- Department of Psychology, Western Washington University, Bellingham, WA, USA
Musicians have a more accurate temporal and tonal representation of auditory stimuli than their non-musician counterparts (Musacchia et al., 2007; Parbery-Clark et al., 2009a; Zendel and Alain, 2009; Kraus and Chandrasekaran, 2010). Musicians who are adept at the production and perception of music are also more sensitive to key acoustic features of speech such as voice onset timing and pitch. Together, these data suggest that musical training may enhance the processing of acoustic information for speech sounds. In the current study, we sought to provide neural evidence that musicians process speech and music in a similar way. We hypothesized that for musicians, right hemisphere areas traditionally associated with music are also engaged for the processing of speech sounds. In contrast we predicted that in non-musicians processing of speech sounds would be localized to traditional left hemisphere language areas. Speech stimuli differing in voice onset time was presented using a dichotic listening paradigm. Subjects either indicated aural location for a specified speech sound or identified a specific speech sound from a directed aural location. Musical training effects and organization of acoustic features were reflected by activity in source generators of the P50. This included greater activation of right middle temporal gyrus and superior temporal gyrus in musicians. The findings demonstrate recruitment of right hemisphere in musicians for discriminating speech sounds and a putative broadening of their language network. Musicians appear to have an increased sensitivity to acoustic features and enhanced selective attention to temporal features of speech that is facilitated by musical training and supported, in part, by right hemisphere homologues of established speech processing regions of the brain.
Introduction
Research investigating the neural mechanisms involved in the processing of music and language has expanded from Bever and Chiarello’s (1974) proposed hemispheric specialization to Tallal and Gaab’s (2006) identification of similar neural areas to the evolving neuroanatomical models of Hickok and Poeppel (2000, 2007). While numerous studies have focused on specialized neural networks for the processing of either speech or music (Peretz et al., 1994; Zatorre et al., 2002; Rogalsky et al., 2011), a growing body of work has revealed that the neural mechanisms involved in the perception and processing of music overlap with those for the perception and processing of speech (Sammler et al., 2007; Wong et al., 2007; Rogalsky et al., 2011; Schulze et al., 2011). Moreover, studies have demonstrated that musical training induces neural changes resulting in enhanced speech perception in musicians (Zatorre and Belin, 2001; Zatorre et al., 2002; Bever and Chiarello, 2009). Specifically, musical training enhances language processing by altering neural networks for perception and processing of speech (Thompson et al., 2003; Schön et al., 2004; Moreno and Besson, 2006; Besson et al., 2007; Parbery-Clark et al., 2009a; Shahin, 2011). In addition to enhancing activity in speech processing areas, musicians may also engage right hemisphere music processing areas for the perception of speech. The present study examined whether alterations stemming from musical training were lateralized to traditional left language areas or extended into right hemisphere homologues for speech processing.
The perception and processing of acoustic features such as onset time and pitch are common to speech and music (Tremblay et al., 2001; Reinke et al., 2003) and are represented hierarchically in the auditory cortex. The primary auditory cortex encodes onset time and pitch, with speech sounds processed mainly in the left auditory cortex (Zatorre and Belin, 2001; Zatorre, 2002). Musicians who engage left hemisphere auditory cortex during the processing and perception of these features during tasks involved in musical training may in turn enhance their ability to perceive temporal aspects of speech sounds (Shahin, 2011). Voice-onset-time (VOT), the duration of the delay between release of closure and start of voicing (Lisker and Abramson, 1964), is one of the most important temporal acoustic cues in speech because it carries linguistically and phonetically relevant information (Ott et al., 2011) that allows us to perceive the difference between a voiced (e.g., /b/) and voiceless (e.g., /p/) stop consonant (Chobert et al., 2012). VOT is also important to the development of phonological representations (Chobert et al., 2012). Studies have demonstrated that musicians are more sensitive to and process voiceless stimuli differently than their non-musician counterparts (Chobert et al., 2011; Ott et al., 2011). However, the neural basis of this difference has not been explored.
Perceptual categorization is another important component to both speech and music. The ability to categorize musical stimuli has been shown to predict categorization of speech stimuli, suggesting that the two processes share a common cognitive mechanism (Overy, 2003; Tallal and Gaab, 2006; Patel and Iversen, 2007; Wong et al., 2007). Speech categorization relies primarily on timbral contrasts and music categorization primarily on pitch contrasts. For example, in speech the range of possible vowel sounds is a continuum, but speakers of a language learn to separate this continuum into discrete vowels. Similarly, the range of possible frequencies in music is continuous, with musicians learning to categorize these frequencies into discrete notes. Musicians have also demonstrated a more accurate temporal and tonal representation of auditory stimuli than their non-musician counterparts (Musacchia et al., 2007; Parbery-Clark et al., 2009a; Zendel and Alain, 2009; Kraus and Chandrasekaran, 2010), though the actual mechanism behind this advantage is less obvious. Moreover, their temporal representations are less susceptible to the negative effects of background noise (Parbery-Clark et al., 2009a). Musical training has also been shown to provide advantages to perceptual and attentional mechanisms for language (Strait and Kraus, 2011). Components of Patel’s (2011) OPERA hypothesis such as: Overlap, Precision, Repetition, and Attention describe how musical training might create these advantages. First there is an anatomical overlap of neural areas that process acoustic features present in both speech and music. Secondly, music requires greater precision than speech and thus places a higher demand on overlapping neural areas. Finally, musical training requires repetition therefore continually engaging these neural areas that have also been shown to be associated with focused attention. Another possibility is that corticofugal mechanisms induce short and long term plasticity resulting in a transfer of training from music to language (Kraus and Chandrasekaran, 2010; Besson et al., 2011; Chobert et al., 2012). Musicians have demonstrated an advantage over non-musicians in their ability to recognize tonal variations in non-native speech sounds (Wong et al., 2007; Cooper and Wang, 2010; Perfors and Ong, 2012). This advantage can be attributed to formal musical training that emphasizes enhanced perception of pitch and may provide them an advantage when learning speech sounds.
When considered together, this literature suggests that musical training is related to neuroplastic changes to the language network as musicians’ become more sensitive to the acoustic features critical to both speech and music. We predict that musical training enhances speech perception and discrimination in musicians by engaging right hemisphere brain regions more typically associated with music processing. This prediction reflects our broader hypothesis that music and language are processed in partially overlapping networks and that the right hemisphere components of this network are enhanced by musical training. Here, we recorded electroencephalography (EEG) to address whether musicians’ engage neural areas that are not typically associated with left hemisphere dominant language networks when discriminating between phonemes differing in voice onset time. An analysis of cortical sources revealed greater right hemisphere engagement for musicians compared to non-musicians.
Materials and Methods
Subjects
Twelve right-handed (evaluated using the Oldfield Handedness Inventory, Oldfield, 1971) monolingual American-English speakers who reported normal hearing were recruited from the music department and general population at Western Washington University and divided into musician and non-musician groups. Musicians (n = 6) were required to have at least 5 years of continuous formal musical training (M = 9.17 years, SD = 2.11) and all played wind instruments. Non-musicians (n = 6) had no musical training and had never played a musical instrument. Participants ranged in age from 19 to 22 years (M = 20.25 years, SD = 0.83). All procedures were conducted with written consent from participants and with the approval of the Western Washington University Human Subjects Committee.
Stimuli
Auditory stimuli were presented at 75 dB via over-ear Sennheiser HD-595 using custom Visual Basic software that controlled the timing and added event markers to the EEG record for subsequent segmentation of individual data epochs. Four synthetic CV stimuli were created in Synthworks (Scion, R&D Inc.), with C consisting of either the voiced unaspirated /d/ or voiceless unaspirated /t/ followed by a 215 ms vowel /α/. The duration of the voiced consonant was 100 ms and the voiceless consonant was 45 ms.
Procedure
In keeping with previous studies (Belin et al., 2000; Parbery-Clark et al., 2009a,b; Kerlin et al., 2010) we used a dichotic listening task. In four tasks participants were presented with different speech sounds in each ear and instructed to attend to a specific aural location or to listen for a specific speech sound. The four tasks were (1) D Sound (2) T Sound (3) Right Ear and, (4) Left Ear. In the D and T Sound tasks, subjects were instructed to focus their attention on the /d/ and /t/ sound respectively regardless of the ear of presentation. In the Right and Left Ear tasks subjects were instructed to focus their attention on their right or left ear respectively. To minimize voiceless dominance, stimuli were onset-aligned rather than aligned to the noise burst and dichotic pairs consisted of all possible VOT (voiced/voiceless) combinations. For each task 120 stimulus pairs were presented consisting of 60 instances of each of the two combinations. In each condition, stimuli were shuffled in a pseudo-randomized order. The condition order was randomized for every subject. We collapsed our measures of performance and event related potentials (ERP) across all dichotic listening tasks. The full analysis of the individual behavioral tasks will be presented in a separate report.
EEG Data Acquisition
Electroenchapalographic signals were recorded continuously from 64 Ag/AgCl active electrodes (Active 2 System, Biosemi, Amsterdam, Netherlands) mounted in an elastic headcap according to a 10–10 configuration (Chatrian et al., 1985). Signals were conducted using a saline-based conductive gel (Signa Gel) and all offsets were maintained below 20 uV. Unreferenced signals were amplified and digitized at 512 Hz using Biosemi Active Two amplifiers and acquisition software. Although electromyography activity was not recorded, all participants were given specific instructions to refrain from moving during the experiment and participants were monitored for evidence of unintended or unconscious movements. The experimenters did not observe any overt movement.
ERP Analysis
Data processing and visualization was accomplished using the EEGLab toolbox running under Matlab 7.0. Continuous data from each participant were referenced to the average potential of all electrodes. For all conditions EEG epochs were extracted in the interval from -100 to 500 ms around the onset of the stimulus. Epoched data was bandpass filtered between 1 and 20 Hz. Trials containing large signals exceeding 100 uV were automatically identified, manually inspected and rejected if they were judged to contain artifacts. Trials were also inspected for EMG contamination. Eye blink and eye movement artifacts were identified and removed in EEGLab (Delorme and Makeig, 2004) using an established independent component analysis (ICA) approach (Jung et al., 2000). For each participant, epoched data were linearly unmixed or decomposed into 64 maximally independent components. Eye blink and other artifact related components were identified based on their characteristic spatiotemporal pattern. The contribution of these components was set to zero and the data were projected back into the original sensor space. This procedure removes the contribution of the artifact without altering the evoked brain response thereby elimination the need to discard large numbers due to excessive blinking (Jung et al., 2000). The resulting trial epochs were used to compute the average evoked response for each participant. A qualitative description of ERP components was based on visual inspection of the grand average ERP, the associated scalp distributions and the global field power of the grand average (see Figure 1).
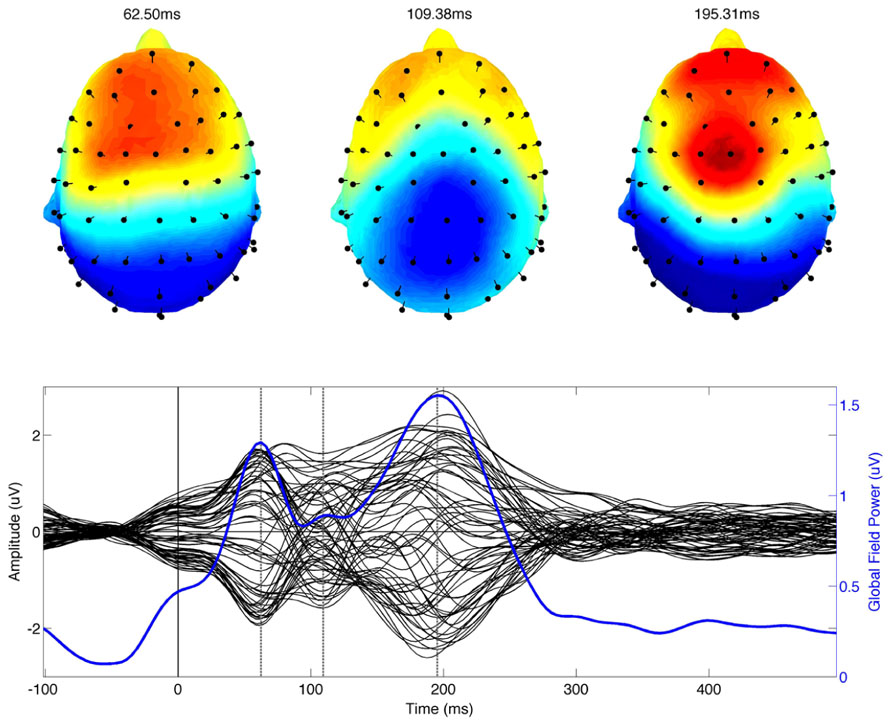
FIGURE 1. The combined grand average of musicians and non-musicians of the event related potential is shown in the bottom panel. The time series from each of the 64 recording electrodes are shown in black on the same axis. The global field power computed using all electrodes is shown in blue. The time of identified ERP (P50m, N100, and P200) components is indicated by vertical dotted lines. The scalp topography of each ERP component is shown in the top row of the figure.
Because our primary hypothesis predicts group differences in ERP source generators for musicians and controls, we performed analysis on cortical sources estimated using standardized low-resolution brain electromagnetic tomography (sLORETA) (Pascual-Marqui et al., 1994, 2002). Before comparing the source distributions, we first identified time points of interest by statistically comparing the ERP time series from the musician and control groups. A non-parametric permutation test using 10000 permutations [as implemented in EEGLAB function “statcond” (Delorme, 2006)] was used to compare ERP of musicians and non-musicians averaged across four electrode montages reflecting our hypothesis that language related activity would originate from left and right inferior frontal and posterior temporal regions. The electrodes included in the montage over the inferior frontal region were AF3, AF7, F5, F7, FC5, and FT7 on the left and AF4, AF8, F6, F8, FC6, and FT8 on the right. For the temporal parietal regions we selected electrodes CP1, CP3, CP5, P1, P3, and P5 on the left, and CP2, CP4, CP6, P2, P4, and P6 on the right. A separate permutation test was run to compare ERP amplitude between musicians and non-musicians for each montage and time points between 3 to 300 ms. This time range was selected because it represents the period during which early auditory processing occurs. We controlled for multiple comparisons using false discovery rate (Benjamini and Yekutieli, 2001) to achieve a corrected p < 0.05. Analysis of the cortical sources was performed at intervals showing significant differences between groups.
To obtain estimates of cortical generators, we applied sLORETA to the average scalp-recorded electric potential distribution of each participant to compute the distribution of current density on a template brain in Talairach coordinates (Talairach and Tournoux, 1988). A parametric two sample t-tests was computed on the amplitude normalized and log-transformed sLORETA images (Thatcher et al., 2005). Tests of skewness confirmed that the log transform generated images that approximated a normal distribution (mean skewness = -0.40) for all participants. Multiple comparisons (voxels = 6239) were controlled using false discovery rate with a corrected p < 0.05. The corrected t threshold in the statistical parametric map was 6.43. The location of cortical regions in which voxels exceeded this threshold was determined using Talairach atlas information available in the LORETA software.
Results
Behavioral Results
Discrimination performance of musicians and non-musicians was measured across all stimuli and tasks. Mean correct responses (musicians, M = 256; non-musicians, M = 264) and a between subjects t-test performed using SPSS showed that musical training did not improve the ability to detect correct stimuli based upon differences in voice onset time [t(10) = 0.470, p = 0.649].
ERP Analysis
Three dominant component peaks were observed in the grand average ERP data and the global field power (Figure 1; lower panel). The scalp distribution of the time of the component peaks corresponds well with the expected P50-N1-P2 complex (Figure 1). The latency of the peak amplitude of the three components was 62.5, 109.38, and 195.31 ms respectively.
Non-parametric tests revealed significant differences in the right temporal parietal montage at three consecutive time points from 46.8 to 54.6 ms, at the approximate time of the P50 ERP component. Activity was significantly more positive for the musicians than the non-musicians. Musicians also showed significantly greater activity in the right superior temporal gyrus (STG; BA 22) and middle temporal gyrus (MTG; BA 39) during the time interval from 46.8 to 54.6 ms. Voxels showing significant differences are shown in Figure 2. The maximum T value of 7.46 was Talairach location at X = 50, Y = -57, Z = 17 in Brodmann area 22.
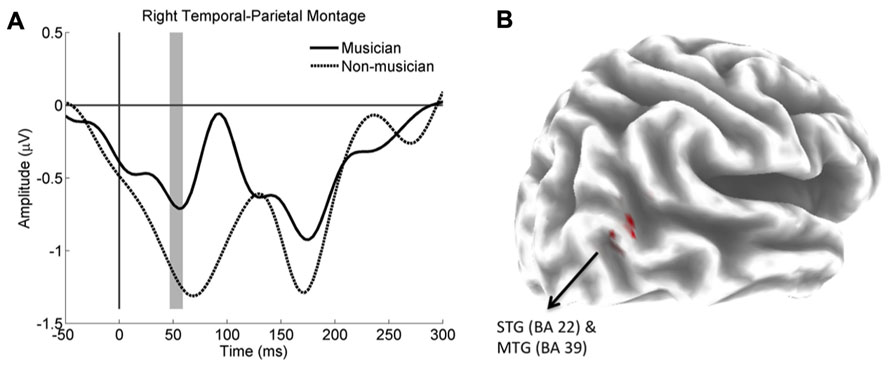
FIGURE 2. (A) Group average ERPs for the right temporal parietal montage. Compare to non-musicians (dotted line), ERP for musicians (solid line) was significantly more positive within a contiguous window 46.8 to 54.6 ms (gray shading). (B) Shows the significant difference between the sLORETA images of the musicians and non-musicians. Musicians had significantly greater activity in the right superior temporal gyrus (STG) and middle temporal gyrus (MTG). Differences are shown in red on a partially inflated template brain.
Although substantial differences in ERP amplitude were also observed at times corresponding approximately to the N1 (~100 ms) and P2 (~175 ms), the differences were not significant after correcting for multiple comparisons.
Discussion
The purpose of this study was to examine how musical training affects the neural organization and representation of speech. We were specifically interested in the early processing of acoustic components of the speech signal. Our hypothesis was that musical training induces alterations to neural areas associated with both music and language, thereby modifying the language network of musicians. In support of our hypothesis, ERP differences between musicians and non-musicians were observed around the time of the P50 response. Analysis of cortical sources revealed greater activity in right hemisphere for musicians during this time frame at the posterior junction of the superior and middle temporal gyri.
Right hemisphere activity in MTG and STG in musicians may reflect enhanced processing of speech sounds. The P50 originates in the STG (Eggermont and Ponton, 2002) and reflects early auditory neurophysiological processes (Ott et al., 2011), in particular, early speech-specific processing of phonemes and syllables (Dehaene-Lambertz et al., 2005). Previous research has shown regions in the superior temporal lobes responsive to perceiving speech sounds (Wise et al., 1991; Mummery et al., 1999; Belin et al., 2000; Binder et al., 2000; Scott et al., 2000). More specifically, the STG and superior temporal sulcus (STS) are sensitive to complex spectrotemporal information (Zatorre et al., 1992; Binder et al., 2000; Scott et al., 2000; Poeppel, 2003). It had been presumed that activity in the temporal lobe regions was lateralized to left dominant STG and STS for phonetic and phonological speech perception (Liebenthal et al., 2005; Möttönen et al., 2006; Hickok and Poeppel, 2007). The major finding of the present study is greater activation of right MTG and STG for musicians. Traditionally, MTG has been implicated in lexical-semantic processing (Demonet et al., 1992; Vandenberghe et al., 1996; Binder et al., 1997; Dronkers et al., 2004). However, more recent research implicates the MTG in phonemic discrimination tasks (Ashtari et al., 2004) and, contrary to previous research, right hemisphere sensitivity to phonemic information may not be limited to a lexical content (Wolmetz et al., 2011). Moreover, activation of the right MTG region at the time of the P50 component of the ERP is compatible with a putative role in encoding of early acoustic features (Schneider et al., 2002).
Another possibility is that the activity we report in STG at 50 ms reflects an enhanced role of right hemisphere for selective attention (Shahin, 2011). The P50 amplitude is thought to reflect top down attentional change and processes associated with working memory, such as our ability to selectively attend to salient stimuli and inhibit processing of irrelevant information (Light and Braff, 2003; Beratis et al., 2009; Sur and Sinha, 2009). Selective attention mechanisms are necessary in the processing of noisy auditory scene situations (Parbery-Clark et al., 2009b; Kerlin et al., 2010) such as the dichotic listening task used in the present study. If selective attention abilities are enhanced in musicians, it would impact how relevant and irrelevant signals are organized in working memory (Sreenivasan and Jha, 2007) and possibly promote relevant acoustical signal intensity while simultaneously suppressing interfering noise (Kerlin et al., 2010). Musicians’ focus on and direct their attention to small changes in acoustical features such as pitch and onset time, thereby developing an acute processing of spectrotemporal acoustical information (Schneider et al., 2002; Marie et al., 2012). This enhanced representation of acoustical information facilitates acoustical feature binding and analysis of the acoustic scene (Treisman and Gelade, 1980; Shinn-Cunningham and Best, 2008), particularly at P50 (Shahin, 2011). Musicians improved performance over non-musicians in auditory tasks requiring focused attention (Strait et al., 2010) may result from improved auditory scene analysis skills that have been shaped by selective attention mechanisms via enhanced acuity to acoustical features (Shahin, 2011). Auditory scene analysis is also influenced by cognitive mechanisms associated with working memory and target detection, both of which are required by musicians when attentively listening to music (Janata et al., 2002) and may lead to improved concurrent sound segregation (Shahin, 2011). Furthermore, segregation of sound is important during dichotic listening tasks and may account for musicians’ recruitment of the right STG.
Previous research examining differences in the processing and analysis of acoustic features between musicians and non-musicians show both functional and structural alterations (Pantev et al., 1998, 2003; Magne et al., 2003; Moreno and Besson, 2005; Chartrand and Belin, 2006; Moreno and Besson, 2006; Baumann et al., 2008) even after only a short exposure to musical training (Magne et al., 2006; Moreno et al., 2009). Taken together, these studies elucidate musicians’ expertise at processing spectrotemporally complex acoustic information. The present study complements this growing body of work by demonstrating recruitment of right hemisphere in musicians for discriminating speech sounds. The increased activation of speech related areas with respect to P50 demonstrate a putative broadening of the speech processing network induced by musical training. We believe these results may reflect enhanced selective attention and increased sensitivity to acoustic features of speech that is facilitated by musical training and supported, in part, by right hemisphere homologues of established speech processing regions of the brain.
In addition to the P50, evidence supports enhancement of later ERP components resulting from musical training. P50, N1, and P2 components have been found to be important for auditory analysis and coding of low-level acoustical features and representing higher-level complex spectrotemporal sound features (Sharma and Dorman, 1999; Steinschneider et al., 1999, 2005; Sharma et al., 2000; Zaehle et al., 2007; Shahin, 2011; Ott et al., 2011). Moreover, earlier occurring components such as the P50 may influence later components such as the N1 and P2 (Gilbert et al., 2001). Previously, N1 (Sharma et al., 2000; Zaehle et al., 2007; Ott et al., 2011) and P2 amplitudes (Tremblay et al., 2001; Tremblay and Kraus, 2002) differed either for voiced versus voiceless stimuli or in musicians versus non-musicians. Although preliminary analysis of the N1 and P2 components in the present data revealed differences between musicians and non-musicians, these findings did not survive statistical correction for multiple comparisons. Thus although the current work supports that musical training influences early acoustic processing, subsequent studies may reveal more subtle differences in later processing as well.
The lack of a behavioral advantage for musicians may stem from the difficulty of the dichotic listening task. Both groups performed at just better than chance level suggesting that they found the task very difficult. Although the dichotic listening task was to ensure attentive auditory processing, future studies may consider altering task demands to be more sensitive to potential performance differences. Another potential limitation of the present study is the small sample size. Although, recent studies that performed between subject experiments with a sample size of 10 or fewer per group also report significant and robust neural differences (Schön et al., 2004; Magne et al., 2006; Besson et al., 2007; Santos et al., 2007), it is important to consider the relatively small number of participants in our study when interpreting these results more broadly.
The role of the right hemisphere and its contribution to speech perception is still a matter of debate. Obleser and Eisner (2009) argue that the right hemisphere plays no role in speech perception. Similarly, left temporal lateralization is supported by a review of studies in which contrasts were related to phoneme-specific processing (Wolmetz et al., 2011). In contrast, however, Hickok and Poeppel (2007) have strongly argued for inclusion of the right hemisphere based upon bilateral activation during speech perception tasks. The present results support this latter position and suggest the possibility that right hemisphere MTG/STG activation in musicians during discrimination of speech consonants differing in VOT results from musical training induced sensitivity and enhanced selective attention to temporal features within the speech signal.
Conflict of Interest Statement
The authors declare that the research was conducted in the absence of any commercial or financial relationships that could be construed as a potential conflict of interest.
References
Ashtari, M., Lencz, T., Zuffante, P., Bilder, R., Clarke, T., Diamond, A., et al. (2004). Left middle temporal gyrus activation during a phonemic discrimination task. Neuroreport 15, 389–393. doi: 10.1097/00001756-200403010-00001
Baumann, S., Meyer, M., and Jäncke, L. (2008). Enhancement of auditory-evoked potentials in musicians reflects an influence of expertise but not selective attention. J. Cogn. Neurosci. 20, 2238–2249. doi: 10.1162/jocn.2008.20157
Belin, P., Zatorre, R. J., Lafaille, P., Ahad, P., and Pike, B. (2000). Voice-selective areas in human auditory cortex. Nature 403, 309–312. doi: 10.1038/35002078
Benjamini, Y., and Yekutieli, D. (2001). The control of the false discovery rate in multiple testing under dependency. Ann. Stat. 29, 1165–1188.
Beratis, I. N., Rabavilas, A., Nanou, E. D., Hountala, C., Maganioti, A. E., Capsalis, C. N., et al. (2009). Effect of initiation-inhibition and handedness on the patterns of the P50 event-related potential component: a low resolution electromagnetic tomography study. Behav. Brain Funct. 5, 1–10. doi: 10.1186/1744-9081-5-51
Besson, M., Chobert, J., and Marie, C. (2011). Transfer of training between music and speech: common processing, attention, and memory. Front. Psychol. 2:94. doi: 10.3389/fpsyg.2011.00094
Besson, M., Schön, D., Moreno, S., Santos, A., and Magne, C. (2007). Influence of musical expertise and musical training on pitch processing in music and language. Restor. Neurol. Neurosci. 25, 399–410.
Bever, T. G., and Chiarello, R. J. (1974). Cerebral dominance in musicians and nonmusicians. Science 185, 537–539. doi: 10.1126/science.185.4150.537
Bever, T. G., and Chiarello, R. (2009). Cerebral dominance in musicians and nonmusicians. J. Neuropsychiatry Clin. Neurosci. 21, 94–97. doi: 10.1176/appi.neuropsych.21.1.94
Binder, J. R., Frost, J. A., Hammeke, T. A., Bellgowan, P. S. F., Springer, J. A., Kaufman, J. N., et al. (2000). Human temporal lobe activation by speech and nonspeech sounds. Cereb. Cortex 10, 512–528. doi: 10.1093/cercor/10.5.512
Binder, J. R., Frost, J. A., Hammeke, T. A., Cox, R. W., Rao, S. M., and Prieto, T. (1997). Human brain language areas identified by functional MRI. J. Neurosci. 17, 353–362.
Chartrand, J. P., and Belin, P. (2006). Superior voice timbre processing in musicians. Neurosci. Lett. 405, 164–167. doi: 10.1016/j.neulet.2006.06.053
Chatrian, G. E., Lettich, E., and Nelson, P. L. (1985). Ten percent electrode system for topographic studies of spontaneous and evoked EEG activity. Am. J. EEG Technol. 25, 83–92.
Chobert, J., François, C., Velay, J. L., and Besson, M. (2012). Twelve months of active musical training in 8-to 10-year-old children enhances the preattentive processing of syllabic duration and voice onset time. Cereb. Cortex doi: 10.1093/cercor/bhs377 [Epub ahead of print].
Chobert, J., Marie, C., Francois, C., Schon, D., and Besson, M. (2011). Enhanced passive and active processing of syllables in musician children. J. Cogn. Neurosci. 23, 3874–3887. doi: 10.1162/jocn_a_00088
Cooper, A., and Wang, Y. (2010). Can musical aptitude and experience predict success in non-native tone word learning? J. Acoust. Soc. Am. 128, 2478. doi: 10.1121/1.3508890
Dehaene-Lambertz, G., Pallier, C., Serniclaes, W., Sprenger-Charolles, L., Jobert, A., and Dehaene, S. (2005). Neural correlates of switching from auditory to speech perception. Neuroimage 24, 21–33. doi: 10.1016/j.neuroimage.2004.09.039
Delorme, A. (2006). “Statistical methods,” in Encyclopedia of Medical Devices and Instrumentation, ed. J. Webster (Hoboken: Wiley Interscience), 240–264.
Delorme, A., and Makeig, S. (2004). EEGLAB: an open source toolbox for analysis of single-trial EEG dynamics including independent component analysis. J. Neurosci. Methods 134, 9–21. doi: 10.1016/j.jneumeth.2003.10.009
Demonet, J. F., Chollet, F., Ramsay, S., Cardebat, D., Nespoulous, J. L., Wise, R., et al. (1992). The anatomy of phonological and semantic processing in normal subjects. Brain 115, 1753–1768. doi: 10.1093/brain/115.6.1753
Dronkers, N. F., Wilkins, D. P., Van Valin R. D. Jr., Redfern, B. B., and Jaeger, J. J. (2004). Lesion analysis of the brain areas involved in language comprehension. Cognition 92, 145–177. doi: 10.1016/j.cognition.2003.11.002
Eggermont, J. J., and Ponton, C. W. (2002). The neurophysiology of auditory perception: from single units to evoked potentials. Audiol. Neurotol. 7, 71–99. doi: 10.1159/000057656
Gilbert, C. D., Sigman, M., and Crist, R. E. (2001). The neural basis of perceptual learning. Neuron 31, 681–697. doi: 10.1016/S0896-6273(01)00424-X
Hickok, G., and Poeppel, D. (2000). Towards a functional neuroanatomy of speech perception. Trends Cogn. Sci. 4, 131–138. doi: 10.1016/S1364-6613(00)01463-7
Hickok, G., and Poeppel, D. (2007). The cortical organization of speech processing. Nat. Rev. Neurosci. 8, 393–402. doi: 10.1038/nrn2113
Janata, P., Tillmann, B., and Bharucha, J. J. (2002). Listening to polyphonic music recruits domain-general attention and working memory circuits. Cogn. Affect. Behav. Neurosci. 2, 121–140. doi: 10.3758/CABN.2.2.121
Jung, T.-P., Makeig, S., Westerfield, M., Townsend, J., Courchesne, E., and Sejnowski, T. J. (2000). Removal of eye activity artifacts from visual event-related potentials in normal and clinical subjects. Clin. Neurophysiol. 111, 1745–1758. doi: 10.1016/S1388-2457(00)00386-2
Kerlin, J. R., Shahin, A. J., and Miller, L. M. (2010). Attentional gain control of ongoing cortical speech representations in a “cocktail party.” J. Neurosci. 30, 620–628. doi: 10.1523/JNEUROSCI.3631-09.2010
Kraus, N., and Chandrasekaran, B. (2010). Music training for the development of auditory skills. Nat. Rev. Neurosci. 11, 599–605. doi: 10.1038/nrn2882
Liebenthal, E., Binder, J. R., Spitzer, S. M., Possing, E. T., and Medler, D. A. (2005). Neural substrates of phonemic perception. Cereb. Cortex 15, 1621–1631. doi: 10.1093/cercor/bhi040
Light, G. A., and Braff, D. L. (2003). Sensory gating deficits in schizophrenia: can we parse the effects of medication, nicotine use, and changes in clinical status? Clin. Neurosci. Res. 3, 47–54. doi: 10.1016/S1566-2772(03)00018-5
Lisker, L., and Abramson, A. S. (1964). A cross-language study of voicing in initial stops: acoustical measurements. Word 20, 384–422.
Magne, C., Schön, D., and Besson, M. (2003). Prosodic and melodic processing in adults and children. Ann. N. Y. Acad. Sci. 999, 461–476. doi: 10.1196/annals.1284.056
Magne, C., Schön, D., and Besson, M. (2006). Musician children detect pitch violations in both music and language better than nonmusician children: behavioral and electrophysiological approaches. J. Cogn. Neurosci. 18, 199–211. doi: 10.1162/jocn.2006.18.2.199
Marie, C., Kujala, T., and Besson, M. (2012). Musical and linguistic expertise influence preattentive and attentive processing of non-speech sounds. Cortex 48, 447–457. doi: 10.1016/j.cortex.2010.11.006
Moreno, S., and Besson, M. (2005). Influence of musical training on pitch processing: event-related brain potential studies of adults and children. Ann. N. Y. Acad. Sci. 1060, 93–97. doi: 10.1196/annals.1360.054
Moreno, S., and Besson, M. (2006). Musical training and language – related brain electrical activity in children. Psychophysiology 43, 287–291. doi: 10.1111/j.1469-8986.2006.00401.x
Moreno, S., Marques, C., Santos, A., Santos, M., Castro, S. L., and Besson, M. (2009). Musical training influences linguistic abilities in 8-year-old children: more evidence for brain plasticity. Cereb. Cortex 19, 712–723. doi: 10.1093/cercor/bhn120
Möttönen, R., Calvert, G. A., Jääskeläinen, I. P., Matthews, P. M., Thesen, T., Tuomainen, J., et al. (2006). Perceiving identical sounds as speech or non-speech modulates activity in the left posterior superior temporal sulcus. Neuroimage 30, 563–569. doi: 10.1016/j.neuroimage.2005.10.002
Mummery, C. J., Ashburner, J., Scott, S. K., and Wise, R. J. S. (1999). Functional neuroimaging of speech perception in six normal and two aphasic subjects. J. Acoust. Soc. Am. 106, 449. doi: 10.1121/1.427068
Musacchia, G., Sams, M., Skoe, E., and Kraus, N. (2007). Musicians have enhanced subcortical auditory and audiovisual processing of speech and music. Proc. Nat. Acad. Sci. U.S.A. 104, 15894–15898. doi: 10.1073/pnas.0701498104
Obleser, J., and Eisner, F. (2009). Pre-lexical abstraction of speech in the auditory cortex. Trends Cogn. Sci. 13, 14–19. doi: 10.1016/j.tics.2008.09.005
Oldfield, R. C. (1971). The assessment and analysis of handedness: the Edinburgh inventory. Neuropsychologia 9, 97–113. doi: 10.1016/0028-3932(71)90067-4
Ott, C. G. M., Langer, N., Oechslin, M. S., Meyer, M., and Jäncke, L. (2011). Processing of voiced and unvoiced acoustic stimuli in musicians. Front. Psychol. 2:195. doi: 10.3389/fpsyg.2011.00195
Overy, K. (2003). Dyslexia and music. Ann. N. Y. Acad. Sci. 999, 497–505. doi: 10.1196/annals.1284.060
Pantev, C., Oostenveld, R., Engelien, A., Ross, B., Roberts, L. E., and Hoke, M. (1998). Increased auditory cortical representation in musicians. Nature 392, 811–814. doi: 10.1038/33918
Pantev, C., Ross, B., Fujioka, T., Trainor, L. J., Schulte, M., and Schulz, M. (2003). Music and learning? Induced cortical plasticity. Ann. N. Y. Acad. Sci. 999, 438–450. doi: 10.1196/annals.1284.054
Parbery-Clark, A., Skoe, E., and Kraus, N. (2009a). Musical experience limits the degradative effects of background noise on the neural processing of sound. J. Neurosci. 29, 14100–14107. doi: 10.1523/JNEUROSCI.3256-09.2009
Parbery-Clark, A., Skoe, E., Lam, C., and Kraus, N. (2009b). Musician enhancement for speech-in-noise. Ear Hear. 30, 653–661. doi: 10.1097/AUD.0b013e3181b412e9
Pascual-Marqui, R. D., Esslen, M., Kochi, K., and Lehmann, D. (2002). Functional imaging with low-resolution brain electromagnetic tomography (LORETA): a review. Methods Find. Exp. Clin. Pharmacol. 24(suppl. C), 91–95.
Pascual-Marqui, R. D., Michel, C. M., and Lehmann, D. (1994). Low resolution electromagnetic tomography: a new method for localizing electrical activity in the brain. Int. J. Psychophysiol. 18, 49–65. doi: 10.1016/0167-8760(84)90014-X
Patel, A. D. (2011). Why would musical training benefit the neural encoding of speech? The OPERA hypothesis. Front. Psychol. 2:142. doi: 10.3389/fpsyg.2011.00142
Patel, A. D., and Iversen, J. R. (2007). The linguistic benefits of musical abilities. Trends Cogn. Sci. 11, 369–372. doi: 10.1016/j.tics.2007.08.003
Peretz, I., Kolinsky, R., Tramo, M., Labrecque, R., Hublet, C., Demeurisse, G., et al. (1994). Functional dissociations following bilateral lesions of auditory cortex. Brain 117, 1283–1301. doi: 10.1093/brain/117.6.1283
Perfors, A., and Ong, J. (2012). “Musicians are better at learning non-native sound contrasts even in non-tonal languages,” in Proceedings of the 34th Annual Conference of the Cognitive Science Society, eds N. Miyake, D. Peebles, and R. P. Cooper (Austin: Cognitive Science Society), 839–844.
Poeppel, D. (2003). The analysis of speech in different temporal integration windows: cerebral lateralization as “asymmetric sampling in time”. Speech Commun. 41, 245–255. doi: 10.1016/S0167-6393(02)00107-3
Reinke, K. S., He, Y., Wang, C., and Alain, C. (2003). Perceptual learning modulates sensory evoked response during vowel segregation. Cogn. Brain Res. 17, 781–791. doi: 10.1016/S0926-6410(03)00202-7
Rogalsky, C., Rong, F., Saberi, K., and Hickok, G. (2011). Functional anatomy of language and music perception: temporal and structural factors investigated using functional magnetic resonance imaging. J. Neurosci. 31, 3843–3852. doi: 10.1523/JNEUROSCI.4515-10.2011
Sammler, D., Grigutsch, M., Fritz, T., and Koelsch, S. (2007). Music and emotion: electrophysiological correlates of the processing of pleasant and unpleasant music. Psychophysiology 44, 293–304. doi: 10.1111/j.1469-8986.2007.00497.x
Santos, A., Joly-Pottuz, B., Moreno, S., Habib, M., and Besson, M. (2007). Behavioural and event-related potentials evidence for pitch discrimination deficits in dyslexic children: improvement after intensive phonic intervention. Neuropsychologia 45, 1080–1090. doi: 10.1016/j.neuropsychologia.2006.09.010
Schneider, P., Scherg, M., Dosch, H. G., Specht, H. J., Gutschalk, A., and Rupp, A. (2002). Morphology of heschl’s gyrus reflects enhanced activation in the auditory cortex of musicians. Nat. Neurosci. 5, 688–694. doi: 10.1038/nn871
Schön, D., Magne, C., and Besson, M. (2004). The music of speech: music training facilitates pitch processing in both music and language. Psychophysiology 41, 341–349. doi: 10.1111/1469-8986.00172.x
Scott, S. K., Blank, C. C., Rosen, S., and Wise, R. J. (2000). Identification of a pathway for intelligible speech in the left temporal lobe. Brain 123, 2400–2406. doi: 10.1093/brain/123.12.2400
Schulze, K., Zysset, S., Mueller, K., Friederici, A. D., and Koelsch, S. (2011). Neuroarchitecture of verbal and tonal working memory in nonmusicians and musicians. Hum. Brain Mapp. 32, 771–783. doi: 10.1002/hbm.21060
Shahin, A. J. (2011). Neurophysiological influence of musical training on speech perception. Front. Psychol. 2:126. doi: 10.3389/fpsyg.2011.00126
Sharma, A., and Dorman, M. F. (1999). Cortical auditory evoked potential correlates of categorical perception of voice-onset time. J. Acoust. Soc. Am. 106, 1078. doi: 10.1121/1.428048
Sharma, A., Marsh, C. M., and Dorman, M. F. (2000). Relationship between N1 evoked potential morphology and the perception of voicing. J. Acoust. Soc. Am. 108, 3030. doi: 10.1121/1.1320474
Shinn-Cunningham, B. G., and Best, V. (2008). Selective attention in normal and impaired hearing. Trends Amplif. 12, 283–299. doi: 10.1177/1084713808325306
Sreenivasan, K. K., and Jha, A. P. (2007). Selective attention supports working memory maintenance by modulating perceptual processing of distractors. J. Cogn. Neurosci. 19, 32–41. doi: 10.1162/jocn.2007.19.1.32
Steinschneider, M., Volkov, I. O., Fishman, Y. I., Oya, H., Arezzo, J. C., and Howard, M. A. (2005). Intracortical responses in human and monkey primary auditory cortex support a temporal processing mechanism for encoding of the voice onset time phonetic parameter. Cereb. Cortex 15, 170–186. doi: 10.1093/cercor/bhh120
Steinschneider, M., Volkov, I. O., Noh, M. D., Garell, P. C., and Howard, M. A. (1999). Temporal encoding of the voice onset time phonetic parameter by field potentials recorded directly from human auditory cortex. J. Neurophysiol. 82, 2346–2357.
Strait, D. L., and Kraus, N. (2011). Can you hear me now? Musical training shapes functional brain networks for selective auditory attention and hearing speech in noise. Front. Psychol. 2:113. doi: 10.3389/fpsyg.2011.00113
Strait, D. L., Kraus, N., Parbery-Clark, A., and Ashley, R. (2010). Musical experience shapes top-down auditory mechanisms: evidence from masking and auditory attention performance. Hear. Res. 261, 22–29. doi: 10.1016/j.heares.2009.12.021
Sur, S., and Sinha, V. K. (2009). Event-related potential: an overview. Ind. Psychiatry J. 18, 70–73. doi: 10.4103/0972-6748.57865
Talairach, J., and Tournoux, P. (1988). Co-Planar Stereotaxic Atlas of the Human Brain. 3-Dimensional Proportional System: An Approach to Cerebral Imaging. New York: Thieme.
Tallal, P., and Gaab, N. (2006). Dynamic auditory processing, musical experience and language development. Trends Neurosci. 29, 382–390. doi: 10.1016/j.tins.2006.06.003
Thatcher, R. W., North, D., and Biver, C. (2005). Parametric vs. non-parametric statistics of low resolution electromagnetic tomography (LORETA). Clin. EEG Neurosci. 36, 1–8. doi: 10.1177/155005940503600103
Thompson, W. F., Schellenberg, E. G., and Husain, G. (2003). Perceiving prosody in speech. Ann. N. Y. Acad. Sci. 999, 530–532. doi: 10.1196/annals.1284.067
Treisman, A. M., and Gelade, G. (1980). A feature-integration theory of attention. Cogn. Psychol. 12, 97–136. doi: 10.1016/0010-0285(80)90005-5
Tremblay, K., Kraus, N., McGee, T., Ponton, C., and Otis, B. (2001). Central auditory plasticity: changes in the N1-P2 complex after speech-sound training. Ear Hear. 22, 79–90. doi: 10.1097/00003446-200104000-00001
Tremblay, K. L., and Kraus, N. (2002). Auditory training induces asymmetrical changes in cortical neural activity. J. Speech Lang. Hear. Res. 45, 564. doi: 10.1044/1092-4388(2002/045)
Vandenberghe, R., Price, C., Wise, R., Josephs, O., and Frackowiak, R. S. (1996). Functional anatomy of a common semantic system for words and pictures. Nature 383, 254–256. doi: 10.1038/383254a0
Wise, R., Chollet, F., Hadar, U., Friston, K., Hoffner, E., and Frackowiak, R. (1991). Distribution of cortical neural networks involved in word comprehension and word retrieval. Brain 114, 1803–1817. doi: 10.1093/brain/114.4.1803
Wolmetz, M., Poeppel, D., and Rapp, B. (2011). What does the right hemisphere know about phoneme categories? J. Cogn. Neurosci. 23, 552–569. doi: 10.1162/jocn.2010.21495
Wong, P. C., Skoe, E., Russo, N. M., Dees, T., and Kraus, N. (2007). Musical experience shapes human brainstem encoding of linguistic pitch patterns. Nat. Neurosci. 10, 420–422.
Zaehle, T., Jancke, L., and Meyer, M. (2007). Electrical brain imaging evidences left auditory cortex involvement in speech and non-speech discrimination based on temporal features. Behav. Brain Funct. 3, 63. doi: 10.1186/1744-9081-3-63
Zatorre, R. J. (2002). “How does the brain process complex sound? Current controversies,” in Journal of Neurology Neurosurgery and Psychiatry, Vol. 72 (London: British Medical Journal Publisher Group), 825.
Zatorre, R. J., and Belin, P. (2001). Spectral and temporal processing in human auditory cortex. Cereb. Cortex 11, 946–953. doi: 10.1093/cercor/11.10.946
Zatorre, R. J., Belin, P., and Penhune, V. B. (2002). Structure and function of auditory cortex: music and speech. Trends Cogn. Sci. 6, 37–46. doi: 10.1016/S1364-6613(00)01816-7
Zatorre, R. J., Evans, A. C., Meyer, E., and Gjedde, A. (1992). Lateralization of phonetic and pitch discrimination in speech processing. Science 256, 846–849. doi: 10.1126/science.1589767
Keywords: musical training, musicians, language, speech processing, speech, transfer effects
Citation: Jantzen MG, Howe BM and Jantzen KJ (2014) Neurophysiological evidence that musical training influences the recruitment of right hemispheric homologues for speech perception. Front. Psychol. 5:171. doi: 10.3389/fpsyg.2014.00171
Received: 14 May 2013; Accepted: 12 February 2014;
Published online: 03 March 2014.
Edited by:
Sarah J. Wilson, University of Melbourne, AustraliaReviewed by:
Psyche Loui, Wesleyan University, USACyrille Magne, Middle Tennessee State University, USA
Michael Brian Gaetz, University of the Fraser Valley, Canada
Copyright © 2014 Jantzen, Howe and Jantzen. This is an open-access article distributed under the terms of the Creative Commons Attribution License (CC BY). The use, distribution or reproduction in other forums is permitted, provided the original author(s) or licensor are credited and that the original publication in this journal is cited, in accordance with accepted academic practice. No use, distribution or reproduction is permitted which does not comply with these terms.
*Correspondence: McNeel G. Jantzen, Department of Psychology, Western Washington University, 516 High Street, Bellingham, WA 98225, USA e-mail: mcneel.jantzen@wwu.edu