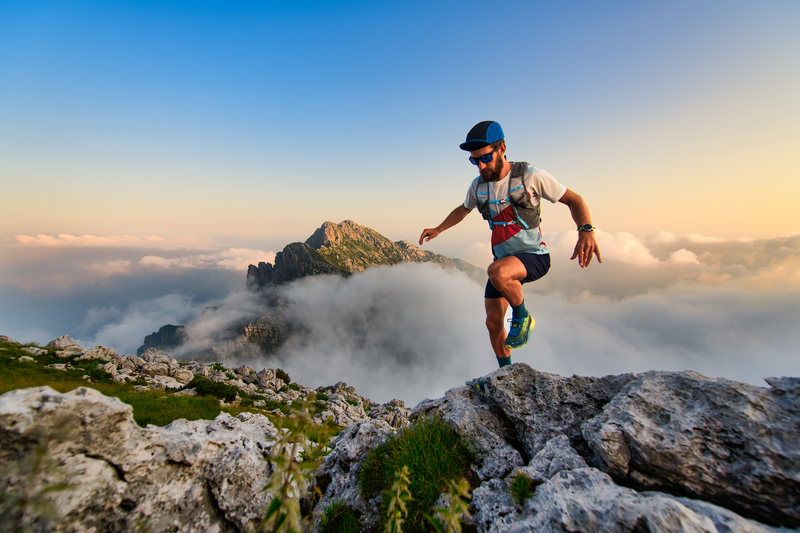
95% of researchers rate our articles as excellent or good
Learn more about the work of our research integrity team to safeguard the quality of each article we publish.
Find out more
ORIGINAL RESEARCH article
Front. Psychol. , 22 June 2012
Sec. Cognition
Volume 3 - 2012 | https://doi.org/10.3389/fpsyg.2012.00206
This article is part of the Research Topic What role does the default mode network play in cognition? View all 7 articles
The default mode network (DMN) has recently attracted widespread interest. Previous studies have found that task-related processing can induce deactivation and changes in the functional connectivity of this network. However, it remains unclear how tasks modulate the underlying effective connectivity within the DMN. Using recent advances in dynamic causal modeling (DCM), we investigated the modulatory effect of (gender judgment) task performance on directed connectivity within the DMN. Sixteen healthy subjects were scanned twice: at rest and while performing a gender judgment task. Group independent component analysis was used to identify independent spatial components. Four subject-specific regions of interest (ROIs) were defined according to the ensuing default mode component: the posterior cingulate cortex, the left lateral parietal cortex, the right lateral parietal cortex, and the medial prefrontal cortex. Effective connectivity among these regions was then characterized with stochastic DCM, revealing enhanced (extrinsic) between region connectivity within the DMN during task sessions – and a universal decrease in (intrinsic) self-inhibition – relative to resting sessions. These results suggest a distributed but systematic modulatory effect of cognitive and attentional set on the effective connectivity subtending the DMN: an effect that increases its sensitivity to inputs and may optimize distributed processing during task performance.
The default mode network (DMN) has received increasing attention in recent years and has been considered as “one of the most intriguing puzzles in cognitive neuroscience” (Gilbert et al., 2007). This network consists of brain regions including precuneus/posterior cingulate cortex (PCC), medial prefrontal cortex (mPFC), and medial, lateral, and inferior parietal cortex (Raichle et al., 2001). Regions in this network have been reported to be the most metabolically active regions, relative to other brain regions. Moreover, DMN regions have been demonstrated to be highly correlated at rest and during passive tasks (Greicius et al., 2003, 2004). In addition, alterations within this network have been reported in subjects with Alzheimer’s disease (Greicius et al., 2004; Rombouts et al., 2005; Wang et al., 2006; Sorg et al., 2007), schizophrenia (Harrison et al., 2007; Calhoun et al., 2008), and depression (Grimm et al., 2009; Sheline et al., 2009).
Previous studies have demonstrated a significant impact of task performance or stimulus-bound processing on spontaneous brain activity within the DMN. On the one hand, cognitive tasks can modulate resting state brain activity in the DMN. A consistent DMN deactivation pattern has been demonstrated by many studies (Shulman et al., 1997; Mazoyer et al., 2001; Greicius et al., 2003; Shannon, 2006). Although the resting state activity of the DMN is high, this activity is decreased when performing goal-directed tasks. This kind of deactivation has been observed using different tasks and different brain imaging techniques. By analyzing data from nine positron emission tomography (PET) studies, Shulman et al. (1997) found consistent blood flow decreases in the DMN across different visual tasks, relative to passive viewing of the same stimuli. Another meta-analysis performed by Mazoyer et al. (2001) included 63 subjects, 370 spatially normalized PET scans, and nine cognitive tasks: the task domains varied from language, mental imagery, mental calculation, reasoning, finger movement, to spatial working memory. Regions of the DMN showed decreased activation during the nine tasks, when compared to a common rest state. Moreover, studies (Shannon, 2006; Buckner et al., 2008) have found that not only the spatial patterns of task-induced deactivation were consistent across different tasks, but also the spatial pattern of blocked task-induced deactivation, event-related task-induced deactivation, and hippocampal functional connectivity converged on the core regions of the DMN.
On the other hand, external stimuli have also been shown to modulate the connectivity of the DMN (Esposito et al., 2006, 2009; Hampson et al., 2006; Marrelec and Fransson, 2011; Newton et al., 2011). Using a working-memory task, Esposito et al. (2006, 2009) found that the functional connectivity within the DMN was spatially modulated by the level of working memory engaged and correlated with task performance. Similar results have also been reported in another study where task performance was positively correlated with the functional connectivity between PCC and the medial frontal gyrus and ventral anterior cingulate cortex (MFG/vACC); both during task and at rest (Hampson et al., 2006). Recently, another study reported that the functional connectivity of the DMN increased as cognitive load increased (Newton et al., 2011). Conversely, Marrelec and Fransson (2011) reported decreases in functional connectivity during task compared to rest. But, as pointed out by the authors, regions of interest (ROIs) were selected using resting state data, which may have deemphasized task responsive regions. In addition, the interaction between the DMN and the other networks has also been shown to be modulated by goal-directed tasks. Treserras et al. (2009) found that the DMN and the sensorimotor network was functionally correlated during task performance, although there were no significant correlation between these two networks at rest.
While an increasing number of studies have suggested a modulatory effect of task and stimuli on the activity and functional connectivity of the DMN, the impact of goal-directed tasks on the effective connectivity of the DMN remain unclear. Functional connectivity is defined as statistical dependencies among regional time series. Any measures such as correlations, coherence, or transfer entropy that describe these dependencies can be used to characterize functional connectivity (Friston et al., 2011a). While functional connectivity measures undirected statistical associations among brain regions, effective connectivity quantifies the directed coupling among regions: effective connectivity refers to the influence that one neural system exerts over another. Analyses of effective connectivity enable one to make inferences about information flow in a network, by comparing the evidence for different models of directed coupling. To better understand how different brain regions interact at rest and during task performance, one needs to study the directed (and possibly reciprocal) effective connections between them.
Studying the effective connectivity of the DMN – and task-specific modulation of effective connectivity – is of great potential significance. It allows us to discover how DMN regions interact with each other and may provide mechanistic insights into the effect of tasks on the activity and connectivity underlying the DMN. In this study, we elucidated the modulatory effect of exogenous (stimulus) processing on the effective connectivity within the DMN. We quantified the effective connectivity within the DMN both at rest and during performance of a gender judgment using functional magnetic resonance imaging (fMRI) data. In brief, group independent component analysis (GICA) analyses were used to decompose the functional images into spatially independent components (ICs). The default mode component was then identified in an automatic manner, using a canonical default mode template derived from previous studies. Activity of the default mode component was then summarized in terms of four ROI. Finally, effective connectivity among these four regions was estimated using stochastic dynamic causal models (DCM). This enabled us to detect directed effective connections – subtending DMN activity – that changed during task-related processing.
Sixteen healthy Chinese subjects (6 males, 10 females, aged 20.4 ± 3.3) participated in this study. All the subjects are right-handed. None were taking any psychoactive drugs or suffered from neurological or psychiatric disease prior to study. All subjects provided informed written consent and this study was approved by the Ethics Committee of Second Xiangya Hospital.
Each subject underwent two sessions of fMRI scan. In one (rest) session, subjects were instructed to lie in the scanner, keep their eyes closed and not to think of anything in particular. The scan lasted for 5 min 6 s during which time 153 images were collected from each subjects.
The second (task) session employed a gender judgment task, in which subjects were asked to discriminate the gender of faces presented visually on a screen. An emotional face was presented for 20 ms, followed by a neutral face for 300 ms. Faces were presented every 3 s. Subjects responded with a button press. This session used a block design: the session began with a 12 s period of preparation, and then the subjects performed a block of the gender judgment task for 45 s. This was followed by a rest block which lasted for 15 s. The whole scan consisted of five task blocks and four rest blocks and the session lasted for 4 min 57 s. A total of 99 scans were collected for each subject.
Data were acquired in the third Xiangya Hospital, Hunan Province, China on a 1.5T MRI scanner (Siemens, Erlangen, Germany). To reduce head motion during scanning, participants’ heads were fixed using foam pads within a standard birdcage head coil. The functional MR images were obtained using gradient-echo T2*-weight echo-planar imaging (EPI) sequence. For the resting state data, the following parameters were used: TR = 2000 ms, TE = 40 ms, FOV = 24 cm, FA = 90°, slice thickness = 5 mm. For the gender judgment task, the parameters were: TR = 3000 ms, TE = 40 ms, FOV = 24 cm, FA = 90°, slice thickness = 4 mm, gap = 1 mm. High resolution T1-weighted 3D images covering the whole brain were also collected for each subject with a 1900 ms TR, and TE of 192 ms, and a FA of 15, yielding 176 slices of 1.0 mm thickness with a FOV of 25 cm.
Data were preprocessed using SPM81. For the rest data, the first 13 functional images for each subject were discarded to ensure signal stabilization. For the task data, the first four images were discarded for each subject. A six-parameter rigid body transformation (three rotations, three translations) was used to realign the functional images to the first image of each session. Then the high resolution structural image was coregistered with the functional images and was subsequently segmented and the (non-linear) mapping between the anatomical image and the standard Montreal Neurological Institute (MNI, Quebec, Canada) template was then used to normalize the functional data. Finally, an 8-mm FWHM Gaussian kernel was used to smooth the data.
We performed a conventional whole brain SPM analysis to identify task-related deactivations during task blocks relative to rest blocks. This analysis represents an alternative way of identifying the default mode, which we used to establish the construct validity of the default mode identified using independent component analyses (see below).
For the task session, subject-specific responses were modeled using a general linear model (GLM) that included the following regressors: (1) a boxcar function convolved with the canonical hemodynamic response function, modeling stimulus-bound processing; (2) the six motion parameters to model the movement correlated effects; (3) one constant regressor to model the baseline, and (4) cosine basis functions, to model possible signal drift and aliased respiratory and cardiac signals. For the first level (within subject) analysis, a t-contrast was used to detect the brain regions that were deactivated by the gender judgment task for each subject. The t-contrast (contrast of parameter estimates) images were then entered into second-level (between-subject) analyses and a one sample t-test was performed to detect deactivations at the group level.
To identify discrete regions for subsequent DCM, we first characterized the DMN – in each subject and session – using ICA: after preprocessing, GICA was performed to decompose the rest and task data into spatial ICs using the GIFT software2. ICA identifies ICs by maximizing their statistical independence. GIFT implements ICA at a group level and decomposes the functional images into spatial independent patterns. For the current study, GICA was performed on the resting state dataset and the task dataset respectively. First, the optimal number of the ICs was estimated using the minimum description length criteria (Li et al., 2007). This number was 22 for the rest data and 26 for the task data. Time series from all subjects were concatenated and decomposed into ICs using the infomax algorithm, resulting in 22 independent spatial maps for the rest data and 26 independent spatial maps for the task data. The corresponding components for each subject were then calculated via a back reconstruction step.
The default mode component for each subject and session was identified in a fully automated manner. First, a DMN template was derived from previous studies (Calhoun et al., 2008; Fair et al., 2008) using the WFU PickAtlas Tool (Version 2.4). In the current study, the template was created using the bilateral precuneus, posterior cingulate, angular, and superior medial frontal cortex. Then the ICs were sorted using a multiple spatial regression (over voxels) of the template with the ICs. The IC with the highest regression coefficient was identified as the default mode component. To identify default mode regions that were expressed consistently over subjects, a one sample t-test was applied to subject-specific default mode components (for each session) to produce a statistical parametric map testing for the group mean DMN, under rest and task conditions.
Subject-specific ROIs were based on the identification of the default mode described above. For each subject and each session, four ROIs were identified: the PCC, the left later parietal cortex (LPC), the right later parietal cortex (RPC), and the mPFC. Subject-specific ROIs were centered on the local maximum of the default mode component that was nearest the maxima in the group mean. For each subject, the time series for all ROIs were extracted from a sphere region (radius = 6 mm). The location of the ROIs and the corresponding responses are shown in Figure 1 for a typical subject. The periods of task performance are indicated by pink regions.
Figure 1. Representative region selection and fMRI responses for a typical subject. (A) Shows the four core regions used to summarize the dynamics of the default mode on a subject-specific basis. These regions were selected as the maximum of subject-specific independent components that were closest to the group maximum as established with a between-subject SPM analysis of the default mode components. (B) Regional responses correspond to the principal eigenvariate of all voxels within 6 mm of the region’s center. Abbreviations: mPFC, medial prefrontal cortex; PCC, posterior cingulate cortex; RPC, right lateral parietal cortex; LPC, left lateral parietal cortex.
Effective connectivity analysis was performed using DCM10 in SPM8. Stochastic DCM tries to explain how neuronal activity in one region is affected by neuronal activity in others based on non-linear stochastic differential equations. Distributed neuronal activity is modeled by hidden neuronal states associated with each region using neuronal evolution or state equations. In addition, hemodynamic state equations model the conversion from hidden neuronal states to observed fMRI signals via hemodynamic states, like blood flow and the deoxyhemoglobin concentration. These stochastic differential equations contain parameters corresponding to effective connectivity mediating influences both within and between regions. Conventional DCMs use deterministic state equations, which do not account for spontaneous neuronal fluctuations or state noise. However, ignoring neuronal fluctuations may lead to an incomplete model and inefficient parameter estimates. Furthermore, deterministic models fail to exploit spontaneous fluctuations in activity to estimate effective connectivity in the absence of a (known) experimental perturbation. Stochastic DCM finesses these problems by including random fluctuations in the state equations. In stochastic DCM, there are three sets of unknown quantities that needed to be estimated; these include the (coupling) parameters of the state equations, the hidden states, and precision of observation noise. Established estimation schemes, such as dynamic expectation maximization (DEM) assume that these quantities are conditional independent. However, we have recently proposed (and validated) a new estimation scheme called generalized filtering, which dispenses this mean-field assumption and can provide more informed online parameter estimates (Friston et al., 2010). See Li et al. (2011) for further details – and an illustrative application to fMRI time series.
We used generalized filtering to invert (fit) a fully connected DCM for each subject and session. The DCM results were then used to optimize the model (discover which connections where present). The parameter estimates from the optimized DCM were then compared at the between subject and session level – as summary statistics – to test for changes in coupling among DMM regions during the task sessions. We use exactly the same DCM for both the rest and task sessions; crucially, we did not include known exogenous inputs for the task sessions but let the fluctuations in neuronal activity model task-induced responses. This represents a more conservative analysis of the differences between the two sessions – in the sense that we did not use prior knowledge about the differences between rest and task sessions.
In more detail: subject-specific stochastic DCMs were fully and reciprocally connected (resulting in 16 connections within and among four nodes) for the rest and task fMRI sessions respectively. This full DCM was inverted using generalized filtering. The best (reduced) model for both the rest and task-related data was recovered using a Bayesian network discovery scheme – post hoc model optimization (Friston et al., 2011b). This optimization uses the estimates from a full model to calculate the model evidence and parameter estimates for all reduced models. The reduced model (and parameter estimates) with the greatest model evidence was selected as the best model. The ensuing DCM (and parameters) was generated for each subject and session. In this study, we assumed that the model was a fixed-effect in the population. Bayesian parameter averaging was then performed over subjects for the rest and task sessions respectively, to assess task-induced changes in connectivity. This parameter averaging procedure treats the posterior distribution of one subject as the prior for the next and provides a single posterior density for the entire group (Kasess et al., 2010). Inference on the presence of a connection at the between-subject level was then made using the posterior density of the group average connection strength.
The standard SPM analysis revealed that the gender judgment task deactivated the core regions of the DMN including the right angular gyrus, the right precuneus, the left inferior parietal gyrus, the right cingulate gyrus, and the left MFG (Table 1). These results are threshold at p < 0.001, uncorrected but survived correction at p < 0.05 for a small volume correction at the regional level.
Group ICA analysis was performed on both the rest and task-related fMRI data to decompose the time series in terms of spatial ICs. The DMN was identified then using a default mode template. Figure 2 shows the mean default mode component (SPM) for both sessions. Figures 2A,B show the mean default mode component for the all the subjects at rest and during performance of the gender judgment task respectively. Figure 2C shows the task-induced deactivation pattern (SPM). Figure 2D shows the overlap of the DMN identified using independent component analysis and task-related deactivation respectively. It can be seen that the spatial pattern of the DMN identified using these different criteria were highly consistent – showing a high degree of overlap with each other. Here the results was displayed at p < 0.005 (uncorrected for display purposes).
Figure 2. Statistical parametric maps following one sample t-tests on the default mode components over subjects (thresholded at p < 0.005 uncorrected). The upper rows show the default mode component at rest (A) and during the task session (B). (C) Shows the task-induced deactivations, detected using a conventional SPM analysis of task-related effects within the task sessions. This represents an alternative way of identifying default mode regions and validates the default modes identified with independent component analysis. This construct validation is shown in terms of the overlap in the lower row (D).
Based on the results of the ICA analyses, four ROIs were defined including the PCC, LPC, RPC, and mPFC. The ROIs and corresponding responses are shown in Figure 1 for a typical subject. Figure 3 shows the estimated hidden (neuronal and hemodynamic) states of the mPFC following inversion (generalized filtering) for a typical resting state session. The upper and middle panels depict the estimated hidden states including neuronal activity, vasodilatory signal, normalized flow, volume, and deoxyhemoglobin content. The lower panel shows the predicted response and prediction error. It can be seen that the conditional expectations or estimates of changes in neuronal activity (and subsequent changes in hemodynamic states) account for most, but not all, of the observed fluctuations in the fMRI time series. Crucially, the slower and larger fluctuations are well predicted; however, there are still high-frequency low amplitude fluctuations about these predictions that constitute an estimate of observation noise.
Figure 3. Exemplar results of the stochastic dynamic causal modeling. These results are taken from a model inversion of resting state data from a single session. The underlying hidden neuronal and hemodynamic states are shown in the upper and middle (A,B). The resulting BOLD time series are shown in terms of the model predictions and prediction error (C). These results are shown as a function of time in scans for a region in the mPFC.
A network discovery scheme was then used to recover the best model from the estimates of the fully connected DCM, using data from all the subjects and both datasets. Figure 4 shows the results of this post hoc optimization. The left panel shows the log evidence over all 65536 reduced models (all permutations of connections between regions). The models on the left of the x axis have fewer connections, while the models on the right have more connections. One can see a general trend, in which models with more parameters have greater evidence. However, the local variations in log evidence mean that some models with fewer connections have more evidence than other models with more connections, despite the fact they cannot fit the data as accurately – these models have greater evidence because they are less complex. Somewhat to our surprise, the fully connected model (the red dot in Figure 4A) had the greatest evidence and is the most plausible explanation for our data. In other words, removing any connection reduced model evidence. This can be intuited from the estimates shown on the right panel of Figure 4. One can see that the 90% Bayesian confidence intervals (pink bars) do not include a zero connection strength for any extrinsic (between region) connection. Usually, this means a connection contributes to model evidence in a substantial way.
Figure 4. This figure presents the results of post hoc model optimization or network discovery. Having inverted a full model with full extrinsic connectivity, we then assessed the log model evidence for all reduced models (models with one or more missing connections). The model with the highest evidence was the full model – as can be seen in (A). This suggests that we can be almost certain the full model is the best explanation for these data. The corresponding conditional parameter estimates are shown over the 16 (intrinsic and extrinsic) connections in (B) as green bars. The associated 90% Bayesian confidence intervals are shown as pink bars.
Figure 5 shows the average optimized DCM and the significant (larger than zero with more than 95% confidence) connections for the resting state and task-related data set. Comparison of the coupling parameters at rest and during task performance reveals an increase (Figure 6) in most (13/16) of the connections within the DMN. Crucially, these increases included both extrinsic (between region) and intrinsic (within-region) coupling. This is important, because within-region coupling (parameters 1, 6, 11, and 16) is negative. This means, that one can summarize our results as a widespread and diffuse increase in extrinsic connectivity that is accompanied by a reduction of intrinsically mediated self-inhibition. In other words, there is an overall increase in the excitability of the DMN that – perhaps counter intuitively – produces task-related deactivation in the constituent regions (see Discussion). This suggests that regional deactivation may be one side of an increase in the amplitude of (task-related) fluctuations.
Figure 5. Significant connections within the average full model under rest (A) and task (B) sessions. Only the connections greater than zero, with more than 95% confidence are shown. The posterior mean for the coupling parameters are shown alongside the connections (arrows). Two-headed arrows indicate bidirectional (reciprocal) connectivity.
Figure 6. Differences between the rest and task conditions for each of the 16 connections. Note that the majority have increased.
Interestingly, we also found significant correlations – over subjects – between task-related changes in neuronal activity and effective connectivity among the DMN regions. Figure 7 shows the correlation between regional BOLD signal changes and (efferent and afferent) effective connection strengths for the mPFC during task performance. Regional changes in BOLD signal in the PCC were significantly (p = 0.04) correlated with the strength of its effective efferent connection to the mPFC (Figure 7A). Similarly, BOLD signal changes in the LPC was significantly (p = 0.006) correlated with the strength of the afferent effective connection from mPFC (Figure 7B). These relationships show that differences in effective connectivity mediate proportional task-related activations and deactivations over subjects.
Figure 7. Correlation between regional BOLD signal changes and effective connection strengths within the DMN. (A) Percent BOLD signal change in the PCC was significantly (p = 0.04) correlated with the strength of its effective connection to the mPFC. (B) Percent BOLD signal change in the LPC was significantly (p = 0.006) correlated with the strength of the effective connection from mPFC to LPC.
In this study, we evaluated resting state effective connectivity within the DMN using stochastic DCM. Significant effective connectivity was detected among four core regions of the DMN including the PCC, LPC, RPC, and mPFC. The same analysis was then applied to fMRI responses obtained during a gender judgment task. Comparison of the effective connectivity strength within the same network showed a modulatory effect of the task on its effective connectivity: with significant increases in the effective connectivity between regions of the DMN; in conjunction with a decrease in (intrinsic) self-inhibition in every region. These results suggest that although the activity of the DMN was decreased during periods of task performance, the effective connectivity among default mode regions increased to render them more excitable.
To the best of our knowledge, this is the first study to investigate resting state effective connectivity and its modulation by task-related processing, using stochastic DCM. Recently, some researcher have tried to investigate directed resting state connectivity between DMN regions using a variety of methods including Granger causality (Uddin et al., 2011; Zhou et al., 2011), Bayesian networks (Wu et al., 2011), and partial directed coherence (Silfverhuth et al., 2011). One key advantage of DCM – over other schemes – is the use of a hemodynamic model to map hidden neuronal states to observed fMRI signals. This is of great importance for fMRI data, where the observed blood-oxygen-level-dependent signals are hemodynamic convolutions of underlying neuronal signals. In this case, “the inevitable effects that hemodynamic system imposes on causal inferences in fMRI data, lead us toward the methods in which causal inferences can take place in latent neuronal level, rather than observed BOLD time series” (Bakhtiari and Hossein-Zadeh, 2012). In the current study, significant bidirectional connections were detected between the PCC and LPC, LPC and RPC as well as RPC and mPFC at rest. Moreover, an inhibitory effect from the PCC to mPFC was observed. Our finding of significant effective connectivity within the DMN at rest is consistent with previous studies of the DMN (Uddin et al., 2011; Wu et al., 2011; Zhou et al., 2011). Despite differences in methodological details, all the three studies detected significant causal interactions between the PCC and mPFC. As the most important nodes of the DMN, the PCC, and mPFC are inferred to be connected in previous reports of functional and anatomy connectivity (Greicius et al., 2003, 2004; Esposito et al., 2006, 2009; Price, 2007). Moreover, the PCC has been reported to be connected with LPC and RPC in humans (Wu et al., 2011; Zhou et al., 2011) and robust anatomical connections with the parietal cortex have also been reported in macaque monkey (Kobayashi and Amaral, 2003, 2007).
In this study, we found task-related effects on the effective connectivity within the DMN. Note that this effective connectivity corresponds to the average effective connectivity over both task and rest periods in the gender judgment sessions. In other words, we did not model changes in connectivity specific to the task blocks – we were more interested in the underlying effective connectivity, while alternating between task performance and rest blocks. In other words, we were interested in the pervasive effects on coupling within the default mode induced by a “non-rest” set or context that includes task performance, task switching, and associated changes in cognitive and attentional set. Task-specific effects were modeled in our DCM by time-dependent fluctuations in activity (hidden neuronal states) that one can associate with event-related and induced neuronal responses.
Within this context, the gender judgment task-induced changes in the coupling of core regions within the DMN. The DMN regions were more tightly connected to each other during task sessions. Bidirectional connections between LPC and mPFC, between PCC and RPC, along with a positive connection from PCC to mPFC were detected during the task sessions, while these connections were not significant at rest. Notably, most of these task-specific connections are to or from the mPFC. This result is in agreement with a recent study that noted increasing cognitive load shifts the DMN functional connectivity pattern from more posterior (PCC) to more anterior (mPFC) regions (Esposito et al., 2009).
Although activity of the DMN decreased during task performance, this was mediated by an increase in excitability and coupling. Our findings are consistent with previous functional connectivity studies that report increased functional connectivity within the DMN during task performance (Esposito et al., 2006, 2009; Hampson et al., 2006; Newton et al., 2011). The coupling parameters in DCM represent connection strengths, describing the strength of a coupling in terms of the rate (in Hz) at which a response is elaborated in the target region (Friston et al., 2003), greater coupling parameters suggest a faster (stronger) response in the target region. In this study, we detected increased effective connectivity within the DMN during task performance. In addition, we found significant correlations between regional BOLD signal changes and effective connection strengths. Larger (faster) BOLD signal changes were associated with stronger effective connections over subjects (Figure 7). In short, increased effective connectivity within the DMN may facilitate faster and large amplitude fluctuations in BOLD signal that appear as activations in task-related regions and deactivations in the DMN. That is to say, increased effective connectivity may enable a more efficient (faster) switching from rest to task mode. This result is consistent with the hypothesis that decreased task-dependent activity in the DMN may be associated with increased engagement of its constituent regions (Hampson et al., 2006).
It may seem paradoxical that a decrease in self-inhibition serves as the best explanation for the deactivation of the default mode during task performance. The resolution of this paradox lies in remembering that we are allowing for differences in both endogenous activity and (time-invariant) connectivity. This means that large (task-related) fluctuations in neuronal activity below average levels during task performance are amplified by decreased self-inhibition and are communicated to other (DMN) regions by enhanced extrinsic connectivity. The net result is a greater task-related deactivation in DMN regions. In other words, there is an antisymmetric effect of connectivity on fluctuations in neuronal activity about average levels – activations are more positive and deactivations are more negative.
In summary, our main result is that a switch in cognitive set from rest to task-orientated modes of activity increases the effective connectivity among the constituents of the DMN and consistently increases their intrinsic excitability. Conventional views of DMN function hypothesize that the DMN is active at rest and suspended during cognitively demanding tasks. However, previous studies have reported an increased engagement of the DMN during task performance (Hampson et al., 2006; Newton et al., 2011). In addition, task performance has also been shown to be correlated with the spatial pattern and the strength of functional connectivity within the DMN (Esposito et al., 2006, 2009; Hampson et al., 2006). Better performance was associated with greater functional connectivity (Hampson et al., 2006). We found that a switch in cognitive set from a rest to task increases effective connectivity in the DMN and increases its intrinsic excitability. In dynamical terms, this implies an increase in sensitivity to exogenous inputs or equivalently, a loss of stability and a move toward a critical regime that might facilitate the processing of sensory input and task-related processing. Thus, our findings may support earlier proposals that the DMN may facilitate or monitor cognitive performance rather than being disengaged during cognitive tasks.
The authors declare that the research was conducted in the absence of any commercial or financial relationships that could be construed as a potential conflict of interest.
This work was funded by National Basic Research Program of China (Baojuan Li, and Dewen Hu for 2011CB707802) and the National Natural Science Foundation of China (Xiang Wang and Shuqiao Yao for 81071104) and supported by the Wellcome Trust (Karl Friston). We are indebted to our two reviewers for guidance in clarifying and elaborating this report.
Bakhtiari, S. K., and Hossein-Zadeh, G. A. (2012). Subspace-based identification algorithm for characterizing causal networks in resting brain. Neuroimage 60, 1236–1249.
Buckner, R. L., Andrews-Hanna, J. R., and Schacter, D. L. (2008). The brain’s default network: anatomy, function, and relevance to disease. Ann. N. Y. Acad. Sci. 1124, 1–38.
Calhoun, V. D., MacIejewski, P. K., Pearlson, G. D., and Kiehl, K. A. (2008). Temporal lobe and “default” hemodynamic brain modes discriminate between schizophrenia and bipolar disorder. Hum. Brain Mapp. 29, 1265–1275.
Esposito, F., Aragri, A., Latorre, V., Popolizio, T., Scarabino, T., Cirillo, S., Marciano, E., Tedeschi, G., and Di Salle, F. (2009). Does the default-mode functional connectivity of the brain correlate with working-memory performances? Arch. Ital. Biol. 147, 11–20.
Esposito, F., Bertolino, A., Scarabino, T., Latorre, V., Blasi, G., Popolizio, T., Tedeschi, G., Cirillo, S., Goebel, R., and Salle, F. D. (2006). Independent component model of the default-mode brain function: assessing the impact of active thinking. Brain Res. Bull. 70, 263–269.
Fair, D. A., Cohen, A. L., Dosenbach, N. U., Church, J. A., Miezin, F. M., Barch, D. M., Raichle, M. E., Petersen, S. E., and Schlaggar, B. L. (2008). The maturing architecture of the brain’s default network. Proc. Natl. Acad. Sci. U.S.A. 105, 4028–4032.
Friston, K. J. (2011a). Functional and effective connectivity: a review. Brain Connectivity 1, 13–36.
Friston, K. J., Li, B., Daunizeau, J., and Stephan, K. E. (2011b). Network discovery with DCM. Neuroimage 56, 1202–1221.
Friston, K., Harrison, L., and Penny, W. (2003). Dynamic causal modelling. NeuroImage 19, 1273–1302.
Friston, K., Stephan, K., Li, B., and Daunizeau, J. (2010). Generalised filtering. Math. Probl. Eng. 2010, 1–35.
Gilbert, S. J., Dumontheil, I., Simons, J. S., Frith, C. D., and Burgess, P. W. (2007). Comment on “wandering minds: the default network and stimulus-independent thought”. Science 317, 43b.
Greicius, M. D., Krasnow, B., Reiss, A. L., and Menon, V. (2003). Functional connectivity in the resting brain: a network analysis of the default mode hypothesis. Proc. Natl. Acad. Sci. U.S.A. 100, 253–258.
Greicius, M. D., Srivastava, G., Reiss, A. L., and Menon, V. (2004). Default-mode network activity distinguishes Alzheimer’s disease from healthy aging: evidence from functional MRI. Proc. Natl. Acad. Sci. U.S.A. 101, 4637–4642.
Grimm, S., Boesiger, P., Beck, J., Schuepbach, D., Bermpohl, F., Walter, M., Ernst, J., Hell, D., Boeker, H., and Northoff, G. (2009). Altered negative BOLD responses in the default-mode network during emotion processing in depressed subjects. Neuropsychopharmacology 34, 932–843.
Hampson, M., Driesen, N. R., Skudlarski, P., Gore, J. C., and Constable, R. T. (2006). Brain connectivity related to working memory performance. J. Neurosci. 26, 13338–13343.
Harrison, B. J., Yucel, M., Pujol, J., and Pantelis, C. (2007). Task-induced deactivation of midline cortical regions in schizophrenia assessed with fMRI. Schizophr. Res. 91, 82–86.
Kasess, C. H., Stephan, K. E., Weissenbacher, A., Pezawas, L., Moser, E., and Windischberger, C. (2010). Multi-subject analyses with dynamic causal modeling. Neuroimage 49, 3065–3074.
Kobayashi, Y., and Amaral, D. (2003). Macaque monkey retrosplenial cortex: II. Cortical afferents. J. Comp. Neurol. 466, 48–79.
Kobayashi, Y., and Amaral, D. G. (2007). Macaque monkey retrosplenial cortex: III. Cortical efferents. J. Comp. Neurol. 502, 810–833.
Li, B., Daunizeau, J., Stephan, K. E., Penny, W., Hu, D., and Friston, K. (2011). Generalised filtering and stochastic DCM for fMRI. Neuroimage 58, 442–457.
Li, Y., Adali, T., and Calhoun, V. (2007). Estimating the number of independent components for functional magnetic resonance imaging data. Hum. Brain Mapp. 28, 1251–1266.
Marrelec, G., and Fransson, P. (2011). Assessing the influence of different ROI selection strategies on functional connectivity analyses of fMRI data acquired during steady-state conditions. PLoS ONE 6, e14788. doi:10.1371/journal.pone.0014788
Mazoyer, B., Zago, L., Mellet, E., Bricogne, S., Etard, O., Houdé, O., Crivello, F., Joliot, M., Petit, L., and Tzourio-Mazoyer, N. (2001). Cortical networks for working memory and executive functions sustain the conscious resting state in man. Brain Res. Bull. 54, 287–298.
Newton, A. T., Morgan, V. L., Rogers, B. P., and Gore, J. C. (2011). Modulation of steady state functional connectivity in the default mode and working memory networks by cognitive load. Hum. Brain Mapp. 32, 1649–1659.
Price, J. L. (2007). Definition of the orbital cortex in relation to specific connections with limbic and visceral structures, and other cortical regions. Ann. N. Y. Acad. Sci. 1121, 54–71.
Raichle, M. E., MacLeod, A. M., Snyder, A. Z., Powers, W. J., Gusnard, D. A., and Shulman, G. L. (2001). A default mode of brain function. Proc. Natl. Acad. Sci. U.S.A. 98, 676–682.
Rombouts, S. A., Barkhof, F., Goekoop, R., Stam, C. J., and Scheltens, P. (2005). Altered resting state networks in mild cognitive impairment and mild Alzheimer’s disease: an fMRI study. Hum. Brain Mapp. 26, 231–239.
Shannon, B. J. (2006). Functional Anatomic Studies of Memory Retrieval and the Default Mode. St. Louis: Washington University in St. Louis.
Sheline, Y. I., Barch, D. M., Price, J. L., Rundle, M. M., Vaishnavi, S. N., Snyder, A. Z., Mintun, M. A., Wang, S., Coalson, R. S., and Raichle, M. E. (2009). The default mode network and self-referential processes in depression. Proc. Natl. Acad. Sci. U.S.A. 106, 1942–1947.
Shulman, G. L., Fiez, J. A., Corbetta, M., Buckner, R. L., Miezin, F. M., Raichle, M. E., and Petersen, S. E. (1997). Common blood flow changes across visual tasks: 11. Decreases in cerebral cortex. J. Cogn. Neurosci. 95, 648–663.
Silfverhuth, M. J., Remes, J., Starck, T., Nikkinen, J., Veijola, J., Tervonen, O., and Kiviniemi, V. (2011). Directional connectivity of resting state human fMRI data using cascaded ICA-PDC analysis. Acta Radiol. 52, 1037–1042.
Sorg, C., Riedl, V., Muhlau, M., Calhoun, V. D., Eichele, T., Laer, L., Drzezga, A., Forstl, H., Kurz, A., Zimmer, C., and Wohlschlager, A. M. (2007). Selective changes of resting-state networks in individuals at risk for Alzheimer’s disease. Proc. Natl. Acad. Sci. U.S.A. 104, 18760–18765.
Treserras, S., Boulanouar, K., Conchou, F., Simonetta-Moreau, M., Berry, I., Celsis, P., Chollet, F., and Loubinoux, I. (2009). Transition from rest to movement: brain correlates revealed by functional connectivity. Neuroimage 48, 207–216.
Uddin, L. Q., Supekar, K. S., Ryali, S., and Menon, V. (2011). Dynamic reconfiguration of structural and functional connectivity across core neurocognitive brain networks with development. J. Neurosci. 31, 18578–18589.
Wang, L., Zang, Y., He, Y., Liang, M., Zhang, X., Tian, L., Wu, T., Jiang, T., and Li, K. (2006). Changes in hippocampal connectivity in the early stages of Alzheimer’s disease: evidence from resting state fMRI. Neuroimage 31, 496–504.
Wu, X., Li, R., Fleisher, A. S., Reiman, E. M., Guan, X., Zhang, Y., Chen, K., and Yao, L. (2011). Altered default mode network connectivity in Alzheimer’s disease – a resting functional MRI and Bayesian network study. Hum. Brain Mapp. 32, 1868–1881.
Keywords: default mode network, effective connectivity, stochastic dynamic causal modeling, independent component analysis, functional magnetic resonance imaging
Citation: Li B, Wang X, Yao S, Hu D and Friston K (2012) Task-dependent modulation of effective connectivity within the default mode network. Front. Psychology 3:206. doi: 10.3389/fpsyg.2012.00206
Received: 28 February 2012; Accepted: 02 June 2012;
Published online: 22 June 2012.
Edited by:
David James Sharp, Imperial College London, UKReviewed by:
Don Tucker, Electrical Geodesics Inc. and University of Oregon, USACopyright: © 2012 Li, Wang, Yao, Hu and Friston. This is an open-access article distributed under the terms of the Creative Commons Attribution Non Commercial License, which permits non-commercial use, distribution, and reproduction in other forums, provided the original authors and source are credited.
*Correspondence: Dewen Hu, Department of Automatic Control, College of Mechatronics and Automation, National University of Defense Technology, 47 Yanwachi Street, Changsha, Hunan 410073, China. e-mail:ZHdodUBudWR0LmVkdS5jbg==
Disclaimer: All claims expressed in this article are solely those of the authors and do not necessarily represent those of their affiliated organizations, or those of the publisher, the editors and the reviewers. Any product that may be evaluated in this article or claim that may be made by its manufacturer is not guaranteed or endorsed by the publisher.
Research integrity at Frontiers
Learn more about the work of our research integrity team to safeguard the quality of each article we publish.