkers will need to integrate neuronal models of flexibility such as the one elaborated for working memory process (Dipoppa and Gutkin, 2013, see below). Indeed, the different types of cortical oscillations must not be understood separately but in close interaction in which subtle physiological mechanisms operate.
In this inaugural article we intent to review the current relevance of the main types of EEG oscillations in order to trace a perspective for future practical applications in sport domain.
The EEG Origin and Information Content
Thanks to its non-invasive nature, EEG recording has been recognized as one of the most routinely neuroimaging approach for studying brain activities (Niedermeyer and da Silva, 1982). Nevertheless, it remains unknown which part of the complex neurophysiological events occurring in the cortical mantle are represented by the surface EEG, in spite of their clinical interest (Libenson, 2012) and numerous applications in psychology (Dickter and Kieffaber, 2014) and in the emergent domains of sport science (Baumeister et al., 2008; Park et al., 2015) and brain computing interface (BCI) (Cheron et al., 2009; Duvinage et al., 2012; Angulo-Sherman and Gutiérrez, 2015). Therefore, in this review, we shall critically revisit the different EEG rhythms which might form a template for future biomarkers of performance.
Neuronal Membrane Potentials and Neuronal Synchrony
The recent approaches coupling electrocorticographic (EcoG), local field potentials (LFP) and dual whole-cell recording in the primary somatosensory barrel cortex in behaving mice (Figure 2) (Poulet and Petersen, 2008) have revealed interesting results about the contribution of the membrane potential (V(m)) and the neuronal synchrony to EEG recording. They demonstrated a perfect synchrony between the V(m) of a large number of pyramidal cell pairs in such a way that LFP and EEG signals represent a close reflection of the rhythmic fluctuation of the synchronized V(m) highly correlated in the resting state and less correlated during movement (Figure 2D). The desynchronized rhythmicity during movement can be due to the influence of afferent signals or to central reverberating activity. In fact, the two oscillatory states (resting and active) are not dependent on afferent information (Poulet and Petersen, 2008). These cortical states are centrally produced and controlled by the thalamus independently from afferent and efferent signals (Figure 2E; Poulet et al., 2012). Interestingly, the inhibitory interneurons of the somatosensory cortex fire at significantly higher frequencies than excitatory neurons during the resting state (Gentet et al., 2010).
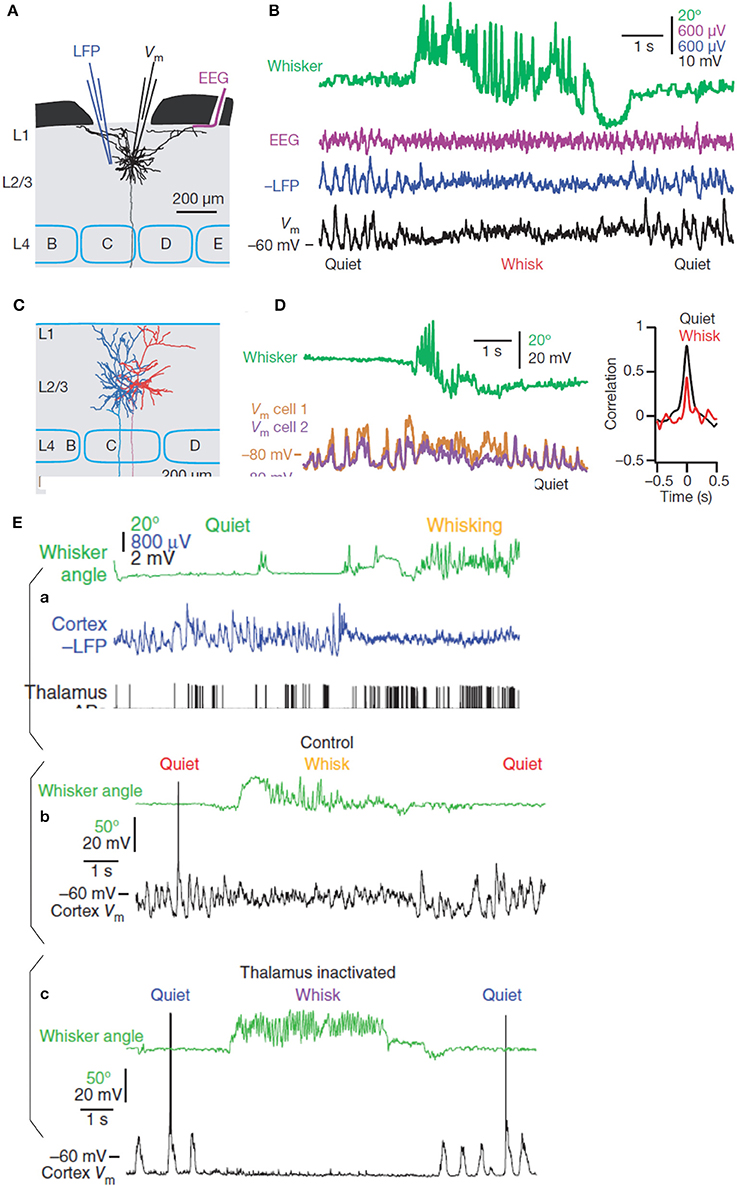
Figure 2. (A) Recording configuration (simultaneous EEG, LFP, and whole-cell recordings) in the mouse somatosensory cortex (Barrel cortex) and reconstruction of one excitatory pyramidal neuron (black) of the layer 2/3. (B) From top to bottom, movement of the whisker (green) defining quiet and whisking periods, EEG signals (red), LFP recording (blue), and membrane potentials (black). (C) Reconstruction of two layer 2/3 pyramidal neurons (blue and red) simultaneously recorded. (D) From top to bottom, whisking movement (green) and superimposition of the membrane potentials of the two pyramidal neurons with their cross-correlation during quiet and whisking movement (modified from Poulet and Petersen, 2008, with permission). (E) Simultaneous recording of a thalamic neuron and cortical LFP showing the increase of the action potential firing rates during the active desynchronized cortical state (a). Behavioral difference of the membrane potential of a pyramidal neuron in presence (b), or in absence of the thalamic influence (c) during quiet and whisking states (see text for more details, modified from Poulet et al., 2012, with permission).
Although whisking movement of mouse is not directly related to sport movement in human these results shed a new light on the mechanism underlying the event related spectral perturbation (ERSP) analysis applied on the EEG recorded in sport. In particular, it highlights the significance of event related desynchronization (ERD) and event related synchronization (ERS) episodes of the EEG signals with respect to selected inhibitory or excitatory neuronal populations.
Unified Brain Rhythms and Continuity Hypothesis between Wake and Sleep States
Self-emerging oscillation occupy a central position because they represent the brain's fundamental organizer of neuronal information (Varela et al., 2001; Buzsáki, 2006; Buzsáki and Watson, 2012; Başar et al., 2013; Cheron et al., 2015). Neuronal oscillators are implicated in a plethora of crucial functions extending from movement control and cognition to sleep. In accordance with the concept of unified brain rhythms, the continuity hypothesis (Pesant and Zadra, 2006; Marzano et al., 2011; Fullagar et al., 2015) proposed that there is a sort of permanency of encoding and retrieval mechanisms of episodic memories across sleep and wakefulness (Scarpelli et al., 2015). Based on these evidences, we suggest that there is continuum of brain oscillatory flux ranging from slow to fast rhythms throughout the day and night that must be taken into account and practically applied in the follow-up of athletes (Fullagar et al., 2015).
The Quality of Sleep Oscillations in Sport: Slow-Wave Oscillation (~1 Hz)
Sport practice has multiple impacts on brain-body relationships, including during following night and day after. This implies that the follow-up of the EEG should also consider the quality of sleep oscillations.
For example, the loss of the slow-wave activity (SWA) occurring during the non-rapid eye movement (NREM) state (Figure 3) is related to slower EEG and impaired performance due to cognitive and memory deficits during wake (Bellesi et al., 2014). The amount of SWA is homeostatically regulated; SWA increase after a learning task concerning specific brain areas is accompanied by improved performance after sleep (Huber et al., 2004). In line with this local plasticity exerted by the SWA it was demonstrated that the immobilization of the subject's arm during the day was accompanied by local SWA reduction during the subsequent sleep (Huber et al., 2006). This is indicative of a local synaptic depression linked to somatosensory and motor evoked potentials in the contralateral sensorimotor cortex and a deterioration of the motor performance.
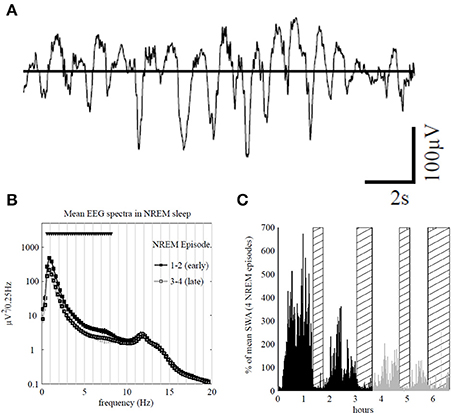
Figure 3. (A) Top traces: representative 16 s electroencephalogram (EEG) traces from the Fp1 channel during early sleep. (B) Average power spectra in non-rapid eye movement (NREM) sleep during episodes 1 and 2 (early sleep, black) and episodes 3 and 4 (late sleep, gray) for Fp1 channel (mean ± SEM, n = 7). Triangles indicate significant bins based on SnPM (P < 0.05, single threshold corrected). (C) Slow-wave activity (SWA; 0.5–4.0 Hz) profile in NREM sleep during the night for an individual subject (average 1-min values, % of the mean of 4 NREM episodes) rapid eye movement (REM) episodes are indicated by hatched areas. Early and late sleep (including REM episodes) are color-coded in black and gray, respectively. Note that 69% of the sleep time corresponds to NREM, 23% of REM and the rest of time refers to waking after sleep onset. In addition, about 17% of the NREM correspond to the SWS (adapted from Riedner et al., 2007, with permission).
Fast Sleep Spindles (13–15 Hz) and New Motor Sequences
It is interesting to note that in spite of the fact that the 1 Hz oscillation largely dominates the EEG frequency spectrum, a clear alpha peak (~12 Hz) corresponding to sleep spindle activity remains stable during all the NREM episodes (Figure 3B). The studies related to the effects of sleep spindles on motor performance showed that fast spindles (13–15 Hz) and not the slow spindles (11–13 Hz) were favorable for the consolidation of new motor sequences (Barakat et al., 2011; Astill et al., 2014). Another example of local cortical plasticity and sleep oscillations was given by the effect of a long-term synaptic potentiation (LTP) produced with transcranial magnetic stimulation (TMS) over the contralateral motor cortex (De Gennaro et al., 2008). Performed during waking, this LTP affected delta and theta EEG power in both NREM and REM states, recorded during the following night, in such a way that SWA was increased in frontal and prefrontal cortex and decreased in the contralateral sites of the stimulated motor cortex (De Gennaro et al., 2008). Similar relationships between SWA and LTP were demonstrated in long-term synaptic depression (LTD) (Bergmann et al., 2008). These experimental evidences corroborate the synaptic homeostasis hypothesis of Tononi and Cirelli (2014), who suggested that sleep is the price the brain pays for plasticity. According to this hypothesis, the oscillatory activity of normal sleep allows the brain to reestablish synaptic and cellular homeostasis which has been confronted to environmental demands implicating energetic supplies and plastic changes occurring during wake. In the same vein, the restorative function of sleep also consists of removing potentially neurotoxic waste products that accumulate in relation to awake brain activity (Xie et al., 2013).
The Sharp Wave-Ripple (SPW-R)
The SPW-R is another crucial oscillation in the memory consolidation process (see details in Figure 4; O'Keefe, 1976; Buzsáki et al., 1992; Ylinen et al., 1995; Buzsáki, 2006). Generated by an intrinsic self-organizing process assuming the mechanism of information transfer from the hippocampus to neocortex during off-line states such as NREM sleep (Buzsáki and Watson, 2012; Watson and Buzsáki, 2015), the SPW-R is considered to link pre-existing and recently acquired information in order to influence future decisions and actions favoring creativity (Buzsáki, 2015).
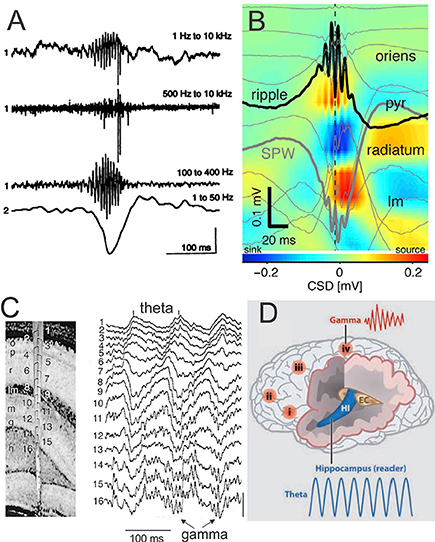
Figure 4. SPW-R is composed by a large and sharp amplitude depolarization (SPW) of about 40-100 ms recorded in the hippocampus CA1 stratum radiatum and most often associated by brief and fast LFP oscillation (~110–200 Hz) known as “ripples” (R) in the CA1 pyramidal layer. The SPW-R represents one of the most synchronous network patterns implicating synchronicity of 10–20% of hippocampus neurons (Chrobak and Buzsáki, 1994). (A) Fast field oscillation in the CA1 region of the dorsal hippocampus. Simultaneous recordings from the CA1 pyramidal layer [electrode 1, wide-band recording (1 Hz–10 kHz)] and stratum radiatum (electrode 2, unit activity 500 Hz–10 kHz and fast field oscillation (100–400 Hz). The second and third traces are digitally filtered derivatives of the wide-band trace (1). Note simultaneous occurrence of fast field oscillations, unit discharges, and sharp amplitude depolarization (electrode 2). Calibrations: 0.5 mV (trace 1), 0.25 mV (traces 2 and 3), and 1.0 mV (trace 4) (from Buzsáki et al., 1992, with permission). (B) Depth profile of averaged sharp-wave ripples (SPW-R) in a freely moving mouse (n = 961 events; vertical site separation: 100 mm). Voltage traces (light gray) are superimposed on current-source density (CSD) map. Black trace: site of maximum amplitude ripple; heavy gray trace: site of maximum amplitude SPW (adapted from Stark et al., 2014, with permission). (C) Depth Profile of theta and gamma oscillations in the rat recorded during exploration (left) with a 16-site silicon probe introduced in the CA1-dentate gyrus axis. Numbers indicate recording sites (100 μm spacing). Note the gradual shift of theta phase from str. oriens to str. lacunosum-moleculare (right) and the emergence of gamma waves superimposed on theta oscillation mainly in the granule cell layer and the hilus. Vertical bar: 1 mV (from Bragin et al., 1995, with permission). (D) Schematic representation of the brain showing location of γ oscillations in different cortical areas (i–iv) and Θ oscillation in the hippocampus (HI) entorhinal cortex (EC). These brain oscillations can influence each other within and across networks by modulating the phase and/or the amplitude of the oscillations (modified from Buzsáki and Watson, 2012, with permission). Abbreviations: p or pyr, pyramidal layer; lm, str. lacunosum-moleculare; o, str. oriens; r, str. radiatum; lm, str. lacunosum-moleculare; g, granule cell layer; h, hilus.
Viscerosensory Brain, Emotion, and Theta Oscillation during REM Sleep
In accordance to functional continuity between sleep and wake state, and its role in memory consolidation (Nishida et al., 2009), theta oscillation may be detected during REM-sleep (Marzano et al., 2011) Various aspects of memory processes have been the focus of increasing interest in sports. The viscerosensory brain activation linking central and peripheral emotion-signaling systems suggests a role for REM sleep in affective brain recalibration as recently demonstrated by testing the social threat discrimination (Goldstein-Piekarski et al., 2015). The influence of emotion in sport has been well-demonstrated (Lane et al., 2011; Uphill et al., 2014). For exemple, better physical performances occurred when the athlete recall anger or happiness emotions in contrast to the emotion-neutral state (Rathschlag and Memmert, 2013). Pleasant (as opposed to unpleasant) emotion is associated with an increase in frontal midline theta power (Sammler et al., 2007).
Prefrontal Cortex and Sport
Interestingly, it was demonstrated that cathodal transcranial direct current stimulation (tDCS) applied over the left dorsolateral prefrontal cotex (PFC) facilitates implicit motor learning in a golf putting task (Zhu et al., 2015). Running enhances cognitive tasks performance associated to astrocytic and neuronal plasticity in the PFC (Brockett et al., 2015). These elements may suppose an important role played by the relative hypoactivation of the PFC during dreaming (Maquet et al., 1996; Hobson et al., 2000) and call for further examination of PFC oscillations the days and nights before sport competition.
The Place Cells and the Grid Cells: A Basic Template for Navigation in Sport
The different hippocampal oscillations are highly relevant for sport performance. In addition to its crucial role in the consolidation of declarative memory (Squire, 1992), the hippocampus and related network are crucial for navigation. Indeed, place cells in the hippocampus (O'Keefe, 1976), head-direction cells in the anterior thalamic nucleus (Stackman and Taube, 1997; Giocomo et al., 2014; Winter et al., 2015), border cells (Solstad et al., 2008), boundary vector cells (Lever et al., 2009; Stewart et al., 2014) and grid cells in the medial entorhinal cortex (MEC) (Hafting et al., 2005; Fyhn et al., 2008; Moser et al., 2008; Ray et al., 2014) must be considered as the most basic elements supporting strategic navigation and self-positional control of the body. They may be thus translated for any action in the space field of human performance (Figure 5).
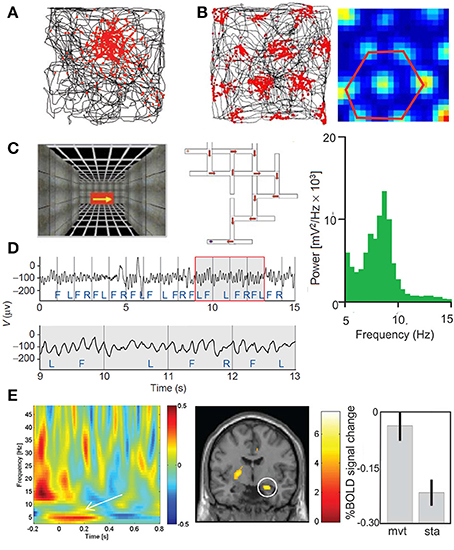
Figure 5. (A) Place cell in the hippocampus (adapted from Moser et al., 2008, with permission) and (B) grid cell in the medial entorhinal cortex. The locations where the neurons discharge (red) are superimposed on the rat's trajectory (black) in the recording enclosure black. Whereas, most of the place cells have a single firing location, the of a grid cell form a periodic triangular matrix of the firing fields covering the entire environment available to the animal. (C–E) Theta oscillations revealed by intracranial EEG and MEG in the human. (C) Navigation in virtual reality-rendered multiple T-junction mazes in which key presses move the subject in the VR maze (F, forward; R, right; and L, left). (D) Sample intracranial EEG recorded in the inferior frontal gyrus (upper trace); theta oscillation is emphasizing in an enlarged view of the boxed region (lower trace). The average power in iEEG for one trial through the VR maze during 21 s is represented by the power frequency plot (green) showing a peak in the theta band (4–12 Hz) (adapted from Kahana et al., 1999, with permission). (E) Movement-related MEG time-frequency effects and related fMRI effects. Plots show MEG signal as baseline corrected log normalized difference scores (dB, z axis) against time (x-axis, seconds) and frequency (y-axis, Hz), averaged across 18 participants. The effect of movement initiation during navigation (left panel) is showed at an exemplary single sensor of interest, (posterior right middle temporal location). Coronal slices showing right hippocampal activation for movement initiation compared to stationary periods (middle panel). Percent signal change at right hippocampal peak-voxel averaged across 14 participants for movement and stationary periods (mean 6 SEM) (right panel) (adapted from Kaplan et al., 2012, with permission).
Following the influence of the oscillatory dynamics of the hippocampus and entorhinal cortex in memory (Squire, 1992) and spatialization (O'Keefe and Burgess, 2005; Buzsáki and Moser, 2013), navigation in a real physical world or in mental space might use similar neuronal processing and might be consequently trained during mental motor imagery tasks (de Lange et al., 2008; Cevallos et al., 2015) or during VR observation (Zarka et al., 2014) or navigation specifically dedicated to sport performance or to neurological rehabilitation and prevention.
Delta Oscillation
Delta-band oscillations have long been believed to reflect periods of deep sleep (Figure 3; Steriade, 2006). However, they have recently been shown to be both stimulus informative (Montemurro et al., 2008) and linked to attentional selection (Lakatos et al., 2008). Following Whittingstall and Logothetis (2009), delta oscillation might represent the cyclical variations in the excitability of the neuronal pool represented by multiple unit activity (MUA) and the network state and might amplify (in case of high-excitability phase) or suppress (in case of low-excitability phase) the input signals. Interestingly, this also corroborates human MEG recordings where the success in visual discrimination task depended on effective coupling between delta oscillation (1–5 Hz) and high-frequency oscillations in the gamma band (~ 63 Hz) (Händel and Haarmeier, 2009). This coupling is also recognized in the conscious access to visual target representation (Nakatani et al., 2014). As skill ability largely depends on visual discrimination, which is also dependent on the cerebellar projections onto the PFC and parietal cortex (Händel et al., 2009), a specific attention should be directed to delta-gamma coupling of the brain rhythm during sport activity. In this context, it is crucial to rule out contamination of EEG signals by EMG activity, movement artifacts, including from physiological activities (Ito et al., 2014; Lechinger et al., 2015). As mentioned above, the phase of delta oscillation plays important role when it facilitates MUA. It is also involved in predictive timing, explaining why the human beat processing is better when the stimulus tempo is in the delta range (1–3 Hz) (Cirelli et al., 2014).
Theta Oscillation
The medial septum-diagonal band of Broca (MS/DBB) reciprocally connected to the supramamillary nucleus plays the role of “theta pacemaker” for the hippocampal theta oscillation (Alonso et al., 1987; Vertes and Kocsis, 1997). It is modulated by different brainstem regions, including the nucleus incertus (NI), located in the dorsal tegmentum. The convergence of experimental observations about the NI and theta oscillation underlines the interest for future investigations of EEG theta in movement performance: (1) the electrical stimulation of the NI evoked theta oscillation in the rat hippocampus (Nuñez et al., 2006); (2) the NI neurons projecting to the cerebellar flocculus discharge in a burst-tonic mode in perfect correlation with the velocity and position of the eye in the cat (Cheron et al., 1995); (3) the theta hippocampal oscillation is synchronized with that in the cerebellum during eye-blink trace conditioning in the rabbit (Hoffmann and Berry, 2009; Wikgren et al., 2010). For all of these reasons future investigations of theta oscillation during head-eye movement in sports should be promising.
A clear theta peak at ~6 Hz has been recorded in hippocampal intracranial EEG (iEEG) in human (Kahana et al., 1999; Figures 5C–E). More recently, a “slow-theta” (~3 Hz) (memory related) and a “fast-theta” (~8 Hz) oscillation (not memory related) were defined (Lega et al., 2012, 2014; Pastötter and Bäuml, 2014).
Phase-Amplitude Theta-Gamma Coupling
Theta oscillations enforce the timing of coordinated firing of neuronal populations, (Buzsáki, 2002; Buzsáki and Chrobak, 2005; Buzsáki and Watson, 2012). Recently, intracranial recordings in human demonstrated that hippocampal and neocortex phase amplitude coupling (PAC) exerted by slow-theta oscillation, respectively at 2.5–5 and 4–9 Hz modulates gamma activity. As episodic memory contains different types of information (semantic, spatial, emotional, and context dependence), it was suggested that the reinstatement of a specific context (integrating brain state dynamics and external elements) expressed into specific neuronal oscillations and spiking was at the basis of a novel model of episodic memory called “SCERT” (spectro-contextual encoding and retrieval theory) (Watrous and Ekstrom, 2014). This theory might offer testable predictions about the possibility to reinstate an episodic memory of a sport event by using VR realistic scenario and or motor imagery able to reproduce the cross-frequency coupling (e.g., delta-gamma or theta-gamma) and phase-synchronization originally present in the hippocampal-neocortical neuronal assemblies during mnemonic formation.
Theta Oscillation and Speed Cells
The dynamic aspect of self-representation in speed running is a crucial element for keeping the good trajectory for the best record. The recent discovery of the “speed cells” in the medial entorhinal cortex in the rat providing linear information to the grid cells (Hafting et al., 2005; Moser et al., 2008) represent a key element of dynamics representation of self-body location (Kropff et al., 2015). This discovery corroborates the fact that delta and theta oscillations recorded in human hippocampus (Watrous et al., 2011) during VR navigation increased their power not only as a function of spatial view (relevant goals and or spatial updating as initially demonstrated by for theta oscillation by Kahana et al., 1999) but also as a function of the speed of the VR image). This is also supported by the subdural grid and intracerebral microelectrode recordings in epileptic patients using a virtual taxi driver task (Caplan et al., 2003). These authors propose that the cortical theta reflects two functions depending of the behavioral context: (1) during goal-seeking, theta oscillation updates the motor plans in function of the incoming sensory information related to wayfinding; (2) during exploratory searching behavior, theta oscillation helps the encoding of coordinated activity in the multiple brain areas involved in building the individual's cognitive map. Theta rhythm also contributes to encoding the “where” and “when” of episodic memory (see Hasselmo and Stern, 2014, for a review).
Theta in Motor Control
Theta oscillation plays also an important function in motor control. Recently, it was demonstrated that the theta power increase (ERS) in the contralateral motor area during the onset of fast ballistic movements (Ofori et al., 2015). Moreover, theta oscillation was phase-locked with the onset of the movement and the theta power correlated with movement acceleration (Ofori et al., 2015). A better performance in expert golfers was reported to be associated with higher fronto-midline EEG theta (FM theta) power and higher parietal upper alpha with respect to novices (Baumeister et al., 2008). In the same vein, Doppelmayr et al. (2008) showed a FM theta power increase for the last 3 s before the riffle shooting only in experts, compared to novices. Inverse modeling (LORETA) revealed theta source in the medial frontal cortex and anterior cingulate area underlying focused attention. Finally, (Bailey et al., 2008) reported an increase in theta activity above resting levels at higher workloads and at fatigue during graded cycle exercise.
The Alpha Oscillation
As the first recorded EEG wave (Berger, 1929), the alpha rhythm mainly appears as spindle-shaped episodes of 10-Hz oscillation that dominate the spontaneous activity of the brain in the relaxed eyes-closed state. When the eyes open the power of the alpha oscillation decreases (arrest reaction) (Figure 6) whereas faster oscillations with smaller amplitude occurred. The transition between these two eye states (closed vs. open) may provide an interesting index of alpha rhythm reactivity and may help to give direct information to the trainer about the global resting state activity of the athlete brain. For this we have defined a suppression coefficient (SC) as the peak value resulting from the subtraction of the power spectrum recorded in the eyes-closed state from that recorded in the eyes-opened state (Cheron et al., 2006).
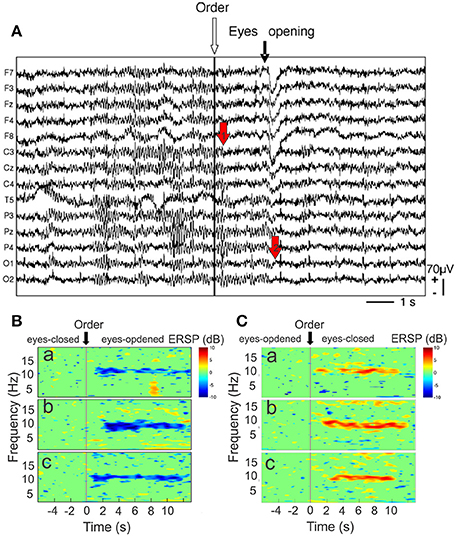
Figure 6. (A) Raw EEG recordings during the arrest reaction. Fourteen EEG channels referenced to linked mastoid (from F7 top) to O2 (bottom). The white arrow indexes to the order to open the eyes. The black arrow points to the onset of the eye movement artifact related to eye opening, mainly recorded by frontal electrodes (F7, F3, FZ, F4, F8). Note that the amplitude of mu rhythm [recorded by central electrodes (C3, CZ, C4)] is reduced before eye opening (red arrow “1”) whereas alpha rhythm (P3, P4, PZ, O1, O2) is only reduced after this movement when the eyes are closed. Event-related spectral perturbation (ERSP) time-locked to the order of eyes opening. (B) ERSP-image plot of the color-coded single trials of the EEG spectrum recorded in P3 channel in one cosmonaut on Earth before the flight (a), in weightlessness (b), and on Earth after the flight (c). The trials are time-locked to the order to open the eyes (stripped line). Blue color indicates a decrease in power. (C) ERSP-image plot for the same electrode, condition and participant as in (B), but time-locked to the order of eyes closing. Red color indicates an increase in power (modified from Cheron et al., 2006, with permission).
The large amplitude of the alpha rhythm would result from a coherent cortical drive from the thalamus coincident with a lack of other sensory input. In this theoretical framework, that is compatible with Crick's searchlight hypothesis (Crick, 1984), a relaxed but well-focused athlete would demonstrate large alpha amplitude before the accomplishment of the motor task. The alpha rhythm has also been considered as a mechanism for increasing signal-to-noise ratios within the cerebral cortex by means of inhibition of unnecessary or conflicting processes to the current task (Klimesch, 1999; Klimesch et al., 2000; Cheron et al., 2006; Sadaghiani et al., 2012). In sport, the perturbation of the reference frame due to the modification of the environmental field and the sensory conflicts which could be produced by a new competitive field associate to psychological pressure could necessitate such a type of regulation.
Generated by interplay of the cortex and the thalamus and modulated by frontocorticothalamic loops, involving the reticular formation, and the pulvinar nucleus (Saalmann and Kastner, 2009, 2011) the 10 Hz oscillations are in fact composed of different rhythms depending of the sensorimotor modalities: the alpha oscillations of the visual system, the mu oscillations of the sensorimotor system (Gastaut, 1952; Arroyo et al., 1993; Ai and Ro, 2014) and tau oscillations of the auditory system (Lehtelä et al., 1997; Walz et al., 2015). ERD of mu rhythm also occurred when individuals (in an identification perspective with the subject on screen) observed movies showing biological motion (e.g., boxing) (Gastaut and Bert, 1954). Mu rhythm is maximally expressed over the sensorimotor areas during a relaxed state (Pfurtscheller and Neuper, 1994; Pfurtscheller et al., 1997) and reduced just before, during movement execution, tactile stimulation and motor imagery a condition interpreted as ERD (Figure 6B) in contrast to an amplitude enhancement referred to ERS (Figure 6C). Interestingly, the association of anodal transcranial direct current stimulation (tDCS) and motor imagery, two conditions known to increase the cortical excitability of the motor cortex (Nitsche and Paulus, 2001; Facchini et al., 2002, respectively), increase the ERD amplitude during motor imagery (Cebolla et al., 2015) in particular at the level of dominant hand (Kasuga et al., 2015). As motor imagery is more and more used in motor skill training (Schuster et al., 2011), the conjunction of tDCS, which is able to induces motor imagery (Speth et al., 2015), VR stimulation and motor imagery controlled by mu oscillation recording should be the future avenue for increasing CNS performance.
The Neural Efficiency Hypothesis
In line with the “neural efficiency” hypothesis suggesting that individuals with higher intelligent quotient (IQ) have lower brain activation than less bright individuals when functioning on the same cognitive tasks (Dunst et al., 2014), the same hypothesis has been extended to expert and athlete performances (Del Percio et al., 2009b, 2010, 2011; Babiloni et al., 2010). Based on the existence of an inverse relationship between IQ and the amount of upper alpha ERD (Grabner et al., 2004), it was proposed to use the classical “arrest reaction” to eye openings to quantify the alpha ERD amplitude in different participant groups. Namely, it was shown that elite karate athletes' brain is characterized by less ERD at the “arrest reaction” with respect to non-athlete participants (Del Percio et al., 2011). This corroborate previous experiments of the same group showing that elite athletes presented less alpha ERD during air pistol shoot than non-athletes (Del Percio et al., 2009a). However, the generalization of the alpha ERD must be cautiously made and greatly depends on the experimental condition. Indeed, during a body–sway paradigm the athletes have demonstrated a greater alpha ERD reflecting a better balance control than non-athlete (Del Percio et al., 2007).
Alpha Oscillation in Binding or Gating
Alpha oscillation is now increasingly regarded as reflecting a global inhibition of the cortex in order to exert a cognitive control of the final performance (Klimesch et al., 2007; Haegens et al., 2010). In this context, two seemingly opposite functions are operational. In the first function, the synchronization of alpha oscillations appears to integrate the multisensory factors into segregated chunks of information necessary for the binding of the inter-personal action perception loops. For example, Babiloni et al. (2008) reported that in golfers, the amplitude of the 10–12 Hz frequency alpha ERD was higher during the successful compared to the unsuccessful putts over the frontal midline (Fz and Cz electrodes), this increase reflecting fine motor control and balance abilities. In contrast, Taliep and John (2014) showed a significantly greater high-alpha (10–12 Hz) ERS prior to ball release. In the second function, the increase in alpha power may exert a “windshield wiper” effect through pulsed inhibition in order to select or gate the incoming signals (Sadaghiani et al., 2012). For example, in golfers, increased alpha activity in the left hemisphere was associated, and able to predict less error in the subsequent golf putt (Crews and Landers, 1993). This is consistent with subsequent finding of an increased alpha power for the best compared to worst shots in elite archers (Salazar et al., 1990). On the contrary, (Hillman et al., 2000) found an increase in alpha across both cerebral hemispheres during the preparatory period preceding shots for rejected (i.e., that were finally not accomplished), compared to executed shots. In the same vein, Dekker et al. (2014) demonstrated that feedback on alpha power (eyes open) based on music training in gymnast produce small improvements in sleep quality, mental, and physical shape. In absence of heart rate deceleration, alpha power was found to be greater in the left than in the right hemisphere during the period preceding the shot in elite archers (Salazar et al., 1990). In addition, this contralateral alpha power was greater for the best than the worst shots.
Alpha Oscillation Phase
Another important function of alpha oscillation is made by its ongoing phase. By selecting the EEG trials that coincide with the P1 component generation of the visual evoked potential related to a recognition task, Gruber et al. (2014) showed that trials presenting alpha phase in the same polarity that the P1 component were associated with shorter target decision times than the rejected trials presenting counter phases to the P1. These results paves the way for precise checking of alpha phase oscillations during sport performance and direct BCI procedure based on instantaneous oscillating phases.
Alpha and Physical Exercise
Many studies showed an association between different stages of exercising and modulations of the alpha rhythm. The findings are however very inconsistent. While the transition from rest to exercise has been linked to an increase in alpha (Nielsen et al., 2001; Rasmussen et al., 2004), the during and after exercise periods have been associated with both upward (Mechau et al., 1998; Rasmussen et al., 2004; Bailey et al., 2008; Schneider et al., 2009; Fumoto et al., 2010; Brümmer et al., 2011; Gutmann et al., 2015; Robertson and Marino, 2015; Mierau et al., 2016) and downward variations (Kubitz and Mott, 1996; Mechau et al., 1998; Doppelmayr et al., 2008, 2012; Schneider et al., 2009; Ludyga et al., 2015). Faced to apparently discrepant results about alpha rhythm behaviors related to exercise, and the existence of complex variation of the resting state EEG dynamics (Goldberg et al., 2008), we would suggest using better controlled paradigms linking alpha oscillation and specific sensory, cognitive, or motor triggering events.
Beta Oscillation
Beta Oscillation in Motor Control
Beta oscillation (15–30 Hz) occupies a central position in the treatment of sensorimotor information and serves as a functional link between different brain regions recorded in the monkey such as the pre-motor (Lebedev and Wise, 2000) motor (M1) (Donoghue et al., 1998; Reimer and Hatsopoulos, 2010) and somatosensory (S1) cortex (Lebedev and Nelson, 1995; Murthy and Fetz, 1996) the supplementary motor area (Hosaka et al., 2015) and the cerebellum (Courtemanche et al., 2002; Courtemanche and Lamarre, 2005). In these regions, beta LFP oscillations have been recorded in quietly sitting normal animals when they are immobile in expectancy and are inhibited by passive or active movements (Courtemanche and Lamarre, 2005). In the cerebellum, beta oscillations were localized in the granular layer where they are synchronized along the rostro-caudal module serving as a coordinated timing template (D'Angelo and De Zeeuw, 2009; Cheron et al., 2015). This oscillation is thus involved in movement preparation during which the Purkinje cell (PC) discharges (the sole output of the cerebellar cortex) is mainly phase-locked to this oscillation just before the execution of movement (Courtemanche and Lamarre, 2005). The phase relationship between beta oscillation, PC firing and movement execution is crucial.
By this way, beta oscillation could play the role of a motor “binding” linking the different commands related to a global gesture as suggested for sensory awareness (Engel and Singer, 2001). In the context of sport competition when exercise-induced-muscle-damage it was reported that the beta oscillation increase in relation to a force output decrease, neurosmuscular alteration and fatigue (Plattner et al., 2014).
In human cortex, Kuo et al. (2014) showed that beta-band (14–30 Hz) ERD (power decreases) was significant during finger movement, expressed in all participants and consistently localized to the primary motor cortex (hand region) and in well-accordance with fMRI localizations. As described for the mu rhythm, beta ERD also occur during both movement execution (Ofori et al., 2015) and observation (Zarka et al., 2014; Cevallos et al., 2015; Babiloni et al., 2016). This evidence was recently reinforced by LFP recordings in the monkey showing modulation of beta power in M1 and premotor cortex during action execution and observation (Waldert et al., 2015).
Beta Oscillation in Sensory Functions
In addition to sensorimotor area, beta oscillations play other important functions in pure sensory domains. For example, prominent increase in beta power (14–16 Hz) was reported for contour compared to non-contour conditions during a visual contour detection task (Volberg and Greenlee, 2014).
Beta oscillations were also modulated by somatosensory input (Pfurtscheller et al., 2001). More recently, in a new paradigm (Cebolla et al., 2014) based on the amplitude increase of the N30 component of the somatosensory evoked potential (SEP) during the observation of another person's hand movement (Rossi et al., 2002), we have demonstrated that beta ERS in a large central-posterior area extending from the contralateral motor cortex through the secondary somatosensory cortex to the insular and in the occipital secondary visual cortex participated to the N30 amplitude increase. In addition, beta phase-locking (ITC) was found in a large region including the premotor, motor, and somatosensory cortex and the angular gyrus (BA39) considered as an important node in the mirror neuron network (MNN) (Cebolla et al., 2014). These results demonstrate that beta oscillation participates in the top-down influence exerted by the MNN on the early evoked SEP component (Cebolla and Cheron, 2015).
Beta Oscillation and Automatic Nervous System
Recently, the low-frequency beta oscillations recorded in the Rolandic rhythms were demonstrated to be related to heart rate regulation by sympathetic activity (Triggiani et al., 2015). Beta oscillation can be thus viewed as a dynamic biomarker involving somatomotor control and ANS signals regulation.
The fact that contrasted results were obtained about the relationship between heart rate deceleration and performance accuracy (Konttinen et al., 1998; Tremayne and Barry, 2001) calls for direct investigation of the EEG rhythms during the preparatory phase of a movement skill. Interestingly, by linking motor imagery of a brisk movement of the leg and the go/no-go paradigm, Pfurtscheller et al. (2013) demonstrated that beta ERD was stronger for the go imagery than in the no-go imagery condition and that the heart rate deceleration was smaller and followed by an acceleration in go as compared to no-go. These authors interpret that this beta ERD increase was due to the increased mental effort linked to the imagery process.
The Gamma Oscillation
Following Steriade (2003) the first description of gamma oscillation was made by Bremer et al. (1960) and described as an “accélération synchronisatrice” of the cortical EEG when the brainstem reticular formation was electrically stimulated.
Binding-by-Gamma Oscillation
The first demonstration of the functional role of neuronal gamma-band synchronization was provided by invasive microelectrode recordings of spikes and LFP in the visual cortex of anesthetized cats (Eckhorn et al., 1988; Gray et al., 1989; Gray and Singer, 1989; Engel et al., 1991) and later confirmed in visual cortex of alert monkey (Kreiter and Singer, 1996; Fries et al., 2001). These important experiments have paved the way for the binding-by-gamma oscillation originally promoted by Freeman (1978) in the olfactory bulb. Novel signal-processing techniques for the detection of transient oscillatory events and the introduction of new source modeling methods (Lachaux et al., 2003; Delorme and Makeig, 2004; Palmero-Soler et al., 2007; Cebolla et al., 2011; Delorme et al., 2015) have facilitated the study of gamma oscillations in human EEG (see Jensen et al., 2007, for a review).
Since pioneering MEG studies (Ribary et al., 1991; Llinás and Ribary, 1993), the interest in the study of gamma oscillation with EEG and MEG increased strongly and their involvement was described in relation to sensorimotor task (Murthy and Fetz, 1992; Donoghue et al., 1998; Aoki et al., 1999), perception (Tallon-Baudry et al., 1996, 1997, 2005; Gruber et al., 2002), attention (Gruber et al., 1999; Gruber and Müller, 2006), working memory (Tallon-Baudry et al., 1998; Lutzenberger et al., 2002) and associative memory (Gruber et al., 2001). In gamma EEG recordings, Yuval-Greenberg et al. (2008) have demonstrated that the induced gamma-band EEG response occurring about 200–300 ms following stimulus onset correspond to miniature saccade dynamics rather than neuronal oscillations. However, this study does not raise any doubt regarding the intracranial recordings and the important role of gamma-band activity in neural function, but it urgently demonstrated the necessity to control not only the concomitant EMG and eye saccades but also the microsaccades events (Keren et al., 2010; Hassler et al., 2011, 2013) during any type of sport behaviors.
Adaptation of Oscillatory Models to Sports Performance
The delayed match to sample (DMS) with distractors task (Miller et al., 1991) has been used for testing the accuracy of a working memory (WM) model based on recurrently coupled networks of spiking neurons (Dipoppa and Gutkin, 2013; Figure 7A). A first visual sample cue is presented to the subject, after a delay period during which a random number of distractor cues are presented, the subject must only respond when the test cue matches the sample cue. This “go, no-go” WM task can be easily transposed in sport competition (starting for speed running or passing ball in soccer). In this later example (Figure 7B), the first visual cue can be represented by the presence in one part of the soccer field of a targeted teammate (n°2 in Figure 7B, gate-in), all the rest of non-targeted teammates corresponding to “no-go signals” already moving during a delay period during which distractor cues may occurred (black birds) (Figure 7C, selective gating). Only when the targeted teammate is recognized in a profitable situation the pass must be accomplished (Figure 7D, gate-out).
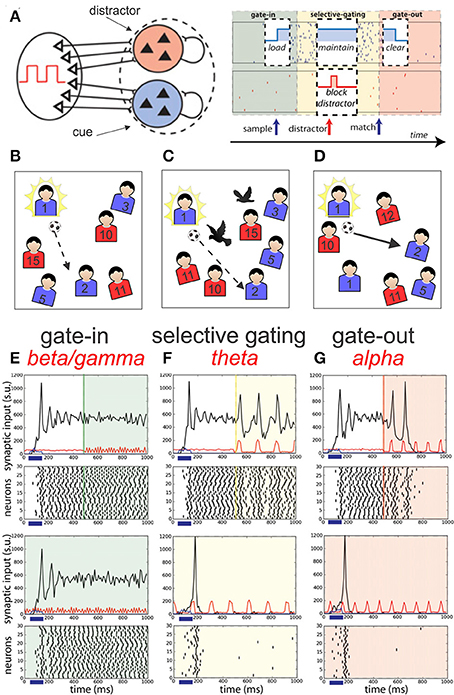
Figure 7. Schematic representation of the network models of Dipoppa and Gutkin (2013) dedicated to a working memory task. (A) Two neuronal populations B (in blue) and R (in red) of recurrent network receiving input by sources modulated by the shared background oscillation. The phases of operations are outlined in a white box showing the succession of the gating modes and operations: (I) Gate-in mode: the sample stimulus (blue arrow) activates population B (load). (II) Selective-gating: the distractor stimulus (red arrow) is not able to activate population R persistently (block distractor) and the memory in population B is held (maintain). (III) Gate-out mode: upon match-stimulus presentation, persistent activity is deactivated in the blue population (clear). Input oscillations enabling the gating modes: beta–gamma band ensures the gate-in mode at the beginning of the task, theta band ensures the selective-gating mode during the delay period (memory maintenance together with protections from the distractors), and alpha band ensures the gate-out mode at the task completion (memory is rapidly cleared). (B–D) Adaptive suggestion of this model into sport context (passing ball in soccer) (see main text for more details). (E–G) Frequency-dependent influence of oscillations on the reverberant spiking persistent state. The network responses (black) to a transient excitatory external stimulus (t = 50–150 ms) (blue bar and signal) depend on the frequency content of the background oscillatory input. First and third rows show the raster histogram of 30 representative neurons; second and fourth rows show average population input from recurrent connections (black), background activity (red), and external stimulus (blue) in arbitrary/normalized units. For each frequency the background oscillation is switched on either after the stimulus presentation (first and second rows) or before (third and fourth rows). (E) Beta–gamma-band oscillations (45 Hz) are compatible with persistent state maintenance: neither erasing nor blocking is seen. (F) Theta-band oscillations (6.5 Hz) maintain an a priori persistent state while blocking de novo activations. (G) Alpha-band oscillations (here 10 Hz) inhibit reverberant activity: the persistent state is deactivated by oscillations onset and is prevented from being activated by the transient stimulus (adapted from Dipoppa and Gutkin, 2013, with permission).
Dipoppa and Gutkin (2013) realized a computational framework that mechanistically links the four main oscillatory bands (alpha, beta, gamma, and theta) into a working memory (WM) network. In this model the coexistence of a ground state and persistent activity is allowed by the beta-gamma oscillation in such a way that transient input can initiate a persistent state corresponding to the gate-in mode of the dynamic gating regime (Figures 7E–G). During this mode the sample or targeted cue is acquired by the network. Then, the theta-band oscillations (3–8 Hz) assume a selective-gating mode maintaining in memory the previously loaded cue, assuming also a protection against distractors. Finally, a gate-out mode is provided by alpha-band oscillations (8–12 Hz) avoiding loading or maintenance of memory facilitating the memory cleaning and task completion.
This model includes two stimulus-selective neural population units (R representing red or B for blue) that are independently activated from a resting state to a persistent state by a sensory stimulus (Figure 7A). Although rather complex this model allows a better understanding of the dynamics links between persistent activities, transient input activation and the influence of different frequency band oscillations introduced in the network. For example, the Figures 7E–G shows that a transient excitatory input given between 50 and 150 ms (blue horizontal bar) on the reverberating network of neurons increases the synaptic activities (black line) showing multiple decreasing peaks reaching a relatively stable regime. Then if a gamma oscillation is added in the network (see green line at 500 ms) the stable level of the acquired synaptic activities is maintained (Figure 7E). The presence of gamma oscillation (45 Hz) at the time arrival of the external excitatory signal is ineffective. If theta oscillation (6.5 Hz) is given (yellow line) in place of the gamma one (Figure 7F), the mean level of activities is maintained but a peak of activities is reactivated at each theta wave. In contrast, if alpha oscillation (10 Hz) is added in the network (red line) (Figure 7G) after two initial reactivation peaks the excitatory level goes to zero. In addition, if theta or alpha oscillations are present at the time arrival of the external input no stable level of excitation are attained.
Event Related Potentials in Sport Domain
As the majority of EEG studies devoted to sports have been included in the previous description of the different EEG rhythms, a review of the event related potentials (ERP) studies in sport is accomplished in the last part of the present review. Independently of the analysis of EEG content per se, two types of ERP studies have been conducted, one about the effect of chronic physical activity and the other about the effect of acute physical exercise.
Effects of Chronic Physical Exercise
The first set, which makes up the majority of the studies, focused on the relationship between neuroelectric modulations associated with cognitive functioning and engagement in chronic physical activity through the life span. Studies using tasks involving stimulus discrimination and attention processes found that chronic engagement in exercise and aerobic fitness are linked to higher capacities, as reflected for the most part by larger P3 amplitudes, themselves indexing increased attentional resources during stimulus encoding (Hamon and Seri, 1989; Polich and Lardon, 1997; Hillman et al., 2005; Pontifex et al., 2009; Chang et al., 2012; Taddei et al., 2012; Getzmann et al., 2013), and by shorter P3 latencies, marking greater cognitive processing speed (Dustman et al., 1990; Hillman et al., 2002, 2004, 2005; Chang et al., 2012; Taddei et al., 2012). These ERP modulations correlated with reduced reaction times and greater response accuracy in the tasks proposed. Only one study found opposite results, i.e., a more efficient capacity in perceptual decision making in baseball batters associated with longer P3 latency and reduced P3 amplitude (Radlo et al., 2001). Studies using the same type of tasks but which have looked into the effects of physical activity on pre-stimulus stages of motor preparation and very early stages of stimulus processing have also reported modulations of earlier components such as greater N1, (Hamon and Seri, 1989; Hung et al., 2004; Berchicci et al., 2015), P1, (Berchicci et al., 2015), P2 (Hamon and Seri, 1989), or N2 amplitudes (Taddei et al., 2012; Winneke et al., 2012), and shorter N2 latency (Taddei et al., 2012). Despite the difference in the tasks used, the majority of authors having investigated the ERP correlates of inhibition (Kamijo and Takeda, 2009; Stroth et al., 2009; Hillman et al., 2014; Chu et al., 2015; Gajewski and Falkenstein, 2015; Kamijo et al., 2015) have also reported increased P3 amplitudes. Greater amplitudes of CNV, indicating greater task preparation processes, as well as diminished N2 amplitudes, reflecting more efficient executive control processes, have also been reported (Stroth et al., 2009). These observations thus suggest a strong positive association between chronic physical activity and the performance in inhibition capacity.
Chronic exercise has also been found to be linked to smaller frontal CNV amplitude during the speed instruction in a Sternberg task (Kamijo et al., 2010, 2011), which indexes more efficient cognitive preparation for working memory. A facilitation of working memory in individuals practising physical activity has also been indexed by an increase in P3 (Chang et al., 2013; Dai et al., 2013) and N1 amplitudes (Chang et al., 2013) and a decrease in P3 latencies (Chang et al., 2013; Fong et al., 2014) in working memory paradigms, which suggests greater allocation and engagement of attentional resources and higher efficiency of stimulus evaluation. Numerous studies (Themanson et al., 2006; Themanson and Hillman, 2006; Hillman et al., 2009a; Pontifex et al., 2011) reported a relationship between higher chronic physical activity and reduced event related negativity (ERN) amplitude and greater post-error response slowing under speeded task instructions. These effects could be interpreted as a decrease in conflict monitoring or an inferior threshold for the moment at which the several processes necessary for the upregulation of cognitive control start to be activated.
Themanson et al. (2008) showed that fitness was associated with larger modulation of ERN amplitude and post-error accuracy crosswise the task instruction conditions during a task requiring variable cognitive demands and Pontifex et al. (2011), through manipulation of stimulus–response compatibility in a modified Flanker task, showed higher response accuracy, larger amplitudes and shorter latencies of the P3 and greater ERN amplitude modulation in higher aerobically fit participants. These two studies indicate increased cognitive flexibility of action monitoring in higher fit individuals. Hillman et al. (2006) and Kamijo and Takeda (2010) also found ERPs indices of higher cognitive flexibility in physically active individuals compared to sedentary ones. However, others researchers (Scisco et al., 2008) did not find any link between physical activity and cognitive performance in a task switching paradigm. This absence of association has been explained in difference in task difficulty of the tasks chosen (Hillman et al., 2012).
Finally, higher fitness appears to be linked to a richer network of words and their meanings, and a greater ability in detecting and correcting syntactic errors as indexed by the greater N400 amplitude and shorter latency and the larger P600 for syntactic violations during the reading of sentences (Scudder et al., 2014). It is important to note that the effects of physical activity on executive functioning-related ERPs are observed across all age groups. Higher levels of fitness indeed increase cognitive performance in adults (Themanson and Hillman, 2006; Bullock and Giesbrecht, 2014), but also in children (Drollette et al., 2014; Hillman et al., 2014) and may also help lightening cognitive decline with age (Hayes et al., 2013).
Effects of Acute Physical Exercise
The second set of researches has focused on studying the effect of acute physical activity (i.e., single bout of exercise lasting from a few seconds to several hours) on ERPs measurements. In this category, two types of work have been conducted. While some explored the effects of activity right following exercise, others studied the modulations of ERPs during exercise.
The studies that have concentrated on the after-effects of a single acute bout of exercise on neuroelectric indices of cognition have focused mainly on the ones reflecting inhibition (Kamijo et al., 2004, 2007, 2009; Hillman et al., 2009b, 2003; Drollette et al., 2014; Chuang et al., 2015; Chu et al., 2015; Kim et al., 2015) and stimulus inhibition discrimination processing (Nakamura et al., 1999; Yagi et al., 1999; Magnié et al., 2000; Kamijo et al., 2004; Duzova et al., 2005; Chang et al., 2012; Pontifex et al., 2015). A handful of studies also explored the correlates of action monitoring (Hillman et al., 2006; Chang et al., 2015) and attention related brain activity (Pontifex et al., 2013; Tsai et al., 2014; Chang et al., 2015).
Overall, these studies, across different cognitive paradigms, showed that exercising during 20–30 min usually lead to greater P3 amplitude and shorter P3 latency, thus reflecting respectively an intensification of the mobilization of attentional processes and an increase in speed of cognitive processing and stimulus classification. Moreover, these exercise-consecutive improvements in cognition abilities seem to be dependent of the intensity of the exercise. In particular, only moderate (in opposition to high or low) intensity exercise appears to be advantageous for ERPs components associated with cognition (Hillman et al., 2012).
The nature of the changes occurring in the brain during exercise has been very much less studied. As far as we know, and this is probably due to technical problems linked to artifacts associated with the gross motor movement intrinsic within physical activity, only four studies have acquired ERP data during acute exercise (Yagi et al., 1999; Grego et al., 2004; Pontifex and Hillman, 2007; Bullock et al., 2015) and different aspects of cognition have been approached by the different authors. Globally, modulations of the N1, P1, P2, and N2 components have been observed, suggesting a complex effect of exercise on the neural indices of information processing, but the small and disparate amount of literature available at this stage renders conclusions hard to be drawn today.
In summary, cumulating evidence demonstrates that higher engagement in physical activity and higher practice of aerobic fitness can have important sustained and transient benefits across the human lifespan. They are linked to modulations of ERPs which attest to increased cognitive functioning, especially as far as executive functions are concerned. Preliminary findings suggest that specific modulations also occur during physical activity participation but further examinations are needed in this domain.
Toward EEG Biomarkers in Sport
The Tables 1, 2 are constructed on the Pubmed search targeting EEG-sport and exercise and Evoked potentials-sport and exercise, respectively. This dichotomy between EEG and evoked potentials studies in sport domain is not surprising and follows the previous distinction existing in clinical studies. The EEG search resulted in about 30 studies, while the evoked potentials resulted in about 55 studies, the earliest dating back from 1989. These relatively small amounts of studies dedicated to sports compared to the large total number of EEG and evoked potentials studies raises serious questioning with regard to the interest in sports in the scientific community. In an optimistic view, we are thus at the beginning of new explorative era for which the establishment of a sound, comprehensive methodology will be helpful to better understand the brain functioning in sport performance.
It also appears that the dynamics aspects of the EEG have only been recently envisaged, in 9 studies (green text in Table 1). The practical conclusions are not obvious at this stage because of the variability in the sports studied, paradigms used, analysis methods and interpretations. In spite of this situation and the few discordances (cited above, Table 2) the conjunction of physiological conclusions adopted thanks to the development of basic research realized (both in animals and human) outside of the sport domain and the more practical investigations may allow to attempt to define the basic line of EEG biomarkers in order to explore the brain activity associated to sports. We may summarize a perspective view about five major EEG biomarkers hierarchically classified following the number of corresponding functions (see Table 3). These biomarkers could be used following EEG dynamics method and practically applied in sport domain. In order to avoid some confusions and insufficiencies in the treatment of these EEG biomarkers we recommended the adoption of an integrated approach (see below).
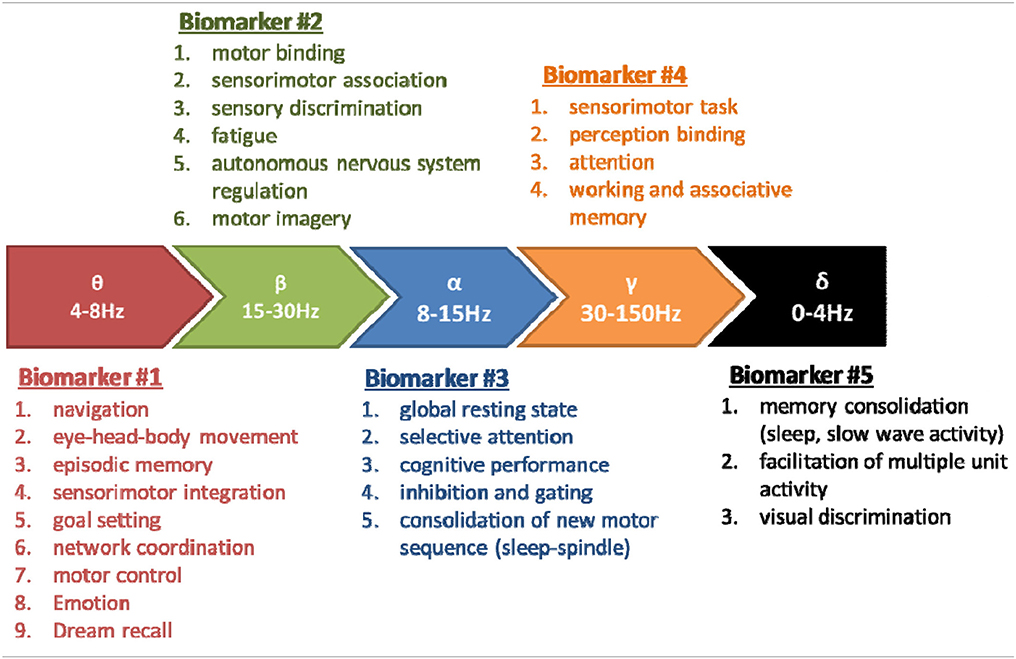
Table 3. Five EEG biomarkers hierarchically organized following the number of corresponding functions.
From Walking Studies to an Integrated Approach of Brain Activities in Sport
The recording of EEG during normal or treadmill walking (Gwin et al., 2010, 2011; Cheron et al., 2012; Gramann et al., 2014a,b) has paved the way for further investigations in sport movement involving higher acceleration and shocks than the cyclic walking movement. Adopting the independent component analysis (ICA) method, already used in walking studies by Gwin et al. (2010, 2011) we have shown contralateral modulations at electrode locations C3–C4 of the mu-beta and gamma oscillations in relation to the respective heel strikes (Cheron et al., 2012). This confirms previous studies (Gwin et al., 2010, 2011; Haefeli et al., 2011) and is line with more recent investigations (Severens et al., 2012; Wagner et al., 2012; Seeber et al., 2014, 2015; Gramann et al., 2014b; Bruijn et al., 2015; García-Cossio et al., 2015; Lisi and Morimoto, 2015). In addition, we have shown that it is possible to use, after some artifact processing, the two most representative independent components of the EEG located over the sensorimotor cortex as input for a dynamic recurrent neural network (DRNN) learning identification of the two principal components (PCk1-2) of the three elevation angles (foot, shank, and thigh) of one lower limb kinematics (Cheron et al., 2012). This could motivate further application joining EEG dynamics and DRNN learning in sport domain. In spite of these advances and in accordance with the recent review of Gramann et al. (2014a) the main obstacle for a direct application of EEG to all the movement phases in sport remains movement artifacts. During treadmill walking the signals issued from EEG cap and accelerometer (placed on the subject's head) exhibit similar time-frequency properties, especially in frequency bands extending from the low-delta to high-gamma bands (1–150 Hz) (Castermans et al., 2014). This finding questioned some conclusions drawn in previous EEG studies where low-delta band (~1 Hz, the fundamental stepping frequency) had been interpreted as related to the control of lower limbs during locomotion. Concerning the gamma band oscillation, the contamination of EEG signals by cranial, facial and neck muscles activities occurring mainly between ~20 and 300 Hz represent another difficulty for which different solutions have been recently reviewed (Muthukumaraswamy, 2013) providing satisfactory options. In contrast to movement and EMG artifacts which are largely distributed throughout the scalp or specifically distributed laterally (close to neck, temporal and frontal region), respectively, the sensorimotor or cognition-related oscillations (e.g., mu and beta) change within narrow frequency bands and in more restricted locations.
Since the early EEG studies of treadmill walking, the EEGLAB tool-box (Delorme and Makeig, 2004) has been used in order to suppress different types of artifacts by the ICA. This has afforded significant progress in the EEG and evoked potentials domains, but the important problem of the movement artifacts occurring during the crucial phase of sport movement still requires new technological advances are. An original procedure described by Kline et al. (2015) consists of recording pure movement artifacts from a standard EEG headset placed on a silicone swim cap. Interestingly, the observed ERD/ERS from 1 to 150 Hz in the artifact data were similar to those obtained in gait-related studies using scalp EEG. Although the same group (Snyder et al., 2015) showed that the vast majority (99%) of the IC were located outside the brain and lacked dipolar characteristic (applying ICA on these pure artifact data), the authors concluded that “Caution should be exercised when interpreting ICA for data that includes semi-periodic artifacts including artifact arising from human walking.” In this context, a hardware system incorporated in each electrode should be developed in order to directly measure the actual movement artifact and reject (subtract) it from the real EEG signal. Another already developed option uses a miniaturized wireless EEG system composed of a ring of 8 electrodes embedded in flexprint biocompatible polyamide placed around the ears (Debener et al., 2015). This system seems to avoid electrode displacement, movement signal contamination, non-stationarities and impedance changes.
Some components of movement artifacts can also be relevant to fine analysis the brain control of movement. For example, blink (Wascher et al., 2014), eye movements including saccades (Cordones et al., 2013) and microsaccades (Yuval-Greenberg et al., 2008), or muscle twitches (Cohen and van Gaal, 2014) can be related to specific brain activities and inform about the current brain state. Rather than just ruling out the artifactual interference of these features on the EEG signals, they should be selectively extracted and explored with respect to preceding and subsequent EEG content.
In an optimistic view, we may therefore anticipate that the general problem of artifact produced by sport movements in the EEG signal will find reasonable solution in a very short time. This will greatly facilitate the generalization of integrated mobile platforms in sport domain as the one proposed by MoBI (mobile brain/body imaging) project for natural cognition (Gramann et al., 2014a,b). MoBI can synchronize multiple physiological parameters (motor, ocular, and autonomic) and sensori-physical environment. In this context, a new open source tool-box called MoBILAB (Ojeda et al., 2014) working with EEGLAB greatly help with the management and the treatment of the multiple streams of data.
Confronted to the large variety of intervening parameters in sport performance we propose a general working methodology which could be applied whatever the highly specialized sport disciplines. This future approach should be made within a technological platform assuming the perfect synchronization between (1) biomechanical recordings (3D movement, force), (2) multiple EMG, (3) high density wireless EEG, (4) eye movement, and (5) signals coming from the autonomous nervous system. Based on the initial feedback of the trainer's team, the targeted movement priorities will be defined and recorded in a first explorative way. The best performance of the best performers will be then compared to less good performance. Sensori-motor strategies based on the dynamic relationship between the multiple EMG profiles and the related kinematics or kinetics will be accurately identified and quantified with the help of DRNN technology (Cheron et al., 2012; Bengoetxea et al., 2014), and the EEG biomarkers of the related performance will be defined and quantified by mean of ERP analysis linked to external or internal movement events and ongoing high density EEG signals allowing further mathematical analysis such as time frequency (ERSP and ITC) (EEGLab procedures, (Delorme and Makeig, 2004; Delorme et al., 2015)) microstates analysis (CARTOOL) (Brunet et al., 2011), coherency/directionality (Nolte et al., 2004; Cheron et al., 2014) and inverse modeling of the ERP, ERS, ERD, and ITC neural generators (swLORETA) (Cebolla et al., 2011, 2014).
Author Contributions
Substantial contributions to the conception or design of the work (GC, GP, JC, AL, AC, CC, MP, TH, AC, and BD). Drafting the work or revising it critically for important intellectual content (GC, GP, GP, and TH). Final approval of the version to be published (GC, GP, BD, and TH). Agreement to be accountable for all aspects of the work in ensuring that questions related to the accuracy or integrity of any part of the work are appropriately investigated and resolved (GC and CC).
Funding
We would like to thank T. D'Angelo, C. de Scoville, M. Dufief, E. Toussaint, E. Hortmanns, and M. Petieau, for expert technical assistance. This work was funded by the Belgian Federal Science Policy Office, the European Space Agency (AO-2004, 118), the Belgian National Fund for Scientific Research (FNRS), the research funds of the Université Libre de Bruxelles and of the Université de Mons (Belgium), the FEDER support (BIOFACT), the MINDWALKER project (“FP7” 2007-2013) supported by the European Commission, the Fonds G. Leibu and the NeuroAtt BIOWIN and Easy Move projects supported by Walloon Country of Belgium.
Conflict of Interest Statement
The authors declare that the research was conducted in the absence of any commercial or financial relationships that could be construed as a potential conflict of interest.
References
Abdollahipour, R., Wulf, G., Psotta, R., and Palomo Nieto, M. (2015). Performance of gymnastics skill benefits from an external focus of attention. J. Sports Sci. 33, 1807–1813. doi: 10.1080/02640414.2015.1012102
Ai, L., and Ro, T. (2014). The phase of prestimulus alpha oscillations affects tactile perception. J. Neurophysiol. 111, 1300–1307. doi: 10.1152/jn.00125.2013
Ali, A., Fawver, B., Kim, J., Fairbrother, J., and Janelle, C. M. (2012). Too much of a good thing: random practice scheduling and self-control of feedback lead to unique but not additive learning benefits. Front. Psychol. 3:503. doi: 10.3389/fpsyg.2012.00503
Alonso, A., Gaztelu, J. M., Buño, W., and García-Austt, E. (1987). Cross-correlation analysis of septohippocampal neurons during theta-rhythm. Brain Res. 413, 135–146.
Angulo-Sherman, I. N., and Gutiérrez, D. (2015). A link between the increase in electroencephalographic coherence and performance improvement in operating a brain-computer interface. Comput. Intell. Neurosci. 2015:824175. doi: 10.1155/2015/824175
Aoki, F., Fetz, E. E., Shupe, L., Lettich, E., and Ojemann, G. A. (1999). Increased gamma-range activity in human sensorimotor cortex during performance of visuomotor tasks. Clin. Neurophysiol. 110, 524–537. doi: 10.1016/S1388-2457(98)00064-9
Arjona, A., Escudero, M., and Gómez, C. M. (2014). Updating of attentional and premotor allocation resources as function of previous trial outcome. Sci. Rep. 4:4526. doi: 10.1038/srep04526
Arroyo, S., Lesser, R. P., Gordon, B., Uematsu, S., Jackson, D., and Webber, R. (1993). Functional significance of the mu rhythm of human cortex: an electrophysiologic study with subdural electrodes. Electroencephalogr. Clin. Neurophysiol. 87, 76–87. doi: 10.1016/0013-4694(93)90114-B
Astill, R. G., Piantoni, G., Raymann, R. J. E. M., Vis, J. C., Coppens, J. E., Walker, M. P., et al. (2014). Sleep spindle and slow wave frequency reflect motor skill performance in primary school-age children. Front. Hum. Neurosci. 8:910. doi: 10.3389/fnhum.2014.00910
Babiloni, C., Del Percio, C., Iacoboni, M., Infarinato, F., Lizio, R., Marzano, N., et al. (2008). Golf putt outcomes are predicted by sensorimotor cerebral EEG rhythms. J. Physiol. 586, 131–139. doi: 10.1113/jphysiol.2007.141630
Babiloni, C., Del Percio, C., Vecchio, F., Sebastiano, F., Di Gennaro, G., Quarato, P. P., et al. (2016). Alpha, beta and gamma electrocorticographic rhythms in somatosensory, motor, premotor and prefrontal cortical areas differ in movement execution and observation in humans. Clin. Neurophysiol. 127, 641–654. doi: 10.1016/j.clinph.2015.04.068
Babiloni, C., Marzano, N., Infarinato, F., Iacoboni, M., Rizza, G., Aschieri, P., et al. (2010). “Neural efficiency” of experts' brain during judgment of actions: a high-resolution EEG study in elite and amateur karate athletes. Behav. Brain Res. 207, 466–475. doi: 10.1016/j.bbr.2009.10.034
Badami, R., VaezMousavi, M., Wulf, G., and Namazizadeh, M. (2012). Feedback about more accurate versus less accurate trials: differential effects on self-confidence and activation. Res. Q. Exerc. Sport 83, 196–203. doi: 10.1080/02701367.2012.10599850
Bailey, S. P., Hall, E. E., Folger, S. E., and Miller, P. C. (2008). Changes in EEG during graded exercise on a recumbent cycle ergometer. J. Sports Sci. Med. 7, 505–511.
Barakat, M., Doyon, J., Debas, K., Vandewalle, G., Morin, A., Poirier, G., et al. (2011). Fast and slow spindle involvement in the consolidation of a new motor sequence. Behav. Brain Res. 217, 117–121. doi: 10.1016/j.bbr.2010.10.019
Başar, E., Başar-Eroğlu, C., Güntekin, B., and Yener, G. G. (2013). Brain's alpha, beta, gamma, delta, and theta oscillations in neuropsychiatric diseases: proposal for biomarker strategies. Suppl. Clin. Neurophysiol. 62, 19–54. doi: 10.1016/B978-0-7020-5307-8.00002-8
Baumeister, J., Reinecke, K., Liesen, H., and Weiss, M. (2008). Cortical activity of skilled performance in a complex sports related motor task. Eur. J. Appl. Physiol. 104, 625–631. doi: 10.1007/s00421-008-0811-x
Bellesi, M., Riedner, B. A., Garcia-Molina, G. N., Cirelli, C., and Tononi, G. (2014). Enhancement of sleep slow waves: underlying mechanisms and practical consequences. Front. Syst. Neurosci. 8:208. doi: 10.3389/fnsys.2014.00208
Bengoetxea, A., Leurs, F., Hoellinger, T., Cebolla, A. M., Dan, B., McIntyre, J., et al. (2014). Physiological modules for generating discrete and rhythmic movements: action identification by a dynamic recurrent neural network. Front. Comput. Neurosci. 8:100. doi: 10.3389/fncom.2014.00100
Berchicci, M., Pontifex, M. B., Drollette, E. S., Pesce, C., Hillman, C. H., and Di Russo, F. (2015). From cognitive motor preparation to visual processing: the benefits of childhood fitness to brain health. Neuroscience 298, 211–219. doi: 10.1016/j.neuroscience.2015.04.028
Berger, H. (1929). Über das Elektrenkephalogramm des Menschen. Arch. Psychiatr. Nervenkr. 87, 527–570. doi: 10.1007/BF01797193
Bergmann, T. O., Mölle, M., Marshall, L., Kaya-Yildiz, L., Born, J., and Roman Siebner, H. (2008). A local signature of LTP- and LTD-like plasticity in human NREM sleep. Eur. J. Neurosci. 27, 2241–2249. doi: 10.1111/j.1460-9568.2008.06178.x
Beyer, L., Weiss, T., Hansen, E., Wolf, A., and Seidel, A. (1990). Dynamics of central nervous activation during motor imagination. Int. J. Psychophysiol. 9, 75–80. doi: 10.1016/0167-8760(90)90008-2
Bragin, A., Jandó, G., Nádasdy, Z., Hetke, J., Wise, K., and Buzsáki, G. (1995). Gamma (40–100 Hz) oscillation in the hippocampus of the behaving rat. J. Neurosci. 15, 47–60.
Bremer, F., Stoupel, N., and van Reeth, P. C. (1960). Nouvelles recherches sur la facilitation et l'inhibition des potentiels évoqués corticaux dans l'éveil réticulaire. Arch. Ital. Biol. 98, 229–247.
Brockett, A. T., LaMarca, E. A., and Gould, E. (2015). Physical exercise enhances cognitive flexibility as well as astrocytic and synaptic markers in the medial prefrontal cortex. PLoS ONE 10:e0124859. doi: 10.1371/journal.pone.0124859
Bruijn, S. M., van Dieën, J. H., and Daffertshofer, A. (2015). Beta activity in the premotor cortex is increased during stabilized as compared to normal walking. Front. Hum. Neurosci. 9:593. doi: 10.3389/fnhum.2015.00593
Brümmer, V., Schneider, S., Strüder, H. K., and Askew, C. D. (2011). Primary motor cortex activity is elevated with incremental exercise intensity. Neuroscience 181, 150–162. doi: 10.1016/j.neuroscience.2011.02.006
Brunet, D., Murray, M. M., and Michel, C. M. (2011). Spatiotemporal analysis of multichannel EEG: CARTOOL. Comput. Intell. Neurosci. 2011, 813870. doi: 10.1155/2011/813870
Bullock, T., Cecotti, H., and Giesbrecht, B. (2015). Multiple stages of information processing are modulated during acute bouts of exercise. Neuroscience 307, 138–150. doi: 10.1016/j.neuroscience.2015.08.046
Bullock, T., and Giesbrecht, B. (2014). Acute exercise and aerobic fitness influence selective attention during visual search. Front. Psychol. 5:1290. doi: 10.3389/fpsyg.2014.01290
Buzsáki, G. (2002). Theta oscillations in the hippocampus. Neuron 33, 325–340. doi: 10.1016/S0896-6273(02)00586-X
Buzsáki, G. (2015). Hippocampal sharp wave-ripple: a cognitive biomarker for episodic memory and planning. Hippocampus 25, 1073–1188. doi: 10.1002/hipo.22488
Buzsáki, G., and Chrobak, J. J. (2005). Synaptic plasticity and self-organization in the hippocampus. Nat. Neurosci. 8, 1418–1420. doi: 10.1038/nn1105-1418
Buzsáki, G., Horváth, Z., Urioste, R., Hetke, J., and Wise, K. (1992). High-frequency network oscillation in the hippocampus. Science 256, 1025–1027. doi: 10.1126/science.1589772
Buzsáki, G., and Moser, E. I. (2013). Memory, navigation and theta rhythm in the hippocampal-entorhinal system. Nat. Neurosci. 16, 130–138. doi: 10.1038/nn.3304
Buzsáki, G., and Watson, B. O. (2012). Brain rhythms and neural syntax: implications for efficient coding of cognitive content and neuropsychiatric disease. Dialogues Clin. Neurosci. 14, 345–367.
Caplan, J. B., Madsen, J. R., Schulze-Bonhage, A., Aschenbrenner-Scheibe, R., Newman, E. L., and Kahana, M. J. (2003). Human theta oscillations related to sensorimotor integration and spatial learning. J. Neurosci. 23, 4726–4736.
Castermans, T., Duvinage, M., Cheron, G., and Dutoit, T. (2014). About the cortical origin of the low-delta and high-gamma rhythms observed in EEG signals during treadmill walking. Neurosci. Lett. 561, 166–170. doi: 10.1016/j.neulet.2013.12.059
Cebolla, A. M., and Cheron, G. (2015). Sensorimotor and cognitive involvement of the beta-gamma oscillation in the frontal N30 component of somatosensory evoked potentials. Neuropsychologia 79, 215–222. doi: 10.1016/j.neuropsychologia.2015.04.033
Cebolla, A. M., Palmero-Soler, E., Dan, B., and Cheron, G. (2011). Frontal phasic and oscillatory generators of the N30 somatosensory evoked potential. NeuroImage 54, 1297–1306. doi: 10.1016/j.neuroimage.2010.08.060
Cebolla, A. M., Palmero-Soler, E., Dan, B., and Cheron, G. (2014). Modulation of the N30 generators of the somatosensory evoked potentials by the mirror neuron system. NeuroImage 95, 48–60. doi: 10.1016/j.neuroimage.2014.03.039
Cebolla, A. M., Petieau, M., Cevallos, C., Leroy, A., Dan, B., and Cheron, G. (2015). Long-lasting cortical reorganization as the result of motor imagery of throwing a ball in a virtual tennis court. Front. Psychol. 6:1869. doi: 10.3389/fpsyg.2015.01869
Cevallos, C., Zarka, D., Hoellinger, T., Leroy, A., Dan, B., and Cheron, G. (2015). Oscillations in the human brain during walking execution, imagination and observation. Neuropsychologia. doi: 10.1016/j.neuropsychologia.2015.06.039
Chang, Y.-K., Huang, C.-J., Chen, K.-F., and Hung, T.-M. (2013). Physical activity and working memory in healthy older adults: an ERP study. Psychophysiology 50, 1174–1182. doi: 10.1111/psyp.12089
Chang, Y.-K., Pesce, C., Chiang, Y.-T., Kuo, C.-Y., and Fong, D.-Y. (2015). Antecedent acute cycling exercise affects attention control: an ERP study using attention network test. Front. Hum. Neurosci. 9:156. doi: 10.3389/fnhum.2015.00156
Chang, Y.-K., Tsai, Y.-J., Chen, T.-T., and Hung, T.-M. (2012). The impacts of coordinative exercise on executive function in kindergarten children: an ERP study. Exp. Brain Res. 225, 187–196. doi: 10.1007/s00221-012-3360-9
Cheron, G., Cebolla, A. M., Petieau, M., Bengoetxea, A., Palmero-Soler, E., Leroy, A., et al. (2009). Adaptive changes of rhythmic EEG oscillations in space implications for brain-machine interface applications. Int. Rev. Neurobiol. 86, 171–187. doi: 10.1016/S0074-7742(09)86013-3
Cheron, G., Duvinage, M., De Saedeleer, C., Castermans, T., Bengoetxea, A., Petieau, M., et al. (2012). From spinal central pattern generators to cortical network: integrated BCI for walking rehabilitation. Neural Plast. 2012, 375148. doi: 10.1155/2012/375148
Cheron, G., Leroy, A., De Saedeleer, C., Bengoetxea, A., Lipshits, M., Cebolla, A., et al. (2006). Effect of gravity on human spontaneous 10-Hz electroencephalographic oscillations during the arrest reaction. Brain Res. 1121, 104–116. doi: 10.1016/j.brainres.2006.08.098
Cheron, G., Leroy, A., Palmero-Soler, E., De Saedeleer, C., Bengoetxea, A., Cebolla, A.-M., et al. (2014). Gravity influences top-down signals in visual processing. PLoS ONE 9:e82371. doi: 10.1371/journal.pone.0082371
Cheron, G., Márquez-Ruiz, J., and Dan, B. (2015). Oscillations, timing, plasticity, and learning in the cerebellum. Cerebellum Lond. Engl. doi: 10.1007/s12311-015-0665-9. [Epub ahead of print].
Cheron, G., Saussez, S., Gerrits, N., and Godaux, E. (1995). Existence in the nucleus incertus of the cat of horizontal-eye-movement-related neurons projecting to the cerebellar flocculus. J. Neurophysiol. 74, 1367–1372.
Chiviacowsky, S., Wulf, G., and Lewthwaite, R. (2012). Self-controlled learning: the importance of protecting perceptions of competence. Front. Psychol. 3:458. doi: 10.3389/fpsyg.2012.00458
Chrobak, J. J., and Buzsáki, G. (1994). Selective activation of deep layer (V-VI) retrohippocampal cortical neurons during hippocampal sharp waves in the behaving rat. J. Neurosci. 14, 6160–6170.
Chu, C.-H., Alderman, B. L., Wei, G.-X., and Chang, Y.-K. (2015). Effects of acute aerobic exercise on motor response inhibition: an ERP study using the stop-signal task. J. Sport Health Sci. 4, 73–81. doi: 10.1016/j.jshs.2014.12.002
Chuang, L.-Y., Tsai, Y.-J., Chang, Y.-K., Huang, C.-J., and Hung, T.-M. (2015). Effects of acute aerobic exercise on response preparation in a Go/No Go Task in children with ADHD: an ERP study. J. Sport Health Sci. 4, 82–88. doi: 10.1016/j.jshs.2014.11.002
Cirelli, L. K., Bosnyak, D., Manning, F. C., Spinelli, C., Marie, C., Fujioka, T., et al. (2014). Beat-induced fluctuations in auditory cortical beta-band activity: using EEG to measure age-related changes. Audit. Cogn. Neurosci. 5:742. doi: 10.3389/fpsyg.2014.00742
Cohen, M. X., and van Gaal, S. (2014). Subthreshold muscle twitches dissociate oscillatory neural signatures of conflicts from errors. NeuroImage 86, 503–513. doi: 10.1016/j.neuroimage.2013.10.033
Cordones, I., Gómez, C. M., and Escudero, M. (2013). Cortical dynamics during the preparation of antisaccadic and prosaccadic eye movements in humans in a gap paradigm. PLoS ONE 8:e63751. doi: 10.1371/journal.pone.0063751
Courtemanche, R., and Lamarre, Y. (2005). Local field potential oscillations in primate cerebellar cortex: synchronization with cerebral cortex during active and passive expectancy. J. Neurophysiol. 93, 2039–2052. doi: 10.1152/jn.00080.2004
Courtemanche, R., Pellerin, J.-P., and Lamarre, Y. (2002). Local field potential oscillations in primate cerebellar cortex: modulation during active and passive expectancy. J. Neurophysiol. 88, 771–782.
Crews, D. J., and Landers, D. M. (1993). Electroencephalographic measures of attentional patterns prior to the golf putt. Med. Sci. Sports Exerc. 25, 116–126. doi: 10.1249/00005768-199301000-00016
Crick, F. (1984). Function of the thalamic reticular complex: the searchlight hypothesis. Proc. Natl. Acad. Sci. U.S.A. 81, 4586–4590. doi: 10.1073/pnas.81.14.4586
D'Angelo, E., and De Zeeuw, C. I. (2009). Timing and plasticity in the cerebellum: focus on the granular layer. Trends Neurosci. 32, 30–40. doi: 10.1016/j.tins.2008.09.007
Dai, C.-T., Chang, Y.-K., Huang, C.-J., and Hung, T.-M. (2013). Exercise mode and executive function in older adults: an ERP study of task-switching. Brain Cogn. 83, 153–162. doi: 10.1016/j.bandc.2013.07.007
Debener, S., Emkes, R., De Vos, M., and Bleichner, M. (2015). Unobtrusive ambulatory EEG using a smartphone and flexible printed electrodes around the ear. Sci. Rep. 5:16743. doi: 10.1038/srep16743
De Gennaro, L., Fratello, F., Marzano, C., Moroni, F., Curcio, G., Tempesta, D., et al. (2008). Cortical plasticity induced by transcranial magnetic stimulation during wakefulness affects electroencephalogram activity during sleep. PLoS ONE 3:e2483. doi: 10.1371/journal.pone.0002483
Dekker, M. K. J., van den Berg, B. R., Denissen, A. J. M., Sitskoorn, M. M., and van Boxtel, G. J. M. (2014). Feasibility of eyes open alpha power training for mental enhancement in elite gymnasts. J. Sports Sci. 32, 1550–1560. doi: 10.1080/02640414.2014.906044
de Lange, F. P., Jensen, O., Bauer, M., and Toni, I. (2008). Interactions between posterior gamma and frontal alpha/beta oscillations during imagined actions. Front. Hum. Neurosci. 2:7. doi: 10.3389/neuro.09.007.2008
Delorme, A., and Makeig, S. (2004). EEGLAB: an open source toolbox for analysis of single-trial EEG dynamics including independent component analysis. J. Neurosci. Methods 134, 9–21. doi: 10.1016/j.jneumeth.2003.10.009
Delorme, A., Miyakoshi, M., Jung, T.-P., and Makeig, S. (2015). Grand average ERP-image plotting and statistics: a method for comparing variability in event-related single-trial EEG activities across subjects and conditions. J. Neurosci. Methods 250, 3–6. doi: 10.1016/j.jneumeth.2014.10.003
Del Percio, C., Babiloni, C., Bertollo, M., Marzano, N., Iacoboni, M., Infarinato, F., et al. (2009a). Visuo-attentional and sensorimotor alpha rhythms are related to visuo-motor performance in athletes. Hum. Brain Mapp. 30, 3527–3540. doi: 10.1002/hbm.20776
Del Percio, C., Babiloni, C., Marzano, N., Iacoboni, M., Infarinato, F., Vecchio, F., et al. (2009b). “Neural efficiency” of athletes' brain for upright standing: a high-resolution EEG study. Brain Res. Bull. 79, 193–200. doi: 10.1016/j.brainresbull.2009.02.001
Del Percio, C., Brancucci, A., Bergami, F., Marzano, N., Fiore, A., Di Ciolo, E., et al. (2007). Cortical alpha rhythms are correlated with body sway during quiet open-eyes standing in athletes: a high-resolution EEG study. NeuroImage 36, 822–829. doi: 10.1016/j.neuroimage.2007.02.054
Del Percio, C., Infarinato, F., Iacoboni, M., Marzano, N., Soricelli, A., Aschieri, P., et al. (2010). Movement-related desynchronization of alpha rhythms is lower in athletes than non-athletes: a high-resolution EEG study. Clin. Neurophysiol. 121, 482–491. doi: 10.1016/j.clinph.2009.12.004
Del Percio, C., Infarinato, F., Marzano, N., Iacoboni, M., Aschieri, P., Lizio, R., et al. (2011). Reactivity of alpha rhythms to eyes opening is lower in athletes than non-athletes: a high-resolution EEG study. Int. J. Psychophysiol. 82, 240–247. doi: 10.1016/j.ijpsycho.2011.09.005
Dickter, C. L., and Kieffaber, P. D. (2014). EEG Methods for the Psychological Sciences, 1st Edn. Los Angeles, CA: SAGE Publications Ltd.
Dipoppa, M., and Gutkin, B. S. (2013). Flexible frequency control of cortical oscillations enables computations required for working memory. Proc. Natl. Acad. Sci. U.S.A. 110, 12828–12833. doi: 10.1073/pnas.1303270110
Donoghue, J. P., Sanes, J. N., Hatsopoulos, N. G., and Gaál, G. (1998). Neural discharge and local field potential oscillations in primate motor cortex during voluntary movements. J. Neurophysiol. 79, 159–173.
Doppelmayr, M., Finkenzeller, T., and Sauseng, P. (2008). Frontal midline theta in the pre-shot phase of rifle shooting: differences between experts and novices. Neuropsychologia 46, 1463–1467. doi: 10.1016/j.neuropsychologia.2007.12.026
Doppelmayr, M., Stabauer, S., Weber, E., Finkenzeller, T., and Amesberger, G. (2012). “Effects of exercise dependency and sensation seeking on risk behavior in ski mountaineers,” in Science and Skiing V, Vol. 5, eds E. Müller, S. Lindinger, and T. Stöggl (Maidenhead: Meyer & Meyer Sport), 127–133.
Drollette, E. S., Scudder, M. R., Raine, L. B., Moore, R. D., Saliba, B. J., Pontifex, M. B., et al. (2014). Acute exercise facilitates brain function and cognition in children who need it most: an ERP study of individual differences in inhibitory control capacity. Dev. Cogn. Neurosci. 7, 53–64. doi: 10.1016/j.dcn.2013.11.001
Dunst, B., Benedek, M., Jauk, E., Bergner, S., Koschutnig, K., Sommer, M., et al. (2014). Neural efficiency as a function of task demands. Intelligence 42, 22–30. doi: 10.1016/j.intell.2013.09.005
Dustman, R. E., Emmerson, R. Y., Ruhling, R. O., Shearer, D. E., Steinhaus, L. A., Johnson, S. C., et al. (1990). Age and fitness effects on EEG, ERPs, visual sensitivity, and cognition. Neurobiol. Aging 11, 193–200. doi: 10.1016/0197-4580(90)90545-B
Duvinage, M., Castermans, T., Petieau, M., Seetharaman, K., Hoellinger, T., Cheron, G., et al. (2012). A subjective assessment of a P300 BCI system for lower-limb rehabilitation purposes. Conf. Proc. Annu. Int. Conf. IEEE Eng. Med. Biol. Soc. 2012, 3845–3849. doi: 10.1109/embc.2012.6346806
Duzova, H., Özisik, H. I., Polat, A., Emre, M. H., and Gullu, E. (2005). Correlations between event-related potential components and nitric oxide in maximal anaerobic exercise among sportsmen trained at various levels. Int. J. Neurosci. 115, 1353–1373. doi: 10.1080/00207450590956387
Eckhorn, R., Bauer, R., Jordan, W., Brosch, M., Kruse, W., Munk, M., et al. (1988). Coherent oscillations: a mechanism of feature linking in the visual cortex? Multiple electrode and correlation analyses in the cat. Biol. Cybern. 60, 121–130. doi: 10.1007/BF00202899
Engel, A. K., Kreiter, A. K., König, P., and Singer, W. (1991). Synchronization of oscillatory neuronal responses between striate and extrastriate visual cortical areas of the cat. Proc. Natl. Acad. Sci. U.S.A. 88, 6048–6052. doi: 10.1073/pnas.88.14.6048
Engel, A. K., and Singer, W. (2001). Temporal binding and the neural correlates of sensory awareness. Trends Cogn. Sci. 5, 16–25. doi: 10.1016/S1364-6613(00)01568-0
Ermutlu, N., Yücesir, I., Eskikurt, G., Temel, T., and Işoğlu-Alkaç, Ü. (2015). Brain electrical activities of dancers and fast ball sports athletes are different. Cogn. Neurodyn. 9, 257–263. doi: 10.1007/s11571-014-9320-2
Facchini, S., Muellbacher, W., Battaglia, F., Boroojerdi, B., and Hallett, M. (2002). Focal enhancement of motor cortex excitability during motor imagery: a transcranial magnetic stimulation study. Acta Neurol. Scand. 105, 146–151. doi: 10.1034/j.1600-0404.2002.1o004.x
Fong, D.-Y., Chi, L.-K., Li, F., and Chang, Y.-K. (2014). The benefits of endurance exercise and Tai Chi Chuan for the task-switching aspect of executive function in older adults: an ERP study. Front. Aging Neurosci. 6:295. doi: 10.3389/fnagi.2014.00295
Freeman, W. J. (1978). Spatial properties of an EEG event in the olfactory bulb and cortex. Electroencephalogr. Clin. Neurophysiol. 44, 586–605. doi: 10.1016/0013-4694(78)90126-8
Fries, P., Reynolds, J. H., Rorie, A. E., and Desimone, R. (2001). Modulation of oscillatory neuronal synchronization by selective visual attention. Science 291, 1560–1563. doi: 10.1126/science.1055465
Ftaiti, F., Kacem, A., Jaidane, N., Tabka, Z., and Dogui, M. (2010). Changes in EEG activity before and after exhaustive exercise in sedentary women in neutral and hot environments. Appl. Ergon. 41, 806–811. doi: 10.1016/j.apergo.2010.01.008
Fullagar, H. H. K., Skorski, S., Duffield, R., Hammes, D., Coutts, A. J., and Meyer, T. (2015). Sleep and athletic performance: the effects of sleep loss on exercise performance, and physiological and cognitive responses to exercise. Sports Med. 45, 161–186. doi: 10.1007/s40279-014-0260-0
Fumoto, M., Oshima, T., Kamiya, K., Kikuchi, H., Seki, Y., Nakatani, Y., et al. (2010). Ventral prefrontal cortex and serotonergic system activation during pedaling exercise induces negative mood improvement and increased alpha band in EEG. Behav. Brain Res. 213, 1–9. doi: 10.1016/j.bbr.2010.04.017
Fyhn, M., Hafting, T., Witter, M. P., Moser, E. I., and Moser, M.-B. (2008). Grid cells in mice. Hippocampus 18, 1230–1238. doi: 10.1002/hipo.20472
Gajewski, P. D., and Falkenstein, M. (2015). Long-term habitual physical activity is associated with lower distractibility in a Stroop interference task in aging: behavioral and ERP evidence. Brain Cogn. 98, 87–101. doi: 10.1016/j.bandc.2015.06.004
García-Cossio, E., Severens, M., Nienhuis, B., Duysens, J., Desain, P., Keijsers, N., et al. (2015). Decoding sensorimotor rhythms during robotic-assisted treadmill walking for brain computer interface (BCI) applications. PLoS ONE 10:e0137910. doi: 10.1371/journal.pone.0137910
Gastaut, H. (1952). Etude électrocorticographique de la réactivité des rythmes rolandiques. Rev. Neurol. 87, 176–182.
Gastaut, H. J., and Bert, J. (1954). EEG changes during cinematographic presentation; moving picture activation of the EEG. Electroencephalogr. Clin. Neurophysiol. 6, 433–444. doi: 10.1016/0013-4694(54)90058-9
Gentet, L. J., Avermann, M., Matyas, F., Staiger, J. F., and Petersen, C. C. H. (2010). Membrane potential dynamics of GABAergic neurons in the barrel cortex of behaving mice. Neuron 65, 422–435. doi: 10.1016/j.neuron.2010.01.006
Getzmann, S., Falkenstein, M., and Gajewski, P. D. (2013). Long-term cardiovascular fitness is associated with auditory attentional control in old adults: neuro-behavioral evidence. PLoS ONE 8:e74539. doi: 10.1371/journal.pone.0074539
Giocomo, L. M., Stensola, T., Bonnevie, T., van Cauter, T., Moser, M.-B., and Moser, E. I. (2014). Topography of head direction cells in medial entorhinal cortex. Curr. Biol. 24, 252–262. doi: 10.1016/j.cub.2013.12.002
Goldberg, I., Ullman, S., and Malach, R. (2008). Neuronal correlates of “free will” are associated with regional specialization in the human intrinsic/default network. Conscious. Cogn. 17, 587–601. doi: 10.1016/j.concog.2007.10.003
Goldstein-Piekarski, A. N., Greer, S. M., Saletin, J. M., and Walker, M. P. (2015). Sleep deprivation impairs the human central and peripheral nervous system discrimination of social threat. J. Neurosci. 35, 10135–10145. doi: 10.1523/JNEUROSCI.5254-14.2015
Grabner, R. H., Fink, A., Stipacek, A., Neuper, C., and Neubauer, A. C. (2004). Intelligence and working memory systems: evidence of neural efficiency in alpha band ERD. Brain Res. Cogn. Brain Res. 20, 212–225. doi: 10.1016/j.cogbrainres.2004.02.010
Gramann, K., Ferris, D. P., Gwin, J., and Makeig, S. (2014a). Imaging natural cognition in action. Int. J. Psychophysiol. 91, 22–29. doi: 10.1016/j.ijpsycho.2013.09.003
Gramann, K., Jung, T.-P., Ferris, D. P., Lin, C.-T., and Makeig, S. (2014b). Toward a new cognitive neuroscience: modeling natural brain dynamics. Front. Hum. Neurosci. 8:444. doi: 10.3389/fnhum.2014.00444
Gray, C. M., König, P., Engel, A. K., and Singer, W. (1989). Oscillatory responses in cat visual cortex exhibit inter-columnar synchronization which reflects global stimulus properties. Nature 338, 334–337. doi: 10.1038/338334a0
Gray, C. M., and Singer, W. (1989). Stimulus-specific neuronal oscillations in orientation columns of cat visual cortex. Proc. Natl. Acad. Sci. U.S.A. 86, 1698–1702. doi: 10.1073/pnas.86.5.1698
Grego, F., Vallier, J.-M., Collardeau, M., Bermon, S., Ferrari, P., Candito, M., et al. (2004). Effects of long duration exercise on cognitive function, blood glucose, and counterregulatory hormones in male cyclists. Neurosci. Lett. 364, 76–80. doi: 10.1016/j.neulet.2004.03.085
Gruber, T., Keil, A., and Müller, M. M. (2001). Modulation of induced gamma band responses and phase synchrony in a paired associate learning task in the human EEG. Neurosci. Lett. 316, 29–32. doi: 10.1016/S0304-3940(01)02361-8
Gruber, T., and Müller, M. M. (2006). Oscillatory brain activity in the human EEG during indirect and direct memory tasks. Brain Res. 1097, 194–204. doi: 10.1016/j.brainres.2006.04.069
Gruber, T., Müller, M. M., and Keil, A. (2002). Modulation of induced gamma band responses in a perceptual learning task in the human EEG. J. Cogn. Neurosci. 14, 732–744. doi: 10.1162/08989290260138636
Gruber, T., Müller, M. M., Keil, A., and Elbert, T. (1999). Selective visual-spatial attention alters induced gamma band responses in the human EEG. Clin. Neurophysiol. 110, 2074–2085. doi: 10.1016/S1388-2457(99)00176-5
Gruber, W. R., Zauner, A., Lechinger, J., Schabus, M., Kutil, R., and Klimesch, W. (2014). Alpha phase, temporal attention, and the generation of early event related potentials. NeuroImage 103, 119–129. doi: 10.1016/j.neuroimage.2014.08.055
Gutmann, B., Mierau, A., Hülsdünker, T., Hildebrand, C., Przyklenk, A., Hollmann, W., et al. (2015). Effects of physical exercise on individual resting state eeg alpha peak frequency. Neural Plast. 2015:e717312. doi: 10.1155/2015/717312
Gwin, J. T., Gramann, K., Makeig, S., and Ferris, D. P. (2010). Removal of movement artifact from high-density EEG recorded during walking and running. J. Neurophysiol. 103, 3526–3534. doi: 10.1152/jn.00105.2010
Gwin, J. T., Gramann, K., Makeig, S., and Ferris, D. P. (2011). Electrocortical activity is coupled to gait cycle phase during treadmill walking. NeuroImage 54, 1289–1296. doi: 10.1016/j.neuroimage.2010.08.066
Haefeli, J., Vögeli, S., Michel, J., and Dietz, V. (2011). Preparation and performance of obstacle steps: interaction between brain and spinal neuronal activity. Eur. J. Neurosci. 33, 338–348. doi: 10.1111/j.1460-9568.2010.07494.x
Haegens, S., Osipova, D., Oostenveld, R., and Jensen, O. (2010). Somatosensory working memory performance in humans depends on both engagement and disengagement of regions in a distributed network. Hum. Brain Mapp. 31, 26–35. doi: 10.1002/hbm.20842
Hafting, T., Fyhn, M., Molden, S., Moser, M.-B., and Moser, E. I. (2005). Microstructure of a spatial map in the entorhinal cortex. Nature 436, 801–806. doi: 10.1038/nature03721
Hamon, J. F., and Seri, B. (1989). Reactivite corticale au cours d'Epreuves de temps de reaction chez les springers. Neurophysiol. Clin. Neurophysiol. 19, 109–122. doi: 10.1016/S0987-7053(89)80051-6
Händel, B., and Haarmeier, T. (2009). Cross-frequency coupling of brain oscillations indicates the success in visual motion discrimination. NeuroImage 45, 1040–1046. doi: 10.1016/j.neuroimage.2008.12.013
Händel, B., Thier, P., and Haarmeier, T. (2009). Visual motion perception deficits due to cerebellar lesions are paralleled by specific changes in cerebro-cortical activity. J. Neurosci. 29, 15126–15133. doi: 10.1523/JNEUROSCI.3972-09.2009
Hasselmo, M. E., and Stern, C. E. (2014). Theta rhythm and the encoding and retrieval of space and time. NeuroImage 85(Pt 2), 656–666. doi: 10.1016/j.neuroimage.2013.06.022
Hassler, U., Barreto, N. T., and Gruber, T. (2011). Induced gamma band responses in human EEG after the control of miniature saccadic artifacts. NeuroImage 57, 1411–1421. doi: 10.1016/j.neuroimage.2011.05.062
Hassler, U., Friese, U., Martens, U., Trujillo-Barreto, N., and Gruber, T. (2013). Repetition priming effects dissociate between miniature eye movements and induced gamma-band responses in the human electroencephalogram. Eur. J. Neurosci. 38, 2425–2433. doi: 10.1111/ejn.12244
Hayes, S. M., Hayes, J. P., Cadden, M., and Verfaellie, M. (2013). A review of cardiorespiratory fitness-related neuroplasticity in the aging brain. Front. Aging Neurosci. 5:31. doi: 10.3389/fnagi.2013.00031
Hillman, C. H., Apparies, R. J., Janelle, C. M., and Hatfield, B. D. (2000). An electrocortical comparison of executed and rejected shots in skilled marksmen. Biol. Psychol. 52, 71–83. doi: 10.1016/S0301-0511(99)00021-6
Hillman, C. H., Belopolsky, A. V., Snook, E. M., Kramer, A. F., and McAuley, E. (2004). Physical activity and executive control: implications for increased cognitive health during older adulthood. Res. Q. Exerc. Sport 75, 176–185. doi: 10.1080/02701367.2004.10609149
Hillman, C. H., Buck, S. M., Themanson, J. R., Pontifex, M. B., and Castelli, D. M. (2009a). Aerobic fitness and cognitive development: event-related brain potential and task performance indices of executive control in preadolescent children. Dev. Psychol. 45, 114–129. doi: 10.1037/a0014437
Hillman, C. H., Castelli, D. M., and Buck, S. M. (2005). Aerobic fitness and neurocognitive function in healthy preadolescent children. Med. Sci. Sports Exerc. 37, 1967–1974. doi: 10.1249/01.mss.0000176680.79702.ce
Hillman, C. H., Kamijo, K., and Pontifex, M. B. (2012). “The relation of ERP indices of exercise to brain health and cognition,” in Functional Neuroimaging in Exercise and Sport Sciences, eds H. Boecker, C. H. Hillman, L. Scheef, and H. K. Strüder (New York: Springer), 419–446. Available online at: http://link.springer.com/chapter/10.1007/978-1-4614-3293-7_18 (Accessed September 16, 2015).
Hillman, C. H., Kramer, A. F., Belopolsky, A. V., and Smith, D. P. (2006). A cross-sectional examination of age and physical activity on performance and event-related brain potentials in a task switching paradigm. Int. J. Psychophysiol. 59, 30–39. doi: 10.1016/j.ijpsycho.2005.04.009
Hillman, C. H., Pontifex, M. B., Castelli, D. M., Khan, N. A., Raine, L. B., Scudder, M. R., et al. (2014). Effects of the FITKids randomized controlled trial on executive control and brain function. Pediatrics 2013:3219. doi: 10.1542/peds.2013-3219
Hillman, C. H., Pontifex, M. B., Raine, L. B., Castelli, D. M., Hall, E. E., and Kramer, A. F. (2009b). The effect of acute treadmill walking on cognitive control and academic achievement in preadolescent children. Neuroscience 159, 1044–1054. doi: 10.1016/j.neuroscience.2009.01.057
Hillman, C. H., Snook, E. M., and Jerome, G. J. (2003). Acute cardiovascular exercise and executive control function. Int. J. Psychophysiol. 48, 307–314. doi: 10.1016/S0167-8760(03)00080-1
Hillman, C. H., Weiss, E. P., Hagberg, J. M., and Hatfield, B. D. (2002). The relationship of age and cardiovascular fitness to cognitive and motor processes. Psychophysiology 39, 303–312. doi: 10.1017/S0048577201393058
Hobson, J. A., Pace-Schott, E. F., and Stickgold, R. (2000). Dreaming and the brain: toward a cognitive neuroscience of conscious states. Behav. Brain Sci. 23, 793–842. discussion: 904–1121. doi: 10.1017/s0140525x00003976
Hoffmann, L. C., and Berry, S. D. (2009). Cerebellar theta oscillations are synchronized during hippocampal theta-contingent trace conditioning. Proc. Natl. Acad. Sci. U.S.A. 106, 21371–21376. doi: 10.1073/pnas.0908403106
Hosaka, R., Nakajima, T., Aihara, K., Yamaguchi, Y., and Mushiake, H. (2015). The suppression of beta oscillations in the primate supplementary motor complex reflects a volatile state during the updating of action sequences. Cereb. Cortex. doi: 10.1093/cercor/bhv163. [Epub ahead of print].
Huber, R., Ghilardi, M. F., Massimini, M., Ferrarelli, F., Riedner, B. A., Peterson, M. J., et al. (2006). Arm immobilization causes cortical plastic changes and locally decreases sleep slow wave activity. Nat. Neurosci. 9, 1169–1176. doi: 10.1038/nn1758
Huber, R., Ghilardi, M. F., Massimini, M., and Tononi, G. (2004). Local sleep and learning. Nature 430, 78–81. doi: 10.1038/nature02663
Hung, T.-M., Spalding, T. W., Maria, D. L. S., and Hatfield, B. D. (2004). Assessment of reactive motor performance with event-related brain potentials: attention processes in elite table tennis players. J. Sport Exerc. Psychol. 26, 317–337.
Ito, J., Roy, S., Liu, Y., Cao, Y., Fletcher, M., Lu, L., et al. (2014). Whisker barrel cortex delta oscillations and gamma power in the awake mouse are linked to respiration. Nat. Commun. 5, 3572. doi: 10.1038/ncomms4572
Jensen, O., Kaiser, J., and Lachaux, J.-P. (2007). Human gamma-frequency oscillations associated with attention and memory. Trends Neurosci. 30, 317–324. doi: 10.1016/j.tins.2007.05.001
Kacem, A., Ftaiti, F., Chamari, K., Dogui, M., Grélot, L., and Tabka, Z. (2014). EEG-related changes to fatigue during intense exercise in the heat in sedentary women. Health 6, 1277–1285. doi: 10.4236/health.2014.611156
Kahana, M. J., Sekuler, R., Caplan, J. B., Kirschen, M., and Madsen, J. R. (1999). Human theta oscillations exhibit task dependence during virtual maze navigation. Nature 399, 781–784. doi: 10.1038/21645
Kamijo, K., Hayashi, Y., Sakai, T., Yahiro, T., Tanaka, K., and Nishihira, Y. (2009). Acute effects of aerobic exercise on cognitive function in older adults. J. Gerontol. B. Psychol. Sci. Soc. Sci. 64B, 356–363. doi: 10.1093/geronb/gbp030
Kamijo, K., Nishihira, Y., Hatta, A., Kaneda, T., Wasaka, T., Kida, T., et al. (2004). Differential influences of exercise intensity on information processing in the central nervous system. Eur. J. Appl. Physiol. 92, 305–311. doi: 10.1007/s00421-004-1097-2
Kamijo, K., Nishihira, Y., Higashiura, T., and Kuroiwa, K. (2007). The interactive effect of exercise intensity and task difficulty on human cognitive processing. Int. J. Psychophysiol. 65, 114–121. doi: 10.1016/j.ijpsycho.2007.04.001
Kamijo, K., O'leary, K. C., Pontifex, M. B., Themanson, J. R., and Hillman, C. H. (2010). The relation of aerobic fitness to neuroelectric indices of cognitive and motor task preparation. Psychophysiology 47, 814–821. doi: 10.1111/j.1469-8986.2010.00992.x
Kamijo, K., Pontifex, M. B., O'Leary, K. C., Scudder, M. R., Wu, C.-T., Castelli, D. M., et al. (2011). The effects of an afterschool physical activity program on working memory in preadolescent children. Dev. Sci. 14, 1046–1058. doi: 10.1111/j.1467-7687.2011.01054.x
Kamijo, K., and Takeda, Y. (2009). General physical activity levels influence positive and negative priming effects in young adults. Clin. Neurophysiol. 120, 511–519. doi: 10.1016/j.clinph.2008.11.022
Kamijo, K., and Takeda, Y. (2010). Regular physical activity improves executive function during task switching in young adults. Int. J. Psychophysiol. 75, 304–311. doi: 10.1016/j.ijpsycho.2010.01.002
Kamijo, K., Takeda, Y., Takai, Y., and Haramura, M. (2015). Greater aerobic fitness is associated with more efficient inhibition of task-irrelevant information in preadolescent children. Biol. Psychol. 110, 68–74. doi: 10.1016/j.biopsycho.2015.07.007
Kaplan, R., Doeller, C. F., Barnes, G. R., Litvak, V., Düzel, E., Bandettini, P. A., et al. (2012). Movement-related theta rhythm in humans: coordinating self-directed hippocampal learning. PLoS Biol. 10:e1001267. doi: 10.1371/journal.pbio.1001267
Kasuga, S., Matsushika, Y., Kasashima-Shindo, Y., Kamatani, D., Fujiwara, T., Liu, M., et al. (2015). Transcranial direct current stimulation enhances mu rhythm desynchronization during motor imagery that depends on handedness. Laterality 20, 453–468. doi: 10.1080/1357650x.2014.998679
Keren, A. S., Yuval-Greenberg, S., and Deouell, L. Y. (2010). Saccadic spike potentials in gamma-band EEG: characterization, detection and suppression. NeuroImage 49, 2248–2263. doi: 10.1016/j.neuroimage.2009.10.057
Kim, S., Kim, S., Kim, M., Kim, M., and Nam, S. (2015). The effect of Tai Chi exercises on the cognitive function in older adults: an examination of P300. Indian J. Sci. Technol. 8, 452. doi: 10.17485/ijst/2015/v8iS7/71294
Klimesch, W. (1999). EEG alpha and theta oscillations reflect cognitive and memory performance: a review and analysis. Brain Res. Brain Res. Rev. 29, 169–195. doi: 10.1016/S0165-0173(98)00056-3
Klimesch, W., Doppelmayr, M., Röhm, D., Pöllhuber, D., and Stadler, W. (2000). Simultaneous desynchronization and synchronization of different alpha responses in the human electroencephalograph: a neglected paradox? Neurosci. Lett. 284, 97–100. doi: 10.1016/s0304-3940(00)00985-x
Klimesch, W., Sauseng, P., and Hanslmayr, S. (2007). EEG alpha oscillations: the inhibition-timing hypothesis. Brain Res. Rev. 53, 63–88. doi: 10.1016/j.brainresrev.2006.06.003
Kline, J. E., Huang, H. J., Snyder, K. L., and Ferris, D. P. (2015). Isolating gait-related movement artifacts in electroencephalography during human walking. J. Neural Eng. 12, 046022. doi: 10.1088/1741-2560/12/4/046022
Konttinen, N., Lyytinen, H., and Viitasalo, J. (1998). Preparatory heart rate patterns in competitive rifle shooting. J. Sports Sci. 16, 235–242. doi: 10.1080/026404198366759
Kreiter, A. K., and Singer, W. (1996). Stimulus-dependent synchronization of neuronal responses in the visual cortex of the awake macaque monkey. J. Neurosci. Off. J. Soc. Neurosci. 16, 2381–2396.
Kropff, E., Carmichael, J. E., Moser, M.-B., and Moser, E. I. (2015). Speed cells in the medial entorhinal cortex. Nature 523, 419–424. doi: 10.1038/nature14622
Kubitz, K. A., and Mott, A. A. (1996). EEG Power Spectral Densities during and after Cycle Ergometer Exercise. Res. Q. Exerc. Sport 67, 91–96. doi: 10.1080/02701367.1996.10607929
Kuo, C.-C., Luu, P., Morgan, K. K., Dow, M., Davey, C., Song, J., et al. (2014). Localizing movement-related primary sensorimotor cortices with multi-band EEG frequency changes and functional MRI. PLoS ONE 9:e112103. doi: 10.1371/journal.pone.0112103
Lachaux, J.-P., Chavez, M., and Lutz, A. (2003). A simple measure of correlation across time, frequency and space between continuous brain signals. J. Neurosci. Methods 123, 175–188. doi: 10.1016/S0165-0270(02)00358-8
Lakatos, P., Karmos, G., Mehta, A. D., Ulbert, I., and Schroeder, C. E. (2008). Entrainment of neuronal oscillations as a mechanism of attentional selection. Science 320, 110–113. doi: 10.1126/science.1154735
Lane, A. M., Beedie, C. J., Devonport, T. J., and Stanley, D. M. (2011). Instrumental emotion regulation in sport: relationships between beliefs about emotion and emotion regulation strategies used by athletes. Scand. J. Med. Sci. Sports 21, e445–451. doi: 10.1111/j.1600-0838.2011.01364.x
Lebedev, M. A., and Nelson, R. J. (1995). Rhythmically firing (20-50 Hz) neurons in monkey primary somatosensory cortex: activity patterns during initiation of vibratory-cued hand movements. J. Comput. Neurosci. 2, 313–334. doi: 10.1007/BF00961443
Lebedev, M. A., and Wise, S. P. (2000). Oscillations in the premotor cortex: single-unit activity from awake, behaving monkeys. Exp. Brain Res. 130, 195–215. doi: 10.1007/s002210050022
Lechinger, J., Heib, D. P. J., Gruber, W., Schabus, M., and Klimesch, W. (2015). Heartbeat-related EEG amplitude and phase modulations from wakefulness to deep sleep: interactions with sleep spindles and slow oscillations. Psychophysiology 52, 1441–1450. doi: 10.1111/psyp.12508
Lega, B., Burke, J., Jacobs, J., and Kahana, M. J. (2014). Slow-theta-to-gamma phase-amplitude coupling in human hippocampus supports the formation of new episodic memories. Cereb. Cortex 26, 268–278. doi: 10.1093/cercor/bhu232
Lega, B. C., Jacobs, J., and Kahana, M. (2012). Human hippocampal theta oscillations and the formation of episodic memories. Hippocampus 22, 748–761. doi: 10.1002/hipo.20937
Lehtelä, L., Salmelin, R., and Hari, R. (1997). Evidence for reactive magnetic 10-Hz rhythm in the human auditory cortex. Neurosci. Lett. 222, 111–114. doi: 10.1016/S0304-3940(97)13361-4
Lever, C., Burton, S., Jeewajee, A., O'Keefe, J., and Burgess, N. (2009). Boundary vector cells in the subiculum of the hippocampal formation. J. Neurosci. Off. J. Soc. Neurosci. 29, 9771–9777. doi: 10.1523/JNEUROSCI.1319-09.2009
Libenson, M. H. (2012). Practical Approach to Electroencephalography, 1st Edn. Philadelphia, PA: Saunders.
Lisi, G., and Morimoto, J. (2015). EEG single-trial detection of gait speed changes during treadmill walk. PLoS ONE 10:e0125479. doi: 10.1371/journal.pone.0125479
Llinás, R., and Ribary, U. (1993). Coherent 40-Hz oscillation characterizes dream state in humans. Proc. Natl. Acad. Sci. U.S.A. 90, 2078–2081. doi: 10.1073/pnas.90.5.2078
Loo, S. K., Lenartowicz, A., and Makeig, S. (2015). Research Review: use of EEG biomarkers in child psychiatry research—current state and future directions. J. Child Psychol. Psychiatry 57, 4–17. doi: 10.1111/jcpp.12435
Ludyga, S., Gronwald, T., and Hottenrott, K. (2015). Effects of high vs. low cadence training on cyclists' brain cortical activity during exercise. J. Sci. Med. Sport Sports. doi: 10.1016/j.jsams.2015.04.003. [Epub ahead of print].
Lutzenberger, W., Ripper, B., Busse, L., Birbaumer, N., and Kaiser, J. (2002). Dynamics of gamma-band activity during an audiospatial working memory task in humans. J. Neurosci. 22, 5630–5638.
Magnié, M.-N., Bermon, S., Martin, F., Madany-Lounis, M., Suisse, G., Muhammad, W., et al. (2000). P300, N400, aerobic fitness, and maximal aerobic exercise. Psychophysiology 37, 369–377. doi: 10.1111/1469-8986.3730369
Maquet, P., Péters, J., Aerts, J., Delfiore, G., Degueldre, C., Luxen, A., et al. (1996). Functional neuroanatomy of human rapid-eye-movement sleep and dreaming. Nature 383, 163–166. doi: 10.1038/383163a0
Marzano, C., Ferrara, M., Mauro, F., Moroni, F., Gorgoni, M., Tempesta, D., et al. (2011). Recalling and forgetting dreams: theta and alpha oscillations during sleep predict subsequent dream recall. J. Neurosci. 31, 6674–6683. doi: 10.1523/JNEUROSCI.0412-11.2011
Mechau, D., Mücke, S., Weiss, M., and Liesen, H. (1998). Effect of increasing running velocity on electroencephalogram in a field test. Eur. J. Appl. Physiol. 78, 340–345. doi: 10.1007/s004210050429
Mierau, A., Felsch, M., Hülsdünker, T., Mierau, J., Bullermann, P., Weiß, B., et al. (2016). The interrelation between sensorimotor abilities, cognitive performance and individual EEG alpha peak frequency in young children. Clin. Neurophysiol. 127, 270–276. doi: 10.1016/j.clinph.2015.03.008
Mierau, A., Schneider, S., Abel, T., Askew, C., Werner, S., and Strüder, H. K. (2009). Improved sensorimotor adaptation after exhaustive exercise is accompanied by altered brain activity. Physiol. Behav. 96, 115–121. doi: 10.1016/j.physbeh.2008.09.002
Miller, E. K., Li, L., and Desimone, R. (1991). A neural mechanism for working and recognition memory in inferior temporal cortex. Science 254, 1377–1379. doi: 10.1126/science.1962197
Montemurro, M. A., Rasch, M. J., Murayama, Y., Logothetis, N. K., and Panzeri, S. (2008). Phase-of-firing coding of natural visual stimuli in primary visual cortex. Curr. Biol. 18, 375–380. doi: 10.1016/j.cub.2008.02.023
Moraes, H., Ferreira, C., Deslandes, A., Cagy, M., Pompeu, F., Ribeiro, P., et al. (2007). Beta and alpha electroencephalographic activity changes after acute exercise. Arq. Neuropsiquiatr. 65, 637–641. doi: 10.1590/S0004-282X2007000400018
Moser, E. I., Kropff, E., and Moser, M.-B. (2008). Place cells, grid cells, and the brain's spatial representation system. Annu. Rev. Neurosci. 31, 69–89. doi: 10.1146/annurev.neuro.31.061307.090723
Murthy, V. N., and Fetz, E. E. (1992). Coherent 25- to 35-Hz oscillations in the sensorimotor cortex of awake behaving monkeys. Proc. Natl. Acad. Sci. U.S.A. 89, 5670–5674. doi: 10.1073/pnas.89.12.5670
Murthy, V. N., and Fetz, E. E. (1996). Oscillatory activity in sensorimotor cortex of awake monkeys: synchronization of local field potentials and relation to behavior. J. Neurophysiol. 76, 3949–3967.
Muthukumaraswamy, S. (2013). High-frequency brain activity and muscle artifacts in MEG/EEG: a review and recommendations. Front. Hum. Neurosci. 7:138. doi: 10.3389/fnhum.2013.00138
Nakamura, Y., Nishimoto, K., Akamatu, M., Takahashi, M., and Maruyama, A. (1999). The effect of jogging on P300 event related potentials. Electromyogr. Clin. Neurophysiol. 39, 71–74.
Nakatani, C., Raffone, A., and van Leeuwen, C. (2014). Efficiency of conscious access improves with coupling of slow and fast neural oscillations. J. Cogn. Neurosci. 26, 1168–1179. doi: 10.1162/jocn_a_00540
Niedermeyer, E., and da Silva, F. L. (1982). Electroencephalography: Basic Principles, Clinical Applications, and Related Fields. Baltimore, MD; Munich: Urban & Scwarzenberg.
Nielsen, B., Hyldig, T., Bidstrup, F., González-Alonso, J., and Christoffersen, G. R. J. (2001). Brain activity and fatigue during prolonged exercise in the heat. Pflüg. Arch. 442, 41–48. doi: 10.1007/s004240100515
Nishida, M., Pearsall, J., Buckner, R. L., and Walker, M. P. (2009). REM sleep, prefrontal theta, and the consolidation of human emotional memory. Cereb. Cortex 19, 1158–1166. doi: 10.1093/cercor/bhn155
Nitsche, M. A., and Paulus, W. (2001). Sustained excitability elevations induced by transcranial DC motor cortex stimulation in humans. Neurology 57, 1899–1901. doi: 10.1212/WNL.57.10.1899
Nolte, G., Bai, O., Wheaton, L., Mari, Z., Vorbach, S., and Hallett, M. (2004). Identifying true brain interaction from EEG data using the imaginary part of coherency. Clin. Neurophysiol. 115, 2292–2307. doi: 10.1016/j.clinph.2004.04.029
Nuñez, A., Cervera-Ferri, A., Olucha-Bordonau, F., Ruiz-Torner, A., and Teruel, V. (2006). Nucleus incertus contribution to hippocampal theta rhythm generation. Eur. J. Neurosci. 23, 2731–2738. doi: 10.1111/j.1460-9568.2006.04797.x
Nybo, L., and Nielsen, B. (2001). Hyperthermia and central fatigue during prolonged exercise in humans. J. Appl. Physiol. 91, 1055–1060.
O'Keefe, J. (1976). Place units in the hippocampus of the freely moving rat. Exp. Neurol. 51, 78–109.
O'Keefe, J., and Burgess, N. (2005). Dual phase and rate coding in hippocampal place cells: theoretical significance and relationship to entorhinal grid cells. Hippocampus 15, 853–866. doi: 10.1002/hipo.20115
Ofori, E., Coombes, S. A., and Vaillancourt, D. E. (2015). 3D Cortical electrophysiology of ballistic upper limb movement in humans. NeuroImage 115, 30–41. doi: 10.1016/j.neuroimage.2015.04.043
Ojeda, A., Bigdely-Shamlo, N., and Makeig, S. (2014). MoBILAB: an open source toolbox for analysis and visualization of mobile brain/body imaging data. Front. Hum. Neurosci. 8:121. doi: 10.3389/fnhum.2014.00121
Olbrich, S., and Arns, M. (2013). EEG biomarkers in major depressive disorder: discriminative power and prediction of treatment response. Int. Rev. Psychiatry 25, 604–618. doi: 10.3109/09540261.2013.816269
Palmero-Soler, E., Dolan, K., Hadamschek, V., and Tass, P. A. (2007). swLORETA: a novel approach to robust source localization and synchronization tomography. Phys. Med. Biol. 52, 1783–1800. doi: 10.1088/0031-9155/52/7/002
Park, J. L., Fairweather, M. M., and Donaldson, D. I. (2015). Making the case for mobile cognition: EEG and sports performance. Neurosci. Biobehav. Rev. 52, 117–130. doi: 10.1016/j.neubiorev.2015.02.014
Pastötter, B., and Bäuml, K.-H. T. (2014). Distinct slow and fast cortical theta dynamics in episodic memory retrieval. NeuroImage 94, 155–161. doi: 10.1016/j.neuroimage.2014.03.002
Pedersen, D. M. (2002). Intrinsic-extrinsic factors in sport motivation. Percept. Mot. Skills 95, 459–476. doi: 10.2466/PMS.95.6.459-476
Pesant, N., and Zadra, A. (2006). Dream content and psychological well-being: a longitudinal study of the continuity hypothesis. J. Clin. Psychol. 62, 111–121. doi: 10.1002/jclp.20212
Pfurtscheller, G., Krausz, G., and Neuper, C. (2001). Mechanical stimulation of the fingertip can induce bursts of beta oscillations in sensorimotor areas. J. Clin. Neurophysiol. 18, 559–564.
Pfurtscheller, G., and Neuper, C. (1994). Event-related synchronization of mu rhythm in the EEG over the cortical hand area in man. Neurosci. Lett. 174, 93–96. doi: 10.1016/0304-3940(94)90127-9
Pfurtscheller, G., Neuper, C., Andrew, C., and Edlinger, G. (1997). Foot and hand area mu rhythms. Int. J. Psychophysiol. 26, 121–135. doi: 10.1016/S0167-8760(97)00760-5
Pfurtscheller, G., Solis-Escalante, T., Barry, R. J., Klobassa, D. S., Neuper, C., and Müller-Putz, G. R. (2013). Brisk heart rate and EEG changes during execution and withholding of cue-paced foot motor imagery. Front. Hum. Neurosci. 7:379. doi: 10.3389/fnhum.2013.00379
Plattner, K., Lambert, M. I., Tam, N., Lamberts, R. P., and Baumeister, J. (2014). Changes in cortical beta activity related to a biceps brachii movement task while experiencing exercise induced muscle damage. Physiol. Behav. 123, 1–10. doi: 10.1016/j.physbeh.2013.08.022
Polich, J., and Lardon, M. T. (1997). P300 and long-term physical exercise. Electroencephalogr. Clin. Neurophysiol. 103, 493–498. doi: 10.1016/S0013-4694(97)96033-8
Pontifex, M. B., and Hillman, C. H. (2007). Neuroelectric and behavioral indices of interference control during acute cycling. Clin. Neurophysiol. 118, 570–580. doi: 10.1016/j.clinph.2006.09.029
Pontifex, M. B., Hillman, C. H., and Polich, J. (2009). Age, physical fitness, and attention: P3a and P3b. Psychophysiology 46, 379–387. doi: 10.1111/j.1469-8986.2008.00782.x
Pontifex, M. B., Parks, A. C., Henning, D. A., and Kamijo, K. (2015). Single bouts of exercise selectively sustain attentional processes. Psychophysiology 52, 618–625. doi: 10.1111/psyp.12395
Pontifex, M. B., Raine, L. B., Johnson, C. R., Chaddock, L., Voss, M. W., Cohen, N. J., et al. (2011). Cardiorespiratory fitness and the flexible modulation of cognitive control in preadolescent children. J. Cogn. Neurosci. 23, 1332–1345. doi: 10.1162/jocn.2010.21528
Pontifex, M. B., Saliba, B. J., Raine, L. B., Picchietti, D. L., and Hillman, C. H. (2013). Exercise improves behavioral, neurocognitive, and scholastic performance in children with attention-deficit/hyperactivity disorder. J. Pediatr. 162, 543–551. doi: 10.1016/j.jpeds.2012.08.036
Poulet, J. F. A., Fernandez, L. M. J., Crochet, S., and Petersen, C. C. H. (2012). Thalamic control of cortical states. Nat. Neurosci. 15, 370–372. doi: 10.1038/nn.3035
Poulet, J. F. A., and Petersen, C. C. H. (2008). Internal brain state regulates membrane potential synchrony in barrel cortex of behaving mice. Nature 454, 881–885. doi: 10.1038/nature07150
Radlo, S. J., Janelle, C. M., Barba, D. A., and Frehlich, S. G. (2001). Perceptual decision making for baseball pitch recognition: using P300 latency and amplitude to index attentional processing. Res. Q. Exerc. Sport 72, 22–31. doi: 10.1080/02701367.2001.10608928
Rasmussen, P., Stie, H., Nybo, L., and Nielsen, B. (2004). Heat induced fatigue and changes of the EEG is not related to reduced perfusion of the brain during prolonged exercise in humans. J. Therm. Biol. 29, 731–737. doi: 10.1016/j.jtherbio.2004.08.047
Rathschlag, M., and Memmert, D. (2013). The influence of self-generated emotions on physical performance: an investigation of happiness, anger, anxiety, and sadness. J. Sport Exerc. Psychol. 35, 197–210.
Ray, S., Naumann, R., Burgalossi, A., Tang, Q., Schmidt, H., and Brecht, M. (2014). Grid-layout and theta-modulation of layer 2 pyramidal neurons in medial entorhinal cortex. Science 343, 891–896. doi: 10.1126/science.1243028
Reimer, J., and Hatsopoulos, N. G. (2010). Periodicity and evoked responses in motor cortex. J. Neurosci. 30, 11506–11515. doi: 10.1523/JNEUROSCI.5947-09.2010
Reinecke, K., Cordes, M., Lerch, C., Koutsandréou, F., Schubert, M., Weiss, M., et al. (2011). From lab to field conditions: a pilot study on EEG methodology in applied sports sciences. Appl. Psychophysiol. Biofeedback 36, 265–271. doi: 10.1007/s10484-011-9166-x
Renfree, A., Martin, L., Micklewright, D., and St Clair Gibson, A. (2014). Application of decision-making theory to the regulation of muscular work rate during self-paced competitive endurance activity. Sports Med. 44, 147–158. doi: 10.1007/s40279-013-0107-0
Ribary, U., Ioannides, A. A., Singh, K. D., Hasson, R., Bolton, J. P., Lado, F., et al. (1991). Magnetic field tomography of coherent thalamocortical 40-Hz oscillations in humans. Proc. Natl. Acad. Sci. U.S.A. 88, 11037–11041. doi: 10.1073/pnas.88.24.11037
Riedner, B. A., Vyazovskiy, V. V., Huber, R., Massimini, M., Esser, S., Murphy, M., et al. (2007). Sleep homeostasis and cortical synchronization: III. A high-density EEG study of sleep slow waves in humans. Sleep 30, 1643–1657.
Robertson, C. V., and Marino, F. E. (2015). Prefrontal and motor cortex EEG responses and their relationship to ventilatory thresholds during exhaustive incremental exercise. Eur. J. Appl. Physiol. 115, 1939–1948. doi: 10.1007/s00421-015-3177-x
Rossi, S., Tecchio, F., Pasqualetti, P., Ulivelli, M., Pizzella, V., Romani, G. L., et al. (2002). Somatosensory processing during movement observation in humans. Clin. Neurophysiol. 113, 16–24. doi: 10.1016/S1388-2457(01)00725-8
Saalmann, Y. B., and Kastner, S. (2009). Gain control in the visual thalamus during perception and cognition. Curr. Opin. Neurobiol. 19, 408–414. doi: 10.1016/j.conb.2009.05.007
Saalmann, Y. B., and Kastner, S. (2011). Cognitive and perceptual functions of the visual thalamus. Neuron 71, 209–223. doi: 10.1016/j.neuron.2011.06.027
Sadaghiani, S., Scheeringa, R., Lehongre, K., Morillon, B., Giraud, A.-L., D'Esposito, M., et al. (2012). α-band phase synchrony is related to activity in the fronto-parietal adaptive control network. J. Neurosci. 32, 14305–14310. doi: 10.1523/JNEUROSCI.1358-12.2012
Salazar, W., Landers, D. M., Petruzzello, S. J., Han, M., Crews, D. J., and Kubitz, K. A. (1990). Hemispheric asymmetry, cardiac response, and performance in elite archers. Res. Q. Exerc. Sport 61, 351–359. doi: 10.1080/02701367.1990.10607499
Sammler, D., Grigutsch, M., Fritz, T., and Koelsch, S. (2007). Music and emotion: electrophysiological correlates of the processing of pleasant and unpleasant music. Psychophysiology 44, 293–304. doi: 10.1111/j.1469-8986.2007.00497.x
Scarpelli, S., D'Atri, A., Gorgoni, M., Ferrara, M., and Gennaro, L. D. (2015). EEG oscillations during sleep and dream recall: state- or trait-like individual differences? Front. Psychol. 6:605. doi: 10.3389/fpsyg.2015.00605
Schneider, S., Brümmer, V., Abel, T., Askew, C. D., and Strüder, H. K. (2009). Changes in brain cortical activity measured by EEG are related to individual exercise preferences. Physiol. Behav. 98, 447–452. doi: 10.1016/j.physbeh.2009.07.010
Schuster, C., Hilfiker, R., Amft, O., Scheidhauer, A., Andrews, B., Butler, J., et al. (2011). Best practice for motor imagery: a systematic literature review on motor imagery training elements in five different disciplines. BMC Med. 9:75. doi: 10.1186/1741-7015-9-75
Scisco, J. L., Leynes, P. A., and Kang, J. (2008). Cardiovascular fitness and executive control during task-switching: an ERP study. Int. J. Psychophysiol. 69, 52–60. doi: 10.1016/j.ijpsycho.2008.02.009
Scudder, M. R., Federmeier, K. D., Raine, L. B., Direito, A., Boyd, J. K., and Hillman, C. H. (2014). The association between aerobic fitness and language processing in children: implications for academic achievement. Brain Cogn. 87, 140–152. doi: 10.1016/j.bandc.2014.03.016
Seeber, M., Scherer, R., Wagner, J., Solis-Escalante, T., and Müller-Putz, G. R. (2014). EEG beta suppression and low gamma modulation are different elements of human upright walking. Front. Hum. Neurosci. 8:485. doi: 10.3389/fnhum.2014.00485
Seeber, M., Scherer, R., Wagner, J., Solis-Escalante, T., and Müller-Putz, G. R. (2015). High and low gamma EEG oscillations in central sensorimotor areas are conversely modulated during the human gait cycle. NeuroImage 112, 318–326. doi: 10.1016/j.neuroimage.2015.03.045
Severens, M., Nienhuis, B., Desain, P., and Duysens, J. (2012). Feasibility of measuring event related desynchronization with electroencephalography during walking. Conf. Proc. Annu. Int. Conf. 2012, 2764–2767. doi: 10.1109/embc.2012.6346537
Snyder, K. L., Kline, J. E., Huang, H. J., and Ferris, D. P. (2015). Independent component analysis of gait-related movement artifact recorded using eeg electrodes during treadmill walking. Front. Hum. Neurosci. 9:639. doi: 10.3389/fnhum.2015.00639
Solstad, T., Boccara, C. N., Kropff, E., Moser, M.-B., and Moser, E. I. (2008). Representation of geometric borders in the entorhinal cortex. Science 322, 1865–1868. doi: 10.1126/science.1166466
Speth, J., Speth, C., and Harley, T. A. (2015). Transcranial direct current stimulation of the motor cortex in waking resting state induces motor imagery. Conscious. Cogn. 36, 298–305. doi: 10.1016/j.concog.2015.07.005
Squire, L. R. (1992). Memory and the hippocampus: a synthesis from findings with rats, monkeys, and humans. Psychol. Rev. 99, 195–231. doi: 10.1037/0033-295X.99.2.195
Stackman, R. W., and Taube, J. S. (1997). Firing properties of head direction cells in the rat anterior thalamic nucleus: dependence on vestibular input. J. Neurosci. 17, 4349–4358.
Stark, E., Roux, L., Eichler, R., Senzai, Y., Royer, S., and Buzsáki, G. (2014). Pyramidal cell-interneuron interactions underlie hippocampal ripple oscillations. Neuron 83, 467–480. doi: 10.1016/j.neuron.2014.06.023
Steriade, M. (2003). Neuronal Substrates of Sleep and Epilepsy. Cambridge: Cambridge University Press.
Steriade, M. (2006). Grouping of brain rhythms in corticothalamic systems. Neuroscience 137, 1087–1106. doi: 10.1016/j.neuroscience.2005.10.029
Stewart, S., Jeewajee, A., Wills, T. J., Burgess, N., and Lever, C. (2014). Boundary coding in the rat subiculum. Philos. Trans. R. Soc. Lond. B. Biol. Sci. 369:20120514. doi: 10.1098/rstb.2012.0514
Stroth, S., Kubesch, S., Dieterle, K., Ruchsow, M., Heim, R., and Kiefer, M. (2009). Physical fitness, but not acute exercise modulates event-related potential indices for executive control in healthy adolescents. Brain Res. 1269, 114–124. doi: 10.1016/j.brainres.2009.02.073
Taddei, F., Bultrini, A., Spinelli, D., and Di Russo, F. (2012). Neural correlates of attentional and executive processing in middle-age fencers. Med. Sci. Sports Exerc. 44, 1057–1066. doi: 10.1249/MSS.0b013e31824529c2
Taliep, M. S., and John, L. (2014). Sport expertise: the role of precise timing of verbal-analytical engagement and the ability to detect visual cues. Perception 43, 316–332. doi: 10.1068/p7530
Tallon-Baudry, C., Bertrand, O., Delpuech, C., and Permier, J. (1997). Oscillatory gamma-band (30–70 Hz) activity induced by a visual search task in humans. J. Neurosci. 17, 722–734.
Tallon-Baudry, C., Bertrand, O., Delpuech, C., and Pernier, J. (1996). Stimulus specificity of phase-locked and non-phase-locked 40 Hz visual responses in human. J. Neurosci. 16, 4240–4249.
Tallon-Baudry, C., Bertrand, O., Hénaff, M.-A., Isnard, J., and Fischer, C. (2005). Attention modulates gamma-band oscillations differently in the human lateral occipital cortex and fusiform gyrus. Cereb. Cortex 15, 654–662. doi: 10.1093/cercor/bhh167
Tallon-Baudry, C., Bertrand, O., Peronnet, F., and Pernier, J. (1998). Induced gamma-band activity during the delay of a visual short-term memory task in humans. J. Neurosci. 18, 4244–4254.
Themanson, J. R., and Hillman, C. H. (2006). Cardiorespiratory fitness and acute aerobic exercise effects on neuroelectric and behavioral measures of action monitoring. Neuroscience 141, 757–767. doi: 10.1016/j.neuroscience.2006.04.004
Themanson, J. R., Hillman, C. H., and Curtin, J. J. (2006). Age and physical activity influences on action monitoring during task switching. Neurobiol. Aging 27, 1335–1345. doi: 10.1016/j.neurobiolaging.2005.07.002
Themanson, J. R., Pontifex, M. B., and Hillman, C. H. (2008). Fitness and action monitoring: evidence for improved cognitive flexibility in young adults. Neuroscience 157, 319–328. doi: 10.1016/j.neuroscience.2008.09.014
Thompson, T., Steffert, T., Ros, T., Leach, J., and Gruzelier, J. (2008). EEG applications for sport and performance. Methods 45, 279–288. doi: 10.1016/j.ymeth.2008.07.006
Tononi, G., and Cirelli, C. (2014). Sleep and the price of plasticity: from synaptic and cellular homeostasis to memory consolidation and integration. Neuron 81, 12–34. doi: 10.1016/j.neuron.2013.12.025
Tremayne, P., and Barry, R. J. (2001). Elite pistol shooters: physiological patterning of best vs. worst shots. Int. J. Psychophysiol. 41, 19–29. doi: 10.1016/S0167-8760(00)00175-6
Triggiani, A. I., Valenzano, A., Del Percio, C., Marzano, N., Soricelli, A., Petito, A., et al. (2015). Resting state Rolandic mu rhythms are related to activity of sympathetic component of autonomic nervous system in healthy humans. Int. J. Psychophysiol. doi: 10.1016/j.ijpsycho.2015.02.009. [Epub ahead of print].
Tsai, C.-L., Chen, F.-C., Pan, C.-Y., Wang, C.-H., Huang, T.-H., and Chen, T.-C. (2014). Impact of acute aerobic exercise and cardiorespiratory fitness on visuospatial attention performance and serum BDNF levels. Psychoneuroendocrinology 41, 121–131. doi: 10.1016/j.psyneuen.2013.12.014
Uphill, M., Groom, R., and Jones, M. (2014). The influence of in-game emotions on basketball performance. Eur. J. Sport Sci. 14, 76–83. doi: 10.1080/17461391.2012.729088
Varela, F., Lachaux, J. P., Rodriguez, E., and Martinerie, J. (2001). The brainweb: phase synchronization and large-scale integration. Nat. Rev. Neurosci. 2, 229–239. doi: 10.1038/35067550
Vertes, R. P., and Kocsis, B. (1997). Brainstem-diencephalo-septohippocampal systems controlling the theta rhythm of the hippocampus. Neuroscience 81, 893–926.
Volberg, G., and Greenlee, M. W. (2014). Brain networks supporting perceptual grouping and contour selection. Front. Psychol. 5:264. doi: 10.3389/fpsyg.2014.00264
Wagner, J., Solis-Escalante, T., Grieshofer, P., Neuper, C., Müller-Putz, G., and Scherer, R. (2012). Level of participation in robotic-assisted treadmill walking modulates midline sensorimotor EEG rhythms in able-bodied subjects. NeuroImage 63, 1203–1211. doi: 10.1016/j.neuroimage.2012.08.019
Waldert, S., Vigneswaran, G., Philipp, R., Lemon, R. N., and Kraskov, A. (2015). Modulation of the Intracortical LFP during Action Execution and Observation. J. Neurosci. 35, 8451–8461. doi: 10.1523/JNEUROSCI.5137-14.2015
Walz, J. M., Goldman, R. I., Carapezza, M., Muraskin, J., Brown, T. R., and Sajda, P. (2015). Prestimulus EEG alpha oscillations modulate task-related fMRI BOLD responses to auditory stimuli. NeuroImage 113, 153–163. doi: 10.1016/j.neuroimage.2015.03.028
Wascher, E., Heppner, H., and Hoffmann, S. (2014). Towards the measurement of event-related EEG activity in real-life working environments. Int. J. Psychophysiol. Off. J. Int. Organ. Psychophysiol. 91, 3–9. doi: 10.1016/j.ijpsycho.2013.10.006
Watrous, A. J., and Ekstrom, A. D. (2014). The spectro-contextual encoding and retrieval theory of episodic memory. Front. Hum. Neurosci. 8:75. doi: 10.3389/fnhum.2014.00075
Watrous, A. J., Fried, I., and Ekstrom, A. D. (2011). Behavioral correlates of human hippocampal delta and theta oscillations during navigation. J. Neurophysiol. 105, 1747–1755. doi: 10.1152/jn.00921.2010
Watson, B. O., and Buzsáki, G. (2015). Sleep, memory & brain rhythms. Daedalus 144, 67–82. doi: 10.1162/DAED_a_00318
Weiss, T., Beyer, L., and Hansen, E. (1991). Motor imagination-a model for motor performances? Int. J. Psychophysiol. 11, 203–205. doi: 10.1016/0167-8760(91)90012-M
West, R. L., and Thorn, R. M. (2001). Goal-setting, self-efficacy, and memory performance in older and younger adults. Exp. Aging Res. 27, 41–65. doi: 10.1080/036107301750046133
Whittingstall, K., and Logothetis, N. K. (2009). Frequency-band coupling in surface EEG reflects spiking activity in monkey visual cortex. Neuron 64, 281–289. doi: 10.1016/j.neuron.2009.08.016
Wikgren, J., Nokia, M. S., and Penttonen, M. (2010). Hippocampo-cerebellar theta band phase synchrony in rabbits. Neuroscience 165, 1538–1545. doi: 10.1016/j.neuroscience.2009.11.044
Winneke, A. H., Godde, B., Reuter, E.-M., Vieluf, S., and Voelcker-Rehage, C. (2012). The association between physical activity and attentional control in younger and older middle-aged adults: an ERP study. GeroPsych. J. Gerontopsychology Geriatr. Psychiatry 25, 207. doi: 10.1024/1662-9647/a000072
Winter, S. S., Clark, B. J., and Taube, J. S. (2015). Spatial navigation. Disruption of the head direction cell network impairs the parahippocampal grid cell signal. Science 347, 870–874. doi: 10.1126/science.1259591
Xie, L., Kang, H., Xu, Q., Chen, M. J., Liao, Y., Thiyagarajan, M., et al. (2013). Sleep drives metabolite clearance from the adult brain. Science 342, 373–377. doi: 10.1126/science.1241224
Yagi, Y., Coburn, K. L., Estes, K. M., and Arruda, J. E. (1999). Effects of aerobic exercise and gender on visual and auditory P300, reaction time, and accuracy. Eur. J. Appl. Physiol. 80, 402–408. doi: 10.1007/s004210050611
Ylinen, A., Bragin, A., Nádasdy, Z., Jandó, G., Szabó, I., Sik, A., et al. (1995). Sharp wave-associated high-frequency oscillation (200 Hz) in the intact hippocampus: network and intracellular mechanisms. J. Neurosci. 15, 30–46.
Yuval-Greenberg, S., Tomer, O., Keren, A. S., Nelken, I., and Deouell, L. Y. (2008). Transient induced gamma-band response in EEG as a manifestation of miniature saccades. Neuron 58, 429–441. doi: 10.1016/j.neuron.2008.03.027
Zarka, D., Cevallos, C., Petieau, M., Hoellinger, T., Dan, B., and Cheron, G. (2014). Neural rhythmic symphony of human walking observation: upside-down and Uncoordinated condition on cortical theta, alpha, beta and gamma oscillations. Front. Syst. Neurosci. 8:169. doi: 10.3389/fnsys.2014.00169
Zhu, F. F., Yeung, A. Y., Poolton, J. M., Lee, T. M. C., Leung, G. K. K., and Masters, R. S. W. (2015). Cathodal transcranial direct current stimulation over left dorsolateral prefrontal cortex area promotes implicit motor learning in a golf putting task. Brain Stimulat. 8, 784–786. doi: 10.1016/j.brs.2015.02.005
Keywords: EEG, biomarkers, sport, brain rythms, cortical activity
Citation: Cheron G, Petit G, Cheron J, Leroy A, Cebolla A, Cevallos C, Petieau M, Hoellinger T, Zarka D, Clarinval A-M and Dan B (2016) Brain Oscillations in Sport: Toward EEG Biomarkers of Performance. Front. Psychol. 7:246. doi: 10.3389/fpsyg.2016.00246
Received: 15 October 2015; Accepted: 08 February 2016;
Published: 26 February 2016.
Edited by:
Pietro Avanzini, University of Parma - Neuroscience Department, ItalyReviewed by:
Maddalena Fabbri Destro, Istituto Italiano di Tecnologia, ItalyMarkus Raab, German Sport University Cologne, Germany
Copyright © 2016 Cheron, Petit, Cheron, Leroy, Cebolla, Cevallos, Petieau, Hoellinger, Zarka, Clarinval and Dan. This is an open-access article distributed under the terms of the Creative Commons Attribution License (CC BY). The use, distribution or reproduction in other forums is permitted, provided the original author(s) or licensor are credited and that the original publication in this journal is cited, in accordance with accepted academic practice. No use, distribution or reproduction is permitted which does not comply with these terms.
*Correspondence: Guy Cheron, Z2NoZXJvbkB1bGIuYWMuYmU=