- 1 Max Planck Institute for Psycholinguistics, Nijmegen, Netherlands
- 2 Key Laboratory of Behavioral Science, Institute of Psychology, Chinese Academy of Sciences, Beijing, China
- 3 Center for the Study of Applied Psychology, South China Normal University, Guangzhou, China
- 4 Centre for Cognitive Neuroimaging, Donders Institute, Radboud University Nijmegen, Nijmegen, Netherlands
Gamma-band neuronal synchronization during sentence-level language comprehension has previously been linked with semantic unification. Here, we attempt to further narrow down the functional significance of gamma during language comprehension, by distinguishing between two aspects of semantic unification: successful integration of word meaning into the sentence context, and prediction of upcoming words. We computed event-related potentials (ERPs) and frequency band-specific electroencephalographic (EEG) power changes while participants read sentences that contained a critical word (CW) that was (1) both semantically congruent and predictable (high cloze, HC), (2) semantically congruent but unpredictable (low cloze, LC), or (3) semantically incongruent (and therefore also unpredictable; semantic violation, SV). The ERP analysis showed the expected parametric N400 modulation (HC < LC < SV). The time-frequency analysis showed qualitatively different results. In the gamma-frequency range, we observed a power increase in response to the CW in the HC condition, but not in the LC and the SV conditions. Additionally, in the theta frequency range we observed a power increase in the SV condition only. Our data provide evidence that gamma power increases are related to the predictability of an upcoming word based on the preceding sentence context, rather than to the integration of the incoming word’s semantics into the preceding context. Further, our theta band data are compatible with the notion that theta band synchronization in sentence comprehension might be related to the detection of an error in the language input.
Introduction
The term semantic unification (in the sense implied by, e.g., Jackendoff, and the related computational model by Vosse and Kempen; Vosse and Kempen, 2000; Jackendoff, 2007, which differs from Shieber’s use that relates to constraint-based lexicalist grammars; Shieber, 1985) refers to the integration of lexically retrieved semantic information into a representation of multiword utterances, as well as the integration of meaning extracted from non-linguistic modalities (Hagoort, 2005; see also Baggio and Hagoort, 2011). As such, semantic unification is at the heart of the combinatorial nature of language. Over the past decades, the process of semantic unification has been studied extensively by means of the N400 component of the event-related potential (ERP; for review, see Lau et al., 2008; Kutas and Federmeier, 2011). From this extensive literature, the notion has emerged that the N400 is related to at least two aspects of semantic unification: semantic integration of words into their preceding context, and the facilitation, based on increased predictability of the upcoming word through the preceding context, of the lexical retrieval of that word.
In recent years, evidence has accumulated that another class of event-related electroencephalographic (EEG) responses, namely event-related changes in gamma-frequency oscillations (i.e., above 30 Hz), are also sensitive to semantic unification operations (see below for detailed arguments). In this paper we present evidence that further specifies the functional significance of the reactivity of gamma-band activity in the context of language comprehension processes.
Event-related changes in EEG (and MEG, for that matter) oscillations are considered to be a reflection of changes in synchronization of underlying neuronal populations (Varela et al., 2001; Fries, 2005). Those changes in synchronization, in turn, have been proposed to constitute the basis of the transient coupling and uncoupling of functional networks in the brain (Singer, 1993, 2011). Thus, studying the reactivity of EEG oscillations provides a window onto the functional network dynamics underlying cognition. The typical ERP procedure of averaging segments of EEG time-locked to experimental events largely cancels out event-related changes in EEG oscillations, because the latter are inherently not phase-locked to the event in question (see Bastiaansen et al., 2012 for a more detailed discussion). One solution to this problem is to perform time-frequency (TF) decompositions of EEG power, or coherence, which captures the increases and decreases in power and/or coherence in specific frequency bands.
Applying such techniques to EEG recorded during language comprehension has shown that different aspects of language comprehension systematically affect power and coherence estimates in different frequency ranges (see Bastiaansen and Hagoort, 2006 for a review). For example, retrieval of word-level information is mostly accompanied by increased neuronal synchronization in the theta frequency range (4–7 Hz; Bastiaansen et al., 2005, 2008). Sentence-level syntactic binding, in contrast, induces neuronal synchronization in the beta frequency band (12–30 Hz; Haarmann et al., 2002; Weiss et al., 2005; Bastiaansen and Hagoort, 2010; Bastiaansen et al., 2010).
Most relevant to our present purpose however, is the notion that there is a relationship between gamma-band neuronal synchronization and sentence-level semantic processing. For example, in one study (Hald et al., 2006), an increase in gamma power (around 40 Hz) was observed in response to a high cloze (HC) critical word (CW) presented in a sentence context. This gamma increase was abolished when the CW was semantically anomalous. Similarly, using EEG coherence analysis, one study (reviewed in Weiss and Mueller, 2003) reported increased gamma-band coherence between left frontal and left temporal electrodes for a semantically correct CW compared to a semantically anomalous CW. In another study (van Berkum et al., 2004) we observed a gamma power increase for referentially correct CWs, that disappeared when these CWs were referentially ambiguous, or did not have a proper referent. What all these findings have in common, is an increase in gamma (∼40 Hz) power or coherence when semantic unification can be routinely performed, and a disruption of this gamma increase when semantic anomalies are encountered. Based on these findings, we have proposed (Varela et al., 2001; Bastiaansen and Hagoort, 2006) that gamma-band neuronal synchronization is related to normal, ongoing semantic unification operations. Two recent studies, in which gamma power time courses were computed across entire sentences, further support this proposition. In the first (Peña and Melloni, 2012), gamma power increases were observed only when Spanish or Italian monolinguals listened to sentences in their own language, not when they listened to sentences spoken in a phonologically related or an unrelated language. Note however that in this study, gamma power changes were observed in a higher gamma-frequency range (around 70 Hz) than in the previously mentioned studies. In the second study (Bastiaansen and Hagoort, 2010) larger gamma power was observed across semantically congruent sentences compared to sentences that were syntactically correct, but semantically anomalous (so-called syntactic prose).
However, in all the papers described here, the conditions in which a disruption of gamma-band synchronization was observed were constituted of severe semantic violations (SVs). These manipulations inherently confound different aspects of language comprehension. The contrast is always between semantically congruent, and predictable words on the one hand, and semantically incongruent, unpredictable words, that violate the “rules” of combinatorial semantics on the other hand. It is therefore unclear whether the observed gamma-band differences are related to differences in the extent to which the incoming word can be semantically integrated into its preceding context, or to differences in the predictability of the incoming word.
In this work, we attempt to disentangle this confound by introducing an additional condition that holds an intermediate position between the congruent and predictable word in a sentence context and its incongruent and unpredictable counterpart. We created three different conditions. In the first condition, sentences were presented that contained a CW that was both semantically congruent (operationally defined as yielding high semantic acceptability ratings in a pre-test) and highly predictable (operationally defined as yielding a HC probability in a pre-test). We termed this the HC condition. In the second condition, termed the low cloze (LC) condition, the CW was also semantically congruent (high semantic acceptability ratings), but unpredictable (having a cloze probability of zero in the pre-test). Finally, in the SV condition, the CW was both incongruent and unpredictable, as indicated by low semantic acceptability ratings and a zero cloze probability.
Subjects were reading sentences in each of these three conditions while their EEG was measured, and we performed an ERP analysis, and a TF analysis of power changes in a wide range of frequencies (from 2 to 80 Hz). For the ERP analysis, we expected to observe a parametric modulation of the N400 amplitude (SV > LC > HC), because the N400 is thought to be related both to the semantic congruency and to the predictability of the CW. For the TF analysis, we hypothesized effects in two different frequency ranges, theta and gamma. For the theta frequency range, under the hypothesis that theta power changes reflect processes related to lexical-semantic retrieval, we expected larger theta power in response to CWs in the LC and SV conditions, given that retrieval is more difficult/more effortful when the lexical item is not predictable on the basis of the preceding sentence context. Our hypotheses concerning gamma power changes were as follows. If gamma-band synchronization is primarily related to the successful semantic integration of the CW into its preceding sentence context, we should observe a gamma power increase after the CW in both the HC and LC conditions, since the CWs in both conditions were semantically congruent with the sentence. No gamma power increase should be observed after the CW in the SV condition. In contrast, if gamma is related to the predictability of the incoming word, a gamma power increase should be observed only in the HC condition, not in the LC or SV conditions. In brief, the presence or absence of a gamma power increase in the intermediate, LC condition determines which of the two hypotheses is supported by our data.
Materials and Methods
Participants
Twenty-eight healthy native speakers of Dutch participated in the experiment. All of them were right-handed, with normal or corrected-to-normal vision, and without neurological impairment. Four participants were excluded from further analysis, because of excessive EMG activity in the EEG recordings, which hinders a good view on gamma-band responsiveness. Thus the final sample consisted of 24 healthy native speakers of Dutch participated in the experiment (19 females; mean age: 23 years; age range: 18–32). All of them signed the informed written consent form according to the local ethics committee and the declaration of Helsinki. After the experiment, they received a small participant fee or course credits.
Stimulus Materials
Experimental items
The stimuli consisted of three types of sentences: HC sentences, LC sentences, and sentences that contained a semantic incongruence (SV). First, sentences with a highly constraining context were constructed (for the HC condition). Each of these sentences was modified by replacing a noun with a semantically unexpected noun that was nevertheless semantically congruent with the context, to form the LC condition. Next the same noun was replaced with a noun that was semantically incongruent with the preceding sentence context, leading to the SV condition sentences. Example stimuli are given in Table 1. We constructed 108 sentences in each condition1, resulting in 324 sentences in total. The CWs across conditions were matched on word length and frequency: log frequency in CELEX (Baayen et al., 1993): HC = 2.71, LC = 2.70, SV = 2.69, F(2,646) = 2.35, p = 0.10.
Pre-tests
In order to validate our stimulus materials, we conducted a cloze probability test (with 20 participants that did not participate in the EEG experiment) and a semantic acceptability rating (with another 20 participants). The cloze probability test was used to quantify the predictability of the CWs, but it should be noted that our experimental materials are not representative of Dutch texts in general. Our use of the term “predictable” or “prediction” should be understood to refer to the predictability of the CW, given the preceding (isolated) sentence context.
We wanted CWs in the HC condition to have a HC probability, and to be semantically acceptable. Likewise, CWs in the LC condition should have a LC probability and be semantically acceptable. Finally, the CWs in the SV condition should have both a LC probability and be semantically unacceptable.
Cloze probabilities of the CWs were obtained by presenting sentences up until the word before the CW position, and participants were instructed to fill in the first noun that came to their mind and made the sentence meaningful. The average cloze probability of the CW in the HC condition was 68% (SD = 19.5, ranging from 33.3 to 100). Then the CWs were replaced by unexpected words to construct the LC and violation sentences.
Semantic acceptability ratings of all sentences were obtained on a 7-point Likert scale, 1 being entirely unacceptable, and 7 being fully acceptable. The average ratings for the HC, LC, and SV conditions were 6.16 (SD = 0.63), 5.40 (SD = 1.02), and 1.77 (SD = 0.63), respectively. The acceptability ratings were significantly different across sentence type: [F(2,646) = 2952, p < 0.001]. The ratings for HC were higher than those for LC [F(1,19) = 462, p < 0.001] and SV [F(1,19) = 1965, p < 0.001], and the ratings for LC were higher than that of SV [F(1,19) = 2352, p < 0.001].
Note that predictability and congruency are inherently not fully orthogonal factors, as semantically incongruent words are by definition unpredictable. Therefore, our study critically hinges on whether predictability and semantic acceptability can be manipulated independently. The behavioral pre-tests on our stimulus materials support this, although there is a small but significant carry-over effect from cloze probability to semantic acceptability, as evidenced by the significant decrease in semantic acceptability ratings from HC (6.16) to LC (5.40). However, we feel that a semantic acceptability score of 5.4 out of 7 for the LC items still indicates that the LC CWs were considered semantically acceptable.
Finally, in order to further assess to which extent the cloze probability and semantic acceptability ratings are related, we computed the correlation between these two scores for the HC condition (for the LC and SV conditions, all cloze probabilities were zero). The correlation coefficient between cloze probability and semantic acceptability for the HC condition was 0.04, and this was not significant (p = 0.40).
Filler items
To avoid sentence wrap-up effects, the CWs in the experimental item set appeared either at the 6th (50%) or the 7th (50%) position in each sentence. In order to avoid the participants expecting the CW in a specific position, 90 incongruent filler sentences were constructed, in which the violating words appeared at the 8th, 9th, or 10th position in the sentence (30 sentences for each CW position). Further, to counterbalance the number of congruent and incongruent sentences, 90 semantically fully congruent filler sentences were also constructed.
Experimental lists
The total item set of 504 sentences (324 experimental, 180 fillers) were split into two halves (one for the current experiment, one for another experiment not reported here, see also text footnote 1). Each half contained 54 HC, 54 LC, and 54 SV sentences. Additionally, 45 filler sentences with the violating word at the 8th, 9th, or 10th position, and another 45 congruent filler sentences. In total, each half thus included 252 sentences. For each half, three experimental lists were constructed, by randomizing presentation order of the sentences. Each participant was assigned one of the lists randomly.
Design and Procedure
Participants were asked to read 252 sentences for comprehension. The sentences were presented in pseudorandom order (162 of which were critical for the current study) in a word-by-word manner (Presentation software, Neurobehavioral Systems). The words were presented as white characters on a black background at the center of a computer screen, positioned ∼80 cm away from the participants.
A trial started with a fixation cross of either 1650, 2400, or 3150 ms duration (2400 ms on average). Each of the 11 words in each sentence was then presented for 300 ms, followed by a 300-ms blank screen. A trial thus took 9 s on average.
Participants were told not to move or blink when individual words appeared. The 252 sentences were presented in six blocks of 42 trials each, about 6 min for each block. The participants were asked to take a self-paced rest between blocks of maximally 1 min. They had to press a button to continue if they wanted to continue before the 1-min rest was over.
EEG Recordings
EEG data were collected from 29 standard channels (10/20 system) ActiCap (Brain Products, Munich, Germany), plus three additional EOG electrodes. The EEG was recorded continuously with a 10-s time constant and a 125-Hz low pass filter, and sampled at 500 Hz. All electrode impedances were kept below 10 kΩ.
EEG Data Analysis
Pre-processing
Brain Vision Analyzer software 1.05 (Brain Products) was used to pre-process the raw EEG data. The EEG data were re-referenced off-line to the average of both mastoids, and filtered with a 1-Hz high pass filter. Horizontal EOG was bipolarly derived from two EOG electrodes placed on the outer canthi of both eyes. Vertical EOG was bipolarly derived from an EOG electrode placed below the left eye, and an anterofrontal EEG electrode located right above the left eye. Then, the data were segmented from −1 to 2.5 s relative to the onset of the CW’s. After that, a semi-automatic artifact rejection procedure was applied. We used three rejection criteria simultaneously: an amplitude criterion of ±90 μV, a gradient criterion (i.e., the maximum admissible voltage step between two adjacent time points) of 50 μV, and a difference criterion (i.e., the maximum admissible absolute difference between two values within each EEG epoch) of 120 μV. On average, 50 trials were left for each condition, with no significant difference in trial numbers between conditions [F(2,46) = 1.504, p = 0.233].
ERP analysis
For the computation of the ERP, we applied an additional low pass filter at 25 Hz. Then, epochs between −0.15and 1 s relative to CW onset were defined, followed by a baseline correction from −0.15 to 0 s preceding CW onset. After that, we averaged all trials in each condition for each subject. To quantify the N400 amplitude differences, a time window of 0.3–0.5 s was selected during which the mean amplitude values were calculated.
Time-frequency analysis
Time-frequency analysis was performed with the Fieldtrip software package, an open-source Matlab toolbox for neurophysiological data analysis (Oostenveld et al., 2011). In order to optimize the tradeoff between time and frequency resolution, TF representations of the single-trial data (epochs from −1 to 2.5 s relative to the CW’s) were computed in two different, partially overlapping frequency ranges. In the low frequency range (2–30 Hz), a 400-ms Hanning window was used to compute power changes in frequency steps of 1 Hz and time steps of 10 ms. In the high frequency range (25–80 Hz), a multitaper approach, as described by (Mitra and Pesaran, 1999), was used for computing the TF representations. Power changes were computed with a 400-ms time-smoothing and a 5-Hz frequency-smoothing window, in 2.5 Hz frequency steps and 10 ms time steps. After acquiring the TF representations of single trials, we averaged the power estimates over trials separately for the three conditions, for each subject. The resulting subject-averaged power changes in the post-stimulus interval were expressed as a relative change from the baseline interval (from −0.5 to −0.15 s).
Time-frequency analysis of the ERP data
A standard TF analysis (based on single-trial data) reveals the EEG amplitude changes that are time-locked, but not phase-locked to the event (i.e., the true oscillations, or so-called induced responses). However, it additionally contains those amplitude changes that are both time- and phase-locked to the eliciting event (the ERPs, or evoked responses). Therefore, differences between conditions observed in the standard TF analysis may potentially be (partially) caused by differences in the (TF contents of the) ERP waveforms. In contrast, a TF analysis of the ERP data (based on subject-averaged ERPs) contains only the evoked responses. So in order to determine whether the results of the standard TF analysis reflects “true” changes in oscillatory dynamics, or whether these results can be accounted for by the (TF contents of the) ERP data, we performed a TF analysis based on the subject-averaged ERP data, and qualitatively compared the results of both analyses.
First, the average ERP in each condition was computed by averaging the epochs from −1 to 2.5 s relative to the CWs. Then the TF representations of the ERP data were obtained in a similar way as described in the Section “Time-Frequency Analysis.” After that, the TF representations were averaged across all the 24 subjects.
Statistical analysis
For a statistical evaluation of the ERP and TF responses, we used a cluster-based random permutation test (Maris and Oostenveld, 2007). This statistical procedure optimally handles the multiple-comparisons problem, while at the same time retaining reasonable statistical power. It naturally takes care of interactions between time points, electrodes and frequency bins by identifying clusters of significant differences between conditions in the time, space, and frequency dimensions. The procedure is briefly described below.
First, for every data point (electrode for the ERP data, electrode by time by frequency for the TF data) of two conditions, a simple dependent-samples t test is performed (giving uncorrected p-values). All adjacent data points exceeding a preset significance level (5%) are grouped into clusters. For each cluster the sum of the t statistics is used in the cluster-level test statistic. Next, a null distribution which assumes no difference between conditions is created. This distribution is obtained by 1000 times randomly assigning the conditions in subjects and calculating the largest cluster-level statistic for each randomization. Finally, the actually observed cluster-level test statistics are compared against the null distribution, and clusters falling in the highest or lowest 2.5th percentile are considered significant.
Results
ERP Data
Figure 1A displays the grand average waveforms elicited by the CW’s in three conditions (HC; LC; and SV) at a representative electrode (Pz). Visual inspection showed that the SV condition elicited the largest N400 amplitude, while the HC condition elicited the smallest N400 amplitude. Figure 1B displays the N400 scalp topographies for the three conditions. Statistical analyses confirmed that the N400 amplitude (in the time window of 0.3–0.5 s) was parametrically modulated by condition, with SV yielding the largest N400 (i.e., the most negative values), LC intermediate, and HC the smallest N400 (SV < HC, p < 0.001; SV < LC, p = 0.01; and LC < HC, p < 0.001). Figure 1C shows the difference topographies of the N400 effects between each two conditions. All of them had a centro-parietal distribution, which is consistent with the classical N400 effect.
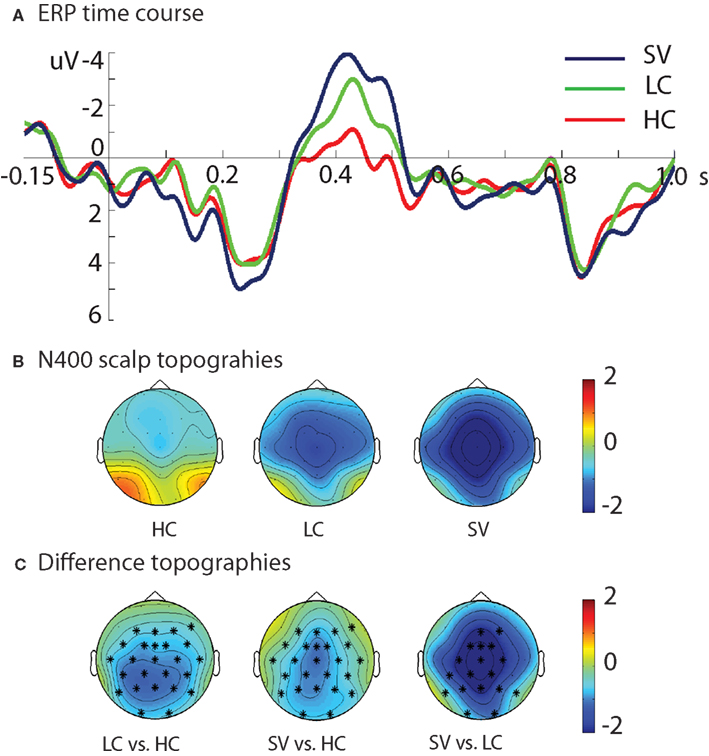
Figure 1. Results of the ERP analysis. (A) ERP time course for each of the three conditions at electrode Pz. (B) Scalp topographies of the N400 amplitude for the three conditions in the time window of 0.3–0.5 s. (C) The difference topographies for all comparisons in the time window of 0.3–0.5 s. The electrodes that showed significant N400 effects were marked by *. HC, high cloze sentences; LC, low cloze sentences; SV, sentences containing a semantic violation.
In addition to the N400 differences, visual inspection of the waveforms also suggested that the SV condition elicited a larger early positivity between 0.1 and 0.3 s than the HC and LC conditions. Post hoc analyses in this time window confirmed this observation: SV > HC, p = 0.02; SV > LC, p = 0.07; LC vs. HC, no significant cluster.
TF Data
The TF representations of each pair of conditions as well as the contrasts between them are shown in Figure 2 (HC–LC), Figure 3 (HC–SV), and Figure 4 (LC–SV). For the high frequencies (30–80 Hz), the contrasts HC–LC and HC–SV both showed one significant cluster (p = 0.048 and 0.005, respectively), in the gamma-frequency range (40–50 Hz) between 0.2 and 1.0 s, while the contrast of LC–SV showed no significant difference.
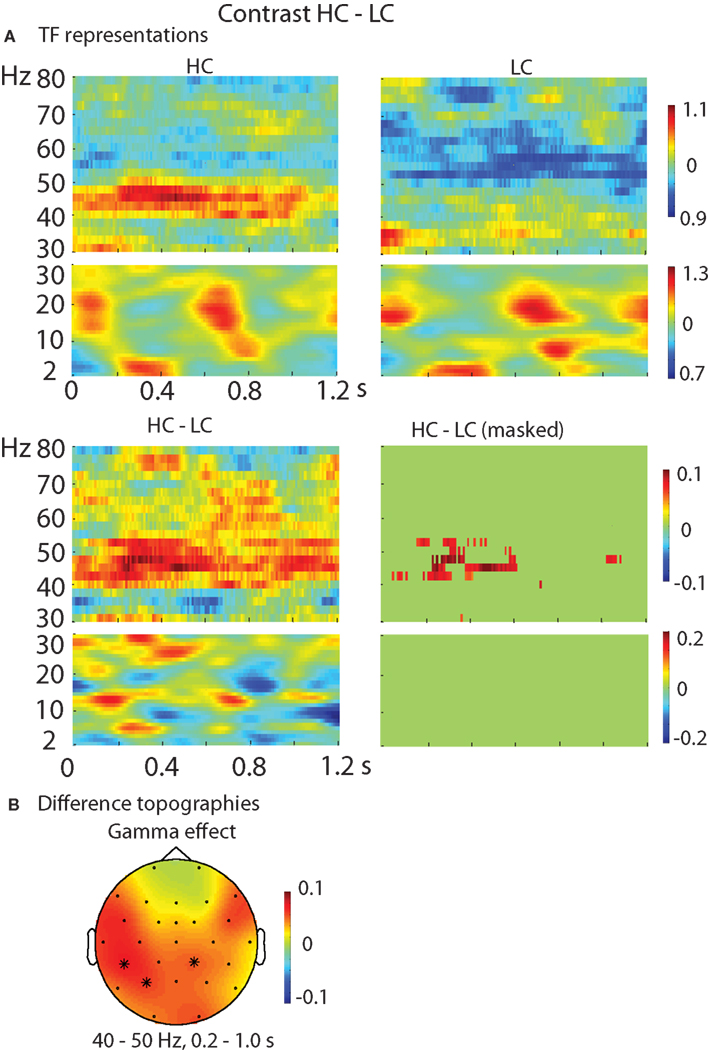
Figure 2. Results of the TF analysis in the HC and LC conditions. (A) The upper panel shows the TF representations of the HC and LC conditions at electrode Pz, separately for the high and low frequency ranges. The lower panel shows the contrast between the HC and LC conditions, with the left one showing the raw difference, and the right one showing the statistically thresholded difference. (B) The difference topographies of HC–LC in the gamma band (40–50 Hz, left) in the time interval of 0.2–1.0 s. The electrodes that showed significant effects over 30% of the selected time intervals were marked by *. HC, high cloze critical words; LC, low cloze critical words.
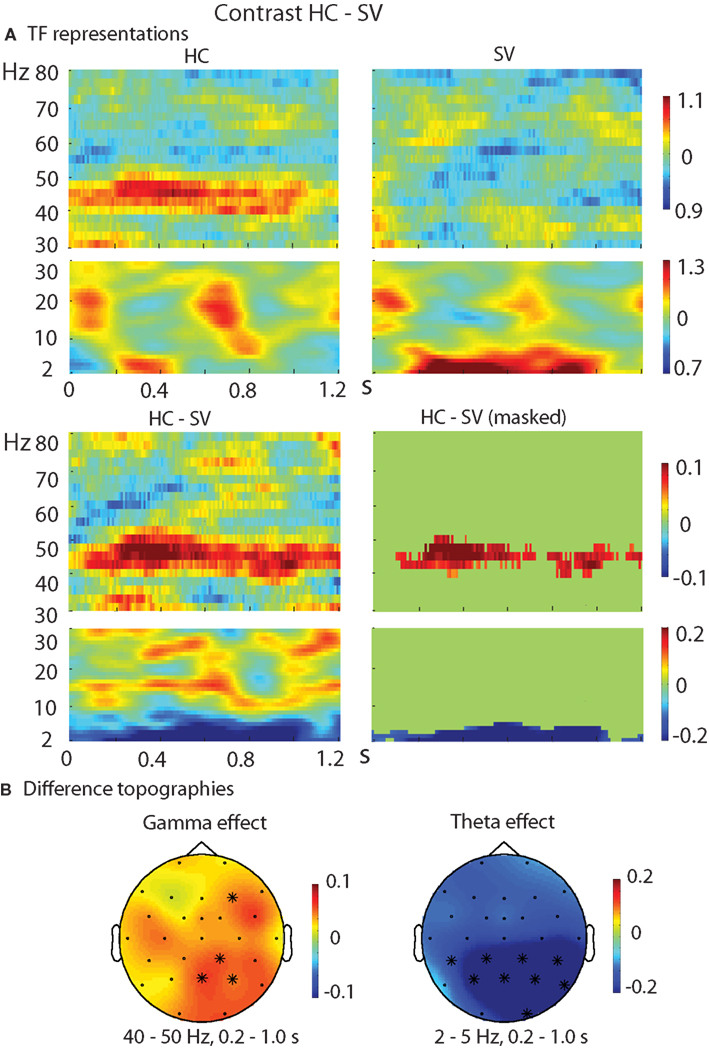
Figure 3. Results of the TF analysis in the HC and SV conditions. (A) The upper panel shows the TF representations of the HC and SV conditions at electrode Pz, in both the high and low frequency ranges. The lower panel shows the contrast between the HC and SV conditions, with the left one showing the raw difference, and the right one showing the statistically thresholded difference. (B) The difference topographies for HC–SV in the gamma band (40–50 Hz, left) and theta band (2–5 Hz, right) in the time interval of 0.2–1.0 s. The electrodes that showed significant effects over a certain percentage of the selected time intervals (30 and 80%, respectively, for the gamma and theta bands) were marked by *. HC, high cloze critical words; SV, semantically incongruent critical words.
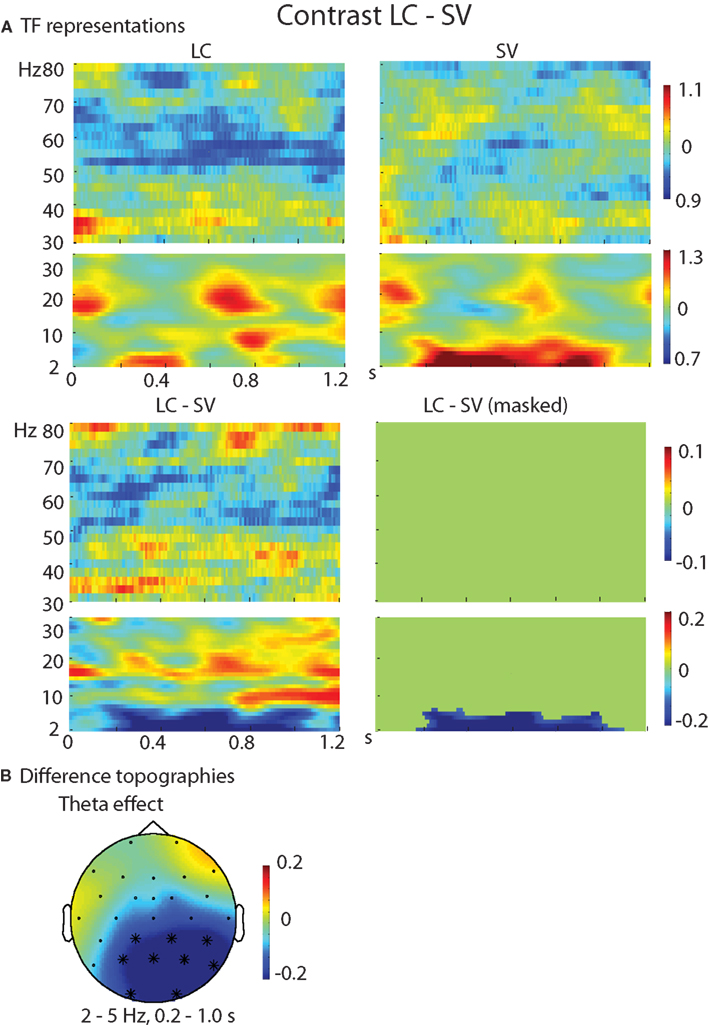
Figure 4. Results of the TF analysis in the LC and SV conditions. (A) The upper panel shows the TF representations of the LC and SV conditions at electrode Pz, in both the high and low frequency ranges. The lower panel shows the contrast between the LC and SV conditions, with the left one showing the raw difference, and the right one showing the statistically thresholded difference. (B) The difference topographies for LC–SV in the theta band (2–5 Hz) in the time interval of 0.2–1.0 s. The electrodes that showed significant effects over 80% of the selected time intervals were marked by *. LC, low cloze critical words; SV, semantically incongruent critical words.
For the low frequencies (2–30 Hz), the contrasts of HC–SV and LC–SV both revealed one significant cluster (p = 0.003 and 0.005, respectively), at the lower edge of the theta frequency range (2–5 Hz) in the time interval of 0.2–1.0 s, while no significant cluster was observed in the contrast of HC–LC. Figure 5 shows the time course of the evolution of both gamma (40–50 Hz) and theta power (2–5 Hz) after CW onset at electrode Pz. Figure 6 shows the scalp topographies for theta and gamma power changes in the relevant time and frequency intervals.
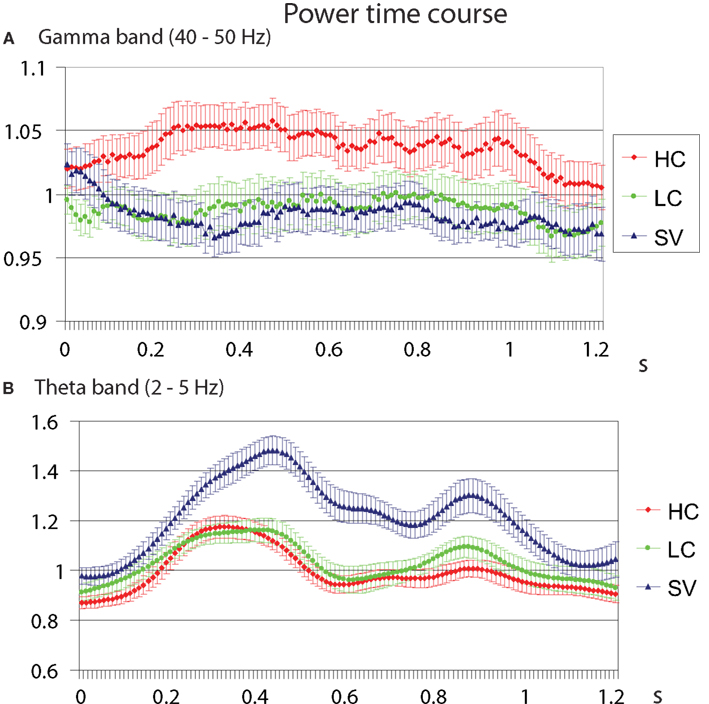
Figure 5. Temporal evolution of the evolution of gamma (A) and theta (B) power between 0 and 1.2 s after the critical word onset, for the three conditions, at electrode Pz. Error bars indicate standard errors around the mean, for illustrative purposes.
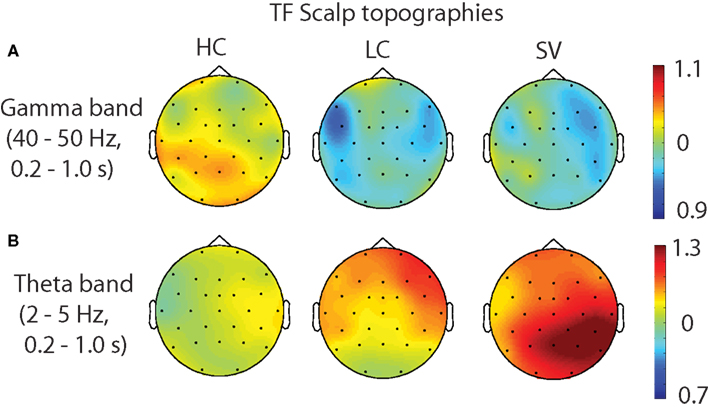
Figure 6. Scalp topographies of each individual condition (A) for the theta band (2–5 Hz) and (B) for the gamma band (40–50 Hz) in the time interval of 0.2–1.0 s. HC, high cloze critical words; LC, low cloze critical words. SV, semantically incongruent critical words.
TF Transform of the ERP
The TF analysis of the ERPs revealed that significantly larger theta power was produced by the SV condition than the HC condition in an interval ranging from 0.2 to 0.6 ms after CW onset (p = 0.008) and theta power in the SV condition was marginally significantly larger than in the LC condition, in a time interval between 0.2 and 0.45 ms after CW onset (p = 0.057). No difference was found between the HC and LC conditions (p > 0.30).
Figure 7 presents the TF representations obtained after the TF analysis on both single trials (Figure 7A) and on ERPs (Figure 7B) in the three conditions. Both analyses revealed that the SV condition elicits a larger theta power (2–5 Hz) than the other two conditions. Importantly however, for the analysis on ERPs, the effect was more restricted in time, and corresponds to the time window of the N400 and its preceding positive peak, whereas for the standard TF analysis, it extends until 1 s after CW onset.
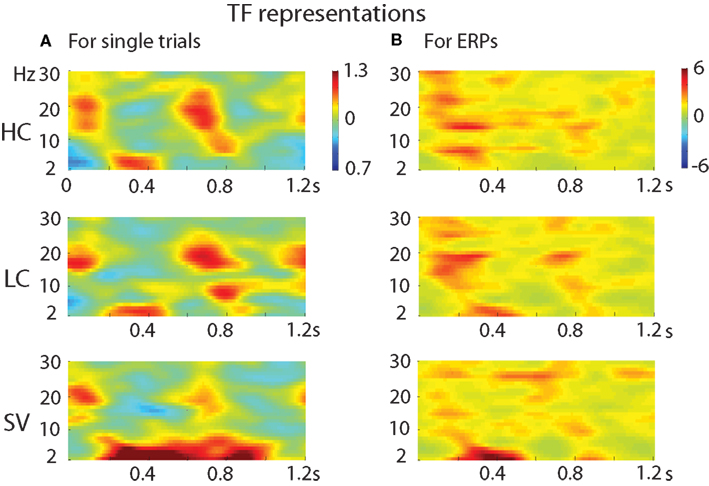
Figure 7. Comparison of TF analyses on (A) single trials and on (B) averaged ERPs in the three conditions.
Discussion
In order to narrow down the functional significance of gamma-band neuronal synchronization during on-line language comprehension, we computed ERPs and frequency band-specific EEG power changes while participants read sentences that contained a CW that was both semantically congruent and predictable (HC), semantically congruent but unpredictable (LC), or semantically incongruent and therefore also unpredictable (SV). In the ERP data, we observed a parametric increase in N400 amplitude from HC to LC to SV. In the gamma-frequency range, we observed a power increase in response to the CW in the HC condition, but not in the LC and the SV conditions. In the theta frequency range, we observed a power increase in the SV condition only.
N400 Effects Are Sensitive both to Predictability and to Congruency
The parametric modulation of N400 amplitude is in line with what we hypothesized, and indicates that in the present study the N400 was sensitive both to semantic congruency of the CW to its preceding sentences context (hence the larger N400 to the SV condition) and to the predictability of the CW (hence the differential responses to HC and LC). This is in line with most studies that have addressed the sensitivity of the N400 to these two factors, and is also in line with the general consensus in the field that predictability (which facilitates lexical retrieval) and congruency are the two factors influencing the amplitude of the N400 component (Lau et al., 2008; Kutas and Federmeier, 2011).
Gamma Power Increases Are Related to Predictability of the Incoming Word
The TF results show that a gamma power increase is observed only in response to words with a HC probability, that is, to words that are predictable on the basis of their preceding sentence context. The gamma increase from LC to HC indicates a sensitivity to predictability, without fully ruling out the possibility of semantic integration playing a role in the generation of gamma, given the small but significant differences in semantic acceptability ratings for these conditions. However, the lack of a gamma increase from SV to LC does rule out that gamma is related to successful semantic integration. In sum, the full pattern of results is only compatible with an interpretation of the observed gamma effect in terms of predictability of an incoming word, rather than with a semantic integration account. Under the latter account, we would also have expected a gamma power increase for unpredictable yet semantically congruent CWs (the LC condition), because those words can be as successfully integrated into the sentence context as their predictable counterparts (the HC words).
The question then arises, what exactly does this gamma power increase reflect? Unpacking the notion of predictability a bit further, one can differentiate between actual prediction of a lexical item, and the downstream consequences of the predictability of the item. In our data, it is clear that it cannot be the actual prediction of the upcoming CW that causes the gamma power increase, because the effect is observed in response to the CW, not prior to it.
A viable hypothesis, however, is that the gamma increase is related to a match between the predicted word and the actually incoming word. As said, it is generally agreed that a power increase is related to an increase in neuronal synchronization in that particular frequency band (Varela et al., 2001; Fries, 2005), and that those changes in synchronization are indicative of the (un)coupling of functional networks (Singer, 1993, 2011). Therefore, we tentatively propose that the observed gamma increase is related to a match between the pre-activation of the neural representation of the predicted word, and the neural representation of the actually incoming word. This would fit into the more domain-general notion that gamma is related to a match between top-down and bottom-up processes, the so-called match-and-utilization model of gamma-band synchronization (Herrmann et al., 2004).
Are Theta Power Increases Related to the Detection of Semantic Errors?
In addition to the gamma-band effects, we observed a power increase between roughly 2–5 Hz (which is at the lower edge of the theta frequency range) for the SV condition only. Comparing the TF analyses on the single-trial data with the TF analysis on the averaged ERP data (Figure 7), one can see that this theta power increase is only partly produced by differences in the ERP waveshape: the theta power increase in the single-trial-based TF analysis (which contains both phase-locked and non-phase-locked changes in theta, cf. Bastiaansen et al., 2012) is longer-lasting (roughly 0.2–1 s post-stimulus) than that in the ERP-based TF analysis (0.2–0.6 s) which contains only phase-locked changes.
The observed pattern of results in the theta frequency range is not in line with what would be expected under the hypothesis that theta is related to lexical retrieval. As said in the introduction, under this hypothesis we expected larger theta power for both the SV and the LC conditions, but we observed it only for the SV condition. It is tempting to relate our present theta effect to the detection of a violation in the sentence, based on (1) the observed pattern in the present study, (2) previous reports of theta power increases in response to syntactic (Bastiaansen et al., 2002b) and semantic (Hald et al., 2006) violations in sentences, and (3) the apparent relationship between theta and error detection/monitoring (e.g., Luu et al., 2004).
However, several arguments can be found against the notion that the present theta effect is related to error detection. First, the scalp topography of theta power changes in Luu et al. (2004) showed a midfrontal maximum. While the previous work from our lab also showed midfrontal theta scalp topographies (Bastiaansen et al., 2002b; Hald et al., 2006), the theta increase we observe here has a right posterior distribution, and is therefore both inconsistent with our previous studies, and with the error-related theta. In addition, theta power changes have also been related to other aspects of language comprehension, such as: increasing working memory load across correct sentences (Bastiaansen et al., 2002a), and lexical retrieval in single-word studies (Bastiaansen et al., 2005, 2008).
Furthermore, outside the language domain, theta oscillations have been related not only to various aspects of memory functions, such as episodic memory, spatial memory, and domain-general working memory (for reviews, see Bastiaansen and Hagoort, 2003; Bird and Burgess, 2008; Nyhus and Curran, 2010; Sauseng et al., 2010), but also to a wide range of other cognitive domains, such as (to name a few) fatigue during vigilance tasks (Lal and Craig, 2001), and, as said, error detection (Luu et al., 2004). In sum, our interpretation of the current theta effects as reflecting the detection of a (semantic) error in the sentence is tentative at best, given the wide involvement of theta in cognitive functioning.
Conclusion
In an attempt to further narrow down the functional role of gamma-band neuronal synchronization in sentence-level language comprehension, we have obtained evidence that gamma power increases are related to the predictability of an upcoming word based on the preceding sentence context, rather than to the integration of the incoming word’s semantics into the preceding context. Thus, gamma functionally contrasts with the N400, which is sensitive to both these aspects of semantic unification.
Conflict of Interest Statement
The authors declare that the research was conducted in the absence of any commercial or financial relationships that could be construed as a potential conflict of interest.
Acknowledgments
This work was supported by the Scientific Foundation of Institute of Psychology, Chinese Academy of Sciences (grant number Y1CX312005) to Lin Wang and the Natural Science Foundation of China (grant number 31100811) to Zude Zhu.
Footnote
- ^We constructed twice as much item sets as needed for the present experiment. One half of the item sets were used in the present experiment, the other half was used for a subsequent simultaneous EEG/fMRI study, that we do not report on in this paper.
References
Baayen, R. H., Piepenbrock, R., and van Rijn, H. (eds). (1993). The CELEX Lexical Database (CD-ROM). Philadelphia: University of Pennsylvania.
Baggio, G., and Hagoort, P. (2011). The balance between memory and unification in semantics: towards a dynamic account of the N400. Lang. Cogn. Proc. 26, 1338–1367.
Bastiaansen, M., and Hagoort, P. (2006). “Oscillatory brain dynamics during language comprehension,” in Event-Related Dynamics of Brain Oscillations, Vol. 159, eds W. Klimesch and C. Neuper (Amsterdam: Elsevier), 182–196.
Bastiaansen, M., and Hagoort, P. (2010). Frequency-based segregation of syntactic and semantic unification. Paper Presented at the 16th Annual Meeting of the Organization for Human Brain Mapping, Barcelona.
Bastiaansen, M., and Hagoort, P. (2003). Event-induced theta responses as a window on the dynamics of memory. Cortex 39, 967–992.
Bastiaansen, M., Magyari, L., and Hagoort, P. (2010). Syntactic unification operations are reflected in oscillatory dynamics during on-line sentence comprehension. J. Cogn. Neurosci. 22, 1333–1347.
Bastiaansen, M., Mazaheri, A., and Jensen, O. (2012). “Beyond ERPs: oscillatory neuronal dynamics,” in Oxford Handbook of Event-Related Potential Components, eds S. Luck and E. Kappenman (New York: Oxford University Press), 31–49.
Bastiaansen, M., Oostenveld, R., Jensen, O., and Hagoort, P. (2008). I see what you mean: theta power increases are involved in the retrieval of lexical semantic information. Brain Lang. 106, 15–28.
Bastiaansen, M., van Berkum, J. J., and Hagoort, P. (2002a). Event-related theta power increases in the human EEG during online sentence processing. Neurosci. Lett. 323, 13–16.
Bastiaansen, M., van Berkum, J. J., and Hagoort, P. (2002b). Syntactic processing modulates the theta rhythm of the human EEG. Neuroimage 17, 1479–1492.
Bastiaansen, M., Van der Linden, M., ter Keurs, M., Dijkstra, T., and Hagoort, P. (2005). Theta responses are involved in lexico-semantic retrieval during language processing. J. Cogn. Neurosci. 17, 530–541.
Bird, C. M., and Burgess, N. (2008). The hippocampus and memory: insights from spatial processing. Nat. Rev. Neurosci. 9, 182–194.
Fries, P. (2005). A mechanism for cognitive dynamics: neuronal communication through neuronal coherence. Trends Cogn. Sci. (Regul. Ed.) 9, 474–480.
Haarmann, H. J., Cameron, K. A., and Ruchkin, D. S. (2002). Neural synchronization mediates on-line sentence processing. EEG coherence evidence from filler-gap constructions. Psychophysiology 39, 820–825.
Hagoort, P. (2005). On Broca, brain, and binding: a new framework. Trends Cogn. Sci. (Regul. Ed.) 9, 416–423.
Hald, L. A., Bastiaansen, M. C., and Hagoort, P. (2006). EEG theta and gamma responses to semantic violations in online sentence processing. Brain Lang. 96, 90–105.
Herrmann, C. S., Munk, M. H., and Engel, A. K. (2004). Cognitive functions of gamma-band activity: memory match and utilization. Trends Cogn. Sci. (Regul. Ed.) 8, 347–355.
Jackendoff, R. (2007). A parallel architecture perspective on language processing. Brain Res. 1146, 2–22.
Kutas, M., and Federmeier, K. D. (2011). Thirty years and counting: finding meaning in the N400 component of the event-related brain potential (ERP). Annu. Rev. Psychol. 62, 621–647.
Lal, S. K., and Craig, A. (2001). A critical review of the psychophysiology of driver fatigue. Biol. Psychol. 55, 173–194.
Lau, E. F., Phillips, C., and Poeppel, D. (2008). A cortical network for semantics: (de)constructing the N400. Nat. Rev. Neurosci. 9, 920–933.
Luu, P., Tucker, D. M., and Makeig, S. (2004). Frontal midline theta and the error-related negativity: neurophysiological mechanisms of action regulation. Clin. Neurophysiol. 115, 1821–1835.
Maris, E., and Oostenveld, R. (2007). Nonparametric statistical testing of EEG- and MEG-data. J. Neurosci. Methods 164, 177–190.
Mitra, P. P., and Pesaran, B. (1999). Analysis of dynamic brain imaging data. Biophys. J. 76, 691–708.
Nyhus, E., and Curran, T. (2010). Functional role of gamma and theta oscillations in episodic memory. Neurosci. Biobehav. Rev. 34, 1023–1035.
Oostenveld, R., Fries, P., Maris, E., and Schoffelen, J. M. (2011). FieldTrip: open source software for advanced analysis of MEG, EEG, and invasive electrophysiological data. Comput. Intell. Neurosci. 2011, 156869.
Peña, M., and Melloni, L. (2012). Brain oscillations during spoken sentence processing. J. Cogn. Neurosci. 24, 1149–1164.
Sauseng, P., Griesmayr, B., Freunberger, R., and Klimesch, W. (2010). Control mechanisms in working memory: a possible function of EEG theta oscillations. Neurosci. Biobehav. Rev. 34, 1015–1022.
Shieber, S. M. (1985). Using restriction to extend parsing algorithms for complex-feature-based formalisms. Paper Presented at the Proceedings of the 23rd Annual Meeting on Association for Computational Linguistics, Stroudsburg.
Singer, W. (1993). Synchronization of cortical activity and its putative role in information processing and learning. Annu. Rev. Physiol. 55, 349–374.
van Berkum, J. J., Zwitserlood, P., Bastiaansen, M., Brown, C., and Hagoort, P. (2004). So who’s “he” anyway? Differential ERP and ERSP effects of referential success, ambiguity and failure during spoken language comprehension. Paper Presented at the Annual Meeting of the Cognitive Neuroscience Society, San Francisco.
Varela, F., Lachaux, J. P., Rodriguez, E., and Martinerie, J. (2001). The brainweb: phase synchronization and large-scale integration. Nat. Rev. Neurosci. 2, 229–239.
Vosse, T., and Kempen, G. (2000). Syntactic structure assembly in human parsing: a computational model based on competitive inhibition and a lexicalist grammar. Cognition 75, 105–143.
Weiss, S., and Mueller, H. M. (2003). The contribution of EEG coherence to the investigation of language. Brain Lang. 85, 325–343.
Keywords: language comprehension, prediction, semantic unification, neuronal oscillations, network dynamics, gamma, theta, EEG
Citation: Wang L, Zhu Z and Bastiaansen M (2012) Integration or predictability? A further specification of the functional role of gamma oscillations in language comprehension. Front. Psychology 3:187. doi: 10.3389/fpsyg.2012.00187
Received: 16 December 2011; Accepted: 22 May 2012;
Published online: 12 June 2012.
Edited by:
Lucia Melloni, Max Planck Institute for Brain Research, GermanyReviewed by:
Doug Davidson, Basque Center on Cognition, Brain, and Language, SpainAyelet Nina Landau, Ernst Strüngmann Institute in Cooperation with Max Planck Society, Germany
Copyright: © 2012 Wang, Zhu and Bastiaansen. This is an open-access article distributed under the terms of the Creative Commons Attribution Non Commercial License, which permits non-commercial use, distribution, and reproduction in other forums, provided the original authors and source are credited.
*Correspondence: Marcel Bastiaansen, Max Planck Institute for Psycholinguistics, P.O. Box 310, 6500 AH Nijmegen, Netherlands. e-mail: marcel.bastiaansen@mpi.nl
†Lin Wang and Zude Zhu have contributed equally to this work.