- 1SoundBrain Lab, Department of Communication Sciences and Disorders, The University of Texas at Austin, Austin, TX, USA
- 2Institute for Mental Health Research, The University of Texas at Austin, Austin, TX, USA
- 3Institute for Neuroscience, The University of Texas at Austin, Austin, TX, USA
- 4Department of Psychology, The University of Texas at Austin, Austin, TX, USA
More than two decades of work in vision posits the existence of dual-learning systems of category learning. The reflective system uses working memory to develop and test rules for classifying in an explicit fashion, while the reflexive system operates by implicitly associating perception with actions that lead to reinforcement. Dual-learning systems models hypothesize that in learning natural categories, learners initially use the reflective system and, with practice, transfer control to the reflexive system. The role of reflective and reflexive systems in auditory category learning and more specifically in speech category learning has not been systematically examined. In this article, we describe a neurobiologically constrained dual-learning systems theoretical framework that is currently being developed in speech category learning and review recent applications of this framework. Using behavioral and computational modeling approaches, we provide evidence that speech category learning is predominantly mediated by the reflexive learning system. In one application, we explore the effects of normal aging on non-speech and speech category learning. Prominently, we find a large age-related deficit in speech learning. The computational modeling suggests that older adults are less likely to transition from simple, reflective, unidimensional rules to more complex, reflexive, multi-dimensional rules. In a second application, we summarize a recent study examining auditory category learning in individuals with elevated depressive symptoms. We find a deficit in reflective-optimal and an enhancement in reflexive-optimal auditory category learning. Interestingly, individuals with elevated depressive symptoms also show an advantage in learning speech categories. We end with a brief summary and description of a number of future directions.
Introduction
Fast and accurate categorization is fundamental to the survival of all organisms. The rabbit must categorize a sound as “friend,” “foe,” or a “gust of wind” to determine whether to approach, run, or continue with the current behavior. The Emergency Medical Technician (EMT) must categorize the ausculatory lung sounds heard through a stethoscope as indicative of “fluid” or “no fluid” when determining whether to conduct additional tests or inform the patient that their lungs are clear. The umpire in cricket must decide if a batsman is “out” or “not out” after weighing auditory and visual evidence. These are all categorization problems because there are many information states but only a small number of courses of action.
The psychological study of category learning is long and rich (Bruner et al., 1956; Smith and Medin, 1981; Estes, 1994; Ashby and Maddox, 2005, 2010). Early research focused on single-system models, whereas recent research focuses on multiple-systems approaches. Surprisingly, nearly all of this work focused on the visual domain with little examination of other modalities, including audition. The overriding aim of this paper is to describe a dual-learning systems theoretical framework that is currently being developed in the auditory domain. We attempt to provide a theoretical scaffolding to the emerging field of auditory cognitive science (Holt and Lotto, 2008). In the next sections, we provide a brief history of category learning research starting with single-system approaches and ending with a neurobiologically inspired dual-learning systems approach. We then examine the extent to which the dual-learning systems approach is neurobiologically viable in the auditory domain. Finally, we develop the dual-learning systems framework to speech category learning. Speech category learning involves the mapping of highly variable acoustic cues to perceptual space, akin to a specific type of categorization problem (Holt and Lotto, 2010). However, thus far, speech category learning has been largely viewed as a perceptually encapsulated process. For example, a rich body of literature has examined categorical perception (Liberman et al., 1967; Kuhl, 1994, 2004). Categorical perception refers to the percept of invariant categories in sensory events that are discrete and along a continuum. Early studies argued that categorical perception is specific to speech and humans (Liberman et al., 1967). Later studies, however, unequivocally demonstrated that categorical perception extends to other non-speech modalities and exists in non-human species (Kuhl and Miller, 1978; Kuhl, 1985). While the focus on understanding the phenomena of categorical perception still continues (Goldstone and Hendrickson, 2010; Fleming et al., 2013), more recent efforts in the speech sciences have argued the need to study speech perception as a categorization problem (Holt and Lotto, 2010), rather than simply a perceptual problem. In contrast to the auditory domain, a rich prior literature exists in the study of categorization. A goal therefore is to extend the rich theoretical understanding of domain-general learning processes involved in visual category learning literature to speech learning. We conclude with a brief summary and a description of a number of exciting lines of future research.
Single System vs. Multiple Systems of Category Learning
Category learning has an extensive history in psychology (Bruner et al., 1956; Smith and Medin, 1981; Nosofsky, 1986b; Estes, 1994; Ashby and Maddox, 2005, 2010). Until the early 1990s, the focus was on developing and testing single-system models of category learning. Three classes of single-system models with multiple instantiations of each were popular during this era: prototype, exemplar, and decision-bound models. Prototype models assume that when asked to assign a stimulus to one of several categories, the participant responds with the category label associated with the most similar prototype (Reed, 1972; Rosch, 1977; Homa et al., 1981; Posner and Petersen, 1990; Smith and Minda, 1998). Exemplar models assume that when asked to assign a stimulus to one of several categories, the participant performs a global match between the representation of the presented stimulus and the memory representation of every exemplar from each contrasting category, selecting the category label associated with the strongest global match (Medin and Schaffer, 1978; Estes, 1986; Hintzman, 1986; Nosofsky, 1986a; Estes, 1994). Decision-bound models assume that the participant learns to assign responses to regions of the perceptual space, and when asked to assign a stimulus to one of several categories, the participant determines into which region the stimulus representation falls and emits the associated response (Ashby and Townsend, 1986; Ashby and Perrin, 1988; Ashby, 1992; Ashby and Maddox, 1993; Maddox and Ashby, 1993). The approach taken by many category learning researchers during this time was to conduct a category learning study and to apply competing models to the data with the aim of identifying the model that provided the best account of the data; the implication being that this “best fitting” model was the correct model (Maddox and Ashby, 1993; McKinley and Nosofsky, 1995; Smith and Minda, 1998). Although a dominant and sometimes fruitful approach, three critical observations cast doubt on this as a viable long-term scientific approach to the study of category learning.
First, research emerged that suggested that many category learning models were mathematically equivalent (Nosofsky, 1990, 1991; Ashby and Maddox, 1993). For example, Ashby and Maddox (1993) (see also Nosofsky, 1990, 1991) showed that prototype, exemplar, and decision-bound models are mathematically equivalent under a broad range of environmental contexts. Thus, in spite of the large differences in psychological processing assumptions across these three classes of models, the models are often equivalent at the level of the data.
Second, a number of results suggested that human category learning is mediated by multiple category-learning systems (Nosofsky et al., 1994; Ashby et al., 1998; Erickson and Kruschke, 1998; Reber et al., 2003; Love et al., 2004; Ashby and O’Brien, 2005). One of the strongest pieces of evidence comes from an examination of both of the category structures in Figure 1, and the learning profiles associated with each category structure. The stimuli represented in Figure 1B were constructed by rotating the items in Figure 1A by 45°. Thus, the two spaces are mathematically equivalent and would be learned to equivalent levels by any standard clustering algorithm. Despite this equivalence, humans show very different learning profiles and introspection when asked to solve these tasks. When faced with the task depicted in Figure 1A, participants start out near chance and then at some point “get it” and perform nearly optimal. In other words, participants’ learning profile is characterized as a step function. In addition, participants are able to describe the strategy that they used accurately. When faced with the task depicted in Figure 1B, participants start out at near chance and then show gradual, incremental learning. Participants are unable to describe the strategy that they used accurately and often say that they went with their “gut” feeling, or “gut reflex.”
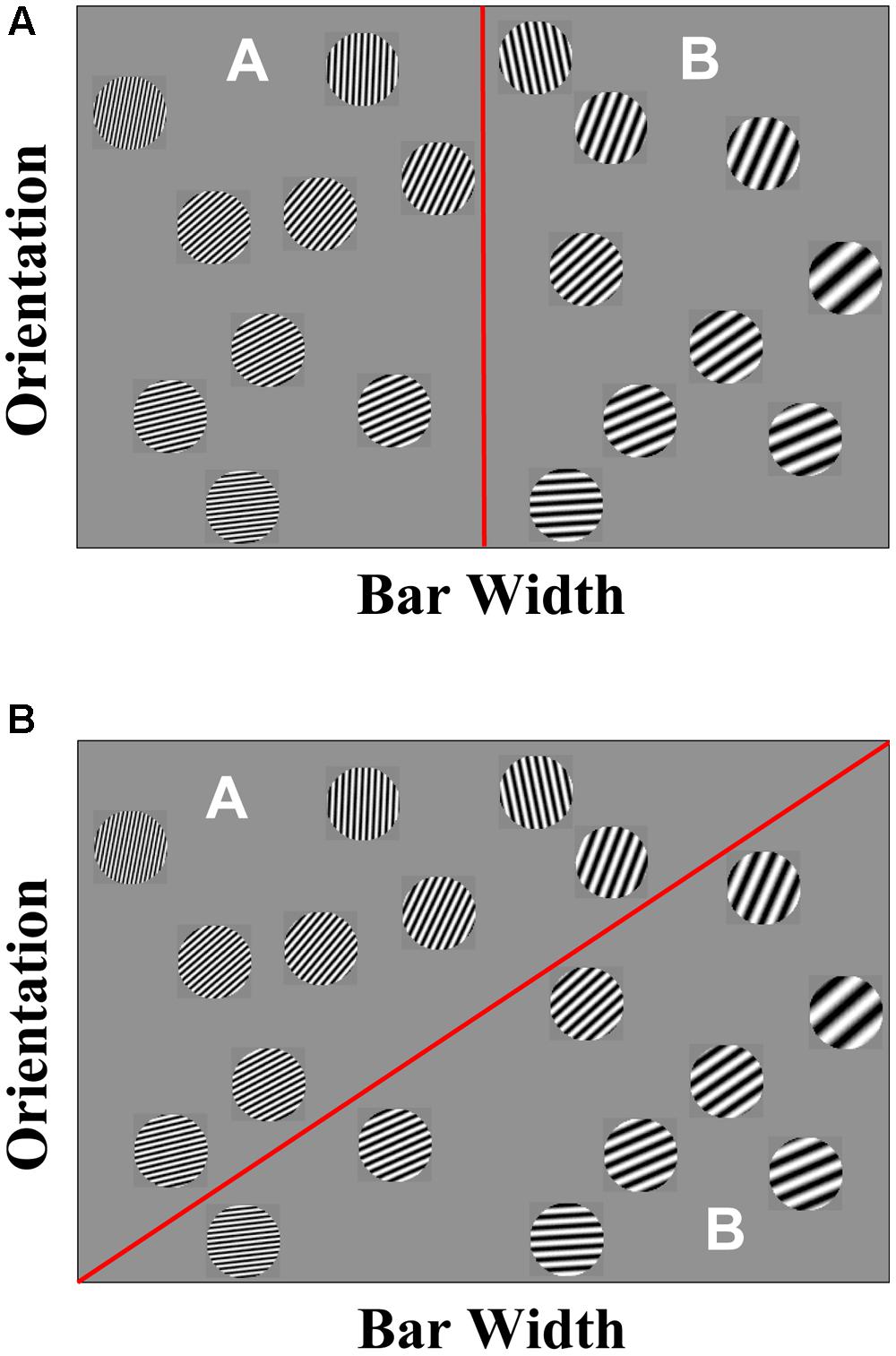
FIGURE 1. (A) Example of a rule-based category learning task in which narrow bar width Gabor patches are in category A and wide bar width Gabor patches are in category B. (B) Example of an information-integration category learning task in which no verbalizable rule can be used to describe the strategy that maximizes accuracy.
This qualitative difference in performance across these structurally equivalent categories led to a number of interesting studies that revealed strong empirical dissociations between the learning of these two category structures. Because single-system models are unable to account simultaneously for more than one or two of these multiple-system results, the field began to question the viability of single-system approaches. Brooks and colleagues suggested one of the earliest multiple-systems approaches, arguing for separate rule-based (RB) and exemplar-based systems (Brooks, 1978; Allen and Brooks, 1991; Regehr and Brooks, 1993). Since then, a number of purely cognitive multiple-systems models have been proposed, with nearly all offering some specific instantiation of Brooks’ RB and exemplar-based systems (Nosofsky et al., 1994; Erickson and Kruschke, 1998; Love et al., 2004).
Finally, a plethora of research examining the neural basis of category learning emerged (Poldrack and Packard, 2003; Nomura et al., 2007). The existence of the neural data weakens the predictive power of the purely cognitive models since they are ambivalent with respect to neuroscience. This revolution opened the door to a number of new methodological approaches.
A Neurobiologically Based Dual-Learning Systems Model (COVIS)
One of the theories of category learning that specifies the constraints imposed by the underlying neurobiology is the COmpetition between Verbal and Implicit Systems (COVIS; Ashby et al., 1998, 2011) model. As we later elaborate, COVIS focuses exclusively on the visual domain. COVIS postulates two learning systems, one reflective and one reflexive1. The reflective system is an explicit learning system in the sense that it formulates and tests specific categorization rules using executive attention and working memory. The critical neural structures include prefrontal cortex, anterior cingulate, and anterior caudate nucleus (Lombardi et al., 1999; Monchi et al., 2001; Ashby and Valentin, 2005; Ashby et al., 2005; Filoteo et al., 2005c; Seger and Cincotta, 2006; Nomura et al., 2007; Schnyer et al., 2009). Figure 1A displays a simple two-category, RB problem using Gabor patches that vary in spatial frequency and spatial orientation as stimuli.
The strategy that maximizes accuracy is to place low spatial frequency items into category A and high spatial frequency items into category B. This strategy is referred to as an RB or reflective strategy. In contrast, the reflexive system is implicit and procedural and learns to associate stimuli lying in different regions of perceptual space with specific motor outputs as a result of reinforcement via trial feedback. Accurate performance in reflexive categorization requires predecisional integration of stimulus components, and it is therefore often referred to as an information-integration (II) strategy. Learning in this system does not rely on working memory and executive attention, and the critical structures are the posterior caudate, putamen and the supplementary motor area (SMA; Ashby and Waldron, 1999; Maddox and Filoteo, 2001; Poldrack et al., 2001; Aron et al., 2004; Filoteo et al., 2005b; Maddox and Filoteo, 2005; Seger and Cincotta, 2005; Nomura et al., 2007; Seger, 2008; Ashby and Crossley, 2011). Figure 1B displays a simple two-category problem. The strategy that maximizes accuracy in Figure 1B (unlike the structure in Figure 1A) is not easily verbalizable, so an II strategy implemented via the reflexive system is most optimal for categorizing these stimuli.
The COVIS model assumes that the reflective and reflexive learning systems compete throughout category learning. In humans, there appears to be a bias toward reflective dominance. Individuals explicitly test category rules and adjust the weight given to that rule depending on its success or failure. The success or failure of rules is assessed by explicit processing of the feedback. After each trial, utility of a particular rule is updated. Through this method of hypothesis testing, relevant decision bounds are learned. The explicit nature of the reflective system requires use of working memory and executive attention to remember which rules have been used, to process the success or failure of these decision bounds, and to switch between rules. COVIS posits that an accurate reflective system prevents the transfer of control to the striatally mediated reflexive system (Ashby and Maddox, 2010). Learners will therefore continue to use reflective system until the reflexive system is more accurate.
In comparison, during reflexive learning, a striatal unit implicitly associates an abstract cortical–motor response with sensory cells in the sensory association cortex. Learning occurs at cortical–striatal synapses. Such synaptic plasticity is enhanced by a dopamine-mediated reinforcement signal. The timing and nature of feedback in a categorization experiment are crucial to the effectiveness of the reflexive learning system, while working memory is not critical to learning. Despite the different circuitries, both the reflective and reflexive learning systems utilize components within the primary and association sensory regions. For further details, the reader is referred to previous review papers on the COVIS model (Ashby and Maddox, 2010; Ashby et al., 2011). See Table 1 for a summary of properties of the reflective and reflexive systems.
The dual-learning systems approach in general, and COVIS in particular, has gained broad support with evidence from behavioral studies conducted in a variety of areas. These include: healthy adult humans (Ashby and Maddox, 2005, 2010; Grimm and Maddox, 2013; Ashby, 2014; Smith et al., 2014), human children, and older adults (Ridderinkhof et al., 2002; Filoteo and Maddox, 2004; Filoteo et al., 2005a; Racine et al., 2006; Minda et al., 2008; Maddox et al., 2010; Huang-Pollock et al., 2011; Gorlick et al., 2012), non-human animals (Smith et al., 2004, 2010, 2011, 2012a,b), various neuropsychological patient groups (Knowlton and Squire, 1993; Knowlton et al., 1994; Squire and Knowlton, 1995; Knowlton, 1999; Keri, 2003; Filoteo et al., 2005b; Filoteo and Maddox, 2007), as well as using brain imaging techniques such as fMRI (Poldrack et al., 1999, 2001; Cincotta and Seger, 2000, 2007; Poldrack and Packard, 2003; Aron et al., 2004; Poldrack and Rodriguez, 2004; Shohamy et al., 2004; Seger and Cincotta, 2005; Seger and Cincotta, 2006; Nomura et al., 2007; Nomura and Reber, 2008; Seger, 2008; Helie et al., 2010; Seger and Miller, 2010; Waldschmidt and Ashby, 2011) and EEG (Folstein and Van Petten, 2004).
Toward an Auditory Version of COVIS
Neuroanatomy
A major focus of this article is to examine the application of the dual-learning systems model to the auditory domain. Previous studies have shown similarities in the organization of the two major sensory domains. In vision, an organizing principle is retinotopy; in audition, topographical organization by frequency (“tonotopy”) has been demonstrated along the auditory pathway. Functionally distinct dorsal and ventral cortical streams are seen both in vision and audition (Romanski et al., 1999; Marois et al., 2000; Rauschecker and Scott, 2009). However, there are some critical differences between the two domains as well. A significant amount of auditory signal processing occurs well before signals reach the auditory midbrain. The visual pathway lacks functional processing centers at the level of the brainstem. The auditory system is subserved by massive efferent (feedback) connectivity that yields substantial top-down control of the lower level auditory centers. In contrast, the efferent connectivity of the visual system is less massive. Functionally, the auditory system is constantly “on” (even when we are asleep) and therefore metabolically more expensive. In monkeys, auditory working memory is less robust and more susceptible to “rewriting” than visual working memory (Scott et al., 2012). In humans, there is a marked difference in recognition memory for visual and auditory objects. The memory for visual images is far greater than for auditory objects (Cohen et al., 2009). Despite these differences, a direct comparison of the two modalities has been challenging due to methodological difficulties in matching the sensory and cognitive load imposed by auditory and visual stimuli. A recent behavioral and computational modeling study matched auditory and visual stimuli on stimulus complexity (static or moving gabor patches vs. moving ripple stimuli) and showed processing similarities between the two modalities in a short-term memory task (Visscher et al., 2007). This study suggests that memory processes are not modality specific. Given inconsistent findings about commonalities/differences between audition and vision, an important question is whether the neural circuitry underlying the dual-learning systems has a parallel in the auditory domain.
The bidirectional connectivity among primary, secondary auditory cortices, and the prefrontal cortex is well established (Rauschecker and Scott, 2009). This connectivity forms a clear basis for a functional reflective auditory system. In contrast, relatively little is known about the functional role of the corticostriatal connectivity in audition. In the next few paragraphs, we review the existing work from animal and human models that argue for a reflexive auditory system. Retrograde tracing experiments in animal models show direct connectivity from the auditory thalamus and auditory cortex to the striatum (LeDoux et al., 1991) In cats, auditory cortical projections to the striatum is tonotopic (Reale and Imig, 1983). Retrograde anatomical labeling studies in primates show that the primary and association auditory cortices are bi-directionally connected to the dorsolateral prefrontal cortex and form many-to-one projections to the striatum (Petrides and Pandya, 1988; Yeterian and Pandya, 1998; Figure 2).
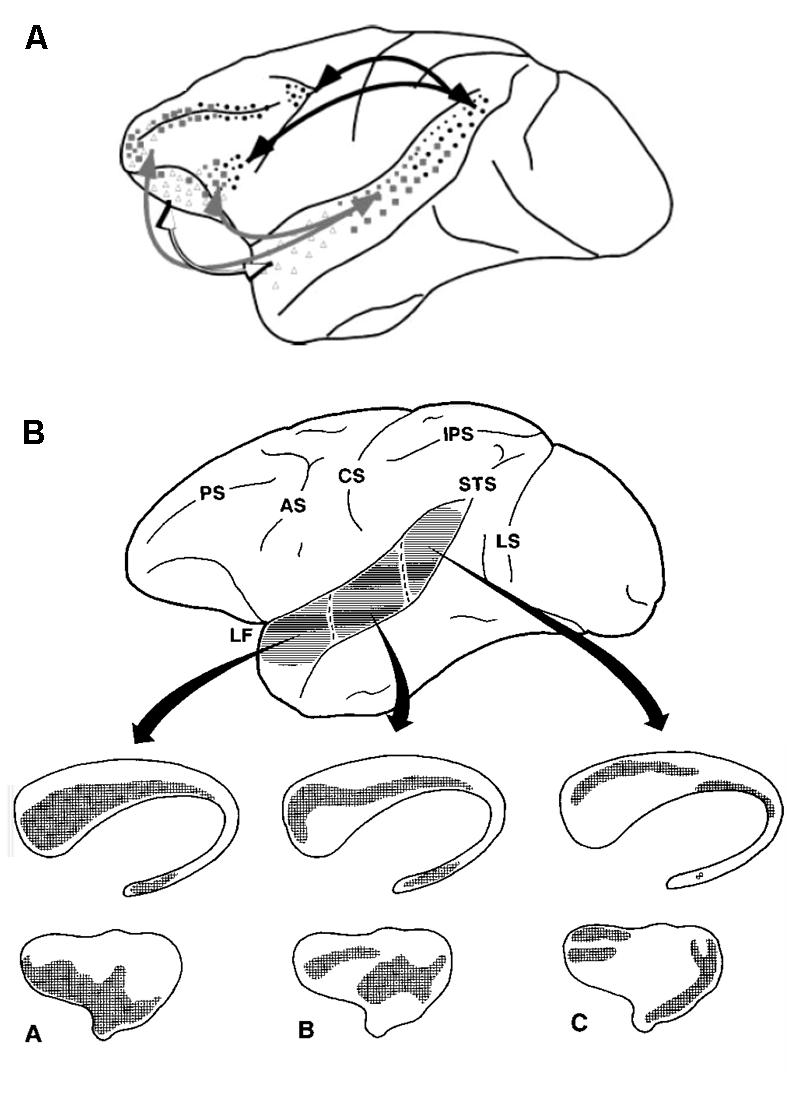
FIGURE 2. Neuroanatomy in support of the (A) reflective, and (B) reflexive auditory category learning systems. Primary and secondary auditory cortices are directly connected to the reflective (A) and reflexive (B) learning systems. Adapted from Petrides and Pandya (1988) and Yeterian and Pandya (1998).
The connections from the primary auditory cortex to the striatum are relatively sparse. In contrast, connections from the belt region, which surrounds the primary auditory cortex, to the caudate and putamen are more dense (Yeterian and Pandya, 1998). Examining responsivity in the striatum to auditory stimulation using c-fos induction, Arnauld et al. (1996) showed dense Fos-IR within the caudal striatum, and relatively sparse labeling in the rostral striatum. This is in contrast to visual stimulation, which resulted in Fos-IR within the rostral striatum (Arnauld et al., 1996). Despite retrograde labeling studies showing diffuse corticostriatal connectivity patterns, the projections from the auditory system largely converge on to the caudal portion of the striatum (Arnauld et al., 1996). While the previous studies have all examined the corticostriatal projection, there is some evidence for a backprojection from the striatum to the auditory cortex via the pallidum. The functional role of this backprojection is unclear (Parent and Hazrati, 1995). From a functional perspective, a recent study showed that decisions on auditory stimuli are functionally determined by corticostriatal connections in rats. Optogenetic stimulation of the corticostriatal neurons biased the animal’s choice (Znamenskiy and Zador, 2013). In humans, a resting-state connectivity study demonstrated functional connectivity between the putamen and the auditory association area. Connectivity is more robust between the auditory cortex and the putamen relative to the caudate (Di Martino et al., 2008).
Despite the fundamental differences between auditory and visual perception, the brain regions associated with auditory processing are interconnected with the brain regions associated with reflective and reflexive category learning. This connectivity is a good indication that the neurobiology associated with the COVIS model is plausible in both the auditory and visual domains. We next need to determine whether processing in these auditory analogs of reflective and reflexive category learning systems behave in a manner similar to those associated with reflective and reflexive visual category learning. Ultimately, we should approach this with all of the same tools that have been used in the visual domain. This includes behavioral dissociation studies, lifespan research, brain imaging techniques (fMRI, EEG), and neuropsychological patient groups. Our group has made headway using some of these approaches and that work will be reviewed here.
Reflective and Reflexive Auditory Learning Systems
Now that we have established that the neurobiology is in place to support a dual-learning systems approach to auditory category learning, we review the empirical evidence in support of dual-learning systems using auditory category learning tasks. The most rigorous tests of dual-learning systems require the use of artificial categories for which the experimenter controls the optimal strategy and constructs one reflective-optimal and one reflexive-optimal task. Figure 3A displays a highly verbalizable reflective-optimal category learning problem that uses tones that vary in duration and frequency as stimuli: short, low-frequency tones are in category A; short, high-frequency tones are in category B; long, low-frequency tones are in category C; and long, high-frequency tones are in category D. In our pilot experiments, learners were able to easily verbalize their strategies for the four categories. The broken lines denote the decision boundaries that maximize accuracy.
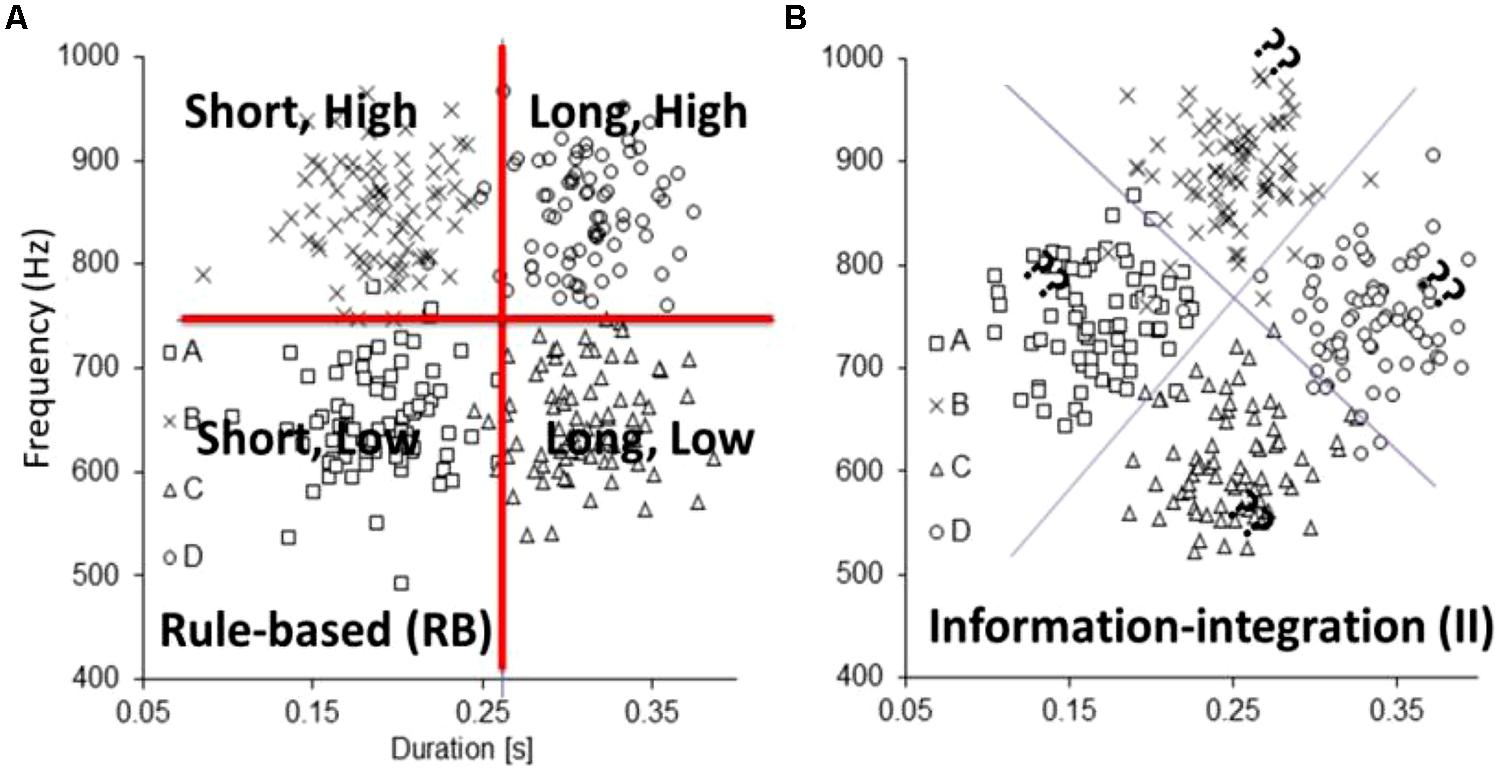
FIGURE 3. Artificial category structures: (A) rule-based, reflective-optimal and (B) information-integration, reflexive-optimal used to study dissociations between reflective and reflexive auditory category learning systems.
Figure 3B displays a reflexive-optimal category learning problem that is constructed by rotating the Figure 3A stimulus space by 45°. The broken lines denote the decision boundaries that maximize accuracy. In this case, no simple verbal description exists to describe this strategy. As a proof of concept, we examined reflective-optimal and reflexive-optimal category learning in the visual domain and compared it with reflective-optimal and reflexive-optimal category learning in the auditory domain. Importantly, the category structures remained the same across the visual and auditory applications; only the specific dimensions changed. Participants showed similar learning profiles across the visual and auditory versions of the reflective-optimal and reflexive-optimal tasks, suggesting that similar mechanisms were in place. As a more rigorous test of the dual-learning systems approach, we examined whether individual differences in working memory capacity were predictive of individual differences in reflective-optimal and reflexive-optimal non-speech auditory category learning. Two lines of work in the visual domain suggest that this should matter. First, a number of researchers (Waldron and Ashby, 2001; Maddox et al., 2004; Zeithamova and Maddox, 2006, 2007; Filoteo et al., 2010) have shown that reflective-optimal visual category learning was impaired when participants were asked to perform a demanding working-memory dual task, whereas reflexive-optimal visual category learning was not affected. Second, and more directly (DeCaro et al., 2008; Tharp and Pickering, 2009; however, see Lewandowsky et al., 2012) showed that increases in working memory capacity were associated with enhanced reflective-optimal visual category learning but did not lead to advantages in reflexive-optimal visual category learning.
We tested this latter result directly in non-speech auditory reflective-optimal and reflexive-optimal category learning. Again, the hypothesis was that working memory would be significantly related to reflective but not reflexive processing. We had 28 young adults (18–35 years) complete the Figure 3A reflective-optimal non-speech auditory category learning task, and 30 young adults (18–35 years) complete Figure 3B reflexive-optimal non-speech auditory category learning task. Working memory capacity was assessed using the digit span portion of the Wechsler Adult Intelligence Scale, 4th edition (WAIS-IV; Wechsler, 2008). In the backward span task, numbers were read at a rate of one number per second with a monotone voice to avoid highlighting any one part of the string of numbers. Participants were required to repeat the string of numbers presented to them backwards and were scored on the sum of correct strings correctly repeated. In the forward span task, participants were required to repeat strings of numbers presented to them and were scored on the sum of strings correctly repeated. A composite span was created by adding the forward and backward spans for each participant. Figures 4A,B display scatterplots of the working memory capacity and reflective-optimal (Figure 4A) or reflexive-optimal (Figure 4B) scores.
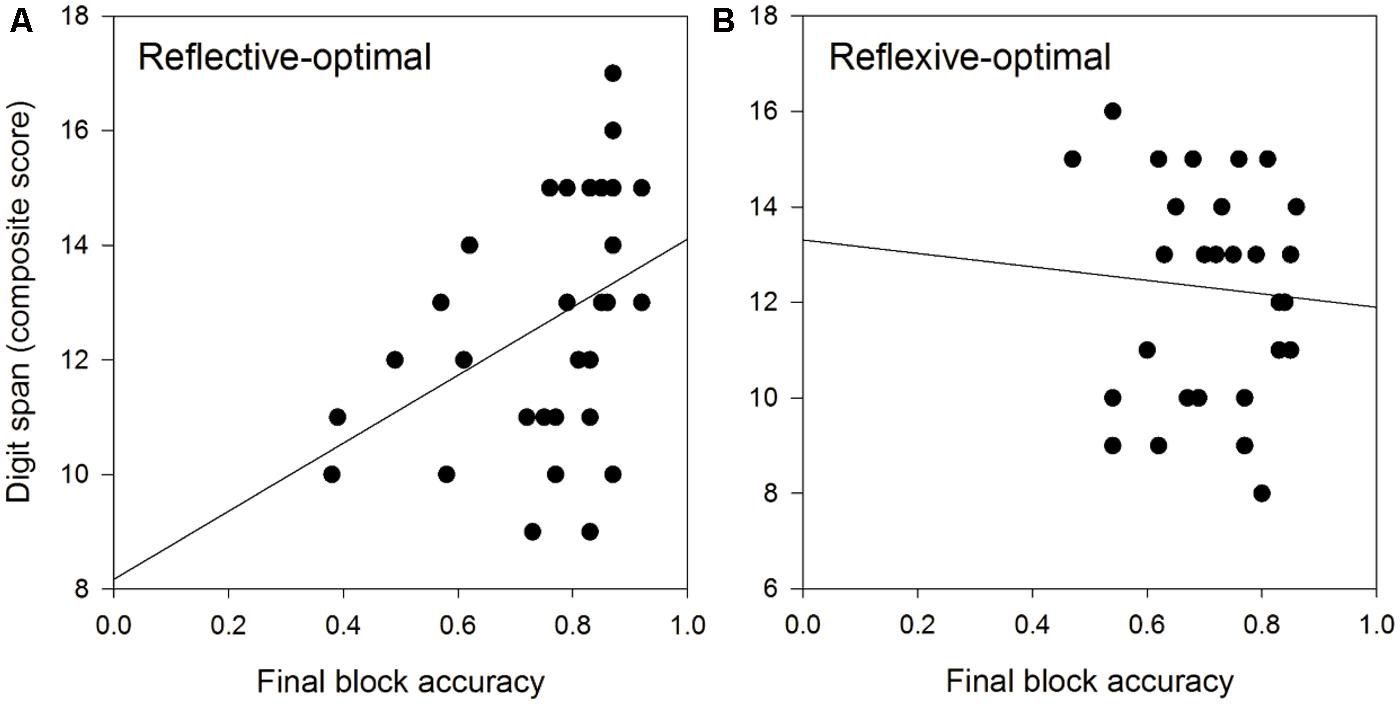
FIGURE 4. (A) Rule-based, reflective-optimal auditory category learning is positively related to working memory span. (B) Information-integration, reflexive-optimal auditory category learning is not significantly related to working memory span.
The solid line denotes the best fitting line. As predicted, working memory capacity was significantly positively related to reflective-optimal performance, as indexed by performance on the final block (r = 0.393, p = 0.028), but was not significantly related to reflexive-optimal performance (r = -0.069, p > 0.05). This is consistent with COVIS prediction that working memory capacity is critical for learning reflective-optimal category structures, but not for learning reflexive-optimal category structures (Maddox and Ashby, 2004; Ashby and Maddox, 2005, 2010). In the next section, we review recent studies applying the COVIS model to speech category learning
Reflective and Reflexive Auditory Systems in Speech Learning
One advantage of extending COVIS to the auditory domain is that it allows the exploration of natural category learning problems. Speech perception can be likened to a categorization problem, in which, multidimensional and highly variable acoustic signals are needed to be parsed into discrete phonological representations. One exciting possibility is that dual-learning systems may underlie speech category learning, which is one of the most difficult human category learning problems. The ability to learn and understand (categorize) speech sounds, either as a first or second language, is a critical skill at which humans are remarkably adept. In fact, as anyone who has experience with the speech recognition systems associated with many “smart” phones knows, the human ability to understand speech far out weights that of even the most sophisticated computer algorithm. The multidimensional and highly variable characteristics of speech signals make speech learning a “difficult” categorization problem, especially for individuals learning novel speech categories in adulthood.
Previous research has theorized several reasons for difficulties in the acquisition of second language (L2) speech categories. These difficulties have been interference caused by existing speech categories, as well as interference due to a “warping” of auditory-perceptual space by prior experience with native speech categories (Flege, 1999; Francis and Nusbaum, 2002; Kilpatrick et al., 2003; Francis et al., 2008). Although difficult, adults can acquire L2 speech categories. Laboratory training paradigms ubiquitously utilize trial-by-trial feedback and high-variability (multiple speakers) training to teach L2 speech categories (Lively et al., 1993; Bradlow et al., 1999; Tricomi et al., 2006; Zhang et al., 2009; Lim and Holt, 2011). Feedback is thought to enhance learning by reducing errors, and multiple-speaker training results in learners refocusing their attention to cues that are relevant for distinguishing speech categories and/or reducing attention to irrelevant cues (Bradlow and Bent, 2008). Although unsupervised training results in some amount of speech learning in adults, the addition of feedback results in substantially larger learning gains (McClelland et al., 2002; Vallabha and McClelland, 2007; Goudbeek et al., 2008). Studies have also examined the role of high-variability (multiple-speaker) training in speech learning. While much of this research has focused on the mechanics of the perceptual system in speech learning, much less is known about the role of the dual-learning systems, which previous studies suggest is critical to learning reflective-optimal and reflexive-optimal category structures. This leads us to an important question: are speech categories similar to reflective-optimal category structures or reflexive-optimal category structures?
Speech categories typically are difficult to verbalize, have multiple dimensions, and are highly variable. Generating and testing hypotheses for categories involving multiple dimensions is resource-intensive. Since the reflective system is dependent on working memory and attention, generating rules/hypotheses for multiple dimensions may not be efficient. Furthermore, the redundancy and variability of cues available during speech perception prevents a simple one-to-one mapping of cues to categories. These suggest that reflexive learning may be most optimal for speech categories. Our hypothesis is therefore that speech learning is reflexive-optimal. During natural visual category learning, the dual-learning systems framework assumes that the reflective and reflexive learning systems compete throughout learning for control (Ashby and Maddox, 2011). Early in category learning, the dual-learning systems model assumes that learners are mostly reflective. They actively test a number of hypotheses and use feedback to validate or invalidate rules. With practice, learners switch to the more automatic, reflexive learning system if the output of this system is more accurate than the reflective system. In line with dual-learning systems predictions, we propose that learning speech category structures is reflexive-optimal and that successful learners may initially use reflective strategies but eventually switch to the more optimal (reflexive) learning system. We have conducted a series of experiments to test this hypothesis. In the next section, we will briefly discuss the major points from these studies.
Application 1: Is Speech Learning Reflective- or Reflexive-Optimal? Chandrasekaran et al. (2014)
As outlined above, our working hypothesis is that speech categories are optimally learned by the reflexive learning system (Chandrasekaran et al., 2014). This is because speech categories are often difficult to verbalize and utilize acoustic cues that are multidimensional, highly redundant, and variable across speakers (Gandour, 1983; Holt and Lotto, 2008, 2010). Creating rules for such complex category structures may not be optimal, since generating and testing rules that involve multiple dimensions is resource intensive. Chandrasekaran et al. (2014) utilized the dissociation logic developed to test COVIS and training manipulations on trial-by-trial feedback (Experiments 1 and 2) and speaker variability (Experiment 3) to examine the relative contribution of the reflective and reflexive learning systems to speech learning success. The reflective and reflexive learning systems have been shown to respond differentially to various training manipulations. For example, delaying the presentation of feedback impairs learning in the reflexive system, but not in the reflective system (Maddox et al., 2003; Maddox and Ing, 2005). This is because the reflexive system is critically dependent on dopamine-mediated stimulus-response implicit reward learning. Delaying feedback interferes with dopamine release, reducing the effectiveness of the association of stimulus-response with reward. Also, rich, informational, “full” feedback that provides the correctness of the response on each trial as well as information about which category was present speeds learning in the reflective system (Maddox et al., 2008) relative to “minimal” feedback that provides only the correctness of the response on each trial. Full feedback promotes the generation and testing of rules that are critical to reflective learning but disrupts the transfer of control to the reflexive system (Maddox et al., 2008). Previous studies have used these timing and feedback manipulations to dissociate the learning systems in artificial visual category learning, but not in natural speech category learning.
Experiment 1 determined the extent to which the immediacy of feedback (immediate vs. delayed) impacts tone category learning. Experiment 2 determined the extent to which the information content of feedback (full versus minimal feedback) impacts tone category learning (Figure 5). Immediate feedback is critical for the reflexive system but not the reflective system (Maddox et al., 2003), while full feedback selectively speeds reflective learning but impairs reflexive learning (Maddox et al., 2008). Based on our working hypothesis, we predicted that feedback manipulations that targeted the reflexive learning system (immediate or minimal feedback) would enhance learning relative to those that target the reflective learning system (delayed or full feedback).
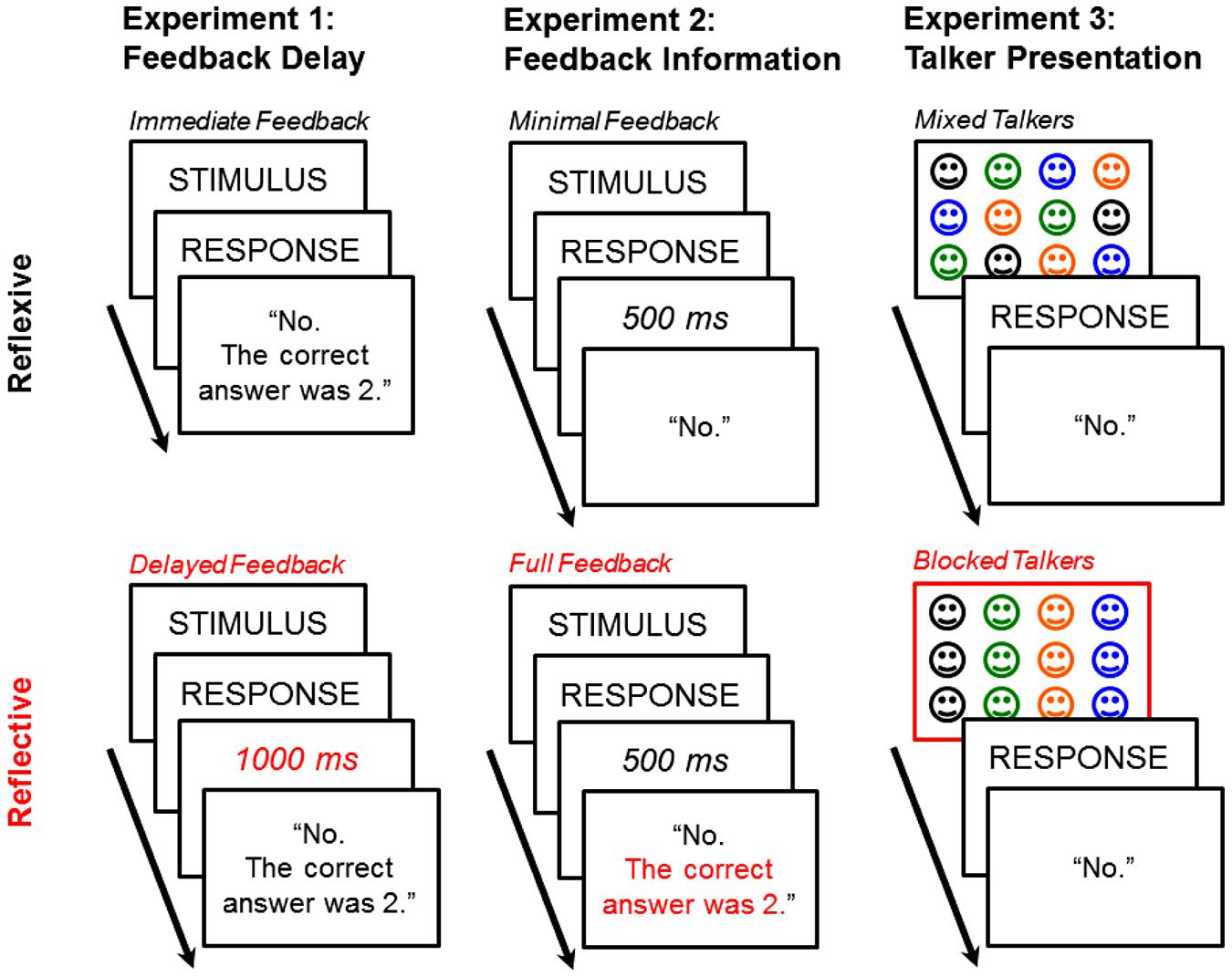
FIGURE 5. Experimental procedures from Chandrasekaran et al. (2014). In Experiments 1–3, we examined the effects of reflexive (top panel) or reflective (bottom panel) training manipulations on tone category learning success.
While dual-learning systems models of visual category learning make specific predictions about feedback processing, they offer no clear prediction about the impact of speaker variability on category learning success. While multi-speaker training is argued to be advantageous in generalizing to speech produced by novel speakers, the role of the order of speaker presentation, if any, has not been systematically examined in previous research. Within the framework of the dual-learning systems, we predicted that systematically blocked speaker presentation (i.e., presenting all stimuli from one speaker) will promote reflective learning, whereas a randomly mixed-speaker presentation will enhance reflexive learning. Our logic here is that blocked speaker presentation promotes faster hypothesis testing and validation, and is therefore less resource intensive for the reflective system than is the mixed-speaker condition. Also, the mixed-speaker presentation does not allow learners to predict the next speaker in advance, disrupting the immediate testing of speaker-specific rules. Therefore, our prediction is that learners are more likely to associate speaker-invariant acoustic cues with implicit reward than speaker-variant cues. Based on the hypothesis that speech learning is optimally learned by the reflexive learning system, we predicted enhanced learning in the mixed-speaker condition, relative to the blocked speaker condition.
Speech Category Learning Task
To study L2 speech category learning, we utilized naturally produced Mandarin tone categories, which are non-native to monolingual English speakers. Mandarin Chinese has four tone categories [ma1 “mother” [T1], ma2 “hemp” [T2], ma3 “horse” [T3], ma4 “scold” [T4]), described phonetically as high level, low rising, low dipping, and high falling, respectively (Figure 6A). Native English speakers find it particularly difficult to learn tone categories (Wang et al., 2003). However, previous studies also show that short-term laboratory training can enhance tone identification and discrimination in native English speakers, although such training paradigms have typically resulted in significant inter-individual differences in learning success (Perrachione et al., 2011).
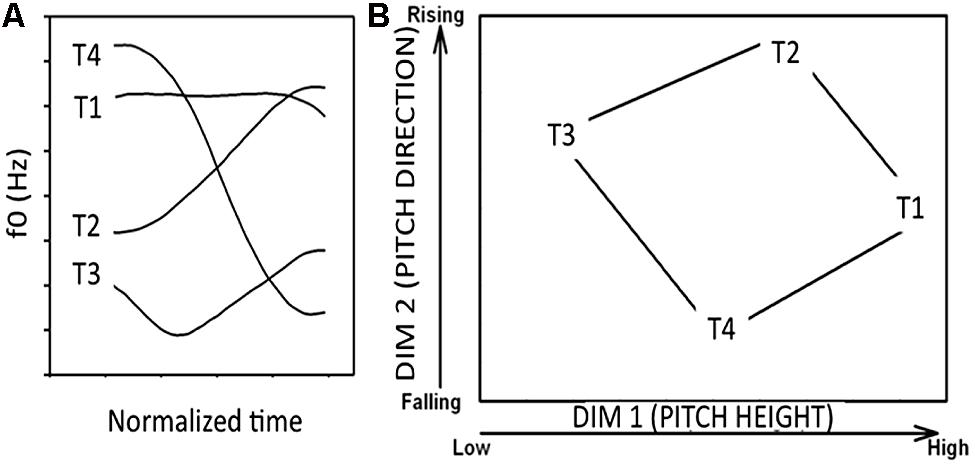
FIGURE 6. (A) Sample fundamental frequency contours of four Mandarin tones (T1: high-level; T2: low-rising; T3: low-dipping; T4: high-falling) produced by a male native Mandarin speaker. (B) The four tones plotted in a two-dimensional perceptual space (x-axis: pitch height, y-axis: pitch direction). Pitch height (dimension 1) and pitch direction (dimension 2) are major cues used to distinguish the tone categories.
A number of dimensions (e.g., pitch height, pitch direction) may serve as cues to tone categorization. The relative perceptual saliency of these dimensions is influenced by the presence or absence of pitch patterns in a language’s tonal inventory (Gandour, 1978, 1983) as well as by the occurrence of abstract rules in a listeners’ phonological system (Hume and Johnson, 2001). Multidimensional scaling studies on tone perception converge on two primary dimensions that underlie the tone space: labeled pitch height and pitch direction (Figure 6).
In Figure 7A, we plot the 80 stimuli used in our experiments (five consonant–vowel segments X four speakers X four tones) along two dimensions [pitch height: average fundamental frequency (x-axis) and pitch direction: slope (y-axis)]. A visual inspection of this space supports our hypothesis that speech category learning is reflexive-optimal (similar to the structure in Figure 3B). That is, category separation is greatest when the dimensions (pitch height and direction) are integrated in a manner that is not easily verbalizable.
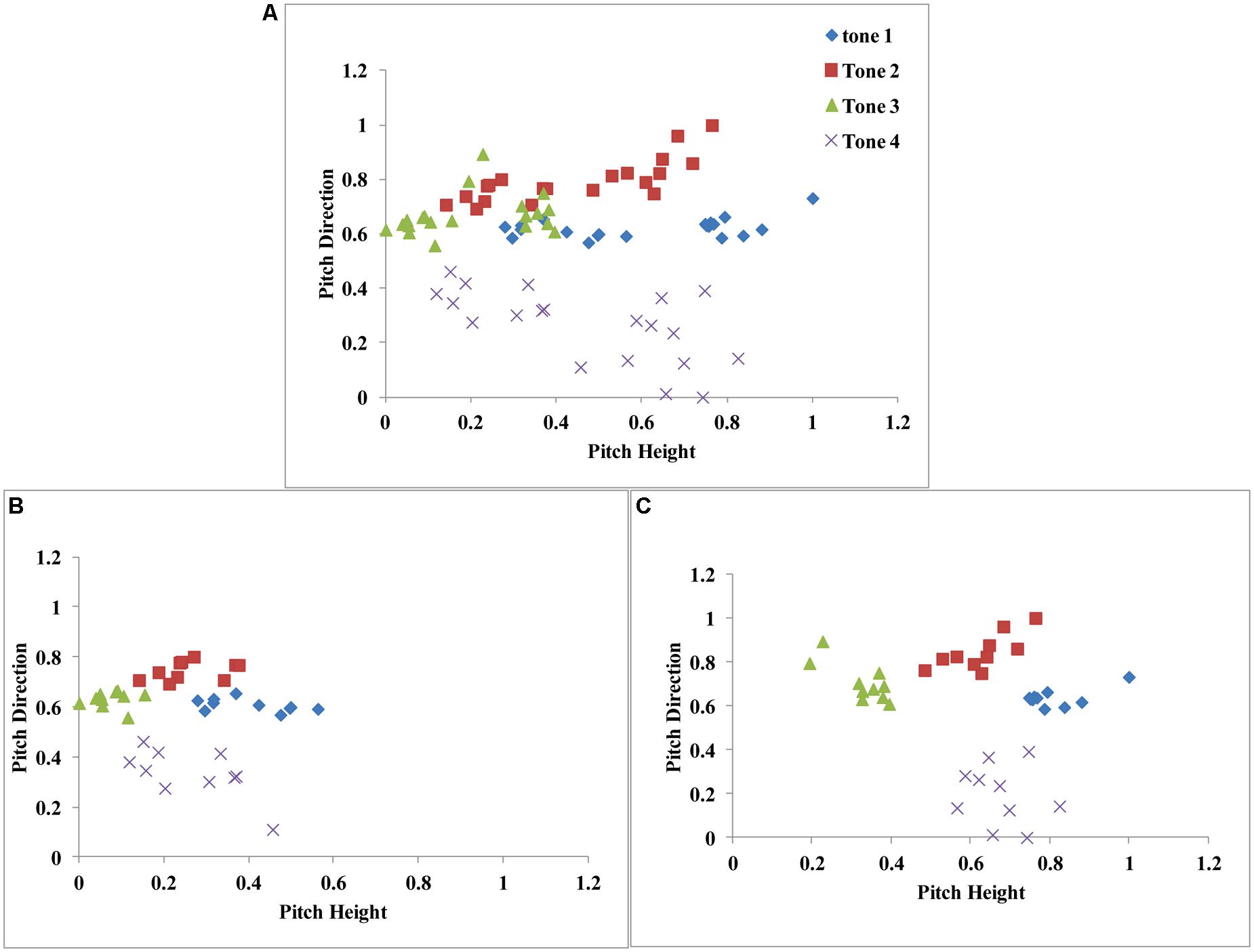
FIGURE 7. (A) Scatterplot of all stimuli from the Mandarin tone category learning experiment. (B) Scatterplot of male-speaker stimuli. (C) Scatterplot of female-speaker stimuli. Stimulus dimensions (pitch height and pitch direction) were normalized between 0 and 1.
Results from Chandrasekaran et al. (2014)
Figure 8 summarizes the results from the three experiments. In all cases, the training manipulation hypothesized to enhance reflexive learning led to better long-term Mandarin tone learning than the training manipulation hypothesized to enhance reflective learning. Taken together, these data provide strong support for the prediction that natural speech category learning is reflexive-optimal.
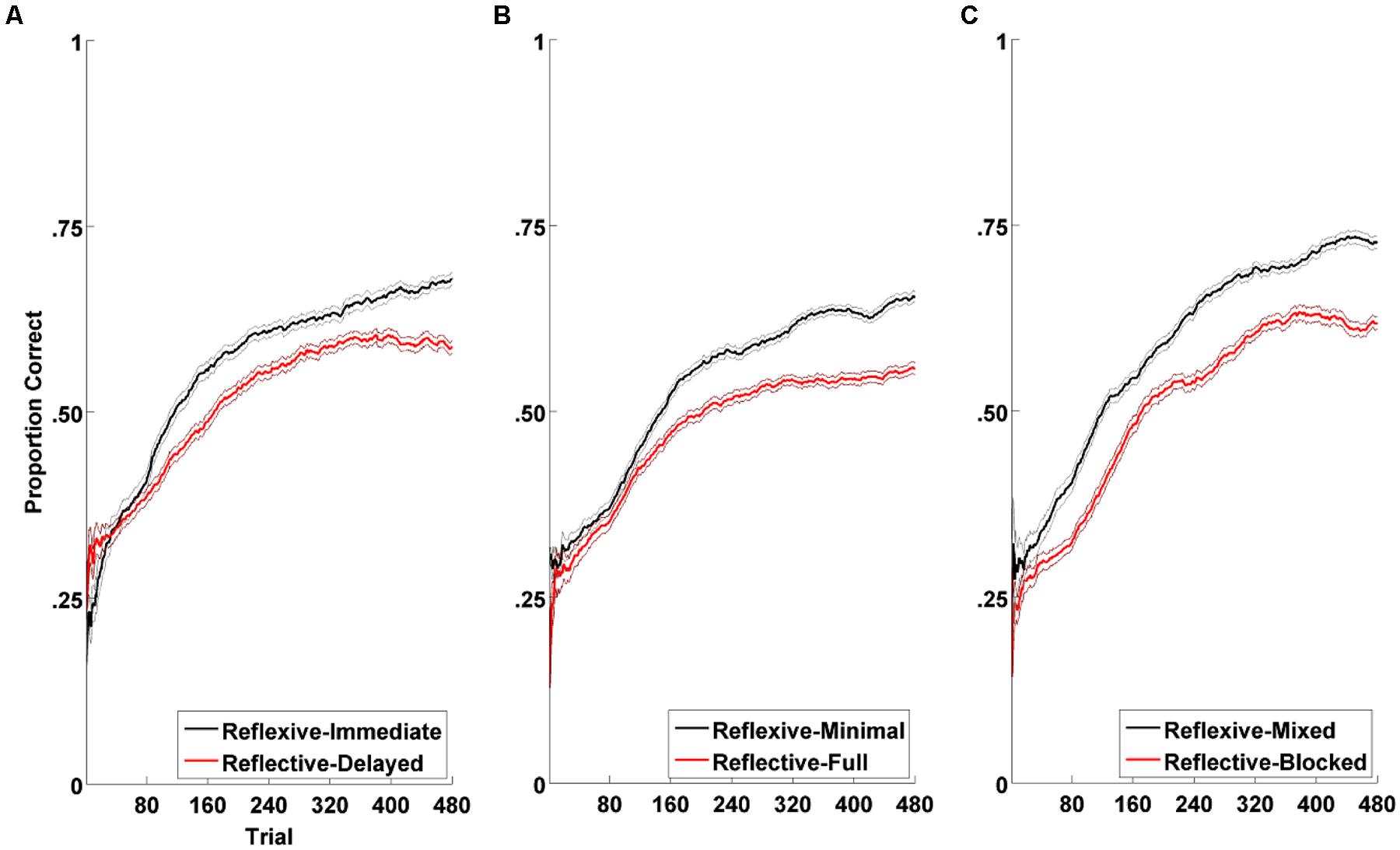
FIGURE 8. Category learning curves across reflexive vs. reflective conditions in all three experiments from Chandrasekaran et al. (2014): (A) Experiment 1: feedback delay (immediate vs. delayed); (B) Experiment 2: feedback information (minimal vs. full); (C) Experiment 3: speaker variability (mixed vs. blocked). Plotted in solid bold lines are the proportions of correct responses across participants within each condition over the course of learning. The black lines denote the reflexive conditions and the red, the reflective conditions. For purposes of visualization of trial-by-trial data, each point in the line denotes the average number of correct responses in a sliding 80-trial window. For trials preceding the 80th trial, cumulative averages were used. Plotted in thin lines are the ranges of standard error of the averages used in the sliding windows. Visual assessment of the learning curves suggest that both conditions result in equivalent degrees of category learning toward the earlier phase of experiment, but that the reflexive condition leads to greater learning than does the reflective condition toward the later phase of the experiment. This pattern is consistent across all three experiments.
Application 2: Computational Models as a Window onto Cognitive Processing: A Reanalysis of Chandrasekaran et al. (2014)
Chandrasekaran et al. (2014) relied on behavioral measures of accuracy to determine whether L2 speech category learning was reflective-optimal or reflexive-optimal. Although a good starting point, one weakness of accuracy-based measures is that the same accuracy rate can often be achieved by using qualitatively different strategies (e.g., reflective or reflexive). Within the domain of category learning, computational models can be utilized that address this shortcoming and can provide important insights into the nature of the strategy (reflective/reflexive) that an individual is applying in a given task. We predict that individuals in the immediate feedback, minimal feedback, and mixed-speaker conditions will utilize reflexive strategies to a greater degree than individuals in the delayed feedback, rich informational feedback, and blocked speaker conditions.
To test this hypothesis, we applied a series of decision-bound models developed by Maddox and Chandrasekaran (in press) on a block-by-block basis at the individual participant level. This was due to problems with interpreting fits to aggregate data (Estes, 1956; Ashby et al., 1994; Maddox, 1999). We assume that the two-dimensional space (pitch height vs. pitch direction) displayed in Figure 7A accurately describes the perceptual representation of the stimuli. Based on the results from our earlier work (Maddox and Chandrasekaran, in press), we also assumed that participants applied category learning strategies separately to the male (Figure 7B) and female (Figure 7C) perceptual spaces. Note that, as long as the major dimensions are known, these modeling procedures can be applied to any type of speech category structure. This offers an exciting new approach to the study of speech categorization.
Model Details
Here we provide a brief description of each model. More details are available in numerous previous publications (e.g., Ashby and Maddox, 1993; Maddox and Ashby, 1993; Maddox and Chandrasekaran, in press). Each model assumes that decision bounds were used to classify stimuli into each of the four Mandarin tone categories (T1, T2, T3, or T4). The model-based approach involves applying three classes of models, with multiple instantiations possible within a class. The first class is computational models of the reflexive procedural learning system. This is instantiated with the Striatal Pattern Classifier (SPC; Ashby and Waldron, 1999; Maddox et al., 2002b). The SPC is a computational model whose processing is consistent with what is known about the neurobiology of the procedural-based category learning system thought to underlie II classification performance (Ashby et al., 1998; Maddox et al., 2002a; Seger and Cincotta, 2005; Ashby and Ennis, 2006; Nomura et al., 2007). The second class is reflective, RB and instantiate hypothesis-testing strategies, such as the application of unidimensional or conjunctive rules. These are verbalizable strategies. The third model is a random responder model that assumes that the participant guesses on each trial. The model parameters were estimated using maximum likelihood procedures (Wickens, 1982; Ashby, 1992) and models were compared using Akaike weights (Wagenmakers and Farrell, 2004). These detailed analyses are available in the original manuscript. We provide the specifics of each model in the next section.
Striatal pattern classifiers
The SPC assumes that stimuli are represented perceptually in higher level auditory areas, such as the superior temporal gyrus. Because of the massive many-to-one (approximately 10,000-to-1) convergence of afferents from the primary and secondary sensory cortices to the striatum (Wilson, 1995; Ashby and Ennis, 2006), a low-resolution map of perceptual space is represented among the striatal units. Within the auditory domain, it is well known that there are direct projections from secondary auditory areas such as superior temporal gyrus and supratemporal plane to the caudate (Hikosaka et al., 1989; Arnauld et al., 1996; Yeterian and Pandya, 1998). During feedback-based learning, the striatal units become associated with one of the category labels so that, after learning is complete, a category response label is associated with each of a number of different regions of perceptual space. In effect, the striatum learns to associate a response with clumps of cells in the auditory cortex. It is important to be clear that the SPC is a computational model that is inspired by what is known about the neurobiology of the striatum. Because of this fact, the striatal “units” are hypothetical and could be interpreted within the language of other computational models (e.g., as “prototypes” in a multiple prototype model like SUSTAIN; Love et al., 2004). In addition, we do not model learning in the SPC in the sense that we do not update association weights between units and category labels. Learning models have been proposed (Ashby and Maddox, 2011) but are not utilized here due to their complexity. The SPC assumes that there is one striatal “unit” in the pitch height–pitch direction space for each category, and a single “noise” parameter that represents the noise associated with the placement of the striatal units. Responses from a hypothetical participant using the SPC are displayed in Figure 9A.
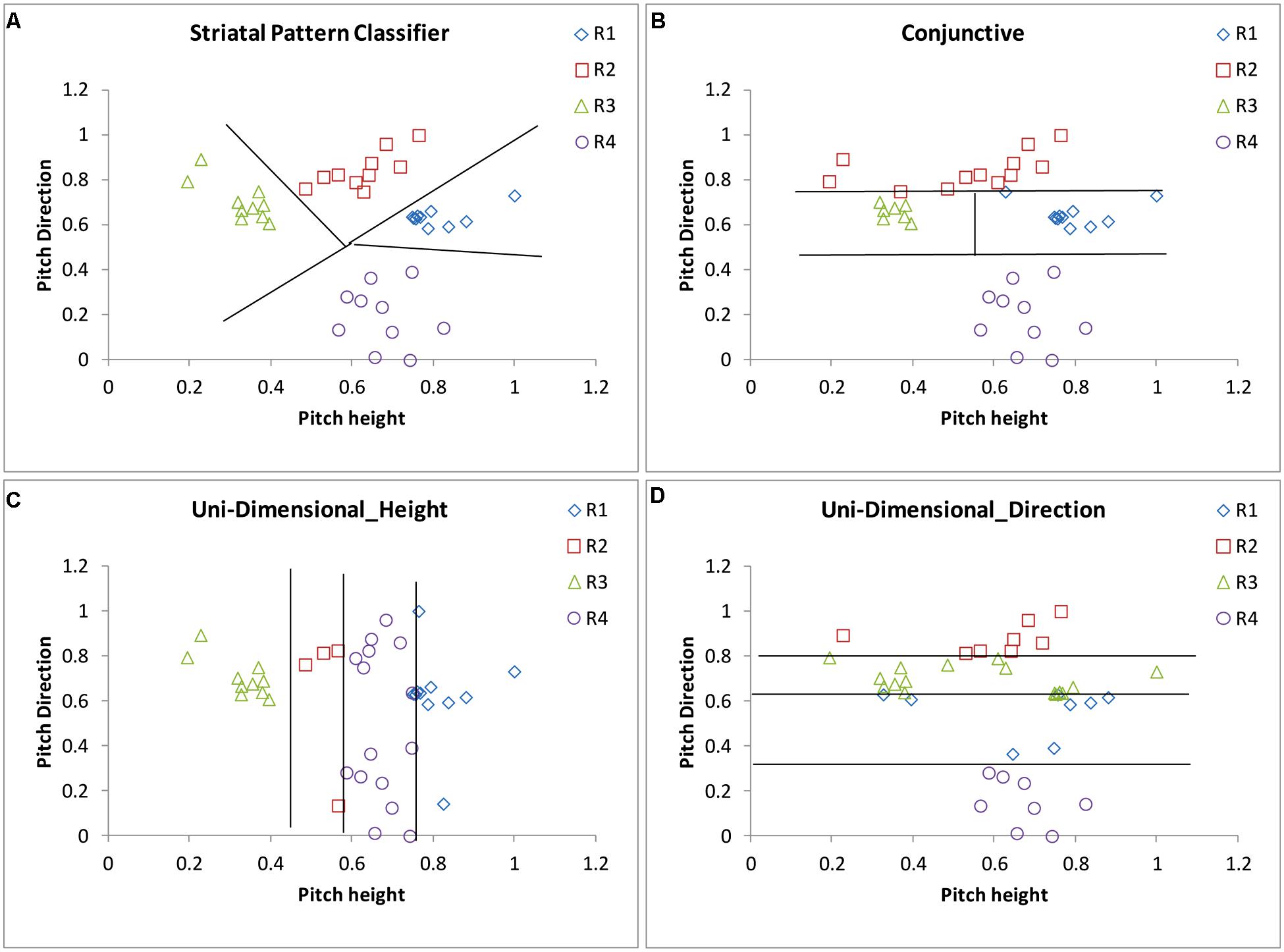
FIGURE 9. Scatterplots of the responses along with the decision boundaries that separate response regions from a hypothetical participant using a version of the (A) Striatal Pattern Classifier, (B) Conjunctive rule-based, (C) Uni-Dimensional Height, and (D) Uni-Dimensional Direction models as applied to the female-speaker stimuli shown in Figure 7C.
Conjunctive rule-based model
A conjunctive RB model that assumes that the participant sets two criteria along the pitch direction dimension and one criterion along the pitch height dimension was also applied to the data. The model assumes that the two criteria along the pitch direction dimension are used to separate the stimuli into those that are of low, medium, or high pitch direction. Low pitch direction items are classified into tone category 4 (T4) and high pitch direction items are classified into tone category 2 (T2). If an item is classified as having medium pitch direction, then the pitch height dimension is examined. The single criterion along the pitch height dimension is used to separate the stimuli into low and high pitch height. Stimuli that have medium pitch direction and low pitch height are classified into tone category 3 (T3) and medium pitch direction items of high pitch height are classified into tone category 1 (T1). Responses from a hypothetical participant using a conjunctive strategy are displayed in Figure 9B.
Unidimensional rule-based model
A unidimensional height RB model that assumes that the participant sets three criteria along the pitch height dimension was also applied to the data. The model assumes that the three criteria along the pitch height dimension are used to separate the stimuli into those that are of low, medium–low, medium–high or high pitch height, with each of these being associated with one of the four tone categories. Notice that this model completely ignores the pitch direction dimension. Although 24 versions of the model are possible given four category labels, some are highly unrealistic [e.g., a model that assumes that tone category 1 (T1) was the lowest in pitch height]. We examined the eight most reasonable variants of the model.
A unidimensional direction RB model that assumes that the participant sets three criteria along the pitch direction dimension was also applied to the data. The model assumes that the three criteria along the pitch direction dimension are used to separate the stimuli into those that are of low, medium-low, medium-high, or high pitch direction with each of these being associated with one of the tone categories. Notice that this model completely ignores the pitch height dimension. Although 24 versions of the model are possible given four category labels, many are highly unrealistic. We examined the two most reasonable variants of the model. Responses from a hypothetical participant using a unidimensional strategy along pitch height are displayed in Figure 9C, and responses from a hypothetical participant using a uni-dimensional strategy along pitch direction are displayed in Figure 9D.
Random responder model
The random responder model assumes a fixed probability of responding tone 1, tone 2, tone 3, and tone 4 but allows for response biases. The model has three free parameters to denote the predicted probability of responding “1,” “2,” or “3” with the probability of responding “4” equal to one minus the sum for the other three categories.
Model Results
As outlined in Application 1, we found better learning when feedback was immediate relative to delayed, when feedback was minimal relative to informationally rich, and when speaker presentation was mixed as opposed to blocked. We assumed that these performance advantages were due to better utilization of the reflexive system. As a test of this hypothesis, we fit the models outlined above to the data from the published study, focusing on the final block. In line with our predictions, we found that 53% of participant’s final block data in the immediate feedback condition was best fit by the SPC, whereas only 43% of participant’s final block data in the delayed feedback condition was best fit by the SPC. Analogously, we found that 53% of participant’s final block data in the minimal feedback condition was best fit by the SPC whereas only 42% of participant’s final block data in the informationally rich feedback condition was best fit by the SPC. Finally, and again in support of our hypothesis, we found that 67% of participant’s final block data in the mixed-speaker condition was best fit by the SPC whereas only 50% of participant’s final block data in the blocked speaker condition was best fit by the SPC.
Application 3: Individual Differences in Speech Category Learning
Speech Category Learning Across the Lifespan
One of our first applications of the dual-learning systems approach in the auditory domain was to examine the effect of normal aging on category learning. Little is known about the learning systems that mediate successful auditory and speech categorization across the lifespan. Normal aging is associated with some deficiencies in reflective and reflexive category learning within the visual domain (Ashby et al., 2003; Maddox et al., 2010), but these have not been explored in the auditory domain. Particularly, previous studies have demonstrated age-related declines in working memory and prefrontal function that may disproportionally impact learning reflective category structures (Daigneault and Braun, 1993; West, 1996; Clapp et al., 2011). We used experimental and computational modeling approaches to examine the extent to which dual-learning systems mediate speech learning in younger and older adults (Maddox et al., 2013). We used the same task outlined in Applications 1 and 2. We did have to make a minor change to get reasonable learning within a single session, and that was to include only one male and one female speaker instead of two male and two female speakers. This change led to only small differences in predicted accuracy across the reflective-conjunctive model and the reflexive-SPC model. However, reflective unidimensional models predicted poor accuracy.
We found an age-related deficit in overall performance that is displayed in Figure 10A. Figure 10B displays the proportion of older and younger adults whose final block of data was best fit by a multi-dimensional model (conjunctive or SPC) or a unidimensional model. Whereas approximately 70% of younger adults were using a multi-dimensional model, only about 30% of older adults were using a multi-dimensional model. Thus, older adults generally perseverated on unidimensional rules when the optimal strategy was to focus on both dimensions. The perseveration on simple unidimensional rules is likely due to a deficit in the reflective learning system. However, due to the fact that we could not separate conjunctive and SPC models, we cannot make a definite conclusion regarding a reflective learning deficit in older adults. This result mirrored previous results in the visual domain, where older adults were slower to transition from RB to procedural rules (Maddox et al., 2010). Next, we examined the final block accuracy rates for older and younger adults as a function of strategy type (Figure 10C). Interestingly, younger adults who used multi-dimensional strategies were more accurate than older adults who used multi-dimensional strategies. However, older and younger adults who used unidimensional strategies yielded about the same (low) accuracy rates. Taken together, these data suggest that younger adults are more likely than older adults to shift from suboptimal uni-dimensional to optimal multi-dimensional strategies, and even when older adults do shift to optimal multi-dimensional strategies, they use these less accurately than younger adults.
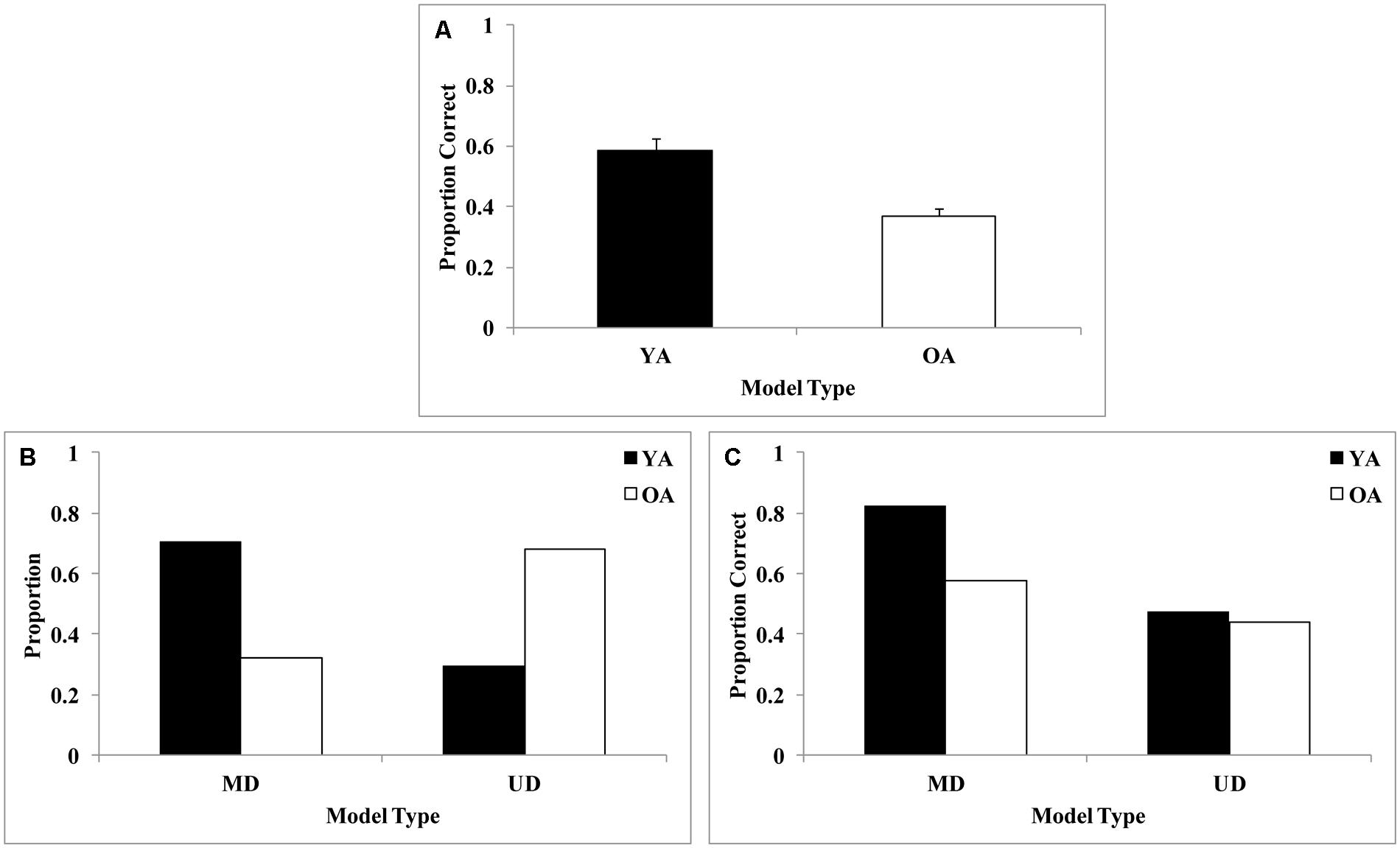
FIGURE 10. (A) Overall accuracy across older adults (OA) and younger adults (YA), (B) final block proportion of multi-dimensional [Striatal Pattern Classifier (SPC)/conjunctive rule-based (CJ)] and uni-dimensional (UD) models, and (C) final block accuracy for each model type by age group from Maddox et al. (2013). In this particular experiment’s stimulus set, SPC and CJ model fits were effectively inseparable, and so have been collapsed in this analysis. Older adults use a greater proportion of simple unidimensional rules, likely due to a deficit in the reflective learning system.
Influence of Depressive Symptoms on Speech Category Learning
A second application of the dual-learning systems approach in the auditory domain was to examine the effect of elevated depressive symptoms on category learning (Maddox et al., 2014). Little is known about the learning systems that mediate successful auditory and speech categorization in individuals with elevated depressive symptoms. Previous studies have shown that individuals with elevated depressive symptoms show deficits in reflective processing (Beevers, 2005; Carver et al., 2009; Beevers et al., 2012; Maddox et al., 2012; Blanco et al., 2013), and because of the deficit in frontally mediated processes, like working memory and cognitive flexibility, we would predict impaired performance on auditory reflective-optimal tasks. We exploited this finding to test critical predictions of the dual-learning systems model in audition. Because the reflective and reflexive systems are dissociable and competitive, we predicted that elevated depressive symptoms would lead to reflective-optimal learning deficits but reflexive-optimal learning advantages. Because natural speech category learning is reflexive in nature, we made the prediction that elevated depressive symptoms would lead to superior speech learning. In support of our predictions, individuals with elevated depressive symptoms showed a deficit in reflective-optimal auditory category learning, but an advantage in reflexive-optimal auditory category learning. In addition, using the same stimuli in Figure 7, we found that individuals with elevated depressive symptoms showed an advantage in learning a non-native speech category structure. Computational modeling suggested that the elevated depressive symptom advantage was due to faster, more accurate, and more frequent use of reflexive category learning strategies in individuals with elevated depressive symptoms.
Summary and Future Directions
Auditory category learning has been traditionally viewed as a perceptually encapsulated process. In contrast, the dual-learning systems theoretical approach tackles learning from an auditory-cognitive categorization perspective. This is an important step toward assessing domain-general influences on auditory and speech processing. Popular dual-learning systems models in vision have been cautious about extending this model beyond vision because the neurobiological plausibility of dual-learning systems in audition has not been extensively studied. Here we argue that the reflective and reflexive learning systems are neurobiologically viable in audition. Moreover, behavioral and computational modeling work clearly demonstrates a functional role for these systems in learning a variety of auditory categories. From a practical standpoint, understanding the role of the dual-learning systems may inform language pedagogy. Extant auditory training programs for language and music pedagogy may be suboptimal because the dynamics of feedback provided are arbitrary and do not target the learning system that is optimal for learning a particular auditory category structure. Our experiments clearly establish the optimal set of feedback characteristics for a broad range of auditory category problems. These training procedures can be easily incorporated into existing auditory training programs and language software, and may have a significant theoretical and practical impact on language and music pedagogy.
Conflict of Interest Statement
The authors declare that the research was conducted in the absence of any commercial or financial relationships that could be construed as a potential conflict of interest.
Acknowledgments
This research was supported by NIDA grant DA032457 and NIA grant AG043425 to W. T. Maddox. The authors thank Rachel Tessmer for reviewing an earlier version of the manuscript. The authors thank the Maddox Lab research assistants for data collection. This research was supported by NIMH grants MH077708 and DA032457 to W. T. Maddox.
Footnotes
- ^ Recent evidence suggests a third system, referred to as the perceptual-representation system, can also mediate category learning under certain conditions (Casale and Ashby, 2008; Zeithamova et al., 2008).
References
Allen, S. W., and Brooks, L. R. (1991). Specializing the operation of an explicit rule. J. Exp. Psychol. Gen. 120, 3–19. doi: 10.1037/0096-3445.120.1.3
Arnauld, E., Jeantet, Y., Arsaut, J., and Demotes-Mainard, J. (1996). Involvement of the caudal striatum in auditory processing: c-fos response to cortical application of picrotoxin and to auditory stimulation. Mol. Brain Res. 41, 27–35. doi: 10.1016/0169-328X(96)00063-0
Aron, A. R., Shohamy, D., Clark, J., Myers, C., Gluck, M. A., and Poldrack, R. A. (2004). Human midbrain sensitivity to cognitive feedback and uncertainty during classification learning. J. Neurophysiol. 92, 1144–1152. doi: 10.1152/jn.01209.2003
Ashby, F. G. (1992). “Multidimensional models of categorization,” in Multidimensional Models of Perception and Cognition, ed. F. G. Ashby (Hillsdale, NJ: Erlbaum).
Ashby, F. G. (2014). Is state-trace analysis an appropriate tool for assessing the number of cognitive systems? Psychon. Bull. Rev. 21, 935–946. doi: 10.3758/s13423-013-0578-x
Ashby, F. G., Alfonso-Reese, L. A., Turken, A. U., and Waldron, E. M. (1998). A neuropsychological theory of multiple systems in category learning. Psychol. Rev. 105, 442–481. doi: 10.1037/0033-295X.105.3.442
Ashby, F. G., and Crossley, M. J. (2011). A computational model of how cholinergic interneurons protect striatal-dependent learning. J. Cogn. Neurosci. 26, 1549–1566. doi: 10.1162/jocn.2010.21523
Ashby, F. G., Ell, S. W., Valentin, V. V., and Casale, M. B. (2005). FROST: a distributed neurocomputational model of working memory maintenance. J. Cogn. Neurosci. 17, 1728–1743. doi: 10.1162/089892905774589271
Ashby, F. G., and Ennis, J. M. (2006). The role of the basal ganglia in category learning. Psychol. Learn. Motiv. 46, 1–36. doi: 10.1016/S0079-7421(06)46001-1
Ashby, F. G., and Maddox, W. T. (1993). Relations between prototype, exemplar, and decision bound models of categorization. J. Math. Psychol. 37, 372–400. doi: 10.1006/jmps.1993.1023
Ashby, F. G., and Maddox, W. T. (2005). Human category learning. Annu. Rev. Psychol. 56, 149–178. doi: 10.1146/annurev.psych.56.091103.070217
Ashby, F. G., and Maddox, W. T. (2010). Human category learning 2.0. Ann. N. Y. Acad. Sci. 1224, 147–161. doi: 10.1111/j.1749-6632.2010.05874.x
Ashby, F. G., and Maddox, W. T. (2011). Human category learning 2.0. Ann. N.Y. Acad. Sci. 1224, 147–161. doi: 10.1111/j.1749-6632.2010.05874.x
Ashby, F. G., Maddox, W. T., and Lee, W. W. (1994). On the dangers of averaging across subjects when using multidimensional scaling or the similarity-choice model. Psychol. Sci. 5, 144–151. doi: 10.1111/j.1467-9280.1994.tb00651.x
Ashby, F. G., Noble, S., Filoteo, J. V., Waldron, E. M., and Ell, S. W. (2003). Category learning deficits in Parkinson’s disease. Neuropsychology 17, 115–124. doi: 10.1037/0894-4105.17.1.115
Ashby, F. G., and O’Brien, J. B. (2005). Category learning and multiple memory systems. Trends Cogn. Sci. 9, 83–89. doi: 10.1016/j.tics.2004.12.003
Ashby, F. G., Paul, E., and Maddox, W. T. (2011). “COVIS 2.0,” in Formal Approaches in Categorization, ed. E. P. A. Wills (New York: Cambridge University Press).
Ashby, F. G., and Perrin, N. A. (1988). Toward a unified theory of similarity and recognition. Psychol. Rev. 95, 124–150. doi: 10.1037/0033-295X.95.1.124
Ashby, F. G., and Townsend, J. T. (1986). Varieties of perceptual independence. Psychol. Rev. 95, 124–150. doi: 10.1037/0033-295X.95.1.124
Ashby, F. G., and Valentin, V. V. (2005). “Multiple systems of perceptual category learning: theory and cognitive tests,” in Categorization in Cognitive Science, eds H. Cohen and C. Lefebvre (New York: Elsevier), 543–578.
Ashby, F. G., and Waldron, E. M. (1999). On the nature of implicit categorization. Psychon. Bull. Rev. 6, 363–378. doi: 10.3758/BF03210826
Beevers, C. G. (2005). Cognitive vulnerability to depression: a dual process model. Clin. Psychol. Rev. 25, 975–1002. doi: 10.1016/j.cpr.2005.03.003
Beevers, C. G., Worthy, D. A., Gorlick, M. A., Nix, B., Chotibut, T., and Maddox, W. T. (2012). Influence of depression symptoms on history independent reward and punishment processing. Psychiatry Res. 207, 53–60. doi: 10.1016/j.psychres.2012.09.054
Blanco, N. J., Otto, A. R., Maddox, W. T., Beevers, C. G., and Love, B. C. (2013). The influence of depression symptoms on exploratory decision-making. Cognition 129, 563–568. doi: 10.1016/j.cognition.2013.08.018
Bradlow, A. R., Akahane-Yamada, R., Pisoni, D. B., and Tohkura, Y. (1999). Training Japanese listeners to identify English /r/ and /l/: long-term retention of learning in perception and production. Percept. Psychophys. 61, 977–985. doi: 10.3758/BF03206911
Bradlow, A. R., and Bent, T. (2008). Perceptual adaptation to non-native speech. Cognition 106, 707–729. doi: 10.1016/j.cognition.2007.04.005
Carver, C. S., Johnson, S. L., and Joormann, J. (2009). Two-mode models of self-regulation as a tool for conceptualizing effects of the serotonin system in normal behavior and diverse disorders. Curr. Dir. Psychol. Sci. 18, 195–199. doi: 10.1111/j.1467-8721.2009.01635.x
Casale, M. B., and Ashby, F. G. (2008). A role for the perceptual representation memory system in category learning. Percept. Psychophys. 70, 983–999. doi: 10.3758/PP.70.6.983
Chandrasekaran, B., Yi, H. G., and Maddox, W. T. (2014). Dual-learning systems during speech category learning. Psychon. Bull. Rev. 21, 488–495. doi: 10.3758/s13423-013-0501-5
Cincotta, C., and Seger, C. A. (2000). An fMRI study of expert and novice categorization. J. Cogn. Neurosci. 99–99.
Cincotta, C. M., and Seger, C. A. (2007). Dissociation between striatal regions while learning to categorize via feedback and via observation. J. Cogn. Neurosci. 19, 249–265. doi: 10.1162/jocn.2007.19.2.249
Clapp, W. C., Rubens, M. T., Sabharwal, J., and Gazzaley, A. (2011). Deficit in switching between functional brain networks underlies the impact of multitasking on working memory in older adults. Proc. Natl. Acad. Sci. U.S.A. 108, 7212–7217. doi: 10.1073/pnas.1015297108
Cohen, M. A., Horowitz, T. S., and Wolfe, J. M. (2009). Auditory recognition memory is inferior to visual recognition memory. Proc. Natl. Acad. Sci. U.S.A. 106, 6008–6010. doi: 10.1073/pnas.0811884106
Daigneault, S., and Braun, C. M. (1993). Working memory and the self-ordered pointing task: further evidence of early prefrontal decline in normal aging. J. Clin. Exp. Neuropsychol. 15, 881–895. doi: 10.1080/01688639308402605
DeCaro, M. S., Thomas, R. D., and Beilock, S. L. (2008). Individual differences in category learning: sometimes less working memory capacity is better than more. Cognition 107, 284–294. doi: 10.1016/j.cognition.2007.07.001
Di Martino, A., Scheres, A., Margulies, D. S., Kelly, A. M. C., Uddin, L. Q., Shehzad, Z.,et al. (2008). Functional connectivity of human striatum: a resting state fMRI study. Cereb. Cortex 18, 2735–2747. doi: 10.1093/cercor/bhn041
Erickson, M. A., and Kruschke, J. K. (1998). Rules and exemplars in category learning. J. Exp. Psychol. Learn. Mem. Cogn. 127, 107–140.
Estes, W. K. (1956). The problem of inference from curves based on group data. Psychol. Bull. 53, 134–140. doi: 10.1037/h0045156
Estes, W. K. (1986). Array models for category learning. Cognit. Psychol. 18, 500–549. doi: 10.1016/0010-0285(86)90008-3
Estes, W. K. (1994). Classification and Cognition. New York: Oxford University Press. doi: 10.1093/acprof:oso/9780195073355.001.0001
Filoteo, J. V., Lauritzen, J. S., and Maddox, W. T. (2010). Removing the frontal lobes: the effects of engaging executive functions on perceptual category learning. Psychol. Sci. 21, 415–423. doi: 10.1177/0956797610362646
Filoteo, J. V., and Maddox, W. T. (2004). A quantitative model-based approach to examining aging effects on information-integration category learning. Psychol. Aging 19, 171–182. doi: 10.1037/0882-7974.19.1.171
Filoteo, J. V., and Maddox, W. T. (2007). “Category learning in Parkinson’s disease,” in Research Progress in Alzheimer’s Disease and Dementia, ed. M. K. Sun (New York: Nova Sciences Publishers), 339–365.
Filoteo, J. V., Maddox, W. T., Ing, A. D., Zizak, V., and Song, D. D. (2005a). The impact of irrelevant dimensional variation on rule-based category learning in patients with Parkinson’s disease. J. Int. Neuropsychol. Soc. 11, 503–513. doi: 10.1017/S1355617705050617
Filoteo, J. V., Maddox, W. T., Salmon, D. P., and Song, D. D. (2005b). Information-integration category learning in patients with striatal dysfunction. Neuropsychology 19, 212–222. doi: 10.1037/0894-4105.19.2.212
Filoteo, J. V., Maddox, W. T., Simmons, A. N., Ing, A. D., Cagigas, X. E., Matthews, S.,et al. (2005c). Cortical and subcortical brain regions involved in rule-based category learning. Neuroreport 16, 111–115. doi: 10.1097/00001756-200502080-00007
Flege, J. E. (1999). “Age of learning and second language speech,” in Second Language Acquisition and the Critical Period Hypothesis, ed. D. Birdsong (Hillsdale, NJ: Lawrence Erlbaum), 101–131.
Fleming, S. M., Maloney, L. T., and Daw, N. D. (2013). The irrationality of categorical perception. J. Neurosci. 33, 19060–19070. doi: 10.1523/JNEUROSCI.1263-13.2013
Folstein, J. R., and Van Petten, C. (2004). Multidimensional rule, unidimensional rule, and similarity strategies in categorization: event-related brain potential correlates. J. Exp. Psychol. Learn. Mem. Cogn. 30, 1026–1044. doi: 10.1037/0278-7393.30.5.1026
Francis, A. L., Ciocca, V., Ma, L., and Fenn, K. (2008). Perceptual learning of Cantonese lexical tones by tone and non-tone language speakers. J. Phonet. 36, 268–294. doi: 10.1016/j.wocn.2007.06.005
Francis, A. L., and Nusbaum, H. C. (2002). Selective attention and the acquisition of new phonetic categories. J. Exp. Psychol. Hum. Percept. Perform. 28, 349–366. doi: 10.1037/0096-1523.28.2.349
Gandour, J. (1978). Perceived dimensions of thirteen tones: a multidimensional scaling investigation. Phonetica 35, 169–179. doi: 10.1159/000259928
Goldstone, R. L., and Hendrickson, A. T. (2010). Categorical perception. Wiley Interdiscip. Rev. Cogn. Sci. 1, 69–78. doi: 10.1002/wcs.26
Gorlick, M. A., Giguere, G., Glass, B. D., Nix, B. N., Mather, M., and Maddox, W. T. (2012). Attenuating age-related learning deficits: emotional valenced feedback interacts with task complexity. Emotion 13, 250–261. doi: 10.1037/a0030071
Goudbeek, M., Cutler, A., and Smits, R. (2008). Supervised and unsupervised learning of multidimensionally varying non-native speech categories. Speech Commun. 50, 109–125. doi: 10.1016/j.specom.2007.07.003
Grimm, L. R., and Maddox, W. T. (2013). Differential impact of relevant and irrelevant dimension primes on rule-based and information-integration category learning. Acta Psychol. (Amst.) 144, 530–537. doi: 10.1016/j.actpsy.2013.09.005
Helie, S., Waldschmidt, J. G., and Ashby, F. G. (2010). Automaticity in rule-based and information-integration categorization. Atten. Percept. Psychophys. 72, 1013–1031. doi: 10.3758/APP.72.4.1013
Hikosaka, O., Sakamoto, Y., and Usui, S. (1989). Functional properties of monkey caudate neruons: III. Activities related to expectation of target and reward. J. Neurophysiol. 61, 814–832.
Hintzman, D. L. (1986). “Schema abstraction” in a multiple-trace memory model. Psychol. Rev. 93, 411–428. doi: 10.1037/0033-295X.93.4.411
Holt, L. L., and Lotto, A. J. (2008). Speech perception within an auditory cognitive science framework. Curr. Dir. Psychol. Sci. 17, 42–46. doi: 10.1111/j.1467-8721.2008.00545.x
Holt, L. L., and Lotto, A. J. (2010). Speech perception as categorization. Atten. Percept. Psychophys. 72, 1218–1227. doi: 10.3758/APP.72.5.1218
Homa, D., Sterling, S., and Trepel, L. (1981). Limitations of exemplar-based generalization and the abstraction of categorical information. J. Exp. Psychol. Hum. Learn. Mem. 7, 418–439. doi: 10.1037/0278-7393.7.6.418
Huang-Pollock, C. L., Maddox, W. T., and Karalunas, S. L. (2011). Development of implicit and explicit category learning. J. Exp. Child Psychol. 109, 321–335. doi: 10.1016/j.jecp.2011.02.002
Hume, E., and Johnson, K. (2001). A model of the interplay of speech perception and phonology. Role Speech Percept. Phonol. 3–26.
Keri, S. (2003). The cognitive neuroscience of category learning. Brain Res. Rev. 43, 85–109. doi: 10.1016/S0165-0173(03)00204-2
Kilpatrick, D. G., Ruggiero, K. J., Acierno, R., Saunders, B. E., Resnick, H. S., and Best, C. L. (2003). Violence and risk of PTSD, major depression, substance abuse/dependence, and comorbidity: results from the National Survey of Adolescents. J. Consult. Clin. Psychol. 71, 692–700. doi: 10.1037/0022-006X.71.4.692
Knowlton, B. J. (1999). What can neuropsychology tell us about category learning? Trends Cogn. Sci. 3, 123–124. doi: 10.1016/S1364-6613(99)01292-9
Knowlton, B. J., and Squire, L. R. (1993). The learning of categories: parallel brain systems for item memory and category level knowledge. Science 262, 1747–1749. doi: 10.1126/science.8259522
Knowlton, B. J., Squire, L. R., and Gluck, M. A. (1994). Probabilistic classification learning in amnesia. Learn. Mem. 1, 106–120.
Kuhl, P. K. (1985). Theoretical contributions of tests on animals to the special-mechanisms debate in speech. Exp. Biol. 45, 233–265.
Kuhl, P. K. (1994). Learning and representation in speech and language. Curr. Opin. Neurobiol. 4, 812–822. doi: 10.1016/0959-4388(94)90128-7
Kuhl, P. K. (2004). Early language acquisition: cracking the speech code. Nat. Rev. Neurosci. 5, 831–843. doi: 10.1038/nrn1533
Kuhl, P. K., and Miller, J. D. (1978). Speech perception by the chinchilla: identification functions for synthetic VOT stimuli. J. Acoust. Soc. Am. 63, 905–917. doi: 10.1121/1.381770
LeDoux, J. E., Farb, C. R., and Romanski, L. M. (1991). Overlapping projections to the amygdala and striatum from auditory processing areas of the thalamus and cortex. Neurosci. Lett. 134, 139–144. doi: 10.1016/0304-3940(91)90526-Y
Lewandowsky, S., Yang, L. X., Newell, B. R., and Kalish, M. L. (2012). Working memory does not dissociate between different perceptual categorization tasks. J. Exp. Psychol. Learn. Mem. Cogn. 38, 881–904. doi: 10.1037/a0027298
Liberman, A. M., Cooper, F. S., Shankweiler, D. P., and Studdert-Kennedy, M. (1967). Perception of the speech code. Psychol. Rev. 74, 431. doi: 10.1037/h0020279
Lim, S. J., and Holt, L. L. (2011). Learning foreign sounds in an alien world: videogame training improves non-native speech categorization. Trends Cogn. Sci. 35, 1390–1405. doi: 10.1111/j.1551-6709.2011.01192.x
Lively, S. E., Logan, J. S., and Pisoni, D. B. (1993). Training Japanese listeners to identify English /r/ and /l/. II: the role of phonetic environment and talker variability in learning new perceptual categories. J. Acoust. Soc. Am. 94, 1242–1255. doi: 10.1121/1.408177
Lombardi, W. J., Andreason, P. J., Sirocco, K. Y., Rio, D. E., Gross, R. E., Umhau, J. C.,et al. (1999). Wisconsin Card Sorting Test performance following head injury: dorsolateral fronto-striatal circuit activity predicts perseveration. J. Clin. Exp. Neuropsychol. 21, 2–16. doi: 10.1076/jcen.21.1.2.940
Love, B. C., Medin, D. L., and Gureckis, T. M. (2004). SUSTAIN: a network model of category learning. Psychol. Rev. 111, 309–332. doi: 10.1037/0033-295X.111.2.309
Maddox, W. T. (1999). On the dangers of averaging across observers when comparing decision bound models and generalized context models of categorization. Percept. Psychophys. 61, 354–375. doi: 10.3758/BF03206893
Maddox, W. T., and Ashby, F. G. (1993). Comparing decision bound and exemplar models of categorization. Percept. Psychophys. 53, 49–70. doi: 10.3758/BF03211715
Maddox, W. T., and Ashby, F. G. (2004). Dissociating explicit and procedural-learning based systems of perceptual category learning. Behav. Processes 66, 309–332. doi: 10.1016/j.beproc.2004.03.011
Maddox, W. T., Ashby, F. G., and Bohil, C. J. (2003). Delayed feedback effects on rule-based and information-integration category learning. J. Exp. Psychol. Learn. Mem. Cogn. 29, 650–662. doi: 10.1037/0278-7393.29.4.650
Maddox, W. T., Ashby, F. G., Ing, A. D., and Pickering, A. D. (2004). Disrupting feedback processing interferes with rule-based but not information-integration category learning. Mem. Cogn. 32, 582–591. doi: 10.3758/BF03195849
Maddox, W. T., and Chandrasekaran, B. (in press). Tests of a dual system model of speech category learning. Biling. Lang. Cogn. doi: 10.1017/S1366728913000783
Maddox, W. T., Chandrasekaran, B., Smayda, K., Yi, H.-G., Koslov, S., and Beevers, C. G. (2014). Elevated depressive symptoms enhance reflexive but not reflective auditory category learning. Cortex 58, 186–198. doi: 10.1016/j.cortex.2014.06.013
Maddox, W. T., Chandrasekaran, B., Smayda, K., and Yi, H.-G. (2013). Dual systems of speech category learning across the lifespan. Psychol. Aging 28, 1042–1056. doi: 10.1037/a0034969
Maddox, W. T., and Filoteo, J. V. (2001). Striatal contributions to category learning: quantitative modeling of simple linear and complex nonlinear rule learning in patients with Parkinson’s disease. J. Int. Neuropsychol. Soc. 7, 710–727. doi: 10.1017/S1355617701766076
Maddox, W. T., and Filoteo, J. V. (2005). “The neuropsychology of perceptual category learning,” in Handbook of Categorization in Cognitive Science, eds. H. Cohen and C. Lefebvre (New York: Elsevier Ltd.), 573–599. doi: 10.1016/B978-008044612-7/50081-0
Maddox, W. T., Gorlick, M. A., Worthy, D. A., and Beevers, C. G. (2012). Depressive symptoms enhance loss-minimization, but attenuate gain-maximization in history-dependent decision-making. Cognition 125, 118–124. doi: 10.1016/j.cognition.2012.06.011
Maddox, W. T., and Ing, A. D. (2005). Delayed feedback disrupts the procedural-learning system but not the hypothesis-testing system in perceptual category learning. J. Exp. Psychol. Learn. Mem. Cogn. 31, 100–107. doi: 10.1037/0278-7393.31.1.100
Maddox, W. T., Love, B. C., Glass, B. D., and Filoteo, J. V. (2008). When more is less: feedback effects in perceptual category learning. Cognition 108, 578–589. doi: 10.1016/j.cognition.2008.03.010
Maddox, W. T., Molis, M. R., and Diehl, R. L. (2002a). Generalizing a neuropsychological model of visual categorization to auditory categorization of vowels. Percept. Psychophys. 64, 584–597. doi: 10.3758/BF03194728
Maddox, W. T., Molis, M. R., and Diehl, R. L. (2002b). Generalizing a neuropsychological model of visual categorization to auditory categorization of vowels. Percept. Psychophys. 64, 584–597. doi: 10.3758/BF03194728
Maddox, W. T., Pacheco, J., Reeves, M., Zhu, B., and Schnyer, D. M. (2010). Rule-based and information-integration category learning in normal aging. Neuropsychologia 48, 2998–3008. doi: 10.1016/j.neuropsychologia.2010.06.008
Marois, R., Leung, H.-C., and Gore, J. C. (2000). A stimulus-driven approach to object identity and location processing in the human brain. Neuron 25, 717–728. doi: 10.1016/S0896-6273(00)81073-9
McClelland, J. L., Fiez, J. A., and Mccandliss, B. D. (2002). Teaching the /r/-/l/ discrimination to Japanese adults: behavioral and neural aspects. Physiol. Behav. 77, 657–662. doi: 10.1016/S0031-9384(02)00916-2
McKinley, S. C., and Nosofsky, R. M. (1995). Investigations of exemplar and decision bound models in large, ill-defined category structures. J. Exp. Psychol. Hum. Percept. Perform. 21, 128–148. doi: 10.1037/0096-1523.21.1.128
Medin, D. L., and Schaffer, M. M. (1978). Context theory of classification learning. Psychol. Rev. 85, 207–238. doi: 10.1037/0033-295X.85.3.207
Minda, J. P., Desroches, A. S., and Church, B. A. (2008). Learning rule-described and non-rule-described categories: a comparison of children and adults. J. Exp. Psychol. Learn. Mem. Cogn. 34, 1518–1533. doi: 10.1037/a0013355
Monchi, O., Petrides, M., Petre, V., Worsley, K., and Dagher, A. (2001). Wisconsin Card Sorting revisited: distinct neural circuits participating in different stages of the task identified by event-related functional magnetic resonance imaging. J. Neurosci. 21, 7733–7741.
Nomura, E. M., Maddox, W. T., Filoteo, J. V., Ing, A. D., Gitelman, D. R., Parrish, T. B.,et al. (2007). Neural correlates of rule-based and information-integration visual category learning. Cereb. Cortex 17, 37–43. doi: 10.1093/cercor/bhj122
Nomura, E. M., and Reber, P. J. (2008). A review of medial temporal lobe and caudate contributions to visual category learning. Neurosci. Biobehav. Rev. 32, 279–291. doi: 10.1016/j.neubiorev.2007.07.006
Nosofsky, R. M. (1986a). Attention, similarity, and the identification-categorization relationship. J. Exp. Psychol. Gen. 115, 39–57. doi: 10.1037/0096-3445.115.1.39
Nosofsky, R. M. (1986b). Attention, similarity, and the identification-categorization relationship. J. Exp. Psychol. Gen. 115, 39–57.
Nosofsky, R. M. (1990). Relations between exemplar-similarity and likelihood models of classification. J. Math. Psychol. 34, 393–418. doi: 10.1016/0022-2496(90)90020-A
Nosofsky, R. M. (1991). Relations between the rational model and the context model of categorization. Psychol. Sci. 2, 416–421. doi: 10.1111/j.1467-9280.1991.tb00176.x
Nosofsky, R. M., Palmeri, T. J., and Mckinley, S. C. (1994). A rule-plus-exception model of classification learning. Psychol. Rev. 101, 53–79. doi: 10.1037/0033-295X.101.1.53
Parent, A., and Hazrati, L.-N. (1995). Functional anatomy of the basal ganglia. I. The cortico-basal ganglia-thalamo-cortical loop. Brain Res. Rev. 20, 91–127. doi: 10.1016/0165-0173(94)00007-C
Perrachione, T. K., Lee, J., Ha, L. Y., and Wong, P. C. (2011). Learning a novel phonological contrast depends on interactions between individual differences and training paradigm design. J. Acoust. Soc. Am. 130, 461–472. doi: 10.1121/1.3593366
Petrides, M., and Pandya, D. N. (1988). Association fiber pathways to the frontal cortex from the superior temporal region in the rhesus monkey. J. Comp. Neurol. 273, 52–66. doi: 10.1002/cne.902730106
Poldrack, R. A., Clark, J., Pare-Blagoev, E. J., Shohamy, D., Creso Moyano, J., Myers, C.,et al. (2001). Interactive memory systems in the human brain. Nature 414, 546–550. doi: 10.1038/35107080
Poldrack, R. A., and Packard, M. G. (2003). Competition among multiple memory systems: converging evidence from animal and human brain studies. Neuropsychologia 41, 245–251. doi: 10.1016/S0028-3932(02)00157-4
Poldrack, R. A., Prabhakaran, S. C. A., and Gabrieli, J. D. (1999). Striatal activation during acquisition of a cognitive skill. Neuropsychology 13, 564–574. doi: 10.1037/0894-4105.13.4.564
Poldrack, R. A., and Rodriguez, P. (2004). How do memory systems interact? Evidence from human classification learning. Neurobiol. Learn. Mem. 82, 324–332. doi: 10.1016/j.nlm.2004.05.003
Posner, M. I., and Petersen, S. E. (1990). The attention system of the human brain. Annu. Rev. Neurosci. 13, 25–41. doi: 10.1146/annurev.ne.13.030190.000325
Racine, C. A., Barch, D. M., Braver, T. S., and Noelle, D. C. (2006). The effect of age on rule-based category learning. Neuropsychol. Dev. Cogn. B Aging Neuropsychol. Cogn. 13, 411–434. doi: 10.1080/13825580600574377
Rauschecker, J. P., and Scott, S. K. (2009). Maps and streams in the auditory cortex: nonhuman primates illuminate human speech processing. Nat. Neurosci. 12, 718–724. doi: 10.1038/nn.2331
Reale, R., and Imig, T. (1983). Auditory cortical field projections to the basal ganglia of the cat. Neuroscience 8, 67–86. doi: 10.1016/0306-4522(83)90026-X
Reber, P. J., Gitelman, D. R., Parrish, T. B., and Mesulam, M. M. (2003). Dissociating explicit and implicit category knowledge with fMRI. J. Cogn. Neurosci. 15, 574–583. doi: 10.1162/089892903321662958
Reed, S. K. (1972). Pattern recognition and categorization. Cogn. Psychol. 3, 382–407. doi: 10.1016/0010-0285(72)90014-X
Regehr, G., and Brooks, L. R. (1993). Perceptual manifestations of an analytic structure: the priority of holistic individuation. J. Exp. Psychol. Gen. 122, 92–114. doi: 10.1037/0096-3445.122.1.92
Ridderinkhof, K. R., Span, M. M., and Van Der Molen, M. W. (2002). Perseverative behavior and adaptive control in older adults: performance monitoring, rule induction, and set shifting. Brain Cogn. 49, 382–401. doi: 10.1006/brcg.2001.1506
Romanski, L. M., Tian, B., Fritz, J., Mishkin, M., Goldman-Rakic, P. S., and Rauschecker, J. P. (1999). Dual streams of auditory afferents target multiple domains in the primate prefrontal cortex. Nat. Neurosci. 2, 1131–1136. doi: 10.1038/16056
Schnyer, D. M., Maddox, W. T., Ell, S., Davis, S., Pacheco, J., and Verfaellie, M. (2009). Prefrontal contributions to rule-based and information-integration category learning. Neuropsychologia 47, 2995–3006. doi: 10.1016/j.neuropsychologia.2009.07.011
Scott, B. H., Mishkin, M., and Yin, P. (2012). Monkeys have a limited form of short-term memory in audition. Proc. Natl. Acad. Sci. U.S.A. 109, 12237–12241. doi: 10.1073/pnas.1209685109
Seger, C. A. (2008). How do the basal ganglia contribute to categorization? Their roles in generalization, response selection, and learning via feedback. Neurosci. Biobehav. Rev. 32, 265–278. doi: 10.1016/j.neubiorev.2007.07.010
Seger, C. A., and Cincotta, C. M. (2005). The roles of the caudate nucleus in human classification learning. J. Neurosci. 25, 2941–2951. doi: 10.1523/JNEUROSCI.3401-04.2005
Seger, C. A., and Cincotta, C. M. (2006). Dynamics of frontal, striatal, and hippocampal systems during rule learning. Cereb. Cortex 16, 1546–1555. doi: 10.1093/cercor/bhj092
Seger, C. A., and Miller, E. K. (2010). Category learning in the brain. Annu. Rev. Neurosci. 33, 203–219. doi: 10.1146/annurev.neuro.051508.135546
Shohamy, D., Myers, C. E., Grossman, S., Sage, J., Gluck, M. A., and Poldrack, R. A. (2004). Cortico-striatal contributions to feedback-based learning: converging data from neuroimaging and neuropsychology. Brain J. Neurol. 127, 851–859. doi: 10.1093/brain/awh100
Smith, E. E., and Medin, D. L. (1981). Categories and Concepts. Cambridge, MA: Harvard University Press. doi: 10.4159/harvard.9780674866270
Smith, J. D., Ashby, F. G., Berg, M. E., Murphy, M. S., Spiering, B., Cook, R. G.,et al. (2011). Pigeons’ categorization may be exclusively nonanalytic. Psychon. Bull. Rev. 18, 414–421. doi: 10.3758/s13423-010-0047-8
Smith, J. D., Beran, M. J., Crossley, M. J., Boomer, J., and Ashby, F. G. (2010). Implicit and explicit category learning by macaques (Macaca mulatta) and humans (Homo sapiens). J. Exp. Psychol. Anim. Behav. Process 36, 54–65. doi: 10.1037/a0015892
Smith, J. D., Berg, M. E., Cook, R. G., Murphy, M. S., Crossley, M. J., Boomer, J.,et al. (2012a). Implicit and explicit categorization: a tale of four species. Neurosci. Biobehav. Rev. 36, 2355–2369. doi: 10.1016/j.neubiorev.2012.09.003
Smith, J. D., Crossley, M. J., Boomer, J., Church, B. A., Beran, M. J., and Ashby, F. G. (2012b). Implicit and explicit category learning by capuchin monkeys (Cebus apella). J. Comp. Psychol. 126, 294–304. doi: 10.1037/a0026031
Smith, J. D., Boomer, J., Zakrzewski, A. C., Roeder, J. L., Church, B. A., and Ashby, F. G. (2014). Deferred feedback sharply dissociates implicit and explicit category learning. Psychol. Sci. 25, 447–457. doi: 10.1177/0956797613509112
Smith, J. D., and Minda, J. P. (1998). Prototypes in the mist: the early epochs of category learning. J. Exp. Psychol. Learn. Mem. Cogn. 24, 1411–1436. doi: 10.1037/0278-7393.24.6.1411
Smith, J. D., Minda, J. P., and Washburn, D. A. (2004). Category learning in rhesus monkeys: a study of the Shepard, Hovland, and Jenkins (1961) tasks. J. Exp. Psychol. Gen. 133, 398–414. doi: 10.1037/0096-3445.133.3.398
Squire, L. R., and Knowlton, B. J. (1995). Learning about categories in the absence of memory. Proc. Natl. Acad. Sci. U.S.A. 92, 12470–12474. doi: 10.1073/pnas.92.26.12470
Tharp, I. J., and Pickering, A. D. (2009). A note on DeCaro, Thomas, and Beilock (2008): further data demonstrate complexities in the assessment of information-integration category learning. Cognition 111, 411–415. doi: 10.1016/j.cognition.2008.10.003
Tricomi, E., Delgado, M. R., Mccandliss, B. D., Mcclelland, J. L., and Fiez, J. A. (2006). Performance feedback drives caudate activation in a phonological learning task. J. Cogn. Neurosci. 18, 1029–1043. doi: 10.1162/jocn.2006.18.6.1029
Vallabha, G. K., and McClelland, J. L. (2007). Success and failure of new speech category learning in adulthood: consequences of learned Hebbian attractors in topographic maps. Cogn. Affect. Behav. Neurosci. 7, 53–73. doi: 10.3758/CABN.7.1.53
Visscher, K. M., Kaplan, E., Kahana, M. J., and Sekuler, R. (2007). Auditory short-term memory behaves like visual short-term memory. PLoS Biol. 5:e56. doi: 10.1371/journal.pbio.0050056
Wagenmakers, E. J., and Farrell, S. (2004). AIC model selection using Akaike weights. Psychon. Bull. Rev. 11, 192–196. doi: 10.3758/BF03206482
Waldron, E. M., and Ashby, F. G. (2001). The effects of concurrent task interference on category learning: evidence for multiple category learning systems. Psychon. Bull. Rev. 8, 168–176. doi: 10.3758/BF03196154
Waldschmidt, G. A., and Ashby, F. G. (2011). Cortical and striatal contributions to automaticity in information-integration categorization. Neuroimage 56, 1791–1802. doi: 10.1016/j.neuroimage.2011.02.011
Wang, Y., Jongman, A., and Sereno, J. A. (2003). Acoustic and perceptual evaluation of Mandarin tone productions before and after perceptual training. J. Acoust. Soc. Am. 113, 1033–1043. doi: 10.1121/1.1531176
Wechsler, D. (2008). Wechsler Adult Intelligence Scale®; 4th Edition (WAIS®;-IV). San Antonio, TX: Harcourt Assessment.
West, R. L. (1996). An application of prefrontal cortex function theory to cognitive aging. Psychol. Bull. 120, 272. doi: 10.1037/0033-2909.120.2.272
Wickens, T. D. (1982). Models for Behavior: Stochastic Processes in Psychology. San Francisco, CA: W. H. Freeman.
Wilson, C. J. (1995). The Contribution of Cortical Neurons to the Firing Pattern of Striatal Spiny Neurons. Cambridge, MA: MIT Press.
Yeterian, E., and Pandya, D. (1998). Corticostriatal connections of the superior temporal region in rhesus monkeys. J. Comp. Neurol. 399, 384–402. doi: 10.1002/(SICI)1096-9861(19980928)399:3<384::AID-CNE7>3.0.CO;2-X
Zeithamova, D., and Maddox, W. T. (2006). Dual task interference in perceptual category learning. Mem. Cogn. 34, 387–398. doi: 10.3758/BF03193416
Zeithamova, D., and Maddox, W. T. (2007). The role of visuo-spatial and verbal working memory in perceptual category learning. Mem. Cogn. 35, 1380–1398. doi: 10.3758/BF03193609
Zeithamova, D., Maddox, W. T., and Schnyer, D. M. (2008). Dissociable prototype learning systems: evidence from brain imaging and behavior. J. Neurosci. 28, 13194–13201. doi: 10.1523/JNEUROSCI.2915-08.2008
Zhang, Y., Kuhl, P. K., Imada, T., Iverson, P., Pruitt, J., Stevens, E. B.,et al. (2009). Neural signatures of phonetic learning in adulthood: a magnetoencephalography study. Neuroimage 46, 226–240. doi: 10.1016/j.neuroimage.2009.01.028
Keywords: dual-learning systems, procedural learning, reflective, reflexive, aging, depression, computational modeling
Citation: Chandrasekaran B, Koslov SR and Maddox WT (2014) Toward a dual-learning systems model of speech category learning. Front. Psychol. 5:825. doi: 10.3389/fpsyg.2014.00825
Received: 01 March 2014; Accepted: 10 July 2014;
Published online: 31 July 2014.
Edited by:
Caren Rotello, University of Massachusetts, USAReviewed by:
Carol Seger, Colorado State University, USAAndrew L. Cohen, University of Massachusetts, USA
Copyright © 2014 Chandrasekaran, Koslov and Maddox. This is an open-access article distributed under the terms of the Creative Commons Attribution License (CC BY). The use, distribution or reproduction in other forums is permitted, provided the original author(s) or licensor are credited and that the original publication in this journal is cited, in accordance with accepted academic practice. No use, distribution or reproduction is permitted which does not comply with these terms.
*Correspondence: Bharath Chandrasekaran, SoundBrain Lab, Department of Communication Sciences and Disorders, The University of Texas at Austin, 1 University Station, A1100, Austin, TX 78712, USA e-mail: bchandra@utexas.edu; W. T. Maddox, Department of Psychology, The University of Texas at Austin, 1 University Station, A8000, Austin, TX 78712, USA e-mail: maddox@psy.utexas.edu
†Bharath Chandrasekaran and W. T. Maddox have contributed equally to this work.