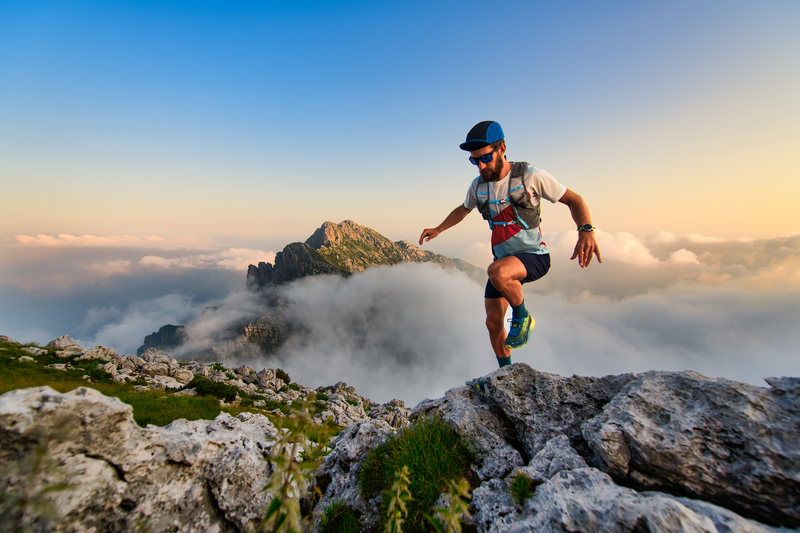
95% of researchers rate our articles as excellent or good
Learn more about the work of our research integrity team to safeguard the quality of each article we publish.
Find out more
HYPOTHESIS AND THEORY article
Front. Psychol. , 18 June 2012
Sec. Cognitive Science
Volume 3 - 2012 | https://doi.org/10.3389/fpsyg.2012.00182
This article is part of the Research Topic Inhibition in the process of feature binding View all 12 articles
How does the brain bind together visual features that are processed concurrently by different neurons into a unified percept suitable for processes such as object recognition? Here, we describe how simple, commonly accepted principles of neural processing can interact over time to solve the brain’s binding problem. We focus on mechanisms of neural inhibition and top-down feedback. Specifically, we describe how inhibition creates competition among neural populations that code different features, effectively suppressing irrelevant information, and thus minimizing illusory conjunctions. Top-down feedback contributes to binding in a similar manner, but by reinforcing relevant features. Together, inhibition and top-down feedback contribute to a competitive environment that ensures only the most appropriate features are bound together. We demonstrate this overall proposal using a biologically realistic neural model of vision that processes features across a hierarchy of interconnected brain areas. Finally, we argue that temporal synchrony plays only a limited role in binding – it does not simultaneously bind multiple objects, but does aid in creating additional contrast between relevant and irrelevant features. Thus, our overall theory constitutes a solution to the binding problem that relies only on simple neural principles without any binding-specific processes.
The term “binding” has several meanings within psychology and neuroscience. The central assumption is that partial representations must in some way be “bound” together into a full representation (Treisman, 1996, 1999). In particular, the term is used in the context of visual processing; however, the issue is relevant in understanding brain and psychological mechanisms in general. The need for binding mechanisms is highlighted by the fact that neurons early in the visual system respond to (and therefore represent) simple visual features while meaningful objects consist of very particular conjunctions of many of these features (e.g., perpendicular lines meeting at their ends compose corners; corners that line up compose rectangles, etc.). Some mechanism appears to be needed to track which of these features belong together; that is, which ones originated from a coherent construct in the real world, and so should be combined to produce an accurate and meaningful internal representation of that construct.
We seek here to clarify the neural mechanisms involved in the process of binding. In doing so, we describe a theory of how binding can be explained using only simple, generic principles of neural processing. Our perspective on binding has much in common with that of other theorists (Reynolds and Desimone, 1999; Shadlen and Movshon, 1999; Treisman, 1999; Bundesen et al., 2005). In fact, the amount of convergence on the binding problem in recent years is striking; the novelty of our contribution is therefore largely in adding specificity to these proposals in terms of the biological mechanisms that underlie binding in the brain.
Our core proposal is that competitive neural inhibition, combined with top-down feedback and learned selectivity for some features over others, accounts for binding in the brain. More specifically, the computational role of inhibition and top-down feedback in binding is to ensure that only neurons with the most support become substantially active and ultimately drive behavior. Cortical inhibition thus performs contrast enhancement by suppressing activity of neurons with significant but lower levels of excitatory input (Kandel et al., 1995; Carandini and Heeger, 2012). Neurons tuned to the less relevant information (such as features from objects outside the focus of attention) are thus out-competed, and so downstream neurons respond only to the most relevant “winning” features.
Top-down feedback supplies an extra set of criteria for which features are most relevant in a given context, supplying useful biases to this competition (Desimone and Duncan, 1995). Top-down feedback can thus be contrasted with feedforward, stimulus-driven signals, that mainly convey information about the sensory environment. However, the neural mechanisms that underlie these two information pathways are exactly the same: standard excitatory synaptic inputs (O’Reilly, 1996; O’Reilly and Munakata, 2000). Putative top-down signals include those from frontal and parietal areas that direct spatial attention (Thompson et al., 2005; Bressler et al., 2008), and those from prefrontal areas that convey information related to the current task or goals (Miller and Cohen, 2001), but might also include those originating from areas only slightly higher up in the visual system that convey “working hypotheses” as to object identities or higher-level features (Fahrenfort et al., 2007; Boehler et al., 2008; Roland, 2010; Koivisto et al., 2011). In each case, the type of information and therefore the exact constraints supplied to the competition are different; but the fundamental computational role in guiding the local competitions that lead to binding the most relevant features is the same.
We motivate our proposal with a recent review by Vanrullen (2009), which posits two distinct types of binding. One is an “on-demand” process for binding together simple but arbitrary feature dimensions into conjunctive representations (e.g., a red circle stimulus in a visual search experiment contain both “red” and “circular” features). Much of research on binding to date has involved visual tasks that use these arbitrary feature conjunctions which have been proposed to be solved by top-down attentional mechanisms as well as inhibitory mechanisms (Treisman, 1996, 1999; Reynolds and Desimone, 1999). A second type of binding, referred to as “hardwired” binding, involves grouping together pre-established conjunctions of features. Experiments using visual object categorization have been used to motivate the need for hardwired binding, with the major finding being that they proceed rapidly in the absence of top-down attentional mechanisms (Riesenhuber and Poggio, 1999b; Serre et al., 2007; Vanrullen, 2007).
We focus here on the case of hardwired binding. However, we propose that the same mechanisms involved in on-demand binding are also present during hardwired binding. Inhibition and top-down feedback interact to select only the most relevant elements of visual features for further processing, eliminating less contextually relevant features, thus minimizing binding errors. We argue that these mechanisms are just as important for activating the learned feature combinations used in visual object recognition as they are in visual tasks involving arbitrary feature combinations.
Thus, our approach focuses on the binding problem inherent in the problem of object recognition, but applies to the problem more generally. When presented with visual information, whether it be in the context of a single isolated object or an array of multiple objects, the brain relies on the same basic neural mechanisms to form a coherent (properly bound) representation. While abstract cognitive strategies may be important for dealing with different tasks (e.g., visual search), it is unlikely that they are implemented differently at the neural level or require special binding processes. Instead, they operate on the same basic representation formed by simple visual processing.
We explicitly demonstrate our proposal using a biologically realistic model of visual processing (O’Reilly et al., under review; see Methods for overview). We demonstrate three particular aspects of our proposal in the context of a realistic object recognition task that requires binding together learned object features into a single, coherent object (i.e., part binding; Treisman, 1996). First, we show how neurons that code complex visual features compete during processing over the full course of recognition. Inhibitory competition ensures that only the most relevant features are active, while less relevant ones are ultimately suppressed. We further show that systematically reducing the number of category-relevant visual features in the stimulus by an occlusion degradation weakens these competition effects, ultimately causing binding errors in which relevant and irrelevant features become co-active in the bound representation. Second, we show how top-down feedback reinforces category-relevant features, including those that may have been weakened by degrading factors like occlusion, providing some robustness to binding errors. Finally, we investigate the case of multiple object recognition, which has special importance in the study of binding as it can produce illusory conjunctions of features across objects (Treisman, 1996, 1999). We find that the same mechanisms of inhibitory competition and top-down feedback contribute to solving the problem of properly binding learned features when selecting among multiple objects.
The novelty of our contribution to the ongoing discussion on binding is a synthesis between binding and object recognition theories using only the general neural mechanisms of neural inhibition and top-down feedback. Others have put forth similar solutions to the binding problem using only general neural mechanisms (e.g., Reynolds and Desimone, 1999; Bundesen et al., 2005), and we expand on this work with explicit simulations that make predictions about the temporal dynamics of these mechanisms during a hardwired binding task. Our theory can be contrasted with more complex theories of binding, especially those that involve multiplexed neural synchrony (e.g., Singer, 1993, 1999; Singer and Gray, 1995; Uhlhaas et al., 2009). While there might be additional binding-related phenomena (such as those involving working memory; see Raffone and Wolters, 2001) that require such mechanisms, the standard object recognition functions of visual cortex targeted by existing work on binding appear to only require the mechanisms that we focus on here. We conclude by discussing some of the predictions and limitations of our model with respect to other binding theories.
In the simplest sense, a bound representation in the brain consists of the current set of actively represented features. The brain represents information in a code distributed across a large number of neurons (Kandel et al., 1995), and thus, can represent many features simultaneously. Binding errors can thus occur when features that belong to different objects in the external world are incorrectly bound together into the brain’s representation of a single object. To minimize binding errors, the brain relies on several mechanisms to ensure that only the features that belong together get bound together in the long run. One such mechanism is neural inhibition.
Within a given brain area, only a small percentage of neurons are ever active at any given time. One reason for this is that cortical neurons inhibit each other through disynaptic connections with local inhibitory neurons. These inhibitory interneurons are known to perform the function of limiting overall activity levels throughout cortical areas. Within an area, connections to and from inhibitory neurons seem to be relatively non-selective (Swadlow and Gusev, 2002), making this competitive effect general: every excitatory neuron competes with every other excitatory neuron, to roughly the same extent. This picture of inhibitory function is, of course, somewhat oversimplified, but it is sufficient to capture the role neural inhibition in solving the binding problem. This competitive inhibition is one mechanism of contrast enhancement (Carandini and Heeger, 2012), and it is useful to think of the mechanism as enhancing contrast between firing rates of neurons representing more- and less-appropriate features.
As an example that illustrates the role of inhibition in hardwired binding, we use the LVis model described in O’Reilly et al. (under review) to demonstrate how the brain binds together a visual representation of a fish for recognition (see Methods for model details). Visual object recognition is thought to be subserved primarily by inferotemporal (IT) cortex, which responds to moderately complex visual features (Logothetis et al., 1995; Tompa and Sary, 2010). IT cortex contains a columnar organization (Tanaka, 1996; Tompa and Sary, 2010), in which columns of neurons that subtend horizontal patches of the cortex code different visual features. While the specific dimensions of stimuli to which a given IT column respond are not yet well-understood (Kourtzi and Connor, 2010), IT neurons can be conceptualized as responding to object “parts” that represent a specific object exemplar at the population level (i.e., combination coding, Ungerleider and Bell, 2011).
As a concrete example, one column of IT neurons might be tuned to a fish’s fin, ideally firing when in the presence of a viewed fish. A neighboring column might be tuned to a completely different visual feature such as a bird’s wing, and thus should be silent when viewing the fish. These columns project onto inhibitory interneurons that create competition among columns (Mountcastle, 1997), effectively making some combinations of columns mutually exclusive.
In Figure 1, we show the firing patterns of simulated columns of IT neurons when presented with a fish stimulus. Initially, a large number of IT neurons fire, some of which belong to columns that code fish-relevant features and some of which belong to columns that do not. The columns selective to fish-relevant features (e.g., a fish fin, a fish tail), however, quickly out-compete columns selective to fish irrelevant features since the former constitute a better fit with the fish stimulus, increasing their initial evoked response. In turn, the columns selective to fish features inhibit columns selective to irrelevant features, effectively stopping irrelevant neurons from firing and becoming part of the bound representation. Thus, competitive inhibition among detected features helps ensure that a valid combination of features ultimately is bound by driving firing of IT neurons, eliminating invalid conjunctions of features that might lead to binding errors.
Figure 1. Neural inhibition in visual binding. We use the LVis model described in O’Reilly et al. (under review) to demonstrate how IT level visual features are suppressed by inhibitory mechanisms over the course of visual processing. tbfTop: Visual occlusion was varied as an independent variable to measure its effect on IT firing patterns during object categorization. Increased occlusion results in a monotonic impairment in categorization accuracy. Bottom: Firing rates were recorded for each IT unit in the model and grouped according to whether they were strongly tuned to the fish category exemplars (dotted lines) or tuned to other categories (solid lines). The first wave of responses from the model’s IT units area code a large number of features, only some of which are category-relevant. Inhibitory competition, however, suppresses the responses of irrelevant non-category units, leaving the features coded by relevant category units to compose the final bound representation. This competitive advantage disappears at higher levels of occlusion (e.g., 50% occlusion) due to fewer category-relevant features being specified in the stimulus.
Inhibition might be especially important when visual objects are highly ambiguous. We demonstrate this idea in Figure 1 by partially occluding the presentation of a fish, which removes diagnostic visual features and impairs recognition accuracy. Other conditions may also create stimulus ambiguity, such as a non-standard view of an object (such as a fish’s underbelly), or an atypical exemplar (an exotic fish, perhaps). Visual occlusion, however, allows us to parametrically measure the effects of ambiguity on activity levels of IT neurons in our model. The general effect of occlusion is an attenuation of the category selective IT response due to the decreased stimulus-driven signal, a finding that has been also demonstrated in neurophysiological studies of occlusion (Kovacs et al., 1995; Nielsen et al., 2006). Moreover, because neurons in category selective columns fire at a lower rate, they indirectly exert weaker levels of inhibition toward competing columns. The result is an overall increase in the response of neurons that are selective to category irrelevant features. Thus, both the weakened response to category-relevant features and the erroneous heightened response to irrelevant features may play a role in binding errors when stimulus conditions are highly ambiguous, leading to impaired recognition accuracy.
It is well-known that the brain contains numerous top-down connections that descend from higher levels of brain systems to lower levels (Felleman and van Essen, 1991; Scannell et al., 1995; Sporns and Zwi, 2004; Sporns et al., 2007). In the context of vision, one commonly suggested function of top-down connections is to convey attentional signals to sensory based areas of visual cortex. These top-down signals can take the form of spatial attention (originating in the frontal eye fields and posterior parietal cortex, Thompson et al., 2005; Bressler et al., 2008) or executive attentional control (as enacted by maintained representations in prefrontal cortex; Miller and Cohen, 2001; Herd et al., 2006).
In the case of spatial attention, top-down feedback about the attended region of space determines which features are relevant by selecting for features within a small spatial area and enhancing them relative to features from neighboring, unattended areas of space. Top-down feedback reflecting executive attentional control works the same way, except that relevancy is determined by more abstract feature dimensions such as color or category (Maunsell and Treue, 2006).
In either case, top-down feedback does not require any representation of what to exclude. Instead, it simply signals what to attend to by providing additional excitatory bias to the sensory representations, causing the representative neurons to fire more strongly. This bias reinforces the activation of relevant features, encouraging their binding at the highest levels of processing. This explanation of attention is a further explication of the biased competition framework of (Desimone and Duncan, 1995), and has been supported by considerable empirical evidence, most notably that of Reynolds and colleagues (see Reynolds and Chelazzi, 2004, for a review).
While top-down feedback has been shown to be crucial for on-demand binding tasks that require the cognitive flexibility to bind arbitrary features together at arbitrary locations (Treisman, 1996, 1999), it is not yet understood whether top-down feedback similarly plays a role in hardwired binding tasks like object recognition and categorization. Computational models have suggested that these tasks can be solved in the brain in a primarily feedforward manner with little to no influence from top-down feedback (Riesenhuber and Poggio, 1999b; Serre et al., 2007; Vanrullen, 2007, 2009). However, there are a number of reports of top-down feedback playing a fundamental role in early visual processes including object recognition (Bar et al., 2006; Fahrenfort et al., 2007; Boehler et al., 2008; Roland, 2010; Koivisto et al., 2011).
In an attempt to reconcile these data, we recently described a computational model of object recognition that contains both feedforward and feedback connections between feature processing layers (O’Reilly et al., under review). One of the key findings, which we review here, is that top-down feedback promotes robust recognition when bottom-up signals are weak and ambiguous due to occlusion (Figure 2). While occlusion generally attenuates neural responses resulting in reduced recognition accuracy, the model often exhibits intact category selective responses and correct recognition, a property that we attribute to top-down feedback. Specifically, top-down reinforcement enhances the responses of neurons at lower levels that may have been weakened due to occlusion. This enhancement is repeated across multiple recurrently connected areas, essentially recovering the occluded visual features and resulting in a complete representation. Conceptually, visible features like the fish’s dorsal fin might evoke a partial response in IT cortex, which could provide reinforcement to the encoding of other relevant features that might not be visible at lower levels like V2 or V4. Similarly, entertaining the possibility that one might be viewing a fish (i.e., partial activation at the “Naming Output” level of our model) can reinforce fish-relevant features encoded by IT columns. Functional neuroimaging experiments have indicated that the brain exhibits a similar object completion process in which visual information is recovered despite its omission from a visual stimulus (Kourtzi and Kanwisher, 2001; Lerner et al., 2004; Johnson and Olshausen, 2005; Juan et al., 2010).
Figure 2. Top-down feedback in “hardwired” binding tasks. (A) As a general rule, the visual system contains bidirectional (both bottom-up and top-down) connections between any two connected areas. The LVis model, depicted here, contains a similar organization, with recurrent connections between hierarchically adjacent areas. (B) Top-down feedback from higher levels enhances the neural responses in lower-level areas, which is crucial for robust binding when stimuli are occluded or otherwise ambiguous. Arrows indicate the enhancement in the representation with respect to the veridical (i.e., unoccluded) representation at different areas within the model. Pale colors indicate the predicted response without top-down feedback, which is asymptotic. The Sem area exhibits a similar enhancement pattern to areas V2/V4 and IT, but is left unannotated on the plot for clarity. V2/V4 = extrastriate cortex; IT = inferotemporal cortex; Sem = amodal semantic responses, such as those demonstrated by anterior IT neurons; Name = named output responses.
Thus far we have focused on the problem of binding visual features into a singular, coherent object, and have proposed that both neural inhibition and top-down feedback play important roles in this process. Do these same mechanisms aid in proper binding when multiple objects are present in a display? Proper binding when multiple objects are present is a challenging problem because high-level visual areas such as IT cortex have receptive fields that span large portions of the visual field (generally 10° to 20°; Kobatake and Tanaka, 1994; Rust and Dicarlo, 2010). Thus, IT neurons respond, by default, to visual features regardless of where they are within the visual display, even when they occur in the context of a second object’s features. Although the large receptive fields of IT neurons are thought to be necessary for promoting tolerance to changes in object position, scale, and rotation (Logothetis et al., 1995; Tanaka, 1996; Riesenhuber and Poggio, 2002; Rolls and Stringer, 2006), they exacerbate the possibility of illusory conjunctions being formed between the features of separate objects.
We propose that neural inhibition combined with top-down feedback can solve the problem of binding when multiple objects are present in a similar manner to the way they aid in binding visual features into singular, coherent objects. We demonstrate the plausibility of this idea in Figure 3. As is the case with single objects presented in isolation, a large number of IT neurons fire initially when multiple objects are present. Grouping these neurons according to the object to which they are selective illustrates the interactions between inhibition and top-down feedback. Generally, neurons that code visual features shared by both objects are the first to respond, since they constitute the best overall fit with the stimulus itself. In the case of the gun and bicycle pictured in Figure 3A, these first responders might be neurons that code the horizontal edges that compose the barrel of the gun and the top tube of the bicycle. Neurons that code unique features for each of the object categories are the next to respond. However, inhibition between these columns of neurons ensures that the features of only one of these objects are selected in the end, “winning” the competition (in this case, the bicycle neurons) and contributing to the final bound representation. When a single object is selected for the bound representation, top-down feedback can reinforce neurons that code meaningful features from that object that may not have initially responded (possibly due to initial inhibitory influences from neurons corresponding to the “losing” object).
Figure 3. Binding multiple objects. (A) The same mechanisms of neural inhibition and top-down feedback extend to binding when multiple objects are present in a display. The competition created from having multiple IT units active that represent multiple objects causes one set of units to “win” and one set to “lose” (in this case, the bicycle units win). Inhibition suppresses the responses from units corresponding to the losing object as well as responses from completely irrelevant units. Top-down feedback serves to reinforce units from the winning object that may not have been initially active. (B) Binding errors occur when completely irrelevant units become erroneously active, leading to the inability to suppress invalid responses. This creates illusory conjunctions of features across the objects in the display, leading to a representation that does not resemble either category.
Binding errors can occur when neurons representing irrelevant features are not entirely out-competed (Figure 3B). This allows invalid feature conjunctions to manifest, which subsequently get reinforced from top-down feedback, resulting in the formation of illusory conjunctions. To determine more specifically how inhibition and top-down feedback contribute to minimizing illusory conjunctions, we tested the effect of removing top-down feedback and both top-down feedback and inhibition from the model1 (see Methods for details). The results of these tests are indicated in Figure 4.
Figure 4. Results for multiple object binding. (A) We tested the effect of removing top-down feedback and both top-down feedback and inhibitory competition from the model. The purely feedforward model missing both of these critical mechanisms made the most recognition errors. (B) Grouping responses according to whether they were corresponded to the model’s output (relevant responses) or not (irrelevant responses) suggests that the reason for the purely feedforward model’s poor performance was that it had a higher overall signal-to-noise ratio (mean relevant response divided by mean irrelevant response). This type of representation could lead to illusory feature conjunctions and thus, recognition errors.
For the LVis model (which contains both inhibition and top-down feedback), illusory conjunctions occurred on only 4.7% of trials. Removing top-down feedback, but leaving inhibition intact, had virtually no effect on the number of illusory conjunctions. However, removing both top-down feedback and inhibition caused illusory conjunctions to occur with much higher frequency, on 19.3% of trials.
We also computed the ratio of relevant IT responses to irrelevant responses (where relevance was determined by whether the responses corresponded to the model’s output) which can be thought of as a kind of “signal-to-noise ratio” (Figure 4B). A decrease in this number reflects lower proportions of relevant information and higher proportions of irrelevant information at the IT level, which could lead to more illusory conjunctions being made. Accordingly, the purely feedforward model, which made the most recognition errors, also exhibited the lowest ratio of relevant to irrelevant information.
Removing feedback from the LVis model also lowered the ratio of relevant to irrelevant information, but recognition performance remained unchanged. This suggests that there is a critical signal-to-noise ratio (in terms of relevant and irrelevant responses) above which recognition remains robust, without many illusory conjunctions. Inhibition was intact in this model, consistent with our proposal that inhibitory competition is the critical mechanism that selects relevant information over irrelevant information, thus providing a relatively stable baseline signal-to-noise ratio. Top-down feedback can further highlight relevant information, increasing the signal-to-noise ratio, but it is unnecessary for well-learned tasks with little ambiguity. Top-down feedback is likely more important in tasks where objects are degraded (e.g., from visual occlusion), which we discussed in the previous section, or in cases where there is more feature overlap across items (e.g., conjunctive visual search).
We have presented an account of binding in the brain that depends only on well-established mechanisms of neural processing that interact over time. Two such mechanisms that we focus on here are neural inhibition and top-down feedback. Together, these mechanisms create an environment of local competition within a brain area that selects only the most relevant features for the bound representation that influences perception and behavior. We have taken a general neural processing approach to explaining how these mechanisms relate to binding. We illustrate the mechanisms explicitly in an object recognition task that requires binding together learned object features into a single, coherent object, as well as a variant of this task that requires selecting from and identifying multiple objects. Despite our focus on “hardwired” binding, we believe that the same mechanisms perform “on-demand” binding (e.g., conjunctive visual search). In on-demand binding, top-down influences bias processing toward items in a particular region of space, and consequently, competitive inhibition eliminates those features in nearby areas of space, allowing a properly bound representation of the novel item.
One natural consequence of our proposal is the suggestion that perception and behavior are largely driven by an interactive process that integrates bottom-up information with dynamic constraints including top-down, conceptual knowledge. It is somewhat surprising then, that a large class of extant theories of visual processing treat early perceptual processing as a feedforward set of stages that simply transform information from one level of the visual system to the next (Riesenhuber and Poggio, 1999b; Serre et al., 2007; Vanrullen, 2007). Models that instantiate this feedforward theory often include a “max” operation that selects the largest response at each processing level, which can be viewed as a form of inhibitory competition that suppresses less relevant responses (Riesenhuber and Poggio, 1999a). These models, however, lack top-down feedback to reinforce relevant information, which can emerge at any time over the course of processing.
Competitive dynamics reflecting inhibitory and top-down influences within visual areas are clear if one examines population level responses. For example, initial IT population responses convey information about many individual object parts, but information about the object as a whole begins to emerge over the full time course of their response (Brincat and Connor, 2006; see also Sugase-Miyamoto et al., 2011). Other single-cell analyses have indicated that the selectivity of IT neurons changes over time, beginning with a quick burst of broadly tuned activity that gradually becomes more selective (Tamura and Tanaka, 2001). Similar temporal dynamics have been demonstrated at other levels of the visual system, such as areas V2 and V4 (Hegde and van Essen, 2004, 2006). The fact that the information content of neural responses changes over time strongly suggests that some aspects of the representation are being selected over others. Our account of binding suggests that relevancy is a significant determining factor of what parts of the representation are ultimately selected for the bound representation at he highest levels.
Our proposal is highly congruent with many previous descriptions of binding (Reynolds and Desimone, 1999; Shadlen and Movshon, 1999; Treisman, 1999; Bundesen et al., 2005). Our contribution is novel in implementing a biologically grounded neural network model that embodies these theories, and in further specifying the mechanisms involved, and how they interact. One notable relation is to the role of top-down feedback in the form of spatial attention in Treisman’s Feature Integration Theory (Treisman, 1996, 1999). Top-down feedback in our model does not directly perform binding, however, but simply prevents mis-binding by highlighting some features over others and relying on competitive inhibition to suppress the others.
Our proposal also has much in common with (Reynolds and Desimone, 1999) biased competition model, which cites the importance of competitive inhibition between populations of neurons and top-down biasing of relevant features. However, the biased competition model has traditionally focused on frontal and parietal cortices as likely sources of the biasing signal. While attentional signals from these areas are clearly capable of biasing perceptual processing (Miller and Cohen, 2001; Thompson et al., 2005; Herd et al., 2006; Bressler et al., 2008), our approach provides a more general characterization of biasing. Specifically, any area that sends feedback to an earlier area has the potential to bias its computations. In our simulations, this allows for representations that are beginning to emerge at high-level areas to bias lower-level areas, which itself can be viewed as a form of emergent feature-based attention.
Theories centering on the role of synchrony have also been proposed as a solution to the binding problem (Singer, 1993, 1999; Singer and Gray, 1995; Uhlhaas et al., 2009). There is ample evidence that neural firing does synchronize to some degree, and that synchrony plays a role in attentive object recognition (Gray et al., 1989; Buzsaki and Draguhn, 2004). We agree that synchrony does play a role in the competitive selection process that is the core of our proposal, acting as another form of contrast enhancement by providing mutual excitation among concurrently active neurons via recurrent feedback and lateral connections (Roland, 2010). Synchrony thus effectively gives the winners of competition an extra advantage in controlling responses at higher levels.
This role of synchrony in sharpening neural competition should be differentiated from early proposals that synchrony can simultaneously bind multiple objects. No data of which we are aware indicates that the brain performs “multiplexed synchrony,” in which neurons representing each object remain in phase with others representing the same object, but reliably out of phase with neurons representing other objects. Theories of multiplexed synchrony for binding have been strongly criticized on the grounds of being both biologically implausible and unnecessary (Shadlen and Movshon, 1999; O’Reilly et al., 2003). While it seems intuitive that we are aware of many objects simultaneously, recent research on change blindness indicates that we do not maintain detailed representations outside the current focus of attention (Beck et al., 2001; Lamme, 2003; Simons and Rensink, 2005).
Because of the level of noise from incidental processing in the brain (compared to models, which are idealized and thus use little to no noise) multiplexed synchrony seems likely to be unstable beyond extremely short time periods. This drawback severely limits the use of this mechanism for binding in working memory, the other case in which intuition and some evidence suggests we maintain several representations simultaneously (Raffone and Wolters, 2001). One alternative to true multiplexed synchrony is that binding in working memory is performed by maintaining separate neural substrates for separate items within prefrontal cortex, as in the model of working memory developed by our group, reviewed in O’Reilly et al. (2010).
Rather than supposing that the brain can represent and interpret several arbitrary conjunctions of features simultaneously, it seems more parsimonious to assume, as in our proposal, that all features represented simultaneously are bound together. Instead of using a particular firing phase to “tag” each neuron as belonging to one object or another, the brain simply represents only one object (or concept, etc.) at a time when binding is difficult, thus serializing a computation that would pose unique difficulties for parallel processing.
While previous work on binding has presented many possible mechanisms and argued that they are needed to solve the brain’s binding problem, the necessity of mechanisms beyond the most basic neural mechanisms has not been clearly demonstrated. We have presented a solution to the binding problem of that relies on only generic neural mechanisms to bind together features into objects. While our proposal clearly demonstrates that the mechanisms of inhibition and top-down feedback contribute in part to solving the brain’s overall binding problem, it is possible that there exist binding-related situations that warrant additional mechanisms and processes (e.g., working memory). Only after attempting to explain these phenomena with basic neural mechanisms (as in the proposals mentioned above) should more complicated theories be considered.
The LVis (Leabra Vision) model and its training/testing methods are briefly described here. See O’Reilly et al. (under review), for a detailed description. The model consists of a hierarchy of feature processing layers that roughly correspond to areas within the ventral stream of the brain – primary visual cortex (V1), extrastriate cortex (V2/V4), inferotemporal cortex (IT) – as well as higher-level layers that represent amodal semantic properties and named output responses (Figure 2A). The model processes grayscale bitmap images with filters that capture the response properties of the retina and lateral geniculate nucleus (LGN) of the thalamus, the results of which are used as inputs to the V1 layer. The model’s V1 layer consists of a retinotopic grid of 3600 units that represent V1-like features at multiple spatial scales. The V2/V4 layer contains 2880 units that receive from neighborhoods of 320 V1 units. Neighboring V2/V4 units receive from overlapping portions of the V1 layer. The IT layer contains 200 units that receive from the entire 2880 V2/V4 units, and thus do not contain a retinotopic organization.
Overall, the model can be viewed as an expansion on a large class of hierarchical feedforward models of visual processing in the brain (Riesenhuber and Poggio, 1999b; Delorme and Thorpe, 2001; Masquelier and Thorpe, 2007; Serre et al., 2007). The primary innovation of the model is that hierarchically adjacent layers (e.g., V1 and V2/V4; V2/V4 and IT) are recurrently connected, providing an account of top-down feedback connections within the brain’s ventral stream. Feedforward connections generally contribute 80–90% of the total input to a receiving layer and feedback connections contribute the remaining 10–20% of the total input. Overall layer activations are controlled using a k-winners-take-all (kWTA) inhibitory competition rule (O’Reilly, 1996; O’Reilly and Munakata, 2000) that ensures only the k most active units remain active over time. The specific k value varies for each layer in the model, but is generally in the range of 10–20% of the number of units in the layer.
The model was trained to categorize images from the CU3D-100 dataset (http://cu3d.colorado.edu) using an extension of the Leabra learning algorithm (O’Reilly, 1996; O’Reilly and Munakata, 2000). The entire dataset consisted of 18,840 total images. Training proceeded for 1000 epochs of 500 trials, each of which consisted of a random image selected from the dataset which was transformed with small variations in position, scale, and planar rotation. Images of two exemplars from each category (4000 images total) were reserved for a generalization test. After training, the final mean generalization accuracy was 91.9%.
Category selective representations were obtained for each of the 100 categories by averaging the response patterns of the model’s IT units to all training and testing images from each category. In general, a distribution of 10–20% of the 200 units were selective to a given category, reflecting the level of kWTA inhibition within the IT layer. The category-relevant units for a given category were then isolated using a simple threshold over the category selective representations. For the fish category used in the simulations here, a value of 0.3 was used such that a higher response level indicated a category-relevant unit while a lower response level indicated a category irrelevant unit. Small variations in this parameter produced very similar results.
To create the plots in Figure 1, the firing rate from each of the model’s IT units was recorded and averaged across every training and testing fish image (180 total images), then grouped according to whether the unit was category-relevant or irrelevant. This procedure was repeated with a visual occlusion manipulation that used a Gaussian-based filter to delete pixels from the input image. The filter was defined as 1.0 within a circle of radius 5% of the image size and then fell off outside the circle as a Gaussian function. The σ parameter of the Gaussian was set to 5% of the image size. The filter was applied to the image a variable number of times, with more applications corresponding to higher levels of occlusion.
To create the plot in Figure 2B, the model was presented with an unoccluded image of the fish and the response pattern was recorded from the model’s V2/V4, IT, Semantic, and Naming Output layers for 50 processing cycles. The model was subsequently presented with a 50% occluded image of the fish, and the resulting response patterns were used to compute the similarity to the unoccluded response patterns for each layer as a function of time. The cosine angle between the unoccluded and occluded response vectors was used as the similarity metric in this calculation.
The multiple object simulations involved training the model to recognize smaller versions of the CU3D-100 stimuli and testing its ability to generalize to presentations of multiple small stimuli. Training methods for these simulations were generally similar to the single object simulations described above, but a subset of the dataset was used. Five (5) exemplars from 5 categories (500 total images) were selected from the full dataset (bicycle, car, donut, doorhandle, and gun). Each image was downscaled to 50% of its size (originally 320 × 320 pixels, downscaled to 160 × 160 pixels) and randomly placed on either the left or right half of a new 320 × 320 image with variation in the y axis position. This was repeated 25 times for each of the 500 original images, resulting in 12500 new images. The model was trained on images from this dataset of 4 exemplars from each category to ensure proper generalization without over fitting. Training proceeded for 50 epochs of 500 trials. This was repeated for five instances of the model using different combinations of the 4 training exemplars from each category and randomized initial weights. After training, the final accuracy over the training stimuli was 100% for each model.
To create the multiple object stimuli that were used for testing, images from each possible pairing of categories were randomly combined with one 160 × 160 image on the left half of a new 320 × 320 image and one 160 × 160 image on the right half. This was repeated 25 times for each category pairing, resulting in 250 new images containing two objects. In testing over these images, the model was ran for 100 cycles, as it often did not fully converge in the standard 50 cycles used in single object presentations. A testing trial was counted as correct if the model’s output matched either of the two categories in the image.
We tested the effect of removing top-down feedback and inhibitory competition from the model on recognition accuracy for the multiple object stimuli. To remove influence from top-down feedback only, unit inputs from top-down feedback connections (e.g., Naming Output to IT, IT to V2/V4) were simply multiplied by zero during the testing phase. Removing influence from both top-down feedback and inhibitory competition required training a variant of the model that contained only feedforward connections (allowing for negative weights between units) with a backpropagation algorithm. This feedforward model required training for 100 epochs of 500 trials on the training stimuli before reaching 100% accuracy. Aside from these differences, the model was architecturally equivalent to the LVis model in terms of layer organization and numbers of units and used otherwise identical training and testing methods.
The same method that was used in the single object simulations was used to isolate category selective representations for each of the 5 categories, except that IT units were further grouped into those shared across category pairings (e.g., gun and bicycle units) as well as those that were unique to each category. These groupings were used to create the plots in Figure 3. Similarity to category selective representations was also measured to determine how much the overall pattern of responses across the IT layer approximated the category selective response to the single objects. The cosine angle between the category selective representation and the IT response vector was used as the similarity metric in this calculation.
To investigate how category-relevant responses were related to a model’s output (Figure 4), the firing rates of units that corresponded to the model’s output were isolated into one grouping (relevant responses) while the firing rates of all other units were isolated into another grouping (irrelevant responses). Our decision to refer to these responses as “relevant” and “irrelevant” was made to keep with the theme of relevant and irrelevant responses when a single object was presented in isolation, but one should note that irrelevant responses encompassed what could be considered to be relevant responses. For example, if a presented stimulus contained a gun and a bicycle and a model responded gun, the responses from units that corresponded to the gun category were considered to be the relevant responses while the units that corresponded to all other categories (including bicycle) were considered to be irrelevant. Other reasonable labels for these two groupings might be selected/unselected or attended/unattended responses.
The authors declare that the research was conducted in the absence of any commercial or financial relationships that could be construed as a potential conflict of interest.
Bar, M., Kassam, K., Ghuman, A., Boshyan, J., and Schmidt, A. (2006). Top-down facilitation of visual recognition. Proc. Natl. Acad. Sci. U.S.A. 103, 449–454.
Beck, D. M., Rees, G., Frith, C. D., and Lavie, N. (2001). Neural correlates of change detection and change blindness. Nat. Neurosci. 4, 645–650.
Boehler, C. N., Schoenfeld, M. A., Heinze, H. J., and Hopf, J. M. (2008). Rapid recurrent processing gates awareness in primary visual cortex. Proc. Natl. Acad. Sci. U.S.A. 105, 8742–8747.
Bressler, S. L., Tang, W., Sylvester, C. M., Shulman, G. L., and Corbetta, M. (2008). Top-down control of human visual cortex by frontal and parietal cortex in anticipatory visual spatial attention. J. Neurosci. 28, 10056–10061.
Brincat, S. L., and Connor, C. E. (2006). Dynamic shape synthesis in posterior inferotemporal cortex. Neuron 49, 17–24.
Bundesen, C., Habekost, T., and Kyllingsbaek, S. (2005). A neural theory of visual attention: bridging cognition and neurophysiology. Psychol. Rev. 112, 291–328.
Buzsaki, G., and Draguhn, A. (2004). Neuroscience neuronal oscillations in cortical networks. Science 304, 1926–1938.
Carandini, M., and Heeger, D. (2012). Normalization as a canonical neural computation. Nat. Rev. Neurosci. 13, 51–62.
Delorme, A., and Thorpe, S. (2001). Face identification using one spike per neuron: resistance to image degradations. Neural. Netw. 14, 795–803.
Desimone, R., and Duncan, J. (1995). Neural mechanisms of selective visual attention. Annu. Rev. Neurosci. 18, 193–222.
Fahrenfort, J. J., Scholte, H. S., and Lamme, V. A. F. (2007). Masking disrupts reentrant processing in human visual cortex. J. Cogn. Neurosci. 19, 1488–1497.
Felleman, D. J., and van Essen, D. C. (1991). Distributed hierarchical processing in the primate cerebral cortex. Cereb. Cortex 1, 1–47.
Gray, C. M., König, P., Engel, A. K., and Singer, W. (1989). Oscillatory responses in cat visual cortex exhibit inter-columnar synchronization which reflects global stimulus properties. Nature 338, 334–337.
Hegde, J., and van Essen, D. C. (2004). Temporal dynamics of shape analysis in macaque visual area v2. J. Neurophysiol. 92, 3030–3042.
Hegde, J., and van Essen, D. C. (2006). Temporal dynamics of 2d and 3d shape representation in macaque visual area v4. Vis. Neurosci. 23, 749–763.
Herd, S. A., Banich, M. T., and O’Reilly, R. C. (2006). Neural mechanisms of cognitive control: an integrative model of Stroop task performance and fMRI data. J. Cogn. Neurosci. 18, 22–32.
Johnson, J. S., and Olshausen, B. A. (2005). The recognition of partially visible natural objects in the presence and absence of their occluders. Vision Res. 45, 3262–3276.
Juan, C., Tiangang, Z., Hua, Y., and Fang, F. (2010). Cortical dynamics underlying face completion in human visual system. J. Neurosci. 30, 16692–16698.
Kandel, E. R., Schwartz, J. H., and Jessell, T. M. (1995). Essentials of Neural Science and Behavior. Norwalk, CT: Appleton & Lange.
Kobatake, E., and Tanaka, K. (1994). Neuronal selectivities to complex object features in the ventral visual pathway. J. Neurophysiol. 71, 856–867.
Koivisto, M., Railo, H., Revonsuo, A., Vanni, S., and Salminen-Vaparanta, N. (2011). Recurrent processing in v1/v2 contributes to categorization of natural scenes. J. Neurosci. 31, 2488–2492.
Kourtzi, Z., and Connor, C. E. (2010). Neural representations for object perception: structure, category, and adaptive coding. Annu. Rev. Neurosci. 34, 45–67.
Kourtzi, Z., and Kanwisher, N. (2001). Representation of perceived object shape by the human lateral occipital complex. Science 293, 1506–1509.
Kovacs, G., Vogels, R., and Orban, G. A. (1995). Selectivity of macaque inferior temporal neurons for partially occluded shapes. J. Neurosci. 15, 1984–1997.
Lamme, V. A. (2003). Why visual attention and awareness are different. Trends Cogn. Sci. (Regul. Ed.) 7, 12–18.
Lerner, Y., Harel, M., and Malach, R. (2004). Rapid completion effects in human high-order visual areas. Neuroimage 21, 516–526.
Lerner, Y., Harel, M., and Malach, R. (2004). Rapid completion effects in human high-order visual areas. Neuroimage 21, 516–526.
Logothetis, N. K., Pauls, J., and Poggio, T. (1995). Shape representation in the inferior temporal cortex of monkeys. Curr. Biol. 5, 552–563.
Masquelier, T., and Thorpe, S. J. (2007). Unsupervised learning of visual features through spike timing dependent plasticity. PLoS Comput. Biol. 3, e31. doi:10.1371/journal.pcbi.0030031
Maunsell, J. H. R., and Treue, S. (2006). Feature-based attention in visual cortex. Trends Neurosci. 29, 317–322.
Miller, E. K., and Cohen, J. D. (2001). An integrative theory of prefrontal cortex function. Annu. Rev. Neurosci. 24, 167–202.
Nielsen, K. J., Logothetis, N. K., and Rainer, G. (2006). Dissociation between local field potentials and spiking activity in macaque inferior temporal cortex reveals diagnosticity-based encoding of complex objects. J. Neurosci. 26, 9639–9645.
O’Reilly, R. C. (1996). Biologically plausible error-driven learning using local activation differences: the generalized recirculation algorithm. Neural Comput. 8, 895–938.
O’Reilly, R. C., Busby, R. S., and Soto, R. (2003). “Three forms of binding and their neural substrates: alternatives to temporal synchrony,” in The Unity of Consciousness: Binding, Integration, and Dissociation, ed. A. Cleeremans (Oxford: Oxford University Press), 168–192.
O’Reilly, R. C., Herd, S. A., and Pauli, W. M. (2010). Computational models of cognitive control. Curr. Opin. Neurobiol. 20, 257–261.
O’Reilly, R. C., and Munakata, Y. (2000). Computational Explorations in Cognitive Neuroscience: Understanding the Mind by Simulating the Brain. Cambridge, MA: The MIT Press.
Raffone, A., and Wolters, G. (2001). A cortical mechanism for binding in visual working memory. J. Cogn. Neurosci. 13, 766–785.
Reynolds, J. H., and Chelazzi, L. (2004). Attentional modulation of visual processing. Annu. Rev. Neurosci. 27, 611–647.
Reynolds, J. H., and Desimone, R. (1999). The role of neural mechanisms of attention in solving the binding problem. Neuron 24, 111–125.
Riesenhuber, M., and Poggio, T. (1999a). Are cortical models really bound by the “binding problem?” Neuron 24, 87–93.
Riesenhuber, M., and Poggio, T. (1999b). Hierarchical models of object recognition in cortex. Nat. Neurosci. 3, 1199–1204.
Riesenhuber, M., and Poggio, T. (2002). Neural mechanisms of object recognition. Curr. Opin. Neurobiol. 12, 162–168.
Roland, P. (2010). Six principles of visual cortical dynamics. Front. Syst. Neurosci. 4:28. doi:10.3389/fnsys.2010.00028
Rolls, E. T., and Stringer, S. M. (2006). Invariant visual object recognition: a model, with lighting invariance. J. Physiol. Paris 100, 43–62.
Rust, N. C., and Dicarlo, J. J. (2010). Selectivity and tolerance (“invariance”) both increase as visual information propagates from cortical area v4 to it. J. Neurosci. 30, 12978–12995.
Scannell, J., Blakemore, C., and Young, M. P. (1995). Analysis of connectivity in the cat cerebral cortex. J. Neurosci. 15, 1463–1483.
Serre, T., Kreiman, G., Kouh, M., Cadieu, C., Knoblich, U., and Poggio, T. (2007). A quantitative theory of immediate visual recognition. Prog. Brain Res. 165, 33–56.
Shadlen, M. N., and Movshon, J. A. (1999). Synchrony unbound: a critical evaluation of the temporal binding hypothesis. Neuron 24, 67–77.
Simons, D. J., and Rensink, R. A. (2005). Change blindness: past, present, and future. Trends Cogn. Sci. (Regul. Ed.) 9, 16–20.
Singer, W. (1993). Synchronization of cortical activity and its putative role in information processing and learning. Annu. Rev. Physiol. 55, 349–374.
Singer, W. (1999). Neuronal synchrony: a versatile code for the definition of relations? Neuron 24, 49–65.
Singer, W., and Gray, C. M. (1995). Visual feature integration and the temporal correlation hypothesis. Annu. Rev. Neurosci. 18, 555–586.
Sporns, O., Honey, C. J., and Kotter, R. (2007). Identification and classification of hubs in brain networks. PLoS ONE 2, e1049. doi:10.1371/journal.pone.0001049
Sporns, O., and Zwi, J. D. (2004). The small world of the cerebral cortex. Neuroinformatics 2, 145–162.
Sugase-Miyamoto, Y., Matsumoto, N., and Kawano, K. (2011). Role of temporal processing stages by inferior temporal neurons in facial recognition. Front. Psychol. 2:141. doi:10.3389/fpsyg.2011.00141
Swadlow, H., and Gusev, A. (2002). Receptive-field construction in cortical inhibitory interneurons. Nat. Neurosci. 5, 403–404.
Tamura, H., and Tanaka, K. (2001). Visual response properties of cells in the ventral and dorsal parts of the macaque inferotemporal cortex. Cereb. Cortex 11, 384–399.
Thompson, K. G., Biscoe, K. L., and Sato, T. R. (2005). Neuronal basis of covert spatial attention in the frontal eye field. J. Neurosci. 25, 9479–9487.
Tompa, T., and Sary, G. (2010). A review on the inferior temporal cortex of the macaque. Brain Res. Rev. 62, 165–182.
Treisman, A. (1999). Solutions to the binding problem: progress through controversy and convergence. Neuron 24, 105–125.
Uhlhaas, P. J., Pipa, G., Lima, B., Melloni, L., Neuenschwander, S., Nikolic, D., and Singer, W. (2009). Neural synchrony in cortical networks: history concept and current status. Front. Integr. Neurosci. 3:17. doi:10.3389/neuro.07.017.2009
Ungerleider, L. G., and Bell, A. H. (2011). Uncovering the visual “alphabet”: advances in our understanding of object perception. Vision Res. 51, 782–799.
Keywords: binding, competitive inhibition, feedback, computational model, object recognition
Citation: Wyatte D, Herd S, Mingus B and O’Reilly R (2012) The role of competitive inhibition and top-down feedback in binding during object recognition. Front. Psychology 3:182. doi: 10.3389/fpsyg.2012.00182
Received: 16 January 2012; Accepted: 20 May 2012;
Published online: 18 June 2012.
Edited by:
Snehlata Jaswal, Indian Institute of Technology Ropar, IndiaReviewed by:
Rufin VanRullen, Centre de Recherche Cerveau et Cognition Toulouse, FranceCopyright: © 2012 Wyatte, Herd, Mingus and O’Reilly. This is an open-access article distributed under the terms of the Creative Commons Attribution Non Commercial License, which permits non-commercial use, distribution, and reproduction in other forums, provided the original authors and source are credited.
*Correspondence: Dean Wyatte, Department of Psychology and Neuroscience, University of Colorado Boulder, 345 UCB, Boulder, CO 80309-0345, USA. e-mail:ZGVhbi53eWF0dGVAY29sb3JhZG8uZWR1
Disclaimer: All claims expressed in this article are solely those of the authors and do not necessarily represent those of their affiliated organizations, or those of the publisher, the editors and the reviewers. Any product that may be evaluated in this article or claim that may be made by its manufacturer is not guaranteed or endorsed by the publisher.
Research integrity at Frontiers
Learn more about the work of our research integrity team to safeguard the quality of each article we publish.