- 1Department of Educational Neuroscience, Faculty of Psychology and Education, LEARN! Institute, VU University Amsterdam, Amsterdam, Netherlands
- 2Scientific Centre for Research in Teaching (LOOK), Ruud de Moor Centrum, Open Universiteit Nederland, Heerlen, Netherlands
- 3Centre for Learning Sciences and Technologies, Open Universiteit Nederland, Heerlen, Netherlands
- 4Faculty of Health, Medicine and Life Sciences, School for Mental Health and Neuroscience, Maastricht University, Maastricht, Netherlands
This study examined differences between boys and girls regarding efficiency of information processing in early adolescence. Three hundred and six healthy adolescents (50.3% boys) in grade 7 and 9 (aged 13 and 15, respectively) performed a coding task based on over-learned symbols. An age effect was revealed as subjects in grade 9 performed better than subjects in grade 7. Main effects for sex were found in the advantage of girls. The 25% best-performing students comprised twice as many girls as boys. The opposite pattern was found for the worst performing 25%. In addition, a main effect was found for educational track in favor of the highest track. No interaction effects were found. School grades did not explain additional variance in LDST performance. This indicates that cognitive performance is relatively independent from school performance. Student characteristics like age, sex, and education level were more important for efficiency of information processing than school performance. The findings imply that after age 13, efficiency of information processing is still developing and that girls outperform boys in this respect. The findings provide new information on the mechanisms underlying boy-girl differences in scholastic performance.
Introduction
During an average school day, students are confronted with a wealth of information. New stimuli have to be attended to and irrelevant information has to be discarded. In a next stage of information processing, relevant information has to be analyzed and linked to previously stored material. As a result of that comparison, information can be discarded or stored in memory for later use. A core device in this process of information flow is working memory, a temporary holding device between new stimuli and already processed information (Baddeley, 1992). Students need to retrieve earlier consolidated information and search among many sources for the most relevant information. A quick and efficient accomplishment of this searching process will be beneficial to their achievement. The present paper investigates whether there are individual differences in the efficiency of information processing in early adolescence. Two narrow age groups were examined (age 13 and 15). Specifically the study sought to evaluate whether boys and girls differ with regard to speed of information processing in adolescence. Furthermore, educational track was considered as a possible source of variability, because positive relations were previously found between processing speed and intelligence (Fry and Hale, 2000; Sheppard and Vernon, 2008). Also, education effects on processing speed have been found in adults samples (Van der Elst et al., 2006; Longman et al., 2007). Given the central role of processing speed in higher cognitive functions (Salthouse, 1996; Fry and Hale, 2000), and its core importance for many aspects of performance in school, any evidence for differences in performance between boys and girls may have important implications for education.
It has been well-established that processing speed continues to improve from childhood into adolescence (age 4–17, e.g., Kail, 1991; Anderson et al., 2001; Luna et al., 2004; Nettelbeck and Burns, 2010; Coyle et al., 2011; McAuley and White, 2011). Age-related change was found to be dependent upon the processing speed measure used (Cepeda et al., 2013). Improvements in processing speed have been related to fine-tuning processes in the brain during this age period, leading to increased efficiency in brain functioning (Giedd, 2004, 2008; Gogtay et al., 2004). Following a developmental cascade model, increases in processing speed during adolescence in turn contribute to improvements in higher cognitive functions, such as working memory (Nettelbeck and Burns, 2010) and reasoning abilities (Nettelbeck and Burns, 2010; Vock et al., 2011). Also, it has been related to increases in general intelligence g (Coyle et al., 2011). Processing speed measures with high task demands (i.e., coding tasks) more strongly correlate with measures of higher cognitive functions than simple reaction time measures do (Nettelbeck and Burns, 2010; Cepeda et al., 2013).
Neuroimaging studies have shown that the developmental changes that take place in adolescence are different for boys and girls. There is a lag in brain development in boys compared to girls (De Bellis et al., 2001; Lenroot et al., 2007; Giedd, 2008). Total cerebral volume and gray matter volumes peak at a later age in boys (14.5 years) than in girls (10.5 years, Giedd, 2008). This suggests that next to age, sex is a possible factor influencing variability in processing speed, and that differences may be expected in young adolescents to the advantage of girls. Still, there is a lot of incongruence in research findings with respect to sex differences in processing speed during adolescence and young adulthood.
The incongruence in research findings with regard to differences between boys and girls may be related to the fact that the examined age ranges were too broad. A large study by Camarata and Woodcock (2006) showed that the magnitude of sex differences in processing speed was influenced by age. The girl advantage was relatively small in young children (9 and younger), larger in young adolescents (age 10–13), and the largest in adolescents aged 14–18. It disappeared almost completely in college students and young adults (17–34 years of age). Previous research examined age ranges that vary over a broad domain: 8–34 years (Anderson et al., 2001; Luna et al., 2004; Silveri et al., 2004; Asato et al., 2006; Camarata and Woodcock, 2006). Hence, the research by Camarata and Woodcock (2006) stresses the need to examine the issue of sex differences in processing speed during adolescence and young adulthood very specifically and in narrow age classes. Conflicting results with respect to sex differences in processing speed may also be explained by differences in processing speed measures. Generally, girls tend to outperform boys on processing speed measures that involve digits and alphabets, whereas boys are faster on simple processing speed measures (Roivainen, 2011). Furthermore, given the large effect of age and education on cognitive performance, sample sizes were often too small (<100 participants) to detect sex differences (Roivainen, 2011). Thus, sex differences in performance speed may only become prominent in large samples and in groups with narrow age ranges.
A sensitive measure to examine individual differences in processing speed is the Letter Digit Substitution Test (LDST, Jolles et al., 1995; Van der Elst et al., 2006, 2012). This coding task requires participants to match pairs of symbols according to a key. Because it involves additional cognitive processes such as working memory, visual scanning, sustained attention, response selection, interference control and monitoring, it is considered a more complex measure of processing speed (Cepeda et al., 2013). Within a given time interval (90 s), participants have to complete as many digit-letter substitutions as possible. The advantage of the LDST over other coding tasks like the Digit Symbol Substitution Test (Wechsler, 1955, 1981) or the Symbol Digit Modalities Test (Smith, 1982) relates to the use of over-learned symbols (letters and numbers) instead of abstract visual symbols. Over-learned symbols do not require complex visual processing and therefore have a lower cognitive load than abstract symbols. Hence, performance on the LDST better reflects efficiency of information processing. The LDST has already shown sensitivity to age, sex, and education level in school-aged children and older adults (Van der Elst et al., 2006, 2012).
This cross-sectional study examined three possible sources of variability in processing speed: age, sex, and educational track. Additionally, it related efficiency of information processing to real-life school performance. The study involved a total of 306 adolescents in grade 7 and grade 9 (aged 13 and 15, respectively). Participants were enrolled in one of the two highest educational tracks of Dutch secondary education. A group administration procedure was applied, enabling rapid and efficient data collection. Successful task performance was dependent on the total number of correctly completed items on the LDST. The hypotheses were: (1) adolescents in grade 9 show better task performance than adolescents in grade 7; (2) girls perform better than boys in both grades; (3) participants in the more difficult educational track perform better than participants in the less difficult track; and (4) higher school performance additionally predicted higher LDST performance. The homogenous population in terms of age range, educational track, and ethnicity, in combination with the large sample size lend the results of this study potential scientific and applied value. Finding differences between boys and girls will have implications for the fine-tuning of didactic procedures to match their working memory capacity.
Materials and Methods
Participants
Participants were recruited from a secondary school in a town in the south-eastern part of the Netherlands. A total number of 370 students from grade 7 and grade 9 participated in the study. All students were enrolled in one of the two highest educational tracks, either havo or vwo. Every student in Dutch secondary school is enrolled in one of three educational tracks that differ in level of difficulty. Approximately 40% of all secondary school children in the Netherlands are enrolled in one of the upper two tracks (Ministry of Education, 2009). Havo (5 years) and vwo (6 years) differ in level of difficulty. A havo diploma gives access to professional education programs, whereas a vwo diploma also allows for entry into university.
LDST data were missing for two of the 370 participating students. Another 62 participants were excluded from data analysis because they met one or more of our exclusion criteria, which were: (1) repeating a class after kindergarten; (2) skipping a class after kindergarten; (3) presence of medical conditions known to influence brain development and/or cognition, such as ADHD, epilepsy, and psychosis (as indicated by a self-report questionnaire); and (4) use of medication affecting the central nervous system. This resulted in a final sample of 306 adolescents (50.3% boys), including 138 adolescents in grade 7 (M age = 12.9, SD = 0.33, range = 12.1–13.9 years, 55% boys) and 168 adolescents in grade 9 (M age = 15.1, SD = 0.36, range = 14.2–16.0 years, 46% boys). Of the 306 selected participants, 143 were enrolled at the higher general secondary educational level (46.9% boys) and 163 at pre-university educational level (53.4% boys).
Procedure
The collaborating school agreed to fit the testing procedure into their regular school schedule. Within a week, all eligible classes of one grade had been tested. Testing took place by means of group administration and was procedurally identical for every class. Every class was tested once for a total duration of 50 min, which is equivalent to the time of one class period. Two trained investigators administered the testing protocol. One of them gave instructions to the participants and kept track of time, while the other walked around to help with potential problems. Additionally, a teacher supported task administration by keeping order in class. Testing circumstances (i.e., investigators, teacher, classroom) were kept similar for every participating class. Each testing session started by asking the participants to complete a short questionnaire on exclusion criteria. Then, several questionnaires and neuropsychological tests were administered, among which the LDST. Instructions for all tasks were given both verbally and on paper. After every instruction, participants practiced the task under supervision of the investigators. When all participants understood the task instructions, the actual task was administered. School grades were retrieved from the school's administration after study completion.
Measures
Letter digit substitution test
The LDST is a coding task that is based on over-learned symbols, i.e., letters and digits (Jolles et al., 1995; Van der Elst et al., 2006). It is a measure of general information processing speed, but also involves other cognitive processes, like working memory, visual scanning, sustained attention, response selection, interference control and monitoring (Baudouin et al., 2009; Cepeda et al., 2013). At the top of the test sheet, a key is presented showing nine boxes with letters and associated numbers between 1 and 9 in a random order. Underneath the key, boxes of letters are shown with blank spaces below. Participants were instructed to replace the blank spaces with the associated digits as fast and accurately as possible, according to the key presented above. First, they practiced the procedure to ensure that they understood the purpose of the test. After completion of the practice items, participants were instructed to complete as many test items as possible within 90 s. The number of correct substitutions made in 90 s served as dependent variable. In a large sample of adults, the test-retest reliability of the LDST was high (r > 0.85; Van der Elst et al., 2008). Furthermore, it was shown that the LDST is sensitive to age, sex, and education level in children aged 8–15 years and in adults (Van der Elst et al., 2006, 2012).
School grades
We obtained grades for Dutch (native language), English (foreign language), and mathematics. Together, these grades can validly estimate school performance (Reed et al., 2010). We used the mid-semester grades, because data collection was conducted in this time period.
Data Analysis
The statistical package SPSS 20.0 was used for all data analyses. A factorial univariate Analysis of Variance (ANOVA) was conducted with number of correct substitutions made in 90 s on the LDST as dependent variable. Independent variables in the model were grade (grade 7 vs. grade 9), sex (boys vs. girls), and educational track (havo vs. vwo). Interaction effects between all pairs of independent variables were examined. Effect sizes were expressed as Cohen's d (Cohen, 1988), which is calculated by dividing the mean difference between each factor level by the pooled standard deviation of these factor levels. Next, LDST performance was correlated with school grades. Then, a regression analysis was performed to examine which factors predicted LDST performance. Number of correct substitutions was the dependent variable, and predictors were school year (0 = grade 7; 1 = grade 9), sex (0 = male; 1 = female), educational track (0 = havo; 1 = vwo) and the average school grade. Level of significance was α = 0.05.
Results
Table 1 presents the number of correct substitutions on the LDST by grade, sex, and educational track. ANOVA showed significant main effects for grade [F(1, 298) = 79.3, p = 0.000], for sex [F(1, 298) = 30.4, p = 0.000], and for educational track [F(1, 298) = 13.0, p = 0.000]. There were no interaction effects between any of the pairs of independent variables [F(1, 298) < 1.39].
Results showed that adolescents in grade 9 outperformed adolescents in grade 7. The size of this effect was large (d = 1.09). Within both grades, a regression analysis with age, sex, and educational track showed that LDST performance was not predicted significantly by age in grade 7 [β = 0.07, t(302) = 0.85, p = 0.40], nor in grade 9 [β = −0.05, t(302) = −0.74, p = 0.46]. This indicates that grade 7 and grade 9 are homogeneous populations with respect to age. In both grades, girls gained significantly higher scores than boys (medium effect size, d = 0.63). There were no interaction effects, indicating that boys and girls did not differ significantly between grades or educational track.
To take a closer look at the data and distribution of boys and girls in this sample, LDST scores were divided into quartiles from the lowest to highest scores per grade (see Figure 1). This gives more insight into the distribution of boys and girls in a group of low, medium, and good performers and enables us to draw inferences about a class situation. We found that the distribution of boys and girls differs significantly between quartiles [X2(3) = 28.8; p = 0.000]. It appears that students with the 25% lowest LDST scores are predominantly boys (ratio 2:1; z = −2.2), whereas students who belong to the 25% best performers on the LDST are predominantly girls (also ratio 2:1; z = 2.5). There was no significant difference in the boy:girl ratio for the second (boys: z = 1.3) and third (boys: z = −1.3) quartile.
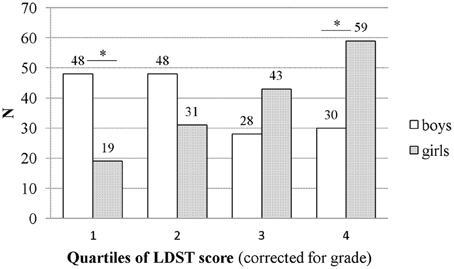
Figure 1. Sex differences in LDST performance divided over quartiles. Note: Quartile 1 = 25% lowest LDST scores; quartile 4 = 25% highest LDST scores. *p < 0.001.
The significant effect for educational track indicates that participants in the vwo track (pre-university education) scored significantly better than participants in the havo track (higher general secondary education). The effect size of educational track was small (d = 0.27).
Relation Between LDST Performance and School Grades
LDST score did not relate to the average school grade (r = 0.091, p = 0.112). This indicates that students who processed information more efficiently did not obtain higher grades in school than students who were slower. To examine the relation between school grades and LDST performance in more detail, school grades were also divided in quartiles. School performance of the lowest and highest performing students was related to LDST performance of students in the lowest and highest LDST quartile. No significant relations were found for any of the four contrasts (see Table 2). This indicates that real-life school performance did not relate to speed of information processing.
The regression model (see Table 3) explained a significant proportion of variance (R2 = 0.313) in LDST score, F(4, 300) = 34.2, p < 0.000. It confirmed that individual differences in LDST performance were explained by school year (β = 0.45), sex (β = 0.27) and educational track (β = 0.16), but not by school grades (β = 0.09). This showed that school grades did not explain additional variance in LDST performance next to school year, sex, and educational track.
Discussion
The purpose of this cross-sectional study was to examine whether age, sex, and educational level explained differences in the speed with which young adolescents process and manipulate information. We focused on two school years which included participants within a narrow age range of two educational tracks. Performance on the LDST was found to be dependent on grade, sex, and educational track. Results indicate that the number of correct substitutions on the LDST increased with age: adolescents in grade 9 performed significantly better than adolescents in grade 7. In both grades, girls obtained significantly higher scores than boys. The highest performing students were predominantly girls (ratio 2:1), whereas the poorest performers were predominantly boys (ratio 2:1). The rate of development was the same for boys and girls, as indicated by the lack of significant interaction effects. Furthermore, it was found that adolescents in the higher educational track outperformed adolescents in the lower educational track. School grades did not explain additional variance in LDST performance. Thus, individual differences in processing speed were explained by student characteristics, not by school performance.
The age effect found in the present study indicates that even after grade 7, there are large improvements in the efficiency with which over-learned material is processed. Thus, information processing in 15-year-old adolescents has evolved substantially by comparison to that of 13-year-old adolescents. This study therefore implies that improvements take place in the efficiency of processing of letters and digits even after childhood. Younger adolescents have less experience with these types of tasks and still have to develop automaticity in these processes. Importantly, our results furthermore indicate that boys and girls differ in processing speed and ability to manipulate new information. This suggests that the development of processing speed follows a sex-specific course in adolescence. In our sample of young adolescents, girls perform better than boys of the same age. The top 25% performers comprised twice as many girls as boys. The opposite pattern is found in the lowest scoring 25%.
Age-related increases in performance have been attributed to protracted brain development. With age, processing becomes more efficient as a result of synaptic pruning and an increase in white matter (Giedd, 2004, 2008; Gogtay et al., 2004). Protracted brain development particularly takes place in the frontal areas, to which executive control (Miller, 2005), working memory (Narayanan et al., 2005), and articulatory rehearsal (Lycke et al., 2008) have been attributed. The poorer performance of boys compared to girls is likely to be the consequence of delayed brain development in boys in the age ranges we studied (De Bellis et al., 2001; Lenroot et al., 2007). The girl advantage in LDST performance may also be related to sex differences in verbal learning, as the ability to manipulate and associate verbal material is an important determinant of successful coding task performance (Piccinin and Rabbitt, 1999; Joy et al., 2004; Cepeda et al., 2013). It has been well-established by cognitive research that girls are better verbal learners than boys (Anderson et al., 2001; Lowe et al., 2003; Meijs, 2008). Congruent with these findings, brain imaging studies have shown both structural (Schlaepfer et al., 1995; Harasty et al., 1997; Luders et al., 2005; Chen et al., 2007; Brun et al., 2009) and functional (Majeres, 1997; Baxter et al., 2003; Clements et al., 2006) differences in brain areas involved in higher order verbal functioning.
Alternatively, sex differences may be explained by motivational factors. During adolescence, large developments take place in students' beliefs and academic self-perceptions, such as their perceived competence and the value they place on doing well (see for instance Bouchey and Harter, 2005). Adolescent boys were found to have less adaptive school motivation patterns than girls, which could possibly explain their lower achievement on school-related tasks (Van Houtte, 2004; Dekker et al., 2013). Boys lower achievement may also be explained by their poorer self-regulation skills (Duckworth and Seligman, 2006). They may have suffered more than girls from the distraction that goes with assessment in a classroom setting.
The effect of educational track reported in this study indicates that higher educated adolescents process and manipulate information more quickly than lower educated adolescents. A comparable education effect has been found in adults (Van der Elst et al., 2006; Longman et al., 2007). This may be attributed to underlying differences in verbal memory. Schneider et al. (2002) found that students in higher educational tracks had better verbal memory than students in lower educational tracks, and that performance improved with age at the same rate for both educational tracks. Furthermore, given the positive relation between complex measures of processing speed and intelligence (Fry and Hale, 2000; Sheppard and Vernon, 2008), the results may also be attributed to differences in intelligence.
Yet, LDST performance was not predicted by school grades. Students who obtained higher grades, did not necessarily perform better on speeded tasks. This indicates that real-life school performance cannot be predicted by performance on one cognitive task only. Rather, numerous other factors will be involved in school grades, like motivation for learning and a structured learning environment. The findings do indicate that information processing speed as measured by the LDST may be a proxy for another dimension in (neuro)psychological functioning than school performance. More likely, it is a proxy for general intelligence. Future research should be done in this domain, to evaluate the role of processing speed in real-life school performance.
The present study does not inform about cultural differences because it was not designed to evaluate these. Previous research in (older) adults has shown that the LDST is a culturally robust test, and that performance was comparable within Europe (Houx et al., 2002) and between Europe and the USA (Moller et al., 1998). Therefore, no differences are expected in LDST performance of children of different Western countries.
In conclusion, our results support the hypothesis that the efficiency with which information is processed and manipulated in early and middle adolescence is still developing over that period. Furthermore, our findings show that sex and educational track, but not school grades, are important sources of variation for this age range. This is relevant for educational practice because many classroom activities involve processing speed. Practical implications of the research are that teachers should provide more guidance to boys, younger students, and students in lower educational tracks. They should be aware that repeated instruction may be more needed in these groups, in particular when multiple tasks are given at the same time. A higher need for clear instructions can be expected in these groups. The findings suggest that girls, older students, and students in higher educational tracks will experience less difficulty with double tasks, tasks that necessitate quick decisions, or tasks under time constraints. Future studies could usefully include older age groups to investigate the protracted development of efficiency of information processing and the stability of the sex difference over time.
Conflict of Interest Statement
The authors declare that the research was conducted in the absence of any commercial or financial relationships that could be construed as a potential conflict of interest.
References
Anderson, V. A., Anderson, P., Northam, E., Jacobs, R., and Catroppa, C. (2001). Development of executive functions through late childhood and adolescence in an Australian sample. Dev. Neuropsychol. 20, 385–406.
Asato, M. R., Sweeney, J. A., and Luna, B. (2006). Cognitive processes in the development of TOL performance. Neuropsychologia 44, 2259–2269. doi: 10.1016/j.neuropsychologia.2006.05.010
Baudouin, A., Clarys, D., Vanneste, S., and Isingrini, M. (2009). Executive functioning and processing speed in age-related differences in memory: contribution of a coding task. Brain Cogn. 71, 240–245. doi: 10.1016/j.bandc.2009.08.007
Baxter, L. C., Saykin, A. J., Flashman, L. A., Johnson, S. C., Guerin, S. J., Babcock, D. R., et al. (2003). Sex differences in semantic language processing: a functional MRI study. Brain Lang. 84, 264–272.
Bouchey, H. A., and Harter, S. (2005). Reflected appraisals, academic self-perceptions, and math/science performance during early adolescence. J. Educ. Psychol. 97, 673–686. doi: 10.1037/0022-0663.97.4.673
Brun, C. C., Lepore, N., Luders, E., Chou, Y. Y., Madsen, S. K., Toga, A. W., et al. (2009). Sex differences in brain structure in auditory and cingulate regions. Neuroreport 20, 930–935. doi: 10.1097/WNR.0b013e32832c5e65
Camarata, S., and Woodcock, R. (2006). Sex differences in processing speed: developmental effects in males and females. Intelligence 34, 231–252. doi: 10.1016/j.intell.2005.12.001
Cepeda, N. J., Blackwell, K. A., and Munakata, Y. (2013). Speed isn't everything: complex processing speed measures mask individual differences and developmental changes in executive control. Dev. Sci. 16, 269–286. doi: 10.1111/desc.12024
Chen, X. H., Sachdev, P. S., Wen, W., and Anstey, K. J. (2007). Sex differences in regional gray matter in healthy individuals aged 44-48 years: A voxel-based morphometric study. Neuroimage 36, 691–699. doi: 10.1016/j.neuroimage.2007.03.063
Clements, A. M., Rimrodt, S. L., Abel, J. R., Blankner, J. G., Mostofsky, S. H., Pekar, J. J., et al. (2006). Sex differences in cerebral laterality of language and visuospatial processing. Brain Lang. 98, 150–158. doi: 10.1016/j.bandl.2006.04.007
Cohen, J. (1988). Statistical Power Analysis for the Behavioral Sciences, 2nd Edn. Hillsdale, NJ: Erlbaum.
Coyle, T. R., Pillow, D. R., Snyder, A. C., and Kochunov, P. (2011). Processing speed mediates the development of general intelligence (g) in adolescence. Psychol. Sci. 22, 1265–1269. doi: 10.1177/0956797611418243
De Bellis, M. D., Keshavan, M. S., Beers, S. R., Hall, J., Frustaci, K., Masalehdan, A., et al. (2001). Sex differences in brain maturation during childhood and adolescence. Cereb. Cortex 11, 552–557. doi: 10.1093/cercor/11.6.552
Dekker, S., Krabbendam, L., Lee, N. C., Boschloo, A., De Groot, R., and Jolles, J. (2013). Sex differences in goal orientation in adolescents aged 10-19: the older boys adopt work-avoidant goals twice as often as girls. Learn. Individ. Dif. 26, 196–200. doi: 10.1016/j.lindif.2012.07.011
Duckworth, A. L., and Seligman, M. E. P. (2006). Self-discipline gives girls the edge: gender in self-discipline, grades, and achievement test scores. J. Educ. Psychol. 98, 198–208.
Fry, A. F., and Hale, S. (2000). Relationships among processing speed, working memory, and fluid intelligence in children. Ann. N.Y. Acad. Sci. 54, 1–34.
Giedd, J. N. (2004). Structural magnetic resonance imaging of the adolescent brain. Ann. N.Y. Acad. Sci. 1021, 77–85. doi: 10.1196/annals.1308.009
Giedd, J. N. (2008). The teen brain: insights from neuroimaging. J. Adolesc. Health 42, 335–343. doi: 10.1016/j.jadohealth.2008.01.007
Gogtay, N., Giedd, J. N., Lusk, L., Hayashi, K. M., Greenstein, D., Vaituzis, A. C., et al. (2004). Dynamic mapping of human cortical development during childhood through early adulthood. Proc. Natl Acad. Sci. 101, 8174–8179. doi: 10.1073/pnas.0402680101
Harasty, J., Double, K. L., Halliday, G. M., Kril, J. J., and McRitchie, D. A. (1997). Language-associated cortical regions are proportionally larger in the female brain. Arch. Neurol. 54, 171–176. doi: 10.1001/archneur.1997.00550140045011
Houx, P. J., Shepherd, J., Blauw, G.-J., Murphy, M. B., Ford, I., Bollen, E. L., et al. (2002). Testing cognitive function in elderly populations: the PROSPER study. J. Neurol. Neurosurg. Psychiatry 73, 385–389. doi: 10.1136/jnnp.73.4.385
Jolles, J., Houx, P. J., Van Boxtel, M. P. J., and Ponds, R. W. H. M. (1995). Maastricht Aging Study: Determinants of cognitive aging. Maastricht: Neuropsych Publishers.
Joy, S., Kaplan, E., and Fein, D. (2004). Speed and memory in the WAIS-III Digit Symbol-Coding subtest across the adult lifespan. Arch. Clin. Neuropsychol. 19, 759–767. doi: 10.1016/j.acn.2003.09.009
Kail, R. (1991). Developmental change in speed of processing during childhood and adolescence. Psychol. Bull. 109, 490–501.
Lenroot, R. K., Gogtay, N., Greenstein, D. K., Wells, E. M., Wallace, G. L., Clasen, L. S., et al. (2007). Sexual dimorphism of brain developmental trajectories during childhood and adolescence. Neuroimage 36, 1065–1073. doi: 10.1016/j.neuroimage.2007.03.053
Longman, R. S., Saklofske, D. H., and Fung, T. S. (2007). WAIS-III percentile scores by education and sex for U.S. and Canadian populations. Assessment 14, 426–432. doi: 10.1177/1073191107304114
Lowe, P. A., Mayfield, J. W., and Reynolds, C. R. (2003). Gender differences in memory test performance among children and adolescents. Arch. Clin. Neuropsychol. 18, 865–878.
Luders, E., Narr, K. L., Thompson, P. M., Woods, R. P., Rex, D. E., Jancke, L., et al. (2005). Mapping cortical gray matter in the young adult brain: effects of gender. Neuroimage 26, 493–501. doi: 10.1016/j.neuroimage.2005.02.010
Luna, B., Garver, K. E., Urban, T. A., Lazar, N. A., and Sweeney, J. A. (2004). Maturation of cognitive processes from late childhood to adulthood. Child Dev. 75, 1357–1372.
Lycke, C., Specht, K., Ersland, L., and Hugdahl, K. (2008). An fMRI study of phonological and spatial working memory using identical stimuli. Scand. J. Psychol. 49, 393–401. doi: 10.1111/j.1467-9450.2008.00672.x
Majeres, R. L. (1997). Sex differences in phonetic processing: speed of identification of alphabetical sequences. Percept. Mot. Skills 85(3 Pt 2), 1243–1251.
McAuley, T., and White, D. A. (2011). A latent variables examination of processing speed, response inhibition, and working memory during typical development. J. Exp. Child Psychol. 108, 453–468. doi: 10.1016/j.jecp.2010.08.009
Meijs, C. J. C. (2008). Verbal Learning in School-Aged Children and the Influence of Child-Related Factors, Test-Related Factors, and Natural Context. Maastricht: Neuropsych Publishers.
Ministry of Education, C. a. S. (2009). Kerncijfers 2004-2008: Onderwijs, Cultuur en Wetenschap [Core Figures 2004–2008: Education, culture and science]. (OCW39.008/1.000/08BK2009B003). Den Haag: Ministry of Education, Culture and Science.
Moller, J. T., Cluitmans, P., Rasmussen, L. S., Houx, P., Rasmussen, H., Canet, J., et al. (1998). Long-term postoperative cognitive dysfunction in the elderly: ISPOCD1 study. Lancet 351, 857–861.
Narayanan, N. S., Prabhakaran, V., Bunge, S. A., Christoff, K., Fine, E. M., and Gabrieli, J. D. (2005). The role of the prefrontal cortex in the maintenance of verbal working memory: an event-related FMRI analysis. Neuropsychology 19, 223–232. doi: 10.1037/0894-4105.19.2.223
Nettelbeck, T., and Burns, N. R. (2010). Processing speed, working memory and reasoning ability from childhood to old age. Pers. Individ. Dif. 48, 379–384. doi: 10.1016/j.paid.2009.10.032
Piccinin, A. M., and Rabbitt, P. M. (1999). Contribution of cognitive abilities to performance and improvement on a substitution coding task. Psychol. Aging 14, 539–551.
Reed, H., Ouwehand, C., Van der Elst, W., Boschloo, A., and Jolles, J. (2010). “Measures of school performance for use in educational neuropsychology,” in Frontiers in Neuroscience Conference Abstract: EARLI SIG22 - Neuroscience and Education (Zurich). doi: 10.3389/conf.fnins.2010.11.00058
Roivainen, E. (2011). Gender differences in processing speed: a review of recent research. Learn. Individ. Dif. 21, 145–149. doi: 10.1016/j.lindif.2010.11.021
Salthouse, T. A. (1996). The processing-speed theory of adult age differences in cognition. Psychol. Rev. 103, 403–428.
Schlaepfer, T. E., Harris, G. J., Tien, A. Y., Peng, L., Lee, S., and Pearlson, G. D. (1995). Structural differences in the cerebral cortex of healthy female and male subjects: a magnetic resonance imaging study. Psychiatry Res. 61, 129–135.
Schneider, W., Knopf, M., and Stefanek, J. (2002). The development of verbal memory in childhood and adolescence: findings from the Munich Longitudinal Study. J. Educ. Psychol. 94, 751–761. doi: 10.1037/0022-0663.94.4.751
Sheppard, L. D., and Vernon, P. A. (2008). Intelligence and speed of information-processing: a review of 50 years of research. Pers. Individ. Dif. 44, 535–551. doi: 10.1016/j.paid.2007.09.015
Silveri, M. M., Tzilos, G. K., Pimentel, P. J., and Yurgelun-Todd, D. A. (2004). Trajectories of adolescent emotional and cognitive development: effects of sex and risk for drug use. Ann. N.Y. Acad. Sci. 1021, 363–370. doi: 10.1196/annals.1308.046
Smith, A. (1982). Symbol Digit Modalities Test, Manual. Los Angeles, CA: Western Psychological Services.
Van der Elst, W., Dekker, S., Hurks, P., and Jolles, J. (2012). The letter digit substitution test: demographic influences and regression-based normative data for school-aged children. Arch. Clin. Neuropsychol. 27, 433–439. doi: 10.1093/arclin/acs045
Van der Elst, W., Van Boxtel, M. P., Van Breukelen, G. J., and Jolles, J. (2006). The Letter Digit Substitution Test: normative data for 1,858 healthy participants aged 24-81 from the Maastricht Aging Study (MAAS): influence of age, education, and sex. J. Clin. Exp. Neuropsychol. 28, 998–1009. doi: 10.1080/13803390591004428
Van der Elst, W., Van Boxtel, M. P., Van Breukelen, G. J., and Jolles, J. (2008). Detecting the significance of changes in performance on the Stroop Color-Word Test, Rey's Verbal Learning Test, and the Letter Digit Substitution Test: the regression-based change approach. J. Int. Neuropsychol. Soc. 14, 71–80. doi: 10.1017/S1355617708080028
Van Houtte, M. (2004). Why boys achieve less at school than girls: the differences between boys' and girls' academic culture. Educ. Stud. 30, 159–173.
Vock, M., Preckel, F., and Rolling, H. (2011). Mental abilities and school achievement: a test of a mediation hypothesis. Intelligence 39, 357–369. doi: 10.1016/j.intell.2011.06.006
Keywords: information processing efficiency, processing speed, development, sex differences, adolescence
Citation: Dekker S, Krabbendam L, Aben A, de Groot R and Jolles J (2013) Coding task performance in early adolescence: a large-scale controlled study into boy-girl differences. Front. Psychol. 4:550. doi: 10.3389/fpsyg.2013.00550
Received: 21 May 2013; Accepted: 02 August 2013;
Published online: 27 August 2013.
Edited by:
Barbara McCombs, University of Denver, USAReviewed by:
Barbara McCombs, University of Denver, USARonnel B. King, Nanyang Technological University, Singapore
Copyright © 2013 Dekker, Krabbendam, Aben, de Groot and Jolles. This is an open-access article distributed under the terms of the Creative Commons Attribution License (CC BY). The use, distribution or reproduction in other forums is permitted, provided the original author(s) or licensor are credited and that the original publication in this journal is cited, in accordance with accepted academic practice. No use, distribution or reproduction is permitted which does not comply with these terms.
*Correspondence: Sanne Dekker, Department of Educational Neuroscience, Faculty of Psychology and Education, LEARN! Institute, VU University Amsterdam, Van der Boechorststraat 1, 1081 BT Amsterdam, Netherlands e-mail:cy5qLmRla2tlckB2dS5ubA==