- 1Departament de Metodologia de les Ciències del Comportament, Facultat de Psicologia, Universitat de Barcelona, Barcelona, Spain
- 2Institut de Recerca en Cervell, Cognició i Conducta, Barcelona, Spain
In the last 15 years, many articles have studied brain connectivity in Mild Cognitive Impairment patients with fMRI techniques, seemingly using different connectivity statistical models in each investigation to identify complex connectivity structures so as to recognize typical behavior in this type of patient. This diversity in statistical approaches may cause problems in results comparison. This paper seeks to describe how researchers approached the study of brain connectivity in MCI patients using fMRI techniques from 2002 to 2014. The focus is on the statistical analysis proposed by each research group in reference to the limitations and possibilities of those techniques to identify some recommendations to improve the study of functional connectivity. The included articles came from a search of Web of Science and PsycINFO using the following keywords: f MRI, MCI, and functional connectivity. Eighty-one papers were found, but two of them were discarded because of the lack of statistical analysis. Accordingly, 79 articles were included in this review. We summarized some parts of the articles, including the goal of every investigation, the cognitive paradigm and methods used, brain regions involved, use of ROI analysis and statistical analysis, emphasizing on the connectivity estimation model used in each investigation. The present analysis allowed us to confirm the remarkable variability of the statistical analysis methods found. Additionally, the study of brain connectivity in this type of population is not providing, at the moment, any significant information or results related to clinical aspects relevant for prediction and treatment. We propose to follow guidelines for publishing fMRI data that would be a good solution to the problem of study replication. The latter aspect could be important for future publications because a higher homogeneity would benefit the comparison between publications and the generalization of results.
Introduction
In recent years, numerous papers have been published on brain connectivity, a key element to understand brain functioning (for example, Cole et al., 2010; Bai et al., 2011; Binnewijzend et al., 2012; Zanto et al., 2014). Brain connectivity has raised great interest in the field of quantitative and computational neuroscience. Computational neuroscience is devoted to identifying new tools for analysis, mathematical, and statistical modeling, and computational resources to deal with brain signal data in any modality.
Currently, several approaches and techniques have been used to quantify the different types of brain connectivity. In the present paper, we focused on those derived from functional Magnetic Resonance Imaging (fMRI): structural connectivity, functional connectivity, and effective connectivity. The reason for this selection lies in the fact that most of the existing papers focused on those three concepts of connectivity. Structural or anatomical connectivity is defined as the set of physical connections between neuronal units. The physical model of anatomic connections is relatively stable in short time periods, but hardly stable in the long term due to the morphological modifications caused by brain plasticity (Symms et al., 2004). In fact, structural MRI has become the accepted standard for routine examination of the brain, offering exquisite anatomical detail and high sensitivity to pathological changes (Symms et al., 2004). On the other hand, functional connectivity is essentially a statistical concept that studies remote neuronal network relationships that show a certain interrelation (Friston et al., 2014). Fluctuations in the blood oxygenation level-dependent (BOLD) signal in functional connectivity may present a valuable data resource for delineating the human neural functional architecture (Cole et al., 2010). On the basis of biological considerations, functional imaging can be regarded as the method that provides dynamic physiological information, whereas structural imaging provides static anatomical information (Symms et al., 2004). Lastly, effective connectivity addresses the direct influence of a brain region on the physiological activity registered in other brain regions (Friston, 1994; Friston et al., 2003). Anatomical imaging is obtained through the study of brain hydrogen and oxygen, and through the diamagnetic changes produced during a task that compromises some cognitive cost, even in a resting state (for more information, see Ogawa et al., 1990; Logothetis et al., 2001; Buxton, 2002; Huettel et al., 2009).
In recent years, there has been a growing interest in studying connectivity through the resting states. Resting-state functional MRI is an imaging method that reflects synaptic activity through changes in blood fluctuations and the oxyhemoglobin: deoxyhemoglobin ratio (Schölvinck et al., 2010; Binnewijzend et al., 2012). In this type of study, the participants may not move at all while they remain with their eyes closed without thinking about anything in particular for a specific period of time. This method permits the investigation of spontaneous activity, and the analysis of the spatiotemporal coherence of fMRI activity reveals several distinct domains of correlated activity in the brain (Schölvinck et al., 2010). The resting state allows us to minimize the amount of noise of the images obtained, given that the participants conduct no activity whatsoever and no stimulus is presented.
A strong debate has emerged of late about the best way to model functional connectivity network estimations, given the crucial role of the statistical model (Gates et al., 2010). The different options in terms of connectivity analysis techniques became clear when the authors mentioned and described four different approaches for connectivity mapping. These approaches were Structural Equation Modeling (SEM) which seemed to appear the most straightforward application, and also the most common, in connectivity estimations. In SEM, covariance patterns of contemporaneous BOLD time series illustrate brain functional connectivity via directed pathways (McIntosh and Gonzalez-Lima, 1994). Another approach was the Dynamic Causal Modeling (DCM) that uses deterministic differential equations to assess how regions relate and estimate external modulation of connections (Friston et al., 2003). The next approach was the vector autoregression (VAR), which estimates the influence that data from ROIs at previous time points have on a given ROI's BOLD activity. Finally, the authors presented an improved version of the unified SEM approach of Kim et al. (2007) to model contemporaneous and sequential relationships among ROIs (Gates et al., 2010), but they recorded the statistical analysis of this type of data.
Other methods of functional connectivity analysis that is important to mention are Granger Causality and the studies of dimensionality. Granger causality is a statistical method for assessing directional influences between simultaneously time series (Zhou et al., 2009). These directions and magnitudes of Granger causality are interpretable in terms of the directions and magnitudes of synaptic transmissions between different neurons and brain areas (Brovelli et al., 2004). On the other hand, the studies of dimensionality allow us to reduce the amount of data to analyze and thus facilitate its later analysis. It is typical to have a large amount of voxels available to conduct the analyses of this type of data. For this reason, the approaches that allowed us to reduce the amount of data while remaining independent between them, as in the case of Independent Component Analysis (ICA), which is the most typical method in this category, are very frequent in this context. In fact, reduction techniques are fundamental to establish reasonable statistical models because the use of ROIs seems logical and sensible.
Therefore, as an especially relevant aspect, we will focus on the different approaches to the study of functional connectivity, which will be itemized below. The images obtained in the study of functional connectivity (functional Magnetic Resonance Imaging—fMRI) allowed us to anatomically and functionally locate the different cognitive processes based on the increase in blood flow and neuronal activity (Bandettini et al., 1997).
Different possibilities exist when choosing the focus of the study of functional brain connectivity. Authors often opt for a particular sampling population. In some cases, the authors intended to study the connectivity of individuals suffering from a specific pathology, mostly to check whether and how that condition is reflected in connectivity. In the last decade, the population of adults suffering from Mild Cognitive Impairment (MCI) has been often chosen from among these subjects. We recognize MCI as the mild but defined deterioration in relation to the previous cognitive performance, confirmed by an observer and clinically quantified by neuropsychological tests (Flicker et al., 1991; Mueller et al., 2005). MCI has been studied on numerous occasions as the decline in cognitive functions between normal aging and Alzheimer's or other types of dementia (Dickerson et al., 2005). It has also been proven that MCI patients have a higher risk of Alzheimer's than those with no such cognitive alterations (Machulda et al., 2003; Han et al., 2011). MCI patients form a remarkably heterogenic group due to the diverse symptomatology they can present (Celone et al., 2006). Additionally, there are different levels of deterioration in MCI, which presents: participants in an early stage of illness could clearly show different connectivity patterns from those in an advanced stage (Machulda et al., 2003).
Brain connectivity in MCI patients has been widely researched recently. The complexity in designing a study on this topic lies not only in the heterogeneity of the disorder, but also in the difficulty of choosing the most valid statistical approach to analyze the fMRI data. This consideration is very important in classical approaches of clinical studies based on the comparison between groups and performance analysis, but it is more relevant if we defend the hypothesis that one particular connectivity structure can show more profound characteristics of MCI patients. Therefore, this current stage where statistical models that estimate connectivity networks are varied and, sometimes, incomparable, make it extraordinarily difficult to know the complexity of connectivity networks in detail. This specificity is not exclusive of the MCI-diagnosed population, but it has a particular relevance in this case due to the relevance of this disease and to its future increased presence because of increased life expectancy.
The choice of MCI to study functional brain connectivity is determined by the fact that it is one of the few pathologies to show brain changes at a structural level, which usually occurs in neurodegenerative disorders. For example, the decrease in the size of the hippocampus and the high atrophy in the medial temporal lobe, as well as the loss of the volume of gray matter in the frontal and parietal regions, are structural changes present in this disorder (Mueller et al., 2012). This distinctive feature makes MCI of great interest, given that we intend to study whether there is functional correspondence in such structural changes and what type it is.
In addition, from a clinical perspective, the study of MCI patients revealed a special interest of certain very important aspects. In the first place, the crucial goal is to determine those variables which would be appropriate predictors of a negative course of the pathology. Thus, it is essential to know that certain elements might indicate that one person with an MCI diagnosis could change from one domain (memory deficits) to multiple domains (language, working memory, etc.) or even develop irreversible dementia. Identifying whether the interaction between tasks, especially those connected to mnemonic performance, and connectivity networks in fMRI might be an option to predict the prognosis (Sandry and Sumowski, 2014). Likewise, a long tradition exists in the study of the effects of Cognitive Reserve (CR), according to the definition by Jones et al. (2011) in the prediction of MCI severity and evolution. Apart from the difficulty of implementing this latent variable (Lojo-Seoane et al., 2012, 2014), it is true that the analysis of possible links between CR and the connectivity network, especially in the Default Mode Network (DMN), should provide some evidence on the matter. The DMN is an extensively known connectivity network involving several brain areas, is remarkably affected in these patients and is also widely studied. Finally, due to the fact that it is a disease related to elderly populations, it seems necessary to determine whether estimated connectivity networks in different tasks might be an indication of risk or frailty in elderly people. This needs to be kept in mind for risk prediction (Sumowski et al., 2014). We must keep in mind the most clinical and applied aspects of the study of connectivity in fMRI paradigms, as it should provide further and deeper evidence on the course of the pathology.
Methodological characteristics and differences between fMRI studies were reported recently by Carp (2012). He explained the flexibility of the 241 articles consulted in terms of methodological details, such as the experimental design, data acquisition, and data analysis. The author noted too many differences in analytic procedures between articles. Finally, he concluded that this high level of analytic flexibility could be a risk factor for bias in scientific research (Carp, 2012), and he advised the use of the guidelines provided by Poldrack et al. (2008) for reporting fMRI data.
As mentioned above, there is a great diversity of statistical approaches for the study of the connectivity models in the MCI population, which makes it difficult to extract conclusions based on different authors. As a consequence of the above described situation, the aim of this paper was to facilitate an in-depth analysis of the mechanisms for statistical modeling used to estimate connectivity in MCI. We will also extract some considerations on the use of some modeling techniques or others in order to assess their advantages and disadvantages.
Additionally, we will try to analyze whether this type of study has provided some evidence on the possibility that connectivity networks in fMRI allow us to further know the role of specific clinical variables in the course of MCI.
Materials and Methods
Article Search
The databases used to conduct the article search were PsycINFO and Web of Science. In order to be included in the present study, the articles were required to comply with the following criteria: (a) be original works whose goal was to study functional connectivity through fMRI in persons with MCI and (b) had to explain the type of analysis of functional brain connectivity applied, as well as the results obtained from it. For these reasons, the key words used to conduct the search were fMRI, MCI, and Functional Connectivity. After a preliminary search with the aforementioned key words, we located 81 articles, two of which were discarded because they did not include a detailed analysis of the sample's functional brain connectivity. Then, all the selected articles contain connectivity models and statistical analysis information. Meta-analyses and reviews with those keywords were selected, but its information appears only in the descriptive sections of this document to avoid repeating information in the other sections. Thus, 79 articles were included in the present paper (identified with * in the bibliography) which contained more than one type of approach to the analysis of functional brain connectivity. For consistency, the search was replicated by two independent researchers. They obtained 100% agreement on the selected articles.
Article Assessment
For each paper selected, we analyzed specific sections in relation to the study of MCI with fMRI. Only the information given in the articles was assessed (no author was contacted to obtain further information). The necessary elements to comprehend the followed procedure in each case were selected for the functional connectivity analysis. For each paper, we assessed the goal of the research, the type of task the participants were required to do (including resting state and all types of tasks), the brain areas involved, the use of regions of interest (ROI) analysis, the connectivity estimation model used, and the data analysis techniques applied. In the results section, we summarized the main results for each section. Those results can be seen in detail for each study included in Table 1.
Sample Characterization
Numerous journals have published articles analyzing the functional brain connectivity of MCI patients. Neurobiology of Aging, Journal of Alzheimer's Disease, NeuroImage, and Human Brain Mapping are the journals with the highest number of studies published included in the present paper.
Table 2 summarizes the journals from articles were taken for analysis.
Journals such as the American Journal of Neuroradiology, Frontiers in Psychology, Journal of Magnetic Resonance Imaging, or European Journal of Radiology have published one article included in the present paper. All journals with one paper selected are included in the Others category.
The articles selected were published between 2002 and 2014, although most of them were published between 2007 and 2013. No articles were found before 2002 with the selected keywords. The exact number of articles by year of publication appears in Figure 1.
Results
The goal of the majority of the papers included was to compare the functional brain connectivity of MCI patients to the functional brain connectivity of Alzheimer's patients or that of adults with no cognitive deterioration. Accordingly, the different studies set forth different types of tasks—-detailed in the following section—in order to study the different connectivity patterns in one or several brain regions—which will also be explained below. In some cases, these regions were defined previously in the aims, and in others, they were mentioned afterwards according to the results obtained.
In some cases, the researchers intended to study the properties of fMRI imaging in relation to its reliability or the reproducibility of the data. Other studies compared fMRI to other types of brain connectivity data, such as PET imaging (Positron Emission Tomography). Lastly, the goal of some authors was to find or suggest possible biomarkers for the detection of MCI in the early stages of the disorder, which would entail a remarkable breakthrough in treatments and therapeutic interventions for these patients. It is important to note that some studies covered more than one of the goals discussed above, such as the study of brain functioning and, at the same time, presented the possibility of finding biomarkers for the early detection of AD.
Table 3 summarizes the main goals defined in the selected papers.
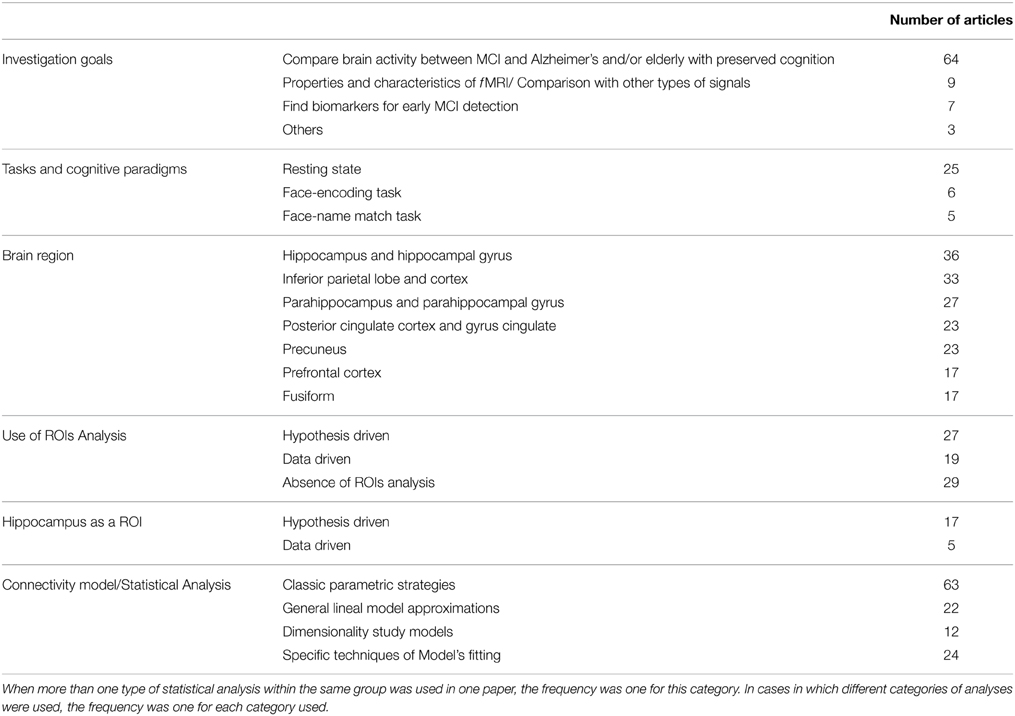
Table 3. Summary of research goals, use of ROI analysis, brain regions, and statistical analysis in the survey.
The tasks chosen by the research teams to study the functional connectivity of MCI patients varied. Many studies opted for memory tasks, mainly semantic or episodic memory, although in some cases, they also used working memory, associative memory, or even emotional memory. Most of the studies with memory tasks presented experiments with different phases in which the data obtained in the information codification phase was distinguished from the data obtained in the recovery phase. In some cases, information fixation phases were also present, as well as neutral or basal phases. This situation reflects the need to evaluate this type of patient in memory domains related to this pathology. It is important to keep in mind that MCI diagnoses always involve the presence of subjective complaints of memory mistakes and, therefore, the generation of cognitive tasks and paradigms is strongly related to common components of memory models.
However, most of the authors decided to apply a resting state paradigm in their studies. As it was explained previously, resting state allows investigating spontaneous activity, and permitting to minimize the noise of the images obtained.
Other types of tasks included problem solving, empathy tasks, sound differentiation, and visual-spatial attention tests. Most of these tasks were visual, although we could find some listening or verbal tasks. We also found papers that combined different types of tasks, or in which more than one task was conducted. More specifically, the resting state was the task most frequently asked of participants, followed by face encoding and face-name matching tasks. The frequencies of such tasks can be seen in Table 3.
The brain regions in which functional connectivity was studied varied. Some researchers suggested studying the whole encephalon, while others preferred to focus research on some specific areas.
The studies frequently focused on the brain regions of the Medial Temporal Lobe (MTL), because MCI patients often present alterations in this connectivity network. Within this lobe, the hippocampus and the regions adjacent to it appeared in almost every paper analyzed. According to the mentioned presence of disease at the DMN in these patients, we found many papers remarking on the changes in connectivity patterns in different areas within the DMN network.
Table 3 shows the brain areas that appeared most frequently in the papers analyzed, disregarding review, or meta-analysis articles, which were excluded in this table because most of the articles in those reviews and meta-analysis were included in our review and we sought to avoid repeated information.
The analysis of the Regions of Interest (ROIs) appears frequently in the papers selected. The most frequently selected ROI is the hippocampus, although often we can also find other regions of the Medial Temporal Lobe (MTL), as well as those areas included within the DMN, such as the ventro- or dorsomedial prefrontal cortex, the retrosplenial and posterior cingulated cortexes, the inferior parietal lobe, and the hippocampus (including the entorhinal and parahippocampal cortexes) (Buckner et al., 2008).
Table 3 shows the number of articles that opted for the analysis of ROIs defined prior to the data collection (Hypothesis Driven); it also shows those that conducted it after an early analysis based on the detection of activated areas (Data Driven). Additionally, it shows those that did not use this type of approach. Review or meta-analysis articles were disregarded. It can be seen that most of the authors chose to conduct the analysis of ROIs, especially the analysis of regions defined prior to the data collection (Hypothesis Driven). However, the Data Driven choice was also made by many research teams. Nevertheless, this description does not contribute to the choice of the statistical model. Due to the diversity in statistical resources employed, it was difficult to identify comparable results.
Connectivity Estimation Model and Data Analysis
As mentioned early in this paper, the authors made numerous proposals to analyze fMRI, and they used a wide variety of models to estimate functional connectivity. In order to organize the different types of analyses and approaches, we decided to put forward a descriptive classification of the different types of models and to do so in four large groups: (i) Classic parametric strategies; (ii) Approaches based on the general linear model; (iii) Studies based on models pertaining to the study of dimensionality; and finally (iv) techniques based on fitting specific models. This classification was generated with the unique proposal of arranging the different approaches and techniques used through a significantly recognizable group. Then, we tried to facilitate the ordering of statistical models that were often used in this field.
The classic parametric strategies were the most used by researchers in this field. Within this group, the authors chose a large number of different analyses, among which we found, for example, correlations, partial and semi-partial correlations, Kendall's coefficient of concordance, t tests, ANOVA, and ANCOVA, the Random Effects Model, the Mixed Effects Model, Dunn and Clarck's Zj statistic, serial correlations and Fisher's transformation of scores into z values. The benefit of these approaches lies in the facility to recognize and replicate them in most cases. The negative aspects reside in the difficulty to accomplish the statistical assumptions of every technique, especially the population normality of the distributions analyzed and the homoscedasticity assumption. In addition, when these techniques are employed in the generation of descriptive models (with limited possibility of inference) the objective remains far from what is required, which is nothing but a complex connectivity model. In addition, it is unusual to find these approaches in independence and self-correlation registers, which further hinders an optimal statistical approach.
We also found approaches to the general linear model with different analyses such as regressions and the discriminating analysis. Along these lines we found different choices from the simplest models [regression models with Ordinary Least Squares estimations (OLS)] to more complex ones based on Structural Equation Models or, occasionally, Path Analysis Models. Regarding the previous paragraph, data on the viability of linear and non-linear (frequently linearized) models is usually unavailable as regards model assumptions or the conditions of application. Most of these models are located within the domain of parametric statistical models, which leads to the same situation we described above. We noticed a scant description of assumptions and adopted modifications to ensure a correct parameter-estimation technique. Likewise, there are no acceptable approaches to the residuals generated by the models studied eventually. Neither are there assessments of the residuals' or the structural errors' independence from one another or between the signal values. To sum up, it seems inarguable that the authors should provide technical data on model fitting. This information would allow us to know in detail to what extent we find more descriptive models than the ones the authors intended to fit. One simple example will serve to illustrate this detail: it is unusual to report the value of the determination coefficient in uniequational and also multiequational models.
Of course, we can find the studies of dimensionality. As we mentioned before, these are widely applied in this field, and many researchers conducted the ICA. However, like in the situations described above, no special tradition exists to offer variability results in dimensionality reduction, either by ICA, as previously mentioned, or by Principal Component Analysis (PCA). In both cases, the selection of maximum explained variance vectors entails a process based on the normalization of the vectors representing the voxels' original values with regard to the ROI defined. Usually, there is no mention of the conditions of sphericity of the voxels selected or of the values that characterize the statistical viability of each ROI. The fact that ROI size is a determining factor to obtain a good solution (either in ICA or PCA) is unimportant. In smaller ROIs the conditions of unidimensionality are easier to obtain than in other techniques. Regarding these considerations, only seldom can we obtain values of explained variance in selected voxels. It could be irrelevant if variance values assumed by ROIs were high, as it usually happens but not always, and these cases go unnoticed because they are not reported.
Lastly, many authors chose for the analysis to conduct a model fit, which was mostly used to fit the data prior to the analysis. In this category, we found numerous examples, such as the Small World Analysis, the Gaussian Random field theory, ROC curves, behavioral vectors, the deconvolution analysis, the segmentation of brain regions, ALFF (Amplitude of Low Frequency Fluctuations) and fALFF (fractional Amplitude of Low Frequency Fluctuations) analyses, the spatial extent analysis, the estimation of deformation fields, the analysis of Regional Homogeneity (ReHo), the Granger Causality Analysis, the analysis of functional synchrony and DICCCOLs, the Dynamic Causal Model, and the Structural Equation Model. As we mentioned above, part of these approaches show the advantages and disadvantages of common linear models. Nevertheless, in our opinion, those are the most reasonable approaches to the study of connectivity, at least so far. The advantages are related to a fundamental issue, which is the fact that those techniques are devoted to the complexity of multiequational structures with a dynamic substratum. It is obvious that they are probably very far away from a feasible representational model, but they still imply a representational model that favors the networks and the comprehension of the structures. However, these techniques still need statistical complexity and, without some previous information, they are very difficult to replicate. For example, it is usual to omit information related to the estimation techniques and their defining values. Convergence criteria are not cited, neither are the values of initial solutions (if there are any) or the conditions of parametrization and reparametrization of estimations. Also, nothing is said about whether the authors opted for full or partial estimations, or whether they opted for colinearity robust estimations (like two-steps techniques) or for techniques based on parameter ponderation (like Weight Least Square). Finally, in this type of approach, the authors usually offer the final results in a simplified way completed with any kind of graph showing the activated areas in the brain, but usually no information is included about parameter intensity and what it means for the connectivity network.
It should be noted that it is common to use more than one analysis in a publication. Accordingly, many authors choose more than one test for each study. Specifically, in 32 papers, the researchers applied analytic techniques pertaining to different categories from the classification above.
Table 3 shows the frequencies of the main analyses and connectivity models proposed by the authors according to the classification used previously. The study of these papers about connectivity and MCI showed an obvious effect that confirms the scarcity of replicable works in an exact way, regarding the statistical models used. This situation, as mentioned above, is not exclusive of this domain but needs to be considered as an important problem to solve. In the following section we offer more details about this situation and some viable recommendations to solve it.
Clinical Results
In this section we intend to emphasize how the articles selected did not tackle, in general, the clinical aspects of the pathology. Only few works noticed the relationship between the estimated connectivity network and the intra- and between-groups effects; in the former case, in order to distinguish networks of specific population groups (for example, control vs. clinical group) and, in the latter case, for longitudinal course studies (for example, estimated networks in repeated measures paradigms). The results presented in these papers focus occasionally on secondary aspects such as those showing the effects of different degrees of severity (Miller et al., 2008) or those regarding the earliest stages of the pathology, when subjective and memory complaints are reported and, therefore, these are very subtle cognitive mistakes (Machulda et al., 2009).
It is true that, in some articles, we can find clinical implications of connectivity estimation. One example is the verification of the existence of compensatory mechanisms in different brain areas. This means that we can find some increased activity in particular regions of MCI patients as compared to normal adults to compensate the deficits in other areas (Krishnan et al., 2006; Bai et al., 2008). Another example is that increased activation in the hippocampus to solve memory tasks seems to predict early detection of Alzheimer's Disease (AD) (Dickerson et al., 2005; Mueller et al., 2012). However, in most of the publications we found the importance of the hippocampus regions on different aspects because it is one of the regions most involved in MCI pathology. Accordingly, changes in hippocampus activity are present in most MCI patients (Greicius et al., 2004; Johnson et al., 2004; Miller et al., 2008; Wang et al., 2011), converters and non-converters to AD, which make it difficult to conclude that changes in hippocampus activity could predict AD.
Therefore, we have seen that there is some clinical information in the articles included herein, but, in general, there is a scarcity of reported information on clinical consequences in the estimation of cognitive networks in MCI.
Conclusions
In this article, we summarized the main features of the studies on functional brain connectivity through fMRI in MCI patients. Based on 79 publications, we described the most relevant elements, especially statistical models for the estimation of connectivity and some considerations on the clinical consequences of those studies.
Thus, most articles aimed to compare the functional brain connectivity network in MCI patients with that of Alzheimer's patients and/or adults with preserved cognition. Many authors opted for semantic or episodic memory tasks, although resting state designs are becoming more frequent. These allowed us to reduce the amount of interference in the data obtained. It seems reasonable to assume that fostering studies based on the DMN involves a simpler experimental system and fewer confounding variables than certain complex cognitive paradigms whose activation correlates are not clear. The brain region that is most frequently activated with significance on the connectivity network estimated in these articles is the hippocampus. However, in resting state designs there is a remarkable presence of other MTL and DMN regions, such as the inferior parietal lobe and the parahippocampal region. All of this is consistent with the definition of the DMN usually assumed. It is also the most studied region in the analysis of ROIs, both in the definitions before and after early connectivity analysis.
Undoubtedly we found the widest variety in the models of statistical estimation of functional brain connectivity chosen, as well as in the data analysis techniques used in relation to the general models. Most authors choose classic parametric strategies. Estimations based on Pearson's correlation were common, and so were estimations of partial and semi-partial correlations in order to isolate the effects as efficiently as possible. It seems evident that this procedure attempted to reduce the perverse effects of colinearity. However, within this category, we still found a large number of diverse analyses. Indeed, parametric statistical tests are frequent, and they are used to contrast specific data between samples and effects. This effect might be caused by the need to compare the different samples, because once they conducted an early connectivity analysis, many authors chose to present the results of the parametric tests in order to compare some specific data. The use of Student's t or ANOVA tests, both included in this category, is frequent in this type of paper, although they are not specific techniques for connectivity estimation. Accordingly, the difference in the values of specific ROIs among groups is often mistaken for differences in the structure and intensity of connectivity networks.
The results obtained in the different publications were diverse, which is consistent with the different goals predetermined by the authors. Still, we found a series of common features regarding functional brain connectivity in MCI patients. First, it was common to observe a reduced brain activity in many of the studied regions, such as the hippocampus or the lateral parietal cortex. Likewise, we also noticed increased brain activity in MTL regions during the task or in DMN regions during the resting state. The authors concluded that these were compensatory mechanisms; that is, in order to solve the task correctly, MCI patients required more activity and involvement from brain regions than the participants without cognitive deterioration. Therefore, the participants seemed to compensate for reduced connectivity in some regions by involving others, or even by increasing the activation within them. Other authors distinguished amnesic MCI patients from non-amnesic ones, and they concluded that the latter showed better performance and more preserved functional connectivity in general. Lastly, many articles proposed possible biomarkers of future cognitive deterioration or future progression to AD. The most frequent biomarkers found in those articles were changes in the DMN and hippocampus connectivity patterns, as well as those in the lateral temporal lobe and the posterior cingulate cortex. The images from fMRI were optimal for this type of study.
Taking into account the aforementioned information available in the articles included herein, we verified that the study of functional brain connectivity in MCI patients was a difficult subject to approach, as noted by Carp (2012) in studying general fMRI articles. The publications we consulted showed great variability concerning the connectivity model chosen. This fact may reflect the difficulty inherent in the choice of an ideal method to analyze brain connectivity. Given the remarkable differences between the techniques, it was difficult to make the right choice. In fact, in most cases, there seemed to be no theoretical justification. This situation also makes difficult to elaborate a meta-analysis of this topic, because of the disparity of methods and approaches, and the lack of statistical details. We can find some meta-analysis regarding clinical results, but it would be very difficult if we want to keep in mind statistical results and functional connectivity models found.
We did not find a previous article about this subject, so we consider that our summary provides a global idea about the state of statistical analysis in functional connectivity studies. We established several relevant aspects:
• The use of such dispersed statistical models prevents the comparison of results in an accumulative and integrated way. In fact, most papers do not justify their choice or establish clearly to what extent the assumptions of each model have been proven, assumed, or simply forgotten. The impossibility to compare results makes it very difficult to make suprastructural estimations of regular connectivity networks.
• Likewise, the complexity inherent to this type of statistical approach involves extreme difficulty in replicating analytical procedures. Not only are the data processing phases opaque, but some of the algorithms used are also underdeveloped. Except for the cases based on the estimations of correlations, it is truly difficult to strictly replicate some analytical procedures.
• In the case of the networks estimated in the papers under consideration, this situation is exactly the same and makes it unfeasible to conduct a simple accumulation of connectivity networks.
• In addition, this implies that each statistical model studies the conception of connectivity in a different way. Regardless of statistical matters, suffice it to say that the connectivity network based on simple parametric tests does not lead to the same result as Bayesian-based complex statistical tests. That being said, they coexist cooperatively in this study sector.
• An attempt to group the different statistical approaches only showed the disparity and lack of specificity we already mentioned. The classification we are proposing is just a general description that allows us to identify the primary areas of statistical interest.
• It is also evident that, in the case of MCI, the results were dependent on aspects outside the phenomenon at hand. The choice of technique has a strong influence on the result on which we base our choices of prediction and knowledge of the characteristics of these patients. Obviously, we should be alerted by the system's fragility.
We believe that this document could provide an idea of the complexity of article replication in functional connectivity studies of MCI patients. We considered that it is important to provide tools to the clinical professionals to better understand the MCI characteristics and elements to focus the diagnosis and treatment for every patient. It would be difficult if every article used a different approach in their analysis, so we aimed to highlight this point to the research community to improve the comparisons of results'.
Regarding the most clinical details about this pathology, our data shows that studies on connectivity networks have not provided, so far, relevant information for the applied field. Thus, we found little information on the CR protector effect on MCI appearance or on how networks show specific patterns for individuals with crystallized CR. Also, there is a lack of information about the use of networks for severity and risk prediction. Additionally, relevant information on the possible effect of frailty in the elderly for network estimation is missing, too. As we mentioned above, we can find some articles that provide possible biomarkers to detect MCI converters to AD, but these biomarkers seem to be far from an actual detection of AD converters. Evidently, this field is very complex and we are in a primary stadium of knowledge, which is insufficient to answer most of these unsolved questions and other characteristics of MCI. However, our paper does show that the problem comes before the study of clinical consequences as the use of technology and dispersed models causes difficulties in this matter. Consequently, we seem to be far from using these studies for clinical categorization in MCI patients.
As regards the differences in the information reported between articles, it could be useful to have guidelines to establish which information should be reported in an article on this topic in order to understand and replicate a study. Following the guidelines proposed by Poldrack et al. (2008) and, mentioned by Carp (2012) would facilitate the unification of information in the articles. Then, it would be possible to easily find the same information in every document and would clarify the statistical approach used. Also, it would allow for a global idea of this topic and would help in the elaboration on meta-analysis in this topic.
Furthermore, all things considered, it seems necessary to establish some recommendations for the MCI field and also for the general scope of connectivity estimation models from fMRI paradigms. They should, i) adopt techniques based on easily estimable statistics, such as correlation coefficients; ii) identify and describe all the phases in statistical analysis and to identify the tools used by its application; iii) provide instructions and analyses in an annex form, to be used in other data-bases or allow for replication; iv) clearly establish the correction mechanisms and their values in cases of classical corrections, such as the Bonferroni correction as well as more elaborated corrections, such as the False Discovery Rate (FDR); v) facilitate the fulfillment of model assumptions; vi) generate works with sufficient sample sizes to support a statistical model compatible with statistical predictor tools; and vii) clearly offer effectuated modifications or settings to the general estimation techniques.
In conclusion, we believe that the aforementioned aspects should be taken into account for future publications on functional brain connectivity in MCI patients. This way, better homogeneity would be achieved in brain connectivity study models and data analyses. This would make it possible to make comparisons between studies and the results would be more easily generalized.
Conflict of Interest Statement
The authors declare that the research was conducted in the absence of any commercial or financial relationships that could be construed as a potential conflict of interest.
Acknowledgments
This study was supported by the Grup de Recerca en Tècniques Estadístiques Avançades Aplicades a la Psicologia (GTEAAP) members of the Generalitat de Catalunya's 2014 SGR 326 Consolidated Research Group (GRC) and was made possible by the PSI2013-41400-P project of Ministerio de Economía y Competitividad of the Spanish Government.
References
*Agosta, F., Rocca, M. A., Pagani, E., Absinta, M., Magnani, G., Marcone, A., et al. (2010). Sensorimotor network rewiring in mild cognitive impairment and Alzheimer's disease. Hum. Brain Mapp. 31, 515–525. doi: 10.1002/hbm.20883
*Alichniewicz, K. K., Brunner, F., Klünemann, H. H., and Greenlee, M. W. (2013). Neural correlates of saccadic inhibition in healthy elderly and patients with amnestic mild cognitive impairment. Front. Psychol. 4:467. doi: 10.3389/fpsyg.2013.00467
*Baglio, F., Castelli, I., Alberoni, M., Blasi, V., Griffanti, L., Falini, A., et al. (2012). Theory of mind in amnestic mild cognitive impairment: an fMRI study. J. Alzheimers Dis. 29, 25–37. doi: 10.3233/JAD-2011-111256
*Bai, F., Liao, W., Watson, D. R., Shi, Y., Yuan, Y., Cohen, A. D., et al. (2011). Mapping the altered patterns of cerebellar resting-state function in longitudinal amnestic mild cognitive impairment patients. J. Alzheimers Dis. 23, 87–99. doi: 10.3233/JAD-2010-101533
*Bai, F., Zhang, Z., Yu, H., Shi, Y., Yuan, Y., Zhu, W., et al. (2008). Default-modenetworkactivitydistinguishes amnestic type mild cognitive impairment from healthy aging: a combined structural and resting-state functional MRI study. Neurosci. Lett. 438, 111–115. doi: 10.1016/j.neulet.2008.04.021
Bandettini, P. A., Kwong, K. K., Tootell, R. B. H., Wong, E. C., Fox, P. T., Belliveau, J. W., et al. (1997). Characterization of Cerebral Blood Oxygenation and flow changes during prolonged brain activation. Hum. BrainMapp. 5, 93–109.
*Binnewijzend, M. A. A., Schoonheim, M. M., Sanz-Arigita, E., Wink, A. M., van der Flier, W. M., Tolboom, N., et al. (2012). Resting-state fMRI changes in Alzheimer's disease and mild cognitive impairment. Neurobiol. Aging 33, 2018–2028. doi: 10.1016/j.neurobiolaging.2011.07.003
*Bokde, A. L. W., Lopez-Bayo, P., Born, C., Dong, W., Meindl, T., Leinsinger, G., et al. (2008). Functional abnormalities of the visual processing system in subjects with mild cognitive impairment: an fMRI study. Psychiatry Res. 163, 248–259. doi: 10.1016/j.pscychresns.2007.08.013
*Bokde, A. L. W., Lopez-Bayo, P., Meindl, T., Pechler, S., Born, C., Faltraco, F., et al. (2006). Functional connectivity of the fusiform gyrus during a face-matching task in subjects with mild cognitive impairment. Brain 129, 1113–1124. doi: 10.1093/brain/awl051
Brovelli, A., Ding, M., Ledberg, A., Chen, Y., Nakamura, R., and Bressler, S. L. (2004). Beta oscillations in a large-scale sensorimotor cortical network: directional influences revealed by Granger causality. Proc. Natl. Acad. Sci. U.S.A. 101, 9849–9854. doi: 10.1073/pnas.0308538101
*Browndyke, J. N., Giovanello, K., Petrella, J., Hayden, K., Chiba-Falek, O., Tucker, K., et al. (2013). Phenotypic regional functional imaging patterns during memory encoding in mild cognitive impairment and Alzheimer's disease. Alzheimers Dement. 9, 284–294. doi: 10.1016/j.jalz.2011.12.006
Buckner, R. L., Andrews-Hanna, J. R., and Schacter, D. L. (2008). The brain's default network: anatomy, function, and relevance to disease. Ann. N.Y. Acad. Sci. 1124, 1–38. doi: 10.1196/annals.1440.011
Buxton, R. B. (2002). Introduction to Functional Magnetic Resonance Imaging. Principles & Techniques. New York, NY: Cambridge University Press.
Carp, J. (2012). The secret lives of experiments: Methods reporting in the fMRI literature. Neuroimage 63, 289–300. doi: 10.1016/j.neuroimage.2012.07.004
*Celone, K. A., Calhoun, V. D., Dickerson, B. C., Altri, A., Chua, E. F., Miller, S. L., et al. (2006). Alterations in memory networks in mild cognitive impairment and alzheimer's disease: an independent component analysis. Neurobiol. Dis. 26, 10222–10231. doi: 10.1523/jneurosci.2250-06.2006
*Clément, F., and Belleville, S. (2009). Test-retest reliability of fMRI verbal episodic memory paradigms in healthy older adults and in persons with mild cognitive impairment. Hum. Brain Mapp. 30, 4033–4047. doi: 10.1002/hbm.20827
*Clément, F., and Belleville, S. (2012). Effect of disease severity on neural compensation of item and associative recognition in mild cognitive impairment. J. Alzheimers Dis. 29, 109–123. doi: 10.3233/JAD-2012-110426
*Clément, F., Gauthier, S., and Belleville, S. (2013). Executive functions in mild cognitive impairment: emergence and breakdown of neural plasticity. Cortex 49, 1268–1279. doi: 10.1016/j.cortex.2012.06.004
Cole, D. M., Smith, S. M., and Beckmann, C. F. (2010). Advances and pitfalls in the analysis and interpretation of resting-state FMRI data. Front. Syst. Neurosci. 4:8. doi: 10.3389/fnsys.2010.00008
*De Rover, M., Pironti, V. A., McCabe, J. A., Acosta-Cabronero, J., Arana, F. S., Morein-Zamir, S., et al. (2011). Hippocampal dysfunction in patients with mild cognitive impairment: a functional neuroimaging study of a visuospatial paired associates learning task. Neuropsychologia 49, 2060–2070. doi: 10.1016/j.neuropsychologia.2011.03.037
*Dickerson, B. C., Salat, D. H., Bates, J. F., Atiya, M., Killiany, R. J., Greve, D. N., et al. (2004). Medial temporal lobe function and structure in mild cognitive impairment. Ann. Neurol. 56, 27–35. doi: 10.1002/ana.20163
*Dickerson, B. C., Salat, D. H., Greve, D. N., Chua, E. F., Rand-Giovannetti, E., Rentz, D. M., et al. (2005). Increased hippocampal activation in mild cognitive impairment compared to normal aging and AD. Neurology 65, 404–411. doi: 10.1212/01.wnl.0000171450.97464.49
*Dickerson, B. C., and Sperling, R. A. (2008). Functional abnormalities of the medial temporal lobe memory system in mild cognitive impairment and Alzheimer's disease: insights from functional MRI studies. Neuropsychologia 46, 1624–1635. doi: 10.1016/j.neuropsychologia.2007.11.030
*Dunn, C. J., Duffy, S. L., Hickie, I. B., Lagopoulos, J., Lewis, S. J. G., Naismith, S. L., et al. (2014). Deficits in episodic memory retrieval reveal impaired default mode network connectivity in amnestic mild cognitive impairment. Neuroimage 4, 473–480. doi: 10.1016/j.nicl.2014.02.010
*Faraco, C. C., Puente, A. N., Brown, C., Terry, D. P., and Stephen Miller, L. (2013). Lateral temporal hyper-activation as a novel biomarker of mild cognitive impairment. Neuropsychologia 51, 2281–2293. doi: 10.1016/j.neuropsychologia.2013.07.023
Flicker, C., Ferris, S. H., and Reisberg, B. (1991). Mild cognitive impairment in the elderly: predictors of dementia. Neurology 41, 1006–1009. doi: 10.1212/WNL.41.7.1006
*Frings, L., Dressel, K., Abel, S., Saur, D., Kümmerer, D., Mader, I., et al. (2010). Reduced precuneus deactivation during object naming in patients with mild cognitive impairment, Alzheimer's disease, and frontotemporal lobar degeneration. Dement. Geriatr. Cogn. Disord. 30, 334–343. doi: 10.1159/000320991
Friston, K. J., Harrison, L., and Penny, W. (2003). Dynamic causal modelling. Neuroimage 19, 1273–1302. doi: 10.1016/S1053-8119(03)00202-7
Friston, K. J., Kahan, J., Biswal, B., and Razi, A. (2014). A DCM for resting state fMRI. Neuroimage 94, 396–407. doi: 10.1016/j.neuroimage.2013.12.009
Friston, K. J. (1994). Functional an effective connectivity in neuroimaging: a synthesis. Hum. Bran Mapp. 2, 56–78. doi: 10.1002/hbm.460020107
Gates, G. A., Gibbons, L. E., McCusrry, S. M., Crane, P. K., Feeney, M. P., and Larson, E. B. (2010). Executive dysfunction and presbycusis in older persons with and without memory loss and dementia. Cogn. Behav. Neurol. 23, 218–223. doi: 10.1097/WNN.0b013e3181d748d7
*Gold, B. T., Jiang, Y., Jicha, G. A., and Smith, C. D. (2010). Functional response in ventral temporal cortex differentiates mild cognitive impairment from normal aging. Hum. Brain Mapp. 31, 1249–1259. doi: 10.1002/hbm.20932
*Graewe, B., Lemos, R., Ferreire, C., Santana, I., Farivar, R., De Weerd, P., et al. (2012). Impaired processing of 3D motion-defined faces in mild cognitive impairment and healthy aging: an fMRI study. Cereb. Cortex 23, 2489–2499. doi: 10.1093/cercor/bhs246
*Greicius, M. D., Srivastava, G., Reiss, A. L., and Menon, V. (2004). Default-mode network activity distinguishes Alzheimer's disease from healthy aging: evidence from functional MRI. Proc. Natl. Acad. Sci. U.S.A. 101, 4637–4642. doi: 10.1073/pnas.0308627101
*Hahn, K., Myers, N., Prigarin, S., Rodenacker, K., Kurz, A., Förstl, H., et al. (2013). Selectively and progressively disrupted structural connectivity of functional brain networks in Alzheimer's disease - revealed by a novel framework to analyze edge distributions of networks detecting disruptions with strong statistical evidence. Neuroimage 81, 96–109. doi: 10.1016/j.neuroimage.2013.05.011
*Haller, S., Montandon, M.-L., Rodriguez, C., Moser, D., Toma, S., Hofmeister, J., et al. (2014). Acute caffeine administration effect on brain activation patterns in mild cognitive impairment. J. Alzheimers Dis. 41, 101–112. doi: 10.3233/JAD-132360
*Hämäläinen, A., Pihlajamäki, M., Tanila, H., Hänninen, Niskanen, E., Tervo, S., Karjalainen, P. A., et al. (2007). Increased fMRI responses during encoding in mild cognitive impairment. Neurobiol. Aging 28, 1889–1903. doi: 10.1016/j.neurobiolaging.2006.08.008
*Hampstead, B. M., Stringer, A. Y., Stilla, R. F., Deshpande, G., Hu, X., Moore, A. B., et al. (2011). Activation and effective connectivity changes following explicit-memory training for face-name pairs in patients with mild cognitive impairment: a pilot study. Neurorehabil. Neural Repair 25, 210–222. doi: 10.1177/1545968310382424
*Han, S. D., Arfanakis, K., Fleischman, D. A., Leurgans, S. E., Tuminello, E. R., Edmonds, E. C., et al. (2012). Functional connectivity variations in mild cognitive impairment: associations with cognitive function. J. Int. Neuropsychol. Soc. 18, 39–48. doi: 10.1017/S1355617711001299
*Han, Y., Wang, J., Zhao, Z., Min, B., Lu, J., Li, K., et al. (2011). Frequency-dependent changes in the amplitude of low-frequency fluctuations in amnestic mild cognitive impairment: a resting-state fMRI study. Neuroimage 55, 287–295. doi: 10.1016/j.neuroimage.2010.11.059
Huettel, S. A., Song, A. W., and McCarthy, G. (2009). Functional Magnetic Resonance Imaging, Second 2nd Edn. Massachusetts, MA: Sinauer Associates.
*Jauhiainen, A. M., Pihlajamäki, M., Tervo, S., Niskanen, E., Tanila, H., Hänninen, T., et al. (2009). Discriminating accuracy of medial temporal lobe volumetry and fMRI in mild cognitive impairment. Hippocampus 19, 166–175. doi: 10.1002/hipo.20494
*Jin, M., Pelak, V., and Cordes, D. (2012). Aberrant default mode network in subjects with amnestic mild cognitive impairment using resting-state functional MRI. Magn. Reson. Imaging 30, 48–61. doi: 10.1016/j.mri.2011.07.007
*Johnson, S. C., Baxter, L. C., Susskind-Wilder, L., Connor, D. J., Sabbagh, M. N., and Caselli, R. J. (2004). Hippocampal adaptation to face repetition in healthy elderly and mild cognitive impairment. Neuropsychologia 42, 980–989. doi: 10.1016/j.neuropsychologia.2003.11.015
*Johnson, S. C., Schmitz, T. W., Moritz, C. H., Meyerand, M. E., Rowley, H. A., Alexander, A. L., et al. (2006). Activation of brain regions vulnerable to Alzheimer's disease: the effect of mild cognitive impairment. Neurobiol. Aging 27, 1604–1612. doi: 10.1016/j.neurobiolaging.2005.09.017
Jones, R. N., Manly, J., Glymour, M. M., Rentz, D. M., Jefferson, A. L., and Stern, Y. (2011). Conceptual and measurement challenges in research on cognitive reserve. J. Int. Neuropsychol. Soc 17, 1–9. doi: 10.1017/S1355617710001748
*Kaufmann, L., Ischebeck, A., Weiss, E., Koppelstaetter, F., Siedentopf, C., Vogel, S. E., et al. (2008). An fMRI study of the numerical Stroop task in individuals with and without minimal cognitive impairment. Cortex 44, 1248–1255. doi: 10.1016/j.cortex.2007.11.009
Kim, J., Zhu, W., Chang, L., Bentler, P. M., and Ernst, T. (2007). Unified structural equation modeling approach for the analysis of multisubject, multivariate functional MRI data. Hum. Brain Mapp. 28, 85–93. doi: 10.1002/hbm.20259
*Kircher, T. T., Weis, S., Freymann, K., Erb, M., Jessen, F., Grodd, W., et al. (2007). Hippocampal activation in patients with mild cognitive impairment is necessary for successful memory encoding. J. Neurol. Neurosurg. Psychiatr. 78, 812–818. doi: 10.1136/jnnp.2006.104877
*Kochan, N. A., Breakspear, M., Slavin, M. J., Valenzuela, M., McCraw, S., Brodaty, H., et al. (2010). Functional alterations in brain activation and deactivation in mild cognitive impairment in response to a graded working memory challenge. Dement. Geriatr. Cogn. Disord. 30, 553–568. doi: 10.1159/000322112
*Krishnan, S., Slavin, M. J., and Tran, T. T. (2006). Mild cognitive impairment: evaluation with 4-T functional MR purpose: methods: results: conclusion: Radiology 240, 177–186. doi: 10.1148/radiol.2401050739
*Lenzi, D., Serra, L., Perri, R., Pantano, P., Lenzi, G. L., Paulesu, E., et al. (2011). Single domain amnestic MCI: a multiple cognitive domains fMRI investigation. Neurobiol. Aging 32, 1542–1557. doi: 10.1016/j.neurobiolaging.2009.09.006
*Li, S.-J., Li, Z., Wu, G., Zhang, M.-J., Franczak, M., and Antuono, P. G. (2002). Alzheimer Disease: evaluation of a functional MR imaging index as a marker. Radiology 225, 253–259. doi: 10.1148/radiol.2251011301
*Liang, P., Li, Z., Deshpande, G., Wang, Z., Hu, X., and Li, K. (2014). Altered causal connectivity of resting state brain networks in amnesic MCI. PLoS ONE 9:e88476. doi: 10.1371/journal.pone.0088476
*Liu, Z., Zhang, Y., Yan, H., Bai, L., Dai, R., Wei, W., et al. (2012). Altered topological patterns of brain networks in mild cognitive impairment and Alzheimer's disease: a resting-state fMRI study. Psychiatry Res. 202, 118–125. doi: 10.1016/j.pscychresns.2012.03.002
Logothetis, N. K., Pauls, J., Augath, M., Trinath, T., and Oeltermann, A. (2001). Neurophysiological investigation of the basis of the fMRI Signal. Nat. 412, 150–157. doi: 10.1038/35084005
Lojo-Seoane, C., Facal, D., Guàrdia-Olmos, J., and Juncos-Rabadán, O. (2014). Structural model for estimating the influence of cognitive reserve on cognitive performance in adults with subjective memory complaints. Arch. Clin. Neuropsychol. 29, 245–255. doi: 10.1093/arclin/acu007
Lojo-Seoane, C., Facal, D., and Juncos-Rabadán, O. (2012). ¿Previene la actividad intelectual el deterioro cognitivo? Relaciones entre reserva cognitiva y deterioro cognitivo ligero. Rev. Esp. Geriatr. Gerontol. 47, 270–278. doi: 10.1016/j.regg.2012.02.006
*Machulda, M. M., Senjem, M. L., Weigand, S. D., Smith, G. E., Ivnik, R. J., Boeve, B. F., et al. (2009). Functional magnetic resonance imaging changes in amnestic and nonamnestic mild cognitive impairment during encoding and recognition tasks. J. Int. Neuropsychol. Soc. 15, 372–382. doi: 10.1017/S1355617709090523
*Machulda, M. M., Ward, H. A., Borowski, B., Gunter, J. L., Cha, R. H., O'Brien, P. C., et al. (2003). Comparison of memory fMRI response among normal, MCI, and Alzheimer's patients. Neurology 61, 500–519. doi: 10.1212/01.WNL.0000079052.01016.78
*Mandzia, J. L., McAndrews, M. P., Grady, C. L., Graham, S. J., and Black, S. E. (2009). Neural correlates of incidental memory in mild cognitive impairment: an fMRI study. Neurobiol. Aging 30, 717–730. doi: 10.1016/j.neurobiolaging.2007.08.024
McIntosh, A. R., and Gonzalez-Lima, F. (1994). Structural equation modeling and its application to network analysis in functional brain imaging. Hum. Brain Mapp. 2, 2–22. doi: 10.1002/hbm.460020104
*Miller, S. L., Fenstermacher, E., Bates, J., Blacker, D., Sperling, R. A., and Dickerson, B. C. (2008). Hippocampal activation in adults with mild cognitive impairment predicts subsequent cognitive decline. J. Neurol. Neurosurg. Psychiatr. 79, 630–635. doi: 10.1136/jnnp.2007.124149
Mueller, S. G., Weiner, M. W., Thal, L. J., Petersen, R. C., Jack, C., Jagust, W., et al. (2005). The Alzheimer's disease neuroimaging initiative. Neuroimaging Clin. N. Am. 15, 869–877. doi: 10.1016/j.nic.2005.09.008
*Mueller, S., Keeser, D., Reiser, M. F., and Teipel, S. (2012). Functional and Structural MR imaging in neuropsychiatric disorders, Part 1: imaging techniques and their application in mild cognitive impairment and Alzheimer Disease. AJNR Am. J. Neuroradiol. 33, 1845–1850. doi: 10.3174/ajnr.A2799
Ogawa, S., Lee, T. M., Kay, A. R., and Tank, D. W. (1990). Brain magnetic resonance imaging with contrast dependent on blood oxygenation. Proc. Natl. Acad. Sci. U.S.A. 87, 9868–9872. doi: 10.1073/pnas.87.24.9868
Parra, M. A., Pattan, V., Wong, D., Beaglehole, A., Lonie, J., Wan, H. I., et al. (2013). Medial temporal lobe function during emotional memory in early Alzheimer's disease, mild cognitive impairment and healthy ageing: an fMRI study. BMC Psychiatry 13:76. doi: 10.1186/1471-244X-13-76
*Petrella, J. R., Sheldon, F. C., Prince, S. E., Calhoun, V. D., and Doraiswamy, P. M. (2011). Default mode network connectivity in stable vs progressive mild cognitive impairment. Neurology 76, 511–517. doi: 10.1212/WNL.0b013e31820af94e
*Pihlajamäki, M., and Sperling, R. (2009). Functional MRI assessment of task-induced deactivation of the default mode network in Alzheimer's disease and at-risk older individuals. Behav. Neurol. 21, 77–91. doi: 10.1155/2009/276384
*Poettrich, K., Weiss, P. H., Werner, A., Lux, S., Donix, M., Gerber, J., et al. (2009). Altered neural network supporting declarative long-term memory in mild cognitive impairment. Neurobiol. Aging 30, 284–298. doi: 10.1016/j.neurobiolaging.2007.05.027
Poldrack, R. A., Fletcher, P. C., Henson, R. N., Worsley, K. J., Brett, M., and Nichols, T. E. (2008). Guidelines for reporting an fMRI study. Neuroimage 40, 409–414. doi: 10.1016/j.neuroimage.2007.11.048
*Protzner, A. B., Mandzia, J. L., Black, S. E., and McAndrews, M. P. (2011). Network interactions explain effective encoding in the context of medial temporal damage in MCI. Hum. Brain Mapp. 32, 1277–1289. doi: 10.1002/hbm.21107
*Puente, A. N., Faraco, C., Terry, D. P., Brown, C., and Miller, L. S. (2014). Minimal functional brain differences between older adults with and without mild cognitive impairment during the stroop. Neuropsychol. Dev. Cogn. B Aging Neuropsychol. Cogn. 21, 346–369. doi: 10.1080/13825585.2013.824065
*Qi, Z., Wu, X., Wang, Z., Zhang, N., Dong, H., Yao, L., et al. (2010). Impairment and compensation coexist in amnestic MCI default mode network. Neuroimage 50, 48–55. doi: 10.1016/j.neuroimage.2009.12.025
*Rombouts, S. A., Barkhof, F., Goekoop, R., Stam, C. J., and Scheltens, P. (2005). Altered resting state networks in mild cognitive impairment and mild Alzheimer's disease: an fMRI study. Hum. Brain Mapp. 26, 231–239. doi: 10.1002/hbm.20160
Sandry, J., and Sumowski, J. F. (2014). Working memory mediates the relationship between intellectual enrichment and long-term memory in multiple sclerosis: an exploratory analysis of cognitive reserve. J. Int. Neuropsychol. Soc. 20, 868–872. doi: 10.1017/S1355617714000630
*Sala-Llonch, R., Bosch, B., Arenaza-Urquijo, E. M., Rami, L., Bargalló, N., Junqué, C., et al. (2010). Greater default-mode network abnormalities compared to high order visual processing systems in amnestic mild cognitive impairment: an integrated multi-modal MRI study. J. Alzheimers Dis. 22, 523–539. doi: 10.3233/JAD-2010-101038
Schölvinck, M. L., Maier, A., Ye, F. Q., Duyn, J. H., and Leopold, D. A. (2010). Neural basis of global resting-state fMRI activity. Proc. Natl. Acad. Sci. U.S.A. 107, 10238–10243. doi: 10.1073/pnas.0913110107
*Smith, J. C., Nielson, K. A., Antuono, P., Lyons, J.-A., Hanson, R. J., Butts, A. M., et al. (2013). Semantic memory functional MRI and cognitive function after exercise intervention in mild cognitive impairment. J. Alzheimers Dis. 37, 197–215. doi: 10.3233/JAD-130467
*Solé-Padullés, C., Bartrés-Faz, D., Junqué, C., Vendrell, P., Rami, L., Clemente, I. C., et al. (2009). Brain structure and function related to cognitive reserve variables in normal aging, mild cognitive impairment and Alzheimer's disease. Neurobiol. Aging 30, 1114–1124. doi: 10.1016/j.neurobiolaging.2007.10.008
*Sperling, R. (2007). Functional MRI studies of associative encoding in normal aging, mild cognitive impairment, and Alzheimer's disease. Ann. N.Y. Acad. Sci. 1097, 146–155. doi: 10.1196/annals.1379.009
*Staffen, W., Ladurner, G., Höller, Y., Bergmann, J., Aichhorn, M., Golaszewski, S., et al. (2012). Brain activation disturbance for target detection in patients with mild cognitive impairment: an fMRI study. Neurobiol. Aging 33, 1002, e1–e16. doi: 10.1016/j.neurobiolaging.2011.09.002
Sumowski, J. F., Rocca, M. A., Leavitt, V. M., Dackovic, J., Mesaros, S., Drulovic, J., et al. (2014). Brain reserve and cognitive reserve protect against cognitive decline over 4.5 years in MS. Neurology 82, 1776–1783. doi: 10.1212/WNL.0000000000000433
Symms, M., Jäger, H. R., Schmierer, K., and Yousry, T. (2004). A review of structural magnetic resonance neuroimaging. J. Neurol. Neurosurg. Psychiatr. 75, 1235–1244. doi: 10.1136/jnnp.2003.032714
*Teipel, S. J., Bokde, A. L. W., Born, C., Meindl, T., Reiser, M., Möller, H.-J., et al. (2007). Morphological substrate of face matching in healthy ageing and mild cognitive impairment: a combined MRI-fMRI study. Brain 130(Pt 7), 1745–1758. doi: 10.1093/brain/awm117
*Trivedi, M. A., Murphy, C. M., Goetz, C., Shah, R. C., Gabrieli, J. D. E., Whitfield-Gabrieli, S., et al. (2008). fMRI activation changes during successful episodic memory encoding and recognition in amnestic mild cognitive impairment relative to cognitively healthy older adults. Dement. Geriatr. Cogn. Disord. 26, 123–137. doi: 10.1159/000148190
*Vannini, P., Almkvist, O., Dierks, T., Lehmann, C., and Wahlund, L.-O. (2007). Reduced neuronal efficacy in progressive mild cognitive impairment: a prospective fMRI study on visuospatial processing. Psychiatry Res. 156, 43–57. doi: 10.1016/j.pscychresns.2007.02.003
*Wang, K., Liang, M., Wang, L., Tian, L., Zhang, X., Li, K., et al. (2007). Altered functional connectivity in early Alzheimer's disease: a resting-state fMRI study. Hum. Brain Mapp. 28, 967–978. doi: 10.1002/hbm.20324
*Wang, Y., Risacher, S. L., West, J. D., McDonald, B. C., Magee, T. R., Farlow, M. R., et al. (2013). Altered default mode network connectivity in older adults with cognitive complaints and amnestic mild cognitive impairment. J. Alzheimers Dis. 35, 751–760. doi: 10.3233/JAD-130080
*Wang, Z., Liang, P., Jia, X., Jin, G., Song, H., Han, Y., et al. (2012). The baseline and longitudinal changes of PCC connectivity in mild cognitive impairment: a combined structure and resting-state fMRI study. PLoS ONE 7:e36838. doi: 10.1371/journal.pone.0036838
*Wang, Z., Liang, P., Jia, X., Qi, Z., Yu, L., Yang, Y., et al. (2011). Baseline and longitudinal patterns of hippocampal connectivity in mild cognitive impairment: evidence from resting state fMRI. J. Neurol. Sci. 309, 79–85. doi: 10.1016/j.jns.2011.07.017
*Wee, C. Y., Yap, P. T., Zhang, D., Wang, L., and Shen, D. (2014). Group-constrained sparse fMRI connectivity modeling for mild cognitive impairment identification. Brain Struct. Funct. 219, 641–656. doi: 10.1007/s00429-013-0524-8
*Wee, C.-Y., Yap, P.-T., Zhang, D., Denny, K., Browndyke, J. N., Potter, G. G., et al. (2012). Identification of MCI individuals using structural and functional connectivity networks. Neuroimage 59, 2045–2056. doi: 10.1016/j.neuroimage.2011.10.015
*Woodard, J. L., Seidenberg, M., Nielson, K. A., Antuono, P., Guidotti, L., Durgerian, S., et al. (2009). Semantic memory activation in amnestic mild cognitive impairment. Brain 132(Pt 8), 2068–2078. doi: 10.1093/brain/awp157
*Yao, H., Liu, Y., Zhou, B., Zhang, Z., An, N., Wang, P., et al. (2013). Decreased functional connectivity of the amygdala in Alzheimer's disease revealed by resting-state fMRI. Eur. J. Radiol. 82, 1531–1538. doi: 10.1016/j.ejrad.2013.03.019
*Yao, H., Zhou, B., Zhang, Z., Wang, P., Guo, Y., Shang, Y., et al. (2014). Longitudinal alteration of amygdalar functional connectivity in mild cognitive impairment subjects revealed by resting-state FMRI. Brain Connect. 4, 361–370. doi: 10.1089/brain.2014.0223
*Yassa, M. A., Stark, S. M., Bakker, A., Albert, M. S., Gallagher, M., and Stark, C. E. L. (2010). High-resolution structural and functional MRI of hippocampal CA3 and dentate gyrus in patients with amnestic mild cognitive impairment. Neuroimage 51, 1242–1252. doi: 10.1016/j.neuroimage.2010.03.040
*Zamboni, G., Drazich, E., McCulloch, E., Filippini, N., Mackay, C. E., Jenkinson, M., et al. (2013). Neuroanatomy of impaired self-awareness in Alzheimer's disease and mild cognitive impairment. Cortex 49, 668–678. doi: 10.1016/j.cortex.2012.04.011
*Zanto, T. P., Pa, J., and Gazzaley, A. (2014). Reliability measures of functional magnetic resonance imaging in a longitudinal evaluation of mild cognitive impairment. Neuroimage 84, 443–452. doi: 10.1016/j.neuroimage.2013.08.063
*Zhang, Z., Liu, Y., Jiang, T., Zhou, B., An, N., Dai, H., et al. (2012). Altered spontaneus activity in Alzheimer's disease and mild cognitive impairment revealed by regional homogeneity. Neuroimage 59, 1429–1440. doi: 10.1016/j.neuroimage.2011.08.049
*Zhou, X., Zhang, J., Chen, Y., Ma, T., Wang, Y., Wang, J., et al. (2014). Aggravated cognitive and brain functional impairment in mild cognitive impairment patients with type 2 diabetes: a resting-state functional MRI study. J. Alzheimers Dis. 41, 925–935. doi: 10.3233/JAD-132354
*Zhou, Y., Dougherty, J. H., Hubner, K. F., Bai, B., Cannon, R. L., and Hutson, R. K. (2008). Abnormal connectivity in the posterior cingulate and hippocampus in early Alzheimer's disease and mild cognitive impairment. Alzheimers Dement. 4, 265–270. doi: 10.1016/j.jalz.2008.04.006
*Zhou, Y., Yu, F., and Duong, T. Q. (2013). White Matter Lesion Load is associated with resting state functional MRI activity and amyloid PET but not FDG in mild cognitive impairment and early Alzheimer's disease patients. J. Magn. Reson. Imaging 1–8. doi: 10.1002/jmri.24550
Zhou, Z., Ding, M., Chen, Y., Wright, P., Lu, Z., and Liu, Y. (2009). Detecting directional influence in fMRI connectivity analysis using PCA based Granger causality. Brain Res. 1289, 22–29. doi: 10.1016/j.brainres.2009.06.096
Keywords: mild cognitive impairment, fMRI, connectivity, statistical analysis, review
Citation: Farràs-Permanyer L, Guàrdia-Olmos J and Peró-Cebollero M (2015) Mild cognitive impairment and fMRI studies of brain functional connectivity: the state of the art. Front. Psychol. 6:1095. doi: 10.3389/fpsyg.2015.01095
Received: 12 March 2015; Accepted: 16 July 2015;
Published: 04 August 2015.
Edited by:
Pietro Cipresso, IRCCS Istituto Auxologico Italiano, ItalyReviewed by:
Krishna P. Miyapuram, Indian Institute of Technology Gandhinagar, IndiaArun Bokde, Trinity College Dublin, Ireland
Copyright © 2015 Farràs-Permanyer, Guàrdia-Olmos and Peró-Cebollero. This is an open-access article distributed under the terms of the Creative Commons Attribution License (CC BY). The use, distribution or reproduction in other forums is permitted, provided the original author(s) or licensor are credited and that the original publication in this journal is cited, in accordance with accepted academic practice. No use, distribution or reproduction is permitted which does not comply with these terms.
*Correspondence: Laia Farràs-Permanyer, Facultat de Psicologia, Universitat de Barcelona, Passeig de la Valld'Hebron, 171, 08035 Barcelona, Spain, laiafarras@ub.edu