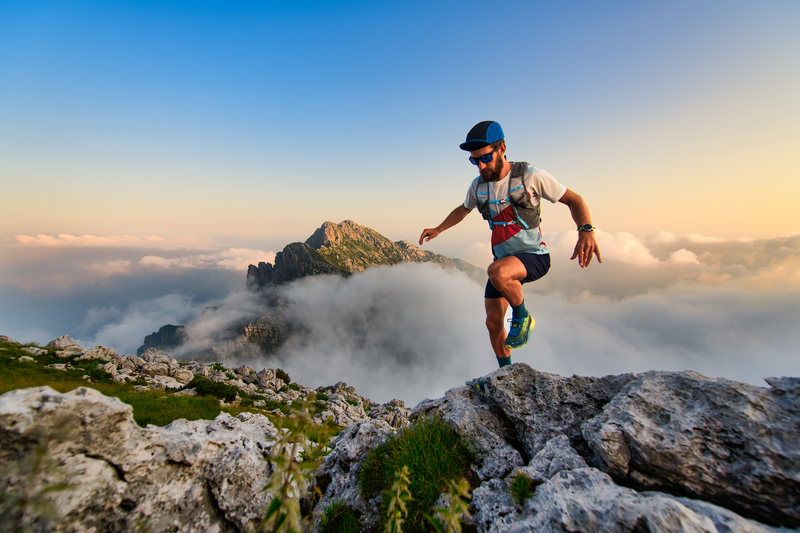
94% of researchers rate our articles as excellent or good
Learn more about the work of our research integrity team to safeguard the quality of each article we publish.
Find out more
ORIGINAL RESEARCH article
Front. Psychol. , 02 July 2012
Sec. Developmental Psychology
Volume 3 - 2012 | https://doi.org/10.3389/fpsyg.2012.00200
This article is part of the Research Topic Grounding Word Learning in Attention, Perception, and Memory View all 12 articles
When looking for the referents of novel nouns, adults and young children are sensitive to cross-situational statistics (Yu and Smith, 2007; Smith and Yu, 2008). In addition, the linguistic context that a word appears in has been shown to act as a powerful attention mechanism for guiding sentence processing and word learning (Landau and Gleitman, 1985; Altmann and Kamide, 1999; Kako and Trueswell, 2000). Koehne and Crocker(2010, 2011) investigate the interaction between cross-situational evidence and guidance from the sentential context in an adult language learning scenario. Their studies reveal that these learning mechanisms interact in a complex manner: they can be used in a complementary way when context helps reduce referential uncertainty; they influence word learning about equally strongly when cross-situational and contextual evidence are in conflict; and contextual cues block aspects of cross-situational learning when both mechanisms are independently applicable. To address this complex pattern of findings, we present a probabilistic computational model of word learning which extends a previous cross-situational model (Fazly et al., 2010) with an attention mechanism based on sentential cues. Our model uses a framework that seamlessly combines the two sources of evidence in order to study their emerging pattern of interaction during the process of word learning. Simulations of the experiments of (Koehne and Crocker, 2010, 2011) reveal an overall pattern of results that are in line with their findings. Importantly, we demonstrate that our model does not need to explicitly assign priority to either source of evidence in order to produce these results: learning patterns emerge as a result of a probabilistic interaction between the two clue types. Moreover, using a computational model allows us to examine the developmental trajectory of the differential roles of cross-situational and sentential cues in word learning.
Learning a language involves mapping words to their corresponding meanings in the outside world. Children learn most of their vocabulary from hearing words in noisy and ambiguous contexts, where there are infinitely many possible mappings between words and concepts (Carey, 1978). They attend to the visual environment to establish such mappings, but given that the visual context is often very rich and dynamic, elaborate cognitive processes are required for successful word learning from observation.
A well-studied mechanism for learning word–world mappings from ambiguous contexts is cross-situational word learning (Quine, 1960; Siskind, 1996; Akhtar and Montague, 1999; Yu and Smith, 2007; Smith and Yu, 2008). This mechanism follows a straightforward bottom-up strategy based on statistical co-occurrence of words and concepts across situations. By observing that a particular object, action, or property is in view more often than others whenever a certain unknown word is uttered, the connection between the word and that object/action/property is strengthened over time. Numerous studies have shown that children and adults draw on cross-situational evidence when learning words from different categories, and in various conditions (e.g., Yu and Smith, 2007; Childers and Paik, 2008; Smith and Yu, 2008; Vouloumanos, 2008; Smith et al., 2011). For instance, Smith and Yu (2008) find that children as young as 1-year-old can quickly track the co-presence of novel nouns and objects across trials when two objects and two spoken words are presented in each trial. Yu and Smith (2007) show that adults perform above chance in learning novel nouns in even more ambiguous conditions (3–4 unknown words and objects per trial). Childers and Paik (2008) report that 2-year-olds are able to use cross-situational evidence for learning not only nouns, but also predicate terms. Moreover, cross-situational learning has been argued to be a graded process: Vouloumanos (2008) and Vouloumanos and Werker (2009) show that adults and children are sensitive to small differences in the word–object co-occurrence rates. These findings all suggest that cross-situational evidence is a rich source of information for handling noise and ambiguity in word learning.
In addition to the cross-situational learning, a variety of attention mechanisms have been proposed to help narrow down relevant parts of an observed scene when learning a word meaning, in order to focus on the referred objects or actions. Carpenter et al. (1998) and Bloom (2000) argue that children use their (innate or acquired) social skills to infer the referent of a word as intended by a speaker. Various studies have shown that caretakers often provide consistent social cues, such as eye-gaze and gesture, when interacting with children (e.g., Tomasello and Todd, 1983), and that children use these cues to facilitate word learning (e.g., Baldwin et al., 1996; Baldwin, 2000; Nappa et al., 2009). In particular, the sentential context of a word is a powerful source of guidance in providing cues for attending to relevant aspects of meaning for the word. The sentential context consists of structures which combine certain types of words in particular ways. Such acquired associations between linguistic forms and word meanings can help the learner make inferences about potential referents of unknown words. It has been suggested that children draw on syntactic cues that the linguistic context provides in order to guide word learning, a hypothesis known as syntactic bootstrapping (Gleitman, 1990; Gillette et al., 1999). Children and adults are shown to be sensitive to structural properties of language, and to the association of such properties with aspects of meaning (e.g., Naigles and Hoff-Ginsberg, 1995; Fisher, 2002; Gertner et al., 2006; Piccin and Waxman, 2007; Lee and Naigles, 2008).
Clearly, the sentential context plays a significant role in human word learning. Nonetheless, only a few studies have examined the interplay of the sentence-level and other well-known word learning mechanisms such as cross-situational learning (see, e.g., Gillette et al., 1999; Lidz et al., unpublished manuscript). In particular, Koehne and Crocker(2010, 2011) investigate the interaction of these two mechanisms in an artificial word learning scenario, and this interaction is the precise focus of our study here. The experiments of Koehne and Crocker (2010) are based on teaching adult participants a semi-natural miniature language. Adult participants are exposed to a variety of learning conditions, in each of which the two sources of word meaning evidence (cross-situational statistics and sentence-level constraints) interact in a different way. For example, the two sources of information may be pointing to the same object as the referent of a target noun, or they might be contradicting each other. The performance of the participants in selecting the correct referent of a novel noun is taken to reflect how these two sources of evidence interact in human word learning. Their results reveal that adults can successfully learn from both cross-situational and sentence-level constraints in parallel. In addition, these results suggest that sentence-level constraints might be modulating the use of cross-situational statistics in certain conditions. While these studies shed light on the nature of both learning mechanisms, a detailed and systematic account of the dynamics and time course of their interplay is still missing.
Computational modeling is a powerful tool for the precise investigation of the hypothesized mechanisms of word learning. Through computational modeling, we can carefully study whether a model that is based on a suggested theory or learning mechanism (and is tested on naturalistic data) shows a pattern of behavior similar to those observed in humans. Most existing computational models of word learning focus on the informativeness of cross-situational evidence in learning word–meaning mappings (Siskind, 1996; Li et al., 2004; Regier, 2005; Yu, 2005; Frank et al., 2007; Fazly et al., 2010). Extensions of these models integrate certain types of social cues such as gaze and gesture (Frank et al., 2007; Yu and Ballard, 2008), or shallow syntactic cues such as lexical categories of words (Yu, 2006; Alishahi and Fazly, 2010). Only a few models explicitly study the role of sentential context in word learning (Niyogi, 2002; Maurits et al., 2009), extremely limiting the possibilities for the syntactic context of the words to be learned.
In sum, there are only a few computational models of word learning that integrate sentence-level syntactic cues. Moreover, there is a complete lack of computational studies of the interplay of cross-situational word learning and sentence-level constraints. Furthermore, none of the existing models investigate the developmental trajectory of word learning, considering information sources other than cross-situational statistics.
We propose a computational approach to fill this gap. Our model integrates the two learning mechanisms using a probabilistic framework. Importantly, there are no specific rules or parameters in the model that indicate which mechanism has a stronger influence in any particular learning situation. Rather, the contribution of each source of evidence is determined by the informativeness of that source, given what is available in the input, and what the model has learned at each stage of learning. We use this model to simulate the experiments of Koehne and Crocker(2010, 2011), and to provide a more detailed explanation of how the two sources of information affect the learned word–meaning mappings. Our model in general behaves similarly to the human participants in the psycholinguistic experiments, and is able to exploit informative linguistic context to boost performance in word learning. The sentential context in our model is represented as a set of categories which are inferable from the linguistic structure of the utterance, and carry aspects of meaning. Cross-situational evidence and context-based cues in our model are integrated in a seamless fashion, and their interaction is a function of the properties of the input as well as the informativeness of each source. In addition to simulating the experimental findings of Koehne and Crocker(2010, 2011), we test our model on varying amounts of input data prior to the artificial word learning trials, and show that the contribution of sentential context is a function of age: in order to efficiently take advantage of this additional attention mechanism, the model has to have received sufficient input data to establish meaningful associations between lexico-syntactic categories and general meaning representations.
Consider a young language learner hearing the sentence daddy is ironing a dax, and trying to find out the meaning of dax. Usually there are many possible interpretations for dax based on the surrounding scene, and the child has to narrow them down using some learning strategy. One such method is to register the potential meanings in the current scene, and compare them to those inferred from the previous usages of the same word (i.e., cross-situational learning). Another way to make an informed guess about the meaning of dax is to pay attention to its sentential context. For example, if the child has already heard some familiar words in a similar context (e.g., mommy is ironing her dress; he is ironing a shirt, etc.), she can conclude that a group of words which can appear in the context “is ironing –” usually refer to clothing items.
We present a computational model based on that of Fazly et al. (2010), that integrates this particular attention mechanism – i.e., guidance by the sentential context, into cross-situational word learning. This model learns word meanings as probabilistic associations between words and semantic features, using an incremental and probabilistic learning mechanism, and drawing only on the word–meaning co-occurrence statistics gradually collected from naturally occurring child-directed input. The model has been shown to accurately learn the meaning of a large set of words from noisy and ambiguous input data, and to exhibit patterns similar to those observed in children in a variety of tasks (see Fazly et al., 2010, for a full set of experiments on this model). However, this model cannot explain effects such as those reported by Koehne and Crocker(2010, 2011), where human subjects clearly use mechanisms other than cross-situational learning in order to guide their attention in a word leaning scenario.
The model presented in this paper extends that of Fazly et al. (2010) in two important aspects. First, it uses a more sophisticated and plausible representation for the semantics of an observed scene. Second, it incorporates an additional learning mechanism based on the sentential context of each word under study, and the restrictions that it imposes on potential referents for that word1. Importantly, our extended model integrates the two sources of information – i.e., cross-situational and context-based evidence – in a seamless fashion. The pattern of interaction between these two information sources is not pre-defined. Instead, they interact in a dynamic way, and as a response to what the model has learned so far, and what information is available from the current context.
In the rest of this section, we first describe how the input data for word learning (an utterance accompanying an observed scene) and the acquired word meanings are represented in our model. We then give an overview of the learning procedure, and explain how cross-situational learning is augmented by a context-based attention mechanism. The mathematical formalization of the model is presented in Section 3.
The input to our word learning model consists of a set of utterance–scene pairs that link an observed scene (what the learner perceives) to the utterance that describes it (what the learner hears). We represent each utterance as a set of words, and the corresponding scene as a set of potential referents. For simplicity, in the rest of this paper we refer to what appears in a scene as a concept or a potential referent of a word – that could be an object, an action, or a property. Each concept in a scene is represented as a set of semantic features (e.g., the referent of the word broccoli is represented as the set {BROCCOLI, VEGETABLE, OBJECT, …}). Figure 1 shows a sample input pair as represented in our model.
The goal of our model is to learn which semantic features are most likely to be part of the meaning of a word. The knowledge of a word meaning must be acquired gradually and by processing noisy and ambiguous input, therefore the representation of meaning must accommodate this uncertainty. We represent the meaning of a word as a probability distribution over all the semantic features. In the absence of any prior knowledge, all features can potentially be part of the meaning of all words. However, as the model receives and processes more usages of the same word, its association with certain semantic features (those which co-occur with the word, or are in line with the sentential cues) strengthens. Similarly, the association of a word with those semantic features which rarely co-occur with it, or are in contrast with the available sentential cues, weakens over time.
Children and adult word learners are sensitive to cues provided by the sentential context of a novel word, such as the selectional preferences of the main verb in the sentence, or the syntactic category of the word as indicated by its surrounding context. For example, a context pattern such as he is Xing over there suggests X to be an action, the big X suggests X to be an object, and She ate X suggests X to be an edible object. These suggestions can significantly reduce the level of uncertainty and ambiguity when searching for the referent of an unknown word in a scene.
We represent such cues in our model as categories. Although the focus of this work is not on what the nature of these categories are and how they are formed, there is ample evidence that adults and children have access to such categories. (We will discuss the notion of categories and their formation in more detail in Section 6.) We assume that an independent categorization module can process each sentence and determine the lexical category for each word based on its surrounding context2. Each category is simply a collection of word forms, weighted by their frequency of occurrence in that category. Note that a word can belong to more than one category, depending on its context.
Since a category is a weighted set of words, it can also have a meaning as a weighted sum of the current meaning of all words which belong to it. In our extended model, category meanings formed as such are one of the main means of guiding attention to relevant objects when processing an utterance–scene pair.
Word learning proceeds incrementally. That is, the knowledge of a word’s meaning is gradually updated each time the learner hears that word being used in a context. The model learns the meaning of a word, by processing each input pair in two steps. The first step is eqnarrayment: upon hearing an utterance paired with a visual scene, the learner has to decide which concept in the scene is likely to be the referent of each word in the utterance. The second step is adjustment: meaning representations for each word in the utterance have to be updated in accordance with the new alignments estimated in the first step. More details on each of these two steps come next.
When aligning words to referents, several factors come into play. One factor is the cross-situational evidence: if a word has frequently co-occurred with a concept, there is a high chance that this co-occurrence is meaningful and the concept is the intended referent of the word. The cross-situational evidence thus far is accumulated in the model’s learned knowledge of word meanings. For a word heard for the first time, we assume that the learned meaning is such that all semantic features are equally likely, and hence all concepts are equally likely to be referents of the word in the context of the current utterance–scene pair. In addition, concepts compete with each other as possible referents of a word, i.e., the concept with the strongest evidence is the one with the strongest alignment with that word.
Another factor that can influence the alignment of a word and a concept is the degree to which the sentential context of the word supports the alignment. For example, if learners are aware of the general properties of direct objects of the verb iron (as a group or category), it is more likely that they pick a clothing item from the scene as the potential referent of dax in the utterance daddy is ironing the dax.
Using these factors, an alignment probability is estimated between every word in the current utterance, and every potential referent in the scene. The alignment probability between a word and a referent reflects the learner’s degree of confidence that the co-occurrence is due to chance or to a meaningful relation between the two.
The estimated alignment probabilities can be used to update the previously learned word meanings. For each word, its meaning is adjusted for each semantic feature that is part of the representation of an aligned referent, in proportion to their alignment probability. Early on in the course of learning, the meaning of a word might change drastically as a result of processing a new input pair. As the model ages, its acquired knowledge of word meanings becomes more robust and stable, and adjustments are done in smaller steps.
Because learning is a gradual process and the meaning of a word is continually changing, it is not obvious at which point in time a word can be considered as properly learned. Intuitively, a word is sufficiently learned when its meaning reflects strong associations with relevant semantic features, and weak associations with irrelevant ones.
In experimental studies of word learning, a common practice for testing whether a word is learned is to ask subjects to pick the right referent for the target word from a set of visible objects (within a scene or on a computer screen). We simulate such a referent selection task in our model by presenting it with a target word and a set of objects as its possible referents. We evaluate the performance of our model in selecting the correct referent, by calculating the probability of choosing the correct referent given the target word as the stimulus. This probability is measured by looking into the similarity of the learned meaning of the target word to the feature-based semantic representation of each of the objects.
In this section we will present a detailed version of the model, and specify how the ideas sketched in the previous section are formally realized.
Given a corpus of utterance–scene pairs, our model learns the meaning of each word w as a time-dependent probability distribution p(t)(.|w) over the semantic features appearing in the corpus. In this representation, p(t)(f |w) is the probability of feature f being part of the meaning of word w at time t. In the absence of any prior knowledge, e.g., when a word is heard for the first time, all features can potentially be part of the meaning of all words. Hence, prior to receiving any usages of a given word, the model assumes a uniform distribution over semantic features as its meaning.
As previously mentioned, we assume that prior to the onset of word learning, the learner has formed a number of categories, each containing a set of word forms. More formally, we assume that the word learning model has access to a categorization function cat(w, U) which at any given time during the course of learning can determine the category of a word w in utterance U. As the model learns meanings of words, the categories that these words belong to are implicitly assigned a meaning as well. Once the word learning process begins, we assign a meaning distribution to each category on the basis of the meanings learned for its members. Formally, at each point in time, we estimate the meaning of a category c, p(t)(.|c), as the average of the meaning distributions of its members. That is, for each feature f :
where |c| is the number of word tokens in category c. Prior to observing any instances of the members of a category in the input, we assume a uniform distribution over all the possible semantic features for each category.
The model proposes a probabilistic interpretation of word learning through an interaction between two types of probabilistic knowledge acquired and refined over time. Given an utterance–scene pair (U(t), S(t)) received at time t, the model first calculates an alignment probability a for each word w ∈ U(t) and each potential referent r ∈ S(t). This alignment is calculated by using the meanings of all the words in the utterance, as well as the meanings of their categories, prior to time t, i.e., p(t−1)(.|w) and p(t−1)(.|cat(w, U(t))), respectively. The model then revises the meanings of all the words in U(t) and their corresponding categories by incorporating the recently calculated alignments for the current input pair. This process is repeated for all the input pairs, one at a time.
The goal is to align all the words w in the utterance with all the potential referents r in the scene. As mentioned before, each concept (or potential referent) is represented as a set of semantic features, that is, r = {f}. Alignment follows a few simple intuitions: the more similar the current learned meaning of a word to a referent, the more likely it is that the two are aligned. Conversely, the more similar the current meaning of a word to a referent in the scene, the less likely that the same word is aligned to another referent in the same scene. This also implies that the alignments between words and referents can be many-to-one (e.g., the big blue box may be aligned to the same referent in the scene).
Recall that for each word in the utterance, we can infer its category based on the sentential context. That is, for all w ∈ U(t), we assume to have access to its category c = cat(w, U(t)). Since categories are also assigned a meaning at each time during learning, we can apply the above intuitions to the categories as well: the more similar the meaning of a word category to a referent, the more likely it is that the word is aligned with the referent, and the less likely it is that the word is aligned with another referent in the same scene.
Combining these intuitions, we calculate alignments as in:
where sim(r, x) determines the similarity between potential referent r and x (which can be a word or a category) at this point in time. Recall that each potential referent r is represented as a set of features {f}, and each word or category is represented as a (time-dependent) probability distribution over features. We convert these representations into vectors over the features, and calculate the cosine of the angle between the two vectors as their similarity. Specifically:
where →vr is a vector over all features, in which those features in r are assigned the value 1|r|, and all other features are assigned a value of 0.→vx is a vector over all features, in which each feature f is assigned its current probability, p(t)(f | x) (note that features unseen with a word/category are always assigned a small unseen probability; see below for further details on how the meaning probabilities are estimated).
We need to update the probabilities p(.|w) for all words w ∈ U(t), based on the evidence from the current input pair reflected in the alignment probabilities. However, word meanings are defined as associations between words and features, whereas alignment probabilities are estimated for words and referents, which are collections of features. Therefore, as evidence for the association between w and f, we take the maximum alignment score for w and any of the existing referents which contain the feature f, and add this to the accumulated evidence assoc(w, f ) from prior co-occurrences of w and f. That is:
where assoc(t−1)(w, f ) is zero if w and f have not co-occurred before (i.e., none of the referents co-occurring with w in the past contains the feature f). The model then uses these association scores to update the meaning of the words in the current utterance, as in:
where assoc(t−1)(w, f ) is zero if w and f have not co-occurred before (i.e., none of the referents co-occurring with w in the past contains the feature f). The model then uses these association scores to update the meaning of the words in the current utterance, as in:
where ℱ is the set of all features seen so far, β is an upper bound on the expected number of semantic features, and λ is a small smoothing factor3. We use smoothing to accommodate noisy input by always leaving some (small) portion of the probability mass to currently unseen features.
Once the meaning probabilities of words are updated, the meaning of their corresponding categories are updated accordingly. For each word w in U(t), the meaning distribution of the corresponding category c = cat(w, U(t)) is incrementally updated as in:
For the evaluation of our model, we focus on the word learning experiments of Koehne and Crocker(2010, 2011) (henceforth K&C). These experiments investigate whether adult learners’ knowledge of the sentential context affects their performance in an artificial word learning scenario. We simulate some of these experiments, and show that the behavior of our model is similar to that of adult participants in these studies. Specifically, we simulate Experiment 2 of K&C (2010) and Experiment 2 of K&C (2011) in order to examine the role of sentential cues as an attention mechanism in narrowing down potential referents of object labels in a controlled word learning setup.
The experiments of K&C are based on teaching German adults a semi-natural miniature language in a step-wise fashion. All these experiments follow the same design: in a first phase, learners are familiarized with a set of restrictive and non-restrictive verbs, all of which have clear equivalents in German (e.g., bermamema, “eat”; tambamema, “take”). In this phase, the participants watch an animated depicted action while hearing a spoken verb, and their task is to memorize the name of the action. (Note that this phase is not supposed to reflect realistic verb learning, but only to set the context for the upcoming phase of noun learning.) In a second phase, the participants are exposed to pairs of static scenes and spoken SVO-sentences: Each sentence consists of one of the recently learned verbs and two novel nouns (in subject and object positions, respectively). Each corresponding scene contains referents of these nouns as well as some distractor objects. Whereas nouns appearing in the subject position are always labels for “man” and “woman,” nouns which appear in direct object position are more varied and hence more difficult to learn. In a third phase, participants are tested on how well they have learned the nouns, using a forced choice referent selection trial for each target noun. Specifically, participants hear each target noun while seeing a number of objects as potential referents on a computer screen, and are asked to click on the correct referent. They are also asked to provide a rating that reflects their confidence level in their selected referent. Figure 2 shows a sample input from these experiments.
Figure 2. A sample input scene from experiments of Koehne and Crocker(2010, 2011), paired with a spoken sentence Si gadis bermamema si worel (the woman will eat the broccoli).
Noun learning in the K&C experiments is partly based on the information provided by the restrictive verbs that participants are familiarized with beforehand. Importantly, all verbs have simple German translations and every adult is familiar with the semantic and syntactic use of these German verbs. In particular, based on years of exposure to usages of verbs such as eat and iron in their first language, the adult participants have learned how these verbs constrain their direct objects by imposing certain semantic restrictions on them. That means that learners bring in substantial information about verb selectional preferences from their mother tongue. In our simulations, we need to first take the model to “adult level” by pre-training it on sufficient information from a first language. Only then we can start training it on the artificial language data from K&C experiments. Finally, we must evaluate the model on a task similar to the vocabulary tests in these experiments. In the following sections, we first explain each of the above stages in simulating the experiments from K&C, i.e., pre-training, artificial novel noun learning, and vocabulary test. We then provide details on how we construct an input-generation lexicon of word meanings, as well as how we set the parameters of our model for the experiments reported in this study.
We want our model to have robust knowledge about the semantic requirements of the subject and direct object of our experimental verbs before we start the noun learning stage (as do adult participants in the experiments of K&C). We automatically extract members of the categories SUBJ(V) and DOBJ(V) for each verb V in our experiments from a large corpus of English text, the British National Corpus (BNC)4.
Each experiment consists of different simulations, representing different human participants, such that in each simulation the model receives a different (randomly generated) pre-training data set. To construct a pre-training data set, we randomly generate N input items (each pairing a member of a category with its correct meaning), whose distribution reflects the relative size of the categories and the frequencies of their members. We train the model on these input pairs, and update the category meanings accordingly. At the end of the pre-training phase, each category is associated a meaning, i.e., a probability distribution over semantic features formed as a weighted average of the learned meanings of its members.
Using a computational model allows us to investigate the effect of the participants’ age on their performance in the artificial noun learning task. To simulate younger or older participants, we can easily manipulate the amount of input in the pre-training phase. We thus provide predictions on the role of previous exposure to first language in the artificial noun learning task, which has not been done in the experiments of K&C (where all participants are young adults).
Training on the artificial language has two phases. First, participants are explicitly taught a small set of verbs in isolation and with enough repetition, and are then tested to make sure that they know all the verbs. Second, participants go through a set of trials, where in each trial an utterance is heard over an image containing an array of familiar objects and characters.
We use automatically generated material similar to those of K&C as our training corpus, where each simulation contains a different set of artificial noun learning trials (with the same constraints specified in the corresponding experiments of Koehne and Crocker, 2010, 2011). Since learning verbs is not the main point of these experiments and it is assumed that participants know all the verbs by the time the trials begin, we simply remove them from our training material. However, we assume that learners know about the relation between each noun and the main verb in the sentence (since they are explicitly told about the SVO word order of the utterance). Therefore we mark each noun by the category it belongs to, e.g., DOBJ(IRON). Each utterance is thus of the form “noun: SUBJ(V), noun: DOBJ(V),” associated with a scene representation containing a number of objects, including the correct referents of the two nouns as well as some distractors (each represented as a set of semantic features). The training example shown in Figure 2 will appear to the model as shown in Figure 3.
Figure 3. Processed version of the training item shown in Figure 2.
To test how well a participant has learned the meaning/referent of a recently taught novel noun, it is common to perform a forced choice vocabulary test or referent selection. In such a task, a target noun is presented along with its correct referent and a number of distractor objects, and the participant is asked to choose the target referent. We present our model with one such trial for each novel noun w that appeared during the artificial novel noun learning (the number of novel words to be learned varies across the experiments). We then use the Shepard-Luce choice rule (Shepard, 1957; Luce, 1959) to calculate the probability of choosing each object r in the scene S as the referent of the target noun, as in:
where sim(r, w) is calculated as in equation (3) (page 8).
We automatically construct a lexicon of semantic features for a number of words, which will be used to find the feature-based representation of potential referents appearing in a scene5. The lexicon contains semantic representations for nouns and pronouns only. For nouns, we extract the semantic features from WordNet (Fellbaum, 1998)6 as follows: We take all the hypernyms (ancestors) for the first sense of the word, where each hypernym is a set of synonym words (or synsets) tagged with their sense number. For each hypernym, we add the first word in the synset of each hypernym to the set of the semantic features of the target word (see Figure 4 for examples). We noted that for some nouns appearing in our experimental data, the first WordNet sense was not the intended meaning in our experiments (e.g., the first WordNet sense of broccoli is its “plant” meaning, whereas its intended meaning in our experiments is the “food” sense). We thus manually correct the senses for nouns appearing in our experiments. For pronouns, we manually add a simple feature-based meaning representation. This is especially important for the pre-training stage where we construct the category meanings reflecting the selectional restrictions of our verbs, since many pronouns appear as the subject or direct object of the verbs in our study.
Figure 4. WordNet hypernym hierarchies for broccoli (a food item) and for skirt (a clothing item), as well as their corresponding meanings in our lexicon, extracted from the hypernym hierarchies.
Each experiment consists of K different simulations, such that in each simulation the model receives a different pre-training data set, as well as a different set of novel noun learning trials (which naturally result in different vocabulary test trials). In experiments reported here, we set the number of simulations K to 10. The parameters λ and β in equation (5) are set according to the criteria explained in (Fazly et al., 2010): λ is set to a small value, 10−5, and β is set to 9000, roughly equal to the total number of semantic features in our lexicon.
We present results and analyses of our simulations of two experiments, where we examine the role of informative linguistic context and its interplay with cross-situational evidence in a controlled word learning setup: (i) K&C 2010-Experiment 2, where the two sources of cross-situational and sentence-level evidence provide complementary information (Section 1); and (ii) K&C 2011-Experiment 2, in which the two sources provide redundant information (Section 2).
This experiment investigates the interaction of cross-situational word learning and sentence-level constraints when they provide complementary information. In Phase 1 (verb learning, see Section 4), participants were familiarized with four restrictive and two non-restrictive verbs. In Phase 2 (noun learning), sentence-scene pairs were provided as learning trials, as explained above. Each scene in Phase 2 contained four objects. Twelve novel nouns were introduced, each belonging to one of three within-subject conditions: In Condition No-R(eferential)U(ncertainty), nouns were always preceded by a restrictive verb and there was only one object in the scene which matched the verbal restriction; in Condition Low-RU, verbs were restrictive but there were two plausible referents in the scene; in Condition High-RU, verbs were non-restrictive, leaving four plausible referents for nouns in the direct object position. This means that while in Conditions No-RU and Low-RU learners could use the sentence-level constraints (i.e., verbal restrictions), in Condition High-RU only co-occurrence information was available. In Condition Low-RU, however, successful learning could be achieved only by using cross-situational statistics in addition to this sentence-context evidence.
As predicted, learning rates and confidence ratings were highest in Condition No-RU and lowest in Condition High-RU (with significant differences in confidence ratings). This means that in those conditions in which sentence-level constraints were available (No-RU and Low-RU), learning was better than in cases where it was not (High-RU), revealing that the sentential context can boost noun learning. The fact that learning in Low-RU was successful moreover reveals that participants applied both co-occurrence statistics and sentence-level constraints in parallel for learning noun meanings. Finally, the difference between Conditions No-RU and Low-RU (reflected in confidence ratings) demonstrates that using cross-situational evidence in Low-RU did not completely compensate for the referential uncertainty that was left after using sentence-level constraints. To summarize, this experiment clearly reveals that cross-situational learning and context-based attention mechanisms can successfully be applied in a complementary way.
We simulate this experiment in our model, using automatically generated pre-training and artificial noun learning data (as described in Sections 4.1 and 4.2). For each condition, we run 10 different simulations, where both data sets (pre-training and artificial) are randomly generated and are thus different for each simulation.
To accurately simulate the knowledge of the adult participants in the experiments of K&C about the selectional preferences of the experimental verbs, we set the size of the pre-training data to a relatively large number, here 5000. In addition, we use a manually cleaned version of the selectional preference information for the verbs, where we remove erroneous words or words whose meaning does not match the intended meaning of an argument of a verb.
A summary of results for the three conditions (averaged over 10 simulations for each) are shown as a bar graph in the right panel of Figure 5 (the left panel shows results from the original K&C experiment). Each bar in this graph reflects the average choice probability Pchoice [estimated by equation (7)] of the correct referents for each of the 4 nouns in a condition, averaged across the simulations. Note that we use a slightly different evaluation measure in our simulations than the one used in the original experiments: rather than measuring the proportion of words learned in each simulation, the graph shows the average of the probability of selecting the correct referent in each trial (as explained in Section 4.3). This decision was made based on the observation that in many trials, the model does not have a strong preference toward one referent over another. In such cases, the referent with the highest absolute probability will be unjustly picked as the “winner” even though the difference between its choice probability and that of the next referent is very small7. Therefore, the corresponding “proportion learned” measure for the computational simulations is a rather unreliable measure, and we decided to instead look at the choice probabilities themselves as a more robust indication of the tendency of the model toward treating each object as the correct referent of the target word. Ultimately, we do not directly compare these measures, but rather examine the relative strength of each measure across conditions, as explained below.
Figure 5. Koehne and Crocker (2010), Experiment 2: the left panel depicts the proportion of nouns learned in each condition in the original experiment, and the right panel shows the mean selection probability of the target object in our simulations.
We evaluate the model simulations of this experiment by analyzing Pchoice of the 12 novel nouns (3 conditions, 4 nouns in each condition) by entering the continuous data into linear mixed effect models using linear regression, with participant and item as random factors (as in K&C 2010). Model comparison is used to evaluate whether the fixed factor Condition has a main effect (Baayen et al., 2008). For pairwise comparisons, we calculate Monte Carlo Markov Chain values (MCMCs; Baayen et al., 2008). Condition has a main effect on learning success [χ(2) = 86.202, p < 0.001] with significantly better rates in both Conditions No-RU and Low-RU than in Condition High-RU (see Table 1, rows 3 and 6) and significantly better rates in Condition No-RU than Condition Low-RU (Table 1, rows 2 and 5).
Table 1. Lmer models and p-values from MCMC sampling for learning prob, Exp. 1 prob ∼ Referential Uncertainty + (1|sub) + (1|item).
As is evident from Figure 5, the model’s learning performance is in line with the experimental results for learning rates reported by K&C (2010). In both cases, the performance in Conditions No-RU and Low-RU is significantly better than in Condition High-RU. These results suggest that our model shows similar overall pattern of behavior as human learners: it can use informative linguistic context to narrow down the set of potential referents in the scene for each novel word in an utterance. Therefore, the model performs better when complementary cross-situational and context-based evidence are available and lead to the same direction.
Also in line with the experimental data, the model performed better in Condition No-RU than in Condition Low-RU. This pattern reveals that while cross-situational word learning was used in addition to sentence-level constraints in Condition Low-RU, this did not completely compensate for the advantage of the perfectly disambiguating sentence constraints in Condition No-RU.
One advantage of computational modeling is that we can closely examine the interactions of the different information sources available to our learner in order to see how they affect learning over time. Figure 6 depicts in grayscale the change in the alignment probabilities over the course of training, for a sample target word (sonis) and its target referent (broccoli), and for the three conditions of K&C 2010-Experiment 2. Recall that in these experiments each word is presented in 4 consecutive trials. Darker squares reflect higher probabilities, and the two lightest colors show alignments that are below or just above the baseline of 0.2 (i.e., the probability of randomly selecting the correct target among the five objects in a scene).
Figure 6. Change in alignment probabilities over time, shown in grayscale, for a sample word (sonis referring to broccoli), and for the three conditions of K&C 2010-Experiment 2. Note that in these experiments each word is presented in 4 consecutive trials (t = 1 ‥ 4). Darker squares reflect higher probabilities. The two lightest colors show alignments that are below or just above the baseline of 0.2, which is the probability of randomly selecting the target referent from a set of five objects present in the scene.
The figure shows visualized alignment probabilities that take into account both word-based (cross-situational) and category-based (sentence-level) evidence, marked as “word and category,” and calculated as in equation (2). To better understand the interactions of the two sources of information, the figure also shows alignments calculated using either one of the two sources, referred to as “word-based” and “category-based” in the figure. The latter two alignments are calculated by using variations of equation (2), in which only the relevant similarity scores are used – sim(r, w) and sim (r’,w) for word-based, and sim(r, c) and sim (r’,c) for category-based.
The example shows that across the three conditions, the cross-situational evidence is not sufficient to result in an alignment higher than the baseline. The category-based alignments are also low in the High-RU condition, but higher and more informative in the No-RU and Low-RU conditions. When we use both sources of information, the alignments increase over time to reasonably high values in the No-RU and Low-RU conditions – with higher values in the No-RU condition – but not in the High-RU condition.
In our simulations reported above, we assume that the model has access to perfect categories representing the selectional preferences of each verb. This assumption is justified in the case of adults, who have a clear image of which semantic restrictions are imposed on each grammatical position based on years of exposure to language usage. However, it is interesting to look at the time course of the development of such knowledge, and how it affects word learning.
To investigate the effect of age, we perform experiments with the original noisy version of the selectional preference information, where we pre-train on different amounts of input. Here a noisy input data set represents the confusion and uncertainty that young learners face when receiving and processing language data. Needless to say, children face many challenges other than a high level of ambiguity when learning their first language (we will discuss some of these challenges in Section 6). However, for the purpose of investigating the developmental pattern of using sentential context, using a more noisy version of the input data seems appropriate.
Figure 7 depicts the performance of the model for two different age groups (i.e., for 500 vs. 5000 input items). Once processing 500 noisy input items, the model has a vague and not very informative conception of each category. By the time the model has received 5000 such input items, informative patterns start to emerge (although not as efficiently as the ones in the “clean” version, depicted in Figure 5). Inferential analyses reveal that for input 500, there is a main effect of factor Condition for the choice probability [χ(2) = 6.703, p = 0.035] and a significant difference between Conditions No-RU and High-RU (p = 0.010). For input 5000, we find a much stronger main effect [χ(2) = 26.994, p < 0.001] and significant differences between not only Conditions No-RU and High-RU (p < 0.001) but also between Conditions Low-RU and High-RU (p = 0.001). In other words, the effect of context is much more clear after the model has received sufficient amount of input data and formed informative categories.
Figure 7. Simulation results for K&C 2010-Experiment 2 on noisy pre-training data sets of different size.
This experiment investigates how cross-situational evidence and sentential context work together when they are independently applicable, that is, when they provide redundant information. In Phase 1 of this experiment, participants learned two restrictive and two non-restrictive verbs. In Phase 2, 16 novel nouns were introduced. Each noun had two potential meanings: The high-frequency referent was depicted in the scene in 83% of all presentations of the noun (e.g., in 83% of all presentation of the noun sonis a sausage co-occurred); the low-frequency referent co-occurred with a noun in only 50% of the time (e.g., sonis co-occurred with jeans). All other objects occurred only once with sonis (i.e., 17% of the time). In addition to this manipulation, each noun was in one of two conditions (manipulated within subjects): In Condition R(estrictive), the noun sometimes followed a restrictive verb. Importantly, this verb supported the high-frequency meaning (e.g., the sausage). In Condition N(on-restrictive), the noun was always preceded by a non-restrictive verb. That means that while in Condition N only cross-situational evidence was available (supporting the high-frequency meaning), in Condition R both cross-situational evidence and sentence-level constraints pointed to the high-frequency meaning.
In the forced choice vocabulary test, there were two different trial types. In Test Type 1, the high-frequency object, the low-frequency object, and two distractor objects were depicted (e.g., for sonis: sausage, jeans, tomato, skirt). In Test Type 2, learners could choose among the low-frequency referent and three distractor objects, one of which shared the semantic category (e.g., food) with the (non-present) high-frequency referent (we refer to this referent as the category associate; e.g., apple for sonis). The participants’ selection of referents show an interesting pattern: In Test Type 1 trials, the high-frequency object was chosen significantly more often than the other objects in both conditions. However, the high-frequency object was chosen significantly more often in Condition R than N whereas the low-frequency object was chosen significantly more often in Condition N than R. In R-trials of Test Type 2, participants selected the category associate significantly more often than all other objects whereas in N-trials, both the category associate and the low-frequency object were preferred over the distractors.
These results reveal that pure cross-situational learning (Condition N) works in a parallel and probabilistic manner: People learned the probability of different potential referents for each noun instead of tracking only the best candidate in a deterministic way. Therefore, they were sensitive to differences between co-occurrence frequencies (83 vs. 50 vs. 17%) and preferred to select the 50% low-frequency object over the 17% distractors when the high-frequency object 83% was not available (Test Type 2). However, this sensitivity to differentiate between 17 and 50% of co-occurrence was blocked when sentence-level constraints were available during learning (Condition R): Learning proceeded in a deterministic way and only the referent which was supported by the verb (the high-frequency object) was memorized. Therefore, the low-frequency object was not chosen more often than the distractors in Test Type 2, when the high-frequency was not available. Instead, participants selected an object which was semantically closest to the verb supported high-frequency object (i.e., the category associate).
These results for Test Type 1 and Test Type 2 are shown in the left panels of Figures 8 and 9, respectively.
Figure 8. Koehne and Crocker (2011), Experiment 2, Test Type 1.
Figure 9. Koehne and Crocker (2011), Experiment 2, Test Type 2.
We simulate this experiment by pre-training the model the same as in K&C 2010-Experiment 2: using 10 random simulations, each using an automatically generated pre-training data set of size 5000, and an automatically generated artificial data set that matches the material used in the original experiments. The bar graphs in the right panels of Figures 8 and 9 summarize the performance of our model for Test Types 1 and 2, respectively.
Again we analyze the probabilities of choosing all of the four possible objects in the vocabulary test (Test Type 1: High-Frequency object, Low-Frequency object, Distractor 1, Distractor 2; Test Type 2: Category Associate, Low-Frequency object, Distractor 1, Distractor 2). Here also the data is analyzed using linear mixed effect models. In line with K&C’s results, we find significant differences between conditions for the probabilities of choosing the High-Frequency target [Test Type 1: χ(1) = 289.010, p < 0.001], the Low-Frequency target [Test Type 1: χ(1) = 234.660, p < 0.001; Test Type 2: χ(1) = 401.960, p < 0.001], and the Category Associate [Test Type 2: χ(1) = 80.705, p < 0.001]: Whereas the High-Frequency object and the Category Associate have a significantly higher probability of being chosen in Condition R than Condition N (see Table 2, row 2; Table 3, row 2), the Low-Frequency target is chosen significantly more often in Condition N than Condition R in both test types (see Table 2, row 4; Table 3, row 4).
Table 2. Lmer models and p-values from MCMC sampling for chosen meanings in conditions (high-frequency meaning choices, low-frequency meaning choices), Test Type 1, Exp. 2. Chosen ∼ Verb Typ e + (1|sub) + (1|item), family = binomial(link = “logit”).
Table 3. Lmer models and p-values from MCMC sampling for chosen meanings in conditions (high-frequency meaning choices, low-frequency meaning choices), Test Type 2, Exp. 2. Chosen ∼ Verb Type + (1|sub) + (1|item), family = binomial(link = “logit”).
For both test types, the overall behavior of our model is very similar to that of the participants in the original experiments of K&C (2011). Firstly, learning in Condition N is parallel and probabilistic: The low-frequency referent is chosen by both our model and the human subjects more often than the distractors, and it is the referent which is most strongly favored by our model when the high-frequency object is not available (Test Type 2). Secondly, when nouns are learned based on sentence-level constraints (Condition R), the model shows a clear preference to choose a referent which is congruent with these constraints (high-frequency object in Test Type 1 and category associate in Test Type 2). Interestingly, as the results from Test Type 2 reveal, this preference is still dominant when this object (i.e., the category associate) has a lower co-occurrence rate than another candidate (the low-frequency object).
Figure 11 depicts in grayscale the change in the alignment probabilities over the course of training, for a sample target word (lebah) and its target high-frequency (HF) and low-frequency (LF) referents (cap and tomato, respectively), and for the two conditions of K&C 2011-Experiment 2. Recall that in these experiments each word co-occurs with both its HF and LF referents in 3 consecutive trials, with its HF referent only in the next 2 trials, and with none of the two referents in the final trial. Here again, the figure shows “word-based,” “category-based,” as well as “word and category” alignments; darker squares reflect higher probabilities; and the two lightest colors show alignments that are below or just above the baseline of 0.2 (since there are 5 objects in a scene).
For this example, we can see that in the Non-restrictive condition, the cross-situational evidence is the only reliable source of information, resulting in mild increases over the baseline for the HF referent only. In contrast, in the Restrictive condition, the sentence-level information for the HF referent (reflected in the category-based alignments for this referent) has a very positive effect on the alignments between the word and its HF referent, causing them to substantially increase over the course of training.
As before, we are interested in studying the role of exposure to linguistic knowledge (in our case, the size of the pre-training data set) on the impact of the sentential context. Similar to the previous simulations, we perform experiments with the original noisy version of the selectional preference information, where we pre-train on different amounts of input (500 and 5000 input items). Again, the noisy input data set reflects the less than perfect conception of each category (or contextual constraint). Results for Test Types 1 and 2 are shown in Figures 10 and 12, respectively.
Figure 10. Simulation results for K&C 2011-Experiment 2, Test Type 1 on a noisy pre-training data sets of different size.
Figure 11. Change in alignment probabilities over time, shown in grayscale, for a sample word (lebah referring to cap and tomato), and for the two conditions of K&C 2011-Experiment 2. Note that in these experiments each word is presented with one or both of its referents in 5 consecutive trials (t = 1 ‥ 5). Darker squares reflect higher probabilities. The two lightest colors show alignments that are below or just above the baseline of 0.2, since there are 5 objects in a scene.
Figure 12. Simulation results for K&C 2011-Experiment 2, Test Type 2 on a noisy pre-training data sets of different size.
We perform inferential statistical tests for input 500 and input 5000, and for Test Types 1 and 2. For Test Type 1, we find a marginal effect of factor Condition for the probability of choosing the high-frequency object [high-freq. object: χ(1) = 2.816, p = 0.093] but no effect for the probability of choosing the low-frequency object for input 500 [low-freq. object: χ(1) = 2.668, p = 0.102]. For input 5000, on the contrary, we find significant effects for both the probability of choosing the high-frequency object and the probability of choosing the low-frequency object [high-freq. object: χ(1) = 53.736, p < 0.001; low-freq. object: χ(1) = 53.17, p < 0.001].
For Test Type 2, surprisingly, analyses for input 500 reveal a significant effect of Condition for the probability of choosing the category associate [χ(1) = 6.759, p < 0.010] and a marginal effect of Condition for choosing the low-frequency object [χ(1) = 2.747, p = 0.100]. For input 5000, on the contrary, we found no effect of Condition, neither for the probability of choosing category associate [χ(1) = 0.077, p = 0.782] nor for the probability of choosing the low-frequency object [χ(1) = 1.455, p = 0.228].
To summarize, although cross-situational evidence can be efficiently used to pick the co-occurring referents at an early stage of learning, a significant effect of context can only be observed after the model has received sufficient amount of input data.
Our goal in this paper is to explicitly model the process of integrating cross-situational learning with guidance from sentential context. In a word learning scenario where both types of information are available to human subjects, adults demonstrate a rather complex behavioral pattern. Simulating such patterns by a computational model gives us insight into the dynamics of the interaction between the two mechanisms at play. Experimental studies of word learning provide us with raw material to constrain the nature of the underlying mechanisms. However, specific learning mechanisms such as our model, which yield the same patterns of behavior given similar input data, present concrete suggestions as to which plausible learning mechanisms might be at play in human word learning.
More specifically, we model a context-based attention mechanism via a set of categories, which we assume are inferable from the linguistic context and carry semantic meaning. We believe that humans can learn associations between such context-induced categories and aspects of meaning, and use these associations for reducing uncertainty while learning the meaning of a novel word. The idea of relying on lexical or syntactic categories in word learning is not new: empirical findings suggest that young children gradually form a knowledge of abstract categories, such as verbs, nouns, and adjectives (e.g., Gelman and Taylor, 1984; Kemp et al., 2005). In addition, several unsupervised computational models have been proposed for inducing categories of words which resemble part of speech categories, by drawing on distributional properties of their context (see for example Redington et al., 1998; Clark, 2000; Mintz, 2003; Parisien et al., 2008; Chrupala and Alishahi, 2010). However, explicit accounts of how such categories can be integrated in a cross-situational model of word learning have been rare.
Importantly, such categories are most probably not innate, but emerge through gradual observation of consistent correlation between certain semantic properties in words, and structural and linguistic roles that they adopt in an utterance. Therefore, we hypothesize that the contribution of context-based mechanisms to word learning is age-dependent: the more exposure a model has to input data, the more informative these categories become in narrowing down the set of potential referents of a word. We investigate this trend in Sections 5.1 and 5.2, where we show that the behavior of the model resembles the experimental patterns more closely when it receives more exposure to training data prior to the artificial language training trials. To our knowledge, such effects have not been experimentally studied on children.
In the experiments reported in this paper, we have focused on the acquisition of nouns and how it can benefit from incorporating verb selectional preferences into cross-situational learning. This approach might seem contradictory to previous findings suggesting that nouns are generally learned before verbs. However, assuming that a small number of basic verbs are learned before the beginning of the artificial word learning trials was the result of our attempt at faithfully replicating the design of Koehne and Crocker’s experiments. But this is not an inherent aspect of our model: we have studied simultaneous acquisition of verbs and nouns in the previous versions of the model (Alishahi and Fazly, 2010; Fazly et al., 2010). We are particularly interested in the effect of sentential context on learning verbs, and are planning to study it more carefully in future.
A desirable aspect of our model is its seamless integration of the two mechanisms under study. There are no specific rules, triggers, or parameters that indicate which mechanism should dominate learning in which condition. Instead, the contribution of each information source is determined by the informativeness of that source, and by what the model has learned so far. This is particularly interesting because it shows that varied, sometimes seemingly complex behavioral patterns, can be a result of a simple learning core and the properties of input data.
In our experimental results, we have simulated two sets of findings by Koehne and Crocker(2010, 2011), which investigate adults’ patterns of learning when the two sources of information (cross-situational and sentence-level) are either complementary or redundant. The results of the model are in line with these experimental findings on adults. Koehne and Crocker (2011) report a third set of findings for situations where the cross-situational and sentence-level evidence provide contradictory cues. However, this study crucially relies on learners’ ability to carry sentence-level constraints learned on one trial (in the absence of a scene), to a following trial (with a scene). Our model in its current form does not incorporate such information: Our model processes each input, updates its knowledge of word meanings, and then forgets all other aspects of information available during that trial, including the sentence-level constraints. We are currently investigating the possibility of adding this ability to our model, enabling us to provide explanations for this rather complex interaction of the two sources of evidence in word learning.
To our knowledge, this is the first computational model that has been developed and used to investigate how sentence-level constraints interact with cross-situational statistics in word learning. Importantly, the sentence-level constraints are incorporated into the model as an extra source of probabilistic evidence giving more or less weight to the evidence from cross-situational statistics. This way of modeling the extra source of evidence as a probabilistic piece of knowledge enables us to investigate the interactions of other sources of information with cross-situational statistics in the future.
The authors declare that the research was conducted in the absence of any commercial or financial relationships that could be construed as a potential conflict of interest.
Akhtar, N., and Montague, L. (1999). Early lexical acquisition: the role of cross-situational learning. First Lang. 19, 347–358.
Alishahi, A., and Fazly, A. (2010). “Integrating syntactic knowledge into a model of cross-situational word learning,” in Proceedings of the 32nd Annual Conference of the Cognitive Science Society, Portland.
Altmann, G. T., and Kamide, Y. (1999). Incremental interpretation at verbs: restricting the domain of subsequent reference. Cognition 73, 247–264.
Baayen, R., Davidson, D., and Bates, D. (2008). Mixed-effects modeling with crossed random effects for subjects and items. J. Mem. Lang. 59, 390–412.
Baldwin, D. (2000). Interpersonal understanding fuels knowledge acquisition. Cartogr. Geogr. Inf. Sci. 9, 40–45.
Baldwin, D., Markman, E. M., Bill, B., Desjardins, R. N., Irwin, J. M., and Tidball, G. (1996). Infants’ reliance on a social criterion for establishing word-object relations. Child Dev. 67, 3135–3153.
Carey, S. (1978). “The child as word learner,” in Linguistic Theory and Psychological Reality, eds M. Halle, J. Bresnan, and G. A. Miller (Cambridge: The MIT Press), 264–293.
Carpenter, M., Nagell, K., Tomasello, M., Butterworth, G., and Moore, C. (1998). Social cognition, joint attention, and communicative competence from 9 to 15 months of age. Monogr. Soc. Res. Child Dev. 63, 174.
Childers, J. B., and Paik, J. H. (2008). Korean- and English-speaking children use cross-situational information to learn novel predicate terms. J. Child Lang. 36, 201–224.
Chrupala, G., and Alishahi, A. (2010). “Online entropy-based model of lexical category acquisition,” in Proceedings of the Fourteenth Conference on Computational Natural Language Learning (Uppsala: Association for Computational Linguistics), 182–191.
Clark, A. (2000). “Inducing syntactic categories by context distribution clustering,” in Proceedings of the 2nd Workshop on Learning Language in Logic and the 4th Conference on Computational Natural Language Learning (Morristown, NJ: Association for Computational Linguistics), 91–94.
Fazly, A., Alishahi, A., and Stevenson, S. (2010). A Probabilistic computational model of cross-situational word learning. Cogn. Sci. 34, 1017–1063.
Fisher, C. (2002). Structural limits on verb mapping: the role of abstract structure in 2.5-year-olds’ interpretations of novel verbs. Dev. Sci. 5, 55–64.
Frank, M. C., Goodman, N. D., and Tenenbaum, J. B. (2007). “A Bayesian framework for cross-situational word-learning,” in Advances in Neural Information Processing Systems 20, Vancouver.
Gelman, S., and Taylor, M. (1984). How two-year-old children interpret proper and common names for unfamiliar objects. Child Dev. 55, 1535–1540.
Gertner, Y., Fisher, C., and Eisengart, J. (2006). Learning words and rules: abstract knowledge of word order in early sentence comprehension. Psychol. Sci. 17, 684–691.
Gillette, J., Gleitman, H., Gleitman, L., and Lederer, A. (1999). Human simulations of vocabulary learning. Cognition 73, 135–176.
Kako, E., and Trueswell, J. C. (2000). “Verb meanings, object affordances, and the incremental restriction of reference,” in Proceedings of the Annual Conference of the Cognitive Science Society, Philadelphia.
Kemp, N., Lieven, E., and Tomasello, M. (2005). Young children’s knowledge of the “determiner” and “adjective” categories. J. Speech Lang. Hear. Res. 48, 592–609.
Koehne, J., and Crocker, M. W. (2010). “Sentence processing mechanisms influence cross-situational word learning,” in Proceedings of the Annual Conference of the Cognitive Science Society, Portland.
Koehne, J., and Crocker, M. W. (2011). “The interplay of multiple mechanisms in word learning,” in Proceedings of the Annual Conference of the Cognitive Science Society, Boston.
Landau, B., and Gleitman, L. R. (1985). Language and Experience: Evidence from the Blind Child. Cambridge, MA: Harvard University Press.
Lee, J. N., and Naigles, L. R. (2008). Mandarin learners use syntactic bootstrapping in verb acquisition. Cognition 106, 1028–1037.
Li, P., Farkas, I., and MacWhinney, B. (2004). Early lexical development in a self-organizing neural network. Neural. Netw. 17, 1345–1362.
Maurits, L., Perfors, A. F., and Navarro, D. J. (2009). “Joint acquisition of word order and word reference,” in Proceedings of the 31st Annual Conference of the Cognitive Science Society, Amsterdam.
Mintz, T. (2003). Frequent frames as a cue for grammatical categories in child directed speech. Cognition 90, 91–117.
Naigles, L., and Hoff-Ginsberg, E. (1995). Input to verb learning: evidence for the plausibility of syntactic bootstrapping. Dev. Psychol. 31, 827–837.
Nappa, R., Wessel, A., McEldoon, K., Gleitman, L., and Trueswell, J. (2009). Use of speaker’s gaze and syntax in verb learning. Lang. Learn. Dev. 5, 203–234.
Niyogi, S. (2002). “Bayesian learning at the syntax-semantics interface,” in Proceedings of the 24th Annual Conference of the Cognitive Science Society, Fairfax, 697–702.
Parisien, C., Fazly, A., and Stevenson, S. (2008). “An incremental Bayesian model for learning syntactic categories,” in Proceedings of the Twelfth Conference on Computational Natural Language Learning, Manchester.
Piccin, T., and Waxman, S. (2007). Why nouns trump verbs in word learning: new evidence from children and adults in the human simulation paradigm. Lang. Learn. Dev. 3, 295–323.
Redington, M., Crater, N., and Finch, S. (1998). Distributional information: a powerful cue for acquiring syntactic categories. Cogn. Sci. 22, 425–469.
Regier, T. (2005). The emergence of words: attentional learning in form and meaning. Cogn. Sci. 29, 819–865.
Shepard, R. (1957). Stimulus and response generalization: a stochastic model, relating generalization to distance in psychological space. Psychometrika 22, 325–345.
Siskind, J. M. (1996). A computational study of cross-situational techniques for learning word-to-meaning mappings. Cognition 61, 39–91.
Smith, K., Smith, A. D. M., and Blythe, R. A. (2011). Cross-situational learning: an experimental study of word-learning mechanisms. Cogn. Sci. 35, 480–498.
Smith, L., and Yu, C. (2008). Infants rapidly learn word-referent mappings via cross-situational statistics. Cognition 106, 1558–1568.
Tomasello, M., and Todd, J. (1983). Joint attention and lexical acquisition style. First Lang. 4, 197.
Vouloumanos, A. (2008). Fine-grained sensitivity to statistical information in adult word learning. Cognition 107, 729–742.
Vouloumanos, A., and Werker, J. F. (2009). Infants’ learning of novel words in a stochastic environment. Dev. Psychol. 45, 1611–1617.
Yu, C. (2005). The emergence of links between lexical acquisition and object categorization: a computational study. Conn. Sci. 17, 381–397.
Yu, C. (2006). “Learning syntax–semantics mappings to bootstrap word learning,” in Proceedings of the 28th Annual Conference of the Cognitive Science Society, Vancouver.
Yu, C., and Ballard, D. H. (2008). A unified model of early word learning: integrating statistical and social cues. J. Neurocomput. 70, 2149–2165.
Keywords: probabilistic modeling, cross-situational word learning, syntactic bootstrapping, context-based attention mechanisms
Citation: Alishahi A, Fazly A, Koehne J and Crocker MW (2012) Sentence-based attentional mechanisms in word learning: evidence from a computational model. Front. Psychology 3:200. doi: 10.3389/fpsyg.2012.00200
Received: 31 October 2011; Accepted: 29 May 2012;
Published online: 02 July 2012.
Edited by:
Larissa Samuelson, University of Iowa, USAReviewed by:
Chen Yu, Indiana University, USACopyright: © 2012 Alishahi, Fazly, Koehne and Crocker. This is an open-access article distributed under the terms of the Creative Commons Attribution Non Commercial License, which permits non-commercial use, distribution, and reproduction in other forums, provided the original authors and source are credited.
*Correspondence: Afra Alishahi, Department of Communication and Information Studies, Tilburg Center for Cognition and Communication, Tilburg University, Warandelaan 2, 5037 AB Tilburg, Netherlands. e-mail:a.alishahi@uvt.nl
Disclaimer: All claims expressed in this article are solely those of the authors and do not necessarily represent those of their affiliated organizations, or those of the publisher, the editors and the reviewers. Any product that may be evaluated in this article or claim that may be made by its manufacturer is not guaranteed or endorsed by the publisher.
Research integrity at Frontiers
Learn more about the work of our research integrity team to safeguard the quality of each article we publish.